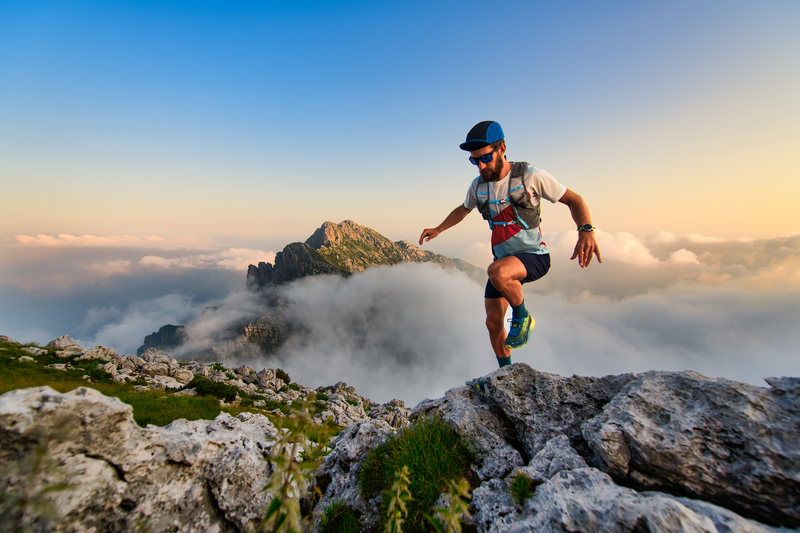
94% of researchers rate our articles as excellent or good
Learn more about the work of our research integrity team to safeguard the quality of each article we publish.
Find out more
ORIGINAL RESEARCH article
Front. Genet. , 24 January 2022
Sec. RNA
Volume 12 - 2021 | https://doi.org/10.3389/fgene.2021.787800
This article is part of the Research Topic RNA-Mediated Epigenetic and Transcriptional Regulation View all 10 articles
Background: From previous studies, we found that there are more than 100 types of RNA modifications in RNA molecules. m6A methylation is the most common. The incidence rate of adenocarcinoma of the esophagogastric junction (AEG) at home and abroad has increased faster than that of stomach cancer at other sites in recent years. Here, we systematically analyze the modification pattern of m6A mRNA in adenocarcinoma at the esophagogastric junction.
Methods: m6A sequencing, RNA sequencing, and bioinformatics analysis were used to describe the m6A modification pattern in adenocarcinoma and normal tissues at the esophagogastric junction.
Results: In AEG samples, a total of 4,775 new m6A peaks appeared, and 3,054 peaks disappeared. The unique m6A-related genes in AEG are related to cancer-related pathways. There are hypermethylated or hypomethylated m6A peaks in AEG in differentially expressed mRNA transcripts.
Conclusion: This study preliminarily constructed the first m6A full transcriptome map of human AEG. This has a guiding role in revealing the mechanism of m6A-mediated gene expression regulation.
In recent years, more and more research studies are based on epigenetics. Epigenetics is a study of reversible and inheritable phenotypes. This study does not involve changes in nuclear DNA sequences (Ng and Gurdon, 2008). RNA epigenetics includes N1-methyladenosine (m1A), N6-methyladenosine (m6A), 5-methylcytidine (m5C), and 7-methylguanosine (m7G) (Zhao et al., 2017). Among them, m6A, discovered in the 1970s, is the most abundant internal modification of mRNA in most eukaryotes (Chen and Wong, 2020). It involves almost all stages of the life cycle, including normal and pathological processes, for example, animal development (Frye et al., 2018), gene expression regulation (Roundtree et al., 2017), and human diseases (Hsu et al., 2017). With the discovery of research, the occurrence and development of many diseases are closely related to the changes in m6A modification, including tumors (Li J. et al., 2021), obesity (Zhao et al., 2014), infertility (Tang et al., 2018), autoimmune diseases (Zhang Y. et al., 2019), neurological diseases (Wu et al., 2019) and so on. Desrosiers et al. (1974) found that about 0.1–0.4% of adenosine in isolated RNA is modified by m6A in Sox. Transcriptome-wide research reveals that m6A modifications are enriched in the 3′-untranslated regions (UTRs) near the stop codons of mRNAs and it has a consensus sequence of RRACH (R = G or A; H = A, C, or U) (Dominissini et al., 2012). m6A modifications are mainly mediated by “writers,” “erasers” and “reader” proteins (Liu et al., 2014). Writers traditionally include methyltransferase-like 3 and 14 proteins (METTL3 and METTL14) and their cofactors WTAP (Wilms tumor suppressor-1-associated protein) (Ping et al., 2014; Schwartz et al., 2014). METTL3 is a member of the S-adenosine-L-methionine-dependent methyltransferase family, and is the main catalytically active enzyme for m6A methylation modification and is highly conserved in eukaryotes (Schöller et al., 2018). METTL14 has no catalytic domain and has no enzymatic activity, but it can form a stable heterodimer complex with METTL3 (Zhang et al., 2020). Therefore, METL3 and METL14 constitute the core and structure of the complex, respectively (Wang et al., 2016). WTAP is a pre-mRNA splicing regulator with independent methylation sites. It is mainly responsible for assisting the targeting of METL3 and METL14 to nuclear sites and can specifically methylate some m6A sites (Zheng et al., 2019; Liu S. et al., 2020; Zhang et al., 2021). There are also new research findings, methyltransferase-like protein 16 (methyltransferase like 16, METL16) (Aoyama et al., 2020), CCCH-type zinc finger protein 13 (Zinc finger CCCH-type containing 13, ZC3H13) (Wen et al., 2018), RNA binding motif protein 15/15B (RNA binding motif protein 15/15B, RBM15/15B) (Wang T. et al., 2020) is also a component of the methyltransferase complex and can participate in m6A methylation modification. Demethylation is achieved by another enzyme family called demethylases (erasers), mainly including FTO and ALKBH5 (Jia et al., 2011; Zheng et al., 2013). In addition to writers and erasers, m6A readers also play an important role in m6A methylation (Shi et al., 2019). Readers which can recognize m6A modification contain the YT521B homology (YTH) domain (Liu et al., 2018). The YTH domain in human cells, including the YTH domain family (YTHDF1-3), YTH domain-containing 1 (YTHDC1), and YTH domain-containing 2 (YTHDC2), have conserved the m6A binding domain (Qin et al., 2021). Recent studies have also reported that eukaryotic initiation factor 3 (eIF3) (Liu T. et al., 2020), heterogenous nuclear ribonucleoprotein A2B1 (heterogenous nuclear ribonucleoprotein A2B1, HNRNPA2B1) (Li K. et al., 2021), insulin-like growth factor 2 messenger ribonucleic acid Binding protein 1/2/3 (insulin-like growth factor 2 mRNA binding protein 1/2/3, IGF2BP1/2/3) (Huang et al., 2018) can also be used as m6A reading protein. However, there are still few studies on the related mechanisms of m6A methylation.
Gastrointestinal (GI) cancers are one of the most common malignancies, accounting for more than one-fourth of the newly diagnosed cancers worldwide (more than 4 million new cases per year) (Macha et al., 2014; Zhang S. et al., 2019). Among the GI cancers, the esophagogastric junction, or esophagogastric junction (EGJ), is a special anatomical site with a remarkably high risk of adenocarcinoma (Keeney and Bauer, 2006). The incidence of adenocarcinoma of the esophagogastric junction (AEG) has been increasing both in the West and East (Steevens et al., 2010; Yamashita et al., 2017; Imamura et al., 2019). There has been much debate as to the optimal therapy for AEG, and the debate continues (Kauppila and Lagergren, 2016). Here, we demonstrate the presence of m6A modification in adenocarcinoma of esophagogastric junction via m6A-methylated RNA immunoprecipitation (MeRIP) sequencing (MeRIP-seq), a powerful strategy for transcriptome-wide mapping of RNA modifications in mRNAs (Lin et al., 2018). We report transcriptome m6A profiling in adenocarcinoma of esophagogastric junction samples and the tumor-adjacent normal tissues for the first time.
Four pairs of matched adenocarcinoma of the esophagogastric junction and para-cancerous tissue samples were derived from adenocarcinoma of esophagogastric junction patients who underwent radical surgery in Affiliated People’s Hospital of Jiangsu University, Zhenjiang from July 2018 to November 2018. Adenocarcinoma of esophagogastric junction tissue was excised from the central part of the tumor; the paired paracancerous tissue was taken from normal tissue that was more than 5 cm from the edge of the tumor and had a negative margin. The collection process for all tissue samples was completed within 30 min after the tumor was isolated. The fresh tissue was cut into tissue pieces of about 5 mm in diameter and quickly placed in a sterilized cryotube and stored in an ultra-low temperature freezer at −80°C.
For each group, four biological replicates were selected, of which every two were combined into one. Then, the total RNA of tissue was extracted using TRIzol reagent (Invitrogen Corporation, CA, United States) in accordance with the manufacturer’s instructions. The Ribo-Zero rRNA Removal Kit (Illumina, Inc., CA, United States) was used to reduce the ribosomal RNA content of total RNAs. Then, the RNA was chemically fragmented into fragments of about 100 nucleotides in length using fragmentation buffer (Illumina, Inc.).
Total RNA was harvested from tissue samples and underwent a quality control (QC) process using NanoDrop ND-1000 (Thermo Fisher Scientific, MA, United States). High-throughput m6A and mRNA sequencing were performed by Cloudseq Biotech, Inc. (Shanghai, China) according to the published procedure with slight modifications. Briefly, fragmented RNA was incubated with anti-m6A polyclonal antibody (Synaptic Systems, 202003, Goettingen, Germany) in IP, immunoprecipitation buffer at 4°C for 2 h (Wang H.-F. et al., 2020). The mixture was then immunoprecipitated by incubation with protein-A beads (Thermo Fisher Scientific) at 4°C for an additional 2 h. Then, bound RNA was eluted from the beads with N6-methyladenosine (Berry & Associates, PR3732, Dexter, United States) in IP buffer and then extracted with Trizol reagent (Thermo Fisher Scientific) according to the manufacturer’s instruction. Purified RNA was used for RNA-seq library generation with NEBNextR Ultra™ RNA Library Prep Kit (New England Biolabs, MA, United States). Both the input samples without immunoprecipitation and the m6A IP samples were subjected to 150 bp paired-end sequencing on Illumina HiSeq sequencer, Illumina, CA, United States.
Paired-end reads were harvested from Illumina HiSeq 4000 sequencer and were quality controlled by Q30. After 3′adapter-trimming and low-quality reads were removed using cutadapt software (v1.9.3) (Kechin et al., 2017), the high-quality clean reads of all libraries were aligned to the reference genome (UCSC HG19) by Hisat2 software (v2.0.4) (Kim et al., 2015). For m6A sequencing, methylated sites on RNAs (m6A peaks) were identified by MACS software (Zhang et al., 2008). Differentially methylated sites were identified by diffReps (Shen et al., 2013). For mRNA sequencing, raw counts were identifiedby HTSeq software (v0.9.1) and normalized by edgeR software. Differentially expressed mRNAs were identified by p-value and fold change. Gene ontology (Geistlinger et al., 2021) and pathway enrichment analysis (Tian et al., 2005) were performed based on the differentially methylated protein coding genes and differentially expressed mRNAs.
Three genes with differentially methylated sites according to m6A-seq were tested by reverse transcription RT-qPCR. A portion of fragmented RNA was saved as the input control. The rested RNA was incubated with anti-m6A antibody-coupled beads. The m6A-containing RNAs were then immunoprecipitated and eluted from the beads.
The following are the gene-specific qPCR primers:
PLCG2:Forward:AGCTTAACCTTCAACCTGTGTG, Reverse:AAGATAGCTTTTACGGTTGGGT
TPR:Forward:TGCTTTTGGAGAAACTAGAGAACA, Reverse:TGGCGTTTCAAAATTGGTGC
DVL1:Forward:AGCTGCTTCTGTGTAAATGCT, Reverse:GTCCCATAAAATTAAACGCTTTT
GAPDH:Forward:GGCCTCCAAGGAGTAAGACC, Reverse:AGGGGAGATTCAGTGTGGTG
Data from three or more independent experiments were presented as the mean ± standard deviation (SD). Statistical analysis was done using SPSS 22.0 and GraphPad Prism 5.0 software. Paired Student t-tests were performed between cancer and adjacent normal samples. One-way analysis of variance was used to access the differences among three or more groups. Differences with p < 0.05 were defined as the threshold for significance.
Human AEG tissues versus tumor-adjacent normal tissues from four patients were selected for transcriptome-wide m6A-sequencing (m6A-seq) and RNA-sequencing (RNA-seq) assays. The original sequencing data IP is 50011084-103480210; Input is 49555862-60311666. After preprocessing of the original data (to remove the connector, to remove low-quality reads, to obtain high-quality clean reads), IP is 11574526-76461856; Input is 49100868-60160794 (Table 1). m6A is known to be a relatively abundant mRNA modification (Chen and Wong, 2020). In general, a total of 5,814 m6A peaks were identified by model-based analysis of ChIP-seq (MACS) (Nilsen, 2014) in the Ca group, representing transcripts of 4,259 genes. In the tumor-adjacent NC group, 4,093 m6A peaks were identified, which correspond with transcripts of 3,174 genes. Among them, 1,039 m6A peaks (only ∼12% of all peaks in cancer and normal groups) were detected within both adenocarcinomas of esophagogastric junction tissues and normal tissues. The low percentage of overlapping m6A peaks within mRNAs suggests differences in the m6A patterns between two groups (Figures 1A,B). By analyzing the distribution of m6A-modified peaks per gene, we found the majority of genes had one to three m6A modified sites, while a relatively small number of genes contain more (Figure 1C).
FIGURE 1. Characteristics of m6A methylation in AEG. (A) The conserved/specific m6A methylation peak. (B) The conserved/specific m6A methylation genes. (C) the distribution of m6A-modified peaks per gene. (D) The RRACH conserved sequence motif for m6A-containing peak regions. (E) The percentage of m6A methylated peaks in transcripts. Each transcript is divided into five parts: 5′UTRs, CDS, 3′UTRs, start codon, stop codon. (F) Accumulation of m6A peaks along with transcripts. m6A, N6-methyladenosine.
RNA methylation and demethylation begin with the motif binding of various binding proteins to methylation sites. A motif is essentially a sequence pattern of nucleic acids with biological significance, and these RNA methylation-related enzymes recognize and bind to these motifs, thereby affecting gene expression (Wang et al., 2019). To determine if the m6A peaks that we identified contained the m6A consensus sequence of RRACH (where R represents purine, A is m6A, and H is a non-guanine base). The m6A methylomes were further mapped by De reme software. The sequence of the top 300 peaks with the largest enrichment factor of each group (50 bp on each side of the vertex) was taken, and the sequence of these peaks was scanned using De reme to find a meaningful motif sequence. Deme (Bailey, 2011) motif analysis of methylated mRNAs revealed the existence of some motifs containing the consensus sequences (RRACH) of m6A modification (Figure 1D).
Then, we analyzed the distribution of m6A in the whole transcriptome of Ca and NC samples. We determined the distribution of m6A reads along with transcripts in the m6A-IP and non-IP (input) samples, respectively. Both total and unique m6A peaks from the two groups were analyzed. m6A peaks were divided into transcription start codon (start c), 5′UTR, coding sequence (CDS), stop codon (stop c) and 3′UTR according to their locations in RNA transcripts. Intriguingly, In general, the m6A peaks were enriched in the vicinity of CDS, the stop codon, and the start codon (Figure 1E). As shown in Figure 1E, about 70% of m6A peaks are located in the intergenic region; more than 60% of them are located near the CDS region and stop codon region; while about 30% of m6A peaks are located in the 5′UTR, start codon, and 3′UTR region. The distribution trend of the two tissues is highly similar, which indicates that the classical recognition sequence of m6A methylation is conserved in human tissues.
To better understand the distribution of m6A peaks in the whole mRNA, we divided each m6A modified mRNA into three regions: 5′UTR, CDS, and 3′UTR, and calculated the distribution proportion of these three regions. It can be seen from Figure 1F that the curve of the whole region of 5′UTR changes very gently until the distribution proportion of m6A peaks increases rapidly near the start codon. On the whole, peaks in the CDS area are highly enriched, however, the curve change in the middle of the CDS area is also relatively gentle, which shows that the distribution of the peaks in the middle of CDS is relatively uniform. But there is a peak of m6A peaks near the stop codon, which indicates that the distribution of m6A peaks increases rapidly when the end of CDS is near the stop codon. In the 3′UTR region, m6A peaks decrease rapidly from the beginning of the 3′UTR to the 3 ′ ends (Figure 1F).
The abundance of the m6A peaks between NC and Ca samples was compared. Among the 1,039 m6A peaks detected in bothsamples, a total of 272 differentially methylated sites were chosen for further study. Among them, there are 188 m6A hypermethylation sites and 84 m6A hypomethylation sites (Figure 2A). According to the Integrative Genomics Viewer (IGV) software, the hypermethylation gene TPR and hypomethylation genes PLCG2 and DVL1 were displayed (Figures 2B–D).
FIGURE 2. Differentially methylated N6-methyladenosine peaks in the tumer and control groups. (A) 188 m6A hypermethylation sites and 84 m6A hypomethylation sites. (B) A representative up-methylated gene in the tumor group relative to the control group. (C,D) two representative down-methylated genes in the tumor group relative to the control group.
In the RNA-seq dataset (m6A-seq input library), we discovered that the global mRNA expression patterns between AEG samples and adjacent normal tissues were significantly different. We calculated gene expression to assign differentially expressed genes (DE genes) of the two tissues. Of the 20,308 mRNAs we have identified, 3,069 were significantly different, while 17,239 were not. Among them, 2,032 is up-expressed and 1,037 is down-expressed (fold change > 2, p < 0.05). The volcano Plot, scatter plot, and the hierarchical clustering of the RNA-seq data were shown in Figures 3A–C.
FIGURE 3. Identification of differentially expressed genes in AEG by RNA-seq. (A) the scatter plot of the RNA-seq data. (B) Volcano Plot of the RNA-seq data. (C) Heat map of RNA-seq data of Ca samples and adjacent normal tissues. Red and Green indicate the upregulation and downregulation of mRNAs, respectively.
By cross-analysis of the m6A-Seq and RNA-seq data, we studied the relationship between the expression level of the m6A modified gene and its mRNA. Using RNA-seq, we found that there were 3,069 differential expression mRNAs, of which 2032 is up-expressed and 1,037 is down-expressed (fold change >2, p < 0.05). In 158 hyper-methylated mRNAs detected by m6A-Seq, we found thirty targets with upregulated mRNA transcripts (fold change > 2, p < 0.05), namely “hyper-up” (Table 2). The expression of 9 hypermethylation mRNA was downregulated (fold change > 2, p < 0.05), namely “hyper-down” (Table 3). In the contrast, 7 of 77 genes with hypomethylated m6A sites showed upregulated mRNA transcripts (fold change > 2, p < 0.05), namely, “hypo-up” (Table 4), and 11 of 77 genes with hypomethylated m6A sites showed downregulated mRNA transcripts (fold change > 2, p < 0.05), namely, “hypo-down” (Table 5). Notably, the numbers of “hyper-up” and “hypo-down” genes were more than those of “hyper-down” and “hypo-up” genes. To describe the relationship between differential m6A modification and its mRNA expression, we plotted a scatter plot. The results show that m6A modifications tend to have a positive correlation of mRNA expression in AEG. However, further analysis of the underlying mechanisms is needed (Figure 4A).
FIGURE 4. Differential expression mRNA harboring differentially methylated N6-methyladenosine sites. (A) Differentially expressed mRNA in AEG and matched normal tissue. (B) The ratio of mRNA expression levels in two samples containing tumor/normal-specific m6A peaks.
We were wondering whether the location of m6A peaks in mRNA transcripts was associated with gene expression levels. To further explore how m6A modification affects mRNA expression, we divided the gene containing m6A sites into PeakStart category (m6A peaks around start codon) and PeakStop (m6A peaks around stop codon). The results showed that the PeakStart category had higher overall expression levels (Figure 4B, note that the expression ratio of the tumor/normal gene is shown).
To uncover the functions of m6A modification in AEG tissues, mRNAs containing DMMSs were selected for GO (gene ontology) enrichment analysis and KEGG pathway analysis. Differently, m6A methylation genes are mainly involved in cell DNA metabolism and cell cycle process (Figures 5A,B). Pathway analysis revealed that mRNAs with hypermethylated and hypomethylated m6A sites were enriched in many pathways involved in cancer pathogenesis, including Pathways in cancer, Basal cell carcinoma, Wnt signaling pathway, HTLV-I infection, ErbB signaling pathway (Figures 6A,B).
FIGURE 5. GO-enrichment analysis for differentially methylated mRNAs (A) The top ten gene ontology terms of biological processes were significantly enriched for the up-methylated genes. (B) The top ten gene ontology terms of biological process significantly enriched for down-methylated genes.
FIGURE 6. Pathway analysis of mRNAs harboring differentially methylated N6-methyladenosine sites. (A) Bubble Plot showing the top ten enrichment scores of the significant enrichment pathway for the hyper-methylated genes. (B) Bubble Plot showing the top ten enrichment scores of the significant enrichment pathways of the hypo-methylated genes.
The differentially expressed genes were selected for ingenuity gene ontology and pathway analysis. It revealed that differentially expressed genes were significantly enriched in biological processes involving DNA metabolic process, cell cycle process (Figures 7A,B). Moreover, pathway analysis showed that nucleotide excision repair, cell cycle, and DNA replication were significantly altered in AEG samples (Figures 8A,B).
FIGURE 7. GO-enrichment analysis for differentially expressed mRNAs. (A) Top 10 biological processes of mRNA enrichment up-regulated by differences. (B) Top 10 biological processes of mRNA enrichment were differentially down-regulated.
FIGURE 8. Pathway analysis for differentially expressed mRNAs. (A) Up-regulated mRNAs related pathway analysis. (B) Down-regulated mRNAs related pathway analysis.
To further confirm the results of our m6A-seq data, we conducted gene-specific MeRIP-qPCR assays for several hypermethylated and hypomethylated genes (TPR, DVL1, and PLCG2). TPR is hypermethylated, but PLCG2, DVL1 are hypomethylated (Table 6 shows the initial detection of methylation of three genes). MeRIP-qPCR results showed that TPR was hypermethylated, while DVL1, PLCG2 were hypomethylated (Figures 9A–C, p < 0.05). We observed the same m6A-level changes in three out of the three genes (100%), demonstrating the reliability of our transcriptome-wide m6A-seq data.
FIGURE 9. Gene-specific m6A qPCR assays and detection of global m6A levels. (A–C) Gene-specific m6A qPCR validation of m6A level changes of three representative hyper-methylated or hypo-methylated genes in the normal control group and the AEG group samples. (D) Relative mRNA level of representative genes was measured by real-time PCR in normal control group and AEG group samples.
Sequentially, we verified the expression of mRNA by qPCR. We chose PLCG2 as the validation gene. The above results show that the expression of PLCG2 is downregulated, and the fold change is 2.935315743 (Table 7). And after verification by qPCR, the results indicate that PLCG2 is downregulated (Figure 9D, p < 0.05). The results of qPCR and MeRIP-qPCR elaborated that the PLCG2 acting as a hypomethylated gene, its expression was down-expressed.
The results of qPCR and MeRIP-qPCR showed that the Melcurve Plots of GAPDH and three mRNAs were single peaks, and the inflection points of each Amplification Plot were obvious, the overall parallelism was good, and the baseline was smooth without rising, indicating that the PCR amplification product specificity, amplification efficiency.
The recent studies have been proven the m6A modification in evolution conservation (Miao et al., 2021; Song et al., 2021), thus, the conservation could be considered in our analysis. Taking PLCG2 as an example, Search for “PLCG2” returns 7 m6A sites located on the PLCG2 transcripts on the ConsRM online platform. One of them are highly conserved, among the top 8% most conserved m6A sites (Figure 10A). In addition, we can also see that the Gene Region where the most conserved m6A site of PLCG2 is located is 3′UTR, which is consistent with our experimental results. Its post-transcriptional regulation involves one RNA binding protein site and two miRNA Targets (Figure 10B).
FIGURE 10. Results of ConsRM online platform. (A) Search for “PLCG2” returns 7 m6A sites. (B) The detailed conservation metrics and other regulatory information of the most conserved m6A site on PLCG2.
Recent technological advances in high-throughput sequencing in combination with antibody enrichment of modifications have accelerated the detection of distribution and abundance for m6A methylation at the transcriptome-wide level (Meyer et al., 2012; Strong et al., 2015). The discovery of m6A demethylases indicates that m6A methylation of mRNA is a reversible and dynamic process with regulatory functions (Jia et al., 2013; Nilsen, 2014).
In recent years, more and more studies have been conducted on the components of m6A writers, erasers, and readers in cancer. Ma et al. (2017) revealed an important role of METTL14 in tumor metastasis and provided a fresh view of m6A modification in tumor progression. Zhang J. et al. (2019) indicated a novel mechanism by which ALKBH5 promotes GC invasion and metastasis by demethylating the lncRNA NEAT1. Tang et al. (2019) found that FTO is essential for the proliferation of pancreatic cancer cells. In our study, we found that the expression level of YTHDF3 was up-regulated (FC > 2, p < 0.05, Table 8), but there was no previous study on the expression level of YTHDF3 in adenocarcinoma of the esophagogastric junction. But limited by the size of our research sample, more research is needed to further explore. But in HCC, Zhou et al. (2019) found that the expression level of YTHDF3 was upregulated.
In this study, we illustrated global m6A modification patterns in AEG samples vs. tumor-adjacent normal tissues, analyzing gene expression and cancer-related pathways modulated by abnormal m6A RNA modifications. From previous studies, we know that m6A modified nucleotides are widely distributed in animal tissues, including the heart, liver, kidney, brain, and lung (Dominissini et al., 2012; Meyer et al., 2012). We figured out that the m6A modification pattern in AEG samples was distinct from that of normal controls, with a higher total m6A level and 1,721 more m6A peaks identified in the Ca group. By analyzing the differently methylated transcripts, cancer-related biological processes and pathways were significantly enriched, indicating the relationship between abnormal m6A modifications and AEG pathogenesis. Such global change of m6A modification profiles could result from the abnormal expression of key m6A enzymes. Nevertheless, only ∼12% of all peaks were detected within both adenocarcinomas of esophagogastric junction tissues and normal tissues. So there are differences between the two kinds of tissues. By analyzing the distribution of m6A-modified peaks per gene, we found the majority of genes had one to three m6A modified sites, while a relatively small number of genes contain more. Similarly, Chen et al. (2020) found that the majority of genes (6268/8526) had one to three m6A modified sites. Using MeRIP-Seq technology, we discovered that the m6A peak is abundant near the CDS and the stop codon, followed by the start codon and the 3′ UTR. However dominant m6A enrichment near stop codons and 3′UTRs is shown in most of mammal mRNA mammal as previously reported (Dominissini et al., 2012), and this m6A distributing type may represent the typical m6A topological pattern in most of the mature mRNA (Meyer et al., 2012; Batista et al., 2014). The extensively higher m6A signals at the stop codon or 3′UTRs may be responsible for RNA stability, signaling for transport, and translocation (Niu et al., 2013; Wang X. et al., 2014). As recently reported, m6A sites in plants are enriched around stop codons within 3′UTRs, start codons, and 5′UTRs (Li et al., 2014; Luo et al., 2014; Wang X. et al., 2014). In order to better understand the distribution of m6A peaks in the whole mRNA, we divided each m6A modified mRNA into three regions: 5′UTR, CDs and 3′UTR, and calculated the distribution proportion of these three regions. We can conclude that the distribution of m6A peaks in the CDS region is increased and there is a summit in the m6A peak near the stop codon of CDS. But in plants, Csepany et al. (1990) found that there was another minor summit of m6A peaks at positions near the start codon of CDS both in callus and leaf.
The consensus motif sequence RRACH has previously been shown to be over represented in m6A motif regions (Wei and Moss, 1977; Harper et al., 1990) and also further been identified in some high throughput m6A RNA sequencing databases (Luo et al., 2014; Wan et al., 2015). Therefore, in our current study, we successfully identified common motifs in the AEG transcriptome. Interestingly, Csepany et al. (1990) failed to find the common RRACH sequence in the m6A motif region of rice, but another different motif sequence was enriched by MEME and HOMER.
We additionally analyzed the relationship between methylation genes and their expression levels. Combined analysis of our m6A-seq and mRNA-seq data revealed 55 genes in the Ca group, which have differently methylated m6A sites along with significant changes of mRNA abundance compared with the NC group (fold change > 2, p < 0.05). It indicated that m6A modifications tend to have a positive correlation of mRNAs expression in AEG. Similar to our results, Luo et al. (2019) found that compared to hypomethylated genes, the expression of hypermethylated mRNAs tended to be upregulated in the HFD group. But some studies have come to different conclusions (Niu et al., 2013; Schwartz et al., 2014). In addition, we found that the overall expression level of methylated genes near the start codon was higher. Luo et al. (2019) also stated that genes in the PeakStart category possess higher overall expression levels. However, in the study of Luo, the enrichment of m6A around the start codon is obvious. Different from the results of Luo, there is no obvious enrichment of m6A around the start codon in our study. Recently, it has been found that the main function of m6A is to mediate the degradation of mRNA in mammalian cells (Batista et al., 2014; Wang Y. et al., 2014; Liu et al., 2014).
In combination with various databases and preliminary screening results, we selected three typical mRNAs for further verification. For example, PLCG2 could promote proliferation through inactivating ERK and NF-κB pathway (Ma et al., 2019), p38 MAPK and JNK MAPK pathways (Chen et al., 2018a), PKCδ-mediated JNK1/2 signaling pathway (Chen et al., 2018b). The TPR contributes to the organization of the nuclear lamina and in cooperation with lamins guards the interphase assembly of nuclear pore complexes (Fišerová et al., 2019). Consistent with the results of the initial screening, TPR was hyper-methylated; DVL1, PLCG2 were hypomethylated. As a hypomethylated gene, the expression of PLCG2 was downregulated. It is further possible to conclude that hypomethylated genes are more prone to low expression. This is similar to the results that m6A modifications tend to have a positive correlation of mRNAs expression in AEG. The relationship between gene methylation and gene expression requires further investigation.
In our study, the human AEG transcriptome modification profile was proposed for the first time and differentially expressed mRNA transcripts were identified through hypermethylation and hypomethylation m6A modification. This may help to further study the mechanism of m6A-mediated gene expression regulation. It is possible to develop new AEG therapeutic strategies by regulating m6A methylation transcripts or m6A-related genes. However, this study still has some limitations: 1) the m6A-Atlas (Tang et al., 2021) database is currently the human genome database containing the most known modification sites. Our research only selected three typical mRNAs for further verification. In the future, we will further select more novel methylated genes in AEG to compare with methylation data in m6A-Atlas, and gradually improve related research. 2) The RMVar (Luo et al., 2021) and RMDisease (Chen et al., 2021) show the potential association between the mutations with m6A. In future work, we will further explore the role of m6A-related mutations in adenocarcinoma of the esophagogastric junction, look for related mechanisms, and find therapeutic targets Point, provide a theoretical basis for the precision treatment of AEG.
This study preliminarily constructed the first m6A full transcriptome map of human AEG. This has a guiding role in revealing the mechanism of m6A-mediated gene expression regulation.
The datasets presented in this study can be found in online repositories. The names of the repository/repositories and accession number(s) can be found below: https://www.ncbi.nlm.nih.gov/geo/GSE189874.
YF provided direction and guidance throughout the preparation of this manuscript. B-BH and J-BH wrote and edited the manuscript. RH, LH, and C-FM collected and prepared the related articles. CZ reviewed and made significant revisions to the manuscript. All authors read and approved the final manuscript.
Jiangsu Innovative Team Leading Talent Fund (CXTDC2016006, QNRC2016446), Jiangsu 333 Talent Fund (BRA2020016), Jiangsu Provincial Key Research and Development Special Fund (BE2015666), Jiangsu Six High Peak Talent Fund (WSW-205,WSW236), Zhenjiang Key Research and Development Fund (SH2021038), Suqian Science and Technology Support Project Fund (K201907).
The authors declare that the research was conducted in the absence of any commercial or financial relationships that could be construed as a potential conflict of interest.
All claims expressed in this article are solely those of the authors and do not necessarily represent those of their affiliated organizations, or those of the publisher, the editors, and the reviewers. Any product that may be evaluated in this article, or claim that may be made by its manufacturer, is not guaranteed or endorsed by the publisher.
AEG, adenocarcinoma of esophagogastric junction; ALKBH5, a-ketoglutarate-dependent dioxygenase alkB homolog 5; eIF3, eukaryotic initiation factor 3; FTO, obesity-associated protein; HNRNPA2B1, heterogenous nuclear ribonucleoprotein A2B1; IGF2BP1/2/3, insulin-like growth factor 2 mRNA binding protein 1/2/3; m6A, N6-methyladenosine; METTL3, methyltransferase-like3; METTL14, methyltransferase-like 14; METTL16, methyltransferase like 16; RBM15/15B, RNA binding motif protein 15/15B; WTAP, Wilms tumor suppressor-1-associated protein; YTHDC1, YTH domain-containing 1; YTHDC2, YTH domain-containing 2; YTHDF1, YTH N6-methyladenosine RNA binding protein 1; YTHDF2, YTH N6-methyladenosine RNA binding protein 2; YTHDF3, YTH N6-methyladenosine RNA binding protein 3; ZC3H13, Zinc finger CCCH-type containing 13.
Aoyama, T., Yamashita, S., and Tomita, K. (2020). Mechanistic Insights into m6A Modification of U6 snRNA by Human METTL16. Nucleic Acids Res. 48 (9), 5157–5168. doi:10.1093/nar/gkaa227
Bailey, T. L. (2011). DREME: Motif Discovery in Transcription Factor ChIP-Seq Data. Bioinformatics (Oxford, England) 27 (12), 1653–1659. doi:10.1093/bioinformatics/btr261
Batista, P. J., Molinie, B., Wang, J., Qu, K., Zhang, J., Li, L., et al. (2014). m6A RNA Modification Controls Cell Fate Transition in Mammalian Embryonic Stem Cells. Cell stem cell 15 (6), 707–719. doi:10.1016/j.stem.2014.09.019
Chen, K., Song, B., Tang, Y., Wei, Z., Xu, Q., Su, J., et al. (2021). RMDisease: a Database of Genetic Variants that Affect RNA Modifications, with Implications for Epitranscriptome Pathogenesis. Nucleic Acids Res. 49 (D1), D1396–D1404. doi:10.1093/nar/gkaa790
Chen, M., and Wong, C.-M. (2020). The Emerging Roles of N6-Methyladenosine (m6A) Deregulation in Liver Carcinogenesis. Mol. Cancer 19 (1), 44. doi:10.1186/s12943-020-01172-y
Chen, X., Lv, Q., Ma, J., and Liu, Y. (2018a). PLCγ2 Promotes Apoptosis while Inhibits Proliferation in Rat Hepatocytes through PKCD/JNK MAPK and PKCD/p38 MAPK Signalling. Cell Prolif 51 (3), e12437. doi:10.1111/cpr.12437
Chen, X., Zhu, X., Liu, Y., Lv, Q., and Ma, J. (2018b). Silencing of Phospholipase C Gamma 2 Promotes Proliferation of Rat Hepatocytes In Vitro. J. Cel. Biochem. 119 (5), 4085–4096. doi:10.1002/jcb.26592
Chen, Y., Zhou, C., Sun, Y., He, X., and Xue, D. (2020). m6A RNA Modification Modulates Gene Expression and Cancer-Related Pathways in clear Cell Renal Cell Carcinoma. Epigenomics 12 (2), 87–99. doi:10.2217/epi-2019-0182
Csepany, T., Lin, A., Baldick, C. J., and Beemon, K. (1990). Sequence Specificity of mRNA N6-Adenosine Methyltransferase. J. Biol. Chem. 265 (33), 20117–20122. doi:10.1016/s0021-9258(17)30477-5
Desrosiers, R., Friderici, K., and Rottman, F. (1974). Identification of Methylated Nucleosides in Messenger RNA from Novikoff Hepatoma Cells. Proc. Natl. Acad. Sci. 71 (10), 3971–3975. doi:10.1073/pnas.71.10.3971
Dominissini, D., Moshitch-Moshkovitz, S., Schwartz, S., Salmon-Divon, M., Ungar, L., Osenberg, S., et al. (2012). Topology of the Human and Mouse m6A RNA Methylomes Revealed by m6A-Seq. Nature 485 (7397), 201–206. doi:10.1038/nature11112
Fišerová, J., Maninová, M., Sieger, T., Uhlířová, J., Šebestová, L., Efenberková, M., et al. (2019). Nuclear Pore Protein TPR Associates with Lamin B1 and Affects Nuclear Lamina Organization and Nuclear Pore Distribution. Cell. Mol. Life Sci. 76 (11), 2199–2216. doi:10.1007/s00018-019-03037-0
Frye, M., Harada, B. T., Behm, M., and He, C. (2018). RNA Modifications Modulate Gene Expression during Development. Science 361 (6409), 1346–1349. doi:10.1126/science.aau1646
Geistlinger, L., Csaba, G., Santarelli, M., Ramos, M., Schiffer, L., Turaga, N., et al. (2021). Toward a Gold Standard for Benchmarking Gene Set Enrichment Analysis. Brief. Bioinformatics 22 (1), 545–556. doi:10.1093/bib/bbz158
Harper, J. E., Miceli, S. M., Roberts, R. J., and Manley, J. L. (1990). Sequence Specificity of the Human mRNA N6-Adenosine Methylasein Vitro. Nucl. Acids Res. 18 (19), 5735–5741. doi:10.1093/nar/18.19.5735
Hsu, P. J., Shi, H., and He, C. (2017). Epitranscriptomic Influences on Development and Disease. Genome Biol. 18 (1), 197. doi:10.1186/s13059-017-1336-6
Huang, H., Weng, H., Sun, W., Qin, X., Shi, H., Wu, H., et al. (2018). Recognition of RNA N6-Methyladenosine by IGF2BP Proteins Enhances mRNA Stability and Translation. Nat. Cel Biol 20 (3), 285–295. doi:10.1038/s41556-018-0045-z
Imamura, Y., Watanabe, M., Toihata, T., Takamatsu, M., Kawachi, H., Haraguchi, I., et al. (2019). Recent Incidence Trend of Surgically Resected Esophagogastric Junction Adenocarcinoma and Microsatellite Instability Status in Japanese Patients. Digestion 99 (1), 6–13. doi:10.1159/000494406
Jia, G., Fu, Y., and He, C. (2013). Reversible RNA Adenosine Methylation in Biological Regulation. Trends Genet. 29 (2), 108–115. doi:10.1016/j.tig.2012.11.003
Jia, G., Fu, Y., Zhao, X., Dai, Q., Zheng, G., Yang, Y., et al. (2011). N6-methyladenosine in Nuclear RNA Is a Major Substrate of the Obesity-Associated FTO. Nat. Chem. Biol. 7 (12), 885–887. doi:10.1038/nchembio.687
Kauppila, J. H., and Lagergren, J. (2016). The Surgical Management of Esophago-Gastric Junctional Cancer. Surg. Oncol. 25 (4), 394–400. doi:10.1016/j.suronc.2016.09.004
Kechin, A., Boyarskikh, U., Kel, A., and Filipenko, M. (2017). cutPrimers: A New Tool for Accurate Cutting of Primers from Reads of Targeted Next Generation Sequencing. J. Comput. Biol. 24 (11), 1138–1143. doi:10.1089/cmb.2017.0096
Keeney, S., and Bauer, T. L. (2006). Epidemiology of Adenocarcinoma of the Esophagogastric junction. Surg. Oncol. Clin. North America 15 (4), 687–696. doi:10.1016/j.soc.2006.07.014
Kim, D., Langmead, B., and Salzberg, S. L. (2015). HISAT: a Fast Spliced Aligner with Low Memory Requirements. Nat. Methods 12 (4), 357–360. doi:10.1038/nmeth.3317
Li, J., Liang, L., Yang, Y., Li, X., and Ma, Y. (2021a). N6-methyladenosine as a Biological and Clinical Determinant in Colorectal Cancer: Progression and Future Direction. Theranostics 11 (6), 2581–2593. doi:10.7150/thno.52366
Li, K., Chen, J., Lou, X., Li, Y., Qian, B., Xu, D., et al. (2021b). HNRNPA2B1 Affects the Prognosis of Esophageal Cancer by Regulating the miR-17-92 Cluster. Front. Cel Dev. Biol. 9, 658642. doi:10.3389/fcell.2021.658642
Li, Y., Wang, X., Li, C., Hu, S., Yu, J., and Song, S. (2014). Transcriptome-wide N6-Methyladenosine Profiling of rice Callus and Leaf Reveals the Presence of Tissue-specific Competitors Involved in Selective mRNA Modification. RNA Biol. 11 (9), 1180–1188. doi:10.4161/rna.36281
Lin, S., Liu, Q., Lelyveld, V. S., Choe, J., Szostak, J. W., and Gregory, R. I. (2018). Mettl1/Wdr4-Mediated m7G tRNA Methylome Is Required for Normal mRNA Translation and Embryonic Stem Cell Self-Renewal and Differentiation. Mol. Cel. 71 (2), 244–255. doi:10.1016/j.molcel.2018.06.001
Liu, J., Yue, Y., Han, D., Wang, X., Fu, Y., Zhang, L., et al. (2014). A METTL3-METTL14 Complex Mediates Mammalian Nuclear RNA N6-Adenosine Methylation. Nat. Chem. Biol. 10 (2), 93–95. doi:10.1038/nchembio.1432
Liu, S., Zhuo, L., Wang, J., Zhang, Q., Li, Q., Li, G., et al. (2020a). METTL3 Plays Multiple Functions in Biological Processes. Am. J. Cancer Res. 10 (6), 1631–1646.
Liu, T., Wei, Q., Jin, J., Luo, Q., Liu, Y., Yang, Y., et al. (2020b). The m6A Reader YTHDF1 Promotes Ovarian Cancer Progression via Augmenting EIF3C Translation. Nucleic Acids Res. 48 (7), 3816–3831. doi:10.1093/nar/gkaa048
Liu, Z.-X., Li, L.-M., Sun, H.-L., and Liu, S.-M. (2018). Link between m6A Modification and Cancers. Front. Bioeng. Biotechnol. 6, 89. doi:10.3389/fbioe.2018.00089
Luo, G.-Z., Macqueen, A., Zheng, G., Duan, H., Dore, L. C., Lu, Z., et al. (2014). Unique Features of the m6A Methylome in Arabidopsis thaliana. Nat. Commun. 5, 5630. doi:10.1038/ncomms6630
Luo, X., Li, H., Liang, J., Zhao, Q., Xie, Y., Ren, J., et al. (2021). RMVar: an Updated Database of Functional Variants Involved in RNA Modifications. Nucleic Acids Res. 49 (D1), D1405–D1412. doi:10.1093/nar/gkaa811
Luo, Z., Zhang, Z., Tai, L., Zhang, L., Sun, Z., and Zhou, L. (2019). Comprehensive Analysis of Differences of N6-Methyladenosine RNA Methylomes between High-Fat-Fed and normal Mouse Livers. Epigenomics 11 (11), 1267–1282. doi:10.2217/epi-2019-0009
Ma, D., Lian, F., and Wang, X. (2019). PLCG2 Promotes Hepatocyte Proliferation In Vitro via NF-Κb and ERK Pathway by Targeting Bcl2, Myc and Ccnd1. Artif. Cell nanomedicine, Biotechnol. 47 (1), 3786–3792. doi:10.1080/21691401.2019.1669616
Ma, J. z., Yang, F., Zhou, C. c., Liu, F., Yuan, J. h., Wang, F., et al. (2017). METTL14 Suppresses the Metastatic Potential of Hepatocellular Carcinoma by Modulating N 6 ‐methyladenosine‐dependent Primary MicroRNA Processing. Hepatology 65 (2), 529–543. doi:10.1002/hep.28885
Macha, M., Seshacharyulu, P., Krishn, S., Pai, P., Rachagani, S., Jain, M., et al. (2014). MicroRNAs (miRNAs) as Biomarker(s) for Prognosis and Diagnosis of Gastrointestinal (GI) Cancers. Curr. Pharm. Des. 20 (33), 5287–5297. doi:10.2174/1381612820666140128213117
Meyer, K. D., Saletore, Y., Zumbo, P., Elemento, O., Mason, C. E., and Jaffrey, S. R. (2012). Comprehensive Analysis of mRNA Methylation Reveals Enrichment in 3′ UTRs and Near Stop Codons. Cell 149 (7), 1635–1646. doi:10.1016/j.cell.2012.05.003
Miao, Z., Zhang, T., Xie, B., Qi, Y., and Ma, C. (2021). Evolutionary Implications of the RNA N6-Methyladenosine Methylome in Plants. Mol. Biol. Evol. 2021, msab299. doi:10.1093/molbev/msab299
Ng, R. K., and Gurdon, J. B. (2008). Epigenetic Inheritance of Cell Differentiation Status. Cell Cycle 7 (9), 1173–1177. doi:10.4161/cc.7.9.5791
Nilsen, T. W. (2014). Internal mRNA Methylation Finally Finds Functions. Science 343 (6176), 1207–1208. doi:10.1126/science.1249340
Niu, Y., Zhao, X., Wu, Y.-S., Li, M.-M., Wang, X.-J., and Yang, Y.-G. (2013). N6-methyl-adenosine (m6A) in RNA: an Old Modification with a Novel Epigenetic Function. Genomics, Proteomics and Bioinformatics 11 (1), 8–17. doi:10.1016/j.gpb.2012.12.002
Ping, X.-L., Sun, B.-F., Wang, L., Xiao, W., Yang, X., Wang, W.-J., et al. (2014). Mammalian WTAP Is a Regulatory Subunit of the RNA N6-Methyladenosine Methyltransferase. Cell Res 24 (2), 177–189. doi:10.1038/cr.2014.3
Qin, S., Mao, Y., Wang, H., Duan, Y., and Zhao, L. (2021). The Interplay between m6A Modification and Non-coding RNA in Cancer Stemness Modulation: Mechanisms, Signaling Pathways, and Clinical Implications. Int. J. Biol. Sci. 17 (11), 2718–2736. doi:10.7150/ijbs.60641
Roundtree, I. A., Evans, M. E., Pan, T., and He, C. (2017). Dynamic RNA Modifications in Gene Expression Regulation. Cell 169 (7), 1187–1200. doi:10.1016/j.cell.2017.05.045
Schöller, E., Weichmann, F., Treiber, T., Ringle, S., Treiber, N., Flatley, A., et al. (2018). Interactions, Localization, and Phosphorylation of the m6A Generating METTL3-METTL14-WTAP Complex. Rna 24 (4), 499–512. doi:10.1261/rna.064063.117
Schwartz, S., Mumbach, M. R., Jovanovic, M., Wang, T., Maciag, K., Bushkin, G. G., et al. (2014). Perturbation of m6A Writers Reveals Two Distinct Classes of mRNA Methylation at Internal and 5′ Sites. Cel Rep. 8 (1), 284–296. doi:10.1016/j.celrep.2014.05.048
Shen, L., Shao, N.-Y., Liu, X., Maze, I., Feng, J., and Nestler, E. J. (2013). diffReps: Detecting Differential Chromatin Modification Sites from ChIP-Seq Data with Biological Replicates. PloS one 8 (6), e65598. doi:10.1371/journal.pone.0065598
Shi, H., Wei, J., and He, C. (2019). Where, when, and How: Context-dependent Functions of RNA Methylation Writers, Readers, and Erasers. Mol. Cel. 74 (4), 640–650. doi:10.1016/j.molcel.2019.04.025
Song, B., Chen, K., Tang, Y., Wei, Z., Su, J., de Magalhães, J. P., et al. (2021). ConsRM: Collection and Large-Scale Prediction of the Evolutionarily Conserved RNA Methylation Sites, with Implications for the Functional Epitranscriptome. Brief. Bioinformatics 22 (6). doi:10.1093/bib/bbab088
Steevens, J., Botterweck, A. A. M., Dirx, M. J. M., Van Den Brandt, P. A., and Schouten, L. J. (2009). Trends in Incidence of Oesophageal and Stomach Cancer Subtypes in Europe. Eur. J. Gastroenterol. Hepatol. 22 (6), 1–678. doi:10.1097/MEG.0b013e32832ca091
Strong, V. E., Wu, A.-w., Selby, L. V., Gonen, M., Hsu, M., Song, K. Y., et al. (2015). Differences in Gastric Cancer Survival between the U.S. And China. J. Surg. Oncol. 112 (1), 31–37. doi:10.1002/jso.23940
Tang, C., Klukovich, R., Peng, H., Wang, Z., Yu, T., Zhang, Y., et al. (2018). ALKBH5-dependent m6A Demethylation Controls Splicing and Stability of Long 3′-UTR mRNAs in Male Germ Cells. Proc. Natl. Acad. Sci. USA 115 (2), E325–E333. doi:10.1073/pnas.1717794115
Tang, X., Liu, S., Chen, D., Zhao, Z., and Zhou, J. (2019). The Role of the Fat Mass and Obesity-associated P-rotein in the P-roliferation of P-ancreatic C-ancer C-ells. Oncol. Lett. 17 (2), 2473–2478. doi:10.3892/ol.2018.9873
Tang, Y., Chen, K., Song, B., Ma, J., Wu, X., Xu, Q., et al. (2021). m6A-Atlas: a Comprehensive Knowledgebase for Unraveling the N6-Methyladenosine (m6A) Epitranscriptome. Nucleic Acids Res. 49 (D1), D134–D143. doi:10.1093/nar/gkaa692
Tian, L., Greenberg, S. A., Kong, S. W., Altschuler, J., Kohane, I. S., and Park, P. J. (2005). Discovering Statistically Significant Pathways in Expression Profiling Studies. Proc. Natl. Acad. Sci. 102 (38), 13544–13549. doi:10.1073/pnas.0506577102
Wan, Y., Tang, K., Zhang, D., Xie, S., Zhu, X., Wang, Z., et al. (2015). Transcriptome-wide High-Throughput Deep m6A-Seq Reveals Unique Differential m6A Methylation Patterns between Three Organs in Arabidopsis thaliana. Genome Biol. 16, 272. doi:10.1186/s13059-015-0839-2
Wang, H.-F., Kuang, M.-j., Han, S.-j., Wang, A.-b., Qiu, J., Wang, F., et al. (2020a). BMP2 Modified by the m6A Demethylation Enzyme ALKBH5 in the Ossification of the Ligamentum Flavum through the AKT Signaling Pathway. Calcif Tissue Int. 106 (5), 486–493. doi:10.1007/s00223-019-00654-6
Wang, T., Kong, S., Tao, M., and Ju, S. (2020b). The Potential Role of RNA N6-Methyladenosine in Cancer Progression. Mol. Cancer 19 (1), 88. doi:10.1186/s12943-020-01204-7
Wang, X., Feng, J., Xue, Y., Guan, Z., Zhang, D., Liu, Z., et al. (2016). Structural Basis of N6-Adenosine Methylation by the METTL3-METTL14 Complex. Nature 534 (7608), 575–578. doi:10.1038/nature18298
Wang, X., Lu, Z., Gomez, A., Hon, G. C., Yue, Y., Han, D., et al. (2014a). N6-methyladenosine-dependent Regulation of Messenger RNA Stability. Nature 505 (7481), 117–120. doi:10.1038/nature12730
Wang, Y., Li, Y., Toth, J. I., Petroski, M. D., Zhang, Z., and Zhao, J. C. (2014b). N6-methyladenosine Modification Destabilizes Developmental Regulators in Embryonic Stem Cells. Nat. Cel Biol 16 (2), 191–198. doi:10.1038/ncb2902
Wang, Y., Zheng, Y., Guo, D., Zhang, X., Guo, S., Hui, T., et al. (2019). m6A Methylation Analysis of Differentially Expressed Genes in Skin Tissues of Coarse and Fine Type Liaoning Cashmere Goats. Front. Genet. 10, 1318. doi:10.3389/fgene.2019.01318
Wei, C.-M., and Moss, B. (1977). Nucleotide Sequences at the N6-Methyladenosine Sites of HeLa Cell Messenger Ribonucleic Acid. Biochemistry 16 (8), 1672–1676. doi:10.1021/bi00627a023
Wen, J., Lv, R., Ma, H., Shen, H., He, C., Wang, J., et al. (2018). Zc3h13 Regulates Nuclear RNA m6A Methylation and Mouse Embryonic Stem Cell Self-Renewal. Mol. Cel. 69 (6), 1028–1038. doi:10.1016/j.molcel.2018.02.015
Wu, R., Li, A., Sun, B., Sun, J.-G., Zhang, J., Zhang, T., et al. (2019). A Novel m6A Reader Prrc2a Controls Oligodendroglial Specification and Myelination. Cel Res 29 (1), 23–41. doi:10.1038/s41422-018-0113-8
Yamashita, H., Seto, Y., Seto, Y., Sano, T., Makuuchi, H., Ando, N., et al. (2017). Results of a Nation-wide Retrospective Study of Lymphadenectomy for Esophagogastric junction Carcinoma. Gastric Cancer 20 (Suppl. 1), 69–83. doi:10.1007/s10120-016-0663-8
Zhang, J., Guo, S., Piao, H.-y., Wang, Y., Wu, Y., Meng, X.-y., et al. (2019a). ALKBH5 Promotes Invasion and Metastasis of Gastric Cancer by Decreasing Methylation of the lncRNA NEAT1. J. Physiol. Biochem. 75 (3), 379–389. doi:10.1007/s13105-019-00690-8
Zhang, S., Orita, H., and Fukunaga, T. (2019b). Current Surgical Treatment of Esophagogastric junction Adenocarcinoma. World J. Gastrointest Oncol. 11 (8), 567–578. doi:10.4251/wjgo.v11.i8.567
Zhang, W., He, L., Liu, Z., Ren, X., Qi, L., Wan, L., et al. (2020). Multifaceted Functions and Novel Insight into the Regulatory Role of RNA N6-Methyladenosine Modification in Musculoskeletal Disorders. Front. Cel Dev. Biol. 8, 870. doi:10.3389/fcell.2020.00870
Zhang, X., Lu, N., Wang, L., Wang, Y., Li, M., Zhou, Y., et al. (2021). Recent Advances of m6A Methylation Modification in Esophageal Squamous Cell Carcinoma. Cancer Cel Int 21 (1), 421. doi:10.1186/s12935-021-02132-2
Zhang, Y., Liu, T., Meyer, C. A., Eeckhoute, J., Johnson, D. S., Bernstein, B. E., et al. (2008). Model-based Analysis of ChIP-Seq (MACS). Genome Biol. 9 (9), R137. doi:10.1186/gb-2008-9-9-r137
Zhang, Y., Wang, X., Zhang, X., Wang, J., Ma, Y., Zhang, L., et al. (2019c). RNA-binding Protein YTHDF3 Suppresses Interferon-dependent Antiviral Responses by Promoting FOXO3 Translation. Proc. Natl. Acad. Sci. USA 116 (3), 976–981. doi:10.1073/pnas.1812536116
Zhao, B. S., Roundtree, I. A., and He, C. (2017). Post-transcriptional Gene Regulation by mRNA Modifications. Nat. Rev. Mol. Cel Biol 18 (1), 31–42. doi:10.1038/nrm.2016.132
Zhao, X., Yang, Y., Sun, B.-F., Zhao, Y.-L., and Yang, Y.-G. (2014). FTO and Obesity: Mechanisms of Association. Curr. Diab Rep. 14 (5), 486. doi:10.1007/s11892-014-0486-0
Zheng, G., Dahl, J. A., Niu, Y., Fedorcsak, P., Huang, C.-M., Li, C. J., et al. (2013). ALKBH5 Is a Mammalian RNA Demethylase that Impacts RNA Metabolism and Mouse Fertility. Mol. Cel. 49 (1), 18–29. doi:10.1016/j.molcel.2012.10.015
Zheng, W., Dong, X., Zhao, Y., Wang, S., Jiang, H., Zhang, M., et al. (2019). Multiple Functions and Mechanisms Underlying the Role of METTL3 in Human Cancers. Front. Oncol. 9, 1403. doi:10.3389/fonc.2019.01403
Keywords: epigenomics, adenocarcinoma of esophagogastric junction, m6A methylation, diagnosis, treatment
Citation: Huang J-B, Hu B-B, He R, He L, Zou C, Man C-F and Fan Y (2022) Analysis of N6-Methyladenosine Methylome in Adenocarcinoma of Esophagogastric Junction. Front. Genet. 12:787800. doi: 10.3389/fgene.2021.787800
Received: 08 November 2021; Accepted: 30 December 2021;
Published: 24 January 2022.
Edited by:
A. Rasim Barutcu, University of Toronto, CanadaReviewed by:
Kunqi Chen, Fujian Medical University, ChinaCopyright © 2022 Huang, Hu, He, He, Zou, Man and Fan. This is an open-access article distributed under the terms of the Creative Commons Attribution License (CC BY). The use, distribution or reproduction in other forums is permitted, provided the original author(s) and the copyright owner(s) are credited and that the original publication in this journal is cited, in accordance with accepted academic practice. No use, distribution or reproduction is permitted which does not comply with these terms.
*Correspondence: Yu Fan, eXVmMzZAc2luYS5jb20=
†These authors have contributed equally to this work and share first authorship
Disclaimer: All claims expressed in this article are solely those of the authors and do not necessarily represent those of their affiliated organizations, or those of the publisher, the editors and the reviewers. Any product that may be evaluated in this article or claim that may be made by its manufacturer is not guaranteed or endorsed by the publisher.
Research integrity at Frontiers
Learn more about the work of our research integrity team to safeguard the quality of each article we publish.