Corrigendum: Systematic pan-cancer analysis of the potential tumor diagnosis and prognosis biomarker P4HA3
- 1Department of Orthopedic and Trauma Surgery, The First Affiliated Hospital of Guangxi Medical University, Nanning, Guangxi, China
- 2Department of Trauma Hand Surgery, The Second Nanning People’s Hospital, Nanning, Guangxi, China
- 3Department of Joint Surgery, The Second Nanning People’s Hospital, Nanning, Guangxi, China
- 4Faculty of Health Sciences, University of Almerìa, Almeria, Spain
- 5Department of Rehabilitation Medicine, Guigang City People’s Hospital, Guigang, China
- 6Department of Rehabilitation Medicine, The First Affiliated Hospital of Guangxi Medical University, Nanning, Guangxi, China
- 7Department of Cardiology, The Affiliated Cardiovascular Hospital of Kunming Medical University (Fuwai Yunnan Cardiovascular Hospital), Kunming, Yunnan, China
Purpose: Prolyl 4-hydroxylase subunit alpha 3 (P4HA3) is implicated in several cancers’ development. However, P4HA3 has not been reported in other cancers, and the exact mechanism of action is currently unknown.
Materials and methods: First, the expression profile of P4HA3 was analyzed using a combination of the University of California Santa Cruz (UCSC) database, Cancer Cell Line Encyclopedia (CCLE) database, and Genotype-Tissue Expression (GTEx) database. UniCox and Kaplan-Meier were used to analyze the predictive value of P4HA3. The expression of P4HA3 was analyzed in clinical staging, immune subtypes, and Molecular subtypes. Secondly, the correlation of P4HA3 with immunomodulatory genes, immune checkpoint genes, RNA modification genes, immune cell infiltration, cancer-related functional status, tumor stemness index, DNA mismatch repair (MMR) genes and DNA Methyltransferase was examined. The role of P4HA3 in DNA methylation, copy number variation (CNV), mutational status, tumor mutational burden (TMB), and microsatellite instability (MSI) was also analyzed. In addition, gene set enrichment analysis (GSEA) was used to explore the potential functional mechanisms of P4HA3 in pan-cancer. Finally, P4HA3-related drugs were searched in CellMiner, Genomics of Drug Sensitivity in Cancer (GDSC), and Cancer Therapeutics Response Portal (CTRP) databases.
Results: P4HA3 is significantly overexpressed in most cancers and is associated with poor prognosis. P4HA3 is strongly associated with clinical cancer stage, immune subtypes, molecular subtypes, immune regulatory genes, immune checkpoint genes, RNA modifier genes, immune cell infiltration, cancer-related functional status, tumor stemness index, MMR Gene, DNA Methyltransferase, DNA methylation, CNV, mutational status, TMB, and MSI are closely related. Available enrichment analysis revealed that P4HA3 is associated with the epithelial-mesenchymal transition and immune-related pathways. There are currently 20 drugs associated with P4HA3.
Conclusion: In human pan-cancer, P4HA3 is associated with poor patient prognosis and multiple immune cells and may be a novel immunotherapeutic target. It may act on tumor progression through the epithelial-mesenchymal transition (EMT) pathway.
Introduction
According to the latest data on cancer from the 2020 statistics, the worldwide cancer burden is expected to increase by 47% by 2040 compared to 2020 (Sung et al., 2021). Cancer immunotherapy is a treatment that fights cancer by boosting the patient’s immune system. Immunotherapy, a vital component of antitumor therapy, is not meeting the needs of the growing number of cancer patients, despite the continuous updating of its treatments. Therefore, searching for new and particular molecular markers of cancer is crucial for developing more effective anti-cancer drugs. The pan-cancer analysis is known to reveal the potential molecular mechanisms and functional roles among cancers systematically and comprehensively (Weinstein et al., 2013). In recent years, pan-cancer-based bioinformatics analysis methods have been highly favored by a wide range of researchers.
The extracellular matrix (ECM), the most abundant component of the tumor microenvironment (TME), plays a crucial role in tumor development (Bonnans et al., 2014; Xiong and Xu, 2016). Collagen is one of the significant components of the ECM and is associated with cancer progression (Armstrong et al., 2004; Koenig et al., 2006; Shintani et al., 2008; Gorres and Raines, 2010; Yang et al., 2014; Peng et al., 2017; Zheng et al., 2017; Yamazaki et al., 2018). It has been shown that cancer cells produce a type 1 collagen (Col I) that is fundamentally different from normal collagen in humans, resulting in a unique extracellular matrix that helps them increase and survive (Chen et al., 2022). Previous studies have found that Col I promotes cancer progression by facilitating tumor cell epithelial-mesenchymal transition (EMT) (Yang et al., 2014). Recent studies have found that loss of Col I in pancreatic cancer enhances T-cell infiltration, thus making anti-PD-1 immunotherapy more effective (Chen et al., 2022). Collagen deposition leads to poor prognosis in cancer patients (Whatcott et al., 2015). In addition, it is involved in tumor fibrosis (Yamauchi et al., 2018). Collagen prolyl 4-hydroxylases (C-P4H) are critical enzymes in collagen synthesis that maintain the triple helix structure’s stability by catalyzing the proline’s hydroxylation (Gjaltema and Bank, 2017). Interfering with prolyl 4-hydroxylase can regulate the process of collagen biosynthesis (Atkinson et al., 2019; D'Aniello et al., 2019). Recent studies have revealed that C-P4H is expressed at elevated levels in several cancers and may be a potential biomarker (Vasta and Raines, 2018). C-P4H is a tetramer composed of two a-subunits and two ß-subunits. Among them, the catalytic a-subunit has three different isozymes such as P4HA1, P4HA2, and P4HA3 (Vuori et al., 1992; Helaakoski et al., 1995; Van Den Diepstraten et al., 2003).
P4HA3 (prolyl 4-hydroxylase subunit alpha 3) is a protein-coding gene that belongs to the prolyl 4-hydroxylase family members. A study found that P4HA3 expression is upregulated in patients with renal cell carcinoma (RCC), promoting cancer growth, invasion, and metastasis (Zhou et al., 2022a). It has been reported that in gastric cancer (GC), P4HA3 is significantly upregulated compared to normal tissue and is associated with unfavorable overall survival (OS) (Song et al., 2018). P4HA3 is located upstream of the PI3K/AKT signaling pathway, and type VI α6 collagen (COL6A6) interacts with P4HA3 to inhibit pituitary adenoma (PA) growth and metastasis by blocking the PI3K/AKT signaling pathway (Long et al., 2019). In addition, it was found that P4HA3 overexpression on the PI3K/AKT signaling pathway reversed the inhibitory effect of COL6A6 on EMT (25). P4HA3 is overexpressed in melanoma and promotes tumor proliferation and invasion (Atkinson et al., 2019). The above findings suggest that P4HA3 is overexpressed in various cancers and is involved in cancer progression. However, its pan-cancer expression level and functional role have not been explored.
In this study, we evaluated the expression of P4HA3 and its relationship with the prognosis of cancer patients. In addition, the relationship between P4HA3 and the tumor immune microenvironment was further analyzed. Our findings provide new insights into the functional role of P4HA3 in human cancer, highlighting the potential mechanisms by which P4HA3 affects the tumor microenvironment and cancer immunotherapy.
Materials and methods
Collection and analysis of P4HA3 expression data
RNA-seq and clinical data of The Cancer Genome Atlas (TCGA) were downloaded from the UCSC Xena database (https://xenabrowser. net/datapages/). The UCSC database includes expression matrices and clinical data of pretreated TCGA tumor patients and normal subjects, including overall survival (OS), disease-specific survival (DSS), disease-free interval (DFI), and progression-free interval (PFI), tumor stage, etc. First, P4HA3 expression data of normal tissues were obtained from the GenotypeTIssup Expression (GTEx, https://commonfund.nih.gov/GTEx) database. And P4HA3 expression data of tumor cell lines were obtained from the Cancer Cell Line Encyclopedia (CCLE, https://portals.broadinstitute.org/ccle/) database. Second, a uniformly normalized pan-cancer dataset was obtained from the UCSC Xena database to analyze the expression levels of P4HA3 in cancer and the differential expression in cancer and normal samples. Meanwhile, we analyzed the expression of P4HA3 in male and female tumor tissues and the corresponding normal tissues using the R package “gganatogram” (Maag, 2018) and visualized it as BodyMap. We analyzed the protein expression level of P4HA3 using The Human Protein Atlas (HPA) database (https://www.proteinatlas.org). Many researchers have used this database to analyze the expression levels of target genes, such as Xiong et al., Zhou et al., Liu et al., and Xu et al. used this database to study the expression levels of PIMREG, NAAA, CD2, and TUBA1C respectively, which tentatively proved their analysis results (Chen et al., 2021; Huang et al., 2021; Zhu et al., 2021; Hu et al., 2022).
Survival and prognostic analysis of P4HA3
To understand the impact of P4HA3 on the survival and prognosis of cancer patients, we used Kaplan-Meier curve analysis and univariate Cox proportional hazard regression to explore the relationship between P4HA3 expression levels, and OS, DSS, DFI, and PFI. A p-value <0.05 was statistically significant.
Analysis of P4HA3 expression in tumor clinical stage, immune subtypes, and molecular subtype
The expression levels of P4HA3 were assessed in each tumor at different clinical stages. Next, the immune subtypes of P4HA3 in 33 tumors, including C1 (wound healing), C2(IFN-gamma dominant), C3 (inflammatory), C4 (lymphocyte depleted), C5 (immunologically quiet), and C6 (TGF-b dominant), were obtained in the TISIDB database (https://doi.org/10.1093/bioinformatics/btz210). In addition, the molecular subtype profile of P4HA3 in 13 tumors was also analyzed. We analyzed the association between P4HA3, immune, and molecular subtypes through TISIDB database of pre-processed gene expression data and phenotypic information of TCGA cancers.
Correlation analysis of P4HA3 with immunomodulatory genes, immune checkpoint genes, and RNA-modified genes
The expression data of 150 marker genes for immune regulation (chemokine (Qiu et al., 2019), receptor (D'Aniello et al., 2019), MHC (Helaakoski et al., 1995), Immunoinhibitor (Song et al., 2018), Immunostimulator (Chae et al., 2019)), 60 marker genes for immune checkpoint-related genes (Inhibitory (Song et al., 2018), Stimulatory (Mariathasan et al., 2018)) and 44 marker genes for RNA modification (m1A (Yamazaki et al., 2018), m5C (Chen et al., 2022), m6A (Helaakoski et al., 1995)) genes were extracted in each sample. Then, the correlations between P4HA3 and immune regulatory genes, immune checkpoint genes, and RNA modification genes were calculated separately using the spearman algorithm.
Analysis of tumor immune cell infiltration
The correlation between P4HA3 and many different types of immune cells was analyzed in the TIMER2.0 database (https://cistrome.shinyapps.io/timer/) using various algorithms such as TIMER, CIBERSORT, CIBERSORT-ABS, QUANTISEQ, XCELL, EPIC, and TIDE. The TIMER 2.0 database provides a more reliable analysis of target gene and immune cell correlations by using state-of-the-art algorithms to calculate the level of immune infiltration in TCGA tumor data. The results were visualized using the R package “ggplot2” (Ito and Murphy, 2013). In addition, we used the ESTIMATE algorithm to calculate the ESTIMATEScore, ImmuneScore, and StromalScore for different tumor types and the spearman algorithm to calculate the correlation coefficient between P4HA3 and the three scores.
Correlation analysis of P4HA3 with single cell level, cancer-related functional status, and tumor stemness index
We obtained 72 single-cell datasets from the CancerSEA database (https://ngdc.cncb.ac.cn/databasecommons/database/id/6092) as well as 14 cancer-related functional states (angiogenesis, apoptosis, cell cycle, cell differentiation, DNA damage, DNA repair, EMT, cellular hypoxia, inflammation onset, cancer cell invasion, metastasis, proliferation cell resting, stem cell properties). The correlation between P4HA3 and 14 cancer-related functional states in each tumor was analyzed and visualized using the R package “ggplot2”. In addition, we obtained DNAss tumor stemness scores calculated from methylation profiles for each cancer from a previous study (Malta et al., 2018). The stemness index and P4HA3 expression data of the samples were first integrated, then the correlation between them was calculated using the spearman algorithm. To further validate our results, tumor stemness scores such as EREG-METHss, DMPss, and ENHss were also performed.
Evaluation of P4HA3 mutation, methylation, TMB, MSI, MMR gene, DNA methyltransferase, and CNV in pancreatic cancer
To understand the mutation characteristics and location of P4HA3 in tumors, we explored using the cBioPortal database (https://www.cbioportal.org/). Meanwhile, using the TCGA database (https://www.cancer.gov/about-nci/organization/ccg/research/structural-genomics/tcga) we analyzed the relationship between the expression level of P4HA3 and the methylation level of its promoter region and visualized it using the R package “ggplot2”. The correlation between P4HA3 expression and tumor mutational burden (TMB) and microsatellite instability (MSI) in different tumors was analyzed in the TCGA database using Spearman’s test, and the results were visualized by the R package “fmsb”. In addition, we also analyzed the correlation between P4HA3 and five DNA mismatch repair (MMR) genes, MLH1, MSH2, MSH6, PMS2, and EpCAM. and their expression levels with the four methyltransferases DNMAT1, DNMT2, DNMT3A, and DNMT3B. Not only that, but we also analyzed the correlation between P4HA3 expression and somatic cell copy number variation (CNV) in pan-cancer.
Enrichment analysis of P4HA3
To further understand the biological functions and potential molecular mechanisms of P4HA3, we used the R package “ClusterProfiler” (Yu et al., 2012) to perform gene set enrichment analysis (GSEA). We also downloaded from the Molecular Signatures Database (MSigDB, https://www.gseamsigdb.org/gsea/index.jsp) a hallmark gene set containing 50 critical pathways affecting cancer. The Normalized Enrichment Score (NES) and False Discovery Rate (FDR) of P4HA3 in each path were calculated separately. p-values <0.05 were considered statistically significant.
Drug sensitivity analysis
To understand the potential drugs targeting P4HA3, we analyzed the relationship between P4HA3 expression and drug sensitivity using the CellMiner database (https://ngdc.cncb.ac.cn/databasecommons/database/id/6092). Meanwhile, to further expand our study, we also explored the correlation between P4HA3 expression and drug sensitivity from the Genomics of Drug Sensitivity in Cancer (GDSC) database (https://www.cancerrxgene.org) and Cancer Therapeutics Response Portal (CTRP) database (https://portals.broadinstitute.org/ctrp.v2.1/).
Results
Expression profile of P4HA3
We analyzed the expression levels of P4HA3 in normal tissues using the GTEx dataset (Figure 1A). The results showed that P4HA3 expression levels were higher in testis, pituitary, prostate, blood vessels, and thyroid. Based on the CCLE dataset, the expression levels of P4HA3 in different tumor cell lines were analyzed (Figure 1B). The results showed that the expression of P4HA3 was relatively similar in various tumor cell lines. In addition, the expression levels of P4HA3 in different tumors were shown in Figure 1C. Data from the TCGA database and GTEx database showed that P4HA3 was expressed in breast invasive carcinoma (BRCA), cholangiocarcinoma (CHOL), colon adenocarcinoma (COAD), lymphoid neoplasm diffuse large B cell lymphoma (DLBC), esophageal carcinoma (ESCA), glioblastoma (GBM), head and neck squamous cell carcinoma (HNSC), lung adenocarcinoma (LUAD), kidney renal clear cell carcinoma (KIRC), acute myeloid leukemia (LAML), lung squamous cell carcinoma (LUSC), pancreatic adenocarcinoma (PAAD), pheochromocytoma and paraganglioma (PCPG), rectum adenocarcinoma (READ), stomach adenocarcinoma (STAD), and thymoma (THYM) were expressed at higher levels than normal tissues in 16 tumors. In contrast, P4HA3 was expressed at lower levels than normal tissues in eight tumors, including liver hepatocellular carcinoma (LIHC), ovarian serous cystadenocarcinoma (OV), skin cutaneous melanoma (SKCM), thyroid carcinoma (THCA), uterine corpus endometrial carcinoma (UCEC), bladder urothelial carcinoma (BLCA), brain lower grade glioma (LGG), and testicular germ cell tumors (TGCT) (Figure 1D). Finally, we analyzed the expression levels of P4HA3 in active body maps using the GEPIA dataset. The results show that P4HA3 was differentially expressed in tumor tissues and corresponding normal tissues, especially in the brain, lung, esophagus, liver, gallbladder, stomach, intestine, thyroid, bladder, and bone (Supplementary Figures 1A, B). The IHC results of COAD, LIHC, LUAD, PAAD, melanoma, BLCA and normal tissues were show in Figure 2. More samples and experiments are needed to validate the expression of P4HA3 in the future. The above results suggest that P4HA3 is highly expressed in various tumors.
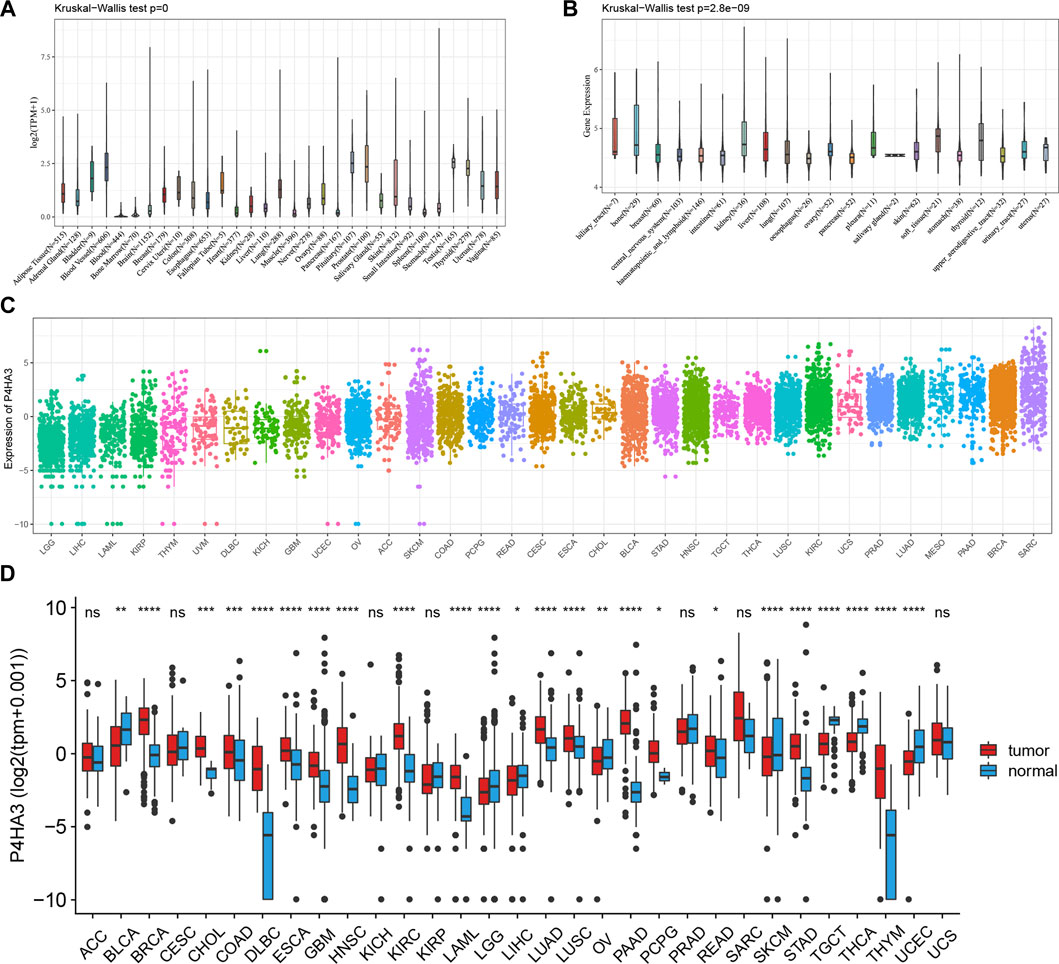
FIGURE 1. Expression of P4HA3 in normal and tumor tissues. (A) P4HA3 expression across 31 regular tissues and (B) 21 tumor cell lines. The mRNA expression landscape of P4HA3 in (C) tumor tissue on TCGA database and (D) expression of P4HA3 in normal and tumor tissues (*p < 0.05, **p < 0.01, and ***p < 0.001; ns: no significance). p values were based on the Wilcoxon rank sum test.
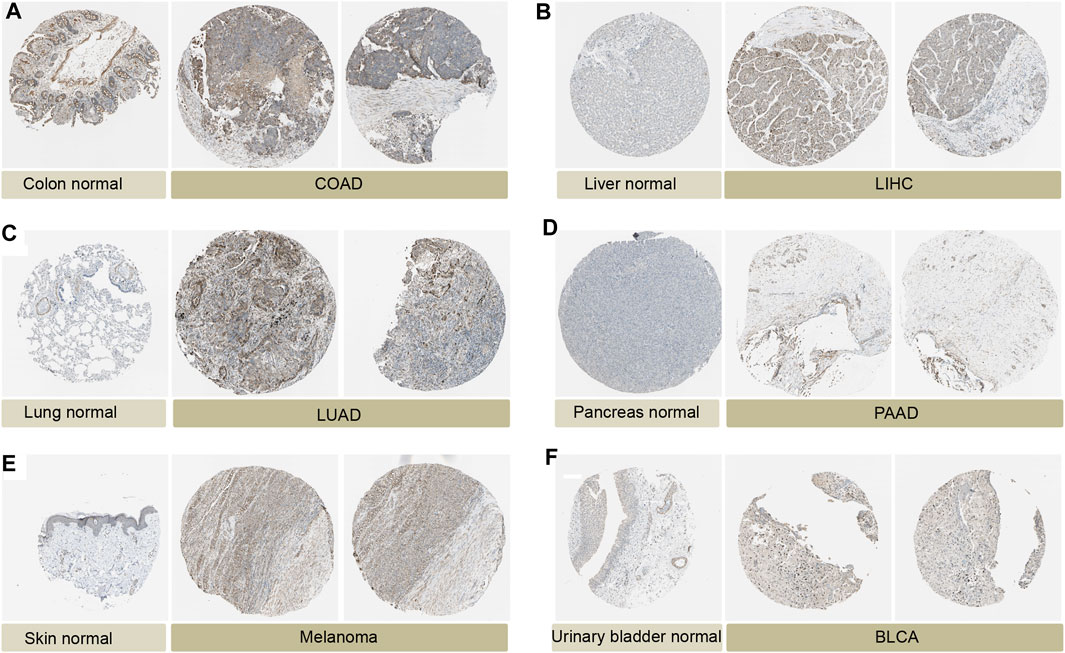
FIGURE 2. Protein expression of P4HA3 in normal and tumor tissues. Immunohistochemical staining in normal tissues and tumour tissues from the HPA database. (A) Colon normal, COAD. (B) Liver normal, LIHC. (C) Lung normal, LUAD. (D) Pancreas normal, GBM. (E) Skin normal, Melanoma. (F) Urinary bladder normal, BLCA.
Survival and prognostic value of P4HA3
Kaplan-Meier survival analysis showed that P4HA3 high expression in BRCA, CESC, COAD, GBM, HNSC, KIRC, kidney renal papillary cell carcinoma (KIRP), LGG, LUSC, OV, READ, STAD, THCA, UCEC, and uveal melanoma (UVM) had a poor prognosis (Figures 3A–O). Univariate COX regression analysis assessed the relationship between P4HA3 expression and OS, DSS, DFI, and PFI. The results showed that P4HA3 was a risk factor for OS in UVM, UCEC, THCA, STAD, PAAD, KIRP, KIRC, kidney chromophobe (KICH), GBM, COAD, cervical squamous cell carcinoma (CESC), and BLCA (Figure 4A); P4HA3 was a risk factor for DSS in UVM, PAAD, mesothelioma (MESO), LUAD, LGG, KIRP, KIRC, KICH, GBM, COAD, CESC, BRCA, BLCA, and adrenocortical carcinoma (ACC) (Figure 4B); P4HA3 was a risk factor for DFI in UVM, UCEC, THCA, PAAD, KIRP, KIRC, KICH, GBM, COAD, CESC, BRCA, and BLCA (Figure 4C); P4HA3 was a risk for PFI in PAAD, KIRP, and CESC (Figure 4D). The survival analysis results suggest that overexpression of P4HA3 is associated with poor prognosis in tumor patients.
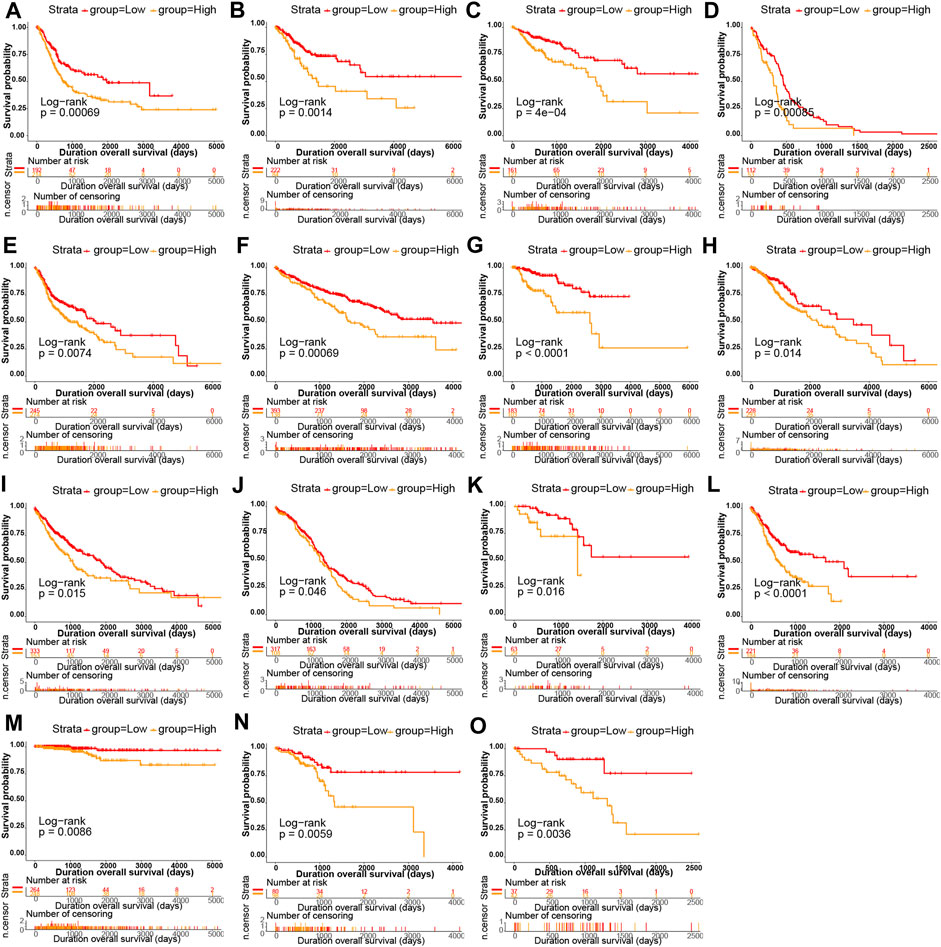
FIGURE 3. The relationship of P4HA3 expression with patients’ overall survival (OS). Kaplan–Meier survival analysis of OS between high- and low-expression groups of P4HA3 in (A) ACC (B) BRCA, (C) CESC (D) CHOL, (E) GBM (F) HNSC, (G) KICH (H) KIRC, (I) LAML (J) LGG, (K) LIHC (L) LUAD, (M) LUNG (N) MESO, and (O) LAML.
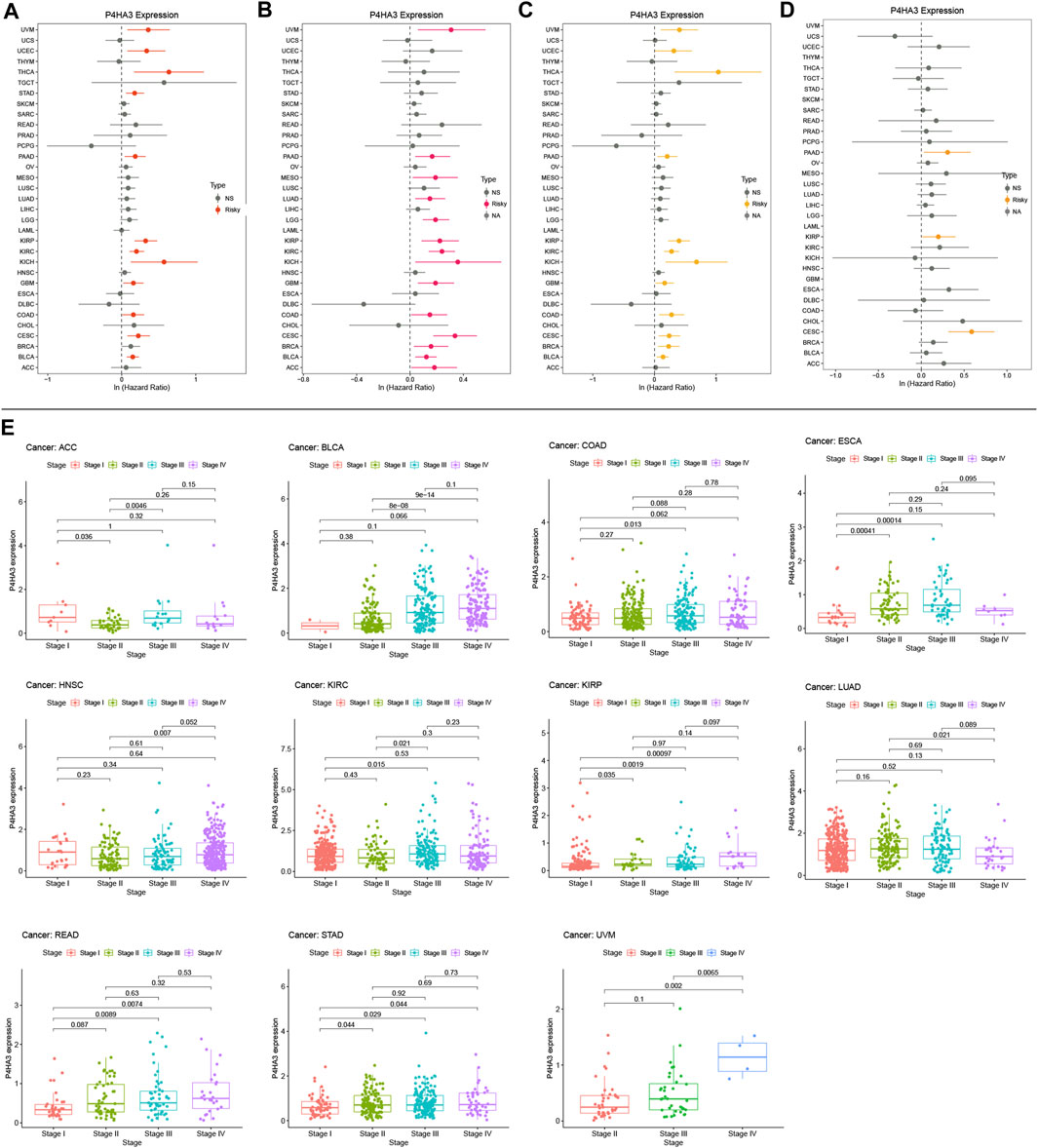
FIGURE 4. Correlation between P4HA3 expression with prognosis and clinical stage in cancer patients. Forest plots of hazard ratios of P4HA3 in (A) overall survival (OS) (B) progression-free interval (PFI) (C) disease-specific survival (DSS), and (D) disease-free interval (DFI). Red and yellow indicates that P4HA3 is a risk factor for the prognosis of cancer patients, while grey represents a protective factor. (E) The difference of P4HA3 expression level between distinct clinical stages in ACC, BLCA, COAD, ESCA, HNSC, KIRC, KIRP, LUAD, READ, STAD, and UVM.
Expression of P4HA3 in different clinical stages of tumors, immune subtypes, and molecular subtypes
After analysis and evaluation, we found that, in terms of clinical staging, P4HA3 was more highly expressed at higher stages of BLCA, COAD, ESCA, KIRP, READ, STAD, and UVM. In comparison, lower expression in higher stages of ACC, HNSC, KIRC, and LUAD (Figure 4E). Regarding immune subtypes, P4HA3 was significantly expressed in 17 tumors (Figure 5). Regarding the molecular subtypes, P4HA3 was expressed considerably in 13 tumors (Figure 6). Thus, the expression level of P4HA3 correlated with the clinicopathological stage, immune subtypes, and molecular subtypes of cancer.
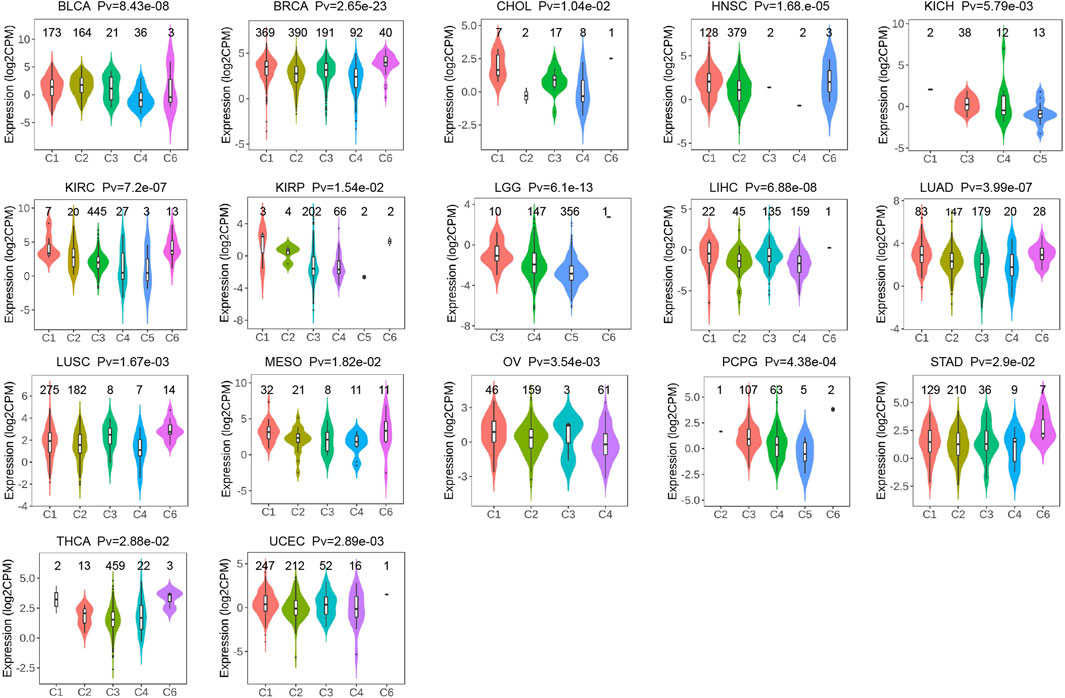
FIGURE 5. The relationship of P4HA3 expression with immune subtypes in BLCA, BRCA, CHOL, HNSC, KICH, KIRC, KIRP, LGG, LIHC, LUAD, LUSC, MESO, OV, PCPG, STAD, THCA, and UCEC. The different colors represent the different immune subtypes.
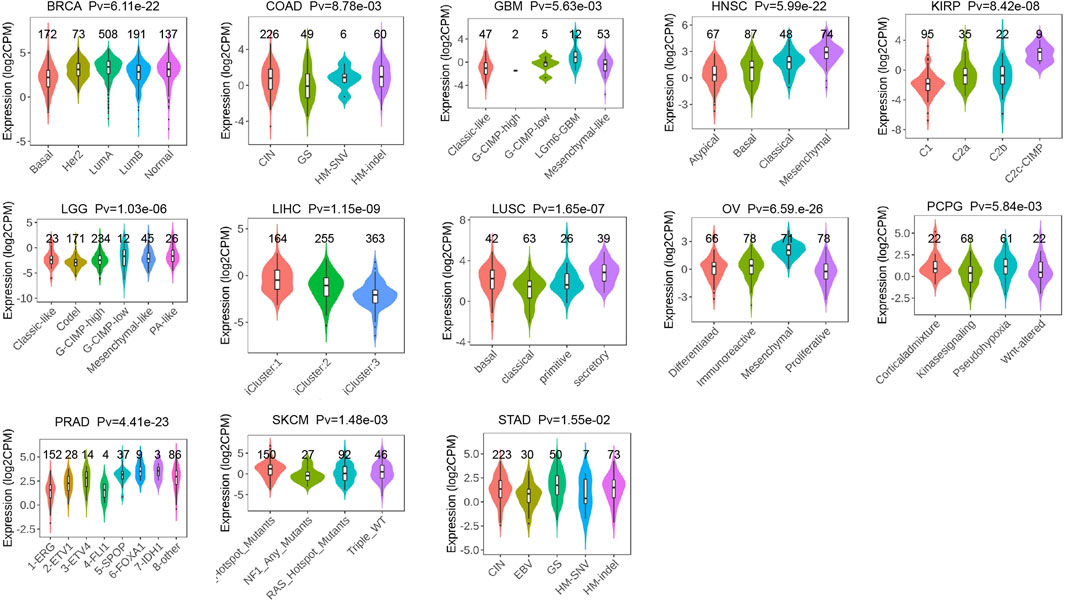
FIGURE 6. The differences of P4HA3 expression levels among distinctive molecular subtypes in BLCA, COAD, GBM, HNSC, KIRP, LGG, LIHC, LUSC, OV, PCPG, PRAD, SKCM, and STAD. The different colors represent the different molecular subtypes.
Correlation analysis of P4HA3 with immunomodulatory genes, immune checkpoint genes, and RNA-modified genes
It was found that in most tumors, P4HA3 was associated with CCL13, CCL18, CXCL12, CCL14, CCL21, CCL2, CCL7, CCL11, CCL26, CXCL3, CXCL8, CCR3, CXCR1, CCR7, CCR1, CXCR4, CCR4, CCR8, CCR10, HLA -DOA, HLA-DPA1, HLA-DPB1, HLA-DRA, B2M, TGFBR1, KDR, TGFBR1, IL10, ADORA2A, CD244, IL2RA, IL6, CD28, CXCR4, TNFRSF4, and CXCL12 immunoregulatory genes were significantly and positively correlated (Figure 7A). It also correlated with immune checkpoint TGFB1, C10orf54, CD276, VEGFA, EDNRB, CTLA4, PDCD1, ENTPD1, TNFSF4, SELP, CD28, TNFRSF4, ICAM1, IL2RE, TNFRSF9, TLR4, IL1B, CX3CL1, and CXCL10 genes were significantly and positively correlated (Figure 7B). In addition, we found that P4HA3 was significantly associated with genes related to m1A, m5c, and m6A in most tumors (Figure 7C). In most tumors, P4HA3 positively correlated with immunomodulatory, immune checkpoint, and RNA-modified genes.
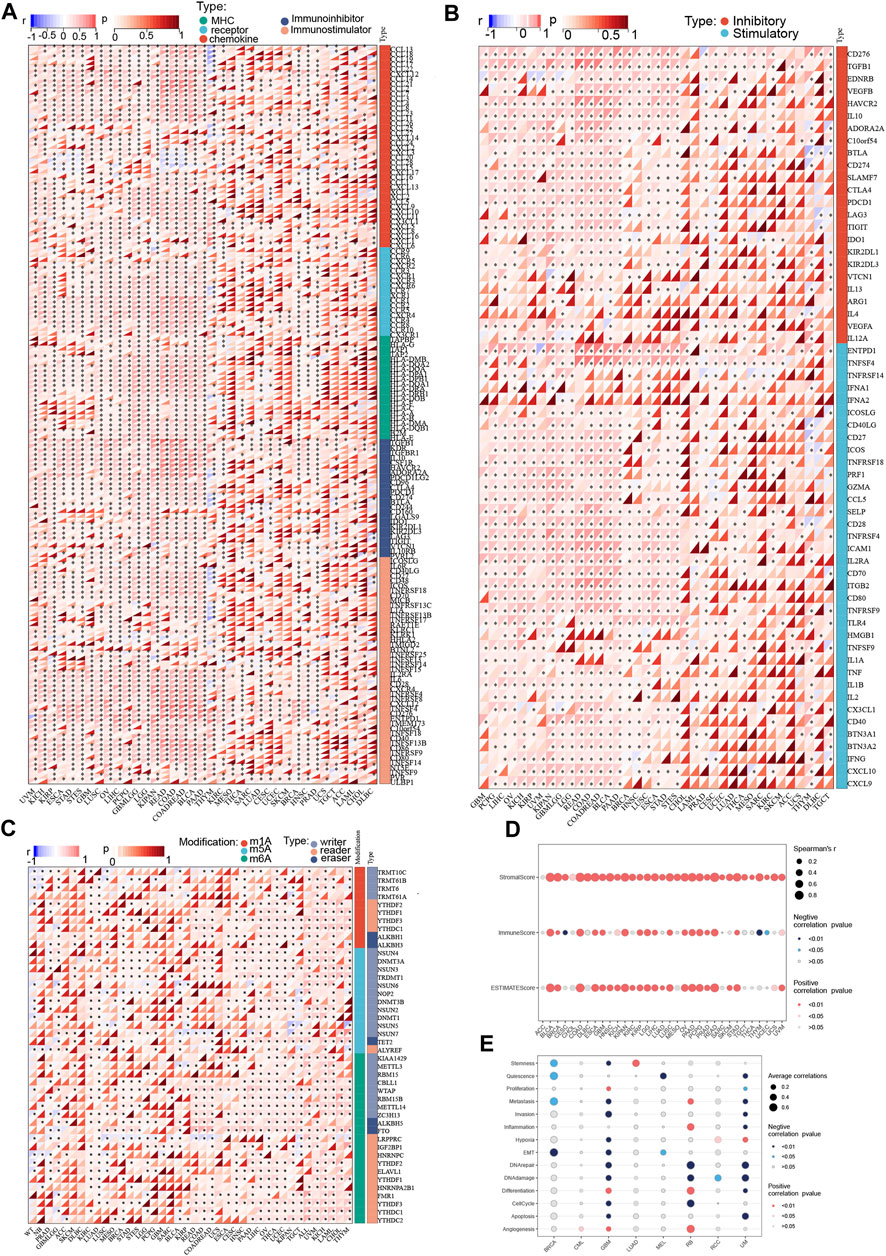
FIGURE 7. Co-expression of P4HA3 with immune-associated genes. Co-expression between P4HA3 and (A) Immunoregulatory genes (B) Immune checkpoint genes, and (C) RNA modifier genes (*p < 0.05, **p < 0.01, and ***p < 0.001). The Relationship of P4HA3 expression with (D) immune infiltration and (E) cancer related functional status analysis.
Correlation analysis of P4HA3 with tumor immune cell infiltration and cancer-related functional status
The results calculated according to the ESTIMATE algorithm showed that P4HA3 in different tumor types such as BLCA, BRCA, COAD, ESCA, GBM, KICH, KIPAN, KIRP, LGG, LIHC, LUSC, OV, PAAD, PCPG, PRAD, READ, STAD, and UVM was associated with significant positive correlations between the three scores of ESTIMATEScore, ImmuneScore, and StromalScore (Figure 7D). The study also showed that P4HA3 was significantly negatively correlated with nine different cancer-related functional statuses in GBM and UM, respectively (Figure 7E). Furthermore, as seen in the correlation heat map, P4HA3 was closely associated with a variety of immune cells such as CD8+ T cells, CD4+ T cells, B cells, neutrophils, myeloid dendritic cells, macrophages, cancer-associated fibroblast (CAF), endothelial cells, and Hematopoietic stem cells in pan-cancer (Figure 8A). Thus, P4HA3 is closely associated with tumor-associated immune cells and functional status.
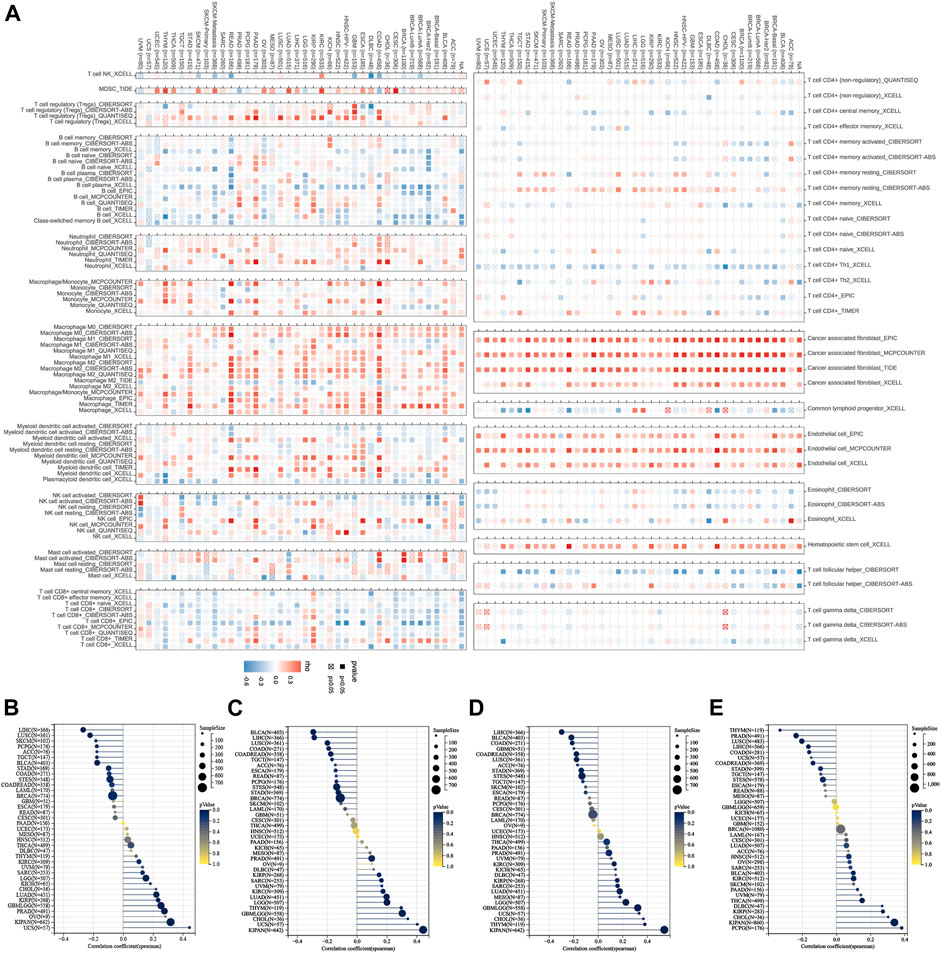
FIGURE 8. The correlations of P4HA3 expression and the infiltration levels of CD4+ T cells, cancer-associated fibroblast, macrophages, and many other immune cells in cancers (A). Positive correlation in red and negative correlation in blue. The Relationship of P4HA3 expression with tumor stemness index from (B) DMPss (C) DNAss, (D) ENHss, and (E) EREG-METHss algorithm.
Correlation analysis of P4HA3 and tumor stemness index
The analysis showed that P4HA3 was significantly positively correlated with different tumor stemness indices such as DMPss (Figure 8B), DNAss (Figure 8C), ENHss (Figure 8D), and EREG-METHss (Figure 8E) in KIPAN, PRAD, GBM, LGG, LUAD, KIRC, KIRP, and SARC. And significant negative correlations were found in LIHC, LUSC, BLCA, STAD, STES, BRCA, COAD, and READ. The results of this analysis suggest that P4HA3 is associated with tumor stemness.
Analysis of P4HA3 associated with mutation, methylation, CNV, TMB, MSI, MMR gene, and DNA methyltransferase
The mutation sites of P4HA3 are shown in Figure 9A. The mutation type was predominantly Amplification, with the highest mutation frequency of P4HA3 in Esophageal Adenocarcinoma (Figure 9B). P4HA3 was significantly negatively correlated with methylation levels in 25 tumors (Figure 9C). P4HA3 had the highest CNV expression levels in TGCT and OV (Figure 9D). The correlation analysis showed that P4HA3 and CNV expression levels were positively correlated in 26 tumors and negatively correlated in seven tumors (Figure 9E). As shown in Figure 9F, P4HA3 was significantly and positively correlated with TMB in UCS, THYM, PRAD, LUAD, LGG, and LAML. And it was significantly negatively correlated with TMB in BRCA, BLCA, UVM, LUSC, LIHC, KIRP, and HNSC. As shown in Figure 9G, P4HA3 was significantly and positively correlated with MSI in UCIEC, PRAD, MESO, COAD, and STAD. And it was significantly negatively correlated with MSI in STAD and LUSC. In addition, we analyzed the correlation between P4HA3 and the level of MMR gene mutations. The results showed that the expression of P4HA3 in KIRC, KIRP, LAML, LIHC, READ, THCA, and UCEC was significantly correlated with the mutation levels of five MMR genes (Figure 9H). Finally, we analyzed the correlation between P4HA3 and the four DNA methyltransferases. The results showed that the expression level of P4HA3 was significantly correlated with at least one DNA methyltransferase in other tumors except for SKCM, STAD, UCEC, UCS, ACC, CESC, GBM, and PCPG (Figure 9I).
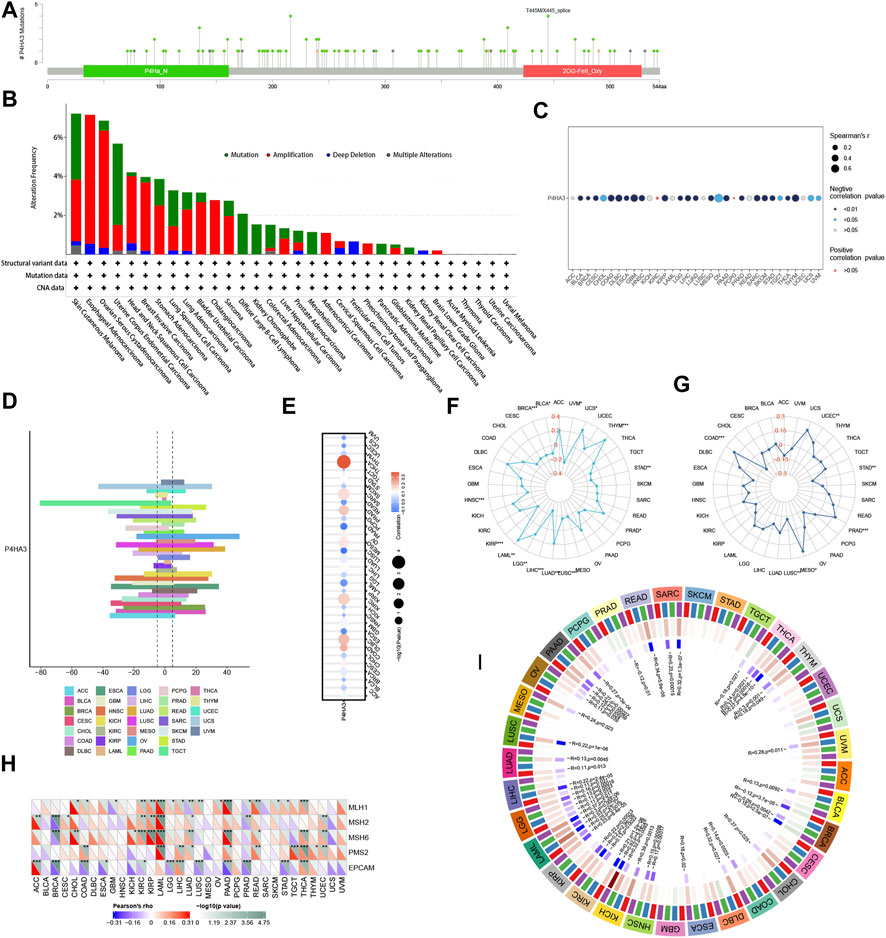
FIGURE 9. (A) Mutation sites of P4HA3 and (B) The mutation frequency and corresponding mutation types of P4HA3 in different cancers. (C) The Relationship of P4HA3 expression with methylation. (D) CNV expression of P4HA3 in human pan-cancer. (E) Correlation analysis of P4HA3 CNV expression with P4HA3 mRNA expression. The Relationship of P4HA3 expression with (F) TMB and (G) MSI. Figure 10: Correlation analysis of P4HA3 expression with (H) MMR genes in human pan-cancer (*p < 0.05, **p < 0.01, ***p < 0.001) and (I) DNA methyltransferases (Red represents DNMAT1, blue represents DNMT2, green represents DNMT3A, and purple represents DNMT3B).
The results of GSEA
The results of the GSEA analysis, as shown in Figure 10, showed that P4HA3 showed a significant positive correlation with UV response dn, TNF-alpha signaling via NFkB, myogenesis, KRAS signaling up, inflammatory response, il6 jak stat3 signaling, hypoxia, epithelial-mesenchymal transition, apical junction, and angiogenesis in most tumors. The enrichment analysis results suggest that P4HA3 is associated with multiple immune-related signaling pathways in tumors.
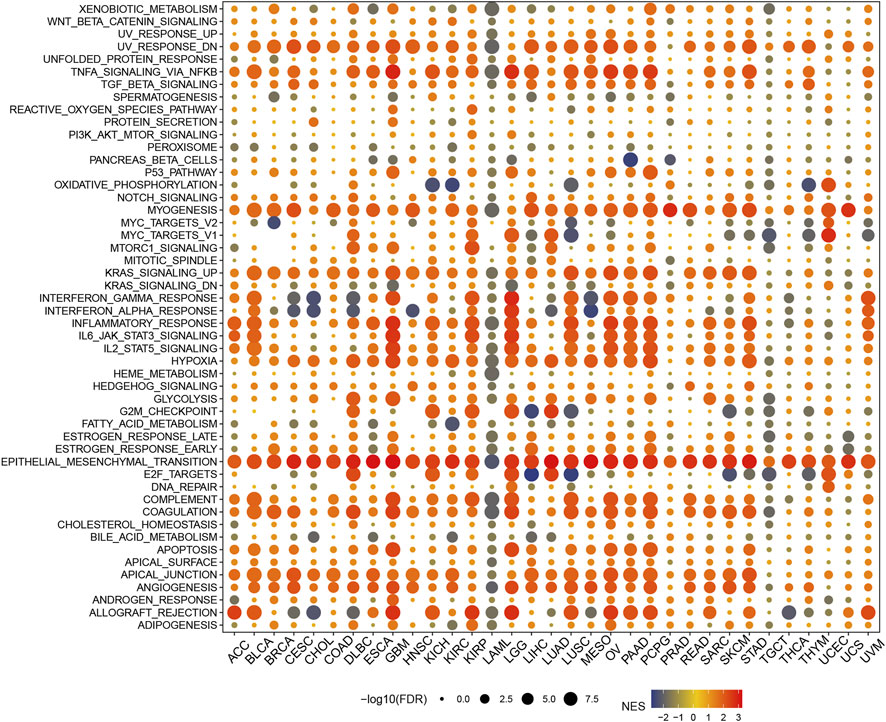
FIGURE 10. The hallmarks gene set enrichment analysis (GSEA) of P4HA3 in pan-cancer. The circle size represents each cancer enrichment item’s false discovery rate (FDR) value, and the color represents the normalized enrichment score (NES) of each enrichment item.
Sensitivity analysis of P4HA3 and drugs
In the CellMiner database, the expression of P4HA3 was correlated with Vincristine, geldanamycin analog, RH1, Tamoxifen, AT-13387, Panobinostat, Tanespimycin, Crizotinib, Vinblastine, Curcumin (+)-JQ1, Acrichine, Lomustine, Daunorubicin, 6-Mercaptopurine, and Belinosta1 were significantly and negatively correlated with 16 drugs (Figure 11A). In the GDSC database (Figure 11B) and the CTRP database (Figure 11C), P4HA3 was significantly positively correlated with various drugs.
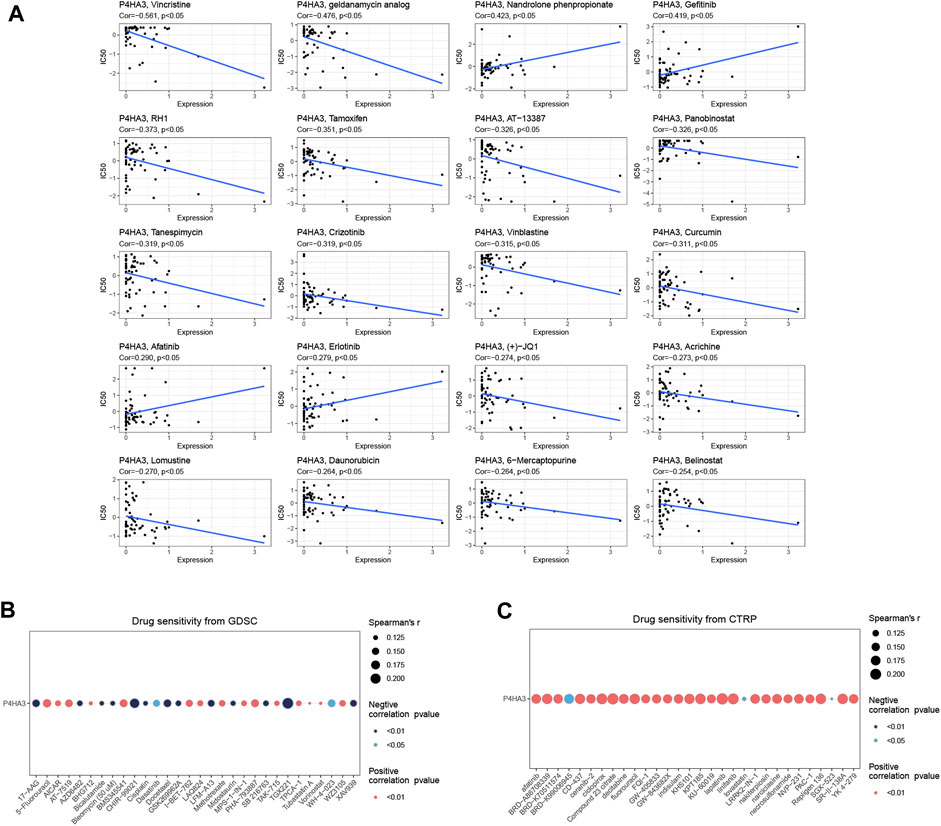
FIGURE 11. Drug sensitivity analysis. (A) In the CellMiner database, P4HA3 was shown to be closely associated with 20 drugs. (B) A Spearman association between P4HA3 and 30 drugs was performed in the GDSC database. (C) A Spearman association between P4HA3 and 30 drugs was performed in the CTRP database.
Discussion
Pan-cancer bioinformatics analysis can help us to understand the gene expression profile and its correlation with genetic mutations, clinicopathology, and prognosis (Ju et al., 2020). Besides, we can also use it to explore the potential mechanisms of gene roles in tumor immunology. Therefore, in this study, we systematically analyzed the expression of P4HA3 in various cancers. We found that P4HA3 was highly expressed in 16 tumors, including BRCA, CHOL, COAD, DLBC, ESCA, GBM, HNSC, LUAD, KIRC, LAML, LUSC, PAAD, PCPG, READ, STAD, and THYM. Combined with the Kaplan-Meier survival analysis results, we found that overexpression of P4HA3 in BRCA, COAD, GBM, HNSC, KIRC, LUSC, READ, and STAD was associated with poor prognosis in patients with these cancers. P4HA3 may serve as a promising biomarker for diagnosing and treating BRCA, COAD, GBM, HNSC, KIRC, LUSC READ, and STAD. Also, we found that the expression level of P4HA3 was closely associated with OS, DSS, DFI, and PFI in patients with multiple cancers, especially in PAAD, KIRP, and CESC. In addition, we analyzed the relationship between the expression of P4HA3 and the clinicopathological stage of cancer. It was found that the level of P4HA3 expression was higher in stage IV than in phase I in BLCA, COAD, ESCA, KIRP, READ, and STAD. This result suggests that the high level of P4HA3 expression correlates with the malignancy of these cancers.
Previous studies have found that collagen increases anti-programmed cell death protein 1 (PD-1)/PD-L1 resistance by depleting CD8+ T cells, which is associated with tumor immunosuppression and drug resistance (Chakravarthy et al., 2018; Mariathasan et al., 2018; Peng et al., 2020). Because of the above findings, we analyzed the relationship between P4HA3 and immune regulatory genes and checkpoint genes. We found that P4HA3 expression levels positively correlated with immune regulatory genes and checkpoint genes in most tumors. In addition, there is growing evidence that understanding tumor immune cell infiltration may assist in optimizing antitumor immunotherapy (Bai et al., 2020; Guo et al., 2020). Therefore, in this study, we also evaluated the correlation between P4HA3 expression and tumor immune cell infiltration levels. The results suggest that P4HA3 is associated with multiple immune cells in most cancers. Among them, the CD8+ T cell infiltration level was negatively correlated with the level of P4HA3 expression in most tumors. In addition, we found that macrophage expression in various tumors showed the strongest positive correlation with P4HA3 expression. A series of previous studies have also demonstrated that tumor-associated macrophages (TAMs) play an immunosuppressive role in tumor development and are associated with poor tumor prognosis (Kersten et al., 2022). TAMs can promote tumor growth through the production of collagen (Qiu et al., 2019). Notably, in this study, we also found a significant positive correlation between the degree of CAFs infiltration and P4HA3 expression in pan-cancer. CAFs are a central component of TME and significantly affect cancer progression, metastasis, treatment, and prognosis (Lavie et al., 2022). They are involved in carcinogenesis as an essential cell type in the ECM that allows collagen deposition and even leads to tumor fibrosis (Lambrechts et al., 2018). These results suggest that P4HA3 may play an essential role in the tumor immune microenvironment.
It is understood that the effectiveness of ICIs is mainly influenced by TMB and MSI (Postow et al., 2015; Dudley et al., 2016). Moreover, many studies also suggest that TMB and MSI may be potential predictive markers associated with tumor immunotherapy response and drug resistance (Yarchoan et al., 2017; Hellmann et al., 2018; Chae et al., 2019; Zhao et al., 2019). MMR Gene mutation status can predict tumor development (Cerretelli et al., 2020). CNA has been recognized as an essential source of genetic variation. In addition, both TMB and CNA are good predictors of the efficacy of ICIs, and the higher the predictive accuracy of the two combined (Liu et al., 2019). Here, we will evaluate the correlation of P4HA3 expression with tumor TMB, MSI, MMR Gene, CNA, and mutational status. Among them, elevated P4HA3 expression levels upregulated TMB of UCS, THYM, PRAD, LUAD, LGG, and LAML, as well as MSI of UCEC, PRAD, MESO, COAD, and STAD. In human cancers, P4HA3 expression was significantly associated with five MMR genes, especially in LAML and THCA. We found a P4HA3 gene variation rate of 2.6% in all TCGA tumors, dominated by missense mutations. In addition, epigenetic modifications are one of the main reasons affecting the effectiveness of various tumor immunotherapies, such as ICIs and immune cell therapies (Zebley et al., 2020). DNA methylation modifications are known to be one of the most important and well-studied epigenetic modifications (Skvortsova et al., 2019). DNA methyltransferases (DNMT) catalyze DNA methylation and are key epigenetic targets for new drug development (Lyko and Brown, 2005). After analysis, P4HA3 expression was positively correlated with DNA methyltransferases in most tumors, especially in LAML. Downregulation of C-P4H in lymphoma is associated with DNA methylation (Hatzimichael et al., 2012). In addition to the most intensively studied DNA methylation, RNA methylation modifications have been increasingly recognized as an essential element of epigenetics in recent years (Roundtree et al., 2017). RNA modifications are widely present in the fundamental biological processes required for cancer development and are closely associated with tumor progression, including N1-methyladenosine (M1A), 5-methylcytosine (M5C), and N6-methyladenosine (M6A) (Zhao et al., 2017). We found that P4HA3 was significantly associated with m1A, m5c, and m6A-related genes in most tumors, especially m6A-related genes. For example, P4HA3 expression was positively correlated with the expression of more than 20 m6A RNA modification regulators in LUAD, BRCA, COADREAD, PAAD, LIHC, OV, THCA, UCEC, KIPAN, UVM, KICH, and LAML. Many previous studies have shown that m6A-related genes are promising targets for cancer immunotherapy (Han et al., 2019; Guo et al., 2021; Zhao et al., 2021). In addition, abnormal m6A methylation can affect the expression levels of tumor target genes (Guo et al., 2021). In summary, P4HA3 may be involved in tumor development by regulating genetic mutation status or epigenetic modifications. Its expression level can be used as a potential predictor to assess the efficacy of immunotherapy in cancer patients.
The ability of human stem cells (SCs) to self-renew and differentiate into mature cells is called stemness (Phi et al., 2018). Many studies have found that cancer stem cells (CSCs) are associated with tumor proliferation, metastasis, recurrence, and drug resistance (Ayob and Ramasamy, 2018; Kuşoğlu and Biray, 2019). Here, we found that P4HA3 was correlated with the dryness index. These findings may help us identify new biomarkers that can predict tumor progression, guide more targeted treatment strategies, and predict prognosis. We performed functional enrichment analysis to understand the potential molecular functions of P4HA3 and related signaling pathways. The results suggest that P4HA3 is closely associated with various biological processes. Among them, P4HA3 was most significantly correlated with epithelial-mesenchymal transition. Many previous studies have shown that P4HA3 promotes tumor cell growth, proliferation, and metastasis in HNSC by activating the EMT process (Wang et al., 2020). Targeting P4HA3 inhibits EMT in colon cancer (Zhou et al., 2022a). TGF-β enables cancer cell invasion and metastasis and inhibits the anti-tumor activity of immune cells (Derynck et al., 2021). TGF-β stimulation increases P4HA3 expression, which is associated with EMT in non-small cell lung cancer (Nakasuka et al., 2021). P4HA3 reverses the inhibitory effect of COL6A6 on EMT in pituitary adenoma (PA) (Long et al., 2019). Reduced levels of P4HA3 expression significantly inhibited the EMT process in colon cancer (Zhou et al., 2022b). Numerous studies have confirmed that EMT is closely related to tumor metastasis (Zhang et al., 2019; Gaballa et al., 2020; Cai et al., 2021). This evidence, combined with our current findings, further demonstrates that P4HA3 may be involved in tumor progression in pan-cancer through multiple biological processes with EMT as the primary role. Finally, we also analyzed the relationship between P4HA3 and drug sensitivity. Twenty drugs were found to be associated with P4HA3.
Our findings suggest that P4HA3 is expressed at high levels in most cancers, which correlates with the spite of cancers and poor prognosis. We can also assess the effect of tumor immunotherapy, tumor immunosuppression, and drug resistance based on the expression of P4HA3. In addition, P4HA3 can affect the infiltration of tumor immune cells and is likely to promote cancer progression through EMT. Therefore, P4HA3 may serve as a promising biomarker for human cancer diagnosis, treatment, and prognosis and may predict the efficacy of anti-tumor immunotherapy response. This study may provide ideas for future researchers to study the role of P4HA3 in human cancers.
Of course, there are some limitations to this study. For example, our study was limited to bioinformatics analysis, which remains to be further validated by basic experiments or clinical trials. In addition, although the present study identified that P4HA3 might affect cancer by participating in some potential signaling pathways, the specific molecular biology of the mechanism of action is not yet clear. These need to be further investigated in depth in the future.
Data availability statement
The original contributions presented in the study are included in the article/Supplementary Material, further inquiries can be directed to the corresponding authors.
Author contributions
SZ, RW, BZ, JN, YL, and YW substantially contributed to conception and design, SZ, RW, and YW conducted the literature search. RW, BZ, and YW contributed to acquisition of data, or analysis and interpretation of data; YW wrote the article. RW and BZ performed data analysis and drafted. SZ and RW revised the article. RAR directed the revisions and touch-ups. All authors gave the final approval of the version to be submitted.
Funding
This work was supported by the National Nature and Science Foundation of China (No. 81901394).
Acknowledgments
We thank the public database TCGA, xiantaoxueshu, xena, UCSCXenaShiny, Sangerbox tools for its assistance in our research.
Conflict of interest
The authors declare that the research was conducted in the absence of any commercial or financial relationships that could be construed as a potential conflict of interest.
Publisher’s note
All claims expressed in this article are solely those of the authors and do not necessarily represent those of their affiliated organizations, or those of the publisher, the editors and the reviewers. Any product that may be evaluated in this article, or claim that may be made by its manufacturer, is not guaranteed or endorsed by the publisher.
Supplementary material
The Supplementary Material for this article can be found online at: https://www.frontiersin.org/articles/10.3389/fgene.2023.1045061/full#supplementary-material
References
Armstrong, T., Packham, G., Murphy, L. B., Bateman, A. C., Conti, J. A., Fine, D. R., et al. (2004). Type I collagen promotes the malignant phenotype of pancreatic ductal adenocarcinoma. Clin. cancer Res. official J. Am. Assoc. Cancer Res. 10 (21), 7427–7437. doi:10.1158/1078-0432.CCR-03-0825
Atkinson, A., Renziehausen, A., Wang, H., Lo Nigro, C., Lattanzio, L., Merlano, M., et al. (2019). Collagen prolyl hydroxylases are bifunctional growth regulators in melanoma. J. investigative dermatology 139 (5), 1118–1126. doi:10.1016/j.jid.2018.10.038
Ayob, A. Z., and Ramasamy, T. S. (2018). Cancer stem cells as key drivers of tumour progression. J. Biomed. Sci. 25 (1), 20. doi:10.1186/s12929-018-0426-4
Bai, X., Wu, D. H., Ma, S. C., Wang, J., Tang, X. R., Kang, S., et al. (2020). Development and validation of a genomic mutation signature to predict response to PD-1 inhibitors in non-squamous NSCLC: A multicohort study. J. Immunother. cancer 8 (1), e000381. doi:10.1136/jitc-2019-000381
Bonnans, C., Chou, J., and Werb, Z. (2014). Remodelling the extracellular matrix in development and disease. Nat. Rev. Mol. Cell Biol. 15 (12), 786–801. doi:10.1038/nrm3904
Cai, J., Gong, L., Li, G., Guo, J., Yi, X., and Wang, Z. (2021). Exosomes in ovarian cancer ascites promote epithelial-mesenchymal transition of ovarian cancer cells by delivery of miR-6780b-5p. Cell death Dis. 12 (2), 210. doi:10.1038/s41419-021-03490-5
Cerretelli, G., Ager, A., Arends, M. J., and Frayling, I. M. (2020). Molecular pathology of Lynch syndrome. J. pathology 250 (5), 518–531. doi:10.1002/path.5422
Chae, Y. K., Davis, A. A., Raparia, K., Agte, S., Pan, A., Mohindra, N., et al. (2019). Association of tumor mutational burden with DNA repair mutations and response to anti-PD-1/PD-L1 therapy in non-small-cell lung cancer. Clin. lung cancer 20 (2), 88–96.e6. doi:10.1016/j.cllc.2018.09.008
Chakravarthy, A., Khan, L., Bensler, N. P., Bose, P., and De Carvalho, D. D. (2018). TGF-β-associated extracellular matrix genes link cancer-associated fibroblasts to immune evasion and immunotherapy failure. Nat. Commun. 9 (1), 4692. doi:10.1038/s41467-018-06654-8
Chen, Y., Meng, Z., Zhang, L., and Liu, F. (2021). CD2 is a novel immune-related prognostic biomarker of invasive breast carcinoma that modulates the tumor microenvironment. Front. Immunol. 12, 664845. doi:10.3389/fimmu.2021.664845
Chen, Y., Yang, S., Tavormina, J., Tampe, D., Zeisberg, M., Wang, H., et al. (2022). Oncogenic collagen I homotrimers from cancer cells bind to α3β1 integrin and impact tumor microbiome and immunity to promote pancreatic cancer. Cancer Cell 40 (8), 818–834.e9. doi:10.1016/j.ccell.2022.06.011
D'Aniello, C., Cermola, F., Palamidessi, A., Wanderlingh, L. G., Gagliardi, M., Migliaccio, A., et al. (2019). Collagen prolyl hydroxylation-dependent metabolic perturbation governs epigenetic remodeling and mesenchymal transition in pluripotent and cancer cells. Cancer Res. 79 (13), 3235–3250. doi:10.1158/0008-5472.CAN-18-2070
Derynck, R., Turley, S. J., and Akhurst, R. J. (2021). TGFβ biology in cancer progression and immunotherapy. Nat. Rev. Clin. Oncol. 18 (1), 9–34. doi:10.1038/s41571-020-0403-1
Dudley, J. C., Lin, M. T., Le, D. T., and Eshleman, J. R. (2016). Microsatellite instability as a biomarker for PD-1 blockade. Clin. cancer Res. official J. Am. Assoc. Cancer Res. 22 (4), 813–820. doi:10.1158/1078-0432.CCR-15-1678
Gaballa, R., Ali, H. E. A., Mahmoud, M. O., Rhim, J. S., Ali, H. I., Salem, H. F., et al. (2020). Exosomes-Mediated transfer of Itga2 promotes migration and invasion of prostate cancer cells by inducing epithelial-mesenchymal transition. Cancers 12 (8), 2300. doi:10.3390/cancers12082300
Gjaltema, R. A., and Bank, R. A. (2017). Molecular insights into prolyl and lysyl hydroxylation of fibrillar collagens in health and disease. Crit. Rev. Biochem. Mol. Biol. 52 (1), 74–95. doi:10.1080/10409238.2016.1269716
Gorres, K. L., and Raines, R. T. (2010). Prolyl 4-hydroxylase. Crit. Rev. Biochem. Mol. Biol. 45 (2), 106–124. doi:10.3109/10409231003627991
Guo, L., Li, X., Liu, R., Chen, Y., Ren, C., and Du, S. (2020). TOX correlates with prognosis, immune infiltration, and T cells exhaustion in lung adenocarcinoma. Cancer Med. 9 (18), 6694–6709. doi:10.1002/cam4.3324
Guo, W., Tan, F., Huai, Q., Wang, Z., Shao, F., Zhang, G., et al. (2021). Comprehensive analysis of PD-L1 expression, immune infiltrates, and m6A RNA methylation regulators in esophageal squamous cell carcinoma. Front. Immunol. 12, 669750. doi:10.3389/fimmu.2021.669750
Han, D., Liu, J., Chen, C., Dong, L., Liu, Y., Chang, R., et al. (2019). Anti-tumour immunity controlled through mRNA m(6)A methylation and YTHDF1 in dendritic cells. Nature 566 (7743), 270–274. doi:10.1038/s41586-019-0916-x
Hatzimichael, E., Lo Nigro, C., Lattanzio, L., Syed, N., Shah, R., Dasoula, A., et al. (2012). The collagen prolyl hydroxylases are novel transcriptionally silenced genes in lymphoma. Br. J. cancer 107 (8), 1423–1432. doi:10.1038/bjc.2012.380
Helaakoski, T., Annunen, P., Vuori, K., MacNeil, I. A., Pihlajaniemi, T., and Kivirikko, K. I. (1995). Cloning, baculovirus expression, and characterization of a second mouse prolyl 4-hydroxylase alpha-subunit isoform: Formation of an alpha 2 beta 2 tetramer with the protein disulfide-isomerase/beta subunit. Proc. Natl. Acad. Sci. U. S. A. 92 (10), 4427–4431. doi:10.1073/pnas.92.10.4427
Hellmann, M. D., Ciuleanu, T. E., Pluzanski, A., Lee, J. S., Otterson, G. A., Audigier-Valette, C., et al. (2018). Nivolumab plus ipilimumab in lung cancer with a high tumor mutational burden. N. Engl. J. Med. 378 (22), 2093–2104. doi:10.1056/NEJMoa1801946
Hu, X., Zhu, H., Chen, B., He, X., Shen, Y., Zhang, X., et al. (2022). The oncogenic role of tubulin alpha-1c chain in human tumours. BMC cancer 22 (1), 498. doi:10.1186/s12885-022-09595-0
Huang, D., Shen, J., Zhai, L., Chen, H., Fei, J., Zhu, X., et al. (2021). Insights into the prognostic value and immunological role of NAAA in pan-cancer. Front. Immunol. 12, 812713. doi:10.3389/fimmu.2021.812713
Ito, K., and Murphy, D. (2013). Application of ggplot2 to pharmacometric graphics. CPT pharmacometrics Syst. Pharmacol. 2 (10), e79. doi:10.1038/psp.2013.56
Ju, Q., Li, X., Zhang, H., Yan, S., Li, Y., and Zhao, Y. (2020). NFE2L2 is a potential prognostic biomarker and is correlated with immune infiltration in brain lower grade glioma: A pan-cancer analysis. Oxidative Med. Cell. Longev. 2020, 3580719. doi:10.1155/2020/3580719
Kersten, K., Hu, K. H., Combes, A. J., Samad, B., Harwin, T., Ray, A., et al. (2022). Spatiotemporal co-dependency between macrophages and exhausted CD8(+) T cells in cancer. Cancer Cell 40 (6), 624–638.e9. doi:10.1016/j.ccell.2022.05.004
Koenig, A., Mueller, C., Hasel, C., Adler, G., and Menke, A. (2006). Collagen type I induces disruption of E-cadherin-mediated cell-cell contacts and promotes proliferation of pancreatic carcinoma cells. Cancer Res. 66 (9), 4662–4671. doi:10.1158/0008-5472.CAN-05-2804
Kuşoğlu, A., and Biray, A. Ç. (2019). Cancer stem cells: A brief review of the current status. Gene 681, 80–85. doi:10.1016/j.gene.2018.09.052
Lambrechts, D., Wauters, E., Boeckx, B., Aibar, S., Nittner, D., Burton, O., et al. (2018). Phenotype molding of stromal cells in the lung tumor microenvironment. Nat. Med. 24 (8), 1277–1289. doi:10.1038/s41591-018-0096-5
Lavie, D., Ben-Shmuel, A., Erez, N., and Scherz-Shouval, R. (2022). Cancer-associated fibroblasts in the single-cell era. Nat. cancer 3 (7), 793–807. doi:10.1038/s43018-022-00411-z
Liu, L., Bai, X., Wang, J., Tang, X. R., Wu, D. H., Du, S. S., et al. (2019). Combination of TMB and CNA stratifies prognostic and predictive responses to immunotherapy across metastatic cancer. Clin. cancer Res. official J. Am. Assoc. Cancer Res. 25 (24), 7413–7423. doi:10.1158/1078-0432.CCR-19-0558
Long, R., Liu, Z., Li, J., and Yu, H. (2019). COL6A6 interacted with P4HA3 to suppress the growth and metastasis of pituitary adenoma via blocking PI3K-Akt pathway. Aging 11 (20), 8845–8859. doi:10.18632/aging.102300
Lyko, F., and Brown, R. (2005). DNA methyltransferase inhibitors and the development of epigenetic cancer therapies. JNCI J. Natl. Cancer Inst. 97 (20), 1498–1506. doi:10.1093/jnci/dji311
Maag, J. L. V. (2018). gganatogram: An R package for modular visualisation of anatograms and tissues based on ggplot2. F1000Research 7, 1576. doi:10.12688/f1000research.16409.2
Malta, T. M., Sokolov, A., Gentles, A. J., Burzykowski, T., Poisson, L., Weinstein, J. N., et al. (2018). Machine learning identifies stemness features associated with oncogenic dedifferentiation. Cell 173 (2), 338–354.e15. doi:10.1016/j.cell.2018.03.034
Mariathasan, S., Turley, S. J., Nickles, D., Castiglioni, A., Yuen, K., Wang, Y., et al. (2018). TGFβ attenuates tumour response to PD-L1 blockade by contributing to exclusion of T cells. Nature 554 (7693), 544–548. doi:10.1038/nature25501
Nakasuka, F., Tabata, S., Sakamoto, T., Hirayama, A., Ebi, H., Yamada, T., et al. (2021). TGF-β-dependent reprogramming of amino acid metabolism induces epithelial-mesenchymal transition in non-small cell lung cancers. Commun. Biol. 4 (1), 782. doi:10.1038/s42003-021-02323-7
Peng, D. H., Rodriguez, B. L., Diao, L., Chen, L., Wang, J., Byers, L. A., et al. (2020). Collagen promotes anti-PD-1/PD-L1 resistance in cancer through LAIR1-dependent CD8(+) T cell exhaustion. Nat. Commun. 11 (1), 4520. doi:10.1038/s41467-020-18298-8
Peng, D. H., Ungewiss, C., Tong, P., Byers, L. A., Wang, J., Canales, J. R., et al. (2017). ZEB1 induces LOXL2-mediated collagen stabilization and deposition in the extracellular matrix to drive lung cancer invasion and metastasis. Oncogene 36 (14), 1925–1938. doi:10.1038/onc.2016.358
Phi, L. T. H., Sari, I. N., Yang, Y-G., Lee, S-H., Jun, N., Kim, K. S., et al. (2018). Cancer stem cells (CSCs) in drug resistance and their therapeutic implications in cancer treatment. Stem Cells Int. 2018, 5416923. doi:10.1155/2018/5416923
Postow, M. A., Callahan, M. K., and Wolchok, J. D. (2015). Immune checkpoint blockade in cancer therapy. J. Clin. Oncol. official J. Am. Soc. Clin. Oncol. 33 (17), 1974–1982. doi:10.1200/JCO.2014.59.4358
Qiu, S., Deng, L., Liao, X., Nie, L., Qi, F., Jin, K., et al. (2019). Tumor-associated macrophages promote bladder tumor growth through PI3K/AKT signal induced by collagen. Cancer Sci. 110 (7), 2110–2118. doi:10.1111/cas.14078
Roundtree, I. A., Evans, M. E., Pan, T., and He, C. (2017). Dynamic RNA modifications in gene expression regulation. Cell 169 (7), 1187–1200. doi:10.1016/j.cell.2017.05.045
Shintani, Y., Maeda, M., Chaika, N., Johnson, K. R., and Wheelock, M. J. (2008). Collagen I promotes epithelial-to-mesenchymal transition in lung cancer cells via transforming growth factor-beta signaling. Am. J. Respir. Cell Mol. Biol. 38 (1), 95–104. doi:10.1165/rcmb.2007-0071OC
Skvortsova, K., Stirzaker, C., and Taberlay, P. (2019). The DNA methylation landscape in cancer. Essays Biochem. 63 (6), 797–811. doi:10.1042/EBC20190037
Song, H., Liu, L., Song, Z., Ren, Y., Li, C., and Huo, J. (2018). P4HA3 is epigenetically activated by slug in gastric cancer and its deregulation is associated with enhanced metastasis and poor survival. Technol. cancer Res. Treat. 17, 1533033818796485. doi:10.1177/1533033818796485
Sung, H., Ferlay, J., Siegel, R. L., Laversanne, M., Soerjomataram, I., Jemal, A., et al. (2021). Global cancer statistics 2020: GLOBOCAN estimates of incidence and mortality worldwide for 36 cancers in 185 countries. CA a cancer J. Clin. 71 (3), 209–249. doi:10.3322/caac.21660
Van Den Diepstraten, C., Papay, K., Bolender, Z., Brown, A., and Pickering, J. G. (2003). Cloning of a novel prolyl 4-hydroxylase subunit expressed in the fibrous cap of human atherosclerotic plaque. Circulation 108 (5), 508–511. doi:10.1161/01.CIR.0000080883.53863.5C
Vasta, J. D., and Raines, R. T. (2018). Collagen prolyl 4-hydroxylase as a therapeutic target. J. Med. Chem. 61 (23), 10403–10411. doi:10.1021/acs.jmedchem.8b00822
Vuori, K., Pihlajaniemi, T., Marttila, M., and Kivirikko, K. I. (1992). Characterization of the human prolyl 4-hydroxylase tetramer and its multifunctional protein disulfide-isomerase subunit synthesized in a baculovirus expression system. Proc. Natl. Acad. Sci. U. S. A. 89 (16), 7467–7470. doi:10.1073/pnas.89.16.7467
Wang, T., Wang, Y. X., Dong, Y. Q., Yu, Y. L., and Ma, K. (2020). Prolyl 4-hydroxylase subunit alpha 3 presents a cancer promotive function in head and neck squamous cell carcinoma via regulating epithelial-mesenchymal transition. Arch. Oral Biol. 113, 104711. doi:10.1016/j.archoralbio.2020.104711
Weinstein, J. N., Collisson, E. A., Mills, G. B., Shaw, K. R., Ozenberger, B. A., Ellrott, K., et al. (2013). The cancer Genome Atlas pan-cancer analysis project. Nat. Genet. 45 (10), 1113–1120. doi:10.1038/ng.2764
Whatcott, C. J., Diep, C. H., Jiang, P., Watanabe, A., LoBello, J., Sima, C., et al. (2015). Desmoplasia in primary tumors and metastatic lesions of pancreatic cancer. Clin. cancer Res. official J. Am. Assoc. Cancer Res. 21 (15), 3561–3568. doi:10.1158/1078-0432.CCR-14-1051
Xiong, G-F., and Xu, R. (2016). Function of cancer cell-derived extracellular matrix in tumor progression. J. Cancer Metastasis Treat. 2, 357–364. doi:10.20517/2394-4722.2016.08
Yamauchi, M., Barker, T. H., Gibbons, D. L., and Kurie, J. M. (2018). The fibrotic tumor stroma. J. Clin. investigation 128 (1), 16–25. doi:10.1172/JCI93554
Yamazaki, S., Higuchi, Y., Ishibashi, M., Hashimoto, H., Yasunaga, M., Matsumura, Y., et al. (2018). Collagen type I induces EGFR-TKI resistance in EGFR-mutated cancer cells by mTOR activation through Akt-independent pathway. Cancer Sci. 109 (6), 2063–2073. doi:10.1111/cas.13624
Yang, M. C., Wang, C. J., Liao, P. C., Yen, C. J., and Shan, Y. S. (2014). Hepatic stellate cells secretes type I collagen to trigger epithelial mesenchymal transition of hepatoma cells. Am. J. cancer Res. 4 (6), 751–763.
Yarchoan, M., Hopkins, A., and Jaffee, E. M. (2017). Tumor mutational burden and response rate to PD-1 inhibition. N. Engl. J. Med. 377 (25), 2500–2501. doi:10.1056/NEJMc1713444
Yu, G., Wang, L. G., Han, Y., and He, Q. Y. (2012). clusterProfiler: an R package for comparing biological themes among gene clusters. Omics a J. Integr. Biol. 16 (5), 284–287. doi:10.1089/omi.2011.0118
Zebley, C. C., Gottschalk, S., and Youngblood, B. (2020). Rewriting history: Epigenetic reprogramming of CD8(+) T cell differentiation to enhance immunotherapy. Trends Immunol. 41 (8), 665–675. doi:10.1016/j.it.2020.06.008
Zhang, X., Sai, B., Wang, F., Wang, L., Wang, Y., Zheng, L., et al. (2019). Hypoxic BMSC-derived exosomal miRNAs promote metastasis of lung cancer cells via STAT3-induced EMT. Mol. cancer 18 (1), 40. doi:10.1186/s12943-019-0959-5
Zhao, B. S., Roundtree, I. A., and He, C. (2017). Post-transcriptional gene regulation by mRNA modifications. Nat. Rev. Mol. Cell Biol. 18 (1), 31–42. doi:10.1038/nrm.2016.132
Zhao, H., Xu, Y., Xie, Y., Zhang, L., Gao, M., Li, S., et al. (2021). m6A regulators is differently expressed and correlated with immune response of esophageal cancer. Front. Cell Dev. Biol. 9, 650023. doi:10.3389/fcell.2021.650023
Zhao, P., Li, L., Jiang, X., and Li, Q. (2019). Mismatch repair deficiency/microsatellite instability-high as a predictor for anti-PD-1/PD-L1 immunotherapy efficacy. J. Hematol. Oncol. 12 (1), 54. doi:10.1186/s13045-019-0738-1
Zheng, X., Liu, W., Xiang, J., Liu, P., Ke, M., Wang, B., et al. (2017). Collagen I promotes hepatocellular carcinoma cell proliferation by regulating integrin β1/FAK signaling pathway in nonalcoholic fatty liver. Oncotarget 8 (56), 95586–95595. doi:10.18632/oncotarget.21525
Zhou, H., Huang, S., Shao, C., Zou, J., Zhou, A., Yu, J., et al. (2022). miR-1266-3p suppresses epithelial-mesenchymal transition in colon cancer by targeting P4HA3. Anal. Cell. Pathol. Amst. 2022, 1542117. doi:10.1155/2022/1542117
Zhou, H., Zou, J., Shao, C., Zhou, A., Yu, J., Chen, S., et al. (2022). Prolyl 4-hydroxylase subunit alpha 3 facilitates human colon cancer growth and metastasis through the TGF-β/Smad signaling pathway. Pathology, Res. Pract. 230, 153749. doi:10.1016/j.prp.2021.153749
Keywords: prolyl 4-hydroxylase subunit alpha 3 (P4HA3), pan-cancer, the tumor microenvironment (TME), collagen, immunotherapy, epithelial-mesenchymal transition (EMT)
Citation: Wu Y, Zhang B, Nong J, Rodrìguez RA, Guo W, Liu Y, Zhao S and Wei R (2023) Systematic pan-cancer analysis of the potential tumor diagnosis and prognosis biomarker P4HA3. Front. Genet. 14:1045061. doi: 10.3389/fgene.2023.1045061
Received: 15 September 2022; Accepted: 13 March 2023;
Published: 22 March 2023.
Edited by:
James Hicks, University of Southern California, United StatesReviewed by:
Hua Zhu, Renmin Hospital of Wuhan University, ChinaChaoyun Yang, Xichang College, China
Copyright © 2023 Wu, Zhang, Nong, Rodrìguez, Guo, Liu, Zhao and Wei. This is an open-access article distributed under the terms of the Creative Commons Attribution License (CC BY). The use, distribution or reproduction in other forums is permitted, provided the original author(s) and the copyright owner(s) are credited and that the original publication in this journal is cited, in accordance with accepted academic practice. No use, distribution or reproduction is permitted which does not comply with these terms.
*Correspondence: Ruqiong Wei, d2VpcnVxaW9uZ3NwYWluQDE2My5jb20=; Shijian Zhao, emhhb3NoaWppYW4xMDI1QDE2My5jb20=
†These authors have contributed equally to this work and share first authorship