- 1Graduate School, Ning Xia Medical University, Yinchuan, China
- 2General Surgery Clinical Medical Center, Gansu Provincial Hospital, Lanzhou, China
- 3Key Laboratory of Molecular Diagnostics and Precision Medicine for Surgical Oncology in Gansu Province, Gansu Provincial Hospital, Gansu, China
- 4NHC Key Laboratory of Diagnosis and Therapy of Gastrointestinal Tumor, Gansu Provincial Hospital, Lanzhou, China
- 5The First Clinical Medical College of Lanzhou University, Lanzhou, China
Background: Dual homeoboxes A pseudogene 8 (DUXAP8) is a newly discovered long noncoding RNA that has been shown to function as an oncogene in a variety of human malignant cancers. By integrating available data, this meta-analysis sought to determine the relationship between clinical prognosis and DUXAP8 expression levels in diverse malignancies.
Materials and methods: A systematic search was performed to identify eligible studies from several electronic databases from their inception to 25 October 2021. Pooled odds ratios and hazard ratios with 95% CI were used to estimate the association between DUXAP8 expression and survival. For survival analysis, the Kaplan-Meier method and COX analysis were used. Furthermore, we utilized Spearman’s correlation analysis to explore the correlation between DUXAP8 and tumor mutational burden (TMB), microsatellite instability (MSI), the related genes of mismatch repair (MMR), DNA methyltransferases (DNMTs), and immune checkpoint biomarkers.
Results: Our findings indicated that overexpression of DUXAP8 was related to poor overall survival (OS) (HR = 1.63, 95% CI, 1.49–1.77, p < 0.001). In addition, elevated DUXAP8 expression was closely related to poor OS in several cancers in the TCGA database. Moreover, DUXAP8 expression has been associated with TMB, MSI, and MMR in a variety of malignancies.
Conclusion: This study revealed that DUXAP8 might serve as a prognostic biomarker and potential therapeutic target for cancer. It can be used to improve cancer diagnosis, discover potential treatment targets, and improve prognosis.
Introduction
Cancer-related deaths have risen dramatically in recent years. Progress in anticancer drug delivery has resulted in tremendous improvements in cancer treatment outcomes, yet cancer survivors’ quality of life and prognosis remains dismal (Bahrami et al., 2018). In part, this is due to the lack of reliable biomarkers for the early identification of the majority of malignancies. In recent years, molecular biomarkers for multiple carcinomas have become more prevalent and may provide further clues for following the disease’s progression (Ilie et al., 2018). Consequently, it is imperative to seek new cancer markers to better characterize the clinical stage, metastasis, and prognosis of most malignancies at an earlier and more accurate point in time (Wang et al., 2021a, 2021b).
An RNA molecule that is more than 200 nucleotides in length but lacks an open reading frame is referred to as Long non-coding RNA (lncRNA) (Johnsson et al., 2014). It has emerged that lncRNAs have a role in a wide range of physiological and pathological processes. Epigenetic regulation, transcriptional and posttranscriptional regulation are only a few of the roles of lncRNAs in diseases (Fan et al., 2017). LncRNAs may play a critical role in the progression of cancer, as evidenced by recent research (Wang et al., 2021a). Collectively, lncRNAs serve as promising markers for cancer patients (Ma et al., 2017a).
Double homeobox A pseudogene 8 (DUXAP8) is a recently discovered lncRNA on 22q11.1. DUXAP8 has a length of around 2,307 bp. DUXAP8 is significantly overexpressed in cancer tissues compared to nearby non-tumor tissues, according to observations (Ma et al., 2017b; Du et al., 2019; Chen et al., 2020a; Chen et al., 2020b; He et al., 2020; Yin et al., 2020; Chen et al., 2021a). DUXAP8 exerts an essential role in tumorigenesis, proliferation, migration, invasion, and inhibition of apoptosis, which means that DUXAP8 acts as an oncogene in the occurrence and development of various malignant tumors (Jiang et al., 2019; Hu et al., 2020; Wang et al., 2020; Wei et al., 2020; Zhang et al., 2020; Guan et al., 2021). In addition, high-quality meta-analysis has been increasingly considered one of the keys and significant tools for achieving evidence (Yao et al., 2016; Tian et al., 2017; Li et al., 2018; Yang, 2018; Yang et al., 2018; Yan et al., 2019).
Thus, we performed this meta-analysis for the first time to explore the clinical prognostic role and functions of DUXAP8 in human cancers. In addition, we employed data mining to investigate the prognostic value of DUXAP8 in a range of tumor types to further validate our results. This study included an in-depth analysis of DUXAP8 expression levels, as well as the relationship with tumor mutational burden (TMB), microsatellite instability (MSI), DNA methyltransferases (DNMTs), and mismatch repair (MMR).
Materials and Methods
Literature Search and Selection
We conducted a systematic search to identify relevant literature from its inception to 25 October 2021, including PubMed (Medline), Embase, and Cochrane Library. The retrieval words include: (“LINC DUXAP8” OR “LincRNA DUXAP8” OR “long non-coding RNA DUXAP8” OR “long noncoding RNA DUXAP8” OR “DUXAP8 lncRNA”) and (“cancer” OR “carcinoma” OR “tumor” OR “tumor” OR “neoplasm” OR “adenoma” OR “sarcoma” OR “melanoma”). Additionally, we searched the reference lists of the primary literature and reviews to find pertinent supplementary literature.
Inclusion and Exclusion Criteria
The inclusion criteria were: 1) articles to study the clinical functions of DUXAP8 in different cancer tissues; 2) clinical trials in which patients were separated into two groups based on their DUXAP8 expression levels; 3) studies that provided OS; 4) studies with sufficient data to generate HR and 95% confidence intervals (CI) or Kaplan-Meier curves; 5) case-control studies. The eliminated criteria included the following content: 1) studies on DUXAP8’s structure and functions; 2) nonhuman studies, reviews, editorials, specialist opinions, letters along with case reports; 3) studies having insufficient original data for survival analysis.
Data Extraction and Quality Assessment
Two researchers independently assessed and obtained all the necessary data from the selected literature. The data extracted from each selected study are shown in Supplementary Table S1. If the relevant data were not directly accessible and only the Kaplan–Meier curves had been provided, we extracted the survival rates from the survival plot graphs and computed the HR, and the 95% CI indirectly (Parmar et al., 1998a; Parmar et al., 1998b). The Newcastle Ottawa Score (NOS) was used to evaluate the quality of the included studies (Stang, 2010). A NOS score of ≥6 indicates a high-quality study.
Analysis of DUXAP8 Expression in Cancer
UCSC Xena, derived from the TCGA database (https://xena.ucsc.edu/), provided us with data on 33 tumors, including RNA sequences, somatic mutations, clinicopathological characteristics, and survival rates. The cell line expression matrix was obtained from the CCLE dataset (https://portals.broadinstitute.org/ccle/about). We use the “Wilcox. test” to determine the difference in DUXAP8 expression levels between tumor and normal tissues in various cancer types. Adrenocortical Carcinoma (ACC), Bladder Urothelial Carcinoma (BLCA), Breast invasive carcinoma (BRCA), Cervical squamous cell carcinoma and endocervical adenocarcinoma (CESC), Cholangiocarcinoma (CHOL), Colon adenocarcinoma (COAD), Lymphoid Neoplasm Diffuse Large B-cell Lymphoma (DLBC), Esophageal carcinoma (ESCA), Glioblastoma multiforme (GBM), Head and Neck squamous cell carcinoma (HNSC), Kidney Chromophobe (KICH), Kidney renal clear cell carcinoma (KIRC), Kidney renal papillary cell carcinoma (KIRP), Acute Myeloid Leukemia (LAML), Brain Lower Grade Glioma (LGG), Liver hepatocellular carcinoma (LIHC), Lung adenocarcinoma (LUAD), Lung squamous cell carcinoma (LUSC), Mesothelioma (MESO), Ovarian serous cystadenocarcinoma (OV), Pancreatic adenocarcinoma (PAAD), Pheochromocytoma and Paraganglioma (PCPG), Prostate adenocarcinoma (PRAD), Rectum adenocarcinoma (READ), Sarcoma (SARC), Skin Cutaneous Melanoma (SKCM), Stomach adenocarcinoma (STAD), Testicular Germ Cell Tumors (TGCT), Thyroid carcinoma (THCA), Thymoma (THYM), Uterine Corpus Endometrial Carcinoma (UCEC), Uterine Carcinosarcoma (UCS), Uveal Melanoma (UVM).
Survival Analysis
We analyzed the relationships between DUXAP8 expression and OS, disease-free interval (DFI), disease-specific survival (DSS), progression-free interval (PFI), age, and clinical stage. For survival analysis, the Kaplan-Meier method and COX analysis were used.
Correlation of DUXAP8 Expression With Tumor Mutational Burden, Microsatellite Instability, DNA Methyltransferases, and Mismatch Repair
TMB is defined as the total number of mutations per megabase of DNA. MSI is the spontaneous loss or gain of nucleotides from short tandem repeat DNA tracts. We utilized Spearman’s correlation analysis to explore the correlation between DUXAP8 and TMB, MSI, the related genes of MMR, DNMTs, and immune checkpoint biomarkers. The resulting heatmap was implemented by using the R-packages “reshape2” and “RColorBrewer”.
Pathway Analysis of DUXAP8
Downloaded gene sets from the Gene Set Enrichment Analysis (GSEA) website (https://www.gsea-msigdb.org/gsea/downloads.jsp) were used in the study. R-package “limma,” “org.Hs.eg.db,” “clusterProfiler,” and “enrichplot” were used to perform both Gene ontology (GO) and Kyoto Encyclopedia of Genes and Genomes (KEGG) of DUXAP8 respectively.
Data Synthesis and Statistical Analysis
The survival result was produced by employing information from the HR and the standard error (SE). During this meta-analysis, HRs were pooled by employing I2 statistics to examine the heterogeneity of the applicable studies. The random-effects model was then used only if there was significant statistical heterogeneity between the studies (chi-squared test, p < 0.1, I2 > 50%). We utilized the fixed-effects model (chi-squared test, p > 0.1, I2 < 50%) where it was not applicable (Zhai et al., 2021). To show the meta-analysis outcomes, we employed forest plots. We used Begg’s test to see if there was any publication bias, and sensitivity analysis to see if the results were consistent. We applied STATA12.0, the R software to integrate and analyze the data. p < 0.05 was considered statistically significant.
Results
Studies Characteristics
Supplementary Figure S1 demonstrates the details concerning the screening process. A systematic search of the databases identified 114 studies published up to 25 October 2021. We excluded duplicate studies, studies irrelevant to the research subject, and studies that did not provide sufficient data. Therefore, there are a total of 25 studies that meet the final analysis conditions (Ma et al., 2017b; Xu et al., 2017; Lian et al., 2018; Lin et al., 2018; Du et al., 2019; Jiang et al., 2019; Chen et al., 2020a; Chen et al., 2020b; He et al., 2020; Hu et al., 2020; Nie et al., 2020; Wang et al., 2020; Wei et al., 2020; Yin et al., 2020; Zhang et al., 2020; Zhao et al., 2020; Chen et al., 2021a; Li et al., 2021a; Arabpour et al., 2021; Yang et al., 2021a; Chen et al., 2021b; Guan et al., 2021; Pang and Yang, 2021; Xing et al., 2021; Zhai et al., 2021). Moreover, the main features of the included studies have been summarized in Supplementary Table S1. The sample size of the 25 studies ranged between 31 and 522, with an average of 198.
All the included studies from 2016 to 2021 have been implemented and published in China except one study carried out in America. In total, 17 cancer types were included in our study: gastric cancer (GC) (Ma et al., 2017b), non-small cell lung cancer (NSCLC) (Yin et al., 2020; Chen et al., 2021a), cervical cancer (CC) (Chen et al., 2020b), oral cancer (OC) (Chen et al., 2020a), colorectal cancer (CRC) (Du et al., 2019; He et al., 2020), papillary thyroid carcinoma (PTC) (Pang and Yang, 2021), LGG (Zhao et al., 2020), PAAD (Lian et al., 2018), hepatocellular carcinoma (HCC) (Jiang et al., 2019; Hu et al., 2020; Wang et al., 2020; Wei et al., 2020; Zhang et al., 2020; Guan et al., 2021), acute myeloid Leukemia (AML) (Zhai et al., 2021), BLCA (Lin et al., 2018), KIRC (Xing et al., 2021), neuroblastoma (Nie et al., 2020), ovarian cancer (Li et al., 2021a), osteosarcoma (Yang et al., 2021a), renal cell carcinoma (RCC) (Xu et al., 2017), melanoma (Chen et al., 2021b), breast cancer (BC) (Arabpour et al., 2021).
Correlation of the DUXAP8 Expression Level With the Overall Survival
There were 25 studies (Ma et al., 2017b; Xu et al., 2017; Lian et al., 2018; Lin et al., 2018; Du et al., 2019; Jiang et al., 2019; Chen et al., 2020a; Chen et al., 2020b; He et al., 2020; Hu et al., 2020; Nie et al., 2020; Wang et al., 2020; Wei et al., 2020; Yin et al., 2020; Zhang et al., 2020; Zhao et al., 2020; Chen et al., 2021a; Li et al., 2021a; Arabpour et al., 2021; Yang et al., 2021a; Chen et al., 2021b; Guan et al., 2021; Pang and Yang, 2021; Xing et al., 2021; Zhai et al., 2021), consisting of 4,757 patients, included for OS analysis. A correlation analysis has been performed to explore between DUXAP8 and the poor OS in patients diagnosed with cancer. It applied the fixed effect model to the studies (I2 = 15.1%, PQ= 0.248). As illustrated in Figure 1A, there was a pooled HR = 1.63 between DUXAP8 and the OS (95% CI, 1.49–1.77, p < 0.001), revealing significantly worse OS in the cancer patients with high expression of DUXAP8.
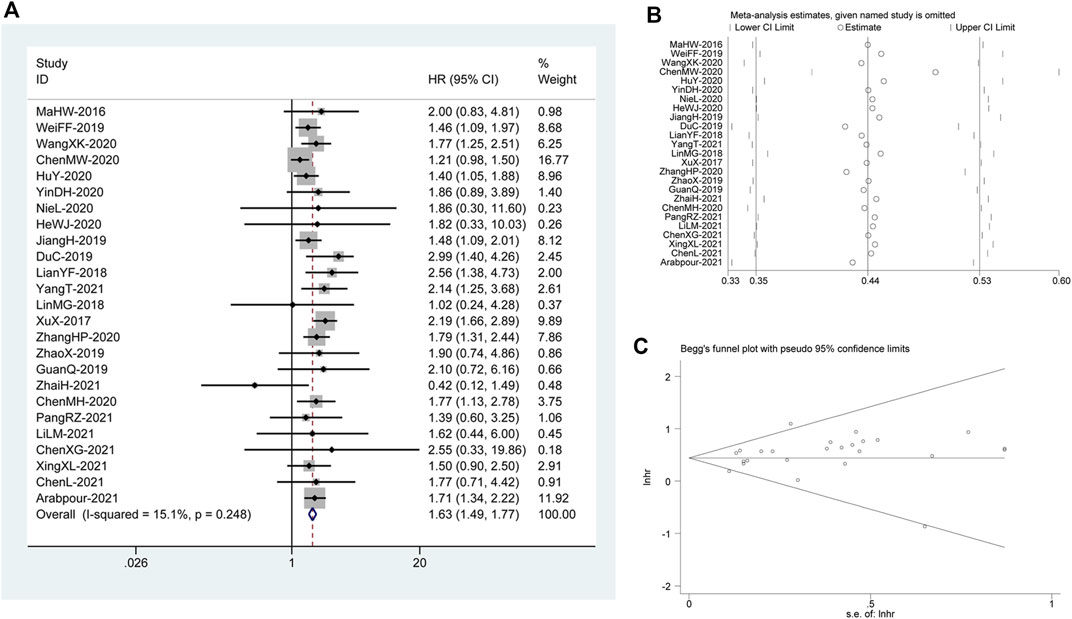
FIGURE 1. (A) Forest plot reflecting the association between OS and DUXAP8 expression level in cancer. (B) Sensitivity analysis for studies about OS. (C): Begg’s funnel plot of DUXAP8 for overall survival.
Publication Bias and Sensitivity Analysis
We constructed Begg’s funnel plot to assess publication bias among the reviews. There was no indication of noticeable OS disparity (p>|t| = 0.164; Figure 1C). In addition, we ran a sensitivity analysis after discarding each paper to confirm the validity of the relationship between DUXAP8 expression and OS. This analysis showed no significant change in the results. Therefore, the meta-analysis results were trustworthy. (Figure 1B).
Subgroup Analysis of the Relationship Between DUXAP8 Expression Level and Overall Survival
Based on the following factors, subgroup analysis was done to evaluate the relationship between DUXAP8 expression levels and OS: follow-up time (<60 or ≥60 months) (Figure 2A), the system of cancer (digestive system, urogenital system, respiratory system, hematologic system or other) (Figure 2B), sample size (<100 or ≥100 tissues) (Figure 2C), sample source (clinical samples or database) (Figure 2D), the quality of included literature (NOS scores) (Figure 2E), and type of cancer (Figure 2F). In these malignancies, the outcomes of the subgroup analysis didn’t change the predictive value of DUXAP8 for OS.
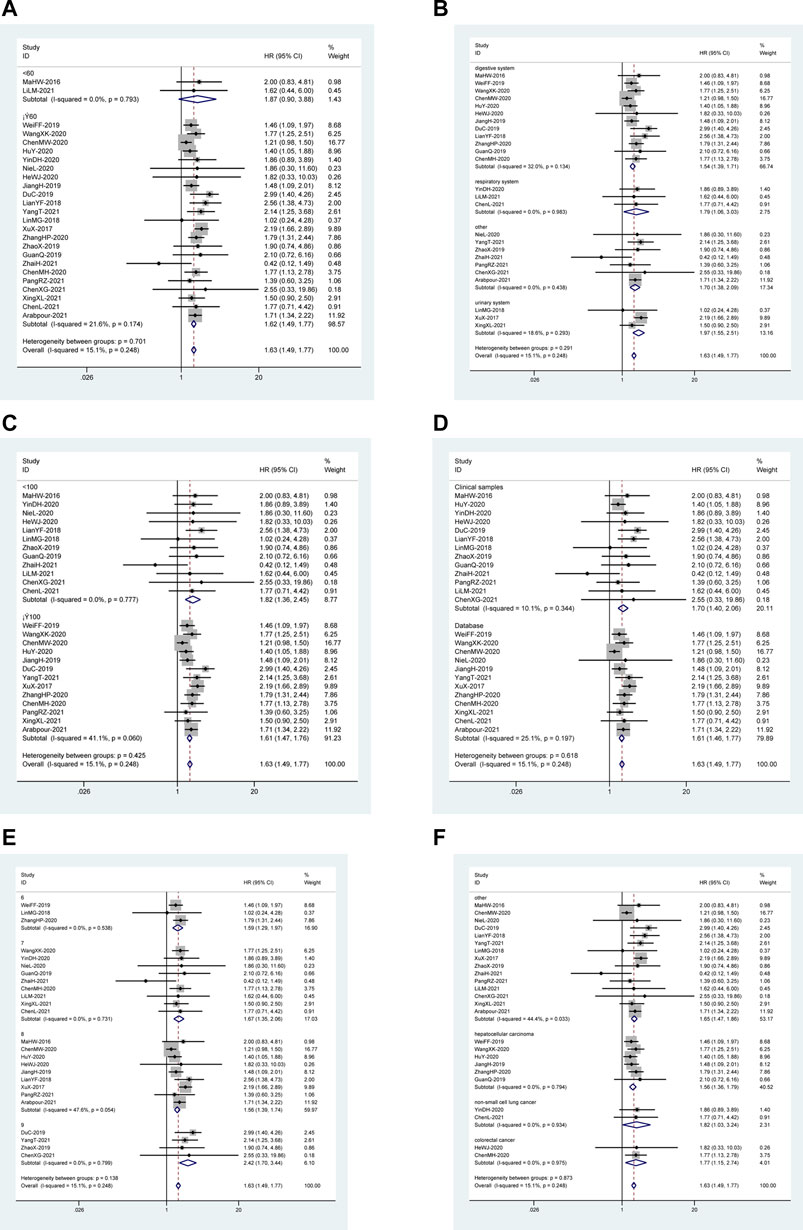
FIGURE 2. Forest plot reflecting the association between OS and lncRNA DUXAP8 expression level in cancer. (A): Subgroup analysis stratified by follow-up time. (B): Subgroup analysis stratified by the system of cancer. (C): Subgroup analysis stratified by sample size. (D): Subgroup analysis stratified by sample source. (E): Subgroup analysis stratified by NOS score. (F): Subgroup analysis stratified by type of cancer.
Multifaceted Prognostic Value of DUXAP8 in Pan-Cancers
To assess DUXAP8’s ability to predict pan-cancer, we evaluated multiple datasets. We used COX analysis to evaluated the DUXAP8-related survival (OS, DSS, DFI, and PFI) (Figure 3). Thus, we discovered that DUXAP8 was a detrimental factor in ACC (OS: HR = 1.910; DSS: HR = 2.037; DFI: HR = 7.031; PFI: HR = 3.228), LIHC (OS: HR = 2.418; DSS: HR = 1.946; DFI: HR = 1.728; PFI:HR = 1.656), KIRP (OS: HR = 5.479; DSS: HR = 6.402; DFI: HR = 6.074; PFI:HR = 4.307), KIRC (OS: HR = 2.459; DSS: HR = 2.808; PFI:HR = 1.919), UCEC (OS: HR = 1.496; DFI: HR = 1.686, p < 0.001; PFI:HR = 1.453), KICH (OS: HR = 18.962; DSS: HR = 21.605; PFI:HR = 7.195), MESO (OS: HR = 1.776; DSS: HR = 1.844; PFI:HR = 2.016), COAD (OS: HR = 1.489; DFI: HR = 2.725; PFI:HR = 1.407), THCA (OS: HR = 3.028; DSS: HR = 3.566), STAD (DSS: HR = 1.395; PFI: HR = 1.301), PRAD (DFI: HR = 2.007; PFI: HR = 1.435), DLBC (OS: HR = 9.983), and HNSC (OS: HR = 1.244).
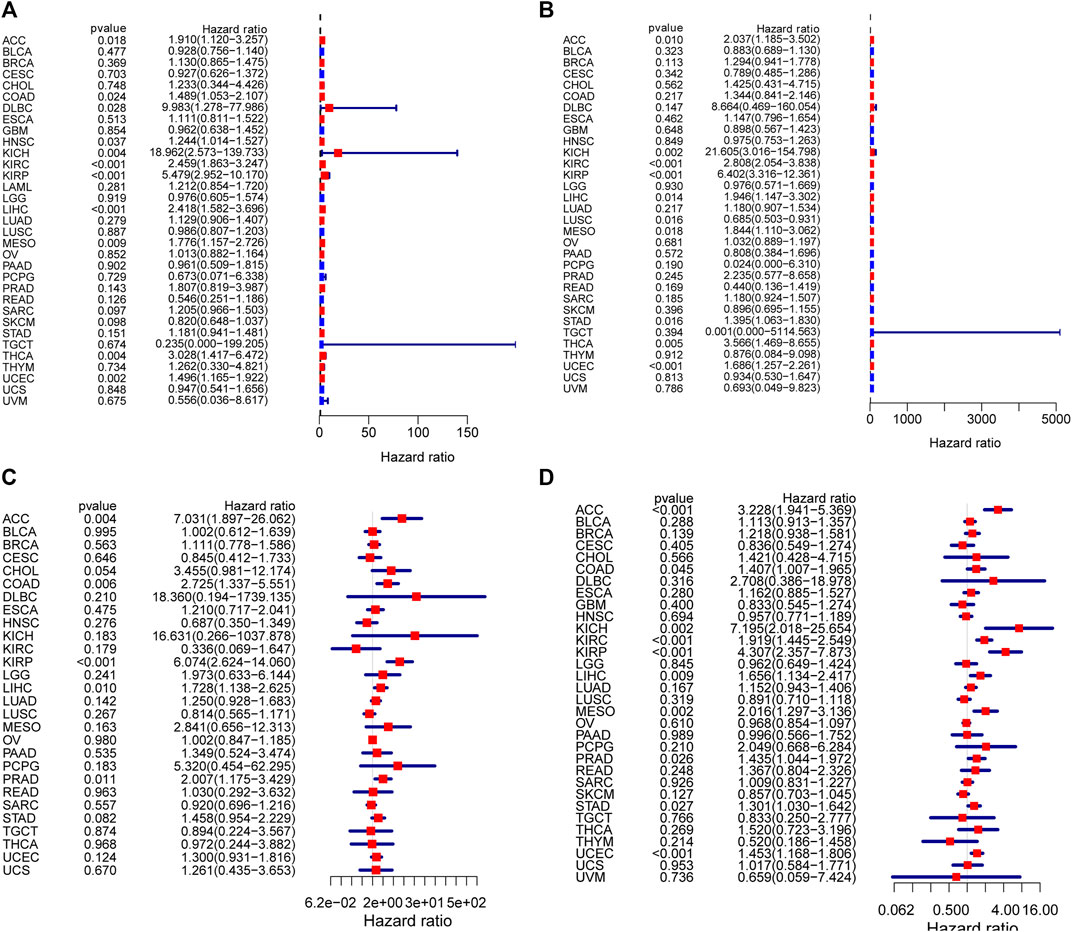
FIGURE 3. Correlation analysis of DUXAP8 expression with survival using the COX method for different types of cancers in TCGA. (A): OS. (B): DSS. (C): DFI. (D): PFI.
We next used Kaplan-Meier method to investigate the DUXAP8-related survival in the TCGA (Supplementary Figure S2). We discovered that low levels of DUXAP8 expression were associated with a poor prognosis, which included SKCM (OS: p = 0.003; DSS: p = 0.033; PFI: p = 0.002), READ (DSS: p = 0.041), LGG (PFI: p = 0.036). Conversely, high levels of DUXAP8 expression were associated with a poor prognosis in KIRC (OS: p < 0.001; DSS: p < 0.001; PFI: p = 0.001), KIRP (OS: p < 0.001; DSS: p = 0.001; PFI: p = 0.015), LIHC (OS: p = 0.001; DFI: p = 0.041; PFI: p = 0.020), UCEC (OS: p = 0.007; DSS: p = 0.022; PFI: p = 0.034), ACC (DSS: p = 0.028; PFI: p < 0.001), BRCA (DSS: p = 0.048), STAD (DSS: p = 0.033, COAD (DFI: p = 0.019), PRAD (DFI: p = 0.019), and MESO (PFI: p = 0.034).
We next used Kaplan-Meier plotter (https://kmplot.com/analysis) to evaluate DUXAP8-related survival (OS and RFS). Interestingly, we were able to verify that DUXAP8 had a protective prognostic role in ESCA (OS: HR = 0.39; RFS, HR = 0.38) (Figures 4A,B), and READ (OS: HR = 0.35) (Figure 4T). In contrast, DUXAP8 expression had a detrimental effect in HNSC (OS: HR = 1.38) (Figure 4C), KIRP (OS: HR = 3.4; RFS, HR = 2.47) (Figures 4E,F), LIHC (OS: HR = 2.15; RFS, HR = 1.54) (Figures 4G,H), LUAD (OS: HR = 1.38; RFS, HR = 1.61) (Figures 4I,J), UCEC (OS: HR = 2.31; RFS, HR = 199) (Figures 4K–L), BRCA (OS: HR = 1.52) (Figure 4Q), ESC (OS: HR = 1.98) (Figure 4R), KIRC (OS: HR = 2.56) (Figure 4S), SARC (OS: HR = 1.57) (Figure 4U). DUXAP8 expression was significantly correlated with patients’ RFS in LUSC, EAC, TGCT, HNSC, and THCA.
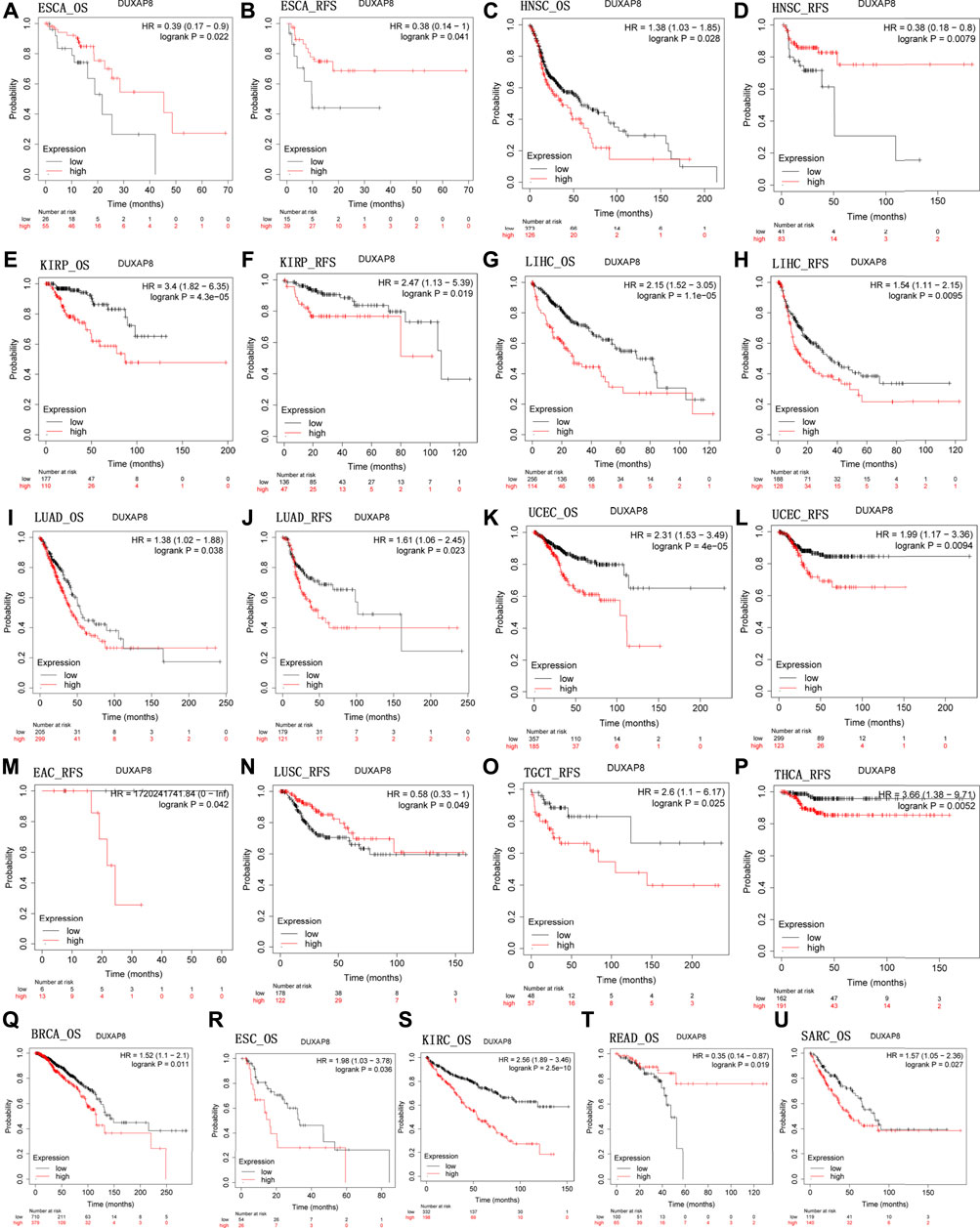
FIGURE 4. Kaplan-Meier survival curves comparing the high and low expression of DUXAP8 gene in various cancer types in Kaplan-Meier Plotter. OS and RFS of (A,B) ESCA, (C,D) HNSC, (E,F) KIRP, (G,H) LIHC, (I,J) LUAD, (K,L) UCEC. RFS of (M) EAC, (N) LUSC, (O) TGCT, and (P) THCA. OS of (Q) BRCA, (R) ESC, (S) KIRC, (T) READ, and (U) SARC. OS, overall survival; RFS, relapse-free survival.
Correlation Analysis of DUXAP8 Expression and Clinicopathology
DUXAP8 expression has been linked to numerous malignancies’ clinicopathological characteristics (Figure 5). Concerning COAD, HNSC, KICH, KIRC, KIRP, and THCA (Figures 5A–F), DUXAP8 was highly expressed in stages III-IV. In particular, patients over the age of 65 had greater DUXAP8 expression in OV, PCPG, SARC, THCA, THYM, and UCEC (Figures 5H–M). DUXAP8 was, on the other hand, highly expressed in individuals under the age of 65, notably in ESCA patients (Figure 5G).
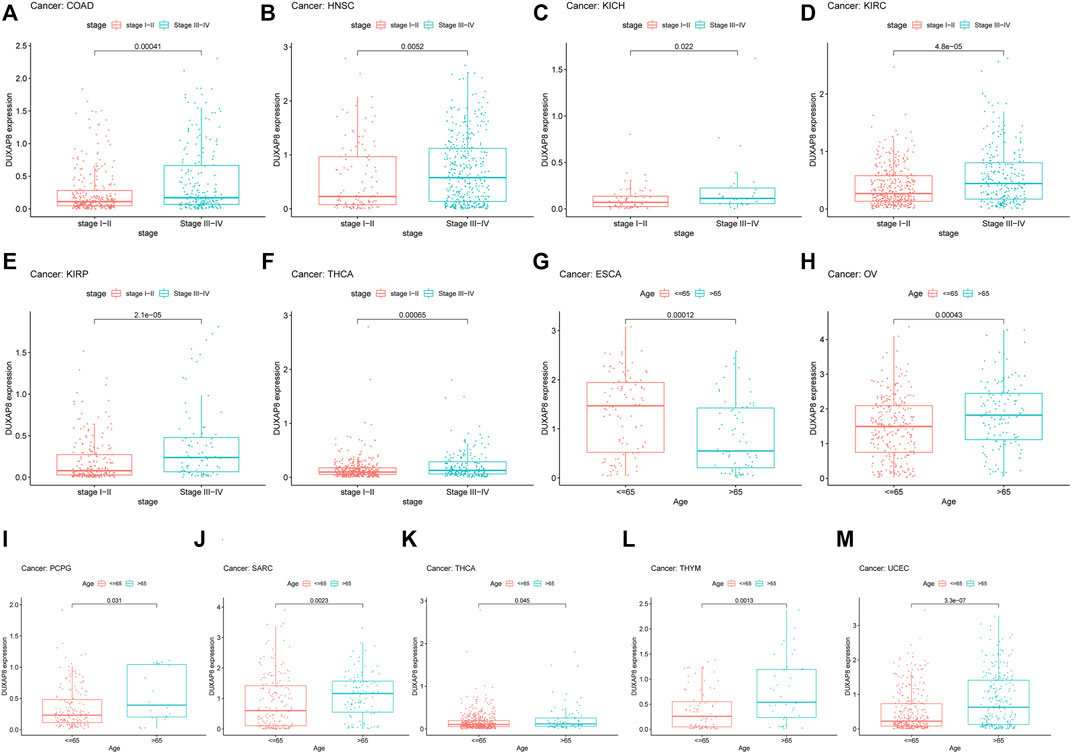
FIGURE 5. Relationship between the DUXAP8 gene expression and clinicopathological features of Pan-cancer. DUXAP8 gene expression is related to the stage in COAD (A), HNSC (B), KICH (C), KIRC (D), KIRP (E), and YHCA (F). DUXAP8 gene expression is associated with age in ESCA (G), OV (H), PCPG (I), SARC (J), THCA (K), THYM (L), and UCEC (M).
Expression of DUXAP8 in Pan-Cancers
We first used GEPIA to investigate DUXAP8 expression in pan-cancer from the TCGA and GTEx databases. DUXAP8 was shown to be highly expressed in BLCA, CHOL, ESCA, HNSC, KIRC, LIHC, UAD, LUSC, OV, SKCM, STAD, THYM, UCEC, and UCS, except for TGCT and LAML, where it was found to be weakly expressed (Figure 6A). Data from the TCGA showed that DUXAP8 was significantly higher in BLCA, CHOL, COAD, ESCA, GBM, HNSC, KIRC, KIRP, LIHC, LUAD, LUSC, PRAD, READ, STAD, THCA, and UCEC (Figure 6B). Figure 6C represents the relative amounts of DUXAP8 expression in several cell lines based on CCLE data.
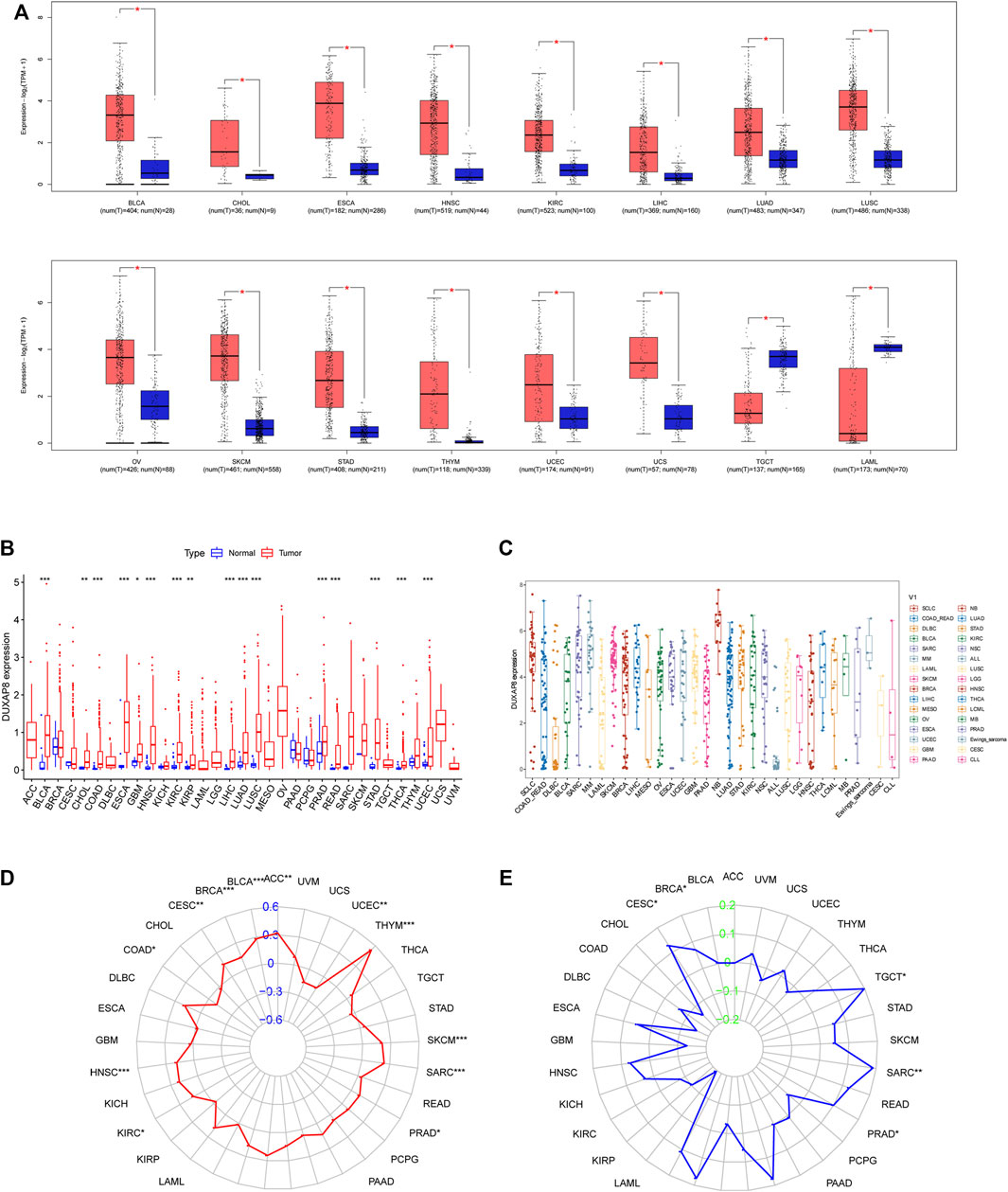
FIGURE 6. (A) DUXAP8 expression levels in different cancer types from TCGA data by Gene Expression Profiling Interactive Analysis (GEPIA). (B) DUXAP8 expression levels in different cancer types from TCGA data. The red fusiformis represents tumor tissue and the blue fusiformis represents normal tissue. p < 0.05, <0.01, <0.001 are represented by “*”, “**”, “***” respectively. (C) The expression distribution of DUXAP8 in different tumor tissues. (D) The radar chart illustrated the association between TMB and DUXAP8 expression in different cancers. (E) The radar chart illustrated the relationship between MSI and DUXAP8 expression in different cancers. The blue curve represents the correlation coefficient, and the green value represents the range.
Correlation of DUXAP8 Expression With Tumor Mutational Burden, Microsatellite Instability, DNA Methyltransferases, and Mismatch Repair
We found that DUXAP8 expression was positively correlated with the TMB in THYM, BLCA, LUAD, SKCM, BRCA, HNSC, SARC, LIHC, LUSC, ACC, CESC, KIRC, PRAD, OV, while negatively correlated with the TMB in UCEC, COAD (Figure 6D). Moreover, DUXAP8 expression was found to be positively correlated to the MSI in LIHC, SARC, TGCT, LGG, BRCA, PRAD, and CESC (Figure 6E). In 29 of the 33 cancer types, TIGIT was correlated with the expression of at least one MMR-related gene (Figure 7A). DUXAP8 expression was positively correlated with DNMTs expression level in most tumors (Figure 7B). DUXAP8 expression was correlated with immune checkpoint biomarkers in most tumors, especially in BRCA, COAD, KRCH, KIRC, KIRP, LIHC, STAD, TGCT, THCA, and THYM (Figure 7C).
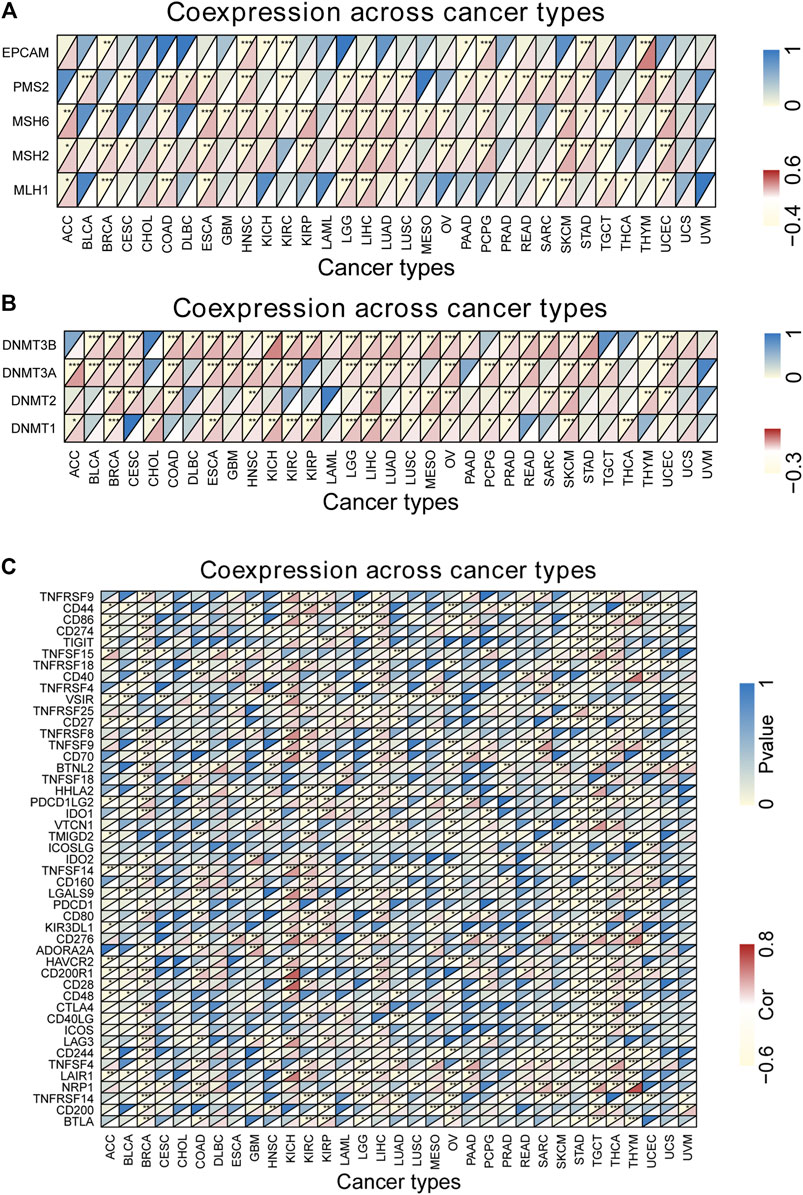
FIGURE 7. Co-expression analysis between DUXAP8 expression and five mismatch repair genes (A), DNA methyltransferase (B) and immune genes (C) in cancers. *p < 0.05; **p < 0.01; ***p < 0.001. Cor, correlation coefficient. The horizontal axis represents cancer types, the vertical axis represents immune genes, and each small rectangular module represents the co-expression of the gene and DUXAP8 in cancer, during them, the upper left corner asterisk and color represent the P-value, and the lower right corner color represents the Cor. *p < 0.05; **p < 0.01; ***p < 0.001. Cor, correlation coefficient.
Pathway Analysis in Pan-Cancers
To investigate the biological function and KEGG pathway of DUXAP8 expression in pan-cancers, we conducted GESA (Figure 8). The results of GO indicated that DUXAP8 was able to regulate the cell cycle, cell junction, cell recognition, cell growth, negative regulation of cellular amide metabolic process, gene silencing, and mRNA binding. The results demonstrate that DUXAP8 expression is associated with several pathways: pentose and glucuronate interconversions, porphyrin and chlorophyll metabolism, retinol metabolism, cytokine receptor interaction, RNA degradation, and regulation of autophagy.
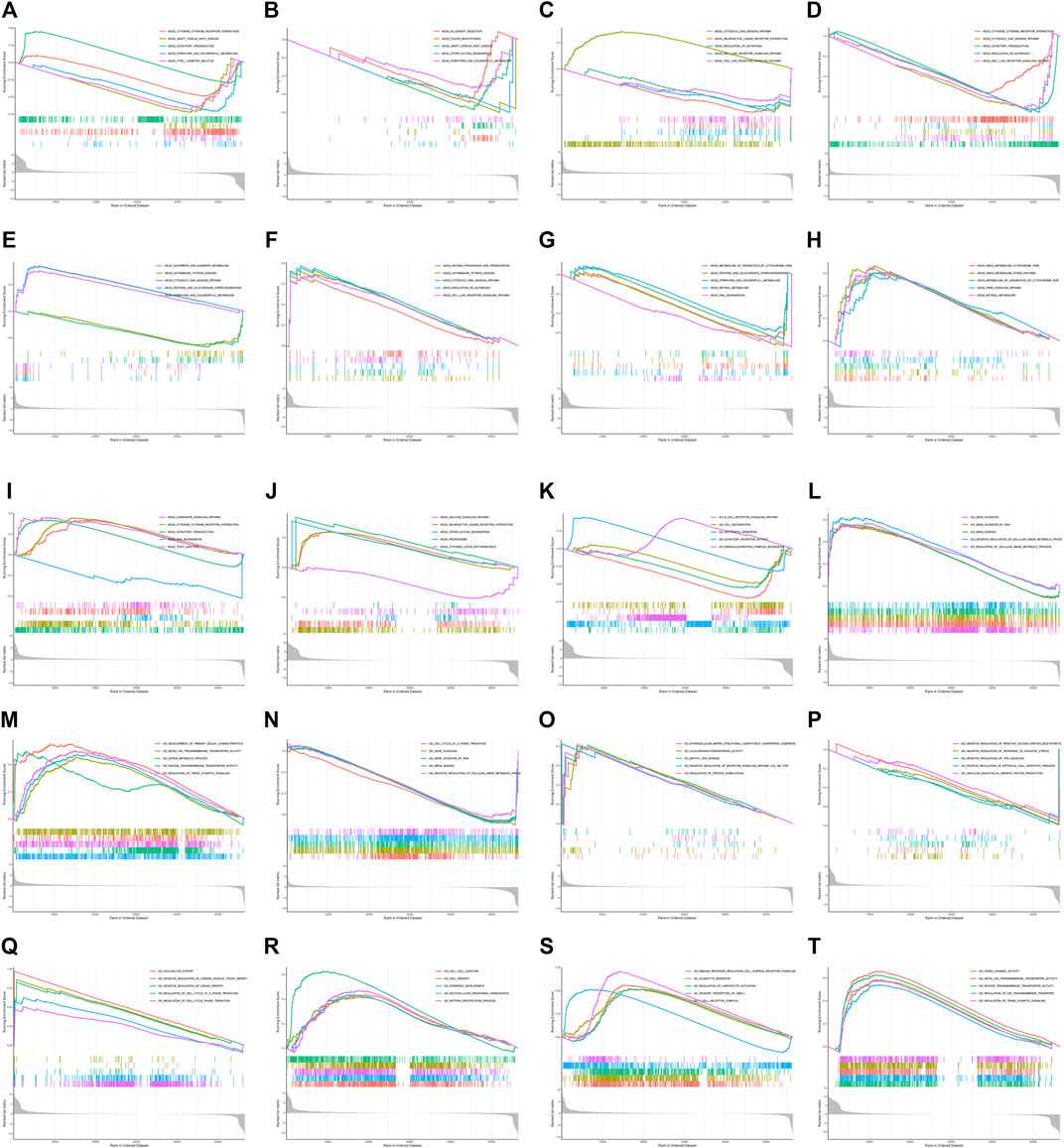
FIGURE 8. (A–J) GO functional annotation of DUXAP8 gene in ACC, BLCA, HNSC, LUSC, OV, PAAD, PCPG, TGCT, THCA, and UVM. (K–T) KEGG pathway analysis of DUXAP8 gene ACC, BLCA, HNSC, LUSC, OV, PAAD, PCPG, TGCT, THCA, and UVM. Different color curves indicate that the DUXAP8 gene regulated different functions or pathways of different cancers, with peaks of curves upward indicating positive regulation and peaks of curves downward representing negative regulation.
Discussion
Great improvements have been achieved in cancer detection and treatment. However, the 5-year survival rate remains relatively low for most cancers. Human health is seriously threatened by cancer. Some lncRNAs have the potential to serve as biomarkers for diagnosing and monitoring tumors due to their specific expression during tumor occurrence and development (Qi and Du, 2013). DUXAP8 is significantly overexpressed in cancer tissues compared to nearby non-tumor tissues, according to observations (Ma et al., 2017b; Du et al., 2019; Chen et al., 2020a; Chen et al., 2020b; He et al., 2020; Yin et al., 2020; Chen et al., 2021a). Thus, we first conducted this meta-analysis to examine if there was a correlation between lncRNA DUXAP8 expression and overall survival in order to better evaluate its predictive potential. Our study revealed a significantly worse OS in cancer patients with high expression of DUXAP8. For this, we concluded that high levels of DUXAP8 expression are associated with a poor prognosis for cancer patients and that DUXAP8 may be a predictor of poor prognosis in cancer patients.
In addition, we employed data mining to investigate the prognostic value of DUXAP8 in a range of tumor types to further validate our results. To assess DUXAP8’s ability to predict pan-cancer, we evaluated multiple datasets. Cox regression model discovered that DUXAP8 was a detrimental factor in ACC, COAD, DLBC, HNSC, KICH, KIRC, KIRP, LIHC, MESO, THCA, and UCEC in the TCGA. Even more, we discovered that high levels of DUXAP8 expression were associated with a poor prognosis in ESCA, HNSC, KIRP, LIHC, LUAD, UCEC, BRCA, ESC, KIRC, READ, and SARC, but with a good prognosis in ESCA, SKCM and READ by Kaplan-Meier method.
We found that DUXAP8 expression was positively correlated with the TMB in THYM, BLCA, LUAD, SKCM, BRCA, HNSC, SARC, LIHC, LUSC, ACC, CESC, KIRC, PRAD, OV, while negatively correlated with the TMB in UCEC, COAD (Figure 6D). Moreover, DUXAP8 expression was found to be positively correlated to the MSI in LIHC, SARC, TGCT, LGG, BRCA, PRAD, and CESC (Figure 6E). DUXAP8 expression was correlated with immune checkpoint biomarkers in most tumors, especially in BRCA, COAD, KRCH, KIRC, KIRP, LIHC, STAD, TGCT, THCA, and THYM. DUXAP8 expression was positively correlated with MMR-related genes level in most tumors.
This study included an in-depth analysis of DUXAP8 expression levels, as well as the relationship with TMB, MSI, MMR, DNMTs, and immune checkpoint biomarkers in 33 cancer types. This study found that the expression of DUXAP8 is significantly correlated with TMB in seven cancer types and MSI in seven cancer types. DUXAP8 expression was positively correlated with MMR-related genes level in most tumors. The research suggested that DUXAP8 expression may have an effect on cancer patients’ response to immune checkpoint therapy, which will benefit the further understanding of immunotherapy’s molecular mechanism in cancer treatment.
DUXAP8 was significantly higher in BLCA, CHOL, ESCA, HNSC, KIRC, LIHC, UAD, LUSC, OV, SKCM, STAD, THYM, UCEC, and UCS, except for TGCT and LAML, where it was found to be weakly expressed. DUXAP8 was significantly higher in BLCA, CHOL, COAD, ESCA, GBM, HNSC, KIRC, KIRP, LIHC, LUAD, LUSC, PRAD, READ, STAD, THCA, and UCEC in the TCGA and GTEx databases. There is accumulating evidence to reveal that DUXAP8 is aberrantly expressed in several malignancies and appears to contribute to the development and progression of multiple cancers, including GC (Ma et al., 2017b), NSCLC (Yang et al., 2019; Ji et al., 2020; Yin et al., 2020; Chen et al., 2021a; Liu et al., 2021a), CC (Chen et al., 2020b), OC (Chen et al., 2020a), CRC (Du et al., 2019; Gong et al., 2019; He et al., 2020; Liang et al., 2021), PTC (Liu et al., 2021b; Pang and Yang, 2021), LGG (Zhao et al., 2020), PC (Lian et al., 2018; Li et al., 2021b), HCC (Jiang et al., 2019; Hu et al., 2020; Wang et al., 2020; Wei et al., 2020; Zhang et al., 2020; Guan et al., 2021), AML (Zhai et al., 2021), BLCA (Jiang et al., 2018; Lin et al., 2018), NB (Nie et al., 2020), ovarian cancer (Meng et al., 2020; Li et al., 2021a), osteosarcoma (Yang et al., 2021a), RCC (Xu et al., 2017; Huang et al., 2018; Xing et al., 2021), melanoma (Chen et al., 2021b), BC (Arabpour et al., 2021; Yang et al., 2021b), esophageal carcinoma (Liu et al., 2018). This is basically consistent with this study.
Although many studies found that lncRNA DUXAP8 serves as an important prognostic factor for patients with a variety of tumors, the underlying systems of how the lncRNA DUXAP8 impacts cancer are still unknown. DUXAP8-related molecular targets, proteins, pathways, and noncoding RNA (microRNAs and circRNAs) were methodically described in this meta-analysis to provide a reference for mechanistic exploration into the carcinogenesis function of DUXAP8 in various cancers (Supplementary Table S2). DUXAP8 induced an EMT phenotype transition and epigenetic alteration via various signaling pathways covering pathways of Wnt/β-catenin (Zhai et al., 2021)in the AML, miR-126-5p/PTEN/PI3K/AKT (Jiang et al., 2018; Lin et al., 2018) in the BLCA, miR-130a-3p (Arabpour et al., 2021; Yang et al., 2021b) in the BC, EZH2 (Ma et al., 2017b; Lian et al., 2018; Du et al., 2019; Gong et al., 2019; Chen et al., 2020a; He et al., 2020) in the CRC, OC and GC, miR-590-5p (Jiang et al., 2019; Hu et al., 2020; Meng et al., 2020; Wang et al., 2020; Wei et al., 2020; Zhang et al., 2020; Li et al., 2021a; Guan et al., 2021) in the ovarian cancer and HCC, miR-126 (Xu et al., 2017; Huang et al., 2018; Xing et al., 2021) in the RCC, miR-3182/NUPR1 (Chen et al., 2021b) in the melanoma, miR-409-3p/HK2/LDHA (Yang et al., 2019; Ji et al., 2020; Yin et al., 2020; Chen et al., 2021a; Liu et al., 2021a) in the NSCLC, miR-448/WTAP/Fak (Lian et al., 2018; Li et al., 2021b) in the PC, miR-223-3p (Liu et al., 2021b; Pang and Yang, 2021) in the PTC, miR-29 (Nie et al., 2020) in the neuroblastoma, miR-635/TOP2A (Yang et al., 2021a) in the osteosarcoma.
Nonetheless, there were several limitations to this meta-analysis. First, only 25 studies with several types of tumors were included in the meta-analysis, so the results need to be further confirmed in a large cohort in the future. Second, it might not be precise enough to calculate HRs and corresponding 95% CIs through survival curves in the place of precisely obtaining them from the primary publications. Third, all included studies divided the cut-off values for high and low lncRNA DUXAP8 expression by inconsistent methods, which made the data less accurate. Fourth, patients from China made up the majority of the eligible trials, which means that they may not accurately represent all cancer patients worldwide. For future clinical trials, it is imperative that high-quality, multi-center studies with a larger sample size be done to confirm and reinforce our preliminary findings.
Conclusion
This study revealed that DUXAP8 might serve as a prognostic biomarker and potential therapeutic target for cancer. It can be used to improve cancer diagnosis, discover potential treatment targets, and improve prognosis. Therefore, combining regular clinical examinations with an evaluation of DUXAP8 expression provides individuals with a targeted prognosis and more treatment options.
Data Availability Statement
The original contributions presented in the study are included in the article/Supplementary Material, further inquiries can be directed to the corresponding author.
Author Contributions
YW and HC conceived and designed the study, and revised the manuscript. YF, XJ, XH, LZ, JR, YL, JY, and HW conducted all data collection and analysis and compiled charts. All authors read and approved the final manuscript.
Funding
This work was funded by the Central to guide local scientific and Technological Development (ZYYDDFFZZJ-1), Natural Science Foundation of Gansu Province, China (No.18JR3RA052), Lanzhou Talent Innovation and Entrepreneurship Project Task Contract (No. 2016-RC-56), Gansu Da Vinci robot high-end diagnosis and treatment team construction project, National Key Research and Development Program (No. 2018YFC1311500), Guiding plan for scientific and technological development of Lanzhou (2019-ZD-102) and Natural Science Foundation of Gansu Province (21JR11RA186).
Conflict of Interest
The authors declare that the research was conducted in the absence of any commercial or financial relationships that could be construed as a potential conflict of interest.
Publisher’s Note
All claims expressed in this article are solely those of the authors and do not necessarily represent those of their affiliated organizations, or those of the publisher, the editors and the reviewers. Any product that may be evaluated in this article, or claim that may be made by its manufacturer, is not guaranteed or endorsed by the publisher.
Acknowledgments
The authors thank the Key Laboratory of Molecular Diagnostics and Precision Medicine for Surgical Oncology in Gansu Province and the DaVinci Surgery System Database (DSSD, www.davincisurgerydatabase.com) for their help and support in the methodology and meta-analysis process.
Supplementary Material
The Supplementary Material for this article can be found online at: https://www.frontiersin.org/articles/10.3389/fgene.2022.907774/full#supplementary-material
Supplementary Figure S1 | Flowchart showing the steps of study selection in this meta-analysis.
Supplementary Figure S2 | Kaplan-Meier survival curves comparing high and low expression of DUXAP8 for different cancer types. (A): OS of KIRC, KIRP, LIHC, SKCM, UCEC. (B) DSS of ACC, BRCA, KIRC, KIRP, READ, SKCM, STAD, and UCEC. (C): DFI of COAD, LIHC, PRAD. (D): PFI of ACC, KIRC, KIRP, LGG, LIHC, MESO, SKCM, and UCEC.
References
Arabpour, M., Layeghi, S. M., Bazzaz, J. T., Naghizadeh, M. M., Majidzadeh-A, K., and Shakoori, A. (2021). The Potential Roles of lncRNAs DUXAP8, LINC00963, and FOXD2-AS1 in Luminal Breast Cancer Based on Expression Analysis and Bioinformatic Approaches. Hum. Cell 34 (4), 1227–1243. doi:10.1007/s13577-021-00539-7
Bahrami, A., Aledavood, A., Anvari, K., Hassanian, S. M., Maftouh, M., Yaghobzade, A., et al. (2018). The Prognostic and Therapeutic Application of microRNAs in Breast Cancer: Tissue and Circulating microRNAs. J. Cell Physiol. 233 (2), 774–786. doi:10.1002/jcp.25813
Chen, L., Wu, C., Wang, H., Chen, S., Ma, D., Tao, Y., et al. (2021). Analysis of Long Noncoding RNAs in Aila-Induced Non-small Cell Lung Cancer Inhibition. Front. Oncol. 11, 2567. doi:10.3389/fonc.2021.652567
Chen, M., Fan, M., Yang, J., and Lang, J. (2020). Identification of Potential Oncogenic Long Non-coding RNA Set as a Biomarker Associated with Colon Cancer Prognosis. J. Environ. Pathol. Toxicol. Oncol. 39 (1), 39–49. doi:10.1615/jenvironpatholtoxicoloncol.2020032351
Chen, M., Zheng, Y., Xie, J., Zhen, E., and Zhou, X. (2020). Integrative Profiling Analysis Identifies the Oncogenic Long Noncoding RNA DUXAP8 in Oral Cancer. Anticancer Drugs 31 (8), 792–798. doi:10.1097/cad.0000000000000936
Chen, X., Gao, J., and Liang, N. (2021). DUXAP8 Knockdown Inhibits the Development of Melanoma by Regulating the miR-3182/NUPR1 P-athway. Oncol. Lett. 22 (1), 495. doi:10.3892/ol.2021.12756
Diboun, I., Wernisch, L., Orengo, C. A., and Koltzenburg, M. (2006). Microarray Analysis after RNA Amplification Can Detect Pronounced Differences in Gene Expression Using Limma. BMC genomics 7, 252. doi:10.1186/1471-2164-7-252
Du, C., Wang, H. X., Chen, P., and Chen, C. H. (2019). STAT3-induced Upregulation of lncRNA DUXAP8 Functions as ceRNA for miR-577 to Promote the Migration and Invasion in Colorectal Cancer through the Regulation of RAB14. Eur. Rev. Med. Pharmacol. Sci. 23 (14), 6105–6118. doi:10.26355/eurrev_201907_18424
Fan, Y-H., Fang, H., Ji, C-x., Xie, H., Xiao, B., and Zhu, X-G. (2017). Long Noncoding RNA CCAT2 Can Predict Metastasis and Poor Prognosis: A Meta-Analysis. Clin. chimica acta; Int. J. Clin. Chem. 466, 120. doi:10.1016/j.cca.2017.01.016
Gong, A., Huang, Z., Ge, H., Cai, Y., and Yang, C. (2019). The Carcinogenic Complex lncRNA DUXAP8/EZH2/LSD1 Accelerates the Proliferation, Migration and Invasion of Colorectal Cancer. J. BUON 24 (5), 1830
Guan, Q., Yuan, B., Zhang, X., Yan, T., Li, J., and Xu, W. (2021). Long Non-coding RNA DUXAP8 Promotes Tumorigenesis by Regulating IGF1R via miR 9 3p in H-epatocellular C-arcinoma. Exp. Ther. Med. 22 (1), 755. doi:10.3892/etm.2021.10187
He, W., Yu, Y., Huang, W., Feng, G., and Li, J. (2020). The Pseudogene DUXAP8 Promotes Colorectal Cancer Cell Proliferation, Invasion, and Migration by Inducing Epithelial-Mesenchymal Transition through Interacting with EZH2 and H3K27me3. Ott Vol. 13, 11059–11070. doi:10.2147/ott.s235643
Hu, Y., Zhang, X., Zai, H.-Y., Jiang, W., Xiao, L., and Zhu, Q. (2020). lncRNA DUXAP8 Facilitates Multiple Malignant Phenotypes and Resistance to PARP Inhibitor in HCC via Upregulating FOXM1. Mol. Ther. - Oncolytics 19, 308–322. doi:10.1016/j.omto.2020.10.010
Huang, T., Wang, X., Yang, X., Ji, J., Wang, Q., Yue, X., et al. (2018). Long Non-coding RNA DUXAP8 Enhances Renal Cell Carcinoma Progression via Downregulating miR-126. Med. Sci. Monit. 24, 7340–7347. doi:10.12659/msm.910054
Ilie, M., Beaulande, M., Hamila, M., Erb, G., Hofman, V., and Hofman, P. (2018). Automated Chromogenic Multiplexed Immunohistochemistry Assay for Diagnosis and Predictive Biomarker Testing in Non-small Cell Lung Cancer. Lung Cancer 124, 90–94. doi:10.1016/j.lungcan.2018.07.037
Ji, X., Tao, R., Sun, L. Y., Xu, X. L., and Ling, W. (2020). Down-regulation of Long Non-coding RNA DUXAP8 Suppresses Proliferation, Metastasis and EMT by Modulating miR-498 through TRIM44-Mediated AKT/mTOR Pathway in Non-small-cell Lung Cancer. Eur. Rev. Med. Pharmacol. Sci. 24 (6), 3152–3165. doi:10.26355/eurrev_202003_20682
Jiang, B., Hailong, S., Yuan, J., Zhao, H., Xia, W., Zha, Z., et al. (2018). Identification of Oncogenic Long Noncoding RNA SNHG12 and DUXAP8 in Human Bladder Cancer through a Comprehensive Profiling Analysis. Biomed. Pharmacother. 108, 500–507. doi:10.1016/j.biopha.2018.09.025
Jiang, H., Shi, X., Ye, G., Xu, Y., Xu, J., Lu, J., et al. (2019). Up-regulated Long Non-coding RNA DUXAP8 Promotes Cell Growth through Repressing Krüppel-like Factor 2 Expression in Human Hepatocellular Carcinoma. Ott Vol. 12, 7429–7436. doi:10.2147/ott.s214336
Johnsson, P., Lipovich, L., Grandér, D., and Morris, K. V. (2014). Evolutionary Conservation of Long Non-coding RNAs; Sequence, Structure, Function. Biochimica Biophysica Acta (BBA) - General Subj. 1840 (3), 1063–1071. doi:10.1016/j.bbagen.2013.10.035
Li, J.-R., Liu, L., Luo, H., Chen, Z.-G., Wang, J.-H., and Li, N.-F. (2021). Long Noncoding RNA DUXAP8 Promotes Pancreatic Carcinoma Cell Migration and Invasion via Pathway by miR-448/WTAP/Fak Signaling Axis. Pancreas 50 (3), 317–326. doi:10.1097/mpa.0000000000001751
Li, L. M., Hao, S. J., Ni, M., Jin, S., and Tian, Y. Q. (2021). DUXAP8 Promotes the Proliferation and Migration of Ovarian Cancer Cells via Down-Regulating microRNA-29a-3p Expression. Eur. Rev. Med. Pharmacol. Sci. 25 (4), 1837–1844. doi:10.26355/eurrev_202102_25078
Li, X., Wei, L., Shang, W., Xing, X., Yin, M., Ling, J., et al. (2018). Trace and Evaluation Systems for Health Services Quality in Rural and Remote Areas: a Systematic Review. J. Public Health 26 (2), 127–135. doi:10.1007/s10389-017-0858-4
Lian, Y., Yang, J., Lian, Y., Xiao, C., Hu, X., and Xu, H. (2018). DUXAP8, a Pseudogene Derived lncRNA, Promotes Growth of Pancreatic Carcinoma Cells by Epigenetically Silencing CDKN1A and KLF2. Cancer Commun. 38 (1), 64. doi:10.1186/s40880-018-0333-9
Liang, H., Wang, J., Zhang, P., Yang, W., Yang, Y., Zhi, Y., et al. (2021). Long Non-coding RNA DUXAP8 Facilitates Cell Proliferation and Induces Apoptosis in Colorectal Cancer via miR-519b/ZNF277 Axis. Ott Vol. 14, 4693–4703. doi:10.2147/ott.s301233
Lin, M. G., Hong, Y. K., Zhang, Y., Lin, B. B., and He, X. J. (2018). Mechanism of lncRNA DUXAP8 in Promoting Proliferation of Bladder Cancer Cells by Regulating PTEN. Eur. Rev. Med. Pharmacol. Sci. 22 (11), 3370–3377. doi:10.26355/eurrev_201806_15158
Liu, W., Zhang, Y., Chen, M., Shi, L., Xu, L., and Zou, X. (2018). A Genome-wide Analysis of Long Noncoding RNA Profile Identifies Differentially Expressed lncRNAs Associated with Esophageal Cancer. Cancer Med. 7 (8), 4181–4189. doi:10.1002/cam4.1536
Liu, Y., Zhang, G., Chen, H., and Wang, H. (2021). Silencing lncRNA DUXAP8 Inhibits Lung Adenocarcinoma Progression by Targeting miR-26b-5p. Biosci. Rep. 41 (1). doi:10.1042/BSR20200884
Liu, Y., Zhang, H., Wang, H., Du, J., Dong, P., Liu, M., et al. (2021). Long Non-coding RNA DUXAP8 Promotes the Cell Proliferation, Migration, and Invasion of Papillary Thyroid Carcinoma via miR-223-3p Mediated Regulation of CXCR4. Bioengineered 12 (1), 496–506. doi:10.1080/21655979.2021.1882134
Ma, H.-w., Xie, M., Sun, M., Chen, T.-y., Jin, R.-r., Ma, T.-s., et al. (2017). The Pseudogene Derived Long Noncoding RNA DUXAP8 Promotes Gastric Cancer Cell Proliferation and Migration via Epigenetically Silencing PLEKHO1 Expression. Oncotarget 8 (32), 52211–52224. doi:10.18632/oncotarget.11075
Ma, Z., Huang, H., Xu, Y., He, X., Wang, J., Hui, B., et al. (2017). Current Advances of Long Non-coding RNA Highly Upregulated in Liver Cancer in Human Tumors. Ott Vol. 10, 4711–4717. doi:10.2147/ott.s136915
Meng, Q., Li, Z., Pan, J., and Sun, X. (2020). Long Noncoding RNA DUXAP8 Regulates Proliferation and Apoptosis of Ovarian Cancer Cells via Targeting miR-590-5p. Hum. Cell 33 (4), 1240–1251. doi:10.1007/s13577-020-00398-8
Newman, A. M., Liu, C. L., Green, M. R., Gentles, A. J., Feng, W., Xu, Y., et al. (2015). Robust Enumeration of Cell Subsets from Tissue Expression Profiles. Nat. Methods 12 (5), 453–457. doi:10.1038/nmeth.3337
Nie, L., Li, C., Zhao, T., Wang, Y., and Liu, J. (2020). LncRNA Double Homeobox A Pseudogene 8 (DUXAP8) Facilitates the Progression of Neuroblastoma and Activates Wnt/β-Catenin Pathway via MicroRNA-29/nucleolar Protein 4 like (NOL4L) axis. Brain Res. 1746, 146947. doi:10.1016/j.brainres.2020.146947
Pang, R., and Yang, S. (2021). lncRNA DUXAP8 Inhibits Papillary Thyroid Carcinoma Cell Apoptosis via Sponging the miR-20b-5p/SOS1 axis. Oncol. Rep. 45 (5), 8051. doi:10.3892/or.2021.8015
Parmar, M. K. P., Torri, V. T., and Stewart, L. S. (1998). Extracting Summary Statistics to Perform Meta-Analyses of the Published Literature for Survival Endpoints. Statistics Med. 17 (24), 2815
Parmar, M. K. B., Torri, V., and Stewart, L. (1998). Extracting Summary Statistics to Perform Meta-Analyses of the Published Literature for Survival Endpoints. Stat. Med. 17 (24), 2815–2834. doi:10.1002/(sici)1097-0258(19981230)17:24<2815::aid-sim110>3.0.co;2-8
Qi, P., and Du, X. (2013). The Long Non-coding RNAs, a New Cancer Diagnostic and Therapeutic Gold Mine. Mod. Pathol. 26 (2), 155–165. doi:10.1038/modpathol.2012.160
Stang, A. (2010). Critical Evaluation of the Newcastle-Ottawa Scale for the Assessment of the Quality of Nonrandomized Studies in Meta-Analyses. Eur. J. Epidemiol. 25 (9), 603–605. doi:10.1007/s10654-010-9491-z
Tian, J., Zhang, J., Ge, L., Yang, K., and Song, F. (2017). The Methodological and Reporting Quality of Systematic Reviews from China and the USA Are Similar. J. Clin. Epidemiol. 85, 50–58. doi:10.1016/j.jclinepi.2016.12.004
Wang, X.-K., Liao, X.-W., Huang, R., Huang, J.-L., Chen, Z.-J., Zhou, X., et al. (2020). Clinical Significance of Long Non-coding RNA DUXAP8 and its Protein Coding Genes in Hepatocellular Carcinoma. J. Cancer 11 (20), 6140–6156. doi:10.7150/jca.47902
Wang, Y., Fu, L., Lu, T., Zhang, G., Zhang, J., Zhao, Y., et al. (2021a). Clinicopathological and Prognostic Significance of Long Non-coding RNA MIAT in Human Cancers: A Review and Meta-Analysis. Front. Genet. 12, 729768. doi:10.3389/fgene.2021.729768
Wang, Y., Lin, K., Xu, T., Wang, L., Fu, L., Zhang, G., et al. (2021b). Development and Validation of Prognostic Model Based on the Analysis of Autophagy-Related Genes in Colon Cancer. Aging. 13 (14), 19028–19047.
Wei, F., Yang, L., Jiang, D., Pan, M., Tang, G., Huang, M., et al. (2020). Long Noncoding RNA DUXAP8 Contributes to the Progression of Hepatocellular Carcinoma via Regulating miR‐422a/PDK2 axis. Cancer Med. 9 (7), 2480–2490. doi:10.1002/cam4.2861
Xing, X.-L., Yao, Z.-Y., Ou, J., Xing, C., and Li, F. (2021). Development and Validation of Ferroptosis-Related lncRNAs Prognosis Signatures in Kidney Renal Clear Cell Carcinoma. Cancer Cell Int. 21 (1), 591. doi:10.1186/s12935-021-02284-1
Xu, X., Xu, Y., Shi, C., Wang, B., Yu, X., Zou, Y., et al. (2017). A Genome-wide Comprehensively Analyses of Long Noncoding RNA Profiling and Metastasis Associated lncRNAs in Renal Cell Carcinoma. Oncotarget 8 (50), 87773–87781. doi:10.18632/oncotarget.21206
Yan, P., Yao, L., Li, H., Zhang, M., Xun, Y., Li, M., et al. (2019). The Methodological Quality of Robotic Surgical Meta-Analyses Needed to Be Improved: a Cross-Sectional Study. J. Clin. Epidemiol. 109, 20–29. doi:10.1016/j.jclinepi.2018.12.013
Yang, K. (2018). Evidence-based Social Science: the Origin, Development and Prospects. J. Librariansh. Inf. Sci. 3, 1e10.
Yang, K., Li, X., and Bai, Z. (2018). Evidence-based Social Science Research Methods: Systematic Review and Meta-Analysis [M]. Lanzhou University Press.
Yang, S.-J., Weng, J.-L., Wei, B., and Du, X.-K. (2019). Long Non-coding RNA DUXAP8 Regulates the Cell Proliferation and Invasion of Non-small-cell Lung Cancer. Open Life Sci. 14, 201–207. doi:10.1515/biol-2019-0022
Yang, T., Guo, J. P., Li, F., Xiu, C., Wang, H., and Duan, X. L. (2021). Long Non-coding RNA-DUXAP8 Regulates TOP2A in the Growth and Metastasis of Osteosarcoma via microRNA-635. Mol. Med. Rep. 24 (1), 12150. doi:10.3892/mmr.2021.12150
Yang, Z., Ding, H., Pan, Z., Li, H., Ding, J., and Chen, Q. (2021). YY1-inudced Activation of lncRNA DUXAP8 Promotes Proliferation and Suppresses Apoptosis of Triple Negative Breast Cancer Cells through Upregulating SAPCD2. Cancer Biol. Ther. 22 (3), 216–224. doi:10.1080/15384047.2021.1881201
Yao, L., Sun, R., Chen, Y.-L., Wang, Q., Wei, D., Wang, X., et al. (2016). The Quality of Evidence in Chinese Meta-Analyses Needs to Be Improved. J. Clin. Epidemiol. 74, 73–79. doi:10.1016/j.jclinepi.2016.01.003
Yin, D., Hua, L., Wang, J., Liu, Y., and Li, X. (2020). Long Non-coding RNA DUXAP8 Facilitates Cell Viability, Migration, and Glycolysis in Non-small-cell Lung Cancer via Regulating HK2 and LDHA by Inhibition of miR-409-3p. Ott Vol. 13, 7111–7123. doi:10.2147/ott.s243542
Zhai, H., Zhao, J., Pu, J., Zhao, P., and Wei, J. (2021). LncRNA-DUXAP8 Regulation of the Wnt/β-Catenin Signaling Pathway to Inhibit Glycolysis and Induced Apoptosis in Acute Myeloid Leukemia. Turk. J. Haematol. 38 (4), 264–272. doi:10.4274/tjh.galenos.2021.2020.0769
Zhang, H., Chu, K., Zheng, C., Ren, L., and Tian, R. (2020). Pseudogene DUXAP8 Promotes Cell Proliferation and Migration of Hepatocellular Carcinoma by Sponging MiR-490-5p to Induce BUB1 Expression. Front. Genet. 11, 666. doi:10.3389/fgene.2020.00666
Keywords: long noncoding RNA, DUXAP8, prognosis, cancers, bioinformatics analysis
Citation: Wang Y, Jiang X, Zhang D, Zhao Y, Han X, Zhu L, Ren J, Liu Y, You J, Wang H and Cai H (2022) LncRNA DUXAP8 as a prognostic biomarker for various cancers: A meta-analysis and bioinformatics analysis. Front. Genet. 13:907774. doi: 10.3389/fgene.2022.907774
Received: 30 March 2022; Accepted: 23 May 2022;
Published: 15 August 2022.
Edited by:
Hifzur R. Siddique, Aligarh Muslim University, IndiaCopyright © 2022 Wang, Jiang, Zhang, Zhao, Han, Zhu, Ren, Liu, You, Wang and Cai. This is an open-access article distributed under the terms of the Creative Commons Attribution License (CC BY). The use, distribution or reproduction in other forums is permitted, provided the original author(s) and the copyright owner(s) are credited and that the original publication in this journal is cited, in accordance with accepted academic practice. No use, distribution or reproduction is permitted which does not comply with these terms.
*Correspondence: Hui Cai, Y2FpYWxvbnRlYW1AMTYzLmNvbQ==
†These authors share first authorship