- 1Department of Crop Science, University of Cape Coast, University Post Office, Cape Coast, ;Ghana
- 2Yam Breeding Unit, International Institute of Tropical Agriculture, Ibadan, ;Nigeria
- 3Department of Agroecology, Faculty of Technical Sciences, Aarhus University, Tjele, Denmark
- 4Department of Agricultural Economics and Extension, University of Cape Coast, University Post Office, Cape Coast, ;Ghana
Background: Yam production in sub-Saharan African countries faces challenges due to susceptibility to yam mosaic virus (YMV) disease, leading to significant yield losses. Dioscorea praehensilis, a semi-cultivated yam species known for its high yield and resistance to YMV, can be utilized as a new variety and a gene source to enhance tuber yield and YMV resistance of White Guinea yam. Investigating the genetic basis of tuber yield-related traits and YMV resistance in D. praehensilis through association mapping will facilitate the traits discovery and deployment into the yam breeding program.
Methodology: A total of 132 D. praehensilis genotypes were evaluated in 2022 and 2023 seasons for tuber yield per plant, tuber size ratio and for mosaic virus severity response. The genotypes were sequenced using SNP markers generated from Diversity Array Technology Platform. The trait association analysis was conducted using mixed linear model implemented in GWASpoly package followed for putative genes analysis.
Results: Population structure and phylogeny analyses using 4,525 single nucleotide polymorphism (SNP) markers grouped the 132 D. praehensilis genotypes into three clusters. Using multiple gene action models which include dominant, additive, and general models, 27 SNP markers were significantly identified to be associated with the tuber yield, tuber size ratio and yam mosaic virus severity. Of these 27 SNPs, we identified three and two SNP markers for tuber yield and yam mosaic virus severity, respectively as stable markers across years. Using gene annotation, we identified 10 putative genes such as Serine/threonine-/dual specificity protein kinase, catalytic domain involved in starch biosynthesis and Ribosomal protein L5 involved in defense signaling against virus diseases. The marker effect revealed that alleles CC and TT were associated with high tuber yield, while AA and AC were linked with low tuber yield. For YMV severity response, alleles CC and CT were linked with low YMV severity, while TT was associated high YMV severity prediction.
Conclusion: The results of this study offer valuable insights into comprehending the functional networks involved in developing strategies to enhance tuber yield and resistance to yam mosaic virus in Dioscorea spp. The markers and candidate genes discovered in this research will serve as crucial genomic resources for selection of D. praehensilis and other yam species.
Introduction
Yam (Dioscorea spp.) is a multispecies crop (~600 species) that ranks fourth among root and tuber crops worldwide and second to cassava in West Africa (FAOSTAT, 2021). It had an estimated global production of 75.14 million tons, from nine million hectares in 2021, with West-Africa accounted for ~97% of the world production (FAOSTAT, 2021). Yam is an important crop that plays a crucial role in alleviating food insecurity and poverty in the tropics and subtropics. It provides high nutritional value in terms of carbohydrates, proteins, fats, fibers, essential minerals, and vitamins (Obidiegwu et al., 2020).
Dioscorea praehensilis, commonly known as the Bush Yam, is a semi-cultivated yam species that is primarily cultivated in West and Central Africa, where it plays a vital role in food security and income generation for local communities (Pitalounani et al., 2017; Adewumi et al., 2021). It possesses several desirable traits, such as high nutritional value, adaptability to diverse agroecological conditions, and resistance to various pests and diseases (Pitalounani et al., 2017; Adewumi et al., 2021).
However, as the world’s population grows, so is the demand for food, and food crops are threatened by poor yield, susceptible to diseases and poor resilience to climatic changes (Godfray et al., 2010; Bradshaw, 2017; FAO, 2019).
Among the significant challenges faced by yam cultivation is the threat of viral diseases, with Yam Mosaic Virus (YMV) being one of the most devastating pathogens affecting yam crops (Thouvenel and Dumont, 1990; Adeniji et al., 2012; Agre et al., 2021). YMV can cause severe reductions in tuber yield and quality, leading to significant economic losses for farmers (Adeniji et al., 2012). Developing yam cultivars with resistance to YMV is crucial to sustain yam production and maintaining food security.
Additionally, enhancing tuber yield and yield-related traits, such as tuber weight, size, and number per plant, is essential to meet the increasing demand for yam in domestic and international markets. Understanding the genetic basis of these traits in D. praehensilis can contribute to the development of improved varieties with enhanced productivity.
Genome-wide association studies (GWAS) have emerged as a powerful approach for dissecting the genetic architecture of complex traits in crops (Stanley et al., 2021). GWAS allows researchers to identify genomic regions associated with phenotypic variation by analyzing a large number of single nucleotide polymorphisms (SNPs) across the genome (Brachi et al., 2011; Gómez et al., 2011; Perseguini et al., 2016; Darvishzadeh, 2016). Application of GWAS has been used in root and tuber crops such as cassava (Esuma et al., 2016; Wolfe et al., 2016; Rabbi et al., 2017; Kayondo et al., 2018; do Carmo et al., 2020; Phumichai et al., 2022) and potato (Rosyara et al., 2016; Sharma et al., 2018; Okada et al., 2019; Naeem et al., 2021) to understand genetic basis of yield- related traits and disease resistance. In yam species, GWAS has been successfully applied to dissect the genetic basis of several traits such as tuber yield and YMV resistance (Agre et al., 2021; Adejumobi et al., 2023; Agre et al., 2023), anthracnose resistance (Adejumobi et al., 2023; Agre et al., 2022), dry matter content, oxidative browning and tuber flesh colour (Agre et al., 2023; Dossa et al., 2023; Ehounou et al., 2022); Gatarira et al., 2020), tuber quality (Asfaw et al., 2023), plant sex and cross-compatibility (Mondo et al., 2021; Asfaw et al., 2022) and plant vigour (Agre et al., 2023; Adejumobi et al., 2023). However, no information is available on the detection of SNP markers and putative genes associated with tuber yield, tuber size ratio and YMV resistance in D. praehensilis. The germplasm of D. praehensilis provides a valuable opportunity to uncover and identify the genomic regions and functional genes responsible for the genetic diversity in resistance to yam mosaic virus and traits related to tuber yield to enhance breeding strategies for marker assisted selection in yam improvement programme. Therefore, this study was conducted to identify quantitative trait nucleotides associated with tuber yield-related traits and YMV resistance in D. praehensilis using association mapping.
Materials and methods
Plant materials
One hundred and thirty-two (132) accessions of D. praehensilis, collected across three predominant yam-producing regions (Central, Eastern, and Western North) of Ghana, were used in this study Supplementary Table S1.
Phenotypic data collection
The experiment was laid out in a 12-by-11 simple lattice design with two replicates. Each plot size consisted of 5 m long ridges containing five plants at 1 m intra- and inter-row spacing. Data were collected on tuber yield per plant (TWPL), tuber size ratio (TSR) and yam mosaic virus (YMV) severity on each of the D. praehensilis genotypes evaluated for two seasons (2022 and 2023 seasons) at Teaching and Research Farm of the University Cape Coast, Ghana. Data related to the TWPL, TSR and YMV severity were recorded on three middle plants basis using the yam crop ontology (Asfaw, 2016).
The tuber yield per plant at harvest was evaluated at 10 months after planting using weighing balance (AND HV – 60KC). We quantitatively measured the size ratio of each tuber (TSR) by calculating the ratio of the length (the longest part of the tubers) to the width (the widest part of the tubers). We assessed the severity of yam mosaic virus (YMV) by visually examining the extent of leaf surface affected by the virus at 30-day intervals between 2 and 6 months after planting. This evaluation was based on a five-point scale ranging from 1 to 5. A score of 1 indicated no visible symptoms of virus infection, while scores of 2 to 5 represented increasing severity of symptoms, including (2) for mild mosaic, vein-banding, green spotting or flecking, curling and mottling on few leaves but no leaf distortion, (3) for low incidence (25–50%) of the mosaic virus on the entire plant, (4) for the severe mosaic on most leaves and leaf distortion, and (5) for severe mosaic and bleaching with severe leaf distortion and stunting.
To determine the area under the disease progression curve (AUDPC) for YMV over time, the severity scores of the virus were utilized. The AUDPC provides a quantitative measure of disease intensity or severity. The trapezoidal method (Campbell and Madden, 1990) was employed to estimate the AUDPC by discretizing the time variable and calculating the average disease intensity or severity between each pair of adjacent time points.
Where: is the number of observations, i is the disease severity at th observation, i is the time at th observation.
Phenotypic data analysis
Phenotypic data obtained from the two seasons were pooled and subjected to a linear mixed model analysis using the lme4 package implemented in R (Pinheiro et al., 2018). The best linear unbiased estimates (BLUEs) for two seasons were obtained by considering genotypes main effect as fixed and location and replication effect as random in the mixed model as follows:
where Yijkl = phenotypic observation for a trait, µ = grand mean, Si = season effect, Rj = replication effect nested in season, Gk = genotype effect, (Gj * Si) = genotype by season interaction, eijkl = random residual error.
Descriptive statistics were utilized to evaluate the variations in tuber yield, tuber size ratio and YMV severity among D. praehensilis accessions. These statistics included means, standard deviations, minimum and maximum values, and coefficients of variation. The lme4 package in R (Zhang et al., 2020) was used to generate the best linear unbiased expectation (BLUE) and two variance components (genotypic and phenotypic variances). Based on the estimated variance components, the broad-sense heritability was calculated as follows:
Where δg2 is genotypic variance, δp2 is phenotypic variance and n is number of observations.
The accessions’ BLUE values for TWPL, YMV and TSR traits derived from the best fit model, were used as input for the GWAS model.
Genotypic procedures
Processing of genotyping data
The DNA was extracted using a technique developed by Intertek-AgriTech (http://www.intertek.com/agriculture/agritech/) and based on the LGC oKtopure™ automated high-throughput ‘sbeadex™’ DNA extraction and purification system (https://www.biosearchtech.com/). Magnetic separation is used in the ‘sbeadex™’ technique to prepare nucleic acids. The first stage in this process is to homogenize leaf tissue samples in 96 deep-well plates using steel bead grinding. The ground tissue is treated with a DNA extraction buffer using LGC’s ‘sbeadex™’ kit for plant DNA preparation (https://www.biosearchtech.com/). Finally, super-paramagnetic particles coated with ‘sbeadexTM’ surface chemistry that catches nucleic acids from a sample are used to purify extracted DNA. Purified DNA is eluted and used in downstream procedures.
High-throughput genotyping was conducted in 96 plex DArTseq protocol, and SNPs were called using the DArT’s proprietary software, DArTSoft, as described by Kilian et al. (2016). Each sequencing result’s reads and tags were mapped to the D. rotundata reference genome v2 (https://drive.google.com/folders/1H5T4xjKAEl9LliR-4qKIR6TypCDe8nj) using Hisat2 (Sugihara et al., 2020; Kim et al., 2015). KDcompute (https://kdcompute.seqart.net/kdcompute, accessed on 30 June 2023) was used to convert the raw HapMap file to a Variant Call Format (VCF). Using the software PLINK 1.9 and VCFtools, SNP-derived markers were filtered to remove low quality SNP markers. Markers with minor allele frequencies <0.05 and low read depth (<5), genotype quality <20; and heterozygosity >50 were removed. In the end, 4,525 informative SNP markers distributed across the 20 yam chromosome were retained and used for the subsequent analysis.
Population structure
To analyze the population structure, the SNP panel (excluding Indels) underwent LD-based pruning using plink2 (Purcell and Chang, 2019) with the command –indep-pairwise 50 10 0.2. The population structure was then assessed using ADMIXTURE version 1.3.0 (Alexander et al., 2009), with the number of clusters (K) ranging from 1 to 10 and a CV value of 10. Each K value was run with 20 replicates, and the outputs were analyzed using pong (Behr et al., 2016). The suitable value for K was determined based on the CV error. Individuals were assigned to a specific population when their membership coefficient in that group was ≥0.50.
GWAS analysis, and gene annotation
The trait association analysis was conducted using mixed linear model implemented in GWASpoly package. The mixed linear model used was , where y represents the phenotypic observations (BLUEs for TWPL, YMV, and TSR), X represents the SNP markers (fixed effect), Z represents the random relation matrix, b represents the estimated SNP effects, u represents the random additive genetic effects, and e represents the random residual errors. For the trait association study, four different gene action models were considered: additive, general, dominant alternative (1-dom-alt), and dominant reference (1-dom-ref) (Rosyara et al., 2016). The GWAS analysis was conducted using BLUEs from multiple locations. The significance of QTNs and reduction of false positive QTNs were determined using a p-value threshold of 0.05 and limit of detection (LOD) thresholds adjusted by the Bonferroni correction method implemented in GwasPoly (Rosyara et al., 2016). To assess the appropriateness of the GWAS model and account for population structure, quantile-quantile (QQ) plots were generated by plotting the negative logarithms of the p-values against their expected values. Manhattan plots were also created to visualize the GWAS results across the entire genome, and zoom mapping was performed on specific chromosomes where significant SNP markers were identified. The effects of these markers were estimated using a multiple regression analysis, with the trait as the response variable and the significant SNP markers as the independent variables. For gene annotation, putative gene were searched in a 5 kb range (upstream and downstream) of the significant SNPs using the yam generic featuring file (GFF3). The functions of the genes associated with the identified SNPs were determined using the Interpro database from the European Molecular Biology Laboratory-European Bioinformatics Institute (EMBL-EBI) (Hunter et al., 2011).
The LD heatmap package (Shin et al., 2006) was used to perform linkage disequilibrium (LD) analysis and create a heatmap to visually represent pairwise LD measurements among SNPs significantly associated with individual traits. Pairwise LD estimates between chromosomes for markers with significant associations were calculated, and the results were plotted based on base pair (bp) distances using the “ggplot2” package in R (R Development Core Team, 2019).
Haplotype estimation and SNP markers effect prediction
The haplotype linked with a significant quantitative trait locus (QTL) was developed using the “ggsignif” and “ggpubr” packages in R (Yin, 2019). The sequence of each haplotype was determined based on 132 genetic materials used for testing and identification. The variant effect prediction was assessed through the adjusted posterior probability, identifying markers with high segregation. These marker effects were then visualized using ggplot2 in R (R Development Core Team, 2019).
Results
Phenotypic traits variation
Significant genotype and season effects (p ≤ 0.001) were observed for tuber yield per plant (TWPL) (Table 1). The response YMV severity were observed to be highly significant (p ≤ 0.001) for D. praehensilis genotype, season of planting and genotype-by-season interaction (Table 1). Significant mean squares were observed for genotype effect (p ≤ 0.001) and season effect (p ≤ 0.01) in tuber size ratio (Table 1).
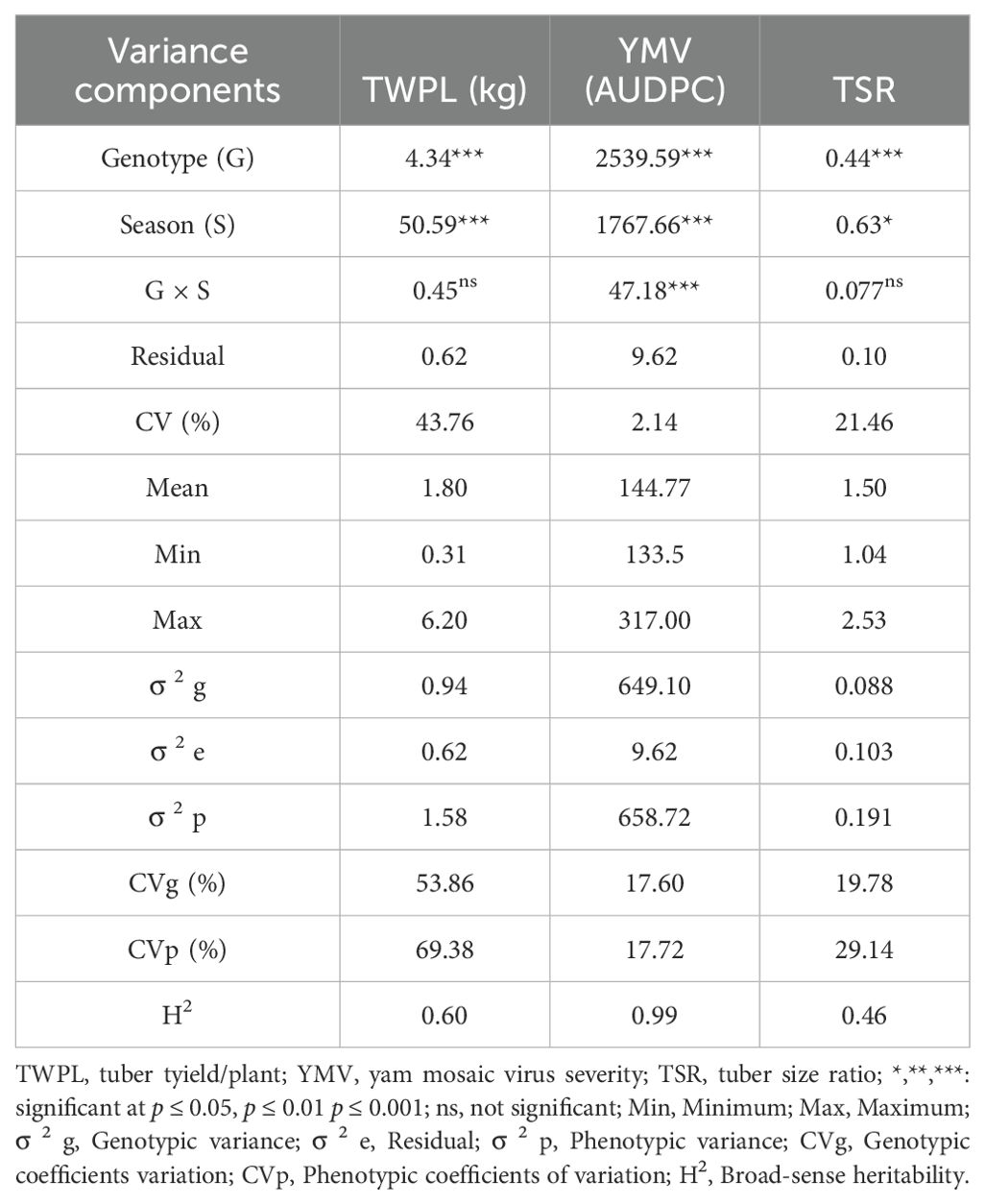
Table 1. Mean squares, means, estimates of variance components and broad-sense heritability of tuber yield, YMV severity and tuber size ratio od yield and other agronomic traits of 132 D. praehensilis accessions evaluated in 2022 and 2023 seasons.
Means for tuber yield per plant (TWPL) (2.11kg) was high with low tuber size ratio (1.46) in 2022, but low tuber yield/plant (1.49kg) with high tuber size ratio (TSR) (1.53) were observed in 2023 (Figure 1). Yam mosaic virus severity was higher in 2023 (146.61 AUDPC) compared to season 2022 (142.93 AUDPC) (Figure 1). The broad-sense heritability varied from 46% for tuber size ratio to 99% for YMV severity response.
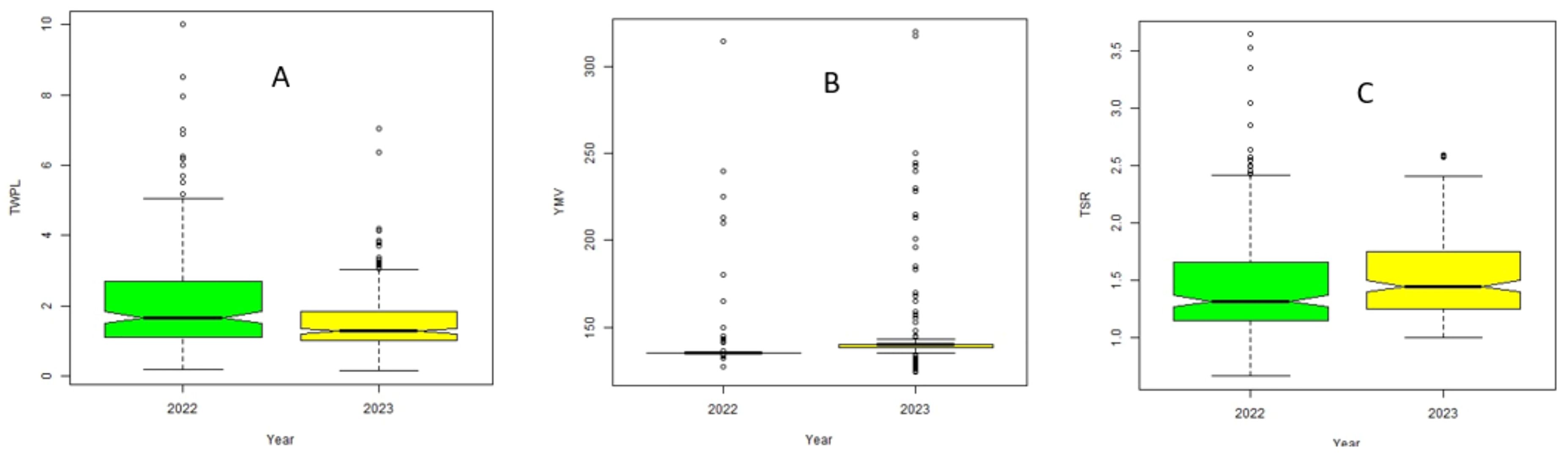
Figure 1. Boxplot showing mean distributions for (A) tuber yield/plant (TWPL); (B) yam mosaic virus (YMV) severity; (C) tuber size ratio (TSR) across 2022 and 2023 seasons.
Markers summary characteristic and population structure
A total of 4,525 SNPs were retained after quality filtering (Table 2; Supplementary Figure S1). The number of SNPs per chromosome ranged from 94 on chromosome 13 to 487 on chromosome 5 (Table 2). Most mutations were transitions (2,757; 60.93%), while transversions accounted for 1,768 SNPs (39.03%) (Supplementary Figure S2). Among the transition mutations, A/G had the highest occurrence rate (1,575), while A/T had the highest occurrence rate among the transversion mutations (668). The polymorphism information content (PIC) of the SNPs ranged from 0.08 on chromosome 4 to 0.138 on chromosome 12, with an average of 0.1. The minor allelic frequency (MAF) ranged from 0.071 to 0.116, with a mean of 0.093. The observed heterozygosity (Ho) varied from 0.096 to 0.187, with an average of 0.127. The expected heterozygosity (He) ranged from 0.098 to 0.175, with an average of 0.124 (Table 2).
Based on the population structure analysis, a clear distinction was observed at K=3, indicating the presence of three subpopulations (Supplementary Figure S3; Supplementary Table S2). Using a membership probability threshold of 50%, 127 accessions were successfully assigned to their respective subpopulations, while the remaining 5 accessions with membership probabilities below 50% were classified as admixt (Supplementary Figure S3; Supplementary Table S2). Subpopulation 1 had the highest number of accessions (78), followed by subpopulation 2 (33), and subpopulation 3 had the lowest number of accessions (16). The subpopulation 1 comprised of accessions from Central region (~ 64%), Eastern region (20.5%) and Western North region (15.4%), while subpopulations 2 and 3 consisted of accessions mostly from Western North regions (Supplementary Figure S3; Supplementary Table S2). The admixt consisted of three and two accessions from Central and Eastern regions, respectively (Supplementary Figure S3; Supplementary Table S2).
Identification of SNP markers associated with tuber yield related traits and yam mosaic virus
GWAS analysis for tuber yield
Marker-trait association analysis identified 20 SNP markers located on chromosomes 1, 3, 4, 5, 6, 7, 9, 10, 12, 13, 15, 18 and 19 to be significant associated with tuber yield across seasons 2022 and 2023 (Table 3; Figure 2). Ten of these SNPs were detected in 2022 season, and eleven were identified in 2023 season. The estimated total phenotypic variation ranged from SNP chrom_06_10592044 (3.33%) to SNP chrom_18_10302290 (20.20%). The LOD scores ranged from 3.17 for SNP chrom_03_14398242 to 4.11 for SNP chrom_12_145723 (Table 3).
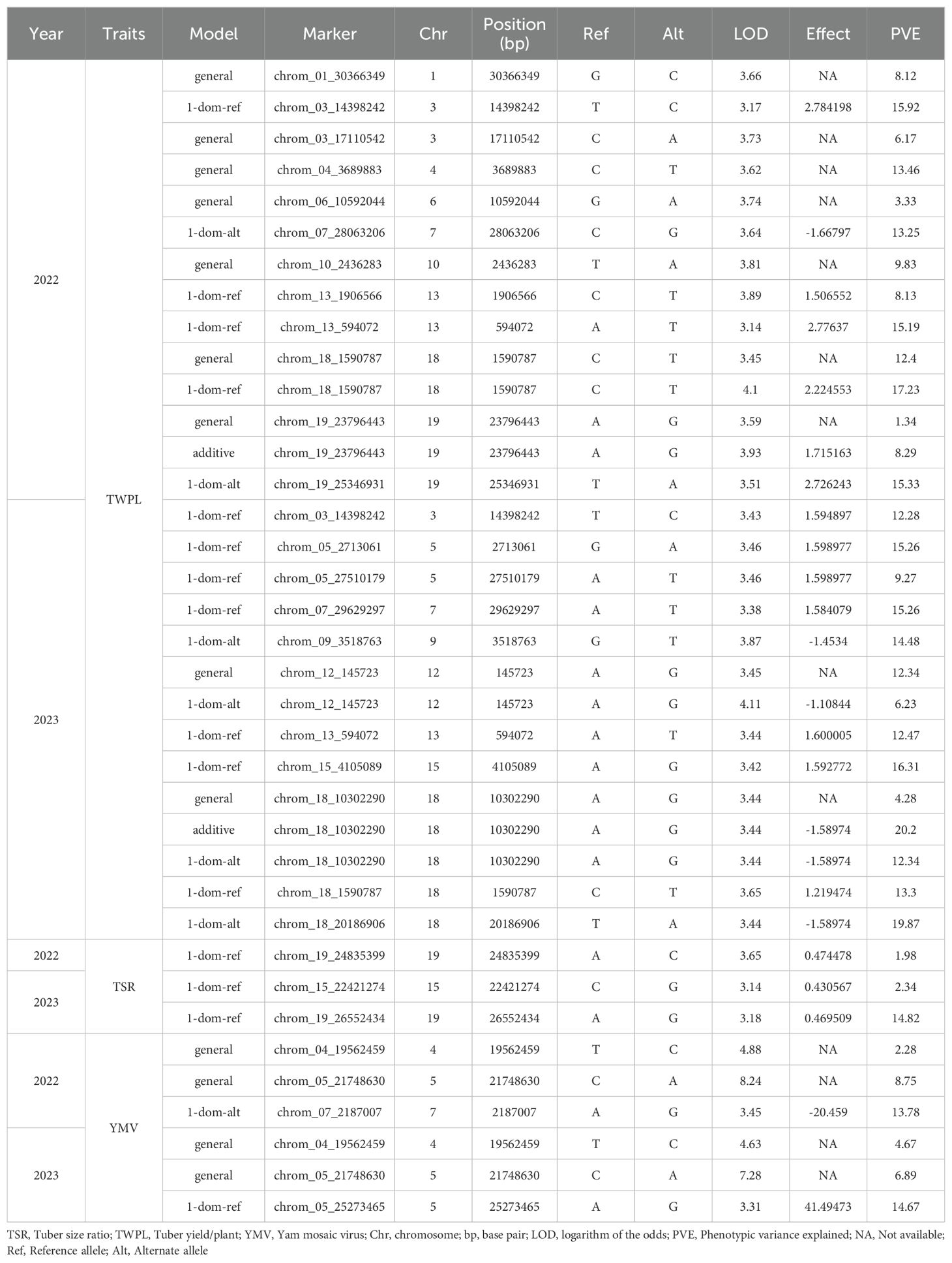
Table 3. SNP markers associated with tuber yield-related traits and response to yam mosaic virus severity of D. praehensilis.
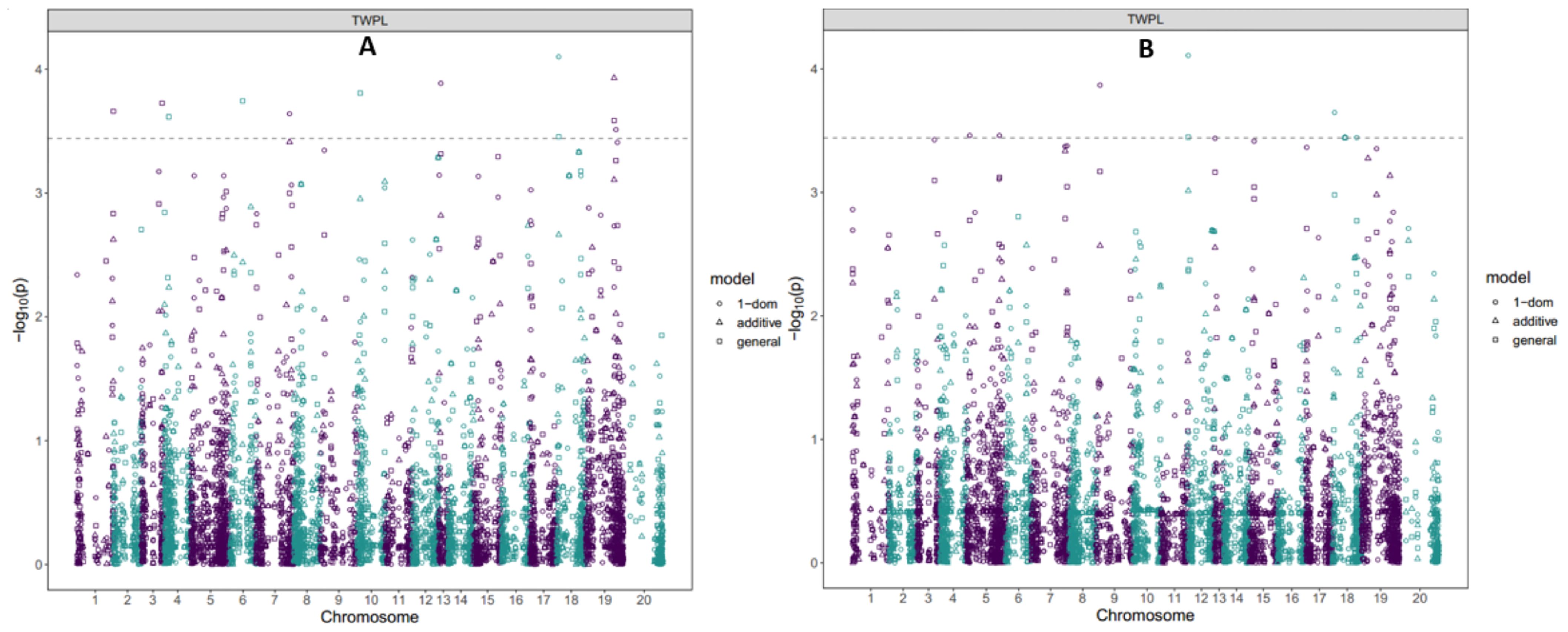
Figure 2. Manhattan plot showing GWAS for SNPs associated with tuber yield at seasons (A) 2022; (B) 2023. SNP variants were considered significant at LOD of 3.5 determined by the Bonferroni correction factor.
Of this SNP markers associated with tuber yield in 2022 season, SNP chrom_18_1590787 was detected by both general and dominant reference (1-dom-ref) gene action models, SNP chrom_19_23796443 was detected by general and additive gene action models, while six SNPs were identified only general gene action model, three SNPs by only dominant reference (1-dom-ref) gene action model and two SNPs by only simple dominant (1-dom-alt) gene action model (Figure 2; Supplementary Figure S4). Of these SNP markers linked with tuber yield in 2023 season, SNP chrom_18_10302290 was detected by general, additive and simple dominant (1-dom-alt) gene action model, SNP chrom_12_145723 was detected by general, and simple dominant (1-dom-alt), seven SNPs were detected by dominant reference (1-dom-ref), and one SNP by simple dominant (1-dom-alt) (Figure 2; Supplementary Figure S4).
GWAS analysis for tuber size ratio
We identified three SNP markers significantly associated with tuber size ratio (TSR) across the two seasons (Table 3; Figure 3). Among the three SNP markers, two (chrom_19_24835399 and chrom_19_26552434) were found on chromosome 19 at physical positions of 24,835,399 and 26,552,434 bp, respectively (Table 3). The LOD scores for these two SNP markers were 3.65 and 3.18, respectively, and together they accounted for 16.80% of the total phenotypic variation (Table 3). The third SNP locus related to the tuber size ratio (TSR) was found on chromosome 15 at a physical position of 22,421,274 bp (chrom_15_22421274). This SNP had a LOD score of 3.14 and explained 2.34% of the total phenotypic variation in the tuber size ratio (Table 3). One of the three SNPs (chrom_19_24835399) was detected in the 2022 season, while the other two (chrom_15_22421274 and chrom_19_26552434) were identified in the 2023 season (Figure 3; Supplementary Figure S5). All three SNP markers were identified through dominant reference (1-dom-ref) gene action model (Figure 3; Supplementary Figure S5).
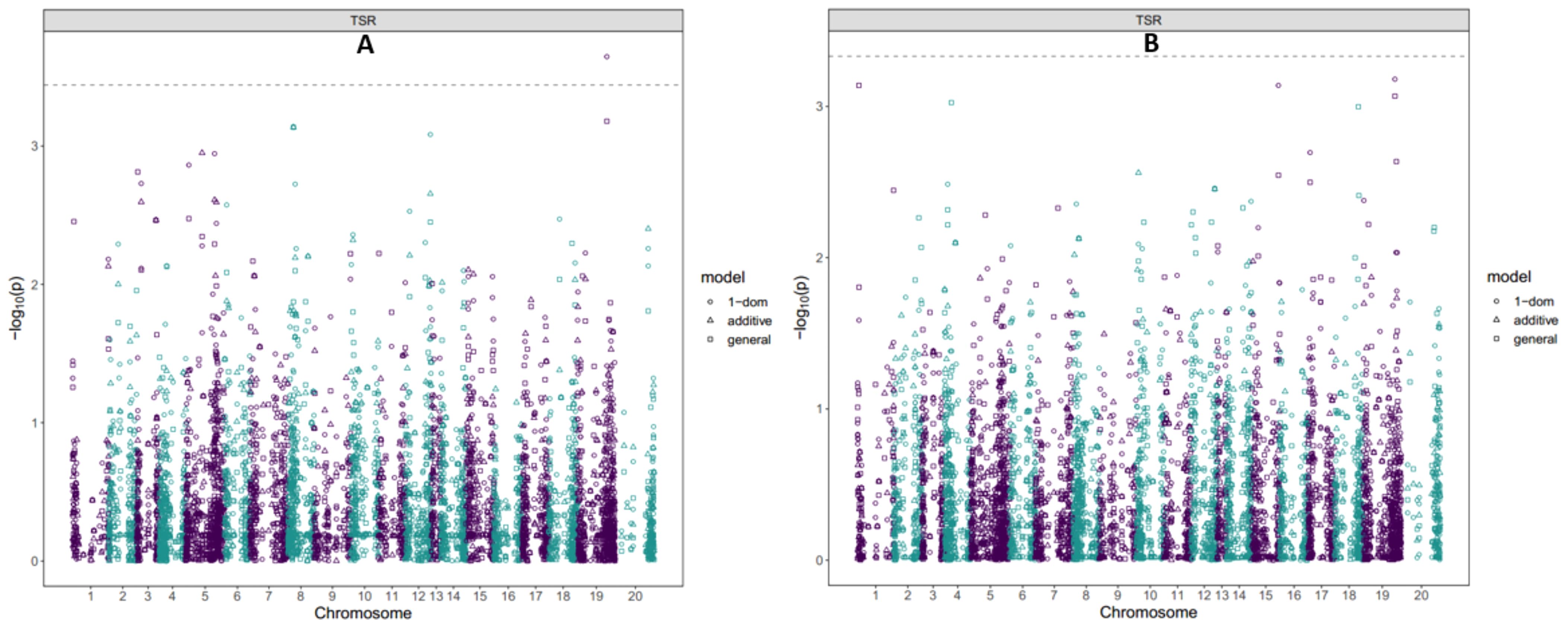
Figure 3. Manhattan plot showing GWAS for SNPs associated with tuber size ratio at seasons (A) 2022; (B) 2023. SNP variants were considered significant at LOD of 3.5 determined by the Bonferroni correction factor.
GWAS analysis for YMV
Four SNP markers were detected to be significantly associated with response to yam mosaic virus severity across the two seasons (Table 3; Figure 4). Two of these SNPs were located on chromosome 5 (chrom_05_21748630 and chrom_05_25273465) with LOD values of 8.24 and 3.31, respectively, and explained 23.42% of total phenotypic variation in response to YMV severity (Table 3). The other two SNPs were located each on chromosome 4 (chrom_04_19562459) and chromosome 7 (chrom_07_2187007) (Table 3; Figure 4), with LOD of 4.63 and 3.45, respectively, and explained phenotypic variation of 4.67 and 13.78%, respectively (Table 3). Two of the SNPs (chrom_04_19562459 and chrom_05_21748630) associated with YMV severity response were detected through general gene action model in both seasons 2022 and 2023, while SNP markers chrom_05_25273465 and chrom_07_2187007 were detected through dominant reference (1-dom-ref) gene action model (Figure 4; Supplementary Figure S6).
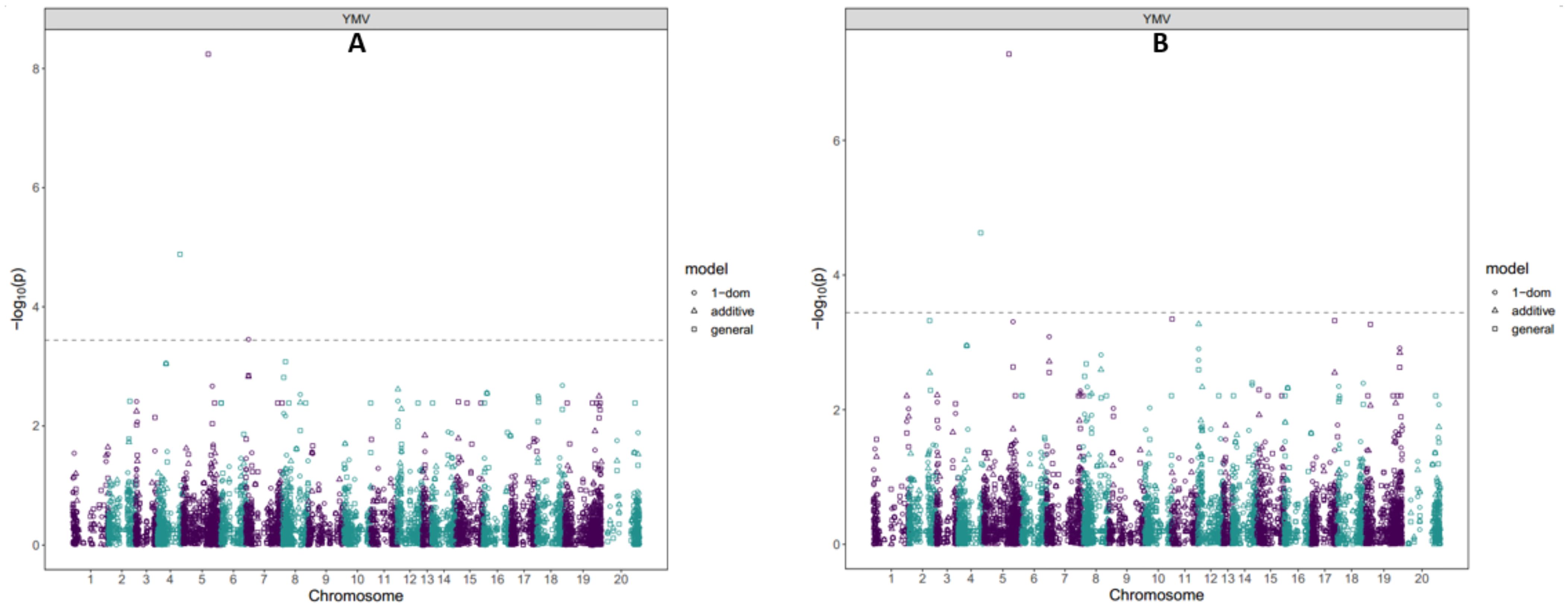
Figure 4. Manhattan plot showing GWAS for SNPs associated with response to yam mosaic virus severity at seasons (A) 2022; (B) 2023. SNP variants were considered significant at LOD of 3.5 determined by the Bonferroni correction factor.
SNP markers interaction
The interaction between seasons and SNP markers related to tuber yield, YMV severity response and tuber size ratio are presented in Table 4. Of the twenty SNP markers that are associated with tuber yield per plant, seven of the SNP markers showed significant SNP by season interaction. Of these seven significant SNPs, three (SNP chrom_03_17110542, SNP chrom_13_594072 and SNP chrom_18_20186906) were stable across the two seasons, two (SNP chrom_07_28063206 and SNP chrom_19_25346931) were specific to season 2022, while other two (SNP chrom_07_29629297 and SNP chrom_12_145723) were specific to season 2023 (Table 4). Of the four SNP markers linked to YMV severity response, two were stable across the seasons, but showed no significant SNP by season interaction, while SNP chrom_07_2187007 showed significant SNP by season interaction with season 2022 (Table 4). For tuber size ratio, neither of the identified SNP markers showed stability across the seasons nor significant interaction with the seasons.
Gene annotations of putative genes associated with tuber yield and yam mosaic virus severity
Through annotation genes, we identified a total of ten and five putative genes associated with tuber yield and yam mosaic virus severity, respectively in the genomic regions of five stable SNP markers across seasons 2022 and 2023 (Table 5). Among these, we found Protein kinase domain (IPR000719), Protein kinase-like domain (IPR011009) and Leucine-rich repeat, cysteine-containing subtype (IPR006553) genes located on genomic region of chrom_03_17110542 (Table 5; Figure 5A). Additionally, Serine/threonine-/dual specificity protein kinase, catalytic domain (IPR002290) and Protein kinase, ATP binding site (IPR017441) genes were detected on SNP marker (chrom_13_594072) associated with tuber yield on chromosome 13 (Table 5; Figure 5B). We also identified Brevis radix (BRX) domain (IPR013591) and Transcription factor BREVIS RADIX, N-terminal domain (IPR027988) genes on the genomic region of SNP chrom_18_20186906 located on chromosome 18 (Table 5; Figure 5C).
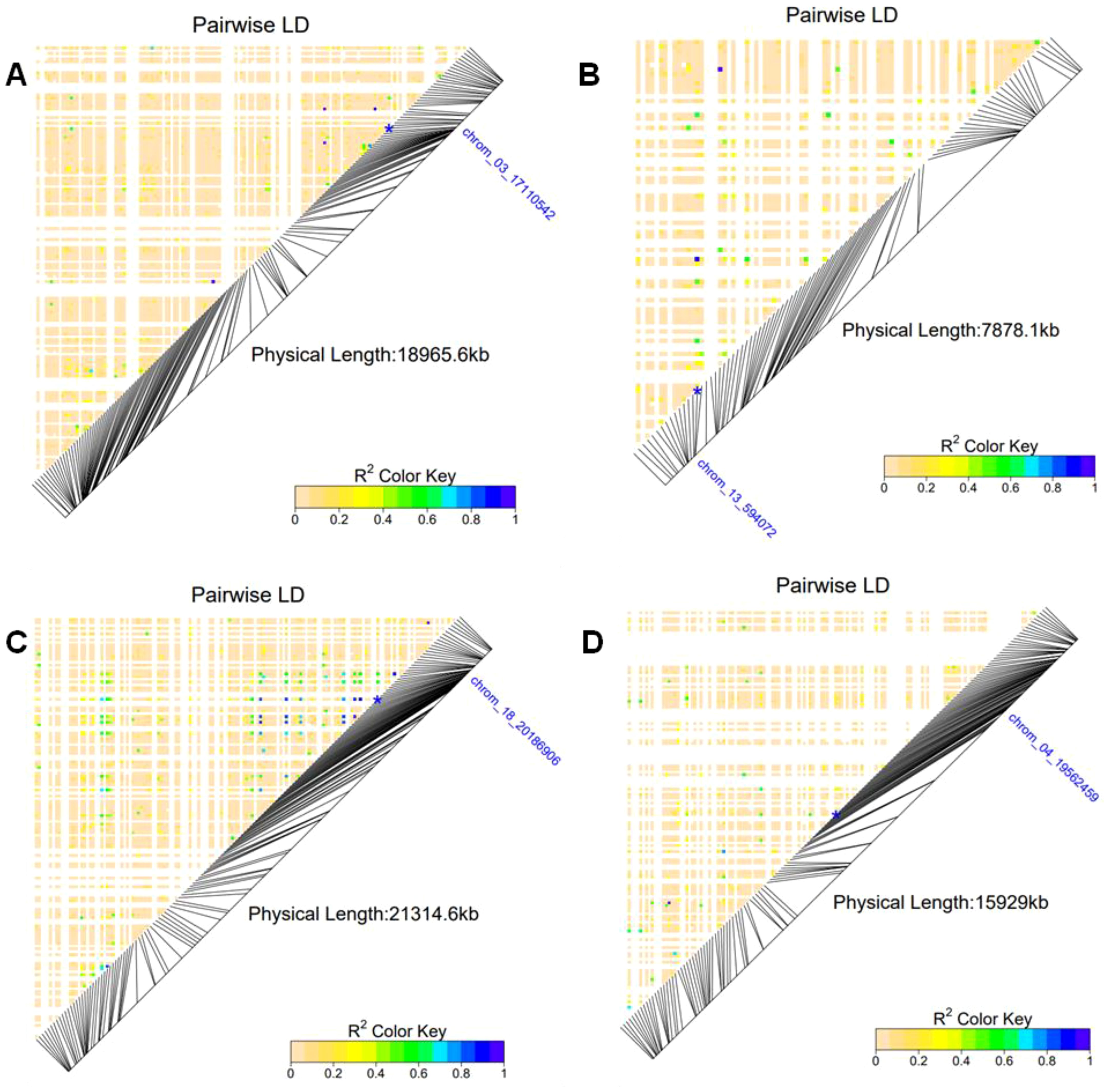
Figure 5. Heatmap LD haplotype blocks for different SNP markers located on different chromosomes associated with tuber yield, and response to YMV severity. (A) SNP chrom_03_17110542, (B) chrom_13_594072 and (C) SNP chrom_18_20186906 associated with tuber yield and (D) SNP chrom_05_21748630 associated with the response YMV severity. The R2 color key indicates the degree of significant association with the putative genes.
For YMV, six putative candidate genes, of which three such as Aspartate/other aminotransferase (IPR000796), Pyridoxal phosphate-dependent transferase (IPR015424), Aminotransferase, class I/classII (IPR004839), histone deacetylase domain (IPR023801) were located on genomic region of chromosome 4, while Ribosomal protein L5 (IPR002132), Peptidase C1A, papain (IPR013128) and Haem peroxidase, plant/fungal/bacterial (IPR002016) were identified near the peak of SNP chrom_05_21748630 (Table 5; Figure 5D).
Marker effects and allele segregation
Marker prediction effects of various alleles associated with tuber yield and yam mosaic virus severity response of D. praehensilis are presented in Table 6 and Figure 6. The three SNPs that are stable across the two seasons for tuber yield showed high significant allele segregation among the haplotypes (Table 6; Figure 6). SNPs chrom_13_594072 and chrom_18_20186906 identified alleles TT and AT to be significantly associated with high tuber yield, while allele AA linked with low tuber yield. SNP chrom_03_17110542 identified allele CC to be significantly linked with high tuber yield, while AC linked with low tuber yield (Table 6; Figure 6). Of the two stable SNPs associated with response to YMV severity, SNP chrom_04_19562459 showed significant allele segregation (Table 6; Figure 6). For this SNP, alleles CC and CT were detected to be significantly associated with YMV resistance in D. praehensilis, while allele TT significantly associated with susceptibility to YMV (Table 6; Figure 6).
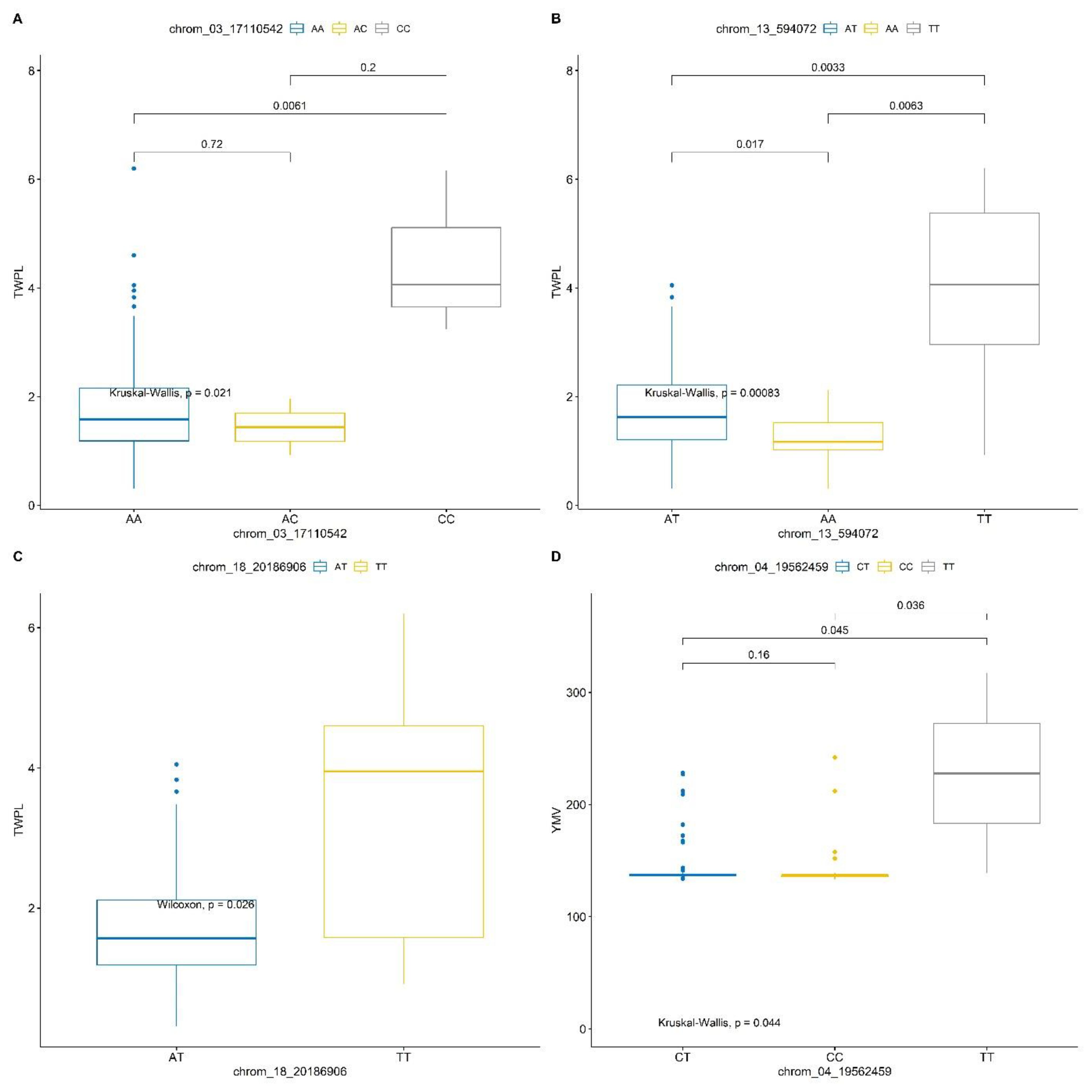
Figure 6. Haplotype prediction effects of significant SNPs associated with tuber yield (A–C) and response to YMV severity (D).
Discussion
In this study, we explored the use of trait association approach to understand and determine the genomic region underlying the tuber yield per plant, the yam mosaic virus and the tuber size ratio in a semi-cultivated yam species. The significant season effects observed in the three traits and the significant genotype x season interaction effect observed in YMV severity response implies the need for multiple evaluations of these traits across seasons and locations to identify genotypes with higher yield and stable resistance to YMV. The significant genotype x season observed in YMV severity response indicates that the behavior of different accessions depends on the specific evaluation environments. Significant accession by year interaction was reported in the GWAS study conducted in multiple yam species from Congo Democratic Republic (Adejumobi et al., 2023). The high broad-sense heritability estimates (≥60%) observed for tuber yield and YMV severity response and moderately high broad-sense heritability estimate (30-60%) for tuber size ratio implies the presence of genetic diversity in the studied traits among the D. praehensilis genotypes and these traits are amenable to genetic improvement through selection. High broad-sense heritability has been reported by Agre et al. (2022) and Adejumobi et al. (2023) on association mapping conducted on D. rotundata and yam multiple species, respectively. The Heritability estimate is a crucial parameter in breeding programs that is used to estimate the response to selection and explain the proportion of phenotypic variation attributed to genetic variations (Falconer and Mackay, 1996).
A total of 27 SNP markers distributed on 13 chromosomes were detected to be linked to the tuber yield, yam mosaic virus severity, and tuber size ratio in D praehensilis. Specifically, 20 of these SNPs were found to be linked to tuber yield, while four were associated to yam mosaic virus severity, and three were related to tuber size ratio. Of the four gene action models used, the dominant reference (1-dom-ref) gene action model was found to detect the highest number of SNPs. Conversely, the additive gene model detected the lowest number of QTNs in all three traits (Table 3). This implies the high efficient nature of this GWAS model in the detection of QTNs linked to complex quantitative traits. Although, dominant reference (1-dom-ref) and general gene action models are very effective in the detection of stable SNPs across several environments.
The majority of the SNPs discovered in this study were found to be specific to individual environments, suggesting frequent interactions between SNPs and the environment. These interactions pose a significant challenge in selecting SNPs for breeding programs, as they are more susceptible to environmental influences (Delfini et al., 2021). Conversely, the stable SNPs demonstrated a notable progression in tuber yield and YMV severity response throughout the study, highlighting the gradual enhancement achieved by accumulating favorable alleles. In this study, most of the identified SNPs had a small effect, except for the SNP (chrom_05_25273465) that was found to have a higher marker effect (41.49) for YMV severity response (Table 3). These small marker effects for SNPs associated with tuber yield-related traits and yam mosaic virus resistance had been reported in the study conducted by Agre et al. (2021) and Adejumobi et al. (2023) and this highlights the complex and quantitative nature of traits in Discorea spp. Since many of the traits studied are controlled by multiple genes, the individual effect of each locus is relatively small. However, it is still important to identify these small-effect loci as they can collectively explain the variation in a trait (Nakano and Kobayashi, 2020). While selecting SNPs with higher effects is preferable for selection assisted by molecular markers (SAM) (Nadeem et al., 2018; Oladosu et al., 2019), the use of small-effect SNPs associated with the traits of interest is considered an important strategy in genomic selection (GS). This is because these SNPs alone can replace the need for high-density genotyping with random SNPs, thereby reducing genotyping costs.
Using D. rotundata reference genome being the closely related yam species to D. praehensilis, ten and five putative genes were identified upstream and downstream of the three and two stable SNPs associated with tuber yield and YMV severity response, respectively. For tuber yield, the putative genes or protein families such as Protein kinase domain, and Protein kinase-like domain harbored on chromosome 3 and Protein kinase, ATP binding site detected on chromosome 13 are involved in enhancing the tolerance of plant to drought and water stress (Zhu et al., 2022). Leucine-rich repeat, cysteine-containing subtype located around the vicinity of the SNP marker (chrom_03_17110542) on chromosome 3 confers resistance virus thereby enhancing tuber development and yield (Bendahmane et al., 2002). Serine/threonine-/dual specificity protein kinase, catalytic domain gene or protein family located at the SNP peak site of chromosome 13 has been reported to play significant role in enhancing process of starch and sugar biosynthesis and stimulated glucose pyrophosphorylase (Geigenberger, 2003). Serine/threonine-/dual specificity protein kinase family has been reported to play a crucial role in the dry matter content of tuber in D. alata (Gatarira et al., 2020). The two putative genes or protein families (Brevis radix (BRX) domain and Transcription factor BREVIS RADIX, N-terminal domain) harbored near SNP peak site of chromosome 18 have been reported to involve in regulating cell proliferation and elongation in the root (Briggs et al., 2006). These genes have also been reported in Arabidopsis thaliana to be involved in root growth (Beuchat et al., 2010).
For YMV severity response, the SNPs on chromosome 4 are near to Aspartate/other aminotransferase, Pyridoxal phosphate-dependent transferase and Aminotransferase, class I/classII, which are involved in nitrogen metabolism and in aspects of carbon and energy metabolism (Sung et al., 1991). These putative genes play a significant role in reducing disease susceptibility in rice and Arabidopsis thaliana (Song et al., 2004). The SNP in chromosome 5 is near to Ribosomal protein L5. Ribosomal protein L5 has been implicated to involve in ribosome assembly and protein translation (Iwasaki et al., 2002). This putative gene involves in defense signaling of host cells by activating immune pathway against virus (Shuo, 2019). Peptidase C1A, papain gene is located at the peak SNP site of chromosome 5 and plays a determinative role in regulating pathogen defense (Liu et al., 2018). It also involved in the synthesis of pathogenesis-related (PR) proteins (Almagro et al., 2008).
The SNP marker-yield trait association demonstrated significant haplotype segregation. Alleles CC and TT were identified as predictors of high tuber yield in D. praehensilis germplasm, whereas alleles AA and AC were associated with low yield. Similarly, Agre et al. (2021) reported the association of allele CC with high tuber yield in white Guinea yam. For YMV, alleles CC and CT were found to predict low YMV disease susceptibility in D. praehensilis, while allele TT was linked to high disease susceptibility.
Conclusion
In this study, a total of 27 unique SNPs were found to be significantly linked to tuber yield-related traits and the severity of yam mosaic virus (YMV). Among these SNPs, five were stable across different cultivation seasons, with three associated with tuber yield and two with YMV severity. However, no stable SNPs were detected for tuber size ratio. These findings highlight the importance of conducting phenotyping in various environments and using multiple detection methods to ensure the reliability of SNPs identified in genome-wide association studies (GWAS).
Furthermore, the study identified several novel genes, including 10 promising genes for tuber yield and five genes for YMV severity response. These genes have the potential to enhance yam genetic improvement for tuber yield and YMV resistance through marker-assisted breeding approaches, after undergoing validation. Additional studies, such as transcriptome analysis and fine mapping with increased marker density, will be needed to validate these associations and candidate genes.
Validating genotypes with favorable alleles will provide valuable breeding materials for improving D. praehensilis in terms of tuber yield-related traits and YMV resistance through marker-assisted selection in future breeding endeavors.
Data availability statement
The datasets presented in this study can be found in online repositories. The names of the repository/repositories and accession number(s) can be found in the article/Supplementary Material.
Author contributions
AA: Data curation, Formal analysis, Methodology, Writing – original draft, Writing – review & editing. IA: Writing – original draft, Writing – review & editing. VO: Supervision, Writing – review & editing. PAs: Supervision, Writing – review & editing. MA: Supervision, Writing – review & editing. KT: Supervision, Writing – review & editing. AS: Writing – review & editing. TO: Writing – review & editing. EA: Writing – review & editing. SA: Writing – review & editing. HM: Writing – review & editing. PAA: Conceptualization, Data curation, Formal analysis, Funding acquisition, Investigation, Methodology, Project administration, Resources, Software, Supervision, Validation, Visualization, Writing – original draft, Writing – review & editing.
Funding
The author(s) declare financial support was received for the research, authorship, and/or publication of this article. We acknowledge, funding support from the Bill and Melinda Gates Foundation (BMGF/PP1052998). We appreciate financial support provided by the International Foundation for Science (IFS) under grant agreement I-3-C-6624-1 and BAYER Foundation and Alexander von Humboldt (AvH) Foundation through the program “AGNES-BAYER Foundation Research Grant for Biodiversity Conservation and Sustainable Agriculture in Sub-Saharan Africa”.
Acknowledgments
Authors acknowledge the assistance of field assistants of Teaching and Research Farm of U.C.C, Cape Coast, Ghana for their assistance in carry field activities and the laboratory supervisor of Yam Breeding Unit, I.I.T.A, Ibadan, Nigeria for his assistance in genotyping activities.
Conflict of interest
The authors declare that the research was conducted in the absence of any commercial or financial relationships that could be construed as a potential conflict of interest.
Publisher’s note
All claims expressed in this article are solely those of the authors and do not necessarily represent those of their affiliated organizations, or those of the publisher, the editors and the reviewers. Any product that may be evaluated in this article, or claim that may be made by its manufacturer, is not guaranteed or endorsed by the publisher.
Supplementary material
The Supplementary Material for this article can be found online at: https://www.frontiersin.org/articles/10.3389/fhort.2024.1459476/full#supplementary-material
References
Adejumobi I. I., Agre P. A., Adewumi A. S., Shonde T. E., Cipriano I. M., Komoy J. L., et al. (2023). Association mapping in multiple yam species (Dioscorea spp.) of quantitative trait loci for yield-related traits. BMC Plant Biol 23, 1–16. doi: 10.1186/s12870-023-04350-4
Adeniji M., Shoyinka S., Ikotun. T., Asiedu R., Hughes J. D., Odu B. (2012). Yield loss in Guinea yam (Dioscorea rotundata poir.) due to infection by yam mosaic virus (YMV) genus potyvirus. IJS 14, 237–244.
Adewumi A. S., Asare P. A., Adu M. O., Taah K. J., Akaba S., Mondo J. M., et al. (2021). A. Farmers’ perceptions on varietal diversity, trait preferences and diversity management of bush yam (Dioscorea praehensilis Benth.) in Ghana. Sci. Afr 12, e00808. doi: 10.1016/j.sciaf.2021.e00808
Agre P. A., Darkwa K., Olasanmi B., Kolade O., Mournet P., Bhattacharjee R., et al. (2022). Identification of QTLs controlling resistance to anthracnose disease in water yam (Dioscorea alata). Genes 13, 347. doi: 10.3390/genes13020347
Agre P. A., Edemodu A., Obidiegwu J. E., Adebola P., Asiedu R., Asfaw A. (2023). Variability and genetic merits of white Guinea yam landraces in Nigeria. Front. Plant Sci. doi: 10.3389/fpls.2023.1051840
Agre P. A., Norman P. E., Asiedu. R., Asfaw A. (2021). Identification of quantitative trait nucleotides and candidate genes for tuber yield and mosaic virus tolerance in an elite population of white Guinea yam (Dioscorea rotundata) using genome-wide association scan. BMC Plant Biol 21, 552. doi: 10.1186/s12870-021-03314-w
Alexander D. H., Novembre J., Lange K. (2009). Fast model-based estimation of ancestry in unrelated individuals. Genome Res 191655–1664. doi: 10.1101/gr.094052.109
Almagro L., Gomez Ros L. V., Belchi-Navarro S., Bru R., Ros Barcelo A., Pedreno M. A. (2008). Class III peroxidases in plant defence reactions. J. Exp. Bot 60, 377–390. doi: 10.1093/jxb/ern277
Asfaw A. (2016). Standard operating protocol for yam variety performance evaluation trial (IITA, Ibadan, Nigeria). pp. 27 (Issue April). doi: 10.13140/RG.2.1.3656.4887
Asfaw A., Agre P., Matsumoto R., Olatunji A. A., Edemodu A., Olusola T., et al. (2023). Genome-wide dissection of the genetic factors underlying food quality in boiled and pounded white Guinea yam. J. Sci. Food Agriculture.
Asfaw A., Mondo J. M., Agre P. A., Asiedu R., Akoroda M. O. (2022). Association mapping of plant sex and cross-compatibility related traits in white Guinea yam (Dioscorea rotundata Poir.) clones. BMC Plant Biol 22, 1–12. doi: 10.1186/s12870-022-03673-y
Behr A. A., Liu K. Z., Liu-Fang G., Nakka P., Ramachandran S. (2016). pong: fast analysis and visualization of latent clusters in population genetic data. Bioinformatics 32, 2817–2823. doi: 10.1093/bioinformatics/btw327
Bendahmane A., Farnham G., Moffett P., Baulcombe D. C. (2002). Constitutive gain-of-function mutants in a nucleotide binding site–leucine rich repeat protein encoded at the Rx locus of potato. Plant J 32, 195–204. doi: 10.1046/j.1365-313X.2002.01413.x
Beuchat J., Scacchi E., Tarkowska D., Ragni L., Strnad M., Hardtke C. S. (2010). BRX promotes Arabidopsis shoot growth. New Phytol 188, 23–29. doi: 10.1111/j.1469-8137.2010.03387.x
Brachi B., Morris G. P., Borevitz J. O. (2011). Genome-wide association studies in plants: the missing heritability is in the field. Genome Biol 12, 232. Available at: http://genomebiology.com/2011/12/10/232.
Bradshaw J. E. (2017). Plant breeding: past, present and future. Euphytica 213, p.60. doi: 10.1007/s10681-016-1815-y
Briggs G. C., Mouchel C. F., Hardtke C. S. (2006). Characterization of the plant-specific BREVIS RADIX gene family reveals limited genetic redundancy despite high sequence conservation. Plant Physiol 140, 1306–1316. doi: 10.1104/pp.105.075382
Campbell C. L., Madden L. V. (1990). Introduction to plant disease epidemiology (John Wiley & Sons).
Darvishzadeh R. (2016). Population structure, linkage disequilibrium and association mapping for morphological traits in sunflower (Helianthus annuus L.). Biotechnol. Biotechnol. Equip 30, 236–246. doi: 10.1080/13102818.2015.1136568
Delfini J., Moda-Cirino V., dos Santos Neto J., Zeffa D. M., Nogueira A. F., Ribeiro L. A. B., et al. (2021). Genome-wide association study identifies genomic regions for important morpho-agronomic traits in mesoamerican common bean. Front. Plant Sci 12. doi: 10.3389/fpls.2021.748829
do Carmo C. D., e Sousa M. B., Brito A. C., de Oliveira E. J. (2020). Genome-wide association studies for waxy starch in cassava. Euphytica. doi: 10.1007/s10681-020-02615-9
Dossa K., Morel A., Houngbo M. E., Mota A. Z., Maledon E., Irep J. L., et al. (2023). Genome-wide association studies reveal novel loci controlling tuber flesh color and oxidative browning in Dioscorea alata. J. Sci. Food Agriculture.
Ehounou A. E., Cormier F., Maledon E., Nudol E., Vignes H., Gravillon M. C., et al. (2022). Identification and validation of QTLs for tuber quality related traits in greater yam (Dioscorea alata L.). Sci. Rep 12, 8423. doi: 10.1038/s41598-022-12135-2
Esuma W., Herselman L., Labuschagne M. T., Ramu P., Lu F., Baguma Y., et al. (2016). Genome-wide association mapping of provitamin A carotenoid content in cassava. Euphytica. doi: 10.1007/s10681-016-1772-5
FAO. (2019). New UN report reveals that hunger in Africa continues to rise. Available online at: https://www.fao.org/news/story/en/item/1180443/icode/ (Accessed 26 November 2021).
FAOSTAT. (2021). Food and Agriculture Organization Cooperate Statistical Database Available online at: http://www.fao.org/faostat/en/#data/QL (Accessed 10th August 2021).
Gatarira C., Agre P., Matsumoto R., Edemodu A., Adetimirin V., Bhattacharjee R., et al. (2020). Genome-wide association analysis for tuber dry matter and oxidative browning in water yam (Dioscorea alata L.). Plants 9, 969. doi: 10.3390/plants9080969
Geigenberger P. (2003). Regulation of sucrose to starch conversion in growing potato tubers. J. Exp 54, 457–465. doi: 10.1093/jxb/erg074
Godfray H. C., Beddington J. R., Crute I. R., Haddad L., Lawrence D., Muir J. F., et al. (2010). Food security: the challenge of feeding 9 billion people. Science 327, 812–818. doi: 10.1126/science.1185383
Gómez G., Álvarez M. F., Mosquera T. (2011). Association mapping, a method to detect quantitative trait loci: statistical bases Mapeo por asociación, un método para la detección de loci de rasgos cuantitativos: bases estadísticas. Agron. Colomb 29, 367–376.
Hunter S., Jones P., Mitchell A., Apweiler R., Attwood T. K., Bateman A., et al. (2011). InterPro new developments in the family and domain prediction database. Nucleic Acids Res 40, 306–312. doi: 10.1093/nar/gkr948
Iwasaki K., Kikukawa S., Kawamura S., Kouzuma Y., Tanaka I., Kimura M. (2002). On the interaction of ribosomal protein L5 with 5S rRNA. Biosci. Biotechnol. Biochem 66, 103–109. doi: 10.1271/bbb.66.103
Kayondo S. I., Pino Del Carpio D., Lozano R., Ozimati A., Wolfe M., Baguma Y., et al. (2018). Genome-wide association mapping and genomic prediction for CBSD resistance in Manihot esculenta. Sci. Rep 8, 1549.
Kilian A., Sanewski G., Ko L. (2016). The application of DArTseq technology to pineapple. Acta Hortic 1111, 181–188. doi: 10.17660/ActaHortic.2016.1111.27
Kim D., Langmead B., Salzbergl S. L. (2015). HISAT: a fast spliced aligner with low memory requirements Daehwan HHS Public Access. Nat. Methods 12, 357–360. doi: 10.1038/nmeth.3317.HISAT
Li J., Mo X., Wang J., Chen N., Fan H., Dai C., et al. (2009). BREVIS RADIX is involved in cytokinin-mediated inhibition of lateral root initiation in Arabidopsis. Planta 229, 593–603. doi: 10.1007/s00425-008-0854-6
Liu H., Hu M., Wang Q., Cheng L., Zhang Z. (2018). Role of papain-like cysteine proteases in plant development. Front. Plant Sci 9. doi: 10.3389/fpls.2018.01717
Mondo J. M., Agre P. A., Asiedu R., Akoroda M. O., Asfaw A. (2021). Genome-wide association studies for sex determination and cross-compatibility in water yam (Dioscorea alata L.). Plants 10, 1–18. doi: 10.3390/plants10071412
Nadeem M. A., Nawaz M. A., Shahid M. Q., Doðan Y., Comertpay G., Yıldı z M., et al. (2018). DNA molecular markers in plant breeding: current status and recent advancements in genomic selection and genome editing. Biotechnol. Biotechnol. Equip 32, 261–285. doi: 10.1080/13102818.2017.1400401
Naeem M., Demirel U., Yousaf M. F., Caliskan S., Caliskan M. E. (2021). Overview on domestication, breeding, genetic gain and improvement of tuber quality traits of potato using fast forwarding technique (GWAS): A review. Plant Breed 140, 519–542. doi: 10.1111/pbr.12927
Nakano Y., Kobayashi Y. (2020). Genome-wide association studies of agronomic traits consisting of field-and molecular-based phenotypes. Rev. Agric. Sci 8, 28–45. doi: 10.7831/ras.8.0_28
Obidiegwu J. E., Lyons J. B., Chilaka C. A. (2020). The dioscorea genus (Yam) - an appraisal of nutritional and therapeutic potentials. Food, 1–45. doi: 10.3390/foods9091304
Okada Y., Monden Y., Nokihara K., Shirasawa K., Isobe S., Tahara M. (2019). Genome-wide association studies (GWAS) for yield and weevil resistance in sweet potato (Ipomoea batatas (L.) Lam). Plant Cell Rep 38, 1383–1392. doi: 10.1007/s00299-019-02445-7
Oladosu Y., Rafii M. Y., Samuel C., Fatai A., Magaji U., Kareem I., et al. (2019). Drought resistance in rice from conventional to molecular breeding: a review. Int. J. Mol. Sci 20, 3519. doi: 10.3390/ijms20143519
Perseguini J. M. K. C., Oblessuc P. R., Rosa J. R. B. F., Gomes K. A., Chiorato A. F., Carbonell S. A. M., et al. (2016). Genome-wide association studies of anthracnose and angular leaf spot resistance in common bean (Phaseolus vulgaris L.). PloS One 11, e0150506. doi: 10.1371/journal.pone.0150506
Phumichai C., Aiemnaka P., Nathaisong P., Hunsawattanakul S., Fungfoo P., Rojanaridpiched C., et al. (2022). Genome-wide association mapping and genomic prediction of yield-related traits and starch pasting properties in cassava. Theor. Appl. Genet, 1–27. doi: 10.1007/s00122-021-03956-2
Pinheiro J., Bates D., DebRoy S., Sarkar D. (2018). R Core Team. nlme: Linear and nonlinear mixed effects models. R Package Version, Vol. 3. 1–137.
Pitalounani W. E. N., Dourma M., Wala K., Woegan Y., Gbogbo A., Batawila K., et al. (2017). Agrodiversity, peasant management and importance of Dioscorea praehensilis Benth. in the Subhumid Zone of Togo. Afr. J. Food Agric. Nutr. Dev 17, 12455–12475. doi: 10.18697/ajfand.79.15930
Purcell S., Chang C. (2019). PLINK2. R package version 2.00. Available online at: https://www.cog-genomics.org/plink/2.0/ (Accessed 20th July 2023).
Purcell P. C., Smith A. M., Halford N. G. (1998). Antisense expression of a sucrose non-fermenting-1-related protein kinase sequence in potato results in decreased expression of sucrose synthase in tubers and loss of sucrose-inducibility of sucrose synthase transcripts in leaves. Plant J 14, 195–202. doi: 10.1046/j.1365-313X.1998.00108.x
Rabbi I. Y., Udoh L. I., Wolfe M., Parkes E. Y., Gedil M. A., Dixon A., et al. (2017). Genome-wide association mapping of correlated traits in cassava: dry matter and total carotenoid content. Plant Genome 10. doi: 10.3835/plantgenome2016.09.0094. plantgenome2016.09.0094.
R Development Core Team (2019). R: A language and environment for statistical computing. R foundation for statistical computing (Vienna, Austria).
Rosyara U. R., De Jong W. S., Douches D. S., Endelman J. B. (2016). Software for genome-wide association studies in autopolyploids and its application to potato. Plant Genome 9. plantgenome2015-08. doi: 10.3835/plantgenome2015.08.0073
Sharma S. K., MacKenzie K., McLean K., Dale F., Daniels S., Bryan G. J. (2018). Linkage disequilibrium and evaluation of genome-wide association mapping models in tetraploid potato. G3: Genes Genomes Genet 8, 3185–3202. doi: 10.1534/g3.118.200377
Shin J. H., Blay S., McNeney B., Graham J. (2006). LDheatmap: An R function for graphical display of pairwise linkage disequilibria between single nucleotide polymorphisms. J. Stat. Software 16, 1–10. doi: 10.18637/jss.v016.c03
Shuo L. (2019). Regulation of ribosomal proteins on viral infection. Cells 8, 508. doi: 10.3390/cells8050508
Song J. T., Lu. H., Greenberg J. T. (2004). Divergent roles in arabidopsis thaliana development and defense of two homologous genes, aberrant growth and death2 and AGD2-like defense response protein1, encoding novel aminotransferases. Plant Cell 16, 353–366. doi: 10.1105/tpc.019372
Stanley A. E., Menkir A., Ifie B., Paterne A. A., Unachukwu N. N., Meseka S., et al. (2021). Association analysis for resistance to Striga hermonthica in diverse tropical maize inbred lines. Sci. Rep 11. doi: 10.1038/s41598-021-03566-4
Sugihara Y., Darkwa K., Yaegashi H., Natsume S., Shimizu M., Abe A., et al. (2020). Genome analyses reveal the hybrid origin of the staple crop white Guinea yam (Dioscorea rotundata). Proc. Natl. Acad. Sci 50, 31987–31992. doi: 10.1073/pnas.2015830117
Sung M. H., Tanizawa K., Tanaka H., Kuramitsu S., Kagamiyama H., Hirotsu K., et al. (1991). Thermostable aspartate aminotransferase from a thermophilic Bacillus species. Gene cloning, sequence determination, and preliminary x-ray characterization. J. Biol. Chem 266, 2567–2572. doi: 10.1016/S0021-9258(18)52282-1
Thouvenel J. C., Dumont R. (1990). Perte de rendement de l'igname infectee par le virus de la mosaique en Côte d'Ivoire.
Wolfe M. D., Rabbi I. Y., Egesi C., Hamblin M., Kawuki R., Kulakow P., et al. (2016). Genome-wide association and prediction reveals genetic architecture of cassava mosaic disease resistance and prospects for rapid genetic improvement. Plant Genome 9. plantgenome2015-11. doi: 10.3835/plantgenome2015.11.0118
Yin L. (2019). R package “CMPlots”. Available online at: https://github.com/YinLiLin/R-CMplot (Accessed January 2023).
Yu J., Pressoir G., Briggs W. H., Vroh Bi I., Yamasaki M., Doebley J. F., et al. (2006). A Unified mixed-model method for association mapping that accounts for multiple levels of relatedness. Nat. Genet 38, 203–208. doi: 10.1038/ng1702
Zhang Y. W., Tamba C. L., Wen Y. J., Li P., Ren W. L., Ni Y. L., et al. (2020). mrMLM v4.0.2: an R platform for multi-locus genome-wide association studies. Genom. Proteom. Bioinform 18, 481–487. doi: 10.1016/j.gpb.2020.06.006
Keywords: bush yam, DArT SNP markers, GWAS, yield-related traits, YMV, GWASpoly
Citation: Adewumi AS, Adejumobi II, Opoku VA, Asare PA, Adu MO, Taah KJ, Stanley AE, Olatunde TE, Afutu E, Akaba S, Mushoriwa H and Agre PA (2024) Exploring quantitative trait nucleotides associated with response to yam mosaic virus severity and tuber yield traits in Dioscorea praehensilis Benth. germplasm via genome-wide association scanning. Front. Hortic. 3:1459476. doi: 10.3389/fhort.2024.1459476
Received: 04 July 2024; Accepted: 17 September 2024;
Published: 04 October 2024.
Edited by:
Sudip Kumar Dutta, ICAR Research Complex For NEH Region, Sikkim Centre, IndiaReviewed by:
Mao Suganami, Fukushima University, JapanGezahegn Girma, Purdue University, United States
Copyright © 2024 Adewumi, Adejumobi, Opoku, Asare, Adu, Taah, Stanley, Olatunde, Afutu, Akaba, Mushoriwa and Agre. This is an open-access article distributed under the terms of the Creative Commons Attribution License (CC BY). The use, distribution or reproduction in other forums is permitted, provided the original author(s) and the copyright owner(s) are credited and that the original publication in this journal is cited, in accordance with accepted academic practice. No use, distribution or reproduction is permitted which does not comply with these terms.
*Correspondence: Paterne A. Agre, cC5hZ3JlQGNnaWFyLm9yZw==; Adeyinka S. Adewumi, YWRld3VtaS5zYWJ1cmlAc3R1LnVjYy5lZHUuZ2g=