- 1School of Agricultural Biotechnology, Punjab Agricultural University, Ludhiana, India
- 2Department of Plant Breeding and Genetics, Punjab Agricultural University, Ludhiana, India
- 3Department of Plant Pathology, Punjab Agricultural University, Ludhiana, India
- 4International Crops Research Institute for the Semi-Arid Tropics (ICRISAT), Hyderabad, India
Root-knot nematode (Meloidogyne graminicola) is one of the emerging threats to rice production worldwide that causes substantial yield reductions. There is a progressive shift of the cropping system from traditional transplanting to direct-seeded water-saving rice production that favored the development of M. graminicola. Scouting and deploying new resistance genes is an economical approach to managing the root-knot nematodes. Here, we report that the inheritance of root-knot nematode resistance in Oryza glaberrima acc. IRGC102206 is governed by a single dominant gene. Traditional mapping coupled with BSA-seq is used to map nematode resistance gene(s) using the BC1F1 population derived from a cross of O. sativa cv. PR121 (S) and O. glaberrima acc. IRGC102206 (R). One major novel genomic region spanning a 3.0-Mb interval on chromosome 6 and two minor QTLs on chromosomes 2 and 4 are the potential genomic regions associated with rice root-knot nematode resistance. Within the QTL regions, 19 putative candidate genes contain 81 non-synonymous variants. The detected major candidate region could be fine mapped to accelerate marker-assisted breeding for root-knot nematode resistance in rice.
Introduction
Rice (Oryza sativa L.) is one of the staple food crops that feed half of the world’s population. Its production has been continuously increasing at a constant pace for the last 10 years. However, to meet the demand of the ever-growing population, there is still a need to increase rice production by 2050 (Ray et al., 2013). Over the changing agro-climatic conditions, various biotic and abiotic stresses have emerged that threaten rice production across the globe. Among biotic stresses, plant-parasitic nematodes pose the foremost warning to rice production worldwide (Mhatre et al., 2017). About 300 nematode species of 35 genera infect rice, and the Meloidogyne genus is the first among the top 10 plant-parasitic nematodes (Nicol et al., 2011). Within the Meloidogyne genus, root-knot nematode (M. graminicola) is the most widespread threat nowadays in almost all rice-growing systems—upland, lowland, deepwater, and irrigated rice—and causes significant yield losses ranging from 20% to 80% (Mantelin et al., 2017; Kumar, 2020).
The second stage juveniles (J2) of root-knot nematode penetrate behind the root cap due to the absence of differentiated endodermis near the root tip (Bridge et al., 2005). After penetration, juveniles migrate intercellularly through the root cortex toward the apical meristem and the cellular differentiation region and establish a permanent feeding site in the vascular tissue to develop giant cells (Williamson, 1998). The metabolically active giant cells serve as a source of nutrients for nematodes to complete their life cycle between 19 and 27 days from juveniles to adults and release eggs around the root surface. These nematodes complete numerous generations in a single rice-growing season to build up a high damaging population in a shorter period (Shrestha et al., 2007). The mechanical disruption caused by the giant cells in metaxylem vessels interferes with the uptake of water and nutrients that strongly impair the root physiology and development (Singh, 2010). The disruption of water translocation and nutrient transport by the root vascular system leads to stunting, chlorosis, and loss of vigor, which eventually result in reduced growth and finally cause significant yield losses of the crop (Mantelin et al., 2017).
Some cultural practices are followed to limit the nematode population below a damaging threshold level. Flooding and crop rotation practices are partially effective and of limited use due to the broad host range of M. graminicola and an unacceptable cost of non-hosts, such as mung bean, mustard, and sesame, for small-scale farmers using rice as a staple food. The use of nematicides is uneconomic, unhealthy for the environment, and unsafe for human health. Alternatively, host resistance is effective and economical to manage root-knot nematode population densities below the threshold levels and gains significance in water-saving practices during the shifting of rice cultivation from irrigation to direct-seeded rice. Most Asian genotypes are susceptible to root-knot nematode, with only a few of them being resistant (Dimkpa et al., 2016). Natural resistance to M. graminicola has been reported in O. glaberrima (African rice) and O. longistaminata (Soriano et al., 1999). But due to the presence of sterility genes and the low yield potential of O. glaberrima, limited efforts have been made to introgress root-knot nematode resistance from O. glaberrima into O. sativa. However, fertility can be retained by recurring backcrossing for a few generations, but backcrossing for several generations increases the risk of losing desirable traits. Moreover, interspecific progenies do not show a similar type of resistance as O. glaberrima (Plowright et al., 1999). Several studies have reported the quantitative nature of resistance against M. graminicola as QTLs for root galling and the number of galls and eggs per root system have been identified using RIL populations (Shrestha et al., 2007; Jena et al., 2013; Galeng-Lawilao et al., 2018). In some crop systems, a single major gene confers resistance to different Meloidogyne species; for example, rkn1 confers resistance to M. incognita in cotton (Wang et al., 2006), Mi from Lycopersicon peruvianum (Roberts, 1995) gives resistance to some of the root-knot nematode species in tomato (Abad et al., 2003), and Hsa-1Og provides resistance against cyst nematode (Heterodera sacchari) in rice (Lorieux et al., 2003). Recently, Mhatre et al. (2017) have reported hypersensitive response (HR) in rice cultivar Zhonghua11 (Asian rice) against M. graminicola and suggested that resistance is due to major genes rather than quantitative resistance. There is still a continuous need to explore and exploit related species of rice for resistance to M. graminicola.
Identification and characterization of genes/QTLs responsible for root-knot nematode resistance are important not only to unveil the molecular mechanisms of resistance but also to deploy the resistance genes for the development of nematode-resilient rice cultivars. Different molecular mapping strategies have been used to map genes/QTLs for several traits in rice. Bulked segregant analysis (BSA) is one of the effective methods to map genes or QTLs from a population having two extreme phenotypic traits (Michelmore et al., 1991; Venuprasad et al., 2009). Recent development in next-generation sequencing technologies has provided effective tools for genome-wide identification of SNPs and other structural variants, and also genotyping (Huang et al., 2009) has accelerated genetic mapping studies and marker development. A “BSA-seq” approach that couples whole-genome re-sequencing and BSA of extreme phenotypes is cost-effective and rapidly identifies genomic regions associated with a trait of interest (Takagi et al., 2013; Deokar et al., 2019). This approach has been used successfully in different crops such as rice, tomato, chickpea, and brassica to map QTLs of different genetic complexities from single genes to major QTLs from last few years (Takagi et al., 2013; Illa-Berenguer et al., 2015; Deokar et al., 2019; Zhang et al., 2020). There is only one report of QTL identification for rice root-knot nematode resistance through BSA-seq analysis using mapping population derived from indica and aus cultivar (Lahari et al., 2019). The present study aims to 1) determine the genetics of M. graminicola resistance in O. glaberrima, 2) identify major locus associated with root-knot nematode resistance through BSA-seq, and 3) explore candidate genes conferring M. graminicola resistance and identification of SNPs within the candidate genes.
Materials and Methods
Plant Materials
The plant material consisted of O. glaberrima acc. IRGC102206, PR121, BC1F1, BC2F1, and BC2F2 populations derived from the cross of PR121 and O. glaberrima acc. IRGC102206 O. glaberrima acc. IRGC102206 were identified as highly resistant and susceptible to M. graminicola, respectively, in a previous study (Kaur, 2020). The resistance was confirmed over 2 years of screening both in the nematode-infested pots and sick plot under field conditions, as the number of galls per plant was significantly lower as compared to susceptible checks. O. glaberrima has characteristics of early maturity, moderate to tall height, seed shattering, lower yield, and more resistance to various diseases and pests. However, PR121 has short stature, bacterial blight resistance, and better lodging tolerance.
Phenotypic Evaluation of Backcross Generations for Nematode Infestation
The BC1F1, BC2F1, and BC2F2 populations were grown in the nursery during cropping seasons 2018–2020. The 25-day-old seedlings were transplanted in irrigated field conditions with plant-to-plant and row-to-row distances of 20 and 30 cm, respectively. Each plant of BC1F1, BC2F1, and BC2F2 after 15 days of transplanting was split into four plantlets, and three replicas of each BC1F1, BC2F1, and BC2F2 plant were transferred to a nematode-infested sick plot with an initial population density of 1 J2/g of soil while one replica was raised under non-infested conditions. Standard agronomic practices were implemented during the raising of the crop, except that the soil was not flooded during screening in the nematode-infested sick plot. Each plant of all populations was uprooted from the nematode-infested sick plot after 60 days of transplanting. The roots were washed immediately under running tap water to count the galls per root system. Root gall index was calculated on a scale of 1–5 as given by Gaur et al. (2001). Rating was done as follows: 1 for 0–1 gall (highly resistant), 2 for 1–10 galls (resistant), 3 for 11–30 galls (moderately resistant), 4 for 30–100 galls (susceptible), and 5 for >100 galls (highly susceptible). The segregation pattern for nematode resistance was checked in each generation using standard chi-square analysis for the goodness of fit. Data for each BC1F1 plant on different morphological characters like plant height (cm), root length (cm), fresh shoot weight (g), and fresh root weight (g) were measured immediately after the uprooting of the plants. The shoots and roots of each plant were packed separately in brown paper bags for drying, to achieve constant weight for measuring dry shoot and root weight (g). Data were analyzed using a generalized linear model (GLM) of SAS software version 9.4 (SAS Institute, Cary, NC, United States).
Traditional Rough Mapping
Genomic DNA was isolated from the young leaves of parents and each BC1F1 plant using the CTAB method (Doyle and Doyle, 1987). The degradation and contamination of DNA were checked on 0.8% agarose gel while DNA was quantified using Thermo scientific NanoDropTM 1000 spectrophotometer. A total of 512 simple sequence repeat (SSR) markers from the universal core genetic map (Orjuela et al., 2010) spanning all 12 rice chromosomes were used for the parental polymorphic survey on PR121 and O. glaberrima acc. IRGC102206. Primer sequences were retrieved from the Gramene database (http://www.gramene.org/; IRGSP, 2005). In vitro amplification using polymerase chain reaction (PCR) was performed in a 96-well PCR plate in Eppendorf and Applied Biosystems master cyclers. The total PCR reaction of 20 μl was prepared using the following components: 100 ng template DNA, 0.50 μM each of forward and reverse primers and 2× Emerald Amp® GT PCR Master Mix containing an optimized buffer, PCR enzyme, dNTP mixture, gel-loading dye (green), and a density reagent. A negative control (without template DNA) was included in each amplification reaction. PCR profile of 95°C for 5 min, followed by 30 cycles of 1 min at 94°C, 1 min at 55–60°C, and 1 min at 72°C with a final extension of 10 min at 72°C was used for amplification. The amplified products were resolved in 3.0% agarose gel, and amplicons were scored by comparing them to parental alleles. The linkage map was constructed using the Kosambi mapping function of QTL IciMapping version 4.1 (Meng et al., 2015) through MAP functionality in a graphic form representing the position of markers within linkage groups by using a threshold LOD score of 3.0. Composite interval mapping (CIM) at a 95% threshold level was used for the identification of QTLs based on 1,000 permutation tests using Windows QTL cartographer version 2.5 (Wang et al., 2012). The position of putative QTLs corresponded to the location (in centiMorgans) of peak LOD scores in the scan of individual chromosomes and was designated according to the chromosome position. The proportion of observed phenotypic variance attributable to the QTL was estimated by the coefficient of determination (R2) using the maximum likelihood of CIM.
Bulked Segregant Analysis Through Whole-Genome Re-Sequencing
The DNA concentration of each sample was normalized to 500 ng/μl, and 5 μg of the total DNA of the individual plant was used for making extreme bulks. An equal amount of DNA from 10 individual plants with few galls (1–2.33 galls) was mixed to generate the resistant DNA bulk. Similarly, an equal amount of DNA from 10 individual plants with higher gall numbers per plant (40–75 galls) was mixed to generate the susceptible DNA bulk (Figure 1). Paired-end sequencing libraries from the two extreme bulk and parents using 2 μg of DNA were prepared according to the Illumina manufacturer’s instructions. The sequencing of libraries using the Illumina HiSeqTM 2500 platform was outsourced from NGB Diagnostics Pvt Ltd., India. Illumina sequencing of genomic libraries for each of the parents and the two bulks (2 × 150 bp) produced 33–35 million reads per sample for a total of 136 million raw reads. The quality of raw reads was assessed using FASTQC (version 0.11.8; Andrews, 2010) with default parameters. Poor-quality sequences were filtered and removed while contaminated adapter sequences and any unwanted bias from their ends were trimmed using Trimmomatic (version 0.39; Bolger et al., 2014). A Phred score of 30 was kept as the overall quality threshold for raw reads. The filtered reads were further re-checked for quality using FASTQC.
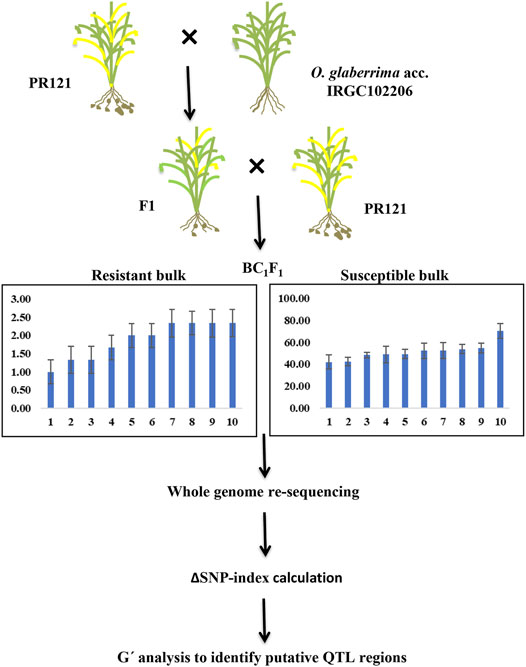
FIGURE 1. Flow chart for BSA-seq using BC1F1 individuals derived from the cross of PR121 (S) and O. glaberrima acc. IRGC102206 (R).
BSA-seq Analysis
High-quality sequences were aligned and mapped to the Oryza sativa Indica Group ASM465v1 reference sequence of cultivar 93-11, available at Ensembl plants (https://plants.ensembl.org/Oryza_indica), using Bowtie 2 algorithm with default parameters (version v2.0.0; Langmead and Salzberg, 2012). The Bowtie 2 default mode is faster than all Burrows-Wheeler Aligner (BWA) modes and more than 2.5 times faster than the BWA default mode. All Bowtie 2 modes aligned a greater number of reads than either BWA or short oligonucleotide alignment program 2 (SOAP2). To keep only uniquely mapping reads, the output SAM files were converted into BAM files, then read groups were added, sorted, and indexed using SAMtools (version 0.1.19; Li et al., 2009). The output BAM files containing uniquely mapped reads were used for SNP Calling through the GATK (Genome Analysis Toolkit) pipeline. Subsequently, GATK’s HaplotypCaller component (version 4.0; Mckenna et al., 2010) was used to perform a joint variant calling of all samples. The indels and missing data were filtered out using the variant filtration parameter of vcftools (https://github.com/vcftools/vcftools). The filtered VCF file in table format was used as an input file for QTLseqr (https://github.com/bmansfeld/QTLseqr) package developed by Mansfeld and Grumet (2018). SNPs with a reference allele frequency of 0.2 from both the bulks were filtered out as these might be due to sequencing or alignment error. In G′ approach, run GprimeAnalysis first counted the number of SNPs within the sliding window, and then a tricube-smoothed ∆SNP index was calculated within a window size of 1.0-Mb genomic region. The ∆SNP index (>0.1) was used to calculate p-values (<0.05) and an FDR(q) of 0.01 to identify potential QTLs associated with root-knot nematode resistance. The G′ determines the statistical significance of QTLs as background noise is less and also addresses the linkage disequilibrium (LD) between SNPs. One important advantage of this method is that p-values can be estimated for each SNP using non-parametric estimation of the null distribution of G′ (Magwene et al., 2011). Comparison of the QTL-seq method (Delta-SNP index) and G′ method by Mansfeld and Grumet (2018) showed that a confidence interval of 99% with the QTL-seq method was not as stringent as using an FDR of 0.01 in the G′ method. All commands and codes for BSA-seq analysis are available at https://github.com/bmansfeld/QTLseqr.
Identification of Candidate Genes
The QTL regions harboring the candidate genes based on the annotation of Oryza sativa indica Group ASM465v1 (https://plants.ensembl.org/Oryza_indica) were identified. To identify non-synonymous SNPs among the candidate genes of the two parents (PR121 and O. glaberrima), nucleotide changes were investigated using ExPASy translate tool (http://web.expasy.org/translate/). Potential candidate genes and their corresponding Ensembl IDs were further subjected to the ShinyGO v0.74 database (Ge et al., 2019) to obtain gene ontology (GO) annotation against O. sativa subsp. indica. GO enrichment was calculated by a p-value cut-off (FDR) at 0.05 for the genes.
Results
Inheritance of Root-Knot Nematode Resistance
The F1s (with medium-sized ligule) generated from the cross of PR121 (S) and O. glaberrima acc. IRGC102206 (R) were partial to completely sterile; therefore these F1s were backcrossed with PR121 to develop the BC1F1 population. A total of 10,800 spikelets of F1 plants were cross-pollinated and a 0.95% seed setting of BC1F1 (103 seeds) was obtained. Out of the 103 seeds, 69 seeds (67%) were germinated in the nursery and transplanted in controlled (normal) conditions. After 25 days of transplanting, three replicas of each BC1F1 plant along with their parental genotypes were screened in the nematode-infested sick plot. Based on gall number, 39 and 30 BC1F1 plants were categorized as resistant and susceptible, respectively, for root-knot nematode resistance that corresponded to a single locus segregation ratio statistically (Table 1). The resistant parent IRGC102206 showed gall number from 0 to 1 while the susceptible parent PR121 exhibited gall number in the range of 64–82 (Figure 2). The average gall number and gall index among BC1F1 individuals ranged from 1.00 to 70.33 and 1.33 to 4.00, respectively (Table 2). The resistant parent O. glaberrima accession IRGC102206 had an overall mean gall index of 1.0 while the susceptible parent PR121 had a mean gall index of 4.0. The segregation pattern in successive generations, that is, BC2F1 and BC2F2, was authenticated, thereby confirming that nematode resistance is governed by a single dominant gene (Table 1). Overall, BC1F1 plants showed stunted growth under nematode infestation. Plant height and root length among BC1F1 plants ranged from 61 to 118 cm and 7.57 to 21.03 cm, respectively. Significant differences were observed among BC1F1 plants for all the traits (Table 2). There was a nominal decrease in growth parameters in the resistant individuals compared to the susceptible plants indicating that the plant growth parameters were affected by nematode infestation.

TABLE 1. Genetic analysis of root-knot nematode resistance in different generations derived from the cross of PR121 (S) and O. glaberrima acc. IRGC102206 (R).
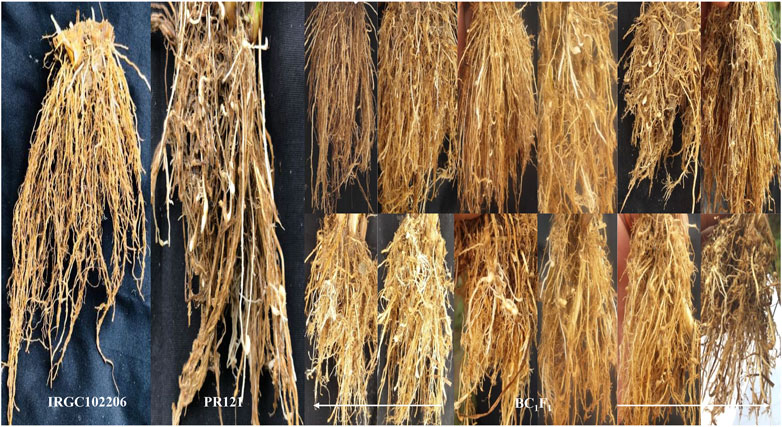
FIGURE 2. Response of O. glaberrima acc. IRGC102206 (R), PR121 (S), and their derived BC1F1 progenies upon M. graminicola infestation.
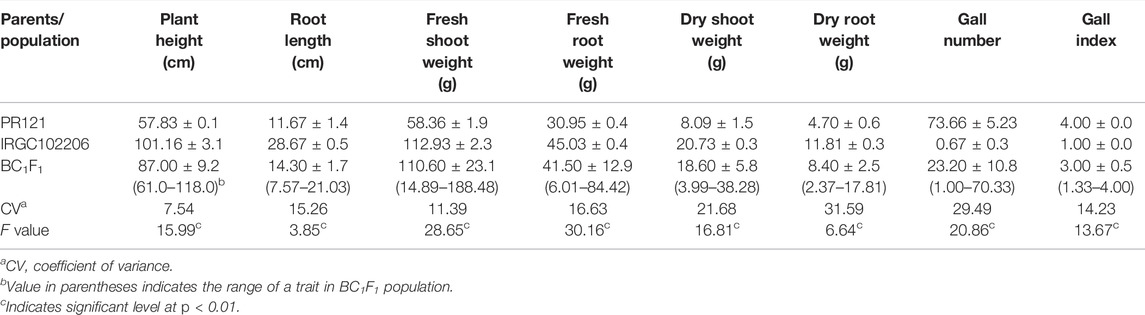
TABLE 2. Means of growth parameters, gall number, and gall index of parents and BC1F1 population derived from the cross of PR121 (S) and O. glaberrima acc. IRGC102206 (R) in nematode-infested conditions.
Traditional Mapping
We also carried out rough QTL mapping using 69 individuals of the BC1F1 population. A total of 512 microsatellite markers spanning 12 chromosomes of rice were used for the parental polymorphic survey and the markers per chromosome varied from 53 (chromosomes 1 and 2) to 31 (chromosome 9). Parental polymorphism among all chromosomes ranged from 7.6% to 50.9% with an average of 31.25%. A comprehensive list of polymorphic SSR markers is given in Supplementary Table S1. A total of 100 polymorphic SSR markers were genotyped and a genetic linkage map was generated with a total map length of 1,901.21 cM, with an average distance of 22.63 cM. One putative QTL associated with gall numbers was detected on chromosome 6 designated as qGN6.1, between the marker interval of RM3183 and RM27001 explaining the phenotypic variance of 41% at an LOD score of 3.95 (Table 3). Two QTLs for dry root weight and dry shoot weight (qDRW3.1 and qDRW3.2) were co-localized on chromosome 3 whereas QTL for fresh root weight, qFRW8.1, was located on chromosome 8 with 17% of total phenotypic variance (Table 3).
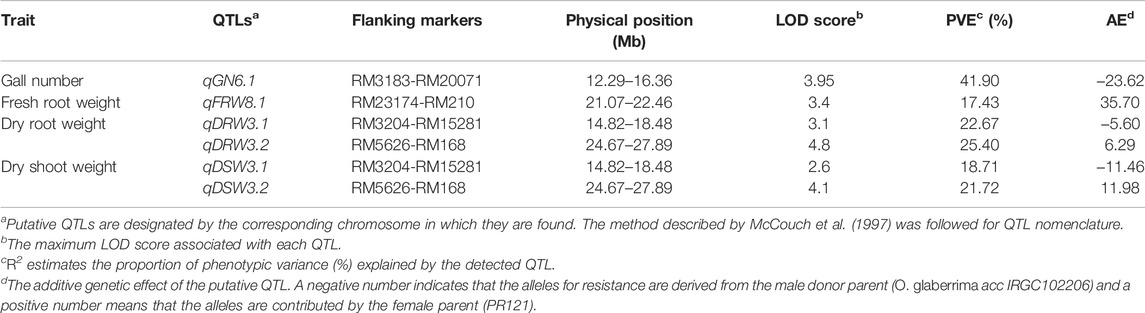
TABLE 3. Chromosomal locations and parameters associated with the quantitative trait loci (QTL) for resistance to rice root-knot nematode in BC1F1 population derived from the cross of PR121 (S) and O. glaberrima acc. IRGC102206 (R).
Whole-Genome Re-Sequencing of Bulked Segregant Analysis Pools
Based on the gall number of BC1F1 population, 10 plants from each of the extreme values of frequency distribution were selected and pooled as resistant bulk (RB) and susceptible bulk (SB), respectively. The whole-genome re-sequencing data from PR121, IRGC102206, resistant, and susceptible bulks were aligned with the O. sativa cultivar 93-11 reference genome using Bowtie 2 algorithm with default parameters. A total of 35.0, 33.6, 34.3, and 34.0 million paired-end reads were generated from IRGC102206, PR121, RB, and SB, respectively (Table 4), and 32–34 million reads of paired-end sequences were retained which were equivalent to 12.8× to 14.9× coverage of the rice genome indicating the high quality of the sequencing data (Supplementary Figure S1). Approximately, 31.23 (96.11%), 29.78 (87.66%), 31.07 (93.33%), and 31.05 (93.86%) million read pairs of PR121, IRGC102206, RB, and SB were uniquely mapped to the reference genome, respectively (Table 4). The GC content of raw reads ranged from 42% to 43% for all the samples.

TABLE 4. Statistical summary of BSA-seq data of parental lines, resistant bulk, and susceptible bulk.
A total of 3,692,066 variants (SNPs/indels) were identified by a joint variant calling with the reference genome through the GATK pipeline. RB had the highest variant (1,641,906) followed by IRGC102206 (1,049,225), PR121 (695,856), and SB (305,079). Maximum SNPs (284,012) were specific to chromosome 1 while chromosome 11 had the least SNPs (123,492). Likewise, the variant rate (SNPs/Mb) also varied among chromosomes, and chromosome 6 possessed the highest variant rate (1 SNP/195 bases) while the lowest variant rate (1 SNP/136 bases) was detected for chromosome 7 (Supplementary Table S2). A total of 1,416,115 SNPs were detected between RB and SB. Based on reference allele frequency (0.20) and maximum total depth (300), 174,651 SNPs were used for QTL identification through BSA-seq analysis.
BSA-seq Identifies Root-Knot Nematode Resistance Major Locus on Chromosome 6
The BSA-seq analysis detected nine putative QTL regions on chromosomes 2, 3, 4, 6, 11, and 12, based on the calculation of Gʹ values of SNP within a window size of 1.0-Mb genomic region across the entire length of chromosomes. QTLs on chromosomes 3, 6, and 12 with large G′ peaks above FDR(q) of 0.01 were considered as major QTL regions responsible for root-knot nematode resistance in rice. Despite the major G′ peaks, five minor G′ peaks at the margin of significance have been identified on chromosomes 2, 3 (left to qNR3.1), 4, and 12 (on both sides of qNR12.1). Two adjacent QTLs on chromosome 3, namely, qNR3.1 and qNR3.2, had peaked in genomic intervals of 22.00–23.3 Mb and 24.0–25.6 Mb, respectively (Figure 3B). Similarly, QTLs on chromosome 12, designated as qNR12.1 and qNR12.2, were located at 5 Mb distance between intervals of 10.5–12.7 Mb and 17.5–22.9 Mb, respectively. qNR12.2 was present at the proximal region on the long arm of chromosome 12 displaying two adjacent component peaks (Figure 3F). The region covered by significant QTLs varied from 0.2 Mb (qNR4.1) to 5.4 Mb (qNR2.2). The direction of the ΔSNP index value and the allele frequency difference (AFD) indicated that qNR2.1, qNR4.1, qNR6.1, and qNR6.2 originated from the donor parent IRGC102206 (Table 5). However, QTLs on chromosomes 3, 11, and 12 originated from a susceptible parent, PR121. The number of SNPs present in qNR3.1, qNR3.2, qNR11.1, qNR12.1, and qNR12.2 region was 192, 330, 328, 691, and 322, respectively.
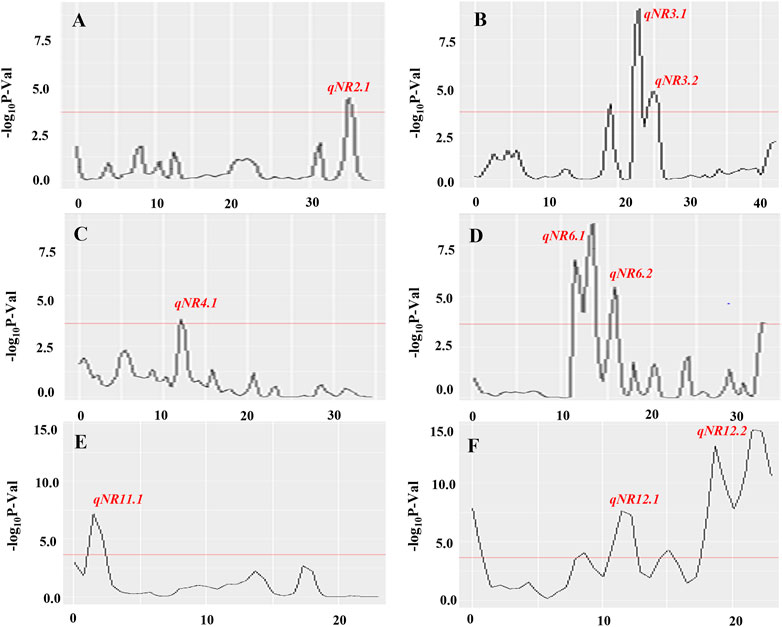
FIGURE 3. Quantitative trait loci for root-knot nematode resistance identified on chromosomes 2 (A), 3 (B), 4 (C), 6 (D), 11 (E), and 12 (F) using BSA-seq analysis. Distribution of –log10 p-value was calculated within a 1.0-Mb sliding window using tricube-smoothed kernel. The Y-axis represents – log10 p-values and the X-axis represents the position of chromosomes in Mb. The red line represents the significance threshold for FDR = 0.01, and the genomic region where –log10 p-value crosses the threshold was considered as significant QTL. Out of 12 chromosomes, significant QTLs identified on six chromosomes are shown.
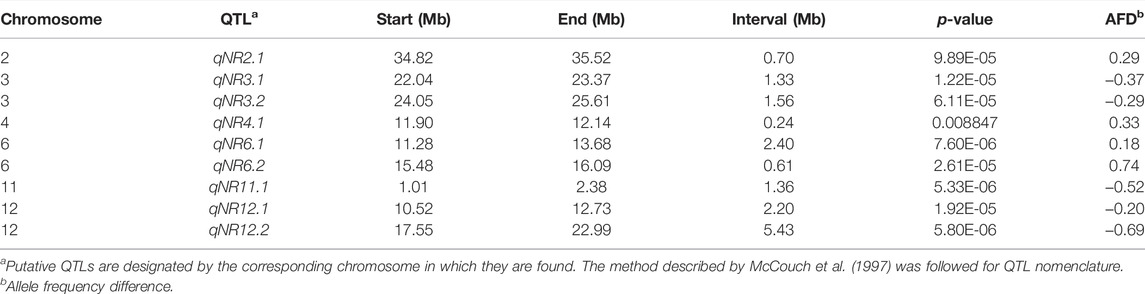
TABLE 5. List of QTLs identified through BSA-seq for rice root-knot nematode (M. graminicola) resistance.
The genomic interval between qNR6.1 and qNR6.2 was 2.4 Mb (11.2–13.6 Mb) and 0.6 Mb (15.4–16.0 Mb), respectively. The main Gʹ peak exhibited a subpeak in the QTL, qNR6.1, region whereas qNR6.2 exhibited a clear sharp peak region and fall near the centromere region (ranging approximately 17.00–22.59 Mb) of the short arm of chromosome 6. These two QTLs must be in continuity suggesting nematode resistance locus spanned within 3.0-Mb regions indicating a significant association for nematode resistance and hence considered as the major locus. The peaks of qNR2.1 and qNR4.1 were at the margin of significance, that is, qNR2.1 peak slightly exceeding and qNR4.1 nearly reaching the threshold; and, therefore, referred to as minor QTLs. The number of SNPs present in qNR2.1, qNR4.1, qNR6.1, and qNR6.2 regions was 345, 103, 264, and 27, respectively. Among all QTLs, qNR6.1 and qNR12.2 showed the highest Gʹ peaks that greatly exceeded the threshold level indicating the candidate regions for root-knot nematode resistance and susceptibility, respectively. Also, the genomic interval for root-knot nematode resistance on chromosome 6 overlapped with the identified gall number QTL (qGN6.1) through SSR markers. Therefore, qNR6.1 and qNR6.2 were selected as promising QTL regions originating from the donor parent for the identification of candidate genes and their sequence variations allied with root-knot nematode resistance.
Candidate Genes Present Within the Identified QTLs
The candidate genes within the QTL regions were identified based on BGI annotation. The genomic interval of candidate genes and their annotation have been summarized in Supplementary Table S3. The total number of candidate genes annotated in QTLs, qNR3.1, qNR3.2, qNR11.1, qNR12.1, and qNR12.2, was 7, 10, 16, 10, and 66, respectively. A total of nine annotated genes were identified within a 2.4-Mb interval of qNR6.1 and three genes within 0.6-Mb interval of qNR6.2. The less number of annotated genes within the QTL (qNR6.1 and qNR6.2) interval is because of low recombination rate in the centromere region. In the qNR2.1 region, seven genes were annotated whereas none of the candidate genes was annotated in the 240-Kb region of qNR4.1. We emphasized on those QTLs which originated from the donor parent to identify non-synonymous SNPs in the candidate genes. Among the nine annotated genes of qNR6.1, eight genes contained a total of 55 non-synonymous SNPs. In the candidate region of qNR6.2, a total of 10 non-synonymous SNPs were detected (Figure 4). The candidate gene encoding glycosyltransferase (BGIOSGA022720) had a maximum number of non-synonymous substitutions by SNPs (15), while peroxidase (BGIOSGA022766) and thioredoxin reductase (BGIOSGA021328) had the least number of non-synonymous SNPs (1). The examination of sequence reads of peroxidase and thioredoxin reductase on Integrative Genome Viewer (IGV) showed that non-synonymous SNPs were specific to the resistant parent; however, the susceptible parent also shared identical alleles to the reference genome (Figure 5). The majority of SNPs have been identified in the coding regions of candidate genes; however, mitogen-activated protein kinase (BGIOSGA022897), fructose-6-P-1-phosphotransferase subunit alpha (BGIOSGA022810), peroxidase (BGIOSGA022766), and thioredoxin reductase (BGIOSGA021328) had intronic SNPs (Table 6). Comparison of amino acid sequences of glycosyltransferase (BGIOSGA021421) and auxin-responsive protein (BGIOSGA022887) revealed that none of the SNPs caused non-synonymous substitutions.
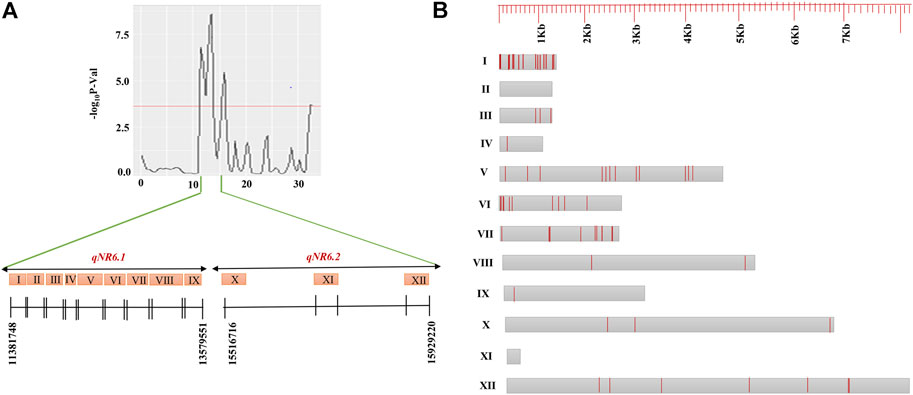
FIGURE 4. Candidate genes identified in QTLs, qNR6.1 (I–IX) and qNR6.2 (X–XII) (A), and intronic and missense variants identified along the total length of genes (B). Gray boxes represent the candidate genes and red lines represent the number of intronic and missense variants in genes.
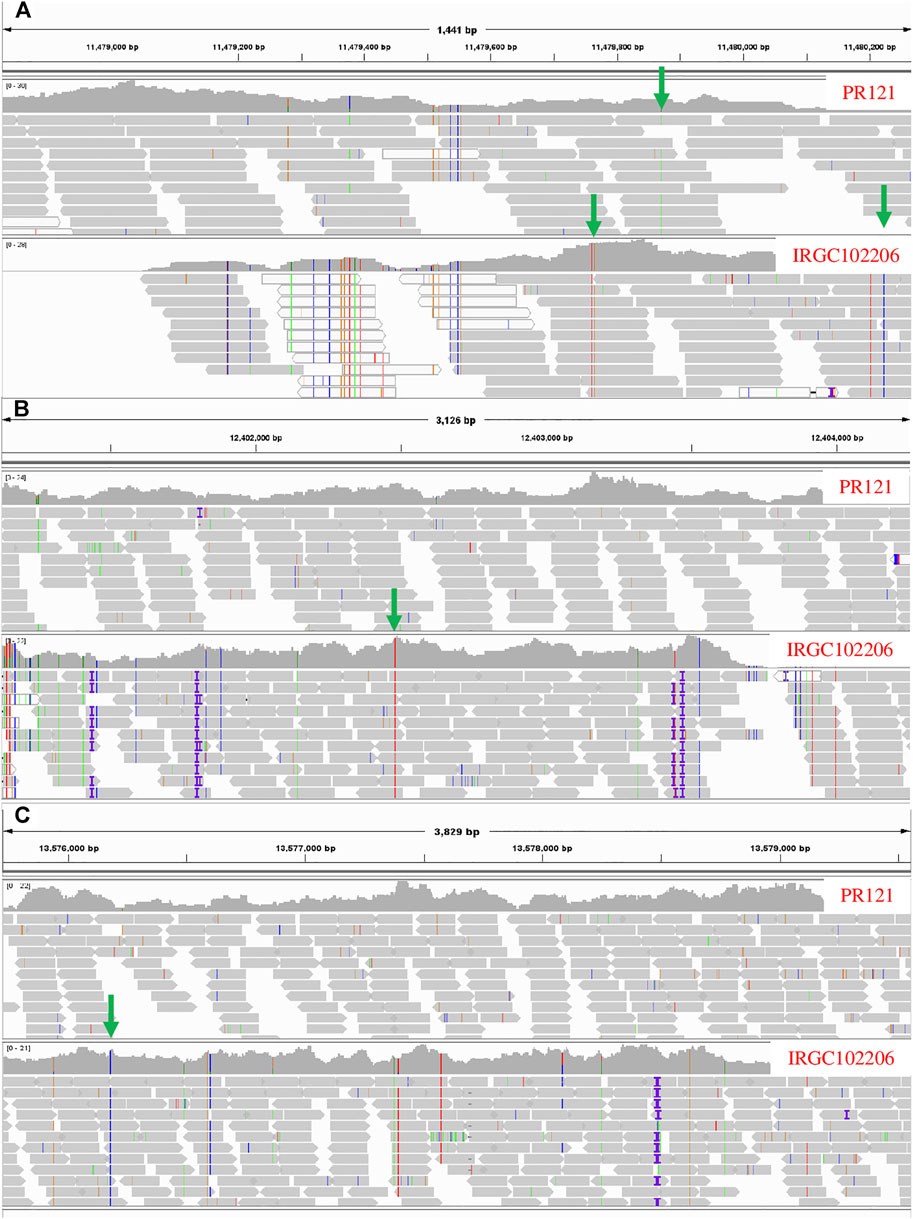
FIGURE 5. Integrative Genome Viewer showing glycosyltransferase (BGIOSGA022727) (A), peroxidase (BGIOSGA022766) (B), and thioredoxin reductase (BGIOSGA021328) (C) candidate genes in the susceptible parent (PR121) and the resistant parent (IRGC102206). The non-synonymous SNPs are highlighted with green arrows.
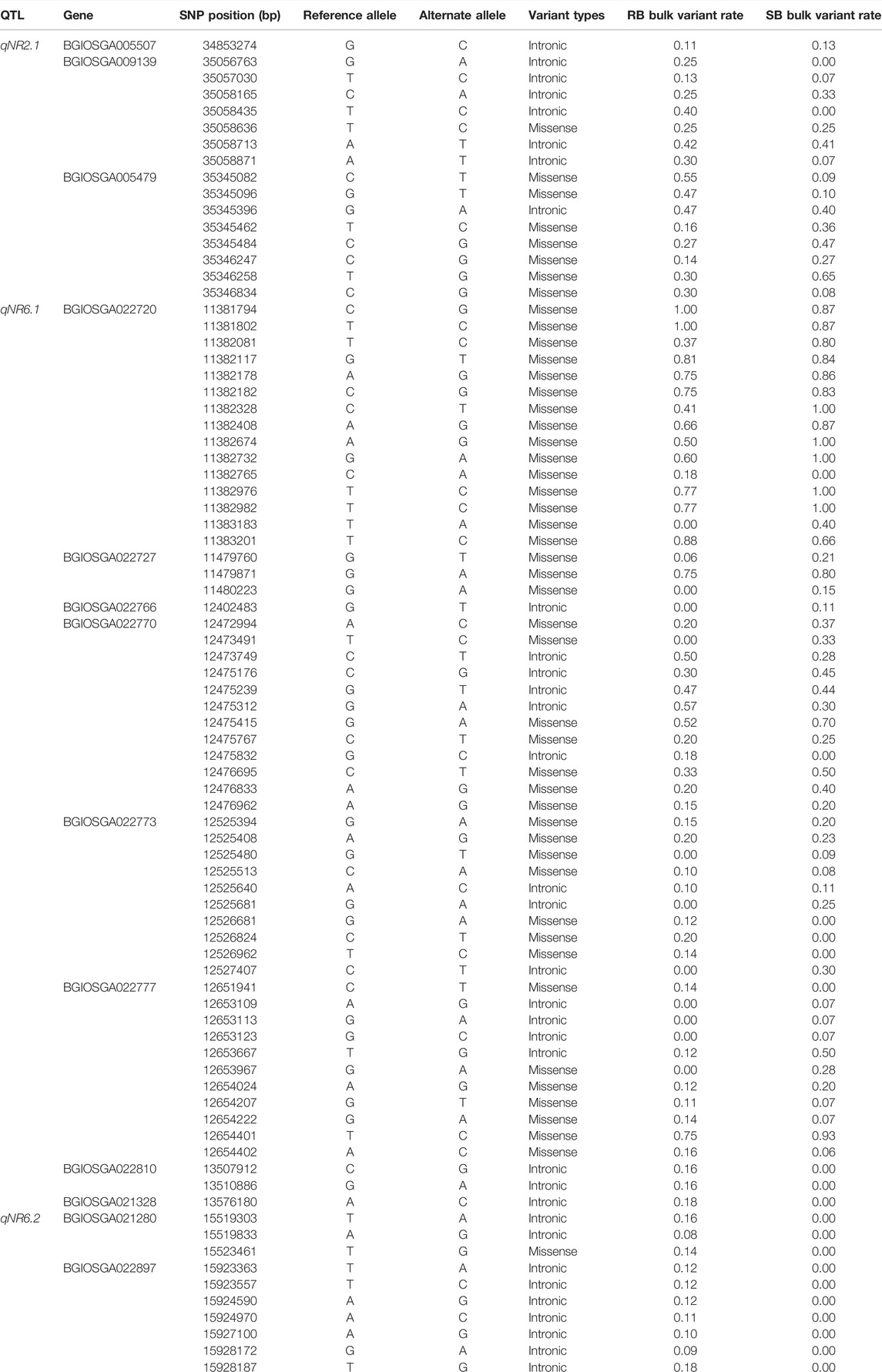
TABLE 6. Identification of non-synonymous SNPs in candidate genes present in putative genomic regions associated with rice root-knot nematode resistance.
In the qNR2.1 region, a total of 85 SNPs were identified within seven candidate genes and only 16 of them had non-synonymous substitution. Among these genes, the miR164 gene (ENSRNA049493468) had no SNP, and none of the SNPs in growth-regulating factor 1 (BGIOSGA005497) and hexosyl transferase (BGIOSGA005471) caused non-synonymous substitutions. Pectin esterase (BGIOSGA005479) genes had 36 synonymous SNPs and 8 non-synonymous SNPs. The maximum non-synonymous SNPs were present in pectinesterase (BGIOSGA005479) followed by a UMP-CMP kinase gene (BGIOSGA009139) among the candidate genes of qNR2.1 (Table 6). Therefore, candidate genes with non-synonymous SNPs could be the preferred genes for further studies. GO annotation showed the involvement of these genes in various biological processes and molecular functions. The candidate genes were predicted to be involved in different types of biosynthetic, metabolic, and catabolic processes (Figure 6). Transferase activity, protein metabolic process, and catabolic process appeared as the top enriched GO terms.
Discussion
Genetics and Mapping of Root-Knot Nematode Resistance in O. glaberrima
M. graminicola is emerging as a devastating pest and affects both upland and irrigated rice causing up to 80% yield losses (Mantelin et al., 2017). It has been observed that intensely galled roots were shorter than the non-infested plants because terminal galls inhibited the roots from further elongation and prevented the plant’s root system to absorb and translocate water (Kaur, 2020). Therefore, the severity of galling has a direct consequence on plant yield. To alleviate the plant damage and yield losses, breeding for rice root-knot nematode resistance is one of the efficient and most economical strategies. With the advent of molecular markers, several nematode resistance genes and QTLs have been identified in different crop species such as tomato, potato, cotton, sugarbeet, and cowpea (Cai et al., 1997; Vander-Vossen et al., 2000; Wang et al., 2006; Wu et al., 2009; Ndeve et al., 2018).
In the past, most studies reported that rice root-knot nematode resistance was a quantitative trait (Amoussou et al., 2004; Prasad et al., 2006; Shrestha et al., 2007; Jena et al., 2013; Galeng-Lawilao et al., 2018). Here, we report that nematode resistance in O. glaberrima acc. IRGC102206 is under dominant monogenic control. Ideally, in BC1F1, 1:1 segregation is expected for heterozygous alleles and homozygous alleles of the recurrent parent. Due to the presence of heterozygous alleles, the trait under consideration should be moderately resistant. However, we got plants of varying resistances, from resistant to moderate resistant, suggesting that there might be non-allelic minor modifying genes that might act in an additive manner such that the phenotype is a resultant of the copy number of the alleles present in the genotype toward resistance. Similarly, the BC2F2 progenies showed wide variation for gall numbers indicating that there are a few modifying minor genes which affect the expression of a major gene to alter the resultant disease reaction. Previously, O. glaberrima variety CG14 has been identified as resistant to M. graminicola (Plowright et al., 1999; Soriano et al., 1999). But O. glaberrima has not been explored further to study the genetics of root-knot nematode resistance due to hybrid sterility in the interspecific crosses. However, introgression of O. glaberrima genes is possible by repeated backcrossing and doubled haploid breeding, even though there is a risk of losing the desirable traits from the parents (Jones et al., 1997). However, Neelam et al. (2020) have successfully transferred the bacterial blight resistance gene xa45(t) into Pusa 44 background and mapped the gene on chromosome 8 from O. glaberrima acc. IRGC102600 by backcross breeding.
So far, no information is available for rice root-knot nematode resistance mapping in African rice cultivars. To the best of our knowledge, this is the first report on the identification of a major locus and a few minor QTLs for root-knot nematode resistance using interspecific BC1F1 population derived from a cross of O. sativa cv. PR121 and O. glaberrima acc. IRGC102206 by traditional QTL mapping and BSA-seq approach. Traditional QTL mapping identified one major large effect QTL for gall number, qGN6.1, explaining 41% of the phenotypic variance localized in the 12.29–16.36 Mb region with increasing alleles from the donor parent. This finding was consistent with the results of BSA-seq as the major locus for nematode resistance overlapped the genomic region between 11 and 16 Mb of chromosome 6, thereby validating the existence of a major QTL for root-knot nematode resistance in this region. We also detected QTLs on chromosomes 2 and 4 with minor effects using the BSA-seq approach. Based on the BC1F1 population, we anticipated that the SNP index of the RB had a mixture of PR121 and IRGC102206 alleles, and SB had PR121 alleles for the genes/QTLs associated with nematode resistance. The SNP index of the QTL regions on chromosomes 2, 4, and 6 agreed with this expectation, but the SNP index of the QTLs on chromosomes 1, 3, 11, and 12 displayed a contrasting blueprint. Thus, the present results inferred that QTLs on chromosomes 2, 4, and 6 probably conferred the nematode resistance, whereas QTLs on chromosomes 1, 3, 11, and 12 likely contributed to the susceptibility. Identification of the higher number of genomic regions contributed by PR121 is likely because we used a backcross population having a higher proportion of recurrent parent (PR121).
Previous studies identified QTLs for root galling, eggs per total root system and eggs per gram of roots, nematode reproduction, and nematode tolerance on chromosomes 1, 3, 4, 5, 7, 8, 9, 11, and 12 (Jena et al., 2013; Dimkpa et al., 2016; Galeng-Lawilao et al., 2018). To date, there is only one report inferring that a single dominant resistance gene (Mg1(t)) located on chromosome 10 in Asian rice cultivar Abhishek confers resistance against M. graminicola (Mhatre et al., 2017). So far, the Hsa-1Og nematode resistance gene has been identified from O. glaberrima TOG5681 on the long arm of chromosome 11 between markers RM206 and RM254 that confers resistance to the cyst nematode (Lorieux et al., 2003). Lahari et al. (2019) identified genomic region from 23 Mb to the bottom of rice chromosome 11 for root-knot nematode resistance through the QTL-seq approach, which might be co-localized with the results reported by Lorieux et al. (2003). Similarly, Dimkpa et al. (2016) also identified an SNP (id11008353) associated with gall number on the long arm of chromosome 11. Also, QTLs, qNR12.1 and qNR12.2, on chromosome 12 have different genomic locations for gall number–associated SNPs (Dimkpa et al., 2016). However, the BSA-seq analysis in our study identified QTL for root-knot nematode resistance on the short arm of chromosome 11 (1.01–2.38 Mb) contributed by the susceptible parent. A root-knot nematode resistance locus from 23 Mbp to the bottom of rice chromosome 11 was identified by Dimkpa et al. (2016). This might be because different sources of root-knot nematode resistance were used to identify QTL regions, as the nature of gene actions varies with the background.
Shrestha et al. (2007) identified a QTL for the gall number on the long arm of chromosome 6 flanked by R2654 and RG778 markers, explaining 9.6% of the total phenotypic variation. The results indicated that QTL, qGN6.1/qNR6.1-6.2, and QTL reported by Shrestha et al. (2007) were present on either sides of the centromere of chromosome 6, suggesting that gall number QTL identified in our population was controlled by a different genetic locus. QTLs/linked markers for root-knot nematode resistance identified in previous studies have been summarized in Supplementary Table S4. In the present study, one QTL for fresh root weight and two QTLs for dry shoot weight and dry root weight were identified on chromosomes 8 and 3 (two QTLs), respectively. The QTLs for dry shoot weight and dry root weight were co-localized. Earlier, the QTL for fresh root weight was mapped on chromosomes 2 and 12 (Galeng-Lawilao et al., 2018). It has to be noteworthy that in the present study, genomic regions identified for different traits through either traditional mapping or BSA-seq are unique. The major QTL could be fine mapped to identify the putative candidate gene for nematode resistance.
Potential Roles of Candidate Genes in Root-Knot Nematode Resistance
Among the 19 candidate genes, 13 genes harbored non-synonymous mutations. Three glycosyltransferase genes were present in the qNR6.1 region, two of which (BGIOSGA022720 and BGIOSGA022727) had a total of 18 non-synonymous substitutions. Glycosyltransferases are involved in carbohydrate biosynthesis and cell-wall synthesis (Egelund et al., 2004). The upregulation of glycosyltransferase genes provided resistance against Pseudomonas syringae and M. incognita and M. hapla in Arabidopsis and tomato, respectively (Langlois-Meurinne et al., 2005; Schaff et al., 2007). However, its role in M. graminicola resistance is not understood currently in rice. In the present study, the peroxidase (BGIOSGA022766) gene located in qNR6.1 plays an important role in ROS detoxification by regulating the H2O2 level and the oxidation of toxic reductants during pathogen attack. A higher level of peroxidase activity was reported in resistant genotypes of tomato during M. incognita infection (Chawla et al., 2013) and sweet potato (Yeon-Woo et al., 2019). Transcriptome profiling of M. graminicola infested rice roots showed the transcript abundance of peroxidases compared to uninfected roots (Kyndt et al., 2012). Rice root-knot nematode interactions revealed higher transcripts for nucleotide binding, catalytic, phosphatase, hydrolase, and ATPase activity after 3 and 7 days post inoculation (dpi) of M. graminicola (Haegeman et al., 2013).
Thioredoxin reductase has a role in ROS signaling and the protection of antioxidant enzymes to establish plant immunity during pathogen attacks. The ROS accumulation during pathogen attack results in oxidative modification of reactive free thiols in S-sulfenic acids (–SOH) of signaling proteins and antioxidant enzymes such as catalase (CAT). Thioredoxin reductase reduces these thiol modifications to enable ROS signaling and protection of antioxidant enzyme activities (Mata-Perez and Spoel, 2019). Similar to thioredoxin reductase, two genes of the qNR6.2 region, acyl-coenzyme A oxidase (BGIOSGA021280) and mitogen-activated protein kinase (BGIOSGA022897), might have a potential role in signaling pathways. Acyl-CoA oxidase metabolizes the pheromone (ascaroside) secreted by plant-parasitic nematode into chemical signals through the peroxisomal β-oxidation pathway that might act as a repellent for nematodes and thus reduces infection (Manohar et al., 2020). Mitogen-activated protein kinases (MAPKs) are intracellular signaling molecules that induce a defense response against root-knot nematode. The differential expression of the MAPK genes occurred after the activation of the effector-triggered immunity (ETI) pathway in rice upon M. graminicola infection as reported by Hatzade et al. (2020). It has been postulated that the genes involved in defense responses, phenylpropanoid, and hormone pathways were induced in response to M. graminicola infection in O. glaberrima line TOG5681, compared to Nipponbare (Petitot et al., 2017).
In the QTL region qNR2.1, the kinesin-like protein gene identified has diverse roles in biotic and abiotic stresses ranging from environmental to developmental processes like cell division, cell expansion, tropisms, and hormonal signaling. Kinesin modulates the cell wall structure and function by affecting the orientation and structure of cellulose microfibrils within the cell wall (Abdelkhalek et al., 2019). Therefore, kinesin-like protein and glycosyltransferase might be involved in the synthesis and maintenance of cell wall integrity during plant–nematode interactions. Growth-regulating factors are transcription factors regulated post-transcriptionally by miRNA396 (miR396) in different plant species (Omidbakhshfard et al., 2015). The growth-regulating factors, GRF1 and GRF3, in Arabidopsis target a plethora of genes having roles in programmed cell death, hormone signaling, and basal defense responses during biotic stresses (Piya et al., 2020). GRF1and GRF3 control the development and differentiation of syncytia during nematode (Heterodera schachtii) infection in Arabidopsis. In the present study, we also identified GRF1 (BGIOSGA009139) in the qNR6.2 region. The presence of non-synonymous SNPs in these candidate genes suggests their potential role for root-knot nematode resistance in rice.
Conclusion
Understanding the genetic basis of root-knot nematode resistance and the development of functional markers to follow marker-assisted breeding is the prerequisite for nematode resistance breeding. In this study, we have identified a major QTL and two minor QTLs for M. graminicola resistance. Also, QTLs for fresh root weight, dry root weight, and dry shoot weight were detected on chromosomes 8 and 3, respectively. These results revealed the novel region of 11.03–16.25 Mb on chromosome 6 harboring qNR6.1 and qNR6.2 that may provide the basis for fine mapping and further exploration of novel genes associated with root-knot resistance in rice. A total of 10 and 3 candidate genes within qNR6.1-qNR6.2 and qNR2.1, respectively, had non-synonymous SNPs that might be important for nematode resistance. The non-synonymous SNPs identified could be converted into KASP markers for effective deployment in rice breeding. The present results will increase our knowledge in understanding the molecular mechanism for root-knot nematode resistance.
Data Availability Statement
The datasets presented in this study can be found in online repositories. The names of the repository/repositories and accession number(s) can be found in the article/Supplementary Material.
Author Contributions
Conceptualization: YV, GM, and KS; methodology: GK, YV, and ND; performed the experiments: GK, KN and PP; analyzed the data: IY, GK, and DB; original draft: GK and YV; finalized the manuscript: GK, YV, and KN; funding: YV. All authors approved the final version.
Funding
This work was supported by the Department of Science and Technology, Ministry of Science and Technology, New Delhi, in the form of Extramural Research Grant (File No. EMR/2016/007539).
Conflict of Interest
The authors declare that the research was conducted in the absence of any commercial or financial relationships that could be construed as a potential conflict of interest.
Publisher’s Note
All claims expressed in this article are solely those of the authors and do not necessarily represent those of their affiliated organizations, or those of the publisher, the editors and the reviewers. Any product that may be evaluated in this article, or claim that may be made by its manufacturer, is not guaranteed or endorsed by the publisher.
Supplementary Material
The Supplementary Material for this article can be found online at: https://www.frontiersin.org/articles/10.3389/fgene.2022.871833/full#supplementary-material
Supplementary Figure S1 | Low quality reads (A) filtered through FastQC to obtain clean 856 reads (B) for reference alignment.
References
Abad, P., Favery, B., Rosso, M.-N., and Castagnone-Sereno, P. (2003). Root-knot Nematode Parasitism and Host Response: Molecular Basis of a Sophisticated Interaction. Mol. Plant Pathol. 4 (4), 217–224. doi:10.1046/j.1364-3703.2003.00170.x
Abdelkhalek, A., Ismail, I. A., Dessoky, E. S., El-Hallous, E. I., and Hafez, E. (2019). A Tomato Kinesin-like Protein Is Associated with Tobacco Mosaic Virus Infection. Biotechnol. Biotechnol. Equip. 33, 1424–1433. doi:10.1080/13102818.2019.1673207
Amoussou, P. L., Ashurt, J., Bridge, J., Green, J., Jones, M., Koyama, M., et al. (2004). Broadly Based Resistance to Nematodes in the Rice and Potato Crops of Subsistence Farmers. DFID Plant Sci. Res. Programme Annu. Rep., 9–14.
Andrews, S. (2010). FastQC: A Quality Control Tool for High Throughput Sequence Data. Available online at: https://www.bioinformatics.babraham.ac.uk/projects/fastqc.
Bolger, A. M., Lohse, M., and Usadel, B. (2014). Trimmomatic: a Flexible Trimmer for Illumina Sequence Data. Bioinformatics 30 (15), 2114–2120. doi:10.1093/bioinformatics/btu170
Bridge, J., Plowright, R. A., and Peng DeLiang, P. D. (2005). “Nematode Parasites of Rice,” in Plant–parasitic Nematodes in Subtropical and Tropical Agriculture. Editors M. Luc, R. A. Sikora, and J. Bridge (Wallingford): CAB International), 87–130. doi:10.1079/9780851997278.0087
Cai, D., Kleine, M., Kifle, S., Harloff, H.-J., Sandal, N. N., and Marcker, K. A. (1997). Positional Cloning of a Gene for Nematode Resistance in Sugar Beet. Science 275, 832–834. doi:10.1126/science.275.5301.832
Chawla, N., Choudhary, K., Kaur, S., and Jindal, S. (2013). Changes in Antioxidative Enzymes in Resistant and Susceptible Genotypes of Tomato Infected with Root-Knot Nematode (Meloidogyne incognita). Indian J. Nematol. 43, 1–12.
Deokar, A., Sagi, M., Daba, K., and Taran, B. (2019). QTL Sequencing Strategy to Map Genomic Regions Associated with Resistance to Ascochyta Blight in Chickpea. Plant Biotechnol. J. 17 (1), 275–288. doi:10.1111/pbi.12964
Dimkpa, S. O., Lahari, Z., Shrestha, R., Douglas, A., Gheysen, G., and Price, A. H. (2016). A Genome-wide Association Study of a Global Rice Panel Reveals Resistance in Oryza Sativa to Root-Knot Nematodes. J. Exp. Bot. 67 (4), 1191–1200. doi:10.1093/jxb/erv470
Doyle, J. J., and Doyle, J. L. (1987). A Rapid DNA Isolation Procedure for Small Quantities of Fresh Leaf Tissue. Phytochem. Bull. 19, 11–15.
Egelund, J., Skjot, M., Geshi, N., Ulvskov, P., and Petersen, B. L. (2004). A Complementary Bioinformatics Approach to Identify Potential Plant Cell Wall Glycosyltransferase-Encoding Genes. Plant Physiol. 136, 2609–2620. doi:10.1104/pp.104.042978
Galeng-Lawilao, J., Kumar, A., and De-Waele, D. (2018). QTL Mapping for Resistance to and Tolerance for the Rice Root-Knot Nematode, Meloidogyne graminicola. BMC Genet. 19 (1), 1–17. doi:10.1186/s12863-018-0656-1
Gaur, H. S., Singh, R. V., Kumar, S., Kumar, V., and Singh, J. V. (2001). AICRP on Nematodes. New Delhi: Division of Nematology. IARI.
Ge, X., Jung, D., and Yao, R. (2019). ShinyGO: a Graphical Enrichment Tool for Animals and Plants. Bioinformatics 36 (28), 2628–2629. doi:10.1093/bioinformatics/btz931
Haegeman, A., Bauters, L., Kyndt, T., Rahman, M. M., and Gheysen, G. (2013). Identification of Candidate Effector Genes in the Transcriptome of the Rice Root Knot Nematode Meloidogyne graminicola. Mol. Plant Pathol. 14 (4), 379–390. doi:10.1111/mpp.12014
Hatzade, B., Singh, D., Phani, V., Kumbhar, S., and Rao, U. (2020). Profiling of Defense Responsive Pathway Regulatory Genes in Asian Rice (Oryza Sativa) against Infection of Meloidogyne graminicola (Nematoda: Meloidogynidae). 3 Biotech 10 (2), 60. doi:10.1007/s13205-020-2055-3
Huang, X., Feng, Q., Qian, Q., Zhao, Q., Wang, L., Wang, A., et al. (2009). High-throughput Genotyping by Whole-Genome Resequencing. Genome Res. 19, 1068–1076. doi:10.1101/gr.089516.108
Illa-Berenguer, E., Van-Houten, J., Huang, Z., and Vander-Knaap, E. (2015). Rapid and Reliable Identification of Tomato Fruit Weight and Locule Number Loci by QTL-Seq. Theor. Appl. Genet. 128, 1329–1342. doi:10.1007/s00122-015-2509-x
Jena, M., Mohapatra, S. L., Panda, R. S., Mohanty, S. K., Thatoi, H. N., and Sahu, S. C. (2013). Genetic Loci Associated with Root-Knot Nematode Resistance in Rice Cv. Ramakrishna. Oryza 50 (2), 132–139.
Jones, M. P., Dingkuhn, M., Alukosnm, G. K., and Semon, M. (1997). Interspecific Oryza Sativa L. X O. Glaberrima Steud. Progenies in Upland Rice Improvement. Euphytica 94, 237–246. doi:10.1023/A:1002969932224
Kaur, G. (2020). Mapping and Transfer of genes/QTLs for Nematode (Meloidogyne graminicola) Resistance from Oryza Glaberrima into Oryza Sativa L. dissertation/PhD’sThesis (Ludhiana: Punjab Agricultural University).
Kumar, V. (2020). Data from: Social Innovation for Agricultural Development: A Study of System of Rice Intensification in Bihar, India. Millenn. Asia 11 (1), 99–118. doi:10.1177/0976399619900615
Kyndt, T., Nahar, K., Haegeman, A., De-Vleesschauwer, D., Hofte, M., and Gheysen, G. (2012). Comparing Systemic Defence-Related Gene Expression Changes upon Migratory and Sedentary Nematode Attack in Rice. Plant Biol. 1, 73–82. doi:10.1111/j.1438-8677.2011.00524.x
Lahari, Z., Ribeiro, A., Talukdar, P., Martin, B., Heidari, Z., Gheysen, G., et al. (2019). QTL-seq Reveals a Major Root-Knot Nematode Resistance Locus on Chromosome 11 in Rice (Oryza Sativa L.). Euphytica 215 (7), 117. doi:10.1007/s10681-019-2427-0
Langlois-Meurinne, M., Gachon, C. M., and Saindrenan, P. (2005). Pathogen-responsive Expression of Glycosyltransferase Genes UGT73B3 and UGT73B5 Is Necessary for Resistance to Pseudomonas syringae Pv. Tomato in Arabidopsis. Plant Physiol. 139 (4), 1890–1901. doi:10.1104/pp.105.067223
Langmead, B., and Salzberg, S. L. (2012). Fast Gapped-Read Alignment with Bowtie 2. Nat. Methods 9 (4), 357–359. doi:10.1038/nmeth.1923
Li, H., Handsaker, B., Wysoker, A., Fennell, T., Ruan, J., Homer, N., et al. (2009). 1000 Genome Project Data Processing Subgroup. The Sequence Alignment/Map Format and SAMtools. Bioinformatics 25 (16), 2078–2079. doi:10.1093/bioinformatics/btp352
Lorieux, M., Reversat, G., Garcia-Diaz, S. X., Denance, C., Jouvenet, N., Orieux, Y., et al. (2003). Linkage Mapping of Hsa-1Og, a Resistance Gene of African Rice to the Cyst Nematode, Heterodera Sacchari. Theor. Appl. Genet. 107, 691–696. doi:10.1007/s00122-003-1285-1
Magwene, P. M., Willis, J. H., and Kelly, J. K. (2011). The Statistics of Bulk Segregant Analysis Using Next Generation Sequencing. PLoS Comput. Biol. 7 (11), e1002255. doi:10.1371/journal.pcbi.1002255
Manohar, M., Tenjo-Castano, F., Chen, S., Zhang, Y. K., Kumari, A., Williamson, V. M., et al. (2020). Plant Metabolism of Nematode Pheromones Mediates Plant-Nematode Interactions. Nat. Commun. 11 (1), 208. doi:10.1038/s41467-019-14104-2
Mansfeld, B. N., and Grumet, R. (2018). QTLseqr: An R Package for Bulk Segregant Analysis with Next-Generation Sequencing. Plant Genome 11 (2). doi:10.3835/plantgenome2018.01.0006
Mantelin, S., Bellafiore, S., and Kyndt, T. (2017). Meloidogyne graminicola: a Major Threat to Rice Agriculture. Mol. Plant Pathol. 18 (1), 3–15. doi:10.1111/mpp.12394
Mata-Perez, C., and Spoel, S. (2019). Thioredoxin-mediated Redox Signaling in Plant Immunity. Plant Sci. 279, 27–33. doi:10.1016/j.plantsci.2018.05.001
McCouch, S. R., Chen, X., Panaud, O., Temnykh, S., Xu, Y., Cho, Y. G., et al. (1997). Microsatellite Marker Development, Mapping and Applications in Rice Genetics and Breeding. Plant Mol. Biol. 35, 89–99. doi:10.1007/978-94-011-5794-0_9
McKenna, A., Hanna, M., Banks, E., Sivachenko, A., Cibulskis, K., Kernytsky, A., et al. (2010). The Genome Analysis Toolkit: a MapReduce Framework for Analyzing Next-Generation DNA Sequencing Data. Genome Res. 20 (9), 1297–1303. doi:10.1101/gr.107524.110
Meng, L., Li, H., Zhang, L., and Wang, J. (2015). QTL IciMapping: Integrated Software for Genetic Linkage Map Construction and Quantitative Trait Locus Mapping in Biparental Populations. Crop J. 3, 269–283. doi:10.1016/j.cj.2015.01.001
Mhatre, P. H., Pankaj, A. S., Singh, A. K., Ellur, R. K., and Kumar, P. (2017). Molecular Mapping of Rice Root-Knot Nematode (Meloidogyne graminicola) Resistance Gene in Asian Rice (Oryza Sativa L.) Using STMS Markers. Indian J. Genet. 77, 163–165. doi:10.5958/0975-6906.2017.00022.0
Michelmore, R. W., Paran, I., and Kesseli, R. V. (1991). Identification of Markers Linked to Disease-Resistance Genes by Bulked Segregant Analysis – a Rapid Method to Detect Markers in Specific Genomic Regions by Using Segregating Populations. Proc. Natl. Acad. Sci. U.S.A. 88, 9828–9832. doi:10.1073/pnas.88.21.9828
Ndeve, A. D., Matthews, W. C., Santos, J. R. P., Huynh, B. L., and Roberts, P. A. (2018). Broad-based Root-Knot Nematode Resistance Identified in Cowpea Gene-Pool Two. J. Nematol. 50, 545–558. doi:10.21307/jofnem-2018-046
Neelam, K., Mahajan, R., Gupta, V., Bhatia, D., Gill, B. K., Komal, R., et al. (2020). High-resolution Genetic Mapping of a Novel Bacterial Blight Resistance Gene Xa-45(t) Identified from Oryza Glaberrima and Transferred to Oryza Sativa. Theor. Appl. Genet. 133 (3), 689–705. doi:10.1007/s00122-019-03501-2
Nicol, J. M., Turner, S. J., Coyne, D. L., den-Nijs, L., Hockland, S., and Maafi, Z. T. (2011). “Current Nematode Threats to World Agriculture,” in Genomics and Molecular Genetics of Plant-Nematode Interactions. Editors J. T. Jones, G. Gheysen, and C. Fenoll (Heidelberg): Springer), 21–43. doi:10.1007/978-94-007-0434-3_2
Omidbakhshfard, M. A., Proost, S., Fujikura, U., and Mueller-Roeber, B. (2015). Growth-Regulating Factors (GRFs): A Small Transcription Factor Family with Important Functions in Plant Biology. Mol. Plant 8 (7), 998–1010. doi:10.1016/j.molp.2015.01.013
Orjuela, J., Garavito, A., Bouniol, M., Moreno-Mayano, L. T., Kimball, J., Wilson, G., et al. (2010). A Universal Core Genetic Map for Rice. Theor. Appl. Genet. 120, 563–572. doi:10.1007/s00122-009-1176-1
Petitot, A. S., Kyndt, T., Haidar, R., Dereeper, A., Collin, M., de-Almeida, E. J., et al. (2017). Transcriptomic and Histological Responses of African Rice (Oryza Glaberrima) to Meloidogyne graminicola Provide New Insights into Root-Knot Nematode Resistance in Monocots. Ann. Bot. 119 (5), 885–899. doi:10.1093/aob/mcw256
Piya, S., Liu, J., Burch-Smith, T., Baum, T. J., and Hewezi, T. (2020). A Role for Arabidopsis Growth-Regulating Factors 1 and 3 in Growth-Stress Antagonism. J. Exp. Bot. 71 (4), 1402–1417. doi:10.1093/jxb/erz502
Plowright, R. A., Coyne, D. L., Nash, P., and Jones, M. P. (1999). Resistance to the Rice Nematodes Heterodera Sacchari, Meloidogyne graminicola and M. incognita in Oryza Glaberrima and O. Glaberrima X O. Sativa Interspecific Hybrids. Nematology 1 (7), 745–751. doi:10.1163/156854199508775
Prasad, P. V. V., Boote, K. J., Allen-, L. H., Sheehy, J. E., and Thomas, J. M. G. (2006). Species, Ecotype and Cultivar Differences in Spikelet Fertility and Harvest Index of Rice in Response to High-Temperature Stress. Field crops Res. 95 (2-3), 398–411. doi:10.1016/j.fcr.2005.04.008
Ray, D. K., Mueller, N. D., West, P. C., and Foley, J. A. (2013). Yield Trends Are Insufficient to Double Global Crop Production by 2050. PLoS One 8 (6), e66428. doi:10.1371/journal.pone.0066428
Roberts, P. A. (1995). Conceptual and Practical Aspects of Variability in Root-Knot Nematodes Related to Host Plant Resistance. Annu. Rev. Phytopathol. 33 (1), 199–221. doi:10.1146/annurev.py.33.090195.001215
Schaff, J. E., Nielsen, D. M., Smith, C. P., Scholl, E. H., and Bird, D. M. (2007). Comprehensive Transcriptome Profiling in Tomato Reveals a Role for Glycosyltransferase in Mi-Mediated Nematode Resistance. Plant Physiol. 144 (2), 1079–1092. doi:10.1104/pp.106.090241
Shrestha, R., Uzzo, F., Wilson, M. J., and Price, A. H. (2007). Physiological and Genetic Mapping Study of Tolerance to Root‐knot Nematode in Rice. New Phytol. 176 (3), 665–672. doi:10.1111/j.1469-8137.2007.02185.x
Singh, P. (2010). The Rice Root-Knot Nematode, Meloidogyne graminicola: An Emerging Problem in Rice-Wheat Cropping System. Indian J. Nematol. 40, 1–11.
Soriano, I. R., Schmit, V., Brar, D. S., Prot, J. C., and Reversat, G. (1999). Resistance to Rice Root-Knot Nematode Meloidogyne graminicola Identified in Oryza Longistaminata and. O. Glaberrima. Nematol. 1 (4), 395–398. doi:10.1163/156854199508397
Takagi, H., Abe, A., Yoshida, K., Kosugi, S., Natsume, S., Mitsuoka, C., et al. (2013). QTL-seq: Rapid Mapping of Quantitative Trait Loci in Rice by Whole-Genome Resequencing of DNA from Two Bulked Populations. Plant J. 74 (1), 174–183. doi:10.1111/tpj.12105
Vander-Vossen, E. A. G., Rouppe vander Voort, J. N. A. M., Kanyuka, K., Bendahmane, A., Sandbrink, H., Baulcombe, D. C., et al. (2000). Homologues of a Single Resistance-Gene Cluster in Potato Confer Resistance to Distinct Pathogens: a Virus and a Nematode. Plant J. 23, 567–576. doi:10.1046/j.1365-313x.2000.00814.x
Venuprasad, R., Dalid, C. O., Del-Valle, M., Zhao, D., Espiritu, M., Sta-Cruz, M. T., et al. (2009). Identification and Characterization of Large-Effect Quantitative Trait Loci for Grain Yield under Lowland Drought Stress in Rice Using Bulk-Segregant Analysis. Theor. Appl. Genet. 120 (1), 177–190. doi:10.1007/s00122-009-1168-1
Wang, C., Ulloa, M., and Roberts, P. A. (2006). Identification and Mapping of Microsatellite Markers Linked to a Root-Knot Nematode Resistance Gene (Rkn1) in AcalaNem X Cotton (Gossypium Hirsutum L.). Theor. Appl. Genet. 112, 770–777. doi:10.1007/s00122-005-0183-0
Wang, S., Basten, C. J., and Zeng, Z. B. (2012). Windows QTL Cartographer 2.5. Raleigh, NC, USA: Department of Statistics, North Carolina State University. Available online at: http://statgen.ncsu.edu/qtlcart/WQTLCart.htm.
Williamson, V. M. (1998). Root-knot Nematode Resistance Genes in Tomato and Their Potential for Future Use. Annu. Rev. Phytopathol. 36, 277–293. doi:10.1146/annurev.phyto.36.1.277
Wu, W. W., Shen, H. L., and Yang, W. C. (2009). Sources for Heat Stable Resistance to Southern Root-Knot Nematode (Meloidogyne incognita) in Solanum lycopersicum. Agric. Sci. China 8, 697–702. doi:10.1016/S1671-2927(08)60267-9
Yeon-Woo, S., Lee, I., Shim, D., Lee, K. L., Nam, K., Yang, J. W., et al. (2019). Transcriptomic Changes in Sweet Potato Peroxidases in Response to Infection with the Root-Knot Nematode Meloidogyne incognita. Mol. Biol. Rep. 46 (4), 4555–4564. doi:10.1007/s11033-019-04911-7
Keywords: BSA-QTLseq, candidate genes, Oryza glaberrima, SNPs, Meloidogyne graminicola
Citation: Kaur G, Yadav IS, Bhatia D, Vikal Y, Neelam K, Dhillon NK, Praba UP, Mangat GS and Singh K (2022) BSA-seq Identifies a Major Locus on Chromosome 6 for Root-Knot Nematode (Meloidogyne graminicola) Resistance From Oryza glaberrima. Front. Genet. 13:871833. doi: 10.3389/fgene.2022.871833
Received: 08 February 2022; Accepted: 02 May 2022;
Published: 14 June 2022.
Edited by:
Sukhwinder Singh, The International Maize and Wheat Improvement Center (CIMMYT), IndiaReviewed by:
Youlu Yuan, Cotton Research Institute (CAAS), ChinaAamir W. Khan, University of Missouri, United States
Copyright © 2022 Kaur, Yadav, Bhatia, Vikal, Neelam, Dhillon, Praba, Mangat and Singh. This is an open-access article distributed under the terms of the Creative Commons Attribution License (CC BY). The use, distribution or reproduction in other forums is permitted, provided the original author(s) and the copyright owner(s) are credited and that the original publication in this journal is cited, in accordance with accepted academic practice. No use, distribution or reproduction is permitted which does not comply with these terms.
*Correspondence: Yogesh Vikal, eXZpa2FsLXNvYWJAcGF1LmVkdQ==