- 1Department of Crop Science, University of Cape Coast, University Post Office, Cape Coast, Ghana
- 2International Institute of Tropical Agriculture, Ibadan, Nigeria
- 3École Supérieure d’Agronomie - Université de Lomé (ESA-UL), Togo, Ghana
- 4Department of Agricultural Economics and Extension, University of Cape Coast, University Post Office, Cape Coast, Ghana
- 5Department of Crop Production, Université Evangélique en Afrique, Bukavu, Democratic Republic of Congo
Introduction: Bush yam (Dioscorea praehensilis Benth.) is an important semi-domesticated food crop in West Africa. Limited information on the genetic architecture and its poor post-harvest tuber quality traits significantly hinder its use as food and source of income. Hence, dissecting the genetics underlying the expression of its post-harvest tuber quality traits is essential for establishing proper breeding schemes.
Methods: In this study, 138 D. praehensilis accessions collected in Ghana were sequenced using Diversity Array Technology (DArTSeq). The materials were profiled for dry matter content (DMC), tuber flesh oxidation (TBOXI) and for tuber flesh hardness (TBhard) during two cropping seasons.
Results and discussion: Diversity assessment using population structure, principal component analysis and hierarchical clustering methods revealed the presence of three major groups. Six genetic models were used for the trait association analysis using multiple random locus mixed linear model (MrMLM). Sixteen SNP markers distributed across the yam genome were identified to be associated with the evaluated traits. The associated SNP markers displayed a phenotypic variance ranged from 4.22% in TBHard to 16.92% in TBOXI. A total 25 putative candidate genes were identified around the SNP markers. The putative genes were identified to play key roles in tuber bulking, oxidative browning and starch hydroxylase. This study provides a valuable insight on the genetics underlying tuber quality traits in bush yam and opens avenues for developing genomic resources to improve D. praehensilis.
1 Introduction
Yam (Dioscorea spp.) is a significant root crop with potential of alleviating poverty and food insecurity in the tropics and sub-tropics (Cormier et al., 2019; Wu et al., 2019). The total global production of yam in 2019 was 74.3 million tons, and West Africa accounted for 69.8 million tons of the total production (FAOSTAT, 2021). Dioscorea is multispecies crop with ~600 species, of which 11 species are produced for food and income and the remaining ones are either wild relatives or semi-cultivated/domesticated species (Scarcelli et al., 2019). Bush yam (D. praehensilis Benth.) is one of the semi-domesticated and wild relative species, widely distributed in rainforest zones of West and Central Africa (in countries like Ghana, Nigeria, Benin, Togo, and Cameroon) (Scarcelli et al., 2019). Dioscorea praehensilis develops large tubers with high starch content (Alexis, 2013), making it ideal as food for alleviating hunger. Today, this wild yam is increasingly valued by rural people for alleviating hunger, especially during periods of food scarcity (Pitalounani et al., 2017). Dioscorea praehensilis shares many morphological, physiological, genetic, and sensory resemblances with the most recognized African Guinea yam (D. cayenensis - D. rotundata complex), being one its progenitors (Dansi et al., 1999; Scarcelli et al., 2019).
Despite these economic potentials of D. praehensilis, farmers and other end-users raised poor post-harvest tuber quality attributes such as tuber flesh oxidative enzymatic browning and postharvest tuber hardening (the inability of tuber flesh to remain soft a few days after harvesting) as some of the major reasons for abandoning D. praehensilis farming (Adewumi et al., 2021). The quality characteristics of yam cultivars are, therefore, critical for the acceptability of their cultivation and consumption. Breeding programs routinely measure tuber quality traits such as starch and sugar content, tuber flesh color, and oxidation because they impact the suitability and market penetration of improved cultivars (Arnau et al., 2016). The spontaneous change in color of harvested crops, either vegetables, fruits or roots and tubers from white or yellow to brown, black or purple is a result of polyphenol oxidation which influences the unacceptable changes in organoleptic characteristics and culinary qualities of agricultural produce (Chi et al., 2014; Graham-Acquaah et al., 2014; González et al., 2020). Polyphenol oxidase acts on phenols and converts them to quinines, resulting in dark-brown precipitates in plant produce (González et al., 2020). This oxidative browning is often associated with changes in the taste and texture (Jukanti, 2017). More than 50% of the economically significant crops is lost due to oxidative browning in tropics and sub-tropics (Jiang et al., 2015).
Lignification and cell wall thickening are major factors resulting in the tuber flesh hardening (Afoakwa and Sefa-Dedeh, 2002). The post-harvest hardening in Dioscorea spp. is divided into forward and backward reactions linked with phytate decrease and an irrevocable reaction linked with total phenol increase (Medoua and Mbofung, 2006). Its mechanism begins with phytate enzymatic hydrolysis and then migrate the released divalent cations to the cell wall, where they cross-react with demethoxylated pectins in the middle lamella. This initiates the lignification process, in which aromatic compounds accumulate on the surface of the cellular wall and react as lignification precursors (Medoua and Mbofung, 2006).
Dry matter content is a critical driver of variety adoption by producers, processors, and consumers in root and tuber crops (Sanchez et al., 2014; Bechoff et al., 2018). Yam varieties with high and moderate dry matter content (more than 30%) are frequently preferred to those with low dry matter content. Dry matter content, like post-harvest tuber quality traits, dry matter content can only be determined on mature storage roots at the end of the growing season.
However, breeding efforts have not fully understood the genetic basis of post-harvest tuber quality attributes and dry matter content in bush yam to facilitate the development of improved cultivars and limited information exists on genetic factors underlying tuber oxidative browning in D. praehensilis. These traits are controlled by quantitatively inherited genes (i.e., polygenic), and thus making the improvement of these traits difficult using conventional breeding approaches (Darkwa et al., 2020). Understanding the genetic basis of these traits variation in bush yam is crucial for developing genomic tools to enhance the selection efficiency, shortening the breeding cycle, and increasing the rate of genetic gain.
Quantitative trait loci (QTL) mapping and genome-wide association studies (GWAS) are popular methods for identifying chromosomic regions that control complex traits (Stanley et al., 2021). GWAS is an effective method for detecting genomic regions associated with important complex quantitative traits and predicting or identifying causative genes (Brachi et al., 2011). The application of the GWAS method has been reported in other yam species. Candidate genes linked to tuber yield and YMV severity have been reported in white yam (Agre et al., 2021). Mondo et al. (2021) also used GWAS to detect genomic regions linked to sex determination and cross-compatibility traits in D. alata. GWAS has also been employed to identify candidate genes associated with oxidative browning and dry matter content in greater yam (Gatarira et al., 2020). The GWAS has also been successful in cassava, another root and tuber crop, in detecting key tuber quality traits: waxy starch (do Carmo et al., 2020), provitamin A carotenoid content (Esuma et al., 2016), dry matter content and total carotenoid (Rabbi et al., 2017). No report exists on using GWAS to identify the genetic mechanisms controlling tuber quality attributes in D. praehensilis.
The current study aimed to identify genomic regions associated with post-harvest tuber quality attributes in a panel of bush yam accessions.
2 Materials and methods
2.1 Genetic materials and experimental site
The GWAS panel used for this study comprised 162 D. praehensilis accessions, of which 71 were collected from the Central region, 25 from the Eastern region, and 66 from the Western North region. Agronomic and tuber quality traits of these accessions are presented in Supplementary Table S1.
The accessions were grown for two seasons, 2020 and 2021, at the Teaching and Research Farm, School of Agriculture, University of Cape Coast, Ghana (5°07´7.6´´N, 1°17´18.9´´W; 15 m above sea level) located in the central region of Ghana with semi-deciduous forest and coastal savannah ecological zones.
2.2 Phenotyping
Phenotypic data were collected on dry matter content, tuber flesh hardness, and tuber flesh oxidation using the yam standard operating protocols (Asfaw, 2016).
Dry matter content was estimated from each accession by sampling pest and disease-free tubers from the replications. Tubers from each accession were washed with running water to remove debris and soil particles. The tuber skin was peeled off, and tuber flesh was grated to smaller sizes to facilitate oven drying. About 100g of grated tuber flesh from each accession was collected into rectangular-shaped aluminum foil bags and dried in an oven at 105°C for 24 hrs. Percentage dry matter content was estimated for each genotype as follows in Equation 1:
Tuber flesh oxidative browning was evaluated by sampling disease and insect-free tubers from each genotype per replicate. These tubers were also washed under running water, air-dried, and the skin was peeled off. The peeled flesh tuber was cut into three portions (head, middle, and tail), and the middle portion was chopped to get small tuber flesh of 5 cm diameter and 0.5 mm thickness 24. Hunter parameters (L*, a*, b*) were used to measure the color of small tuber flesh (5 cm diameter, 0.5 mm thickness) using a potable chromometer or colorimeter (CHN Spec, CS-10, Baoshishan, China) immediately the surface was cut and exposed to air (0 min) and 60 min after the cut surface was exposed to air. The brightness coordinate L* is used to measure the whiteness of a sample ranging from black (0) and white (100), a* coordinate is a redness (positive value) or greenness (negative value), and b* coordinate represents the yellowness (positive value) or blueness (negative value) (Abano et al., 2012). White and black tiles were used to calibrate the colorimeter before each measurement. The color change () was estimated using the formula in Equation 2:
Where is total color change, is the change between white and black, is the change between red and green, while is the change between yellow and blue.
Oxidative browning was calculated using the formula in Equation 3:
Where is the initial color change, while is the final color change.
The procedure employed by Siadjeu et al. (2016) with slight modification was used in assessing the postharvest hardening of bush yam (D. praehensilis) accessions. The tuber flesh samples of 5 cm diameter and 1 cm thickness from each accession in each replicate were assessed for tuber flesh hardening using a digital penetrometer at a 6.00 mm probe. Three measurements were taken from each accession in each replicate, and the averages were calculated and expressed in Newton.
2.3 Genotyping
DNA samples were extracted for each accession using the LGC oKtopure™ automated high-throughput ‘sbeadex™’ DNA extraction and purification system (https://www.biosearchtech.com/), which is frequently used at Intertek-AgriTech (http://www.intertek.com/agriculture/agritech/). The ‘sbeadex™’ technology prepares nucleic acids using magnetic separation. The first stage in this process is to homogenize leaf tissue samples in 96 deep-well plates using steel bead grinding. LGC’s plant DNA preparation ‘sbeadex™’ kit (https://www.biosearchtech.com/) was used to incubate the ground tissue with a DNA extraction buffer. Finally, super-paramagnetic particles coated with ‘sbeadexTM’ surface chemistry absorb nucleic acids from a sample and are used to purify extracted DNA. Purified DNA is eluted and used in downstream operations.
According to Kilian et al. (2016) high-throughput genotyping was carried out using the 96-plex DArTseq methodology, and SNPs were called using the DArT’s proprietary software, DArTSoft. [20]. Reads and tags found in each sequencing result were aligned to the D. rotundata reference genome v2 (https://drive.google.com/drive/folders/1H5T4xjKAEl9LliR-4qK_IR6TypCDe8nj) with Hisat2 (Kim et al., 2015; Sugihara et al., 2020. The raw HapMap file generated was first converted to a Variant Call Format (VCF) using KDcompute (https://kdcompute.seqart.net/kdcompute). SNP-derived markers were filtered to remove unwanted SNP markers for quality control using the software PLINK 1.9 and VCFtools. Markers and 24 accessions with more than 20% missing data were removed. Rare SNPs with 5% minor allele frequencies and low coverage read depth (<5) were also eliminated. In the end, only 4,525 informative SNP markers and 138 D. praehensilis accessions were used for the subsequent association analysis.
2.4 Statistical analyses
Only 138 of the 162 accessions investigated in the study provided both phenotypic and genotypic data, which were included in future data analysis.
2.4.1 Phenotypic data analysis
Analysis of variance combined across the two growing seasons using lme4 package in R (R Development Core Team, 2019) was computed for collected post-harvest tuber quality traits and dry matter content based on a linear mixed model (LMM) analysis with restricted maximum likelihood procedure in R. The linear model used was as follows in Equation 4:
Where Yijk = value of the observed quantitative trait; μ = population mean; Gh = effect of the hth accession; Si = effect of the ith growing season; (Gh × Si) is the accessions × season interaction associated with accession h and season i; Rij = effect of the jth replicate (superblock) in seasons ith; Bk = effect of the kth incomplete block within the jth replicate; and εhijk = experimental error. In this analysis, accessions were considered fixed while all other factors were random. The variations in the quantitative traits of D. praehensilis accessions were assessed using descriptive statistics such as means, standard deviations, minimum and maximum values and coefficients of variation. Pearson’s correlation coefficients in corrplot package (R Development Core Team, 2019) were used to assess relationships among the evaluated traits. The lme4 package in R (R Development Core Team, 2019) was used to generate the best linear unbiased expectation (BLUE), and the variance components. The broad-sense heritability was calculated based on the estimated variance components as follows in Equation 5:
Where = genotypic variance, = phenotypic variance and n = number of observation.
2.4.2 Population structure analysis
To explore the genetic relationship among the bush yam accessions, principal component analysis (PCA) was conducted using factorMiner package in R (R Development Core Team, 2019). The phylogenetic tree was generated using phangorn R package (Bates, 2010). The population stratification among the bush yam accessions was assessed using Admixture method in R package ‘adegenet (Jombart et al., 2010). After varying the number of clusters from 1 to 40, by employing cross validation using the Bayesian Information Criterion (BIC). The optimal number of clusters was determined using k-means analysis. Accessions with membership probabilities (MP) ≥50% were assigned to genetic groups by admixture. While accessions with MP<50% were considered as admixt (Salazar et al., 2017).
2.4.3 Genome wide association study analysis
The GWAS analysis was conducted using the multi-locus models implemented in marl v4.0.2 (Zhang et al., 2020) with six genetic models. These models included: multi-locus random-SNP-effect Mixed Linear Model (Wang et al., 2016), fast multi-locus random-SNP-effect EMMA (FASTmrEMMA) (Yang-Jun et al., 2017), polygenic-background-control- based least angle regression plus empirical Bayes (pLARmEB) (Zhang et al., 2017), fast mrMLM (FASTmrMLM) (Tamba and Zhang, 2018) and pKWmEB (Ren et al., 2018). To account for the genetic error and to avoid the false discovery we made use of the Kinship matrix (K) and the population structure (Q) as covariate.
The negative logarithms (-log10) of the p-values were plotted against their expected p-values to generate quantile–quantile (Q–Q) plots, which fit the appropriateness of the GWAS model with the null hypothesis of no association and to determine how well the model accounted for population structure. Adjusted false discovery rate (FDR) was used to decide the limit of detection (LOD) score to reduce false positive QTNs and it was set to 3 cut-off point for QTNs in all measured traits.
2.4.4 Identification of putative genes
The Generic File Format (GFF3) file was used to search for probable candidate genes within the relevant genomic domain (downstream and upstream) at a specific range window of 1 MB at 500 kb. The LD heatmap package (Shin et al., 2006) was utilized to conduct LD analysis and generate a visual representation in the form of a heatmap, illustrating the pairwise LD measurements among SNPs showing significant associations with individual traits. The estimation of pairwise LD estimates between chromosomes for markers displaying significant associations was carried out, and the plotting was performed based on base pair (bp) distance utilizing the “ggplot2” package in R (R Development Core Team, 2019). The yam generic feature format (GFF3) of the reference genome was used to identify the main gene in the inter-genic region using the SNPReff. The European Molecular Biology Laboratory-European Bioinformatics Institute (EMBL-EBI) public database Interpro was utilized to determine the functions of the genes associated with discovered SNPs (Hunter et al., 2012).
2.4.5 Haplotype estimation and SNP markers effect prediction for stable SNPs
Haplotype associated with significant QTN was developed using “ggsignif and ggpubr” packages implemented in R (Yin, 2019), and the sequence of each haplotype was defined based on the 138 genetic materials considered as testing and or identification population. The variant effect prediction was evaluated through the adjusted posterior probability, and the markers with high segregation were identified. Marker effects were then plotted for visualization using ggplot2 in R (R Development Core Team, 2019).
3 Results
3.1 Phenotypic traits variation and correlation among the post-harvest traits
Estimate of variance components, coefficients of variation and means, minimum and maximum values for post-harvest tuber quality attributes among bush yam accessions are presented in Table 1. The variance estimates for the genotype effect were significant (p< 0.05) for the three evaluated traits. In contrast, the season and genotype by season interaction effects were significant (p< 0.05) for only tuber flesh hardness (Table 1).
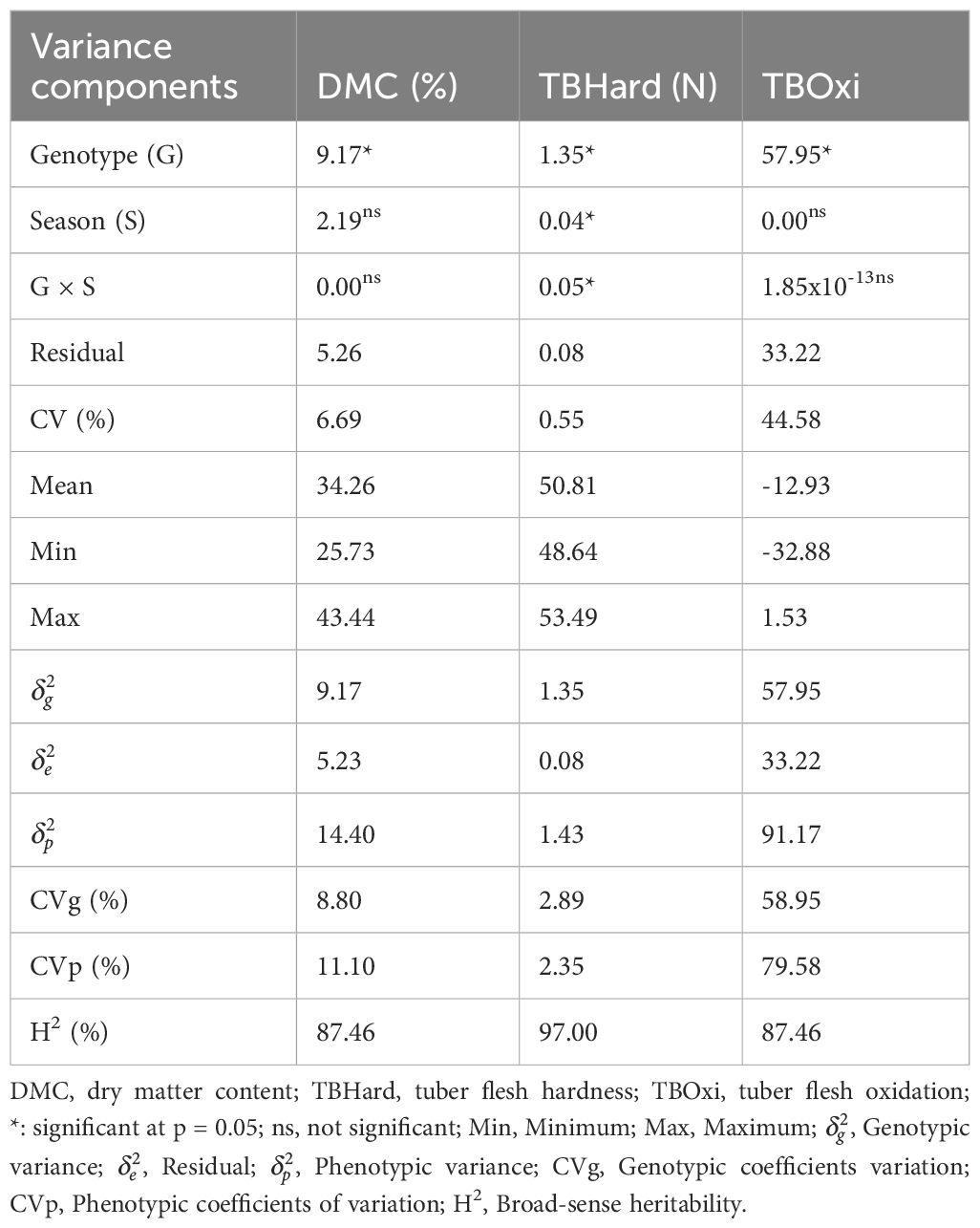
Table 1 Estimate of variance components, coefficients of variation, means, and minimum and maximum values and genetic parameters of dry matter contents and tuber quality traits in D. praehensilis germplasm.
The coefficients of variation ranged from 0.55% for tuber flesh hardness to 44.58% for tuber flesh oxidation. Dry matter content ranged from 25.73 to 43.44%, with an average of 34.28%, tuber flesh hardness recorded a mean value (50.86 N) with a range of 48.64 to 53.49N and tuber flesh oxidation varied from -32.88 to 1.53, with an average of -12.93 (Table 1).
High broad-sense heritability (>60%) was recorded for the evaluated post-harvest tuber quality traits, with a range of 87.46% for dry matter contents and tuber flesh oxidation, respectively to 97.00% for tuber flesh hardness (Table 1). The genotypic coefficients of variation ranged from 2.89% for tuber flesh hardness to 58.95% for tuber flesh oxidation, while phenotypic coefficients of variation varied from 2.35% for tuber flesh hardness to 79.58% for tuber flesh oxidation.
Tuber dry matter content showed a positive relationship with tuber flesh oxidation (r = 0.25; p< 0.01), but showed negative correlations with tuber flesh hardness (r = -0.23; p< 0.01) (Supplementary Figure S1). Tuber flesh oxidation revealed a significant negative correlation with tuber flesh hardness (r = -0.40; p< 0.001).
3.2 Population structure
Through the Bayesian Information Criteria (BIC), a rapid elbow was observed at K=3 and was used as the maximum number of clusters to group the 138 bush yam accessions into 3 sub-populations (Figure 1). Using 50% membership probability threshold, 133 accessions were assigned to the three different sub-populations. The remaining five accessions with a membership probability of less than 50% were designated as admixt (Supplementary Table S2). Sub-population 1 has the highest proportion of accessions (60%), followed by sub-population 2 (~24%) and the least was sub-population 3 (~12%) (Figure 1). Sub-population 1 was represented mainly by the accessions from the Central region, while those members in sub-populations 2 and 3 are majorly from Western North region of collection (Supplementary Table S2). The phylogenetic tree also revealed three clusters (Figure 2). The size of the clusters varied across the identified groups. The accessions from Western North region had highest members in cluster 1 (~94%) and cluster 2 (~66%), while accessions from Central region had highest members in cluster 3 (~56%). The accessions from Western North regions are mostly grouped together in cluster 1 since they are closely similar to one another. The principal component analysis (PCA) explained 71.1% of the total variation on the first two component and the accessions were not grouped based on origin of collection (Figure 3). The PCA plot revealed some level of variation among the accessions from each region, but some of the accessions are closely related to one another, especially accessions from central and Eastern regions (Figure 3).
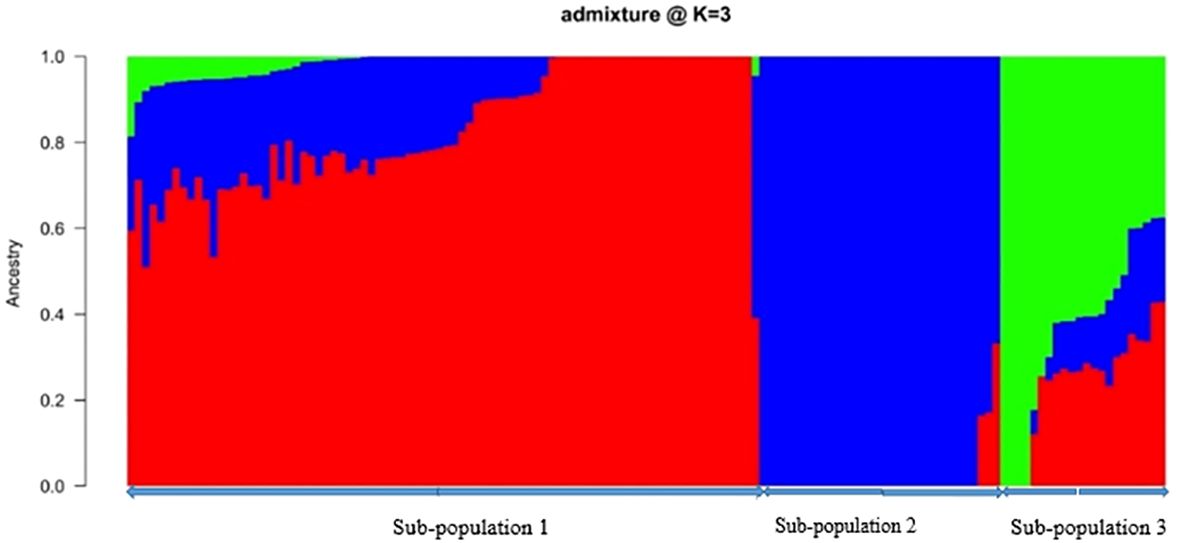
Figure 1 Population structure plot of 138 bush yam accessions (k = 3 using 4,525 SNP markers. Each color represents a region in a sub-population. The colors represent regions of collection: Central (red), Western North (blue), Eastern (green) based on a membership coefficient of ≥ 50%.
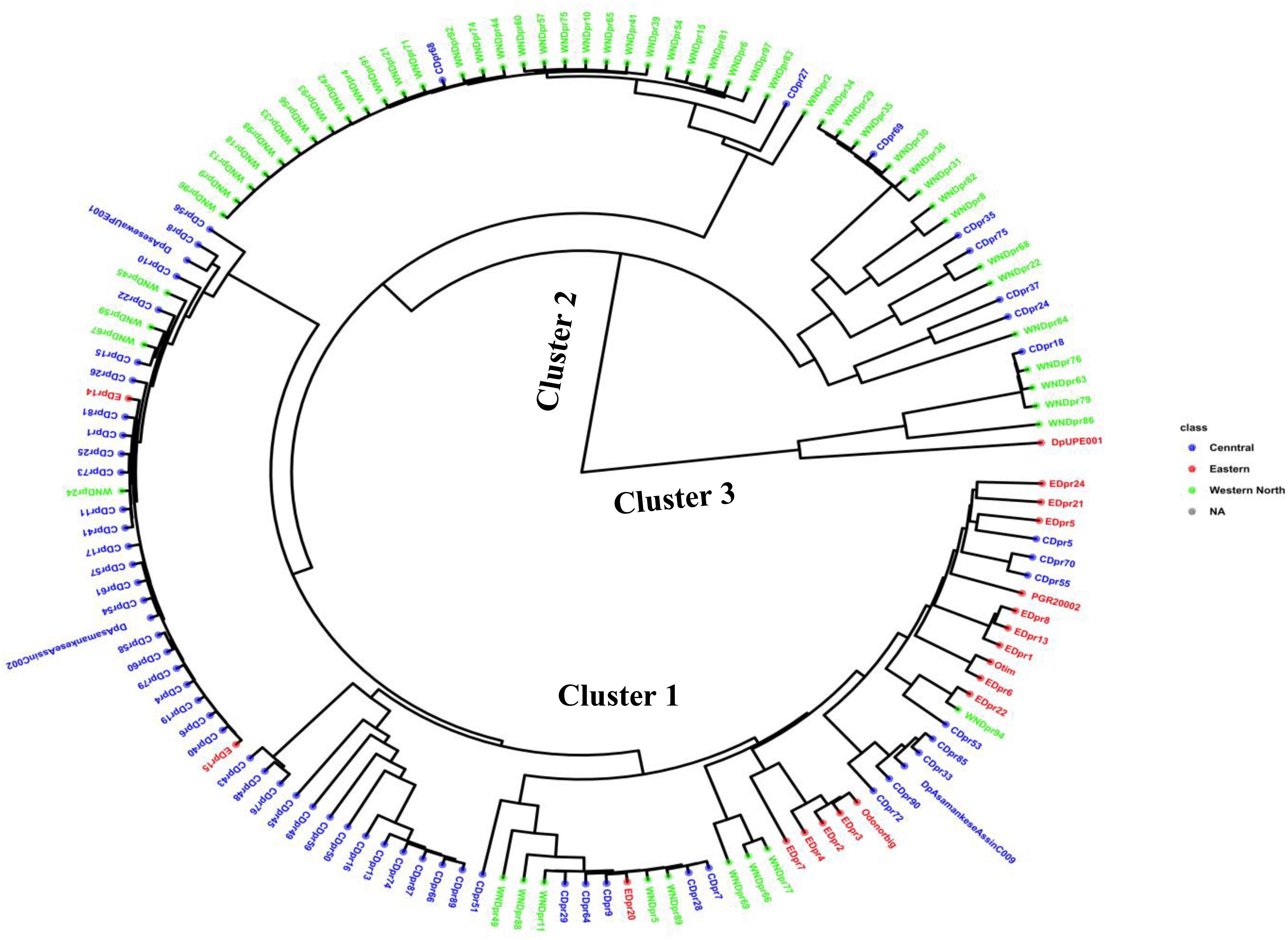
Figure 2 Phylogenetic tree showing the genetic relationship among 138 accessions using 4,525 SNP markers based on Ward2 method.
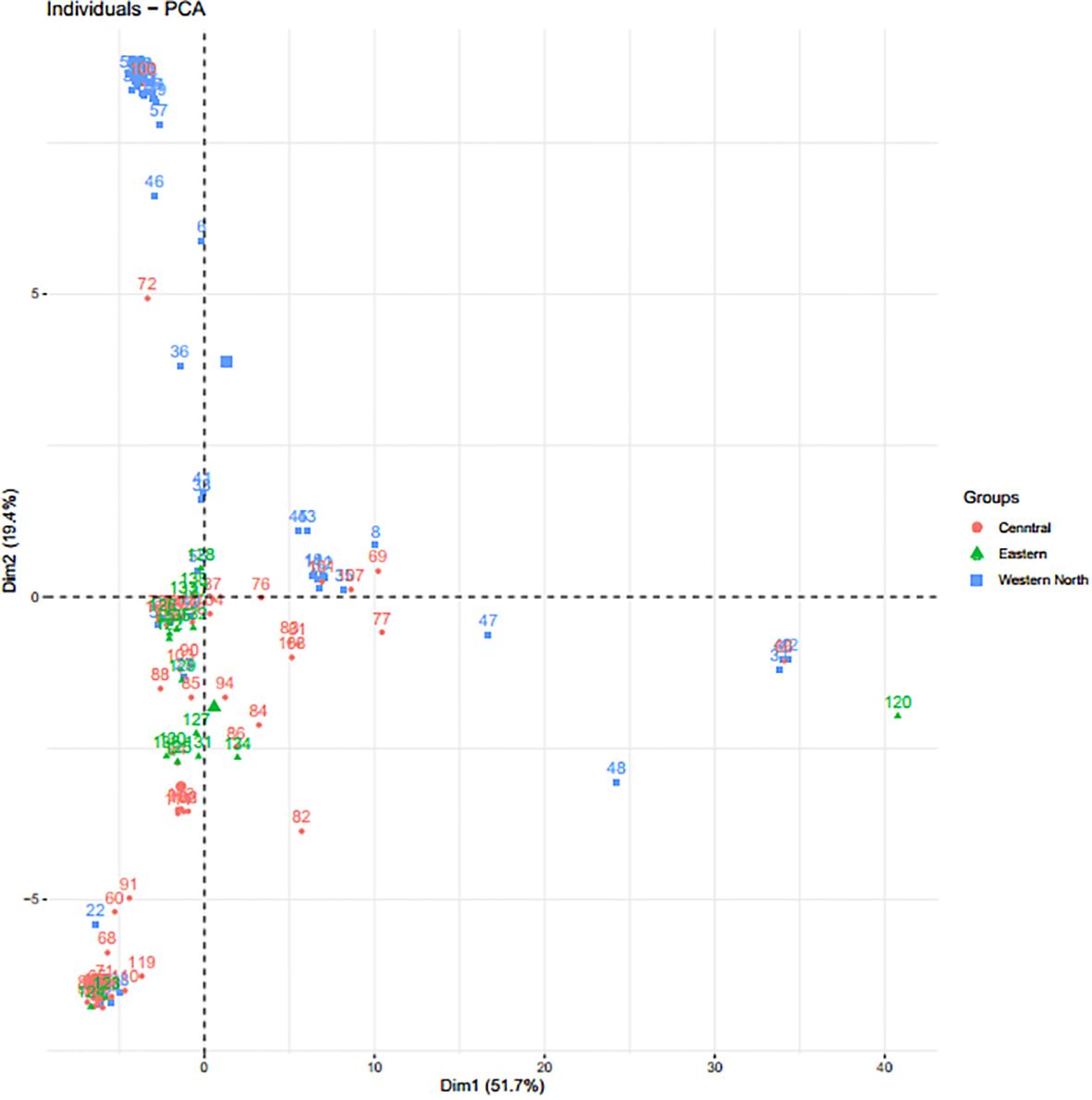
Figure 3 Principal component analysis based on 133 bush yam accessions using 4,525 SNP markers. Each color represents the region of collection, and each dot represents the individual within the region of collection.
3.3 Genome-wide association scan for post-harvest tuber quality traits
3.3.1 GWAS multi-locus models for QTN detection
A total of 16 significant SNPs associated with post-harvest tuber quality traits of D. praehensilis were detected using five multiple-locus GWAS models FASTmrEMMA, FASTmrMLM, mrMLM, pKWmEB and pLARmEB, across 20 chromosomes. In season 2020, for DMC, FASTmrEMMA model detected one SNP, while three of the models (FASTmrMLM, mrMLM and pLARmEB) detected SNP chrom_9_179515. For TBHard, SNP chrom_14_3890023 identified on chromosome 14 was detected by model pKWmEB. For TBOXI, SNP chrom_19_30094257 was detected by models FASTmrMLM, mrMLM and pLARmEB, chrom_19_30908452 was detected by FASTmrMLM and mrMLM, while chrom_03_16800771 and chrom_17_884171 were detected by models FASTmrEMMA and pLARmEB, respectively (Table 2). In season 2021, for DMC, two SNPs were detected each by mrMLM and pLARmEB and one SNP by FASTmrEMMA. For TBHard, 2 SNP markers chrom_19_2992038 and chrom_19_29680098 located on chromosome 19 were detected by each of FASTmrMLM and mrMLM models. For TBOXI, each of the identified SNPs were detected by two different models each, except chrom_16_1769569, which was detected by model FASTmrEMMA (Table 2).
3.3.2 GWAS scan for DMC
Marker-trait association analysis conducted using the 2020 season data set detected two SNPs significantly associated with DMC on chromosomes 4 and 19, with SNPs chrom_04_5165938 and chrom_19_179515 accounted for total phenotypic variations of 12.85 and 6.48%, respectively, (Table 2; Figure 4A). Using 2021 season phenotypic data set, five SNP markers were significantly linked with DMC and were located chromosomes 2, 3, 5, 7 and 17 with estimated total phenotypic variation ranging from chrom_05_245603 (0.00%) to chrom_02_21104405 (14.01%) (Table 2; Figure 4B). The marker-trait association analysis combining data sets from 2020 and 2021 seasons identified one significant SNP marker (chrom_07_4136960) on chromosome 7 associated with DMC with average LOD score and MAF values of 4.13 and 0.30, respectively, with average estimated total phenotypic variation of 9.18% (Table 2; Figure 4C). Of the seven SNPs that were associated with DMC, only SNP chrom_07_4136960 showed stability across the seasons as it was detected in season 2021 as well combined analysis for seasons 2021 and 2022 (Table 2).
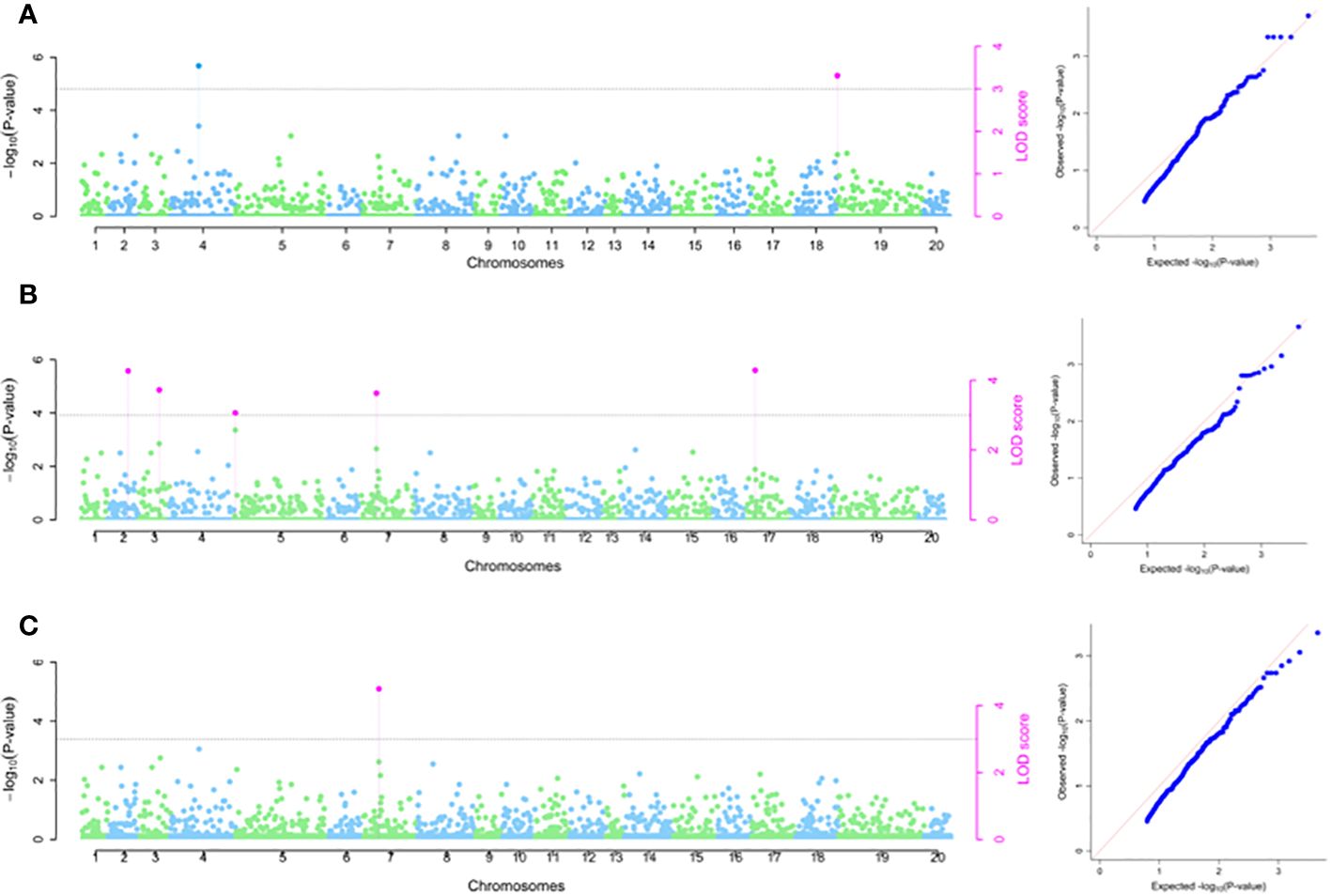
Figure 4 SNP markers identified to be associated with dry matter content. (A) 2020 phenotypic data; (B) 2021phenotypic data and (C) Combined 2020 and 2021 phenotypic data. The graph refers to the Manhattan and the quantile–quantile (Q-Q) plot.
3.3.3 GWAS scan for TBhard
Based on 2020 phenotypic data, one SNP marker (chrom_14_3890023) located on chromosome 14 was significantly associated with TBHard and accounted for total phenotypic variation of 12.13% and LOD score of 3.23 (Table 2; Figure 5A). For 2021 season, GWAS revealed two significant SNP markers on chromosome 19 with estimated average total phenotypic variations of 7.54% for SNP chrom_19_2992038 and 11.88% for SNP chrom_19_29680098 (Table 2; Figure 5B). For combined analysis of data sets from 2020 and 2021 seasons, two significant SNP markers located on chromosome 19 were identified with average estimated total phenotypic variations of 6.97% for SNP chrom_19_2992038 and 14.53% for SNP chrom_19_29680098, respectively (Table 2; Figure 5C). Of the three SNPs that were identified to be associated with TBHard, two SNPs chrom_19_2992038 and chrom_19_29680098 showed stability across the seasons as they were detected in season 2021 as well combined analysis for seasons 2021 and 2022 (Table 2).
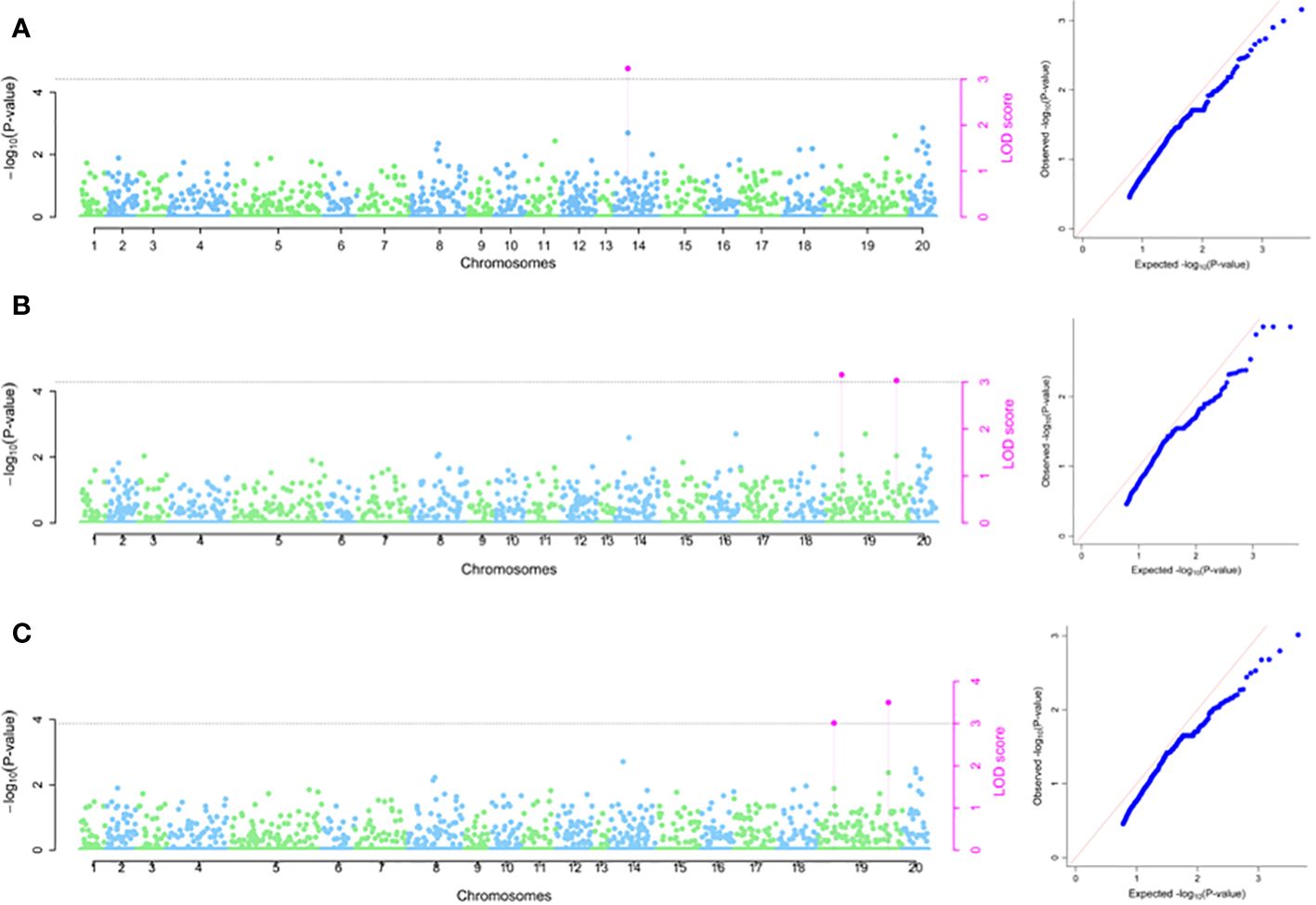
Figure 5 SNP markers associated with tuber flesh hardness (A) 2020 phenotypic data; (B) 2021 phenotypic data and (C) Combined 2020 and 2021 phenotypic data. The graph refers to the Manhattan and the quantile–quantile (Q-Q) plot.
3.3.4 GWAS scan for TBOXI
Using 2020 phenotypic data set, four SNP markers were identified on chromosomes 3, 17 and 19, with LOD scores ranging from chrom_19_30094257 (3.19) to chrom_03_16800771 (4.94) (Table 2; Figure 6A). The estimated phenotypic variation accounted for in tuber flesh oxidation ranged from SNP chrom_19_30908452 (4.25%) to SNP chrom_03_16800771 (13.97%) (Table 2). For 2021 phenotypic data set, three SNP markers were detected on chromosomes 16, 17 and 19 with estimated phenotypic variations varied from chrom_17_19182614 (4.03%) to chrom_19_30094257 (15.61%) (Table 2; Figure 6B). Using combined phenotypic data sets from 2020 and 2021 seasons, five significant SNP markers on chromosomes 3, 16, 17 and 19 were detected with estimated phenotypic variation ranged from 4.51% for SNP chrom_17_19182614 to 16.92% for SNP chrom_03_16800771 (Table 2; Figure 6C). Five SNPs showed stability for TBOXI as they were detected across the seasons as well as detected in the combined analysis for seasons 2020 and 2021 (Table 2).
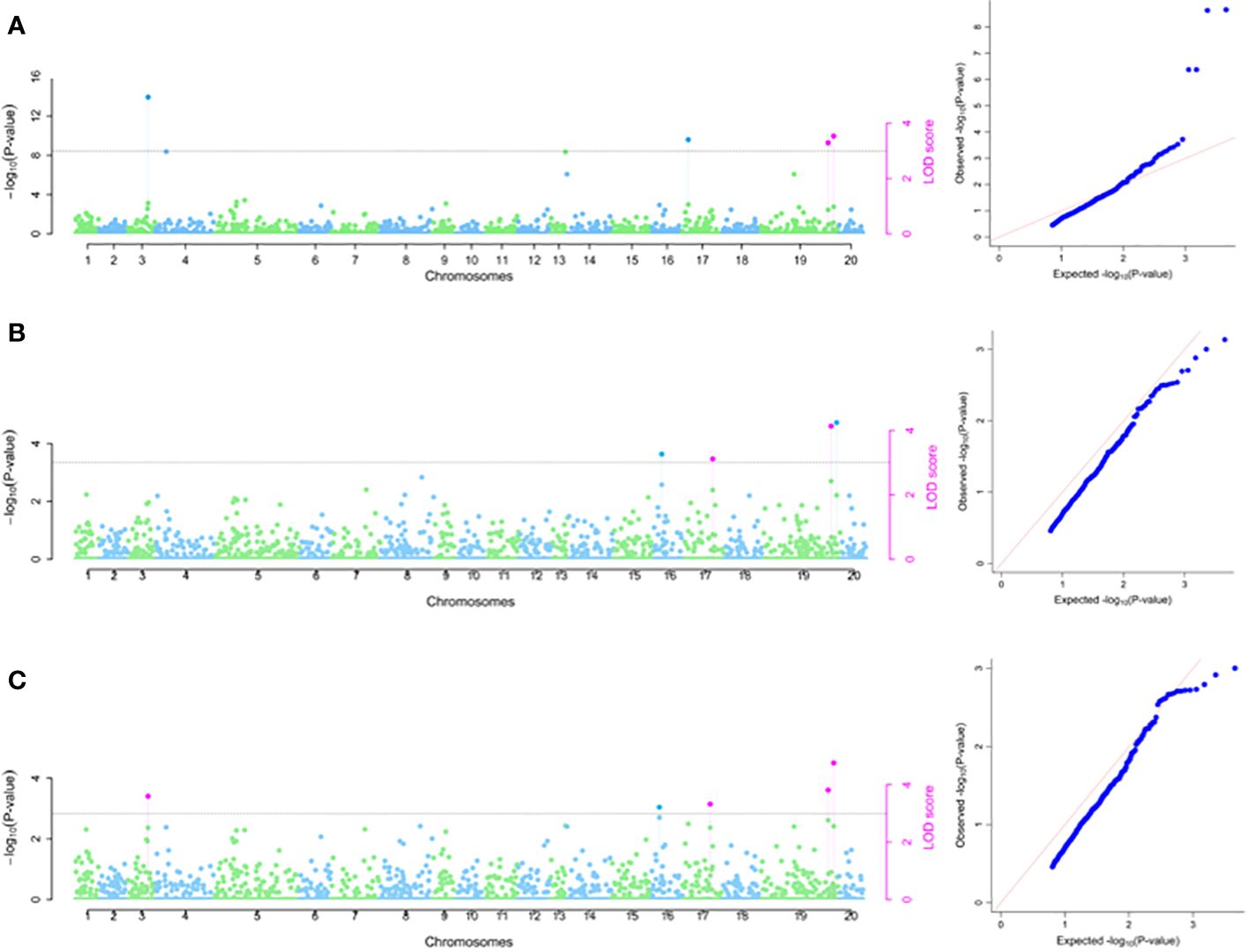
Figure 6 SNP markers associated with tuber flesh oxidation. (A) using 2020 phenotypic data (B) using 2021 phenotypic data and (C) combined 2020 and 2021 phenotypic data. The graph refers to the Manhattan and the quantile–quantile (Q-Q) plot.
3.4 Identification of putative genes associated with post-harvest quality traits in D. praehensilis
Through gene annotation, seven (7) putative genes belonging to glycoside hydrolase gene family were identified on SNP markers associated with DMC, and these genes were distributed on chromosome 7 (Table 3; Figure 7A). For tuber flesh oxidation, we identified 13 putative genes, and the most promising are serine/threonine-protein kinase genes, tetratricopeptide-like genes, leucine-rich repeat genes, glycoside hydrolase genes, six-hairpin glycosidase-like genes, P-loop containing nucleoside triphosphate hydrolase genes, Alpha/beta hydrolase fold-1 genes, tudor domain, Leucine-rich repeat-containing N-terminal, type 2, and glycoside hydrolase, family 9, active site. (Table 3; Figure 7C). On the other hand, five (5) candidate genes (ATPase, AAA-type, core, P-loop containing nucleoside triphosphate hydrolase, AAA+ ATPase domain, F-box domain and Leucine-rich repeat, cysteine-containing subtype) were identified near peak of the significant SNP associated with tuber flesh hardness (Table 3; Figure 7B).
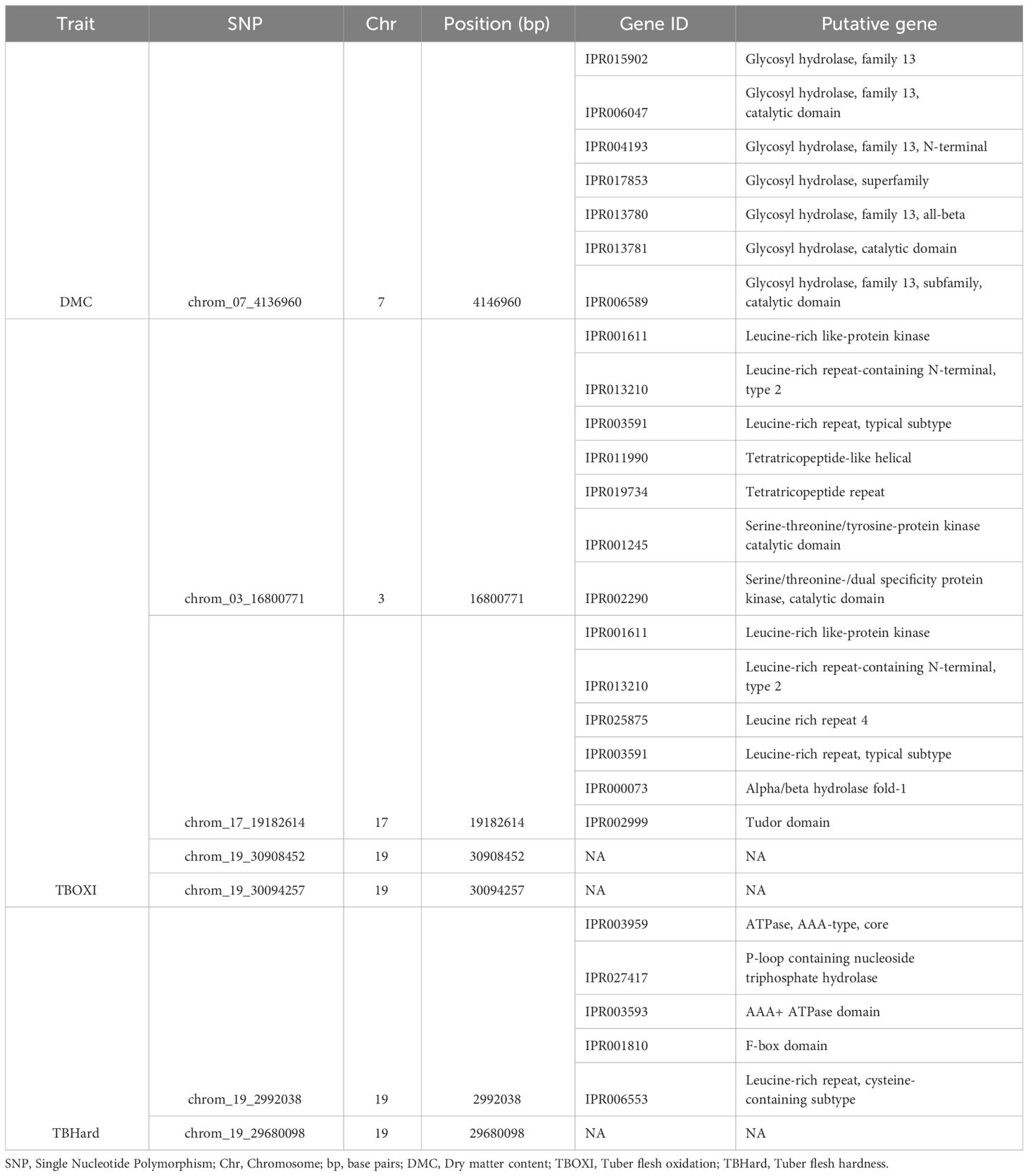
Table 3 Candidate genes within chromosomic regions associated with post-harvest tuber quality traits.
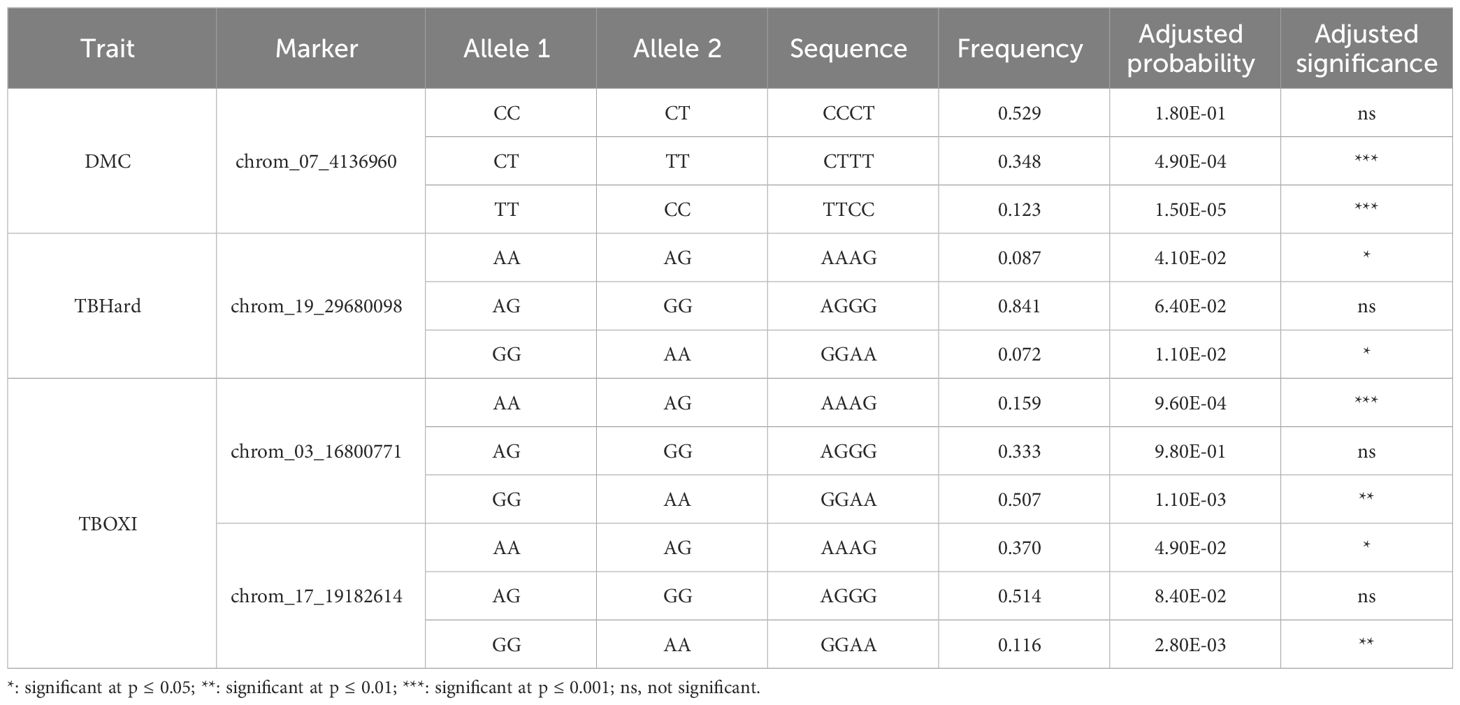
Table 4 Allele frequencies and marker effects of stable SNPs associated with post-harvest tuber quality traits.
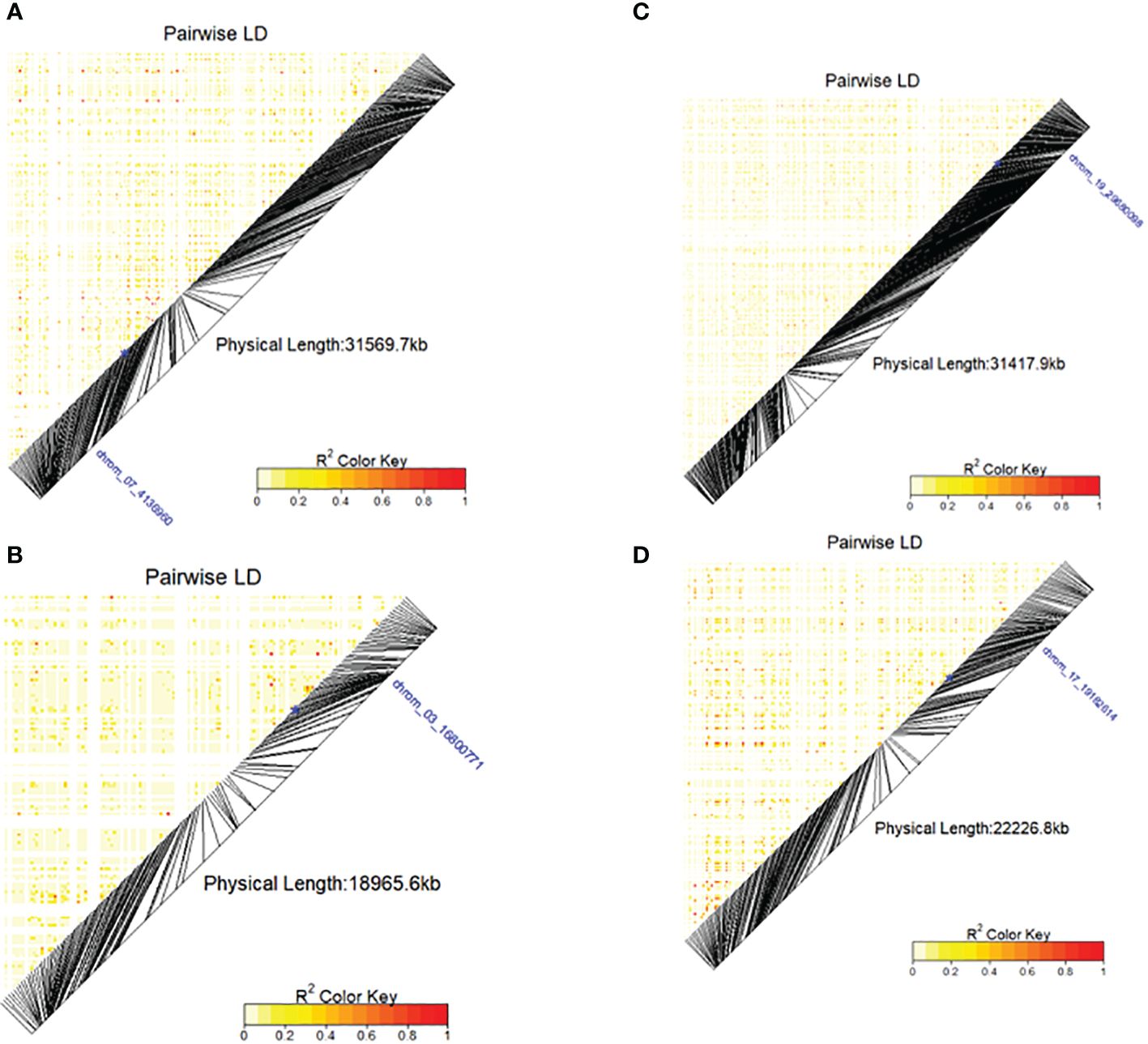
Figure 7 Heatmap LD haplotype blocks for different SNP markers located on different chromosomes associated with DMC, TBHard and TBOXI. (A) SNP chrom_07_4136960 was associated with the DMC, (B) chrom_19_2992038 associated with the TBHard, (C) SNP chrom_03_16800771 and (D) SNP chrom_17_19182614 associated with the TBOXI. The R2 color key indicates the degree of significant association with the putative genes.
3.5 Haplotype segregation frequencies and SNP markers effects
The frequencies and marker prediction effects of various alleles associated with post-harvest tuber quality traits of D. praehensilis are presented in Table 4 and Figure 8. The only stable SNP chrom_07_4136960 for DMC showed high significant allele segregation among the haplotypes. This stable SNP detected alleles TT and CT to be associated with high dry matter content, while allele CC was identified to be associated with low dry matter content (Table 4; Figure 8). For tuber flesh hardness, SNP chrom_19_29680098 of the two stable SNPs displayed highly significant segregation among the alleles (Table 4; Figure 8). Allele AA was detected to significantly associate with low tuber flesh hardness, while allele GG was linked to high tuber flesh hardness (Table 4; Figure 8). Of the four stable SNPs identified with TBOXI, two SNPs (chrom_03_16800771 and chrom_17_19182614) revealed highly significant allele segregation (Table 4; Figure 7). The two SNP markers on Chromosomes 3 and 17 identified haplotypes AA and AG to be linked with lower tuber flesh oxidation and allele GG to be linked with higher tuber flesh oxidation (Table 4; Figure 8).
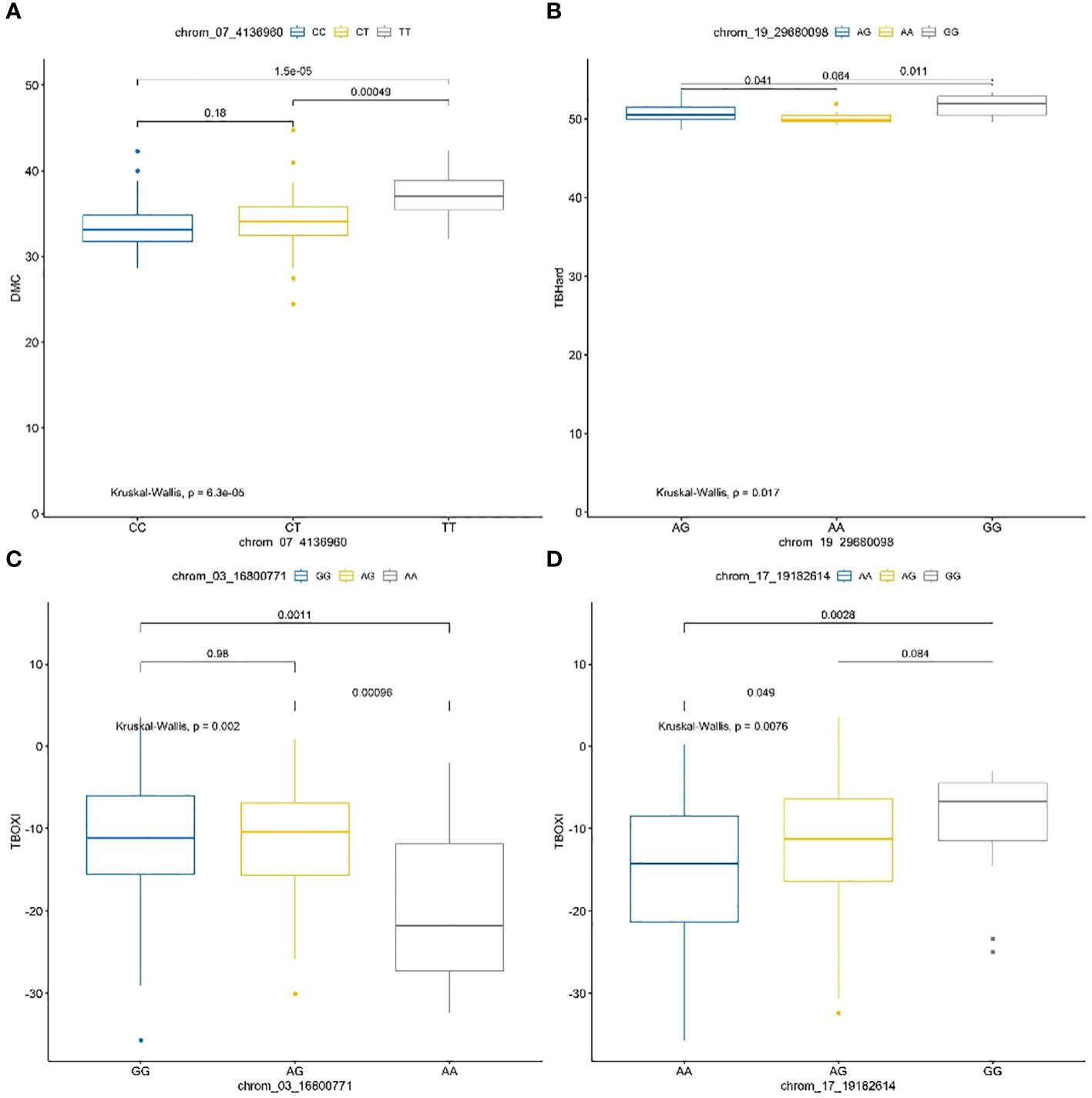
Figure 8 Haplotype prediction effects of significant SNPs associated with dry matter content (A), tuber flesh hardness (B) and tuber flesh oxidation (C, D).
4 Discussion
In this study, we evaluated the performance of 138 bush yam collected from Ghana and profiled for tuber quality. Through the phenotypic evaluation, we obtained high broad sense heritability (≥60%) for the three evaluated traits, explaining the predominant role of genetic factors in these traits. The high heritability observed in this study make selection for these post-harvest quality traits possible in yam breeding program. High heritability traits improve the sensitivity of detecting SNPs in an association panel, allowing the identification of a true association between a marker and a putative gene (Brachi et al., 2011; Agre et al., 2021). In a previous study, and in a similar QTL mapping, Arnau et al., 2023 reported as well high heritability for DMC in Dioscorea alata population.
The population structure, principal component analysis and phylogenic tree grouped the bush yam accessions used in this study into three subpopulations, suggesting variation among the evaluated germplasm. The considerable genetic diversity suggests that the genotypes tested have the potential for genetic improvement in terms of dry matter content, tuber flesh oxidation, and tuber flesh hardness. Agre et al. (2021) and Stanley et al. (2021) have also reported the importance of population structure, and the kinship in preventing false discovery in GWAS analysis.
Genome-wide association studies have been frequently employed to identify the genetic basis of complex characteristics (Morris et al., 2013; Sukumaran et al., 2018). However, complex genetic structures and structured features might cause erroneous signals and indirect correlations in genome-wide association studies.
This study used five MLM models to detect genomic regions linked with post-harvest tuber quality traits (DMC, TBOXI and TBHard) in D. praehensilis germplasm. Of the 16 SNPs associated with DMC, TBOXI and TBHard, FASTmrEMMA detected 4 SNPs, FASTmrMLM detected 8 SNPs, mrMLM detected 8 SNPs, pLARmEB detected 5 SNPs, while pKWmEB detected 3 SNPs (Table 2). This suggests different levels of detection for each model. The MLMs utilized in this research identified potential candidate genes for the traits under investigation, highlighting their effectiveness in GWAS. These outcomes reinforce the notion that MLMs are valuable in pinpointing QTNs and candidate genes in plant species (Karikari et al., 2020; Agre et al., 2021). The results of this investigation established an association between post-harvest tuber quality traits and single nucleotide polymorphisms.
Identifying QTL and genes that influence bush yam’s post-harvest tuber quality characteristics is critical for its improvement and marker-assisted breeding. The GWAS study revealed the genetic basis of post-harvest tuber quality traits in D. praehensilis. A whole-genome scan for phenotypic and allelic variation in post-harvest tuber quality traits discovered genome regions with significant -log10 values on nine chromosomes (chromosomes 2, 3, 4, 5, 7, 14, 16, 17, and 19). Many agronomic and tuber quality attributes have been studied using genome-wide association mapping in Dioscorea spp. These traits include tuber dry matter, tuber flesh color and oxidative browning in water yam (Gatarira et al., 2020; Dossa et al., 2023), sex determination and cross-compatibility in water yam (Mondo et al., 2021), and tuber yield, tuber quality traits, yam mosaic virus resistance and sex determination and cross compatibility in white yam (Agre et al., 2021, 2023; Asfaw et al., 2022). This study identified seven significant markers for DMC on chromosomes 2, 3, 4, 5, 7, 17 and 19 and six significant marker for tuber flesh oxidation on chromosomes 3, 16, 17 and 19 (Table 2), which differed from SNPs identified by Gatarira et al. (2020) on the two traits. There were no similar genomic regions detected, but additional SNP markers associated with dry matter content and tuber flesh oxidation were detected. The significant markers co-located with candidate genes for the evaluated traits could be essential for developing functional markers that would be useful for marker-assisted selection to enhance the development of D. praehensilis for post-harvest tuber quality traits. Detection of significant marker-trait association using GWAS has been reported in some yam species such as D. rotundata (Agre et al., 2021, 2023) and D. alata (Gatarira et al., 2020; Mondo et al., 2021; Dossa et al., 2023) and other root and tuber crops such as cassava (Uchendu et al., 2021).
This study detected putative candidate genes within the genomic regions of the target traits. A total of 24 putative candidate genes were identified upstream, and downstream SNP association with dry matter content, tuber flesh oxidation, and tuber hardness.
The seven candidate genes or protein families linked with dry matter content (Table 3), are mostly Glycoside hydrolase, family 13. Glycoside hydrolase family has also been reported to play significant role in dry matter content of water yam (Gatarira et al., 2020; Arnau et al., 2023). The glycoside hydrolase family 13 is a family of glycoside hydrolases, which are a widespread group of enzymes that hydrolyze the glycosidic bond between two or more carbohydrates, or between a carbohydrate and a non-carbohydrate moiety (Ryttersgaard et al., 2002). Glycoside Hydrolase Family 13 is engaged in a variety of enzymatic metabolisms of carbohydrate molecules found in numerous plant tissues (Minic, 2008).
Tuber flesh oxidation is a vital post-harvest tuber quality trait hindering the marketability of bush yam. Polyphenol oxidation has been reported in the previous studies to be majorly attributed to tuber flesh oxidation (Rinaldo et al., 2022). Dossa et al. (2023) and Gatarira et al. (2020) identified several genes associated with tuber flesh oxidation on chromosomes 5 and 2, respectively, while the present study identified twelve putative candidate genes on chromosomes 3, 7, 16, 17 and 19. The report from this study corroborates the study of Ehounou et al. (2022) who identified QTLs associated with tuber flesh oxidation on chromosomes 16 and 19. The most important of these genes are Leucine-rich repeat (LRR) families. Leucine-rich repeats (LRR) consist of 2-45 motifs of 20-30 amino acids in length that generally folds into an arc or horseshoe shape (Enkhbayar et al., 2004). LRRs occur in proteins ranging from viruses to eukaryotes, and appear to provide a structural framework for the formation of protein-protein interactions (Kobe and Kajava, 2001). Proteins containing LRRs include tyrosine kinase receptors, cell-adhesion molecules, virulence factors, and extracellular matrix-binding glycoproteins, and are involved in a variety of biological processes, including signal transduction, cell adhesion, DNA repair, recombination, transcription, RNA processing, disease resistance, apoptosis, and the immune response (Ng and Xavier, 2011). LRRs have been reported to play significant role in promoting oxidative stress resistance in Arabidopsis thaliana (Yang et al., 2022).
Five putative candidate genes were found in association with tuber flesh hardness (Table 3). Leucine-rich repeat (LRR) genes have been reported to play significant role in the textural properties of D. alata (Mota et al., 2023). The specific functions and underlying mechanisms in relation to tuber flesh hardness are still not clear. Further functional study can elaborate on their potential roles.
The SNP marker-DMC trait association exhibited high allele segregation. The allele TT on chromosome 7 predicts high dry matter content in the diversity panel used in the study, while allele CC was identified to associate with low dry matter content. Allele TT has been reported to be associated with higher dry matter content in the study conducted on diversity panel of D. alata (Gatarira et al., 2020). For the TBHard, we found allele AA to be responsible for low tuber flesh oxidation, while allele GG was associated with high tuber flesh hardness. Haplotypes AA and AG were predicted to be responsible for low tuber flesh oxidation, while allele GG was linked with high tuber flesh oxidation. Gatarira et al. (2020) also predicted the influence of allele AG on low tuber flesh oxidation in the study conducted on the diversity panel of D. alata.
The identified putative candidate genes and SNPs connected to these essential economic traits could aid in the development of new breeding strategies to stockpile superior alleles for these crucial traits in future bush yam improvement programs. However, some of the unique regions identified in this study have not previously been identified and explored in Dioscorea species.
5 Conclusions
We accessed the trait association in bush yam using six genetic model and detect 16 markers associated with DMC, TBOXI and TBHard. The SNP markers explained high phenotypic variance and could be investigated for marker assisted selection. Through the gene annotation we discovered 25 putative candidate genes linked to the evaluated traits. Further genetic studies involving transcript/transcriptome analysis, will be required to validate associations and candidate genes identified in this study to accelerate genetic improvement of agronomic and tuber quality traits in bush yam germplasm.
Data availability statement
The datasets presented in this study can be found in online repositories. The names of the repository/repositories and accession number(s) can be found below: https://figshare.com/, 23713059.
Author contributions
AA: Conceptualization, Data curation, Formal analysis, Investigation, Methodology, Resources, Visualization, Writing – original draft, Writing – review & editing. PAs: Conceptualization, Supervision, Methodology, Validation, Writing – review & editing. OA: Writing – review & editing. IA: Writing – original draft, Writing – review & editing. MA: Conceptualization, Supervision, Writing – review & editing. KT: Conceptualization, Supervision, Writing – review & editing. VO: Writing – review & editing. AS: Writing – review & editing. SA: Writing – review & editing. JM: Writing – original draft, Writing – review & editing. HM: Writing – review & editing. PAg: Conceptualization, Data curation, Formal analysis, Funding acquisition, Investigation, Methodology, Resources, Software, Supervision, Validation, Visualization, Writing – original draft, Writing – review & editing.
Funding
The author(s) declare financial support was received for the research, authorship, and/or publication of this article. We acknowledge, funding support from the Bill and Melinda Gates Foundation (BMGF/PP1052998).
Acknowledgments
Authors acknowledge the assistance of field assistants of Teaching and Research Farm of U.C.C, Cape Coast, Ghana for their assistance in carry field activities and the laboratory supervisor of Yam Breeding Unit, I.I.T.A, Ibadan, Nigeria for his assistance in genotyping activities. We appreciate support from the African trans-regional cooperation through academic mobility (ACADEMY) project, reference number 2017-3052/001-001, funded by the European Union Commission and African Union within the framework of “Intra Africa Mobility Scheme”. Additional financial support was provided by the International Foundation for Science under grant agreement I-3-C-6624-1 and BAYER Foundation and Alexander von Humboldt (AvH) Foundation through the program “AGNES-BAYER Foundation Research Grant for Biodiversity Conservation and Sustainable Agriculture in Sub-Saharan Africa”.
Conflict of interest
The authors declare that the research was conducted in the absence of any commercial or financial relationships that could be construed as a potential conflict of interest.
Publisher’s note
All claims expressed in this article are solely those of the authors and do not necessarily represent those of their affiliated organizations, or those of the publisher, the editors and the reviewers. Any product that may be evaluated in this article, or claim that may be made by its manufacturer, is not guaranteed or endorsed by the publisher.
Supplementary material
The Supplementary Material for this article can be found online at: https://www.frontiersin.org/articles/10.3389/fhort.2024.1373327/full#supplementary-material
References
Abano E. E., Ma H., Qu W. (2012). Influence of combined microwave-vacuum drying on drying kinetics and quality of dried tomato slices. J. Food Qual. 35, 159–168. doi: 10.1111/j.1745-4557.2012.00446.x
Adewumi A. S., Asare P. A., Adu M. O., Taah K. J., Akaba S., Mondo J. M., et al. (2021). Farmers’ perceptions on varietal diversity, trait preferences and diversity management of bush yam (Dioscorea praehensilis Benth.) in Ghana. Sci. Afr. 12, e00808. doi: 10.1016/j.sciaf.2021.e00808
Afoakwa E. O., Sefa-Dedeh S. (2002). Textural and microstructural changes associated with post-harvest hardening of trifoliate yam (Dioscorea dumetorum) pax tubers. Food Chem. 77, 279–284. doi: 10.1016/S0308-8146(01)00343-0
Agre P., Norman P. E., Asiedu R., Asfaw A. (2021). Identification of quantitative trait nucleotides and candidate genes for tuber yield and mosaic virus tolerance in an elite population of White Guinea Yam (Dioscorea rotundata) using genome-wide association scan. BMC Plant Biol., 1–17. doi: 10.1186/s12870-021-03314-w
Agre P. A., Edemodu A., Obidiegwu J. E., Adebola P., Asiedu R., Asfaw A. (2023). Variability and genetic merits of white Guinea yam landraces in Nigeria. Front. Plant Sci. doi: 10.3389/fpls.2023.1051840
Alexis S. D. (2013). Technical sheet of some wild yam (Dioscorea) species starch functional properties. IOSRPHR 03, 66–72. doi: 10.9790/3013-0361066-72
Arnau G., Desfontaines L., Ehounou A. E., Marie‐Magdeleine C., Kouakou A. M., Leinster J., et al (2023). Quantitative trait loci and candidate genes for physico‐chemical traits related to tuber quality in greater yam (Dioscorea alata L.). J. Sci. Food Agric.
Arnau G., Maledon E., Nudol E., Gravillon M. C. (2016). “Progress and challenges in gene/c improvement of yam (Dioscorea alata L.),” in Electronic Proceedings of World Congress on Root and Tuber Crops (World Congress on Root and Tuber Crops. 1, Nanning (Chine, Nanning).
Asfaw A. (2016). Standard operating protocol for yam variety performance evaluation trial (Ibadan, Nigeria: IITA), 27. doi: 10.13140/RG.2.1.3656.4887
Asfaw A., Mondo J. M., Agre P. A., Asiedu R., Akoroda M. O. (2022). Association mapping of plant sex and cross-compatibility related traits in white Guinea yam (Dioscorea rotundata Poir.) clones. BMC Plant Biol. 22, 1–12. doi: 10.1186/s12870-022-03673-y
Bechoff A., Tomlins K. I., Chijioke U., Ilona P., Westby A., Boy E. (2018). Physical losses could partially explain modest carotenoid retention in dried food products from biofortified cassava. PloS One 13, e0194402. doi: 10.1371/journal.pone.0194402
Brachi B., Morris G. P., Borevitz J. O. (2011). Genome-wide association studies in plants: the missing heritability is in the field. Genome Biol. 12, 232. doi: 10.1186/gb-2011-12-10-232
Chi M., Bhagwat B., Lane W. D., Tang G., Su Y., Sun R., et al. (2014). Reduced polyphenol oxidase gene expression and enzymatic browning in potato (Solanum tuberosum L.) with artificial microRNAs. BMC Plant Biol. 14, 1–18. doi: 10.1186/1471-2229-14-62
Cormier F., Lawac F., Maledon E., Gravillon M. C., Nudol E., Mournet P., et al. (2019). A reference high-density genetic map of greater yam (Dioscorea alata L.). Theor. Appl. Genet. 132, 1733–1744. doi: 10.1007/s00122-019-03311-6
Dansi A., Mignouna H. D., Zoundjihékpon J., Sangare A., Asiedu R., Quin F. M. (1999). Morphological diversity, cultivar groups and possible descent in the cultivated yams (Dioscorea cayenensis/D. rotundata) complex in Benin Republic. Genet. Resour. Crop Evol. 46, 371–388. doi: 10.1023/A:1008698123887
Darkwa K., Agre P., Olasanmi B., Iseki K., Matsumoto R., Powell A., et al. (2020). Comparative assessment of genetic diversity matrices and clustering methods in white Guinea yam (Dioscorea rotundata) based on morphological and molecular markers. Sci. Rep. 10. doi: 10.1038/s41598-020-69925-9
do Carmo C. D., e Sousa M. B., Brito A. C., de Oliveira E. J. (2020). Genome-wide association studies for waxy starch in cassava. Euphytica. doi: 10.1007/s10681-020-02615-9
Dossa K., Morel A., Houngbo M. E., Mota A. Z., Maledon E., Irep J. L., et al. (2023). Genome-wide association studies reveal novel loci controlling tuber flesh color and oxidative browning in Dioscorea alata. J. Sci. Food Agric. doi: 10.1002/jsfa.12721
Ehounou A. E., Cormier F., Maledon E., Nudol E., Vignes H., Gravillon M. C. (2022). Identification and validation of QTLs for tuber quality related traits in greater yam (Dioscorea alata L.). Sci. Rep. 12 (1), 8423.
Enkhbayar P., Kamiya M., Osaki M., Matsumoto T., Matsushima N. (2004). Structural principles of leucine-rich repeat (LRR) proteins. Proteins 54, 394–403. doi: 10.1002/prot.10605
Esuma W., Herselman L., Labuschagne M. T., Ramu P., Lu F., Baguma Y., et al. (2016). Genome-wide association mapping of provitamin A carotenoid content in cassava. Euphytica. doi: 10.1007/s10681-016-1772-5
FAO FAOSTAT. (2021). Food and Agriculture Organization Cooperate Statistical Database. Available at: http://www.fao.org/faostat/en/#data/QL (Accessed 10th August 2021).
Gatarira C., Agre P., Matsumoto R., Edemodu A., Adetimirin V., Bhattacharjee R., et al. (2020). Genome-wide association analysis for tuber dry matter and oxidative browning in water yam (Dioscorea alata L.). Plants 9, 969. doi: 10.3390/plants9080969
González M. N., Massa G. A., Andersson M., Turesson H., Olsson N., Fält A. S., et al. (2020). Reduced enzymatic browning in potato tubers by specific editing of a polyphenol oxidase gene via ribonucleoprotein complexes delivery of the CRISPR/cas9 system. Front. Plant Sci. 10. doi: 10.3389/fpls.2019.01649
Graham-Acquaah S., Ayernor G. S., Bediako-Amoa B., Saalia F. K., Afoakwa E. O. (2014). Spatial distribution of total phenolic content, enzymatic activities and browning in white yam (Dioscorea rotundata) tubers. J. Food Sci. Technol. 51, 2833–2838. doi: 10.1007/s13197-012-0760-6
Hunter S., Jones P., Mitchell A., Apweiler R., Attwood T. K., Bateman A., et al. (2012). InterPro in 2011: New developments in the family and domain prediction database. Nucleic Acids Res. 40, 306–312. doi: 10.1093/nar/gkr948
Jiang Y., Duan X., Qu H., Zheng S. (2015). Browning: enzymatic browning. Encyclopedia Food Health, 508–514. doi: 10.1016/B978-0-12-384947-2.00090-8
Jombart T., Devillard S., Balloux F. (2010). Discriminant analysis of principal components: a new method for the analysis of genetically structured populations. BMC Genet. 11, 1–15. doi: 10.1186/1471-2156-11-94
Karikari B., Wang Z., Zhou Y., Yan W., Feng J., Zhao T. (2020). Identification of quantitative trait nucleotides and candidate genes for soybean seed weight by multiple models of genome-wide association study. BMC Plant Biol. 20, 404. doi: 10.1186/s12870-020-02604-z
Kilian A., Sanewski G., Ko L. (2016). The application of DArTseq technology to pineapple. Acta Hortic. 1111, 181–188. doi: 10.17660/ActaHortic.2016.1111.27
Kim D., Langmead B., Salzberg S. L. (2015). HISAT: a fast spliced aligner with low memory requirements Daehwan HHS Public Access. Nat. Methods 12, 357–360. doi: 10.1038/nmeth.3317.HISAT
Kobe B., Kajava A. V. (2001). The leucine-rich repeat as a protein recognition motif. Curr. Opin. Struct. Biol. 11, 725–732. doi: 10.1016/S0959-440X(01)00266-4
Medoua G. N., Mbofung C. M. F. (2006). Hard-to-cook defect in trifoliate yam Dioscorea dumetorum tubers after harvest. Int. Food Res. J. 39, 513–518. doi: 10.1016/j.foodres.2005.10.005
Minic Z. (2008). Physiological roles of plant glycoside hydrolases. Planta 227, 723. doi: 10.1007/s00425-007-0668-y
Mondo J. M., Agre P. A., Asiedu R., Akoroda M. O., Asfaw A. (2021). Genome-wide association studies for sex determination and cross-compatibility in water yam (Dioscorea alata L.). Plants 10, 1–18. doi: 10.3390/plants10071412
Morris G. P., Ramu P., Deshpande S. P., Hash C. T., Shah T., Upadhyaya H. D., et al. (2013). Population genomics and genome-wide association studies of agroclimatic traits in sorghum. PNAS 110, 453–458. doi: 10.1073/pnas.1215985110
Mota A. Z., Dossa K., Lechaudel M., Cornet D., Mournet P., Lopez D., et al. (2023). Genomic insights into greater yam tuber quality traits. bioRxiv. doi: 10.1101/2023.03.17.532727
Ng A., Xavier R. J. (2011). Leucine-rich repeat (LRR) proteins: integrators of pattern recognition and signaling in immunity. Autophagy 7, 1082–1084. doi: 10.4161/auto.7.9.16464
Pitalounani W. E. N., Dourma M., Wala K., Woegan Y., Gbogbo A., Batawila K., et al. (2017). Agrodiversity, peasant management and importance of Dioscorea praehensilis Benth. In the Subhumid Zone of Togo. Afr. J. Food Agric. Nutr. Dev. 17, 12455–12475. doi: 10.18697/ajfand.79.15930
Rabbi I. Y., Udoh L. I., Wolfe M., Parkes E. Y., Gedil M. A., Dixon A., et al. (2017). Genome-wide association mapping of correlated traits in cassava: dry matter and total carotenoid content. Plant Genome 10, plantgenome2016.09.0094. doi: 10.3835/plantgenome2016.09.0094
R Development Core Team (2019). R: A Language and Environment for Statistical Computing (R Foundation for Statistical Computing: Vienna, Austria).
Ren W. L., Wen Y. J., Dunwell J. M., Zhang Y. M. (2018). pKWmEB: integration of Kruskal-Wallis test with empirical Bayes under polygenic background control for multi-locus genome-wide association study. Heredity 120, 208–218. doi: 10.1038/s41437-017-0007-4
Rinaldo D., Sotin H., Pétro D., Le-Bail G., Guyot S. (2022). Browning susceptibility of new hybrids of yam (Dioscorea alata) as related to their total phenolic content and their phenolic profile determined using LCUV-MS. LWT 162, 113410.
Ryttersgaard C., Lo Leggio L., Coutinho P. M., Henrissat B., Larsen S. (2002). Aspergillus aculeatus β-1, 4-galactanase: substrate recognition and relations to other glycoside hydrolases in clan GH -A. Biochem. 41 (51), 15135–15143.
Salazar E., González M., Araya C., Mejía N., Carrasco B. (2017). Genetic diversity and intra-racial structure of Chilean Choclero corn (Zea mays L.) germplasm revealed by simple sequence repeat markers (SSRs). Sci. Hortic. 225, 620–629. doi: 10.1016/j.scienta.2017.08.006
Sanchez T., Ceballos H., Dufour D., Ortiz D., Morante N., Calle F., et al. (2014). Prediction of carotenoids, cyanide and dry matter contents in fresh cassava root using NIRS and Hunter color techniques. Food Chem. 151, 444–451. doi: 10.1016/j.foodchem.2013.11.081
Scarcelli N., Cubry P., Akakpo R., Thuillet A. C., Obidiegwu J., Baco M. N., et al. (2019). Yam genomics supports West Africa as a major cradle of crop domestication. Sci. Adv. 5, 1–8. doi: 10.1126/sciadv.aaw1947
Shin J. H., Blay S., McNeney B., Graham J. (2006). LDheatmap: an R function for graphical display of pairwise linkage disequilibria between single nucleotide polymorphisms. J. Stat. Software 16, 1–10. doi: 10.18637/jss.v016.c03
Siadjeu C., Akdowa Panyoo E., Mahbou Somo Toukam G., Bell J. M., Nono B., Medoua G. N. (2016). Influence of cultivar on the postharvest hardening of trifoliate yam (Dioscorea dumetorum) tubers. Adv. Agric. 2016, 1–7. doi: 10.1155/2016/2658983
Stanley A. E., Menkir A., Ifie B., Paterne A. A., Unachukwu N. N., Meseka S., et al. (2021). Association analysis for resistance to Striga hermonthica in diverse tropical maize inbred lines. Sci. Rep. 11. doi: 10.1038/s41598-021-03566-4
Sugihara Y., Darkwa K., Yaegashi H., Natsume S., Shimizu M., Abe A., et al. (2020). Genome analyses reveal the hybrid origin of the staple crop white Guinea yam (Dioscorea rotundata). PNAS 50, 31987–31992. doi: 10.1073/pnas.2015830117
Sukumaran S., Reynolds M. P., Sansaloni C. (2018). Genome-wide association analyses identify QTL hotspots for yield and component traits in durum wheat grown under yield potential, drought, and heat stress environments. Front. Plant Sci. 9. doi: 10.3389/fpls.2018.00081
Tamba C. L., Zhang Y. M. (2018). A fast mrMLM algorithm for multi-locus genome- wide association studies. BioRxiv, 341784. doi: 10.1101/341784
Uchendu K., Njoku D. N., Paterne A., Rabbi I. Y., Dzidzienyo D., Tongoona P., et al. (2021). Genome-wide association study of root mealiness and other texture-associated traits in cassava. Front. Plant Sci. 12. doi: 10.3389/fpls.2021.770434
Wang S. B., Feng J. Y., Ren W. L., Huang B., Zhou L., Wen Y. J., et al. (2016). Improving power and accuracy of genome-wide association studies via a multi-locus mixed linear model methodology. Sci. Rep. 6, 19444. doi: 10.1038/srep19444
Wu W., Chen C., Zhang Q., Ahmed J. Z., Xu Y., Huang X., et al. (2019). A comparative assessment of diversity of greater yam (Dioscorea alata) in China. Sci. Hortic. 243, 116–124. doi: 10.1016/j.scienta.2018.08.016
Yang L., Gao C., Jiang L. (2022). Leucine-rich repeat receptor-like protein kinase AtORPK1 promotes oxidative stress resistance in an AtORPK1-AtKAPP mediated module in Arabidopsis. Plant Sci. 315, 111147. doi: 10.1016/j.plantsci.2021.111147
Yang-Jun W., Hanwen Z., Yuan-Li N., Bo H., Jin Z., Jian-Ying F., et al. (2017). Methodo- logical implementation of mixed linear models in multi-locus genome- wide association studies. Brief. Bioinform. 19, 700–712.
Yin L. (2019). R package “CMPlots”. Available at: https://github.com/YinLiLin/R-CMplot (Accessed January 2023).
Zhang J., Feng J. Y., Ni Y. L., Wen Y. J., Niu Y., Tamba C. L., et al. (2017). pLARmEB: integration of least angle regression with empirical Bayes for multi-locus genome-wide association studies. Heredity 118, 517–524. doi: 10.1038/hdy.2017.8
Keywords: SNP markers, trait association mapping, gene annotation, yam, trait discovery
Citation: Adewumi AS, Asare PA, Akintayo OT, Adejumobi II, Adu MO, Taah KJ, Afutu E, Opoku VA, Stanley AE, Akaba S, Mondo JM, Mushoriwa H and Agre PA (2024) Genetic architecture of post-harvest tuber quality traits in bush yam (Dioscorea praehensilis Benth.) germplasm through association mapping. Front. Hortic. 3:1373327. doi: 10.3389/fhort.2024.1373327
Received: 19 January 2024; Accepted: 19 March 2024;
Published: 08 April 2024.
Edited by:
Nicola Busatto, Fondazione Edmund Mach, ItalyReviewed by:
Takuya Morimoto, Kyoto Prefectural University, JapanMukesh Choudhary, ICAR-Indian Institute of Maize Research, India
Copyright © 2024 Adewumi, Asare, Akintayo, Adejumobi, Adu, Taah, Afutu, Opoku, Stanley, Akaba, Mondo, Mushoriwa and Agre. This is an open-access article distributed under the terms of the Creative Commons Attribution License (CC BY). The use, distribution or reproduction in other forums is permitted, provided the original author(s) and the copyright owner(s) are credited and that the original publication in this journal is cited, in accordance with accepted academic practice. No use, distribution or reproduction is permitted which does not comply with these terms.
*Correspondence: Paterne A. Agre, p.agre@cgiar.org; Adeyinka S. Adewumi, adewumi.saburi@stu.ucc.edu.gh