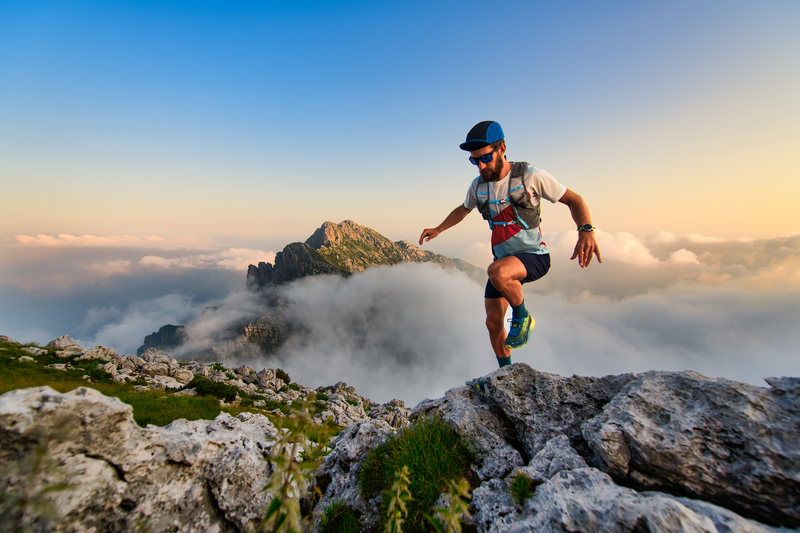
95% of researchers rate our articles as excellent or good
Learn more about the work of our research integrity team to safeguard the quality of each article we publish.
Find out more
ORIGINAL RESEARCH article
Front. Hortic. , 19 August 2024
Sec. Breeding and Genetics
Volume 3 - 2024 | https://doi.org/10.3389/fhort.2024.1448159
Introduction: Chile pepper (Capsicum annuum L.) mechanization is a promising alternative to traditional hand harvesting due to the costs associated with manual harvest, as well as the increasing unavailability of skilled manual chile harvesters. This study aimed to identify single nucleotide polymorphisms (SNPs) associated with mechanical harvestability (MH) and yield-related traits using multi-locus genome-wide association mapping approaches in a C. annuum association mapping population.
Methods: A C. annuum association mapping panel for mechanical harvest was manually direct seeded in an augmented block design in two locations. After filtration, imputation, and quality control 27,291 single nucleotide polymorphism (SNP) markers were used for association analyses. Six multi-locus GWAS models were implemented to identify marker trait association.
Results and Discussion: Multi-locus GWAS models identified 12 major SNP markers (R2 > 10) across nine chromosomes associated with plant architecture, easy destemming traits, and yield parameters. The presence of a major QTL in chromosome P2, dstem2.1, identified recently to be associated with destemming force, was confirmed. Mature green and mature red yield shared three SNP markers mapped on chromosome P3, P5, and P6 explaining 11.94% to 25.15% of the phenotypic variation. Candidate gene analysis for the significant loci identified 19 candidate genes regulating different phytohormone biosynthesis/signaling, metabolic processes, transcription, methylation, DNA repair/replication, and RNA splicing, with potential roles in controlling plant architecture and morphology. The diverse positions of the associated SNPs suggest the complex nature of these quantitative traits, involvement of multiple genetic factors, and novel significant marker-trait associations. Results from this study will be relevant for genetic improvement of mechanical harvestability traits in New Mexican chile pepper using molecular markerassisted breeding and selection.
Chile pepper (C. annuum L.) mechanization is an advantageous alternative to traditional manual harvesting that can reduce the production costs by at least 40% and can minimize dependency on manual labor (Funk et al., 2011). The efforts toward chile pepper mechanization were intensified following the termination of the Bracero Program in 1964 which was initiated in 1942 to deal with the farm labor shortages by recruiting temporary workers from Mexico (Walker and Funk, 2014). Ernest Riggs was the pioneer who made the first known attempt with mechanical harvesting of chile pepper in the US using an inclined, counter-rotating brush or flap design employed to propel through the rows of chile plants to dislodge the fruit (Marshall and Boese, 1998).
The efficiency of mechanical harvesting in chile peppers is influenced by plant architecture and diverse fruit morphology characterized by variations in length, width, and shape, encompassing spherical, round, conical, and elongated fruit types (Khokhar et al., 2022). Supporting production and harvesting approaches need to be implemented for different types of pepper with varying plant and fruit morphology. The Southwestern US is dominated by New Mexican (NM) pod type (red and green) chile peppers with at least 20 cultivars belonging to these types previously released by the New Mexico State University (NMSU) Chile Pepper Breeding Program (Coon et al., 2023).
Since the 1970s, mechanization efforts in the southwest US were directed toward the NM- pod-type red chile peppers which resulted in a nearly complete transition from manual to machine-driven harvest for this type (Walker and Funk, 2014). However, NM pod type green chile pepper mechanization is more challenging due to issues including fruit breakage and the need for destemming (removal of calyx and pedicel) for processed green chile. Destemming without breaking the fruit is one of the most important and challenging traits required for widespread adoption of NM pod type mechanical harvest. The stem and calyx of the fruit are considered foreign matter which can compromise the quality and reduce the value of the finished products (Wall et al., 2003). Due to the destemming requirement, jalapeño harvesting has largely been displaced from the US with almost 95% of the US processing demands currently being met by importing jalapeños from the countries where fruits are manually harvested and destemmed (Funk and Marshall, 2010). Considering the differences in fruit quality and morphology, as well as plant architecture, mechanization in green chile pepper requires a “systems approach”, where varieties are bred, and production practices are modified to accommodate mechanical harvesting (Funk et al., 2011).
The objective of this study was to implement genome-wide association studies (GWAS) to identify the genomic loci underpinning plant architecture and destemming traits in New Mexican chile pepper (Capsicum spp.). GWAS is one of the molecular breeding approaches used to detect genomic regions controlling complex quantitative traits using single nucleotide polymorphism (SNP) markers (Tibbs-Cortes et al., 2021; Zhu et al., 2008). This study employed multi-locus GWAS to determine quantitative trait loci (SNP) and candidate genes associated with complex quantitative traits such as plant architecture, destemming traits, and yield-related parameters in New Mexican chile pepper. Results will be relevant for genetic improvement of current pepper germplasm for mechanical harvestability traits using molecular marker-assisted breeding and selection.
A C. annuum association mapping panel for mechanical harvest (MH-CAMP; N= 90 genotypes) was manually direct seeded to a flood-irrigated, furrowed field in an augmented block design in two locations in May 2022: Leyendecker Plant Science Research Center (LPSRC), Las Cruces, NM, and at the NMSU Los Lunas Agricultural Science Center (LLASC), Los Lunas, NM, USA. Direct seeding at the LPSRC, Las Cruces, NM, resulted in poor germination, hence, this environment was excluded for data collection and analyses. After germination, thinning (i.e., removal of excess seedlings) was performed for the MH-CAMP at the LLASC, Los Lunas, NM, to maintain the plant-to-plant distance of ∼25 cm (10 inches) in 4.5 m (15 ft) plots with 1 m (3 ft) between plots to maintain at least 15 plants per plot and genotype. Cultural and management practices for growing chile peppers in Southern New Mexico were conducted according to Bosland and Walker (2014).
The replicated checks in each block were used as a reference to calculate the error and blocking effects (Federer et al., 2001). Un-replicated test genotypes were considered random effects, whereas the replicated checks were regarded as fixed effects (Federer, 1961) in the model. Augmented design is one of the most flexible and reliable experimental designs which can evaluate large diversity panels with an unequal number of genotypes in each block without compromising the critical differences among the tested treatments, thus leading to efficient utilization of time, resources, and accurate results (Federer et al., 2001; Burgueño et al., 2018). The MH-CAMP consisted of 10 blocks, with blocks 3, 7, and 10 comprising of 10 genotypes each. Blocks 1, 2, 4, 5, 6, and 8 had 9 genotypes each, and block 9 consisted of 7 genotypes. All blocks contained three checks, all having New Mexican pod types, namely, ‘NuMex Odyssey’ (Walker et al., 2021), ‘NuMex Iliad’, and ‘PDJ BLK7–4’ (Curry Chile & Seed Co., Pearce, AZ).
At maturity (after ~116 days (about 4 months) of manual direct seeding; September 2022), five plants from each block were selected at random to record plant architecture traits (Figure 1A), where the average of five plants for each trait was accounted for analysis. Plant morphology-related traits were measured in the field based on Joukhadar et al. (2018). Briefly, plant height (PHT) was the measurement from the bottom to the top of the plants. Plant width (PWDT) was calculated by measuring the widest point across the canopy of the plants. PHT and PWDT were measured in centimeters. Branches other than the main stem within 10 centimeters of the soil line were counted as the total number of basal branches (NBB). Height to first primary branch (HTFPB) was measured from the bottom of the plant to the first bifurcation of main stem in centimeters. Distance to first node (DTFN) was measured from the bottom of the main stem to the first node in centimeters.
Figure 1. (A) Plant architecture in chile pepper. PHT, plant height; PWDT, plant width; DTFPB, distance to first primary branch; DTFN, distance to first node; and BB, basal branches. (B) Scoring system to rate the destemmed red (DSRR) and green (DSRG) fruits. Scale: 1= perfect separation of stem and calyx from the fruit; 2= small breakage from the fruit; 3= medium breakage from the fruit; 4= large breakage from the fruit; and 5= complete breakage of the fruit.
A total of 5–8 red and green fruits from each plot collected after ~150–157 days (about 5 months; October 2022) of direct seeding were used to determine destemming rate and destemming force. The destemming force was measured using a Mark-10 M4–200 Digital Force Gauge (Copiague, NY, US). The gauge was equipped with a wire mesh Kellems grip (Hubbell Inc., Shelton, CT, USA) to get a better grip of the fruit for accurate measurements. The digital force gauge can simulate the force inserted by incline counter-rotating double open-helix harvester during harvesting (Funk and Walker, 2010; Hill et al., 2023). Five mature fruits (red and green) randomly selected from five plants per genotype were used to measure the destemming force. The destemming force (in Newtons, N) was the force needed to remove the stem and calyx from the mature red (DSFR) and mature green (DSFG) fruit samples. A scoring system was used to rate the destemmed red (DSRR) and green (DSRG) fruits, where 1= perfect separation of stem and calyx from the fruit; 2= small breakage from the fruit; 3= medium breakage from the fruit; 4= large breakage from the fruit; and 5= complete breakage of the fruit (Figure 1B). For yield parameters, mature green yield (GRN) and mature red yield (RED) were measured as the total weight of ten randomly selected fruits from five individual plants from each genotype for green and red samples, respectively.
Descriptive analysis of the traits was conducted using means, standard errors, and maximum/minimum values. The genotypic variances (GV), phenotypic variances (PV) and environmental variances (EV) were obtained from ANOVA table using an approach outlined by Federer (1961), whereas phenotypic coefficients of variation (PCV) and genotypic coefficients of variation (GCV) were calculated as described by Burton (1951). Coefficient of variation (CV) was estimated according to Johnson and Leone (1964). Similarly, broad-sense heritability (H2) was calculated following the methodology proposed by Lush (1940).
Seeds were grown in standard greenhouse conditions for cultivating chile peppers (Sharma et al., 2017) at the Fabián García Science Center greenhouse, Las Cruces, NM, USA (32°16′46.7″N, 106°46′24.7″W). After 30–45 days (about 1 and a half months) of sowing, a tissue sample was taken from a single plant per genotype in 1.2 mL Qiagen® collection microtubes. DNA extraction and quantification were performed using the Qiagen DNEasy® 96 plant extraction kits and Picogreen® (Thermofisher Scientific, MA, USA), respectively at the University of Minnesota Genomics Center (https://genomics.umn.edu/). Samples were normalized to 10 ng/µL and genotyping-by-sequencing (GBS) was performed using the Illumina NovaSeqTM 6000 sequencer (Illumina, CA, USA) with single-end 1×100 sequencing (Lozada et al., 2021). The raw FASTQ files were demultiplexed using Illumina ‘bcl2fastq’ software. Burrows-Wheeler Aligner was employed to align these FASTQ files to the ‘Zunla-1’ (C. annuum L.) reference genome (Qin et al., 2014). Freebayes Bayesian genetic variant identifier was used for variant calling across all samples (Garrison and Marth, 2012). Variant call format (VCF) files were processed using VCFtools, where variants with minor allele frequency <1% were removed. The VCF files were converted to the HapMap format and data imputation using the LD k-nearest neighbor genotype imputation function was performed using TASSEL v.5.2.77 software (Bradbury et al., 2007).
Linkage disequilibrium (LD) analysis was performed using TASSEL v.5.2.89 (Bradbury et al., 2007). A sliding window approach with a window size of 50 (0.05 kb) was implemented to calculate LD coefficients (r2) for pairwise intrachromosomal markers. To examine the relationship between LD and physical distance of genome-wide SNP markers, a non-linear regression model was fitted to the LD coefficients values (r2) against physical distance (in Mb) using a custom R script to identify the intersection point between the regression curve and critical value of r2 at which LD started to decay (Hill and Weir, 1988; Remington et al., 2001).
The genetic diversity parameters including minor allele frequency (MAF), expected heterozygosity (He), SNP density and polymorphism information content (PIC) were calculated using an R script (https://github.com/mohsinali1990/My_scripts/blob/462dbd14cb99397aceee97789de77861cd28a59f/TasselDiversityOut.R). STRUCTURE v.2.3.4 (Pritchard et al., 2000) was employed to investigate genetic stratification for the MH-CAMP, where an admixture model was implemented with burn-in of 1000 iterations, and 1000 Monte Carlo Markov Chain (MCMC) replicates and number of clusters (K) were set between 1 and 5. The POPHELPER 2.3.1 package (Francis, 2017) in R4.1.2 was used in identifying the optimal number of K as per Evanno method (Evanno et al., 2005) that best represent the MH-CAMP.
Muti-locus GWAS models were implemented to identify the marker-trait association for plant architecture, destemming traits, and yield parameters using the multi-locus random-SNP effect Mixed Linear Model (https://cran.r-project.org/web/packages/mrMLM/index.html) package in R (Zhang et al., 2020). Multi-locus GWAS models were preferred over single-locus models due to their efficient computational approach of using less stringent thresholds of significance for the initial screening of the markers followed by a likelihood test to confirm the true quantitative trait loci (Lozada et al., 2022; Vikas et al., 2022). A total of six GWAS models, viz., (1) mrMLM (Wang et al., 2016), (2) ISIS EMBLASSO (Tamba et al., 2017), (3) pLARmEB (Zhang et al., 2017), (4) FASTmrEMMA (Wen et al., 2018), (5) pKWmEB (Ren et al., 2018), and (6) FASTmrMLM (Tamba and Zhang, 2018) were implemented for association analyses. Phenotypic traits and BLUP values were calculated using a custom R script (https://github.com/mighster/BLUPs_Heritability/blob/master/BLUP_Tutorial.R). A kinship-principal component (K-PC) model was used to perform GWAS, where the first two principal components were included as covariate. The K-PC model minimizes the confounding effects of population structure that could lead to the identification of false positive marker-trait associations (Price et al., 2006). An LOD score of > 3.0 was set as a threshold for significant marker-trait association (Zhang et al., 2019).
EnsemblPlants (Bolser et al., 2016) was used to perform candidate gene analysis where genes within 0.5 Mb proximity of the SNP marker were considered as candidates. Annotation file for ‘Criollo de Morellos 334’ (CM-334; Genome assembly (GA): ASM512225v2) (C. annuum L.) (Kim et al., 2014) was downloaded from the EnsemblPlants website (https://plants.ensembl.org/index.html). Genes were annotated based on biological process and molecular function for major SNP associated with plant architecture, destemming rate, destemming force, and yield parameters.
Analysis of variance (ANOVA) revealed significant statistical differences (P ≤ 0.01) among the means of plant architecture and destemming traits, and yield parameters (Table 1). The coefficient of variation (CV) ranged between 11.04 (DSFG) and 64.95 (NBB). Phenotypic coefficient of variation (PCV) was higher compared to genotypic coefficient of variation (GCV). Low to high broad-sense heritability (H2) values ranging between 0.06 (DSRG) and 0.87 (DSFG) were reported (Table 2). A moderate to weak Pearson correlation was observed between plant architecture traits, whereas destemming rate and destemming force were negatively correlated with plant architecture. PHT, PWDT, and NBB were negatively correlated with DSFG, DSFR, DSRR, DSRG, GRN, and RED (Figure 2). The DSFR and DSFG were positively correlated with GRN and RED. The principal component (PC) biplot further supported the weak phenotypic correlation between the plant architecture traits (Figure 3). Destemming traits and yield parameters formed separate clusters indicating a strong phenotypic correlation for respective parameters. The first two principal components, PC1 and PC2, were associated with 33.1% and 18%, of variation, respectively. BLUP values represented the estimated random genetic effect of each genotype on plant architecture, fruit destemming and yield parameters (Supplementary Table S1). Traits such as PHT, PWDT, and DTFN showed moderate variability, whereas DSFR and DSFG displayed higher values for variances. The average PHT BLUP was 43.31; PWDT had a mean BLUP value of 32.45, whereas DTFN had an average BLUP of 3.41. Traits with lower standard deviations (NBB, GRN, RED) were relatively stable across genotypes, whereas DSFR and DSFG showed higher values for standard deviation.
Table 1. Analysis of variance for plant architecture, destemming traits, and yield parameters in the Capsicum population.
Table 2. Summary statistics and genetic variability components of plant architecture, destemming traits, and yield parameters.
Figure 2. Pearson correlation coefficients between plant architecture (PHT, PWDT, NBB, HTFPB, DTFN), destemming force (DSFG, DSFR), destemming rate (DSRG, DSRR), and yield parameters (GRN, RED). PHT, plant height; PWDT, plant width; NBB, number of basal branches; HTFPB, height to first primary branch; DTFN, distance to first node; DSFG, destemming force for green; DSFR, destemming force for red; DSRG, destemming rate for green; DSRR, destemming rate for red; GRN, mature green yield; RED, mature red yield. *Significant at the 0.05 probability level. **Significant at the 0.01 probability level. ***Significant at the 0.001 probability level. ns, Non-significant.
Figure 3. Principal component analysis (PCA) biplot for plant architecture (PHT, PWDT, NBB, HTFPB, DTFN), destemming force (DSFG, DSFR), destemming rate (DSRG, DSRR) and yield parameters (GRN, RED). Length and angle of the vectors represent the correlation and corresponding principal component (PC), contributing more to its variation. cos2 refers to the squared cosine of the angle between the original variables and the PCs. PHT, plant height; PWDT, plant width; NBB, number of basal branches; HTFPB, height to first primary branch; DTFN, distance to first node; DSFG, destemming force for green; DSFR, destemming force for red; DSRG, destemming rate for green; DSRR, destemming rate for red; GRN, mature green yield; RED, mature red yield.
Single nucleotide polymorphism (SNP) markers (N= 404,188) were obtained using genotyping-by-sequencing (GBS). After filtration, imputation, and quality control in TASSEL 5.2.89 software (Bradbury et al., 2007), 27, 291 SNP markers across 12 chromosomes were retained for further analyses. Chromosome P3 had the highest number of SNP (3,554) followed by P1 (2,927), P2 (2,739), and P6 (2,438) whereas lowest number of SNP was reported for chromosome P5 (1,700), P7 (1,702), and P11 (1,771). The marker density across 12 chromosomes ranged between 7.66 (P7) and 15.51 Mb (P8). The whole genome SNP density was 10.31 markers per Mb (Supplementary Table S2). Adenine (A) and Thymine (T) were the most common nucleotides, each representing 24% of occurrence across the SNP sites, whereas Guanine (G) and Cytosine (C) each represented 23% of the observed nucleotides (Supplementary Table S3). Transition substitution (52%) was higher than the transversion substitution (48%). The ‘C/T’ and ‘G/A’ type transition substitution were predominant, each accounted for 14% of the substitutions, whereas, ‘A/T’, ‘T/A’, and ‘G/T’ transversions, had an equal frequency of 7%.
Genetic diversity parameters such as MAF, He and PIC were estimated for the MH-CAMP (Supplementary Table S4). The MAF, He, and PIC were higher in the pericentromeric regions of all 12 chromosomes (Figure 4). The MAF values ranged between 0.20 and 0.24 with an average of 0.22. The observed range of He was between 0.29 to 0.33, with an average of 0.31. The PIC had a mean of 0.25. We inferred two subpopulations for MH-CAMP according to the Evanno method, where maximum value was reported for ΔK=2 (Figures 5A, B). Cluster 1 consisted of 64 genotypes, whereas 24 genotypes were grouped in cluster 2 (Supplementary Table S5).
Figure 4. The Circos plot demonstrates SNP density, minor allele frequency, expected heterozygosity, and polymorphic information content of the 27,291 SNP markers used for multi-locus GWAS. The numbers outside the Circos plot represent the chromosome number for C. annuum L. (chromosomes 1–12). Please refer to Supplementary Table S4 for the values of the different diversity parameters.
Figure 5. Population structure and linkage disequilibrium (LD) of the MH-CAMP. (A) Identification of the optimal number of clusters using the Evanno method revealed K = 2 as the highest value for ΔK. (B) Subpopulations inferred by K-means structure analysis. (C) The critical value for LD (r2 = 0.20) was represented by the red dashed line, whereas the blue solid line is the non-linear regression curve. The intersection between the critical value and the curve for non-linear regression is the LD decay distance for the population (~0.12 Mb).
Analysis of linkage disequilibrium revealed 1,349,251 intrachromosomal pairs for the MH-CAMP, with a mean coefficient of LD (r2) of 0.15 (Supplementary Table S2). Among these intrachromosomal pairs, 452,533 (33%) were in significant (P < 0.05) LD, whereas 62,751 (5%) pairs were in complete LD (r2 = 1.0). The r2 for intrachromosomal pairs in significant LD across 12 chromosomes ranged between 0.33 Mb (P12) and 0.42 Mb (P1). Chromosome P3 had the maximum number of significant intrachromosomal pairs (P < 0.05) in LD (55,080), followed by P1 (51,722), P2 (50,108), and P9 (42,973). Average distance between the pairs in significant LD (~2.64 Mb) was lower than the pairs in complete LD (~4.19 Mb). Chromosome P12 (10.59 Mb) has the highest average markers distance for the pairs in complete LD, followed by P4 (5.46 Mb), P7 (4.34 Mb), P10 (4.16 Mb), and P11 (4.16 Mb). The LD for MH-CAMP reported a decline at a physical distance of ~0.12 Mb as indicated by the critical value of r2 = 0.20 across the genome (Figure 5C).
Multi-locus GWAS models identified 87 SNP markers associated with plant architecture, destemming traits, and yield parameters (Supplementary Table S6). Among these, 25 SNP markers were significant across at least two multi-locus GWAS models. Across the different traits, NBB had a maximum number of SNP (14), whereas PHT and HTFPB had equal number of SNP (8), followed by PWDT (6) and DTFN (4). For the destemming traits, DSFG (13) had the maximum number of significant SNP followed by DSRG (7), DSRR (6), and DSFR (1). A total of 11 SNP markers were associated with GRN, whereas nine were identified for RED yield. No multi-trait SNP were found for plant architecture and destemming traits. However, mature GRN and RED yield shared three common SNP (SCM002814.1_260456773, SCM002816.1_1749348, and SCM002817.1_210746241) mapped on chromosomes P3, P5, and P6, respectively (Table 3; Figure 6). SCM002817.1_210746241 (P6) explained 25.15% of phenotypic variation for both GRN and RED (Table 3; Figure 6). For PHT, SCM002822.1_1169164 on chromosome P11 explained 12.69% of phenotypic variation, whereas SCM002820.1_20749917 on chromosome P9 explained 10.50% of variation for PWDT. SCM002814.1_248773989 on chromosome P3 was linked with NBB, explaining 10.09% of phenotypic variation. SCM002816.1_186377753 (P5) and SCM002818.1_219380408 (P7) each explained approximately 13% of phenotypic variation and were associated with HTFPB and DTFN, respectively. For destemming rate, SCM002822.1_177952684 and SCM002821.1_2290233 were linked with DSRG and DSRR with R2 values of 19.27% and 24.34% at 177.95 Mb and 2.29 Mb on chromosomes P11 and P10, respectively (Table 3; Figure 6). For destemming force, two SNP markers (SCM002813.1_140023085 and SCM002814.1_261284271) were mapped on chromosome P2 and P3, associated with DSFR and DSFG, explaining over 10% of the phenotypic variation for the trait, respectively.
Table 3. Major marker-trait associations (R2 > 10%) identified for plant architecture, destemming, and yield-related traits in the MH-CAMP using multi-locus GWAS models.
Figure 6. Manhattan plots showing genome-wide SNP markers associated with plant architecture, destemming traits, and yield parameters using multi-locus models in the MH-CAMP. All dots above the dotted horizontal line were significant marker-trait associations (LOD score > 3.0). The SNP significant across at least two multi-locus GWAS models were represented by pink dots.
A total of 242 genes across seven chromosomes (P2, P3, P5, P6, P9, P10, P11) were found to be associated with major SNP for plant architecture, destemming rate, destemming force, and yield parameters (Supplementary Table S7). Out of 242, ~34% of the candidate genes were accounted for GRN and RED (n= 83). DSFG (n= 47) and DSFR (n= 33) accounted for ~33% of the candidate genes, whereas ~20% of the genes were identified for DSRG (n= 5) and DSRR (n= 44). PHT, PWDT and NBB combined had the lowest number (n= 29) of candidate genes. The candidate genes were related with diverse biological processes and molecular functions. A total of 19 candidate genes were found to be associated with phytohormone biosynthesis/signaling, metabolic processes, transcription, methylation, DNA repair/replication, RNA splicing and defense response among others (Supplementary Table S7).
Breeding and selection for plant architecture and destemming traits can improve the machine harvest potential of chile pepper. Selection of taller plants with fewer basal branches, greater distance to the first primary branch, and easy destemming are the fundamentals of developing chile pepper cultivars that are amenable to mechanization. In major cereals, the modification of plant architecture had significant impact in wheat (Triticum aestivum L.), where yield was improved after selection for shorter and sturdier stem conferred by the reduced height genes (Peng et al., 1999; Hedden, 2003), resulting in the ‘Green Revolution’ in the 1960’s (Evenson and Gollin, 2003). Likewise, efforts were successful in redesigning tomato (Solanum lycopersicum L) for modified plant architecture amenable for mechanized production (Webb and Bruce, 1968). Chile pepper mechanization is more complex and requires a “systems approach”, integrating plant breeding, production practices, harvester and processing plant machinery design (Funk et al., 2011) to select for taller genotypes with strong stems, and easy destemming. This study aimed to dissect the genetic architecture of machine harvestability traits using a C. annuum association mapping population evaluated in New Mexico, USA. The genetic diversity of the Capsicum panel was explored, and significant marker-trait associations were mapped using multi-locus GWAS approaches. Finally, candidate genes with roles related to controlling plant morphology and architecture were identified for the significant SNP markers.
Phenotypic diversity was detected among a panel of 90 genotypes for machine harvestability traits, in agreement with previous observations (Usman et al., 2014; Rosmaina et al., 2016; Saisupriya et al., 2022) on chile pepper germplasm. We reported a higher difference between PCV and GCV with moderate heritability, indicating a fair proportion of genetic variance for plant architecture, destemming, and yield traits. Our findings indicate that variation among genotypes were also influenced by genotype × environmental interactions, in contrast with other studies (Ahmed et al., 2022; Bhutia et al., 2015; Vyas et al., 2021), where the genetic variance was documented for plant height, quality and yield parameters in chile pepper. These discrepancies in the estimation of genetic effects controlling various quantitative traits might be due to the differences in the genetic makeup of the genotypes under study, environmental factors affecting the phenotypic expression of the traits, and variation in the statistical methods used for data analysis and data acquisition (Bhutia et al., 2015). We further evaluated the genetic diversity parameters such as He and PIC to assess the genetic variation in the MH-CAMP. The genetic variability expressed by He and PIC is instrumental for understanding the molecular characterization of the population occurred due to selection forces on the chile pepper germplasm (Liu et al., 2019). Akyavuz et al. (2018) reported moderate genetic diversity based on the He and PIC for Turkish pepper (C. annuum L.) germplasm evaluated using SNP markers. Our findings agreed with the observations made by Naegele et al. (2016) where genetic differentiation was assessed for fruit morphology in chile pepper diversity panel using He and PIC. The MH-CAMP includes breeding lines, cultivars, landraces, and heirlooms contributing toward a rich genetic landscape of the panel to ensure robust association studies leading into valuable insights about plant architecture, destemming, and yield parameters.
GWAS is one of the recent advances in the field of molecular breeding implemented in different crops (Malik et al., 2021; Yoosefzadeh-Najafabadi et al., 2021; Khan et al., 2022; Lozada et al., 2022) to identify genetic variations associated with complex traits. Large diversity panels can improve the statistical power of the GWAS results by providing better mapping resolution because of higher recombination events occurring within the population (Brachi et al., 2011). A number of previous studies in different crops have demonstrated the potential of performing association mapping even under relatively small population sizes: C. annuum L. (Nimmakayala et al., 2016), wheat (Triticum aestivum L.) (Ahmed et al., 2022; Neumann et al., 2011), cucumber (Cucumis sativus L.) (Kumar et al., 2022), and sequoia (Sequoia sempervirens L. and Sequoiadendron giganteum L.) (De La Torre et al., 2022), where the association mapping population size ranged between 78 and 96 genotypes. The current study employed a panel of 90 lines to find marker-trait associations (MTAs) for plant architecture, destemming traits, and yield parameters. A small population size might lead to the detection of significant marker-trait associations, if such are, under the influence of a single gene or small set of genes with large effects (Otyama et al., 2019). Only a small number of major effect SNP markers for the evaluated traits were identified using the Capsicum population (Table 3), a potential consequence of the population size used for genome-wide mapping (Nimmakayala et al., 2016). In the current study, utilizing the MH-CAMP resulted in the identification of previously mapped marker-trait association in chromosome 2 (dstem2.1; Hill et al., 2023) for easy destemming, which demonstrated the robustness of using multi-locus models for association mapping of a highly heritable trait even under a relatively small population size.
Destemming force and destemming rate are one of the most important traits for chile pepper mechanization. Chromosomes P11, P12 and P2, P3 hosted SNP markers for destemming rate and destemming force, respectively, consistent with the identification of SNP on similar chromosomal regions (Hill et al., 2023). Multi-locus GWAS identified the association of a SNP marker, SCM002813.1_140023085 with destemming force, thus confirming the presence of a major QTL, dstem2.1, recently reported by Hill et al. (2023) on the long arm of chromosome P2. The location of the other identified SNP for destemming force and destemming rate were ~150 to 250 Mb from the locus identified by Hill et al. (2023), indicating that potential novel loci for Capsicum destemming were identified. We have observed the similar trend for yield parameters, where SNP markers for GRN and RED were detected on chromosomes P5 and P6, which agreed with observations made on New Mexican diversity panel of chile pepper (Lozada et al., 2022).
Multiple SNP markers associated with PHT at chromosome P11 were previously detected and found to be related with fruit length and plant width (Yarnes et al., 2013; Lozada et al., 2022). We identified highly significant SNP for PHT at chromosome P11 which was found ~59 Mb away from the reported coordinate; however, the SNP detected for PWDT in our study was found on chromosome P9, possibly a consequence of ascertainment bias arising from smaller population size (Lachance and Tishkoff, 2013). Our findings for NBB agreed with the observations of Solomon et al. (2019), where SNP markers were reported on chromosome P3 (between 225–227 Mb) for this trait.
The associated SNPs implicated the complex nature of these quantitative traits suggesting involvement of multiple genetic factors in phenotypic trait variation, making it difficult to select for these complex traits. For the improvement of complex traits under the influence of unique SNP markers, more advanced tools such as genomic selection are required. Genomic selection predicts the breeding values of the complex trait using whole-genome marker profiles without relying on the effects of individual SNP giving comprehensive overview of the traits, whereas multi-trait GWAS accounts for the correlation and pleiotropic effects while analyzing multiple traits simultaneously (Ibrahim et al., 2020). These tools can significantly contribute to optimizing the breeding methodologies for the traits associated with unique SNPs with large effects.
A total of 19 candidate genes regulating phytohormone biosynthesis/signaling, metabolic processes, transcription, methylation, DNA repair/replication, RNA splicing associated with major SNP were identified for plant architecture (PHT, PWDTH, HTFPB, DTFN and NBB), destemming (DSFR, DSFG, DSRR and DSRG), and yield parameters (GRN and RED). Of the 19 candidate genes, one was associated with the auxin transport, two were affiliated with cytokinin-activated signaling pathway, seven were linked with metabolic processes, five were tied with transcription, two with DNA repair/replication, and one each was associated with RNA splicing and methylation (Table 4). Auxin and cytokinin are two important phytohormones and their regulation can play a crucial role in controlling overall plant architecture. Cytokinin is associated with the development and growth of overall plant shape (Kyozuka, 2007; Gao, 2020). Shoot meristem activity which is regulated by cytokinin signaling affects plant height and branching patterns. On later growth stages, cytokinin signaling also influences the lateral bud outgrowth that makes plants bushy with more basal branches, therefore regulating the plant width and branching density (Werner et al., 2003; Azizi et al., 2015). Different mutant genes for cytokinin showed abnormal branching pattern and poor reproductive growth in rice (Oryza sativa L.) (Kurakawa et al., 2007), pear (Pisum sativum L.) (Ward and Leyser, 2004), and Chrysanthemum spp (Dierck et al., 2016), indicating the influence of cytokinin signaling on vegetative and reproductive growth in different crop species.
Table 4. Candidate genes with known biological functions associated with the major SNP markers identified for plant architecture, destemming, and yield parameters.
We also identified candidate genes for auxin transport. Auxin is an important phytohormone that can regulate plant growth and development including cell elongation, cell division and differentiation (Teale et al., 2006). The movement of auxin from dormant buds to other parts of the plant can promote their outgrowth. Auxins stimulate strigolactone biosynthesis for the overexpression of TB1/BRC1 which act in axillary buds, inhibiting the branching in pea plants (Braun et al., 2012). Accumulation of auxin in axillary bud regulated by the expression of different proteins was found to alter the shoot structure in cucumber (Shen et al., 2019) and sweet crabapple (Malus coronaria L.) (Zhao et al., 2020) suggesting the role of auxin in regulating plant shoot architecture. Plants produce new organs throughout their life cycle using stem cells that are regulated by thousands of genes controlled by key transcriptional regulators which control developmental processes (Kaufmann et al., 2010).
The upregulation and downregulation of genes are influenced by transcription factors indicating their important role in regulating important hormones affecting the expression of plant architecture and morphology-related traits. Down regulation of novel transcription factor, SlGT-26 confer dwarfing and salt tolerance (Li et al., 2023), whereas overexpression of the MADS-box transcription factor, SlMBP22 influence auxin and gibberellin signaling in tomato (Li et al., 2020). Overexpression of PagKNAT2/6b transcription factor altered the plant architecture in Poplar 84K (Populus alba × P. glandulosa) (Song et al., 2021).
Candidate genes for epigenetic factors such as methylation were also identified. Methylation can contribute toward the growth and development of plants by regulating gene expression, integrity, and mobility of the genome (Chachar et al., 2022). Although the role of epigenetic factors in regulating the expression of the genes associated with plant architecture and destemming is not fully established, denser methylation profile has been reported for chile peppers compared to potato (Solanum tuberosum L.) and tomato (Ramchiary and Kole, 2019). Our results demonstrated that epigenetics could play a role in controlling plant architecture and morphology in Capsicum.
Mechanical harvesting offers a cost-effective solution for boosting chile pepper production. Our study identified 87 SNP markers across 12 chromosomes linked to plant architecture, destemming, and yield parameters. Twelve major markers were detected, with three common SNP markers shared among yield parameters. A SNP marker for DSFG was confirmed at chromosome 2. A moderate correlation was observed between plant architecture traits, whereas destemming rate and destemming force were negatively correlated with plant architecture. Candidate gene analysis revealed 19 potential genes regulating different phytohormone biosynthesis/signaling, metabolic processes, transcription, methylation, DNA repair/replication, and RNA splicing. The findings of this study will contribute toward the development of molecular markers for marker-assisted selection and genomic prediction of plant architecture and destemming traits in New Mexican chile pepper.
The original contributions presented in the study are publicly available. This data can be found here: FigShare, https://doi.org/10.6084/m9.figshare.26015656 and https://doi.org/10.6084/m9.figshare.26015674.
EK: Investigation, Data curation, Formal analysis, Methodology, Visualization, Writing – original draft, Writing – review & editing. DL: Conceptualization, Funding acquisition, Investigation, Project administration, Supervision, Writing – original draft, Writing – review & editing. MA: Formal analysis, Software, Visualization, Writing – review & editing. MK: Data curation, Writing – review & editing. SN: Data curation, Writing – review & editing. SW: Funding acquisition, Resources, Writing – review & editing.
The author(s) declare financial support was received for the research, authorship, and/or publication of this article. This work was supported by the New Mexico Chile Association Competitive Grants Program (2021–2023) for DL and SW.
The Authors are grateful for the assistance of Danise Coon (Plant and Environmental Sciences, NMSU), and Mark Marsalis, Dennis Price, and Charles Havlik (NMSU Los Lunas Agricultural Science Center, Los Lunas, New Mexico), in direct seeding and collecting phenotypic data from the MH-CAMP.
The authors declare that the research was conducted in the absence of any commercial or financial relationships that could be construed as a potential conflict of interest.
All claims expressed in this article are solely those of the authors and do not necessarily represent those of their affiliated organizations, or those of the publisher, the editors and the reviewers. Any product that may be evaluated in this article, or claim that may be made by its manufacturer, is not guaranteed or endorsed by the publisher.
The Supplementary Material for this article can be found online at: https://www.frontiersin.org/articles/10.3389/fhort.2024.1448159/full#supplementary-material
Ahmed Z., Khalid M., Ghafoor A., Shah M. K. N., Raja G. K., Rana R. M., et al. (2022). SNP-Based Genome-Wide Association Mapping of Pollen Viability Under Heat Stress in Tropical Zea mays L. Inbred Lines. Frontiers in Genetics, 13. doi: 10.3389/fgene.2022.819849
Akyavuz R., Taskin B., Koçak M., Yildiz M. (2018). Exploring the genetic variations and population structure of Turkish pepper (Capsicum annuum L.) genotypes based on peroxidase gene markers. 3 Biotech. 8, 1–9. doi: 10.1007/s13205-018-1380-2
Azizi P., Rafii M. Y., Maziah M., Abdullah S. N. A., Hanafi M. M., Latif M. A., et al. (2015). Understanding the shoot apical meristem regulation: a study of the phytohormones, auxin and cytokinin, in rice. Mech. Dev. 135, 1–15. doi: 10.1016/j.mod.2014.11.001
Bhutia N. D., Seth T., Shende V. D., Dutta S., Chattopadhyay A. (2015). Estimation of heterosis, dominance effect and genetic control of fresh fruit yield, quality and leaf curl disease severity traits of chilli pepper (Capsicum annuum L.). Scientia Hortic. 182, 47–55. doi: 10.1016/j.scienta.2014.11.017
Bolser D., Staines D. M., Pritchard E., Kersey P. (2016). Ensembl plants: integrating tools for visualizing, mining, and analyzing plant genomics data. In Plant bioinformatics (Springer), pp. 115–140. doi: 10.1007/978-1-4939-3167-5_6
Bosland P. W., Walker S. J. (2014). Growing Chiles in new Mexico. New Mexico State Univ. Coop. Ext. Serv. Guide H-230. Available online at: https://pubs.nmsu.edu/_h/H230.pdf.
Brachi B., Morris G. P., Borevitz J. O. (2011). Genome-wide association studies in plants: the missing heritability is in the field. Genome Biol. 12, 1–8. doi: 10.1186/gb-2011-12-10-232
Bradbury P. J., Zhang Z., Kroon D. E., Casstevens T. M., Ramdoss Y., Buckler E. S. (2007). TASSEL: software for association mapping of complex traits in diverse samples. Bioinformatics 23, 2633–2635. doi: 10.1093/bioinformatics/btm308
Braun N., de Saint Germain A., Pillot J.-P., Boutet-Mercey S., Dalmais M., Antoniadi I., et al. (2012). The pea TCP transcription factor PsBRC1 acts downstream of strigolactones to control shoot branching. Plant Physiol. 158, 225–238. doi: 10.1104/pp.111.182725
Burgueño J., Crossa J., Rodríguez F., Yeater K. M. (2018). Augmented designs-experimental designs in which all treatments are not replicated. Appl. Stat Agricult. Biol. Environ. Sci., 345–369. doi: 10.2134/appliedstatistics.2016.0005.c13
Burton G. W. (1951). Quantitative inheritance in pearl millet (Pennisetum glaucum) 1. Agron. J. 43, 409–417. doi: 10.2134/agronj1951.00021962004300090001x
Chachar S., Chachar M., Riaz A., Shaikh A. A., Li X., Li X., et al. (2022). Epigenetic modification for horticultural plant improvement comes of age. Scientia Hortic. 292, 110633. doi: 10.1016/j.scienta.2021.110633
Coon D., Walker S., Lozada D., Guzman I., Bosland P. W. (2023). The Chile Cultivars of New Mexico State University. New Mexico State Univ. Coop. Ext. Serv. Circular CR-706 1–20. Available online at: https://pubs.nmsu.edu/_circulars/CR706.pdf.
De La Torre A. R., Sekhwal M. K., Puiu D., Salzberg S. L., Scott A. D., Allen B., et al. (2022). Genome‐wide association identifies candidate genes for drought tolerance in coast redwood and giant sequoia. The Plant Journal 109(1), 7–22. doi: 10.1111/tpj.15592
Dierck R., De Keyser E., De Riek J., Dhooghe E., Van Huylenbroeck J., Prinsen E., et al. (2016). Change in auxin and cytokinin levels coincides with altered expression of branching genes during axillary bud outgrowth in Chrysanthemum. PloS One 11, e0161732. doi: 10.1371/journal.pone.0161732
Evanno G., Regnaut S., Goudet J. (2005). Detecting the number of clusters of individuals using the software STRUCTURE: a simulation study. Molecular Ecology 14 (8), 2611–2620. doi: 10.1111/j.1365-294X.2005.02553.x
Evenson R. E., Gollin D. (2003). Assessing the impact of the green revolution 1960 to 2000. Science 300, 758–762. doi: 10.1126/science.1078710
Federer W. T. (1961). Augmented designs with one-way elimination of heterogeneity. Biometrics 17, 447–473. doi: 10.2307/2527837
Federer W. T., Reynolds M., Crossa J. (2001). Combining results from augmented designs over sites. Agron. J. 93, 389–395. doi: 10.2134/agronj2001.932389x
Francis R. M. (2017). pophelper: an R package and web app to analyse and visualize population structure. Mol. Ecol. Resour. 17, 27–32. doi: 10.1111/1755-0998.12509
Funk P. A., Walker S. J. (2010). Evaluation of five green Chile cultivars utilizing five different harvest mechanisms. Appl. Eng. Agric. 26, 955–964. doi: 10.13031/2013.35906
Funk P. A., Walker S. J., Herbon R. P. (2011). A systems approach to Chile harvest mechanization. Intl. J. Veg. Sci. 17, 296–309. doi: 10.1080/19315260.2010.549167
Funk P. A., Marshall D. E. (2010). Pepper Harvester development. Proceedings of the American Society of Agricultural and Biological Engineers (ASABE). Annual International Meeting. Pittsburgh, PA.
Gao S. (2020). “Function and mechanism study of plant cytokinins,” in Proceedings of the 2020 10th International Conference on Biomedical Engineering and Technology, Tokyo Japan, September 15 - 18, 2020, Association for Computing Machinery New York NY United States. 80–84. doi: 10.1145/3397391.3397395
Garrison E., Marth G. (2012). Haplotype-based variant detection from short-read sequencing. ArXiv Preprint ArXiv:1207.3907. doi: 10.48550/arXiv.1207.3907
Hedden P. (2003). The genes of the green revolution. Trends Genet. 19, 5–9. doi: 10.1016/S0168-9525(02)00009-4
Hill T. A., Cassibba V., Joukhadar I., Tonnessen B., Havlik C., Ortega F., et al. (2023). Genetics of Destemming in Pepper: A step towards mechanical harvesting. Front. Genet. 14. doi: 10.3389/fgene.2023.1114832
Hill W. G., Weir B. S. (1988). Variances and covariances of squared linkage disequilibria in finite populations. Theor. Popul. Biol. 33, 54–78. doi: 10.1016/0040-5809(88)90004-4
Ibrahim A. K., Zhang L., Niyitanga S., Afzal M. Z., Xu Y., Zhang L., et al. (2020). Principles and approaches of association mapping in plant breeding. Trop. Plant Biol. 13, 212–224. doi: 10.1016/0040-5809(88)90004-4
Johnson N. L., Leone F. C. (1964). Statistics and experimental design in engineering and the physical sciences (New York: Wiley).
Joukhadar I. S., Walker S. J., Funk P. A. (2018). Comparative mechanical harvest efficiency of six New Mexico pod–type green Chile pepper cultivars. HortTechnol. Hortte 28, 310–318. doi: 10.21273/HORTTECH03999-18
Kaufmann K., Pajoro A., Angenent G. C. (2010). Regulation of transcription in plants: mechanisms controlling developmental switches. Nat. Rev. Genet. 11, 830–842. doi: 10.1038/nrg2885
Khan M. I., Kainat Z., Maqbool S., Mehwish A., Ahmad S., Suleman H. M., et al. (2022). Genome-wide association for heat tolerance at seedling stage in historical spring wheat cultivars. Front. Plant Sci. 13. doi: 10.3389/fpls.2022.972481
Khokhar E. S., Lozada D. N., Nankar A. N., Hernandez S., Coon D., Kaur N., et al. (2022). High-throughput Characterization of Fruit Phenotypic Diversity among New Mexican Chile Pepper (Capsicum spp.) Using the Tomato Analyzer Software. HortScience 57, 1507–1517. doi: 10.21273/HORTSCI16815-22
Kim S., Park M., Yeom S.-I., Kim Y.-M., Lee J. M., Lee H.-A., et al. (2014). Genome sequence of the hot pepper provides insights into the evolution of pungency in. Capsicum species. Nat. Genet. 46, 270–278. doi: 10.1038/ng.2877
Kumar R., Das Munshi A., Behera T. K., Jat G. S., Choudhary H., Talukdar A., et al. (2022). Association mapping, trait variation, interaction and population structure analysis in cucumber (Cucumis sativus L.). Genetic Resources and Crop Evolution 69(5), 1901–1917. doi: 10.21203/rs.3.rs-982298/v1
Kurakawa T., Ueda N., Maekawa M., Kobayashi K., Kojima M., Nagato Y., et al. (2007). Direct control of shoot meristem activity by a cytokinin-activating enzyme. Nature 445, 652–655. doi: 10.1038/nature05504
Kyozuka J. (2007). Control of shoot and root meristem function by cytokinin. Curr. Opin. Plant Biol. 10, 442–446. doi: 10.1016/j.pbi.2007.08.010
Lachance J., Tishkoff S. A. (2013). SNP ascertainment bias in population genetic analyses: why it is important, and how to correct it. Bioessays 35(9), 780–786. doi: 10.1002/bies.201300014
Li F., Chen G., Xie Q., Zhou S., Hu Z. (2023). Down-regulation of SlGT-26 gene confers dwarf plants and enhances drought and salt stress resistance in tomato. Plant Physiol. Biochem. 203, 108053. doi: 10.1016/j.plaphy.2023.108053
Li F., Chen X., Zhou S., Xie Q., Wang Y., Xiang X., et al. (2020). Overexpression of SlMBP22 in tomato affects plant growth and enhances tolerance to drought stress. Plant Sci. 301, 110672. doi: 10.1016/j.plantsci.2020.110672
Liu J., Rasheed A., He Z., Imtiaz M., Arif A., Mahmood T., et al. (2019). Genome-wide variation patterns between landraces and cultivars uncover divergent selection during modern wheat breeding. Theor. Appl. Genet. 132, 2509–2523. doi: 10.1007/s00122-019-03367-4
Lozada D. N., Barchenger D. W., Coon D., Bhatta M., Bosland P. W. (2022). Multi-locus association mapping uncovers the genetic basis of yield and agronomic traits in Chile pepper (Capsicum spp.). Crop Breed Genet. Genom. 4, 2–28. doi: 10.20900/cbgg20220002
Lozada D. N., Bhatta M., Coon D., Bosland P. W. (2021). Single nucleotide polymorphisms reveal genetic diversity in New Mexican Chile peppers (Capsicum spp.). BMC Genomics 22, 1–12. doi: 10.1186/s12864-021-07662-7
Lush J. L. (1940). Intra-sire correlations or regressions of offspring on dam as a method of estimating heritability of characteristics. Proc. Am. Soc. Anim. Nutr., 293–301. doi: 10.2527/jas1940.19401293x
Malik P., Kumar J., Singh S., Sharma S., Meher P. K., Sharma M. K., et al. (2021). Single-trait, multi-locus and multi-trait GWAS using four different models for yield traits in bread wheat. Mol. Breed. 41, 1–21. doi: 10.1007/s11032-021-01240-1
Marshall D. E., Boese B. N. (1998). Breeding Capsicum for mechanical harvest. Part2-Equipment Proc. 10, 61–64.
Naegele R. P., Mitchell J., Hausbeck M. K. (2016). Genetic diversity, population structure, and heritability of fruit traits in Capsicum annuum. PLoS One 11(7), e0156969. doi: 10.1371/journal.pone.0156969
Neumann K., Kobiljski B., Denčić S., Varshney R. K., Börner A. (2011). Genome-wide association mapping: a case study in bread wheat (Triticum aestivum L.). Molecular Breeding 27, 37–58. doi: 10.1007/s11032-010-9411-7
Nimmakayala P., Abburi V. L., Saminathan T., Alaparthi S. B., Almeida A., Davenport B., et al. (2016). Genome-wide diversity and association mapping for capsaicinoids and fruit weight in Capsicum annuum L. Scientific Reports 6(1), 1–14. doi: 10.1038/srep38081
Otyama P. I., Wilkey A., Kulkarni R., Assefa T., Chu Y., Clevenger J., et al. (2019). Evaluation of linkage disequilibrium, population structure, and genetic diversity in the US peanut mini core collection. BMC Genomics 20, 1–17. doi: 10.1186/s12864-019-5824-9
Peng J., Richards D. E., Hartley N. M., Murphy G. P., Devos K. M., Flintham J. E., et al. (1999). ‘Green revolution’ genes encode mutant gibberellin response modulators. Nature 400, 256–261. doi: 10.1038/22307
Price A. L., Patterson N. J., Plenge R. M., Weinblatt M. E., Shadick N. A., Reich D. (2006). Principal components analysis corrects for stratification in genome-wide association studies. Nat. Genet. 38, 904–909. doi: 10.1038/ng1847
Pritchard J. K., Stephens M., Donnelly P. (2000). Inference of population structure using multilocus genotype data. Genetics 155, 945–959. doi: 10.1093/genetics/155.2.945
Qin C., Yu C., Shen Y., Fang X., Chen L., Min J., et al. (2014). Whole-genome sequencing of cultivated and wild peppers provides insights into Capsicum domestication and specialization. Proc. Natl. Acad. Sci. 111, 5135–5140. doi: 10.1073/pnas.1400975111
Ramchiary N., Kole C. (2019). The Capsicum Genome (Switzerland: Springer Nature). doi: 10.1007/978-3-319-97217-6
Remington D. L., Thornsberry J. M., Matsuoka Y., Wilson L. M., Whitt S. R., Doebley J., et al. (2001). Structure of linkage disequilibrium and phenotypic associations in the maize genome. Proc. Natl. Acad. Sci. 98, 11479–11484. doi: 10.1073/pnas.20139439
Ren W.-L., Wen Y.-J., Dunwell J. M., Zhang Y.-M. (2018). pKWmEB: integration of Kruskal-Wallis test with empirical Bayes under polygenic background control for multi-locus genome-wide association study. Heredity 120, 208–218. doi: 10.1038/s41437-017-0007-4
Rosmaina S., Hasrol F., Yanti J. (2016). Estimation of variability, heritability and genetic advance among local chili pepper genotypes cultivated in peat lands. Bulgarian J. Agric. Sci. 22, 431–436.
Saisupriya P., Saidaiah P., Pandravada S. R. (2022). Analysis of genetic variability, heritability and genetic advance for yield and yield related traits in chilli (Capsicum annuum L.). Int. J. Bio-Res. Stress Manage. 13, 387–393. doi: 10.23910/1
Sharma H., Shukla M. K., Bosland P. W., Steiner R. L. (2017). Soil moisture sensor calibration, actual evapotranspiration, and crop coefficients for drip irrigated greenhouse Chile peppers. Agric. Water Manage. 179, 81–91. doi: 10.1016/j.agwat.2016.07.001
Shen J., Zhang Y., Ge D., Wang Z., Song W., Gu R., et al. (2019). CsBRC1 inhibits axillary bud outgrowth by directly repressing the auxin efflux carrier CsPIN3 in cucumber. Proc. Natl. Acad. Sci. 116, 17105–17114. doi: 10.1073/pnas.1907968116
Solomon A. M., Han K., Lee J.-H., Lee H.-Y., Jang S., Kang B.-C. (2019). Genetic diversity and population structure of Ethiopian Capsicum germplasms. PloS One 14, e0216886. doi: 10.1371/journal.pone.0216886
Song X., Zhao Y., Wang J., Lu M.-Z. (2021). The transcription factor KNAT2/6b mediates changes in plant architecture in response to drought via down-regulating GA20ox1 in Populus alba× P. glandulosa. J. Exp. Bot. 72, 5625–5637. doi: 10.1093/jxb/erab201
Tamba C. L., Ni Y.-L., Zhang Y.-M. (2017). Iterative sure independence screening EM-Bayesian LASSO algorithm for multi-locus genome-wide association studies. PloS Comput. Biol. 13, e1005357. doi: 10.1093/jxb/erab201
Tamba C. L., Zhang Y.-M. (2018). A fast mrMLM algorithm for multi-locus genome-wide association studies. Biorxiv, 341784. doi: 10.1101/341784
Teale W. D., Paponov I. A., Palme K. (2006). Auxin in action: signalling, transport and the control of plant growth and development. Nat. Rev. Mol. Cell Biol. 7, 847–859. doi: 10.1038/nrm2020
Tibbs-Cortes L., Zhang Z., Yu J. (2021). Status and prospects of genome‐wide association studies in plants. The Plant Genome 14 (1), e20077. doi: 10.1002/tpg2.20077
Usman M. G., Rafii M. Y., Ismail M. R., Malek M. A., Abdul Latif M. (2014). Heritability and genetic advance among chili pepper genotypes for heat tolerance and morphophysiological characteristics. Sci. World J. 2014, 2–14. doi: 10.1155/2014/308042
Vikas V. K., Pradhan A. K., Budhlakoti N., Mishra D. C., Chandra T., Bhardwaj S. C., et al. (2022). Multi-locus genome-wide association studies (ML-GWAS) reveal novel genomic regions associated with seedling and adult plant stage leaf rust resistance in bread wheat (Triticum aestivum L.). Heredity 128, 434–449. doi: 10.1038/s41437-022-00525-1
Vyas D., Kar S., Thakur P. (2021). Genetic variability, heritability and correlation association studies for yield and its components in Chilli (Capsicum annum L.). The Pharma Innovation Journal 10(8):1681–1683.
Walker S. J., Funk P. A. (2014). Mechanizing Chile peppers: challenges and advances in transitioning harvest of New Mexico’s signature crop. HortTechnol. Hortte 24, 281–284. doi: 10.21273/HORTTECH.24.3.281
Walker S. J., Funk P., Joukhadar I., Place T., Havlik C., Tonnessen B. (2021). ‘NuMex odyssey’, a New Mexico–type green Chile pepper for mechanical harvest. HortScience 56, 1605–1607. doi: 10.21273/HORTSCI15793-21
Wall M. M., Walker S., Wall A. D., Hughs E., Phillips R. (2003). Yield and quality of machine harvested red Chile peppers. HortTechnology 13, 296–302. doi: 10.21273/HORTTECH.13.2.0296
Wang S.-B., Feng J.-Y., Ren W.-L., Huang B., Zhou L., Wen Y.-J., et al. (2016). Improving power and accuracy of genome-wide association studies via a multi-locus mixed linear model methodology. Sci. Rep. 6, 1–10. doi: 10.1038/srep19444
Ward S. P., Leyser O. (2004). Shoot branching. Curr. Opin. Plant Biol. 7, 73–78. doi: 10.1016/j.pbi.2003.10.002
Webb R. E., Bruce W. M. (1968). Redesigning the tomato for mechanized production. Yearbook Agric., 103–107.
Wen Y.-J., Zhang H., Ni Y.-L., Huang B., Zhang J., Feng J.-Y., et al. (2018). Methodological implementation of mixed linear models in multi-locus genome-wide association studies. Briefings Bioinf. 19, 700–712. doi: 10.1093/bib/bbw145
Werner T., Motyka V., Laucou V., Smets R., Van Onckelen H., Schmülling T. (2003). Cytokinin-deficient transgenic Arabidopsis plants show multiple developmental alterations indicating opposite functions of cytokinins in the regulation of shoot and root meristem activity. Plant Cell 15, 2532–2550. doi: 10.1105/tpc.014928
Yarnes S. C., Ashrafi H., Reyes-Chin-Wo S., Hill T. A., Stoffel K. M., Van Deynze A. (2013). Identification of QTLs for capsaicinoids, fruit quality, and plant architecture-related traits in an interspecific Capsicum RIL population. Genome 56, 61–74. doi: 10.1139/gen-2012-0083
Yoosefzadeh-Najafabadi M., Torabi S., Tulpan D., Rajcan I., Eskandari M. (2021). Genome-wide association studies of soybean yield-related hyperspectral reflectance bands using machine learning-mediated data integration methods. Front. Plant Sci. 12. doi: 10.3389/fpls.2021.777028
Zhang J., Feng J.-Y., Ni Y. L., Wen Y. J., Niu Y., Tamba C. L., et al. (2017). pLARmEB: integration of least angle regression with empirical Bayes for multilocus genome-wide association studies. Heredity 118, 517–524. doi: 10.1038/hdy.2017.8
Zhang Y.-M., Jia Z., Dunwell J. M. (2019). Editorial: the applications of new multi-locus GWAS methodologies in the genetic dissection of complex traits. Front. Plant Sci. 10. doi: 10.3389/fpls.2019.00100
Zhang Y.-W., Tamba C. L., Wen Y.-J., Li P., Ren W.-L., Ni Y.-L., et al. (2020). mrMLM v4. 0.2: an R platform for multi-locus genome-wide association studies. Genom. Proteomics Bioinf. 18, 481–487. doi: 10.1016/j.gpb.2020.06.006
Zhao D., Wang Y., Feng C., Wei Y., Peng X., Guo X., et al. (2020). Overexpression of MsGH3. 5 inhibits shoot and root development through the auxin and cytokinin pathways in apple plants. Plant J. 103, 166–183. doi: 10.1111/tpj.14717
Keywords: chile pepper mechanization, genome-wide association study, genotyping-by-sequencing, quantitative trait loci, single nucleotide polymorphisms
Citation: Khokhar ES, Lozada DN, Ali M, Khan MI, Nourbakhsh SS and Walker S (2024) Marker-trait association analysis for easy fruit destemming and mechanical harvestability traits in New Mexican chile pepper (Capsicum annuum L.). Front. Hortic. 3:1448159. doi: 10.3389/fhort.2024.1448159
Received: 12 June 2024; Accepted: 30 July 2024;
Published: 19 August 2024.
Edited by:
Sudip Kumar Dutta, ICAR Research Complex For NEH Region, Sikkim Centre, IndiaReviewed by:
Jagesh Kumar Tiwari, Indian Institute of Vegetable Research (ICAR), IndiaCopyright © 2024 Khokhar, Lozada, Ali, Khan, Nourbakhsh and Walker. This is an open-access article distributed under the terms of the Creative Commons Attribution License (CC BY). The use, distribution or reproduction in other forums is permitted, provided the original author(s) and the copyright owner(s) are credited and that the original publication in this journal is cited, in accordance with accepted academic practice. No use, distribution or reproduction is permitted which does not comply with these terms.
*Correspondence: Dennis Nicuh Lozada, ZGxvemFkYUBubXN1LmVkdQ==
Disclaimer: All claims expressed in this article are solely those of the authors and do not necessarily represent those of their affiliated organizations, or those of the publisher, the editors and the reviewers. Any product that may be evaluated in this article or claim that may be made by its manufacturer is not guaranteed or endorsed by the publisher.
Research integrity at Frontiers
Learn more about the work of our research integrity team to safeguard the quality of each article we publish.