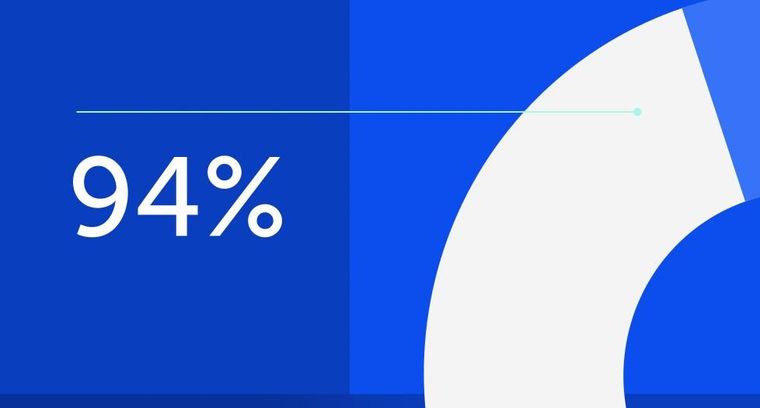
94% of researchers rate our articles as excellent or good
Learn more about the work of our research integrity team to safeguard the quality of each article we publish.
Find out more
ORIGINAL RESEARCH article
Front. Genet., 11 April 2022
Sec. Computational Genomics
Volume 13 - 2022 | https://doi.org/10.3389/fgene.2022.872238
This article is part of the Research TopicMedical Knowledge-Assisted Machine Learning Technologies in Individualized MedicineView all 31 articles
Background: Increasing evidence have depicted that DNA repair–related genes (DRGs) are associated with the prognosis of colorectal cancer (CRC) patients. Thus, the aim of this study was to evaluate the impact of DNA repair–related gene signature (DRGS) in predicting the prognosis of CRC patients.
Method: In this study, we retrospectively analyzed the gene expression profiles from six CRC cohorts. A total of 1,768 CRC patients with complete prognostic information were divided into the training cohort (n = 566) and two validation cohorts (n = 624 and 578, respectively). The LASSO Cox model was applied to construct a prediction model. To further validate the clinical significance of the model, we also validated the model with Genomics of Drug Sensitivity in Cancer (GDSC) and an advanced clear cell renal cell carcinoma (ccRCC) immunotherapy data set.
Results: We constructed a prognostic DRGS consisting of 11 different genes to stratify patients into high- and low-risk groups. Patients in the high-risk groups had significantly worse disease-free survival (DFS) than those in the low-risk groups in all cohorts [training cohort: hazard ratio (HR) = 2.40, p < 0.001, 95% confidence interval (CI) = 1.67–3.44; validation-1: HR = 2.20, p < 0.001, 95% CI = 1.38–3.49 and validation-2 cohort: HR = 2.12, p < 0.001, 95% CI = 1.40–3.21). By validating the model with GDSC, we could see that among the chemotherapeutic drugs such as oxaliplatin, 5-fluorouracil, and irinotecan, the IC50 of the cell line in the low-risk group was lower. By validating the model with the ccRCC immunotherapy data set, we can clearly see that the overall survival (OS) of the objective response rate (ORR) with complete response (CR) and partial response (PR) in the low-risk group was the best.
Conclusions: DRGS is a favorable prediction model for patients with CRC, and our model can predict the response of cell lines to chemotherapeutic agents and potentially predict the response of patients to immunotherapy.
With the third highest incidence rate in the world, colorectal cancer (CRC) is a serious threat to human health (Bray et al., 2018). Nowadays, due to lifestyle changes, there is an increasingly high incidence of mortality from CRC (Zheng et al., 2014). As one of the most common gastrointestinal tumors in general surgery, CRC is a multifactorial disease with extremely complex pathogenesis (Migliore et al., 2011). At present, the early diagnosis of CRC has involved epigenetics, genomics, and so on (Marcuello et al., 2019). DNA repair is a series of processes by which a cell recognizes and corrects damage to the DNA molecules that encode its genome (Zinovkina, 2018; Burdak-Rothkamm and Rothkamm, 2021), and it is extremely important for maintaining the stability of the genome and protecting the genome from damage by endogenous and environmental agents (Friedberg, 2001). It is estimated that human cells suffer more than
Due to the limited options for capturing the molecular heterogeneity of the disease and the lack of consideration and sufficient validation of other gene expressions, few of the prognostic models of early stage CRC have been applied in clinical practice (Guinney et al., 2015; Phipps et al., 2015). Thus, an accurate method is needed to identify effective prognostic models to assess the disease-free survival (DFS) of patients with CRC. The aim of the present study is to examine the interrelationships between DNA repair–related genes (DRGs) and CRC, to determine an effective prognostic model to evaluate the DFS of patients with CRC and provide guidance for clinicians in early diagnosis and treatment.
We retrospectively analyzed the gene expression profiles of CRC samples from six public cohorts. Totally, 1,768 samples were available for analysis in the current study. The CIT/GSE39582 (n = 566) was used for training the model, and The Cancer Genome Atlas colorectal cancer (TCGA, n = 624) was selected to serve as a validation-1 cohort. The remaining four microarray data sets (GSE14333, GSE33113, GSE37892, and GSE39084) were merged into a validation-2 cohort (n = 578) (Table 1). The transcriptome RNA-sequencing data of the CRC samples were from the TCGA data portal, and other microarray data sets were acquired directly from the GEO database. The institutional review board of our hospital approved this study, and data were collected from 12 May to 10 October 2020.
Firstly, a complete list of DRGs was available online from MSigDB (version 6.2, https://www.gsea-msigdb.org/gsea/msigdb). We identified a list of candidate genes differentially expressed between relapsed samples and non-relapsed samples by using the “limma” R package (Diboun et al., 2006). The genes with an absolute log2-fold change of more than 1 and an adjusted p < 0.05 were considered for subsequent analysis. In order to minimize over-fitting risk, we applied a Cox proportional hazards regression model on CRC samples combined with the least absolute shrinkage and selection operator (LASSO) (Tibshirani, 1997). The penalty parameter was estimated by 10-fold cross-validation in the training data set at the minimum partial likelihood deviance.
We divided patients into high-risk and low-risk groups by determining the optimal threshold through the time-dependent receiver operating characteristic (ROC) curve (survivalroc, version 1.0.3) at 5 y in the training data set. The ROC curve was estimated by the Kaplan–Meier estimation method. We performed univariate and multivariate Cox regression analyses of the cohort to verify that the 11-DRG signature was independent of other clinical features.
To evaluate the biological functions of the DNA repair–related gene signature (DRGS), enrichment analysis for differentially expressed genes in different groups was applied using the R package “gProfileR.” We used the Bioconductor package “HTSanalyzeR” to perform Gene Set Enrichment Analysis (GSEA) to predict significant dysregulated pathways (Subramanian et al., 2005; Wang et al., 2011). Gene sets of cancer hallmarks from MSigDB (Liberzon et al., 2015) were examined.
To further explore the clinical application of our model, we used Genomics of Drug Sensitivity in Cancer (GDSC) to analyze the differences of chemotherapeutic drugs between the high-risk group and the low-risk group.
As known, immunotherapy is a hot topic, and we want to know whether this model can predict immunotherapy. We verified our model by using the data provided in the article “Interplay of somatic alterations and immune infiltration modulates response to PD-1 blockade in advanced clear cell renal cell carcinoma (ccRCC)” published in Nature Medicine (Braun et al., 2020). We constructed the DRGS in the data set of advanced clear cell renal cell carcinoma and divided it into the high-risk and low-risk groups according to the cutoff of our original model. The overall survival (OS) curve was drawn using the Kaplan–Meier method. In addition, we selected some immune-related indicators in the data set and compared the differences of these indicators between the high- and low-risk groups by t-test. Besides, we also analyzed the OS curve of the objective response rate (ORR) of immunotherapy.
The tumor immune dysfunction and exclusion (TIDE) algorithm can be used to predict the tumor response to immune checkpoint inhibition treatment and the function of genes regulating tumor immunity, so as to effectively predict the effect of immune checkpoint inhibition treatment.
All the statistical analyses were performed on R (version 3.4.3, www.r-project.org). The hazard ratios were calculated using the “survcomp” package28 (version: 1.28.4) (Schröder et al., 2011). The LASSO regression was implemented using “glmnet” R package (version: 2.0.16). Cox regression analysis was used for single-factor and multifactor analyses of the results, and the receiver operating characteristic (ROC) curve and C-index were used to evaluate the model. A p-value of less than 0.05 was defined as statistical significance in all tests.
A total of 1,768 CRC patients were included in the analysis. The CIT data set (GSE39582, n = 566) was used as the training cohort and genes with relatively high variation were maintained as candidates (Table 1, Figure 1). With median absolute deviation >0.5 and excluding the genes expressed less in the median expression level, 1,286 genes were screened out of 1,376 DRGs measured on all platforms from the data sets. In addition, in order to improve the robustness of the identification for the limited sample size, we further selected DRGs by using the Cox proportional hazards regression against 1,000 randomized trials (80% portion of samples each time) to assess the correlation between each candidate gene and patients’ DFS in the training cohort. A total of 46 DRGs were robustly associated with individual patients’ DFS. In order to minimize the over-fitting risk, we applied a Cox proportional hazards regression model to the CRC samples combined with the LASSO. By using LASSO Cox regression, 11 prognostic DRGs were selected and combined for the construction of DRGS (Figures 2A,B). The risk scores were calculated by the formula designed by the Cox regression model. The total risk score was imputed as follows (−0.1145 × POLR2B) + (−0.0653 × RAD1) + (0.0370 × CDA) + (0.1711 × NPR2) + (−0.0328 × UBE2D2) + (−0.0992 × BCL2) + (−0.0473 × PLD6) + (0.0896 × ERBB2) + (0.1220 ×ARPC1B) + (−0.1086 × FUT4) + (−0.0765 × PSME2). The time-dependent ROC curve analysis showed that the optimal cutoff to stratify high- and low-risk groups was −0.147 (Figure 2C).
FIGURE 2. (A) Identification and selection of prognostic genes by LASSO Cox proportional hazards regression. (B) Establishment of 11 DNA repair–related genes signature from the LASSO COX regression. (C) Optimal cutoff point of the prognostic gene signature at 5-y OS endpoint from the ROC curve. (D) Heat map of the 11 DNA repair–related genes in two risk groups.
Six colorectal cancer transcription data sets containing prognostic data were selected to assess the prognostic ability of the DRGS. The GSE39582 data set (n = 566) was used as a training data set (Figure 2D). The TCGA CRC dataset was enrolled as validation-1 cohort (n = 624), and additional data sets from the GEO were combined as validation-2 cohort (n = 578). Among the patients in the training and validation cohorts, more recurrences were found in the high-risk group than in the low-risk group (Figures 3A,D,G). When applied to a follow-up duration, the promising prognostic values of 2-, 3-, and 5-year AUC were 0.640, 0.664, and 0.653, respectively, in the training cohort. In the validation-1 cohort, the values of 2-, 3-, and 5-year AUC were 0.620, 0.628, and 0.606, respectively. Furthermore, in the validation-2 cohort, the values of 2-, 3-, and 5-year AUC were 0.645, 0.631, and 0.638, respectively (Figures 3B,E,H). The DRGS significantly stratified patients into the high- and low-risk groups in the training cohort (HR = 2.40, 95% CI = 1.67–3.44, p < 0.001), validation-1 cohort (HR = 2.20, p < 0.001, 95% CI = 1.38–3.49), and validation-2 cohort (HR = 2.12, p < 0.001, 95% CI = 1.40–3.21) (Figures 3C,F,I). Besides, the OS in the low-risk group was better than in the high-risk group (Supplementary Figure 1).
FIGURE 3. (A,D,G) Distribution of the DRGS risk score and its correlation to recurrence in the training, validation-1, and validation-2 cohort. (B,E,H) Time-dependent ROC analysis of disease-free survival for CRC patients in the training, validation-1, and validation-2 cohorts at the time points of 2, 3, and 5 y. (C,F,I) Kaplan–Meier curves comparing survival of patients within the low- and high-risk groups in the training cohort, validation-1, and validation-2 cohorts. p-values were calculated using log-rank tests.
Compared to the risk scores calculated using the FDA-approved assay Oncotype DX colon algorithm, we found that the DRGS achieved better survival correlation in the training cohort (C-index, 0.78 vs 0.60), validation-1 cohort (C-index, 0.65 vs 0.51), and validation-2 cohort (C-index, 0.66 vs 0.62) (Table 2).
To further investigate whether the DRGS could serve as an independent predictor of prognosis, univariate and multivariate Cox proportional hazards regression analyses were performed. As expected, age, sex, tumor stage, tumor location, and pathologic gene status were associated with outcomes for CRC patients (Table 3). In the univariate analysis, DRGS, MMR status, and KRAS mutation status were significantly correlated with worse prognosis in the training cohort. After adjusting for clinical features such as age, gender, tumor location, and molecular types, the DRGS remained an independent prognostic factor in the multivariate analyses in both validation cohorts.
Gene Ontology (GO) analysis revealed that some biological process pathways (extracellular region, cell proliferation, and cell adhesion) were the main enriched pathways in the high-risk group (Figure 4A). In addition, the GSEA in the high-risk group when compared with the low-risk groups shown that the metastasis-related pathways (i.e., angiogenesis, KRAS signaling, epithelial mesenchymal transit, and myogenesis pathways) were enriched in the high-risk group (Figure 4B, Supplementary Table S1). Similarly, we obtained consistent results in the TCGA and validation-2 cohorts (Supplementary Figure 2). These findings suggest that the enrichment of pathways provided evidence of molecular mechanisms affected by the DRGS and thus can predict the prognosis of CRC.
FIGURE 4. (A) Gene ontology of the differentially expressed genes between the two risk groups. “GeneRatio” is the percentage of total differential genes in the given GO term. (B) GSEA showed several metastasis-related processes enriched in the high-risk group, including angiogenesis, KRAS signaling, epithelial mesenchymal transit (EMT), and myogenesis signal pathways.
As known, MSI/MMR-deficient (dMMR) is widely considered as a promising biomarker, suggesting greater efficacy for immune checkpoint inhibitor (ICB) (Zhao et al., 2019). In order to further investigate the clinical application of our model, we used GDSC to analyze the differences of chemotherapeutic drugs between the high-risk and low-risk groups. We selected 48 kinds of cell lines related to CRC. After dividing the cell lines into the high-risk and low-risk groups according to the cutoff of our model, we selected the chemotherapeutic drugs commonly used in clinics to see the IC50 of the cell lines in the high-risk and low-risk groups. We can see that among the chemotherapeutic drugs such as oxaliplatin, 5-fluorouracil, and irinotecan, the IC50 of the cell line in the low-risk group was lower (Figure 5). It showed that the cell lines in the low-risk group were more sensitive to these three drugs.
FIGURE 5. CRC cell lines in the GDSC database were divided into the high-risk and low-risk groups based on DNA repair–related signature and the differences in response to chemotherapies between the two groups were analyzed. (A) Relationship between the cell line of the high-risk and low-risk groups and IC50 of oxaliplatin. (B) Relationship between the cell line of the high-risk and low-risk groups and IC50 of fluorouracil. (C) Relationship between the cell line of the high-risk and low-risk groups and IC50 of irinotecan.
To examine whether the DRGS could predict the survival for ccRCC patients, the patients were divided into the high-risk and the low-risk groups according to the cutoff of our original model. The cutoff was still −0.147, and the prognosis data of these patients were analyzed. The OS of the high-risk group was worse than that of the low-risk group in ccRCC patients (HR = 1.45, 95% CI = 1.09–1.92, p = 0.0103) (Figure 6A). When it comes to the ORR of immunotherapy, we can clearly see the ORR with complete response (CR) and partial response (PR) that had better OS for both the high-risk and low-risk groups (p < 0.001). Notably, the OS of the low-risk group with the CR + PR was the best (Figure 6B).
FIGURE 6. Patients in the advanced clear cell renal cell carcinoma (ccRCC) database were divided into the high-risk and low-risk groups based on the DNA repair–related signature. (A) Kaplan–Meier curves comparing the survival of patients within the low- and high-risk groups in the ccRCC database. (B) OS curve of the objective response rate (ORR) of immunotherapy in ccRCC database.
We applied the TIDE algorithm which can predict the response to immunotherapy. The low-risk group had a lower TIDE score in GSE39582 and TCGA data sets, indicating that this subgroup was most likely to benefit from immunotherapy. Besides, the low-risk group had higher interferon gamma (IFNG), higher microsatellite instability (MSI) score, and lower cancer-associated fibroblasts (CAFs) amount, which confirmed the more activated immune landscape in this subgroup (Figure 7).
FIGURE 7. Tumor immune dysfunction and exclusion (TIDE) algorithm was validated in the training set GSE39582 (A,B,C,D) and the validation set TCGA (E,F,G,H).
Colorectal cancer is the leading cause of death among gastrointestinal cancers. The incidence and mortality from colorectal cancer are increasing year by year, and its prognosis is closely related to early diagnosis (Siegel et al., 2016; Siegel et al., 2017). Numerous studies have highlighted the biomarkers that are associated with the pathogenesis and biology of CRC (Shah et al., 2014; De Rosa et al., 2016; Lech et al., 2016; Das et al., 2017), and many multigene prognostic signatures have been developed for CRC (Shah et al., 2014; Kandimalla et al., 2018; Ozawa et al., 2018; Gao et al., 2019; Kandimalla et al., 2019). Unfortunately, the accuracy of their prognosis predictions remains uncertain (Fung et al., 2014). We still need much more effort to achieve good prognostic CRC prediction, which is still considered a challenge.
In recent years, some studies have found some new results in DNA pathway repair and DRGs research. DRGs inactivation may disrupt genomic integrity, which may increase the risk of accumulation of gene mutations associated with cancer development (Bouwman and Jonkers, 2012). MSI/dMMR is widely considered as a promising biomarker, suggesting greater efficacy for ICB despite some limitations (Zhao et al., 2019). In this study, our purpose was to identify and validate a reliable DRGS and improve the accuracy of survival prediction for CRC patients.
A total of 1,768 CRC patients from one training cohort and two validation cohorts were included in this study. Our prognostic DRGS can stratify CRC patients into two groups with different survival outcomes. A multivariate analysis suggested that the DRGS remained an independent prognostic factor and was significantly associated with poor prognosis in CRC. Furthermore, the C-index results of the DRGS showed its clinical superiority to Oncotype DX. Thus, it offers a significantly promising prognostic biomarker potential compared to the clinicopathological risk factors that are currently in use. The GSEA revealed that the metastasis-related pathways (i.e., angiogenesis, KRAS signaling, epithelial mesenchymal transit, and myogenesis pathways) were enriched in the high-risk group, all of which were well known to play a crucial role in the progression and proliferation of CRC in numerous studies (Cooks et al., 2013; De Simone et al., 2015; Lu et al., 2016). Further studies are required to clarify the effects of DNA repair in order to identify more targets and improve the prognosis of CRC patients.
In order to further investigate the clinical application of our model, we divided the CRC cell lines in the GDSC database into the high-risk group and low-risk group according to the DRGS and analyzed the differences in chemotherapy response between the two groups. We can see that among the chemotherapeutic drugs such as oxaliplatin, 5-fluorouracil, and irinotecan, the IC50 of the cell line in the low-risk group was lower. It showed that the cell lines in the low-risk group were more sensitive to these three drugs. On the contrary, the cell lines in the high-risk group were more insensitive to these three chemotherapeutic drugs. This indicated that our model could predict the response of cell lines to chemotherapeutic agents. This may provide some guidance for clinical medication.
We knew that MSI/dMMR was widely considered as a potential biomarker for predicting ICB (Zhao et al., 2019). We wanted to know whether our model can predict immunotherapy, so we verified our model by using the data provided in the article that “Interplay of somatic alterations and immune infiltration modulates response to PD-1 blockade in advanced clear cell renal cell carcinoma (ccRCC)” published in Nature Medicine (Braun et al., 2020). From the OS curve of the high- and low-risk groups, we could see that the OS of the high-risk group was worse in the ccRCC patients, and it suggested that our model can also well predict the OS of patients with ccRCC. When it comes to the ORR of immunotherapy, we can clearly see the ORR with CR + PR that had better OS in both the high-risk and low-risk groups. Notably, the OS of the low-risk group with CR + PR was the best. This indicated that our model can potentially predict the response of patients to immunotherapy. Our model can be used to further identify cancer patients who are more suitable for immunotherapy.
To further demonstrate that our model can predict the response to immunotherapy, we used the TIDE algorithm for validation in the training and validation data sets. From the results, we can see that the TIDE score of the high-risk group was higher than that of the low-risk group, indicating that the high-risk group was less sensitive to immunotherapy than the low-risk group. That is to say, the low-risk group was more effective for immunotherapy. IFNG, produced by T cells in the immune system and natural killer cells, is a potent viral inhibitor (Jorgovanovic et al., 2020). MSI, caused by defects in MMR genes, is an important molecular marker for prognosis and the development of adjuvant treatment regimens in colorectal and other solid tumors (Boland and Goel, 2010). CAFs are a group of activated fibroblasts with significant heterogeneity and plasticity in the tumor microenvironment, which have significant tumor-promoting functions (Chen and Song, 2019). The low-risk group had higher IFNG, higher MSI score, and lower cancer CAFs amount, which showed that the immune landscape of the low-risk group was more active. The consistent results of the training and validation data sets not only proved the reliability and robustness of our model but also proved that our model can predict the response to immunotherapy, which may bring some clinical benefits to CRC patients.
As for how to apply the model to the clinic, we can detect these 11 genes for patients. Because it is a small panel of genes, it can avoid the waste of large medical resources and reduce the problem of high diagnostic cost for patients as much as possible. By detecting the 11 small panel genes, we calculated the risk score of patients and grouped them. With the help of the prediction model, not only patients can make more favorable choices for themselves but also doctors can make better clinical decisions according to the patient’s risk score.
There are some limitations to our study. First, this is a retrospective study, although we validated the signature in independent data sets. In addition, the samples from primary tumor or metastatic disease may have inconsistent genetic heterogeneity, which could lead to sampling bias (NEJM Group, 2012; Mimori et al., 2018). In addition, systematic errors result from analyzing samples of disparate databases or the influence of measuring instruments, and not all batch effects can be eliminated based on their complexity. In verifying whether the model could predict immunotherapy response to CRC, we used immunotherapy data sets from ccRCC as there is currently a lack of data sets for public immunotherapy response to CRC. However, we also used the TIDE algorithm to further verify that our model can predict the immunotherapy benefit of patients. Therefore, we have sufficient evidence to prove that our model can predict the benefit of immunotherapy for patients. Although we investigated as many genes as possible, further clinical and pharmacological tests are needed to validate our results.
In summary, our work provides an accurate prognostic approach for estimating the survival outcomes of CRC patients. Further prospective studies are needed to evaluate the clinical application of this signature for the prognosis of CRC.
The data sets generated and analyzed during the current study are available in the TCGA cohort data downloaded from Broad GDAC Firehose (http://gdac.broadinstitute.org/). Other microarray data sets were acquired directly from the GEO database (https://www.ncbi.nlm.nih.gov/geo/query).
M-YL and WW contributed equally to this study. M-YL, WW, XH and FG contributed to the study concept and design, acquisition, analysis, and interpretation of data and in drafting of the manuscript. M-EZ, DC, DF, C-HL, W-BK, Z-PH, XD, CH and QZ contributed to the data collections and manuscript reviews. All authors read and approved the final manuscript.
This study was supported by the National Natural Science Foundation of China (No. 82002221, FG), the Sun Yat-sen University 100 Top Talent Scholars Program – China (No. P20190217202203617, FG), Guangzhou Basic and Applied Basic Research Fund (No. 202102020820, FG), The Sixth Affiliated Hospital of Sun Yat sen University Startup Fund for Returnees (No. R20210217202501975, FG), Project funded by China Postdoctoral Science Foundation (No. 2020M683121, MZ).
The authors declare that the research was conducted in the absence of any commercial or financial relationships that could be construed as a potential conflict of interest.
All claims expressed in this article are solely those of the authors and do not necessarily represent those of their affiliated organizations, or those of the publisher, the editors, and the reviewers. Any product that may be evaluated in this article, or claim that may be made by its manufacturer, is not guaranteed or endorsed by the publisher.
The Supplementary Material for this article can be found online at: https://www.frontiersin.org/articles/10.3389/fgene.2022.872238/full#supplementary-material.
Supplementary Figure 1 | (A) Distribution of the DRGS risk score and its correlation to the survival status. (B) Time-dependent ROC analysis of overall survival for CRC patients at the time points of 5 and 10 y. (C) Kaplan–Meier curves comparing overall survival of patients within the low and high-risk groups. P-values were calculated using log-rank tests.
Supplementary Figure 2 | (A) GSEA showed several metastasis-related processes enriched in the high-risk group in the TCGA validation dataset. (B) GSEA showed several metastasis-related processes enriched in the high-risk group in the validation-2 cohort.
DRGs, DNA repair-related genes; DRGS, DNA repair-related gene signature; CRC, colorectal cancer; DEGs, differentially expressed genes; HR, hazard ratio; GEO, Gene Expression Omnibus; GSEA, Gene Set Enrichment Analysis; LASSO, least absolute shrinkage and selection operator; OS, overall survival; ROC, receiver operating characteristic; and TCGA, The Cancer Genome Atlas.
Boland, C. R., and Goel, A. (2010). Microsatellite Instability in Colorectal Cancer. Gastroenterology 138 (6), 2073–2087. doi:10.1053/j.gastro.2009.12.064
Bouwman, P., and Jonkers, J. (2012). The Effects of Deregulated DNA Damage Signalling on Cancer Chemotherapy Response and Resistance. Nat. Rev. Cancer 12 (9), 587–598. doi:10.1038/nrc3342
Braun, D. A., Hou, Y., Bakouny, Z., Ficial, M., Sant’ Angelo, M., Forman, J., et al. (2020). Interplay of Somatic Alterations and Immune Infiltration Modulates Response to PD-1 Blockade in Advanced clear Cell Renal Cell Carcinoma. Nat. Med. 26 (6), 909–918. doi:10.1038/s41591-020-0839-y
Bray, F., Ferlay, J., Soerjomataram, I., Siegel, R. L., Torre, L. A., and Jemal, A. (2018). Global Cancer Statistics 2018: GLOBOCAN Estimates of Incidence and Mortality Worldwide for 36 Cancers in 185 Countries. CA: A Cancer J. Clinicians 68 (6), 394–424. doi:10.3322/caac.21492
Burdak-Rothkamm, S., and Rothkamm, K. (2021). DNA Damage Repair Deficiency and Synthetic Lethality for Cancer Treatment. Trends Mol. Med. 27, 91–92. doi:10.1016/j.molmed.2020.09.011
Chen, X., and Song, E. (2019). Turning Foes to Friends: Targeting Cancer-Associated Fibroblasts. Nat. Rev. Drug Discov. 18 (2), 99–115. doi:10.1038/s41573-018-0004-1
Cooks, T., Pateras, I. S., Tarcic, O., Solomon, H., Schetter, A. J., Wilder, S., et al. (2013). Mutant P53 Prolongs NF-Κb Activation and Promotes Chronic Inflammation and Inflammation-Associated Colorectal Cancer. Cancer Cell 23 (5), 634–646. doi:10.1016/j.ccr.2013.03.022
Das, V., Kalita, J., and Pal, M. (2017). Predictive and Prognostic Biomarkers in Colorectal Cancer: A Systematic Review of Recent Advances and Challenges. Biomed. Pharmacother. 87, 8–19. doi:10.1016/j.biopha.2016.12.064
De Rosa, M., Rega, D., Costabile, V., Duraturo, F., Niglio, A., Izzo, P., et al. (2016). The Biological Complexity of Colorectal Cancer: Insights into Biomarkers for Early Detection and Personalized Care. Therap Adv. Gastroenterol. 9 (6), 861–886. doi:10.1177/1756283X16659790
De Simone, V., Franzè, E., Ronchetti, G., Colantoni, A., Fantini, M. C., Di Fusco, D., et al. (2015). Th17-type Cytokines, IL-6 and TNF-α Synergistically Activate STAT3 and NF-kB to Promote Colorectal Cancer Cell Growth. Oncogene 34 (27), 3493–3503. doi:10.1038/onc.2014.286
Diboun, I., Wernisch, L., Orengo, C. A., and Koltzenburg, M. (2006). Microarray Analysis after RNA Amplification Can Detect Pronounced Differences in Gene Expression Using Limma. BMC Genomics 7, 252. doi:10.1186/1471-2164-7-252
Friedberg, E. C. (2001). How Nucleotide Excision Repair Protects against Cancer. Nat. Rev. Cancer 1 (1), 22–33. doi:10.1038/35094000
Fung, K. Y., Nice, E., Priebe, I., Belobrajdic, D., Phatak, A., Purins, L., et al. (2014). Colorectal Cancer Biomarkers: to Be or Not to Be? Cautionary Tales from a Road Well Travelled. World J. Gastroenterol. 20 (4), 888–898. doi:10.3748/wjg.v20.i4.888
Gao, F., Wang, W., Tan, M., Zhu, L., Zhang, Y., Fessler, E., et al. (2019). DeepCC: a Novel Deep Learning-Based Framework for Cancer Molecular Subtype Classification. Oncogenesis 8 (9), 44. doi:10.1038/s41389-019-0157-8
Guinney, J., Dienstmann, R., Wang, X., de Reyniès, A., Schlicker, A., Soneson, C., et al. (2015). The Consensus Molecular Subtypes of Colorectal Cancer. Nat. Med. 21 (11), 1350–1356. doi:10.1038/nm.3967
Iyama, T., and Wilson, D. M. (2013). DNA Repair Mechanisms in Dividing and Non-dividing Cells. DNA Repair 12 (8), 620–636. doi:10.1016/j.dnarep.2013.04.015
Jorgovanovic, D., Song, M., Wang, L., and Zhang, Y. (2020). Roles of IFN-γ in Tumor Progression and Regression: a Review. Biomark Res. 8, 49. doi:10.1186/s40364-020-00228-x
Kandimalla, R., Gao, F., Matsuyama, T., Ishikawa, T., Uetake, H., Takahashi, N., et al. (2018). Genome-wide Discovery and Identification of a Novel miRNA Signature for Recurrence Prediction in Stage II and III Colorectal Cancer. Clin. Cancer Res. 24 (16), 3867–3877. doi:10.1158/1078-0432.CCR-17-3236
Kandimalla, R., Ozawa, T., Gao, F., Wang, X., Goel, A., Nozawa, H., et al. (2019). Gene Expression Signature in Surgical Tissues and Endoscopic Biopsies Identifies High-Risk T1 Colorectal Cancers. Gastroenterology 156 (8), 2338–2341. e3. doi:10.1053/j.gastro.2019.02.027
Knijnenburg, T. A., Wang, L., Zimmermann, M. T., Chambwe, N., Gao, G. F., Cherniack, A. D., et al. (2018). Genomic and Molecular Landscape of DNA Damage Repair Deficiency across the Cancer Genome Atlas. Cell Rep 23 (1), 239–e6. doi:10.1016/j.celrep.2018.03.076
Lech, G., Slotwinski, R., Slodkowski, M., and Krasnodebski, I. W. (2016). Colorectal Cancer Tumour Markers and Biomarkers: Recent Therapeutic Advances. World J. Gastroenterol. 22 (5), 1745–1755. doi:10.3748/wjg.v22.i5.1745
Liberzon, A., Birger, C., Thorvaldsdóttir, H., Ghandi, M., Mesirov, J. P., and Tamayo, P. (2015). The Molecular Signatures Database Hallmark Gene Set Collection. Cel Syst. 1 (6), 417–425. doi:10.1016/j.cels.2015.12.004
Lindahl, T., and Wood, R. D. (1999). Quality Control by DNA Repair. Science 286 (5446), 1897–1905. doi:10.1126/science.286.5446.1897
Lu, Y.-X., Ju, H.-Q., Wang, F., Chen, L.-Z., Wu, Q.-N., Sheng, H., et al. (2016). Inhibition of the NF-Κb Pathway by Nafamostat Mesilate Suppresses Colorectal Cancer Growth and Metastasis. Cancer Lett. 380 (1), 87–97. doi:10.1016/j.canlet.2016.06.014
Marcuello, M., Vymetalkova, V., Neves, R. P. L., Duran-Sanchon, S., Vedeld, H. M., Tham, E., et al. (2019). Circulating Biomarkers for Early Detection and Clinical Management of Colorectal Cancer. Mol. Aspects Med. 69, 107–122. doi:10.1016/j.mam.2019.06.002
Migliore, L., Migheli, F., Spisni, R., and Coppedè, F. (2011). Genetics, Cytogenetics, and Epigenetics of Colorectal Cancer. J. Biomed. Biotechnol. 2011, 1–19. doi:10.1155/2011/792362
Mimori, K., Saito, T., Niida, A., and Miyano, S. (2018). Cancer Evolution and Heterogeneity. Ann. Gastroenterol. Surg. 2 (5), 332–338. doi:10.1002/ags3.12182
Nejm Group (2012). Intratumor Heterogeneity and Branched Evolution. New Engl. J. Med. 366, 2132–2133. doi:10.1056/nejmc1204069
Ozawa, T., Kandimalla, R., Gao, F., Nozawa, H., Hata, K., Nagata, H., et al. (2018). A MicroRNA Signature Associated with Metastasis of T1 Colorectal Cancers to Lymph Nodes. Gastroenterology 154 (4), 844–848. doi:10.1053/j.gastro.2017.11.275
Phipps, A. I., Limburg, P. J., Baron, J. A., Burnett-Hartman, A. N., Weisenberger, D. J., Laird, P. W., et al. (2015). Association between Molecular Subtypes of Colorectal Cancer and Patient Survival. Gastroenterology 148 (1), 77–87. doi:10.1053/j.gastro.2014.09.038
Schröder, M. S., Culhane, A. C., Quackenbush, J., and Haibe-Kains, B. (2011). Survcomp: an R/Bioconductor Package for Performance Assessment and Comparison of Survival Models. Bioinformatics 27 (22), 3206–3208. doi:10.1093/bioinformatics/btr511
Shah, R., Jones, E., Vidart, V., Kuppen, P. J. K., Conti, J. A., and Francis, N. K. (2014). Biomarkers for Early Detection of Colorectal Cancer and Polyps: Systematic Review. Cancer Epidemiol. Biomarkers Prev. 23, 1712–1728. doi:10.1158/1055-9965.EPI-14-0412
Siegel, R. L., Miller, K. D., and Jemal, A. (2016). Cancer Statistics, 2016. CA: A Cancer J. Clinicians 66 (1), 7–30. doi:10.3322/caac.21332
Siegel, R. L., Fedewa, S. A., Anderson, W. F., Miller, K. D., Ma, J., Rosenberg, P. S., et al. (2017). Colorectal Cancer Incidence Patterns in the United States, 1974-2013. J. Natl. Cancer Inst. 109 (8), djw322. doi:10.1093/jnci/djw322
Subramanian, A., Tamayo, P., Mootha, V. K., Mukherjee, S., Ebert, B. L., Gillette, M. A., et al. (2005). Gene Set Enrichment Analysis: A Knowledge-Based Approach for Interpreting Genome-wide Expression Profiles. Proc. Natl. Acad. Sci. 102, 15545–15550. doi:10.1073/pnas.0506580102
Tibshirani, R. (1997). The Lasso Method for Variable Selection in the Cox Model. Stat. Med. 16 (4), 385–395. doi:10.1002/(sici)1097-0258(19970228)16:4<385::aid-sim380>3.0.co;2-3
Wang, X., Terfve, C., Rose, J. C., and Markowetz, F. (2011). HTSanalyzeR: an R/Bioconductor Package for Integrated Network Analysis of High-Throughput Screens. Bioinformatics 27 (6), 879–880. doi:10.1093/bioinformatics/btr028
Zhao, P., Li, L., Jiang, X., and Li, Q. (2019). Mismatch Repair Deficiency/microsatellite Instability-High as a Predictor for Anti-PD-1/pd-L1 Immunotherapy Efficacy. J. Hematol. Oncol. 12 (1), 54. doi:10.1186/s13045-019-0738-1
Zheng, Z.-X., Zheng, R.-S., Zhang, S.-W., and Chen, W.-Q. (2014). Colorectal Cancer Incidence and Mortality in China, 2010. Asian Pac. J. Cancer Prev. 15 (19), 8455–8460. doi:10.7314/apjcp.2014.15.19.8455
Keywords: DNA repair–related genes, prognostic, colorectal cancer, immunotherapy, microsatellite instability
Citation: Lv M-Y, Wang W, Zhong M-E, Cai D, Fan D, Li C-H, Kou W-B, Huang Z-P, Duan X, Hu C, Zhu Q, He X and Gao F (2022) DNA Repair–Related Gene Signature in Predicting Prognosis of Colorectal Cancer Patients. Front. Genet. 13:872238. doi: 10.3389/fgene.2022.872238
Received: 09 February 2022; Accepted: 07 March 2022;
Published: 11 April 2022.
Edited by:
Ka-Chun Wong, City University of Hong Kong, Hong Kong SAR, ChinaReviewed by:
Chieh-Lin Jerry Teng, Taichung Veterans General Hospital, TaiwanCopyright © 2022 Lv, Wang, Zhong, Cai, Fan, Li, Kou, Huang, Duan, Hu, Zhu, He and Gao. This is an open-access article distributed under the terms of the Creative Commons Attribution License (CC BY). The use, distribution or reproduction in other forums is permitted, provided the original author(s) and the copyright owner(s) are credited and that the original publication in this journal is cited, in accordance with accepted academic practice. No use, distribution or reproduction is permitted which does not comply with these terms.
*Correspondence: Xiaosheng He, aGV4c2hlbmdAbWFpbC5zeXN1LmVkdS5jbg==; Feng Gao, Z2FvZjU3QG1haWwuc3lzdS5lZHUuY24=
†These authors have contributed equally to this work
Disclaimer: All claims expressed in this article are solely those of the authors and do not necessarily represent those of their affiliated organizations, or those of the publisher, the editors and the reviewers. Any product that may be evaluated in this article or claim that may be made by its manufacturer is not guaranteed or endorsed by the publisher.
Research integrity at Frontiers
Learn more about the work of our research integrity team to safeguard the quality of each article we publish.