- 1National Institute of Agricultural Botany (NIAB), East Malling, West Malling, United Kingdom
- 2Cocoa Research Institute of Ghana, Tafo, Ghana
- 3Teagasc Environment Research Centre, Wexford, Ireland
Postharvest spoilage of strawberry grown under protection, caused by Mucor spp. and Rhizopus spp., has recently become more common in the UK, but there is insufficient knowledge to develop and implement integrated management against Mucor and Rhizopus. Field sampling was conducted to obtain field data for developing models to predict the infection of Mucor and Rhizopus on strawberry fruit. Fruits were exposed to naturally occurring inoculum for a period of 24 hours before surface-sterilisation and incubation to estimate the level of infection by Mucor and Rhizopus. The observed incidence data, together with climatic conditions and inoculum trap counts, were used analysed firstly within the framework of (1) generalised linear model (GLM), and (2) classification tree. Field sampling confirmed previous research that ripening/ripe strawberry fruits are more susceptible to infection by Mucor and Rhizopus. Climatic variability, particularly in vapour pressure deficit, appears to be more important in influencing the rotting incidence of both Mucor and Rhizopus. However, the predictability of both Mucor and Rhizopus, whether as a continuous variable (incidence) in the GLM analysis or as a categorical risk in classification tree analysis, is too low to be of practical value based on those predictors used in the present study. Thus, current management may have to be based on scheduled preharvest application of alternative products to reduce infection and local pathogen inoculum as well as adopting management practice to minimise pathogen inoculum in the planting. Future research is needed to develop methods for rapid yet accurate in situ quantification of pathogen inoculum to improve disease risk predictions.
1 Introduction
Postharvest strawberry losses are largely caused by Botrytis cinerea, Mucor and Rhizopus spp (Bautista-Baños et al., 2003). IPM research on strawberry diseases has been so far focussed on B. cinerea that can infect almost every part of the strawberry plant including leaves, petiole, flowers and fruit. Predicative models for B. cinerea have been developed, implemented and used in practice (Xu et al., 2000; Xu and Berrie, 2014; Cordova et al., 2018). Under protected cropping systems, rapid removal of field heat immediately after harvesting and subsequent cool-chain management are sufficient to manage grey mould without the need to use fungicides (Berrie et al., 2022), similar to managing grey mould on protected raspberry crops (O'Neill et al., 2012). This is because nearly all crop losses due to B. cinerea under protected cropping result from post-harvest rot developing from preharvest latent infections of flowers.
In recent years, postharvest spoilage of strawberry (grown under protection to extend the length of the production season) caused by Mucor spp. and Rhizopus spp. has become more common in the UK. In comparison, little research has been directed to understand and manage fruit rotting caused by Mucor spp. and Rhizopus spp. Infection by Mucor and Rhizopus lead to soft rot disease (Dennis and Davis, 1977). Rhizopus spp. (mainly R. stolonifer and occasionally R. sexualis) and Mucor spp. (mainly M. piriformis, M. mucedo, and M. hiemalis) were reported as the main species responsible for Rhizopus and Mucor rots, respectively (Maas, 1998). Rhizopus stolonifer is one of the causal agents of soft rot disease in strawberry (Romanazzi et al., 2013; Kwinda et al., 2015). In the UK, M. piriformis was recently considered as the main pathogen of strawberry but the exact Rhizopus species identities causing strawberry decay is uncertain (Agyare, 2017). Postharvest losses of strawberry due to Rhizopus rot can increase rapidly by the contact spread from one fruit to another because of the production of long extending hyphae colonising fruits (Harris and Dennis, 1980).
We showed recently that that M. piriformis and Rhizopus had very similar epidemiological characteristics on strawberry (Agyare et al., 2020). Both pathogens are unable to infect flowers, and fruit became increasingly susceptible from the green development stage onwards. Spore germination under in vitro conditions requires near-saturation humidity. Infection of attached ripe or close to ripe fruit is affected by temperature and relative humidity, but not as much as for B. cinerea. Post-infection rot development is not much affected by temperature and humidity conditions that commonly occur under field conditions in the UK. On attached ripe fruit, rot symptoms can become visible within 3-5 five days of inoculation. There are small, though statistically significant, differences among cultivars in their susceptibility to the two pathogens. The levels of infection under field conditions varied greatly during the summer and autumn in the UK (Agyare, 2017).
Unlike B. cinerea on strawberry grown under protection, pre-harvest management intervention may be necessary for Mucor and Rhizopus under moderate to high predicted disease risks as both the pathogens can infect fruit and rapidly lead to visual pre-harvest fruit decaying. Tunnel ventilation management can greatly affect humidity and temperature at the canopy level, which may affect inoculum dispersal and fruit infection. Fungicides and alternative products (including biopesticides) tested so far do not have high efficacies against the two pathogens (Agyare, 2017). There has been insufficient knowledge to predict and manage the infection of Mucor and Rhizopus on strawberry.
There were too few combinations of climatic variables in our previous study (Agyare et al., 2020) to develop predictive models; thus we conducted field sampling to develop models to predict the risk of infection of strawberry fruit by Mucor and Rhizopus. Specifically, fruit in commercial and experimental plantings were exposed to naturally occurring inoculum for a period of 24 hours. Levels of fruit infection within each 24 h period was estimated, together with weather conditions and inoculum trapping data. Then we applied regression analysis and recursive tree analysis to the data to develop predictive models.
2 Materials and methods
Field determination of fruit infection by Mucor and Rhizopus spp. was based on recent findings that fruits become susceptible to Mucor and Rhizopus spp. from ‘pale yellow/white’ onwards with the susceptibility increasing with increasing fruit ripeness and infection can complete within a few hours (Agyare, 2017). To relate the incidence of fruit infection under field scale fruit productions to the inoculum level and weather conditions, the shorter the period of fruit exposure to pathogen inoculum, the better it is.
2.1 Sampling fruit
Field sampling was conducted in 2018, 2019 and 2023 at three sites in Kent, England; the three sites were within 10 km from each other. Table 1 gives the summary of field samples collected in each year. During the fruiting period in the summer and autumn, about 100 fruit trusses (depending on the availability) with all fruits not beyond the stage of pale yellow/white were bagged with paper bags. Thus, at the time of bagging all fruit in the selected trusses were unlikely to be already infected by Mucor or Rhizopus. Two weeks after bagging, the bags were removed at 9 am to expose fruit to naturally occurring inoculum. All exposed fruit trusses were collected and taken to the lab 24 h after exposure. During the 24 h exposure period, no fungicidal products (including biopesticides) were applied to the planting. The exposed fruits were immediately surface sterilised with 5% sodium hypochlorite solution and washed several times with sterilised distilled water (SDW). The fruits were then divided into two maturity classes (yellow and red/ripe), kept on seeding trays containing paper tissue, covered with a plastic bag, and incubated at ambient conditions (ca. 20-25°C) for 3-7 days. Individual fruits were placed far apart from each other. Fruit was assessed daily for visible rotting; rot pathogens growing on the fruits were identified based on morphological characteristics (Agyare, 2017). Thus, each batch of sampled trusses generated two data points: one for yellow (immature) and the other for red/ripe fruit. This process was repeated to obtain as many samples as possible (Table 1).
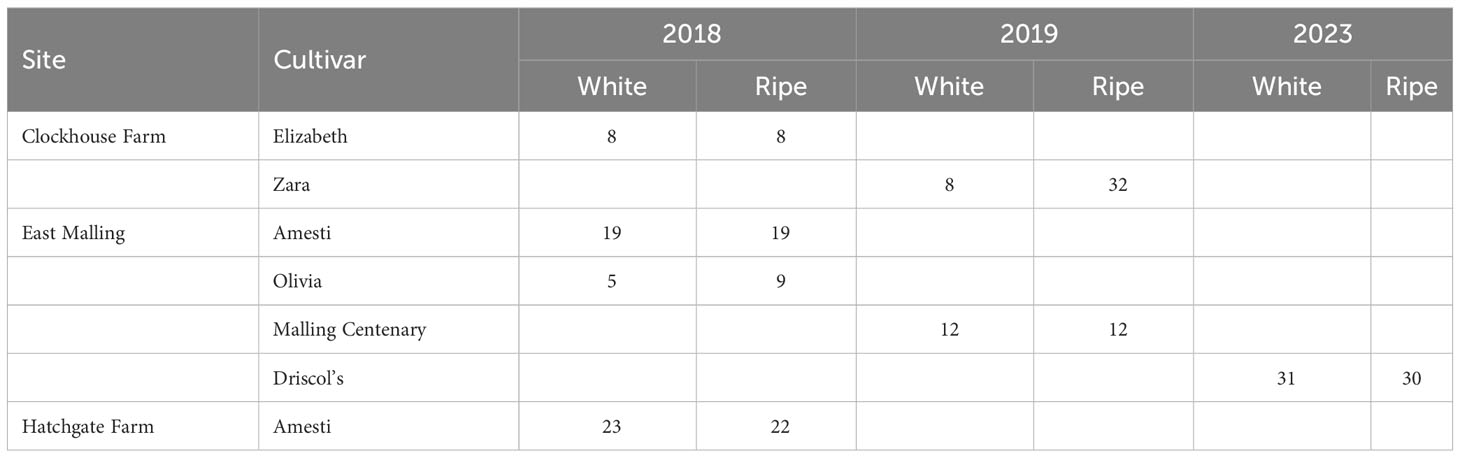
Table 1 Number of samples of strawberry fruit at the white and ripe stage obtained to determine the level of fruit infection by Mucor and Rhizopus spp. within a period of 24 h exposure to natural pathogen inoculum in 2018, 2019 and 2023 [only those samples with the total number of fruits of at least 20 were included].
Weather data [temperature, relative humidity (RH) and dew temperature (DewT)] were captured by USB502 data logger (www.mccdaq.com) at an interval of 20 min. Vapour pressure deficit (VPD) was then calculated from temperature and RH.
2.2 Trapping Mucor and Rhizopus inoculum
Inoculum during each 24 h exposure period was trapped based on the MYA-K selective media method (Agyare, 2017). The basal medium MYA (malt yeast agar) was prepared (malt extract 20 g l-1, yeast extract 2 g l-1 and agar 15 g l-1), autoclaved at 121°C for 15 mins and kept in 50°C water bath for addition of chloramphenicol and ketoconazole. Chloramphenicol (0.25 g) was dissolved in 5 ml 98% absolute ethanol, and filter sterilised with 0.22 µm sterile acrodisc filter (GelmanSciences). Ketoconazole (0.25 g) was dissolved in 2 ml 95% absolute ethanol and filter sterilised with the 0.22 µm sterile acrodisc filter. The prepared chloramphenicol and ketoconazole solutions were added to the 50°C molten MYA medium (500 ml). The medium was then thoroughly mixed and poured into 90 mm Petri plates.
Sterile filter papers (90 mm Fisherbrand 11566873) were placed in 90 mm Petri plate lids in a sterile flow cabinet. A pipette was used to add 1 ml of SDW onto the paper to stabilise the paper on the lid. The lids were covered with the inverted Petri plates. One plate was exposed at each of three randomly selected positions within each planting. The same positions for inoculum trapping were maintained during all 24 h exposure periods in the same planting. The filter papers containing trapped spores were transferred to MYA-K selective media with the exposed paper surface facing up using sterile forceps in a flow cabinet. These were then incubated at 21°C for 4-7 days and inspected daily for fungal growth. The fungal colonies growing on the selective media-contained paper were identified based on morphology and counted (Figure 1).
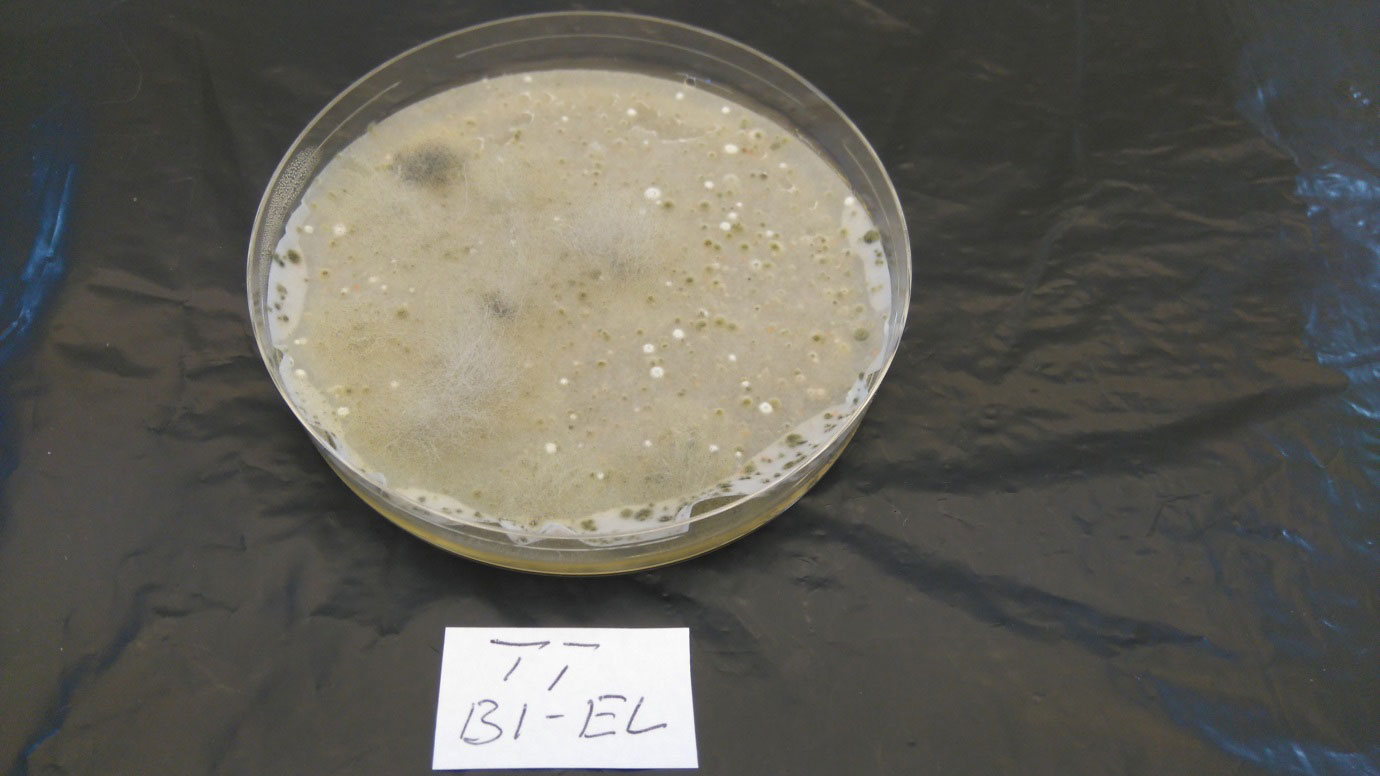
Figure 1 MYA-K selective medium showing colony growth of trapped spores from filter-paper inoculum sampling.
2.3 Data analysis
Two statistical approaches were used to model the relationships of disease incidence with weather conditions and inoculum strength. Firstly, data were analysed within the GLM (generalised linear model) framework, in which residual errors were assumed to follow a quasibinomial distribution and the logit was used as the link function. Secondly, the incidence data were transformed into three categories: low, moderate and high, and then subjected to classification tree analysis, which was conducted with the rpartScore package in R (Galimberti et al., 2012). The criteria for classifying disease incidences into the three categories were based primarily on the need to have enough cases in the categories of moderate and high risks, but also considered the minimum sample size in the present study. The rpartScore package was designed specifically for building classification trees for ordinal responses within the general framework of classification and regression tree (CART) analysis. In the present study, classification trees were built on the generalised Gini impurity function and their predictive performance was assessed based on the misclassification costs. There is an in-built capability of cross-validation in rpartScore; in the present study, a 10-fold cross-validation was used. Fitted trees were pruned based on the rule of one standard error of predictive performance (one-se-pr) (Breiman et al., 1984), namely the final tree was the smallest/simplest tree with the average relative predictive performance within one-se-pr of the minimum average relative predictive performance of all tree sizes. The goodness of fit was statistically assessed by the linear-by-linear association test (implemented in the R coin package (https://rdocumentation.org/packages/coin/versions/1.4-3).
Each 24-h period was divided into day (9:00 am to 20:59 pm) and night (21:00 pm to 8:59 am next day). Average temperature, RH, VPD, and DewT were calculated for each day and night period; similarly, the coefficient of variation (CV) of each of the four variables was also calculated for each time period. Furthermore, 24 h (daily) average of each of the four variables was calculated, together the corresponding CVs. The cross product between log-transformed 24 h inoculum counts and each of the four daily average variables was calculated to represent the interaction between inoculum and weather conditions. Inoculum counts were logarithmically (on the natural base) transformed as ln(x+1.5) instead of the usual ln(x+1.0) to prevent zero values when inoculum was multiplied by other predictors. Table 2 give the summary of all predictors used in modelling. Two sets of predicators were used for developing GLM and classification tree models – (1) all predictors and (2) all predictors with those inoculum-related variables excluded.
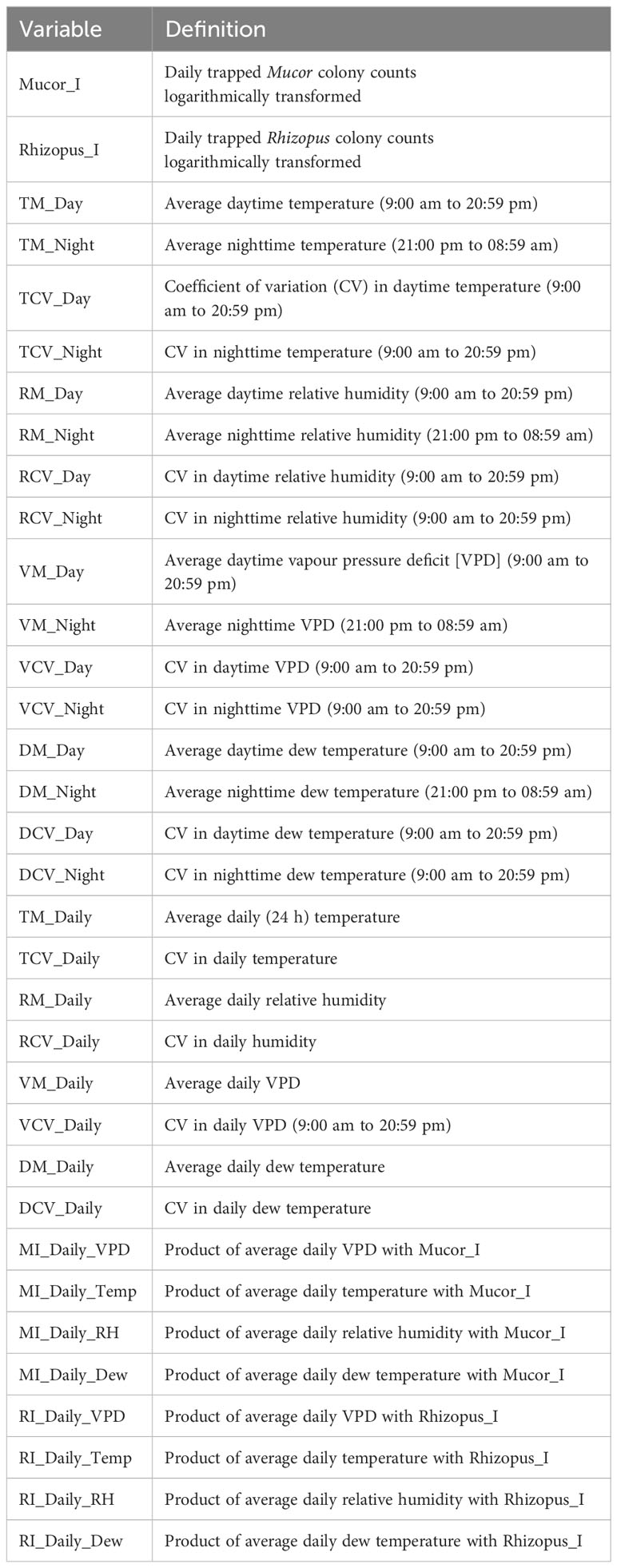
Table 2 Summary of all predictors used to develop models for incidence of strawberry fruit rotting caused by Mucor and Rhizopus in 24-h exposure periods.
All analyses were carried out in R version 3.6.0 (Team, 2019).
3 Results
3.1 Observed fruit infection and weather conditions
A total of 266 samples of strawberry fruit at the white or ripe stage were obtained in the three years. Only 238 of the 264 samples were retained for statistical analysis (Table 1) because the remaining 26 samples had fewer than 20 fruit and hence were excluded. Figure 2 shows the average daytime and nighttime values of temperature, RH, VPD and DewT for all 24 h exposure periods. Overall, weather conditions were less variable and moister in 2023 than in 2018 and 2019.
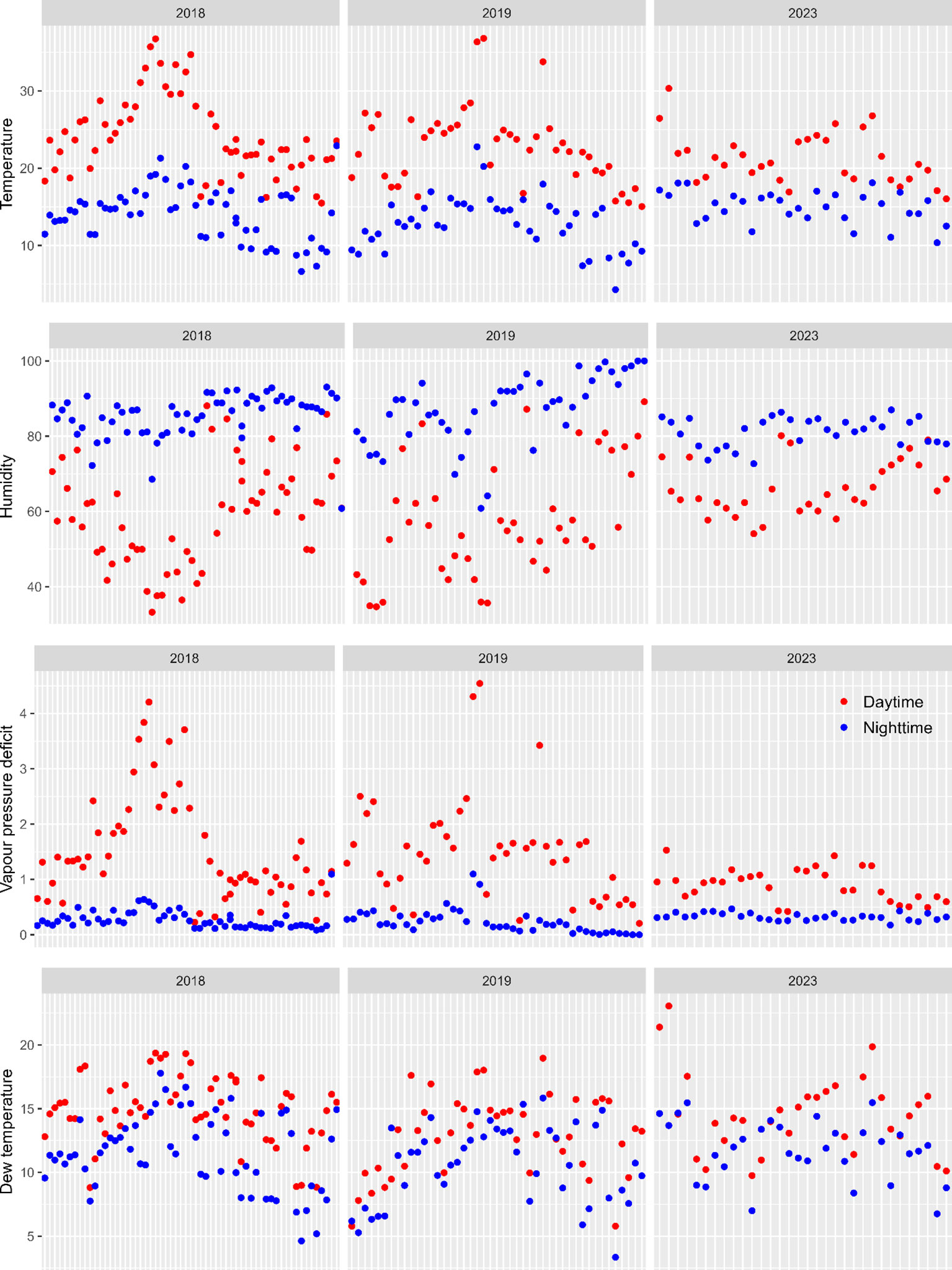
Figure 2 Average day (9:00 am to 20:59 pm; red) and night (21:00 pm to 8:59 am next day; blue) values of temperature, humidity, vapour pressure deficit and dew temperature for all 24 h periods (x-axis) in which strawberry fruit were exposed to natural inoculum in strawberry plantings under protection.
There were large differences in both Mucor and Rhizopus incidences among the three years (Figure 3). Overall, Mucor incidence was 2.2%, 5.0% and 7.2% for 2018, 2019 and 2023, respectively; the corresponding values for Rhizopus were 4.3%, 19.3% and 3.4%. On average, disease incidence was higher on ripe fruit than on white fruit: 12.1% vs 2.8% (Rhizopus) and 6.4% vs 1.4% (Mucor). Incidences on white fruit were mostly close to zero, particularly for Mucor (Figure 3). The incidence varied greatly among individual 24 h exposure periods. For Rhizopus, incidence was zero in 64 of the 132 ripe fruit samples, and in 69 of the 106 white fruit samples. For Mucor, no rotting was observed in 50 of the 132 ripe fruit samples, and in 78 of the 106 white fruit samples. Trapped inoculum counts were variable, particularly in 2018; inoculum counts were lower in 2023 than in the other two years (Figure 3).
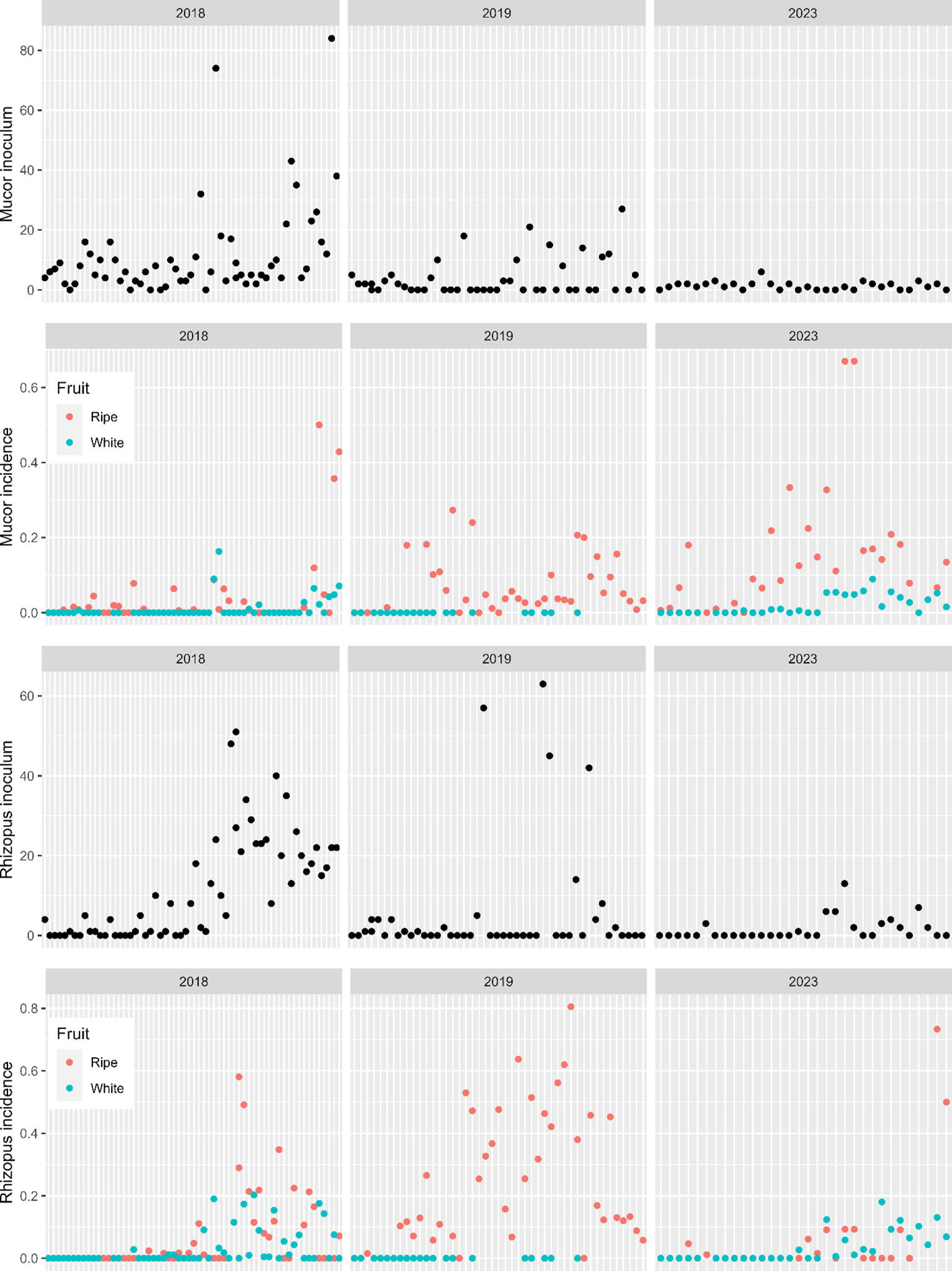
Figure 3 Observed incidence of infection of strawberry fruit by Mucor and Rhizopus spp. during individual 24 h exposure periods (x-axis) under protection, and the corresponding trapped inoculum counts based on selective media.
3.2 GLM analysis of fruit rot incidence
Both Pearson and Spearman correlation (r) of rotting incidences with other variables is given in Supplementary Table 1. Pearson correlation of the Rhizopus rotting incidence with summary weather variables was low, ranging from -0.161 to 0.317, but statistically significant (P < 0.05) for TM_Night (r = -0.161), TCV_Day (r = 0.260), RM_Night (r = 0.135), RCV_Day (r = 0.285), VM_Night (r = -0.145), VCV_Day (r = 0.317), TCV_Daily (r = 0.142), and VCV_Daily (r = 0.252). For the Mucor rotting incidence, Pearson correlation ranged from -0.162 to 0.105 and statistically significant (P < 0.05) for TM_Day (r = -0.146), RCV_Night (r = -0.143), VM_Day (r = -0.145) and RCV_Daily (r = -0.162). Daily trapped inoculum counts were not significantly corelated with the disease incidence for both pathogens. Pearson correlation of inoculum counts with weather variables was also low: from -0.132 to 0.148 and from -0.148 to 0.206 for Mucor and Rhizopus, respectively.
For both Mucor and Rhizopus, GLM models fitted the data poorly (Figure 4). For Mucor, the best model consisted of four predictors: fruit stage (P < 0.01) and three variables describing daily CV in RH (RCV_Daily, P < 0.001, slope = -8.09), temperature (TCV_Daily, P < 0.05, slope = 6.32) and DewT (DCV_Daily, P < 0.05, slope = -6.71). However, the Pearson correlation between fitted and observed values was only 0.37. After the four predictors were included, there were still large significant differences among the three years.
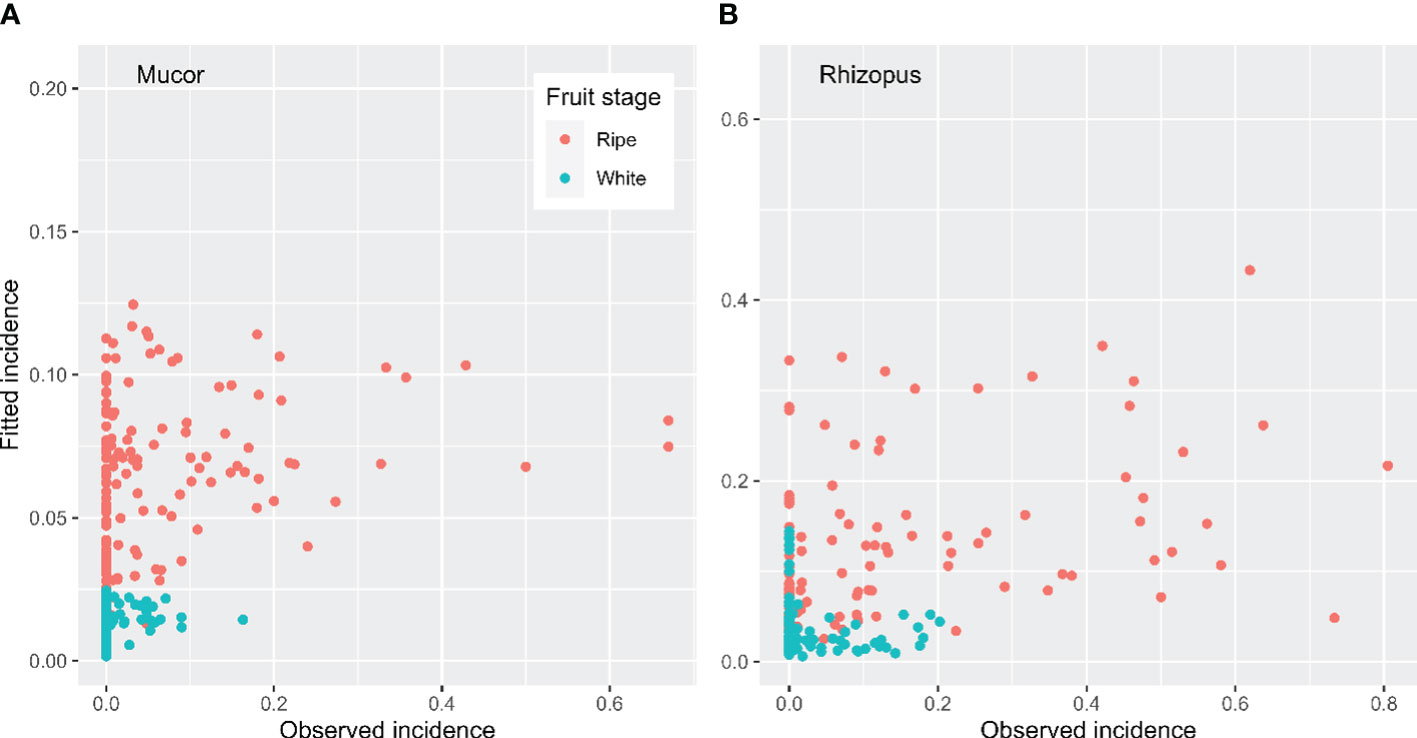
Figure 4 Fitted incidence of fruit rotting caused by (A) Mucor and (B) Rhizopus spp. in 24 h exposure periods. GLM was used to relate observed incidences to weather conditions and trapped inoculum during the exposure periods.
For Rhizopus, the best GLM model consisted of four predictors: fruit stage (P < 0.001) and three variables describing daytime CV in RH (RCV_Day, P < 0.001, slope = 9.01), temperature (TCV_Day, P < 0.01, slope = -8.26) and VPD (VCV_Day, P < 0.001, slope = 3.87). The Pearson correlation between fitted and observed values was only 0.48. After the four predictors were included, the differences among the three years were no longer statistically significant.
Goodness of fit failed to improve when GLM analysis was applied to only ripe fruit data. Three CV variables in daily RH (RCV_Daily), temperature (TCV_Daily) and DewT (DCV_Daily) were included for Mucor, and only the daytime CV of RH (RCV_Day) was needed for Rhizopus. However, the Pearson correlation between fitted and observed values were only 0.27 for Mucor and 0.38 for Rhizopus.
3.3 Classification trees
For Mucor, risk for a given 24 h exposure period was classified as ‘low’, ‘moderate’ and ‘high’ when the observed incidence was < 1%, in the range of 1% to 7.5%, and ≥ 7.5%, respectively. Similarly, for Rhizopus, it was classified as ‘low’, ‘moderate’ and ‘high’ when the observed incidence was < 5%, in the range of 5% to 15%, and ≥ 15%, respectively. There were 144, 53 and 41 cases when Mucor risk was in the low, moderate and high category; the corresponding values for Rhizopus were 159, 41 and 38.
The classification tree for Mucor when all predictors were included had three splits and four terminal nodes (Figure 5). The first split was determined by fruit development stage – all white fruit samples were given the low risk. The next split level was determined by the product of inoculum counts with average daily VPD (MI_Daily_VPD): values higher than 0.716 led to low risks. Average nighttime RH (RM_Night) determined the final split: RH higher than 87.3% led to moderate risks and otherwise high risks.
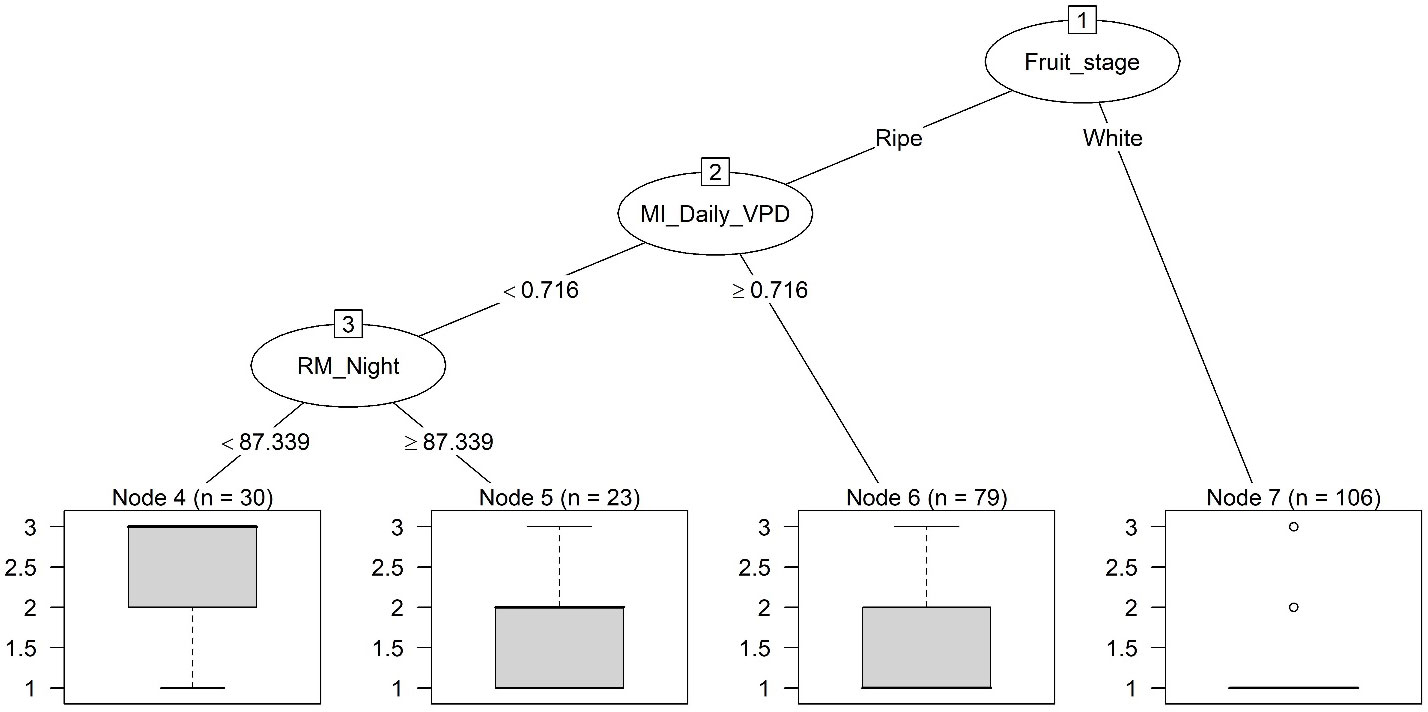
Figure 5 Classification trees of categorical risks of strawberry fruit infection by Mucor in a 24-h exposure period. All weather and inoculum-related predictors were included. There were three levels of risks: low, moderate and high, corresponding to a nominal numeric value of 1, 2 and 3, respectively. The thick horizontal line is the predicted risk, and the box plot represents observed data under each terminal node. Refer to Table 2 for definition of predictors.
The classification tree for Mucor when inoculum-related predictors were excluded had five splits at four levels and six terminal nodes (Figure 6). The first split was also determined by fruit development stage – all white fruit samples were given the low risk. The 2nd and 3rd level splits were determined by variability in weather conditions (Figure 6), initially by the CV in daily VPD (VCV_VPD) and then by the CV in nighttime VPD (VCV_Night). The final split was determined by daily average RH (RM_Daily). Lower variability in VPD coupled with high RH led to high disease risks. However, extreme high daily variability in VPD (CV > 98.1%) also led to moderate disease risks, compared to the risk under moderate variability in VPD (CV in the range of 74.3% to 98.1%) (Figure 6).
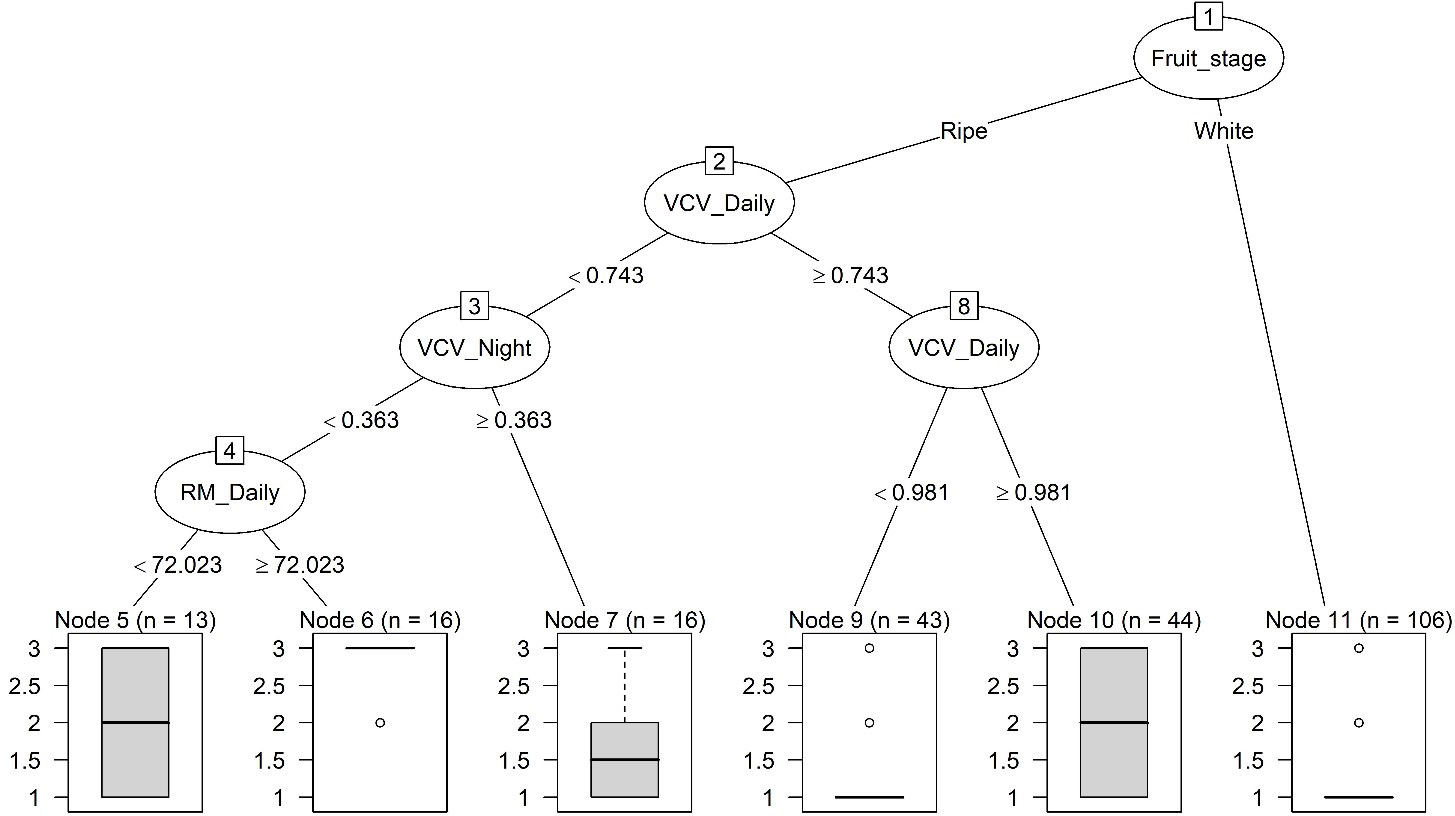
Figure 6 Classification trees of categorical risks of strawberry fruit infection by Mucor in a 24-h exposure period. Only weather predictors were included. There were three levels of risks: low, moderate and high, corresponding to a nominal numeric value of 1, 2 and 3, respectively. The thick horizontal line is the predicted risk, and the box plot represents observed data under each terminal node. Refer to Table 2 for definition of predictors.
Both sets of predictors (all, and those excluding inoculum-related predictors) produced the same tree for Rhizopus. This simple tree only had two splits and three terminal nodes (Figure 7). The first split occurred at the condition of whether the daytime CV of VPD (VCV_Day) was < 0.39. If this condition was satisfied, a low risk was predicted. If the condition was not satisfied, a low risk was also given for white fruit and a moderate risk for ripe fruit. Thus, this decision tree did not generate any prediction of high risks (Table 2).
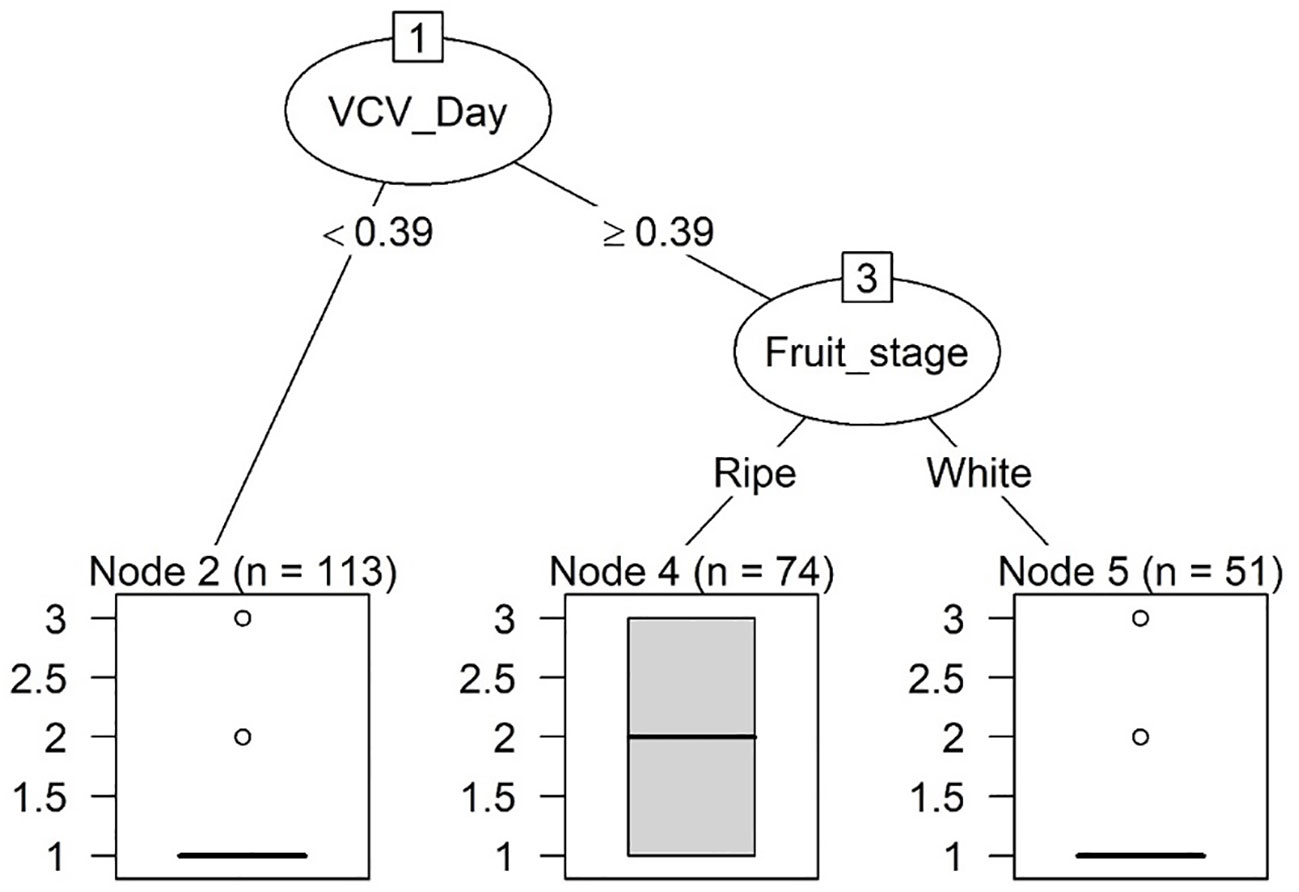
Figure 7 Classification trees of categorical risks of strawberry fruit infection by Rhizopus in a 24-h exposure period. All predictors (including inoculum-related ones) were included. There were three levels of risks: low, moderate and high, corresponding to a nominal numeric value of 1, 2 and 3, respectively. The thick horizontal line is the predicted risk, and the box plot represents observed data under each terminal node. Refer to Table 2 for definition of predictors.
Although all fitted risk values were highly associated with observed values for all three trees, there were misclassifications for many samples (Table 3). The Mucor tree based on all predictors correctly predicted the risk level for 163 out of the total 238 samples (68.5%), whereas the weather-only tree gave correct predictions for 160 cases (67.2%). For the Rhizopus, the tree correctly predicted risks for 155 cases (65.1%).
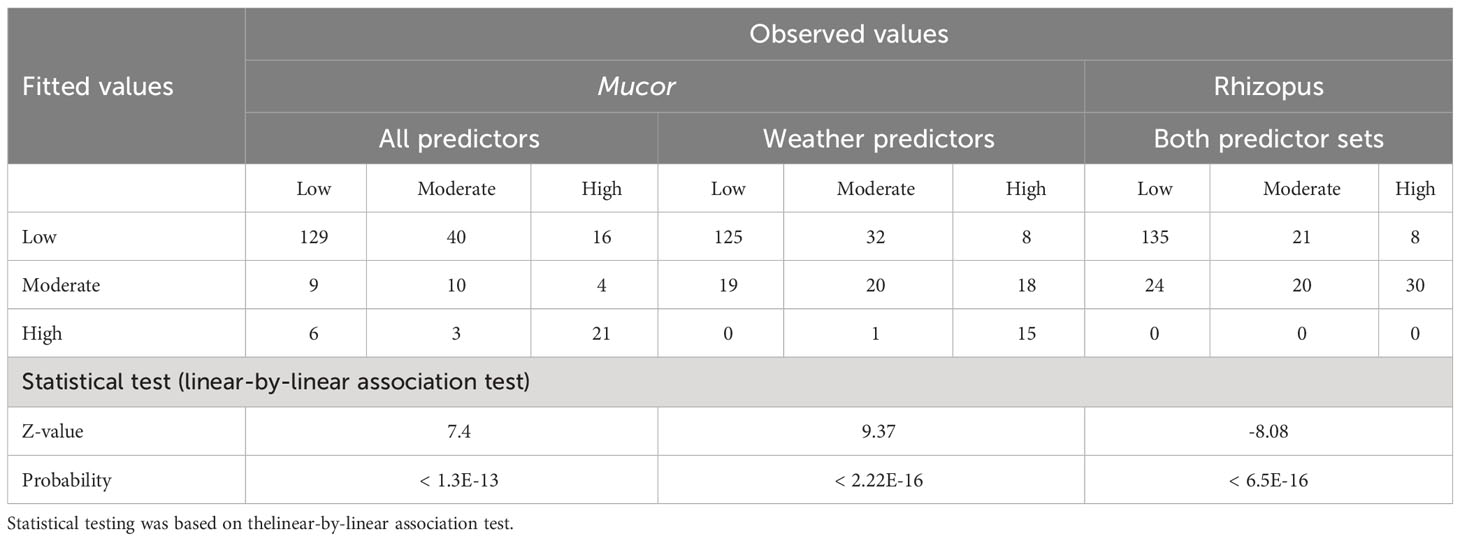
Table 3 Summary of classification tree analysis of daily risks of strawberry fruit infection by Mucor and Rhizopus.
4 Discussion
The present study confirmed previous research that ripening/ripe strawberry fruits are more susceptible to infection by Mucor and Rhizopus. It is primarily the variability of vapour pressure deficit that appears to be the important weather conditions affecting rot development for both Mucor and Rhizopus. However, the predictability of both Mucor and Rhizopus based on the current set of predictors is too low to be of practical value.
White/yellow fruit were much less susceptible to Mucor and Rhizopus than ripe fruit, agreeing with previous research (Agyare et al., 2020). The classification tree model for Mucor gives low risks for white fruits. However, for several samples the incidence of Rhizopus on white fruits reached > 15%. Indeed, the fitted classification tree for Rhizopus did not have the fruit age as the first split criterion. Thus, for predicting and managing soft rot development on white fruits, infection by Mucor may be ignored but not Rhizopus.
The present study suggested that it is the variability in climactic conditions, particularly vapour pressure deficit, rather than the absolute level, which appears to be more important in influencing infection of strawberry fruit by Mucor and Rhizopus. The importance of variability in climatic conditions on rot incidences may be explained by two reasons. Firstly, substantial changes in air moisture may increase inoculum mortality and/or reduce spore germination/infection. Spore germination is more affected by relative humidity than by temperature (Agyare et al., 2020). Secondly, substantial changes in weather conditions may increase spore dispersal from the ground to the canopy. Spreading plant pathogens by water splash is an important mechanism of fungal inoculum dispersal (Fitt et al., 1989; Madden, 1997). However, under protected production without overhead watering, aerial inoculum dispersal is the main mechanism for fungal inoculum movement. Initial dislodging of spores from sporing bodies on the ground level into air to reach sufficient height (around 1 m for the table-top production of strawberry in the sampled planting) may be increased under rapid/large changing climatic conditions (e.g. changes in relative humidity/vapour pressure deficit) in addition to disturbance by fruit picking and other management practice.
In both GLM and classification tree models for both Mucor and Rhizopus, only once was an inoculum-related variable included – this is the interaction (product) term between logarithmically-transformed inoculum and daily average vapour pressure deficit in the Mucor classification tree model. A high value (> 0.716) of this interaction term led to lower risks. For this term, high values may result from high vapour pressure deficit and/or high inoculum. However, high inoculum leading to low disease risks does not sound biologically plausible. It is thus more likely that high vapour pressure deficit (drier conditions) is responsible for this classification criterion. Interaction terms are in general difficult to interpret biologically for field observational data as for interpretation of inter-correlated predictors in GLM. Another issue may affect the interpretation of this interaction term (also the general model performance) is the temporal pattern of inoculum availability and weather conditions within a 24 h period. Unlike in controlled inoculation studies, there is no synchrony between inoculum arrival (hence inoculation) and specific weather condition, which always presents problems for evaluating disease predicative models under commercial conditions.
Whether the incidence is modelled as a continuous variable or an ordinal variable, the present study showed low predictability of soft rot incidences on strawberry fruits. This low predictability may be explained by several reasons in addition to the aforementioned asynchrony between inoculation and specific weather condition. Firstly, this low predictability is consistent with the conclusion from our previous artificial inoculation research under controlled conditions that climatic conditions are unlikely to be the main factor limiting infection of fruit by M. piriformis and Rhizopus for the main strawberry production season in the UK (Agyare et al., 2020). Secondly, inoculum may have not been accurately quantified in the present study, which probably explains the lack of relationship of disease incidence with inoculum strength. We used a selective media method that relies on passive inoculum impaction and hence does not sample equal air volume among sampling periods unlike Burkard trap, a commonly used spore sampler (Frenz, 1999; Blanco et al., 2004). Accurate differentiation of fungal colonies in the media based on morphology may not be always possible and relies on specific expertise. Finally, varietal differences in disease susceptibility, although contributing far less to fruit rotting than fruit age (Agyare et al., 2020), may be included into the model to improve model performance. Including varietal information into a model may improve its predictive performance but also limits its applicability because of the rapid cultivar turnover in commercial strawberry production.
A number of alternative products when applied preharvest were found to have significantly reduced strawberry post-harvest rotting, including Mucor and Rhizopus (Romanazzi et al., 2016). A few biocontrol agents were effective against Mucor and Rhizopus, but the efficacy varied largely with strawberry cultivars (Błaszczyk et al., 2022). Given the low predictability of soft rot infection, scheduled application of these products may be necessary at least for now instead of based on predicted disease risks. Other management strategies may include suppressing inoculum and cool-chain management. Under protected cropping systems, rapid removal of field heat immediately after harvesting and subsequent cool-chain management are sufficient to manage grey mould without the need to use fungicides for strawberry (Berrie et al., 2022) and raspberry (O'Neill et al., 2012). Such a cool chain is more likely to have some effects against Rhizopus than Mucor since the latter can survive and grow under temperature in the range of 0-4°C better than Rhizopus. However, it should be noted that cold-tolerant Rhizopus strains were found to cause strawberry soft rots in New Zealand (Siefkes-Boer et al., 2009).
Soil management under protection for table-top strawberry production may be still necessary because population dynamics of Mucor piriformis in pear orchards is affected by soil moisture and temperature (Spotts and Cervantes, 1994). Practical management practices include using cover crops to ensure good ground vegetation. To investigate the effectiveness of these measures as well as to quantify inoculum strength for predicting disease risks, there is an urgent need to develop methods for rapid yet accurate in situ quantification of pathogen inoculum. Recently, qPCR quantification has been combined with Burkard samplers to quantify airborne inoculum (Cao et al., 2016; Pizolotto et al., 2022). Other approaches include detection and quantification based on immune response (Meyer et al., 2000; Yucel et al., 2005) and pathogen specific volatiles (Jiang et al., 2023).
In summary, fruit are more susceptible to Mucor and Rhizopus at the ripe stage than at the white/yellow stage. Variability in climatic conditions, particularly in vapour pressure deficit, appears to be the most important factor influencing soft rot development. However, the overall predictability of infection of fruit by Mucor and Rhizopus is low. Future research should focus on developing (1) tools for accurate and rapid in situ inoculum quantification and (2) strategies for minimising local inoculum.
Data availability statement
The raw data supporting the conclusions of this article will be made available by the authors, without undue reservation.
Author contributions
XX: Conceptualization, Formal analysis, Funding acquisition, Project administration, Resources, Supervision, Writing – original draft, Writing – review & editing. SA: Data curation, Writing – review & editing, Methodology. EB: Data curation, Methodology, Writing – review & editing. TP: Data curation, Writing – review & editing, Project administration, Supervision.
Funding
The author(s) declare financial support was received for the research, authorship, and/or publication of this article. This research was funded by Innovate UK (project number 132749) and Biotechnology and Biological Sciences Research Council (BB/X01181X/1), UK.
Acknowledgments
We thank our colleagues Joyce Robinson, Georgina Fagg and Jennier Kingsnorth for helping field sampling.
Conflict of interest
The authors declare that the research was conducted in the absence of any commercial or financial relationships that could be construed as a potential conflict of interest.
The author(s) declared that they were an editorial board member of Frontiers, at the time of submission. This had no impact on the peer review process and the final decision.
Publisher’s note
All claims expressed in this article are solely those of the authors and do not necessarily represent those of their affiliated organizations, or those of the publisher, the editors and the reviewers. Any product that may be evaluated in this article, or claim that may be made by its manufacturer, is not guaranteed or endorsed by the publisher.
Supplementary material
The Supplementary Material for this article can be found online at: https://www.frontiersin.org/articles/10.3389/fhort.2024.1373717/full#supplementary-material
References
Agyare S. (2017). “The Epidemiology and Management of Strawberry Rot Complex,” in School of Energy, Environment and Agrifood. (Cranfield University, Cranfield), 306.
Agyare S., Magan N., Xu X. (2020). Conditions for infection of strawberry fruit by M. piriformis and Rhizopus spp. Eur. J. Plant Pathol. 157, 65–75. doi: 10.1007/s10658-020-01981-3
Bautista-Baños S., Garcıía-Domıínguez E., Barrera-Necha L. L., Reyes-Chilpa R., Wilson C. L. (2003). Seasonal evaluation of the postharvest fungicidal activity of powders and extracts of huamuchil (Pithecellobium dulce): Action against Botrytris cinerea, Penicillium digitatum and Rhizopus stolonifer of strawberry fruit. Postharvest Biol. Technol. 29, 81–92. doi: 10.1016/S0925-5214(02)00244-2
Berrie A., Passey T., Xu X. (2022). Integrating management of powdery mildew with Botrytis in protected strawberries in the UK. Crop Prot. 154, 105902. doi: 10.1016/j.cropro.2021.105902
Blanco C., de los Santos B., Barrau C., Arroyo F. T., Porras M., Romero F. (2004). Relationship among concentrations of Sphaerotheca macularis conidia in the air, environmental conditions, and the incidence of powdery mildew in strawberry. Plant Dis. 88, 878–881. doi: 10.1094/PDIS.2004.88.8.878
Błaszczyk J., Nawrocki J., Łysiak G. P. (2022). The effect of the method of plant protection on the quality of remontant strawberry cultivars grown in a gutter system under covers. Agriculture 12, 1–12. doi: 10.3390/agriculture12122041
Breiman L., Friedman J., Olshen R. A., Stone C. J. (1984). Classification and regression trees. (New York: Taylor & Francis).
Cao X., Yao D., Zhou Y., West J. S., Xu X., Luo Y., et al. (2016). Detection and quantification of airborne inoculum of Blumeria graminis f. sp. tritici using quantitative PCR. Eur. J. Plant Pathol. 146, 225–229. doi: 10.1007/s10658-016-0908-8
Cordova L. G., Ellis M. A., Wilson L. L., Peres N. A. (2018). Evaluation of the Florida strawberry advisory system for control of Botrytis and anthracnose fruit rots in Ohio. Plant Health Prog. 19, 182–187. doi: 10.1094/PHP-10-17-0062-RS
Dennis C., Davis R. P. (1977). Susceptibility of strawberry varieties to post-harvest fungal spoilage. J. Appl. Bacteriology 42, 197–206. doi: 10.1111/j.1365-2672.1977.tb00685.x
Fitt B. D. L., McCartney H. A., Walklate P. J. (1989). The role of rain in dispersal of pathogen inoculum. Annu. Rev. Phytopathol. 27, 241–270. doi: 10.1146/annurev.py.27.090189.001325
Frenz D. A. (1999). Comparing pollen and spore counts collected with the Rotorod Sampler and Burkard spore trap. Ann. Allergy Asthma Immunol. 83, 341–349. doi: 10.1016/S1081-1206(10)62828-1
Galimberti G., Soffritti G., Maso M. D. (2012). Classification trees for ordinal responses in R: The rpartScore package. J. Stat. Software 47, 1–25. doi: 10.18637/jss.v047.i10
Harris J. E., Dennis C. (1980). Distribution of Mucor piriformis, Rhizopus sexualis and R. stolonifer in relation to their spoilage of strawberries. Trans. Br. Mycological Soc. 75, 445–450. doi: 10.1016/S0007-1536(80)80125-2
Jiang L., Dumlao M. C., Donald W. A., Steel C. C., Schmidtke L. M. (2023). Rapid in-field volatile sampling for detection of Botrytis cinerea infection in wine grapes. Molecules 28, 5227. doi: 10.3390/molecules28135227
Kwinda G. T., Jacobs A., Rong I. H., Lebelo S. L. (2015). Mucorales from selected spoilt fruit commodities in the Gauteng Province, South Africa. Afr. Plant Prot. 18, 1–5.
Madden L. V. (1997). Effects of rain on splash dispersal of fungal pathogens. Can. J. Plant Pathol. 19, 225–230. doi: 10.1080/07060669709500557
Meyer U. M., Spotts R. A., Dewey F. M. (2000). Detection and quantification of Botrytis cinerea by ELISA in pear stems during cold storage. Plant Dis. 84, 1099–1103. doi: 10.1094/PDIS.2000.84.10.1099
O'Neill T., Wedgwood E., Berrie A. M., Allen J., Xu X. M. (2012). Managing grey mould on raspberry grown under protection without use of fungicides during flowering and fruiting. Agron. Sustain. Dev. 32, 673–682. doi: 10.1007/s13593-011-0063-8
Pizolotto C. A., Harrington M., Brown L., Murdock M., Harrington S., Marshall J., et al. (2022). A real-time PCR assay for Erysiphe betae and its effectiveness when used with different spore trapping methods. Eur. J. Plant Pathol. 162, 329–341. doi: 10.1007/s10658-021-02405-6
Romanazzi G., Feliziani E., Landi L. (2016). Preharvest treatments with alternatives to conventional fungicides to control postharvest decay of strawberry. Int. Soc. Hortic. Sci. (ISHS) Leuven Belgium pp, 111–118. doi: 10.17660/ActaHortic.2016.1117.19
Romanazzi G., Feliziani E., Santini M., Landi L. (2013). Effectiveness of postharvest treatment with chitosan and other resistance inducers in the control of storage decay of strawberry. Postharvest Biol. Technol. 75, 24–27. doi: 10.1016/j.postharvbio.2012.07.007
Siefkes-Boer H. J., Boyd-Wilson K. S. H., Petley M., Walter M. (2009). Influence of coldstorage temperatures on strawberry leak caused by Rhizopus spp. New Z. Plant Prot. 62, 243–249. doi: 10.30843/nzpp.2009.62.4827
Spotts R. A., Cervantes L. A. (1994). Effects of soil moisture, temperature and nutrient availability on the population dynamics of Mucor piriformis. Mycological Res. 98, 342–346. doi: 10.1016/S0953-7562(09)80462-0
Team R. C. D. (2019). R: A Language and environment for statistical computing. (Vienna, Austria: R Foundation for Statistical Computing).
Xu X. M., Berrie A. M. (2014). Use of a disease forecasting system to manage strawberry grey mould. Acta Hortic. 1049, 613–619. doi: 10.17660/ActaHortic.2014.1049.95
Xu X., Harris D. C. C., Berrie A. M. M. (2000). Modeling infection of strawberry flowers by Botrytis cinerea using field data. Phytopathology 90, 1367–1374. doi: 10.1094/PHYTO.2000.90.12.1367
Keywords: strawberry soft rot, classification, recursive trees, fruit maturity, variability in climatic conditions
Citation: Xu X, Agyare S, Browne E and Passey T (2024) Predicting infection of strawberry fruit by Mucor and Rhizopus spp. under protected conditions. Front. Hortic. 3:1373717. doi: 10.3389/fhort.2024.1373717
Received: 20 January 2024; Accepted: 21 February 2024;
Published: 07 March 2024.
Edited by:
Paul M. Severns, University of Georgia, United StatesReviewed by:
Clarence Codod, University of California, Davis, United StatesOzkan Kaya, Erzincan Horticultural Research Institute, Türkiye
Copyright © 2024 Xu, Agyare, Browne and Passey. This is an open-access article distributed under the terms of the Creative Commons Attribution License (CC BY). The use, distribution or reproduction in other forums is permitted, provided the original author(s) and the copyright owner(s) are credited and that the original publication in this journal is cited, in accordance with accepted academic practice. No use, distribution or reproduction is permitted which does not comply with these terms.
*Correspondence: Xiangming Xu, eGlhbmdtaW5nLnh1QG5pYWIuY29t