- 1University of Applied Sciences Upper Austria, Hagenberg im Mühlkreis, Austria
- 2Technische Hochschule Ingolstadt, Ingolstadt, Germany
- 3Johannes Kepler University Linz, Linz, Austria
As vehicle automation advances, drivers of automated vehicles become more disengaged from the primary driving task. Windshield displays provide a large screen space supporting drivers in non-driving related activities. This article presents user preferences as well as task and safety issues for 3D augmented reality windshield displays in automated driving. Participants of a user study (n = 24) customized two modes of content presentation (multiple content-specific windows vs. one main window), and could freely adjust visual parameters for these content windows using a simulated “ideal” windshield display in a virtual reality driving simulator. We found that user preferences differ with respect to contextual aspects. Additionally, using one main content window resulted in better task performance and lower take-over times, but the subjective user experience was higher for the multi-window user interface. These findings help automotive interface designers to improve experiences in automated vehicles.
1 Introduction
Today, drivers perform more and more activities while driving, such as the primary driving task as well as engaging with the vehicle’s infotainment system (e.g., operating the music player, accessing navigation data, checking vehicle information) (Kern and Schmidt, 2009). Automated driving (AD) further pushes drivers’ demand for non-driving related tasks (NDRTs) (Pfleging et al., 2016). In conditionally/highly (SAE level 3 and 4) and fully automated driving (SAE level 5) (SAE On-Road Automated Vehicle Standards Committee, 2021), the primary driving function is conducted by the automated vehicle (AV), and this generates new opportunities to utilize AVs as mobile entertainment carriers and offices (e.g., Riener et al., 2016; Schartmüller et al., 2018).
Many non-driving related activities require input from the driver, or give feedback to the driver, such as visually (e.g., Damböck et al., 2012), auditory (e.g., Horswill and Plooy, 2008), and olfactory (e.g., Dmitrenko et al., 2019), or a combination (i.e., multimodal interaction/feedback, e.g., Pfleging et al., 2012). Currently, a wide range of displays in the vehicle offers visual output, including dashboard, tablet, and head-up displays (Haeuslschmid et al., 2016a). Head-up displays (HUDs) visualize information in the drivers’ field of view towards the outside road environment, and thereby enable the driver to quickly check information while observing the road (Haeuslschmid et al., 2016a; Riegler et al., 2019c). Beyond that, windshield displays (WSDs) extend HUDs to the entire windscreen, providing an even larger viewing and interaction area, thereby making this display type suitable for visualizing world-fixed navigation information (Fu et al., 2013), presenting nearby points of interest (POIs) (Häuslschmid et al., 2015), and enabling in-vehicle work, entertainment, and social interaction activities (Riegler et al., 2018), among others. Another potential of WSDs is to improve user trust and acceptance in AVs by increasing their system transparency (Wintersberger et al., 2017b). AR also helps to better anticipate lane change maneuvers, which has the potential to increase drivers’ situational awareness (Langlois and Soualmi, 2016). With regard to elderly drivers as well as visually or cognitively impaired drivers, the use AR HUD interfaces resulted in improved reaction times of older drivers as well as decreased collision occurrences, as compared to a head-down display (HDD), or dashboard interface (Charissis et al., 2011). Hwang et al. (2016), Calvi et al. (2020) show in experimental studies that an in-vehicle AR HUD might positively contribute to both the visual safety driving behavior, and their psychological driving safety, such as tension and stress, of elderly drivers. As more drivers increasingly demand adaptive and personalized interfaces and devices (Meixner et al., 2017; Riegler et al., 2019a), WSDs provide a novel opportunity for the car industry to minimize visual clutter in the instrument cluster and center stack, and show digital information on the WSD, tailored to the individual needs of drivers and passengers. Therefore, WSDs can be utilized to establish a single user interface (UI) for all in-vehicle infotainment systems (Gabbard et al., 2014), and even for outside interactive activities (e.g., Colley et al., 2018). Especially in automated driving, WSDs can be supportive in informing the driver to transfer control of the vehicle in case of so-called take-over requests (TORs) (Wintersberger et al., 2018). As more attention is paid to personalization of smart devices such as smartphones (e.g., customizable content through apps, layouts, and themes), in-vehicle interfaces will become more tailored to the automotive users in terms of adaptation and personalization (Häuslschmid et al., 2019). In addition, technological advances in augmented reality (AR) may lead to new opportunities for presenting information on a WSD by providing world-fixed visualizations (i.e., the content appears to be anchored in the real world) (Gabbard et al., 2014). Accordingly, “floating” 2D or 3D AR objects are a novel way to realize such an adaptive interface. Consequently, designers of AR WSD interfaces should take this trend into account by allowing drivers to customize how content is displayed on the WSD. Although the potential of WSDs for AVs has been highlighted, limited research has been conducted to evaluate user preferences related to personalization of content and layout in AR WSDs, as well as their impact on task and take-over performance. Adaptive automotive UIs are challenging, as drivers of SAE level 3 (L3) and level 4 (L4) conditionally AVs may choose to place content in a way that emphasizes entertainment functions, for example, however, such a layout may be considered unsafe for handing over control to the driver in case of emergencies.
Consequently, we explore and evaluate AR WSDs in respect to user preferences including content type and layout, as well as their effect on task and take-over performance for SAE L3 automated vehicles. For this purpose, we have drivers create their own personalized WSD layout in a virtual reality (VR) driving simulator, and compare the benefits and drawbacks of having multiple content-specific windows with one single main window for content presentation. Based on analysis of user preferences, task and take-over performances, we discuss the potential impact of personalized AR-supported windshield display user interfaces.
2 Related Work
For manual and assisted driving (up to SAE L2), the potential of AR applications has already been shown in prototypical user studies (e.g., Medenica et al., 2011; Rane et al., 2016). Traditional approaches utilizing HUDs often focus on improving safety. For example, Smith et al. (2015) could demonstrate that presenting information directly in a driver’s field of view can lead to better driving performance and less distraction. An additional application for AR HUDs is navigation, where digital arrows are superimposed on the outside environment to assist navigation (e.g., Kim and Dey, 2009; Bark et al., 2014). Recent research has focused on NDRTs and passenger experiences, in which drivers/passengers perform office tasks or engage in entertainment activities. However, in conditionally automated vehicles, such tasks are conducted with the need to keep the driver in the loop (i.e., without the driver losing control of the vehicle, even in AD mode) (Dillmann et al., 2021), as they must be able to assume control of the vehicle in the event of an emergency (Frison et al., 2019). Therefore, it can be very convenient for the driver to gaze in the direction of the traffic while using the windshield as a display (Smith et al., 2016). Additional research on engaging in work-related tasks in the vehicle shows that participants prefer a WSD to a head-down display (such as traditional dashboard or center stack displays) (Schartmüller et al., 2018). AR content can be visualized in a way that keeps the driver’s visual focus on the road by reducing or even eliminating glances between the primary driving task and other activities (Schroeter and Steinberger, 2016). As a result, the implementation of TORs, i.e., notifying the driver to accept control of the vehicle, could take advantage from the driver’s forward gaze direction and the WSD’s large screen space. Applications that use WSDs are expected to improve in-vehicle experience for drivers and passengers, and facilitate the evolution of vehicles in the transition from manual to automated driving (Kun et al., 2017). The systematic review of AR applications for automated vehicles by Riegler et al. (2021a) shows increasing research efforts for designing driver and passenger experiences. In this research, we focus on automated driving (SAE L3 and higher), because AR features are expected to accompany driving automation (Wiegand et al., 2019; Riegler et al., 2020b), and can help to build trust in automated driving (AD) (Wintersberger et al., 2017b). For now, however, the realization of AR WSDs is impracticable both for technical and financial reasons (Gabbard et al., 2014). Therefore, the suitability and acceptance of AR WSDs and future use cases can be demonstrated and evaluated using software simulations, in many cases employing virtual reality (VR) technology (e.g., Riegler et al., 2020a; Gerber et al., 2020). Advances in optics research demonstrate the feasibility of visualizing stereoscopic 3D images with an eyetracking-based light-field display and actual head-up display optics, and the resulting 3D AR HUD system has the potential to form the mainstream technology for a wide range of automotive AR applications (Lee et al., 2020).
2.1 Augmented Reality Windshield Display Personalization
Exploratory research on personalization of large head-up as well as windshield display content has been conducted. Haeuslschmid et al. (2016b) investigated a generalizable view management concept for manual vehicles (SAE L0–2) that takes into account drivers’ tasks, context, resources, and abilities for efficient information retrieval. The results show content-specific areas, for example safety-critical, and personal areas, on the WSD preferred by the study participants. Additionally, Häuslschmid et al. (2019) explored user preferences and safety aspects for 3D WSDs in manual driving. They found that while personalization is desired by users, their customized layouts do not always enable safe driving, compared to a one-fits-all layout. Therefore, Häuslschmid et al. suggest using a one-fits-all layout because the information uptake was found to be higher for these layouts, but with the disadvantage that individual user preferences are not sufficiently considered. In the context of automated driving, Riegler et al. (2018), Riegler et al. (2019e) investigated windshield display content, window sizes, and window transparency for SAE L3 and L5 AVs. Their experiment aimed at investigating driver-passengers’ requirements and preferences for WSDs based on different content types, such as warnings, vehicle-related information, work-, entertainment-, and social media content, among others. The study was conducted on a simulated 2D WSD, and the content windows were drawn and attributed in a fixed distance from the driver. Results show that drivers are aware of the implications of conditionally and fully automated driving, as content windows were utilized in more peripheral areas of the WSD in SAE L3 driving, while the entire WSD was applied for SAE L5 driving. However, as their study was focussed on initial exploration of user preferences for WSDs, no task or take-over performance was assessed. Further findings by Riegler et al. reveal that world-fixed AR visualizations could result in better task and take-over performance, compared to screen-fixed content presentations. However, user experience results showed no clear preference (Riegler et al., 2020c). Therefore, variable, continuous-depth, distance in content presentation on the WSD should be evaluated further, from a personalization perspective.
Our state-of-the art research suggests that there are two directions for presenting content on a 3D AR windshield display: 1) a single main window, containing all content in sub-menus, thereby utilizing only a single section of the WSD, similar to today’s in-vehicle infotainment systems (IVIS) displayed on a tablet in the center console of the vehicle, and 2) multiple content-specific windows, utilizing the entire windscreen design space for individual content areas. In this paper, we explore this research gap pertaining to the personalization of windshield display content in automated driving for SAE L3 AVs, and further assess the user-centered WSD design with task and take-over performance measures. The goal is therefore to investigate personalization parameters for 3D AR WSDs and to make qualitative and quantitative statements regarding the layout, i.e., a one-fits-all main window vs. multiple content-specific floating windows, as well as resulting recommendations for the design of content for such large displays in an automotive context.
3 Research Questions
Since windshield displays enable the driver/passengers to engage in NDRTs with a large AR interface in conditionally and fully automated vehicles, we determine visual properties and their implications on user experience, workload, as well as task and take-over performance for 3D WSDs with the following research questions:
• RQ1: Which, and how many areas on 3D WSDs do drivers of conditionally automated vehicles prefer for displaying information? We hypothesize that drivers would, if provided, personalize content presentation on the 3D WSD.
• RQ2: Do drivers have a clear preference regarding window presentation (multiple content-specific windows vs. one main window)? We hypothesize that drivers have a clear preference regarding the window(s) placement on the 3D WSD.
• RQ3: Which window parameters (such as size, transparency, content types) are desired to be customized? We hypothesize that drivers have a clear preference regarding window parameters.
• RQ4: How does the driver-selected WSD personalization impact task and take-over performance? We hypothesize that both task and take-over performance are impacted by driver’s WSD personalization.
• RQ5: How does the level of vehicle automation (conditional, full) influence drivers’ preferences for 3D WSDs? We hypothesize that drivers are aware of the implications of different levels of automation and adjust the content presentation on the 3D WSD accordingly.
4 Design and Implementation
We used the open-source VR driving simulator AutoWSD (Riegler et al., 2019b; Riegler et al., 2019d), and adapted it to allow participants to freely move and adjust content windows on a simulated 3D AR windshield display while driving through a virtual city or highway scene. We utilized VR in order to keep the experiment in a safe and controlled environment (De Winter et al., 2012; Riegler et al., 2021b). Additionally, the current limited availability of highly automated vehicles makes it necessary to explore automated driving scenarios by other means, such as VR simulations. Research shows that participants’ subjective and psychological responses in VR environments are closely coupled to their experience and behaviors in a real-world setting (Slater et al., 2006). Pettersson et al. (2019) conducted a comparison study with a VR setup and a field study, and found that while VR studies cannot completely substitute field studies, they can add value to conventional driving simulators and desktop testing. In particular, VR can be used to communicate the general principles or “the story” behind a user study. Another comparison study conducted by Langlois et al. (2016) showed that the virtual environment is able to replicate relative distance perception between virtual objects (e.g., vehicles, pedestrians, road objects) and overlays. Therefore, Langlois et al. (2016) recommend that user experiments can be carried out in virtual spaces with simulated AR HUDs or WSDs.
We designed four scenarios, one for each WSD condition, in order to avoid learning effects related to traffic scenes and take-over requests. We kept the scenarios short (approx. 8 min), to minimize potential simulator sickness. We further designed a “warm-up” setting to allow participants to become familiar with the virtual reality environment. During the scenario, the WSD sequentially displayed semantic sentences, with the purpose of measuring situational awareness and task performance (Daneman and Carpenter, 1980). These sentences were provided in German and English language, according to the preferred language of the study subjects. An example for a semantically correct sentence would be “After finishing all tests the class celebrated for a whole week.” An example for a semantically incorrect sentence would be “The spider goes on vacation by plane.” Since we evaluated SAE level 3 automated driving [On-Road Automated Driving (ORAD) Committee, 2021], we did not assume subjects to place their hands on the steering wheel throughout the drive, so we implemented a speech interface for subjects to perform the task (Naujoks et al., 2016). To this end, we resorted to the offline version of the Microsoft Speech API, which is capable of processing and recognizing keywords or phrases, such as “Yes” and “No,” and their German equivalents.
Additionally, for each scenario, we designed a take-over that required subjects to grasp the steering wheel and/or press the brake pedal, and steer the vehicle out of the danger zone to avoid a collision. When such a take-over prompt was given, the WSD displayed a “Take over” warning message along with a warning tone, as it was found that such multimodal feedback was preferred by drivers (Bazilinskyy et al., 2018). We placed the warning message for both scenarios at the respective warnings window, and chose the background color red, as research found this color to aid drivers in anticipating or reacting to emergencies and warnings (Yun et al., 2018; Han and Ju, 2021).
Figure 1 shows both presentation variants for condition M (multiple content-specific windows), i.e., the flat, screen-fixed 2D baseline (left), and the potential personalization of the 3D WSD (right). Accordingly, condition S refers to the placements and characteristics of a single window used for all content types (see Figure 2).
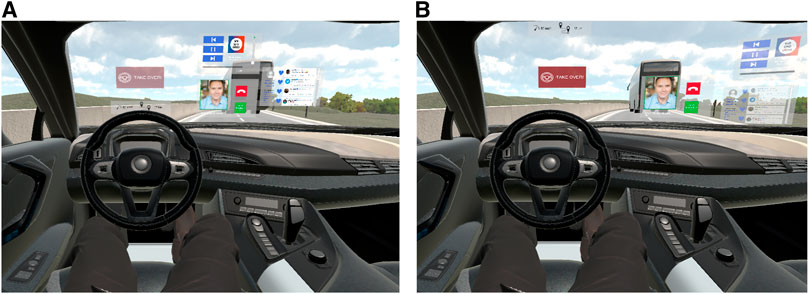
FIGURE 1. Condition M: Multiple floating windows displayed on a 3D WSD for SAE L3 AD. (A) Baseline, screen-fixed 2D WSD content proposed by Riegler et al. (2018) for SAE L3. (B) Example personalization using 3D WSD content for SAE L3.
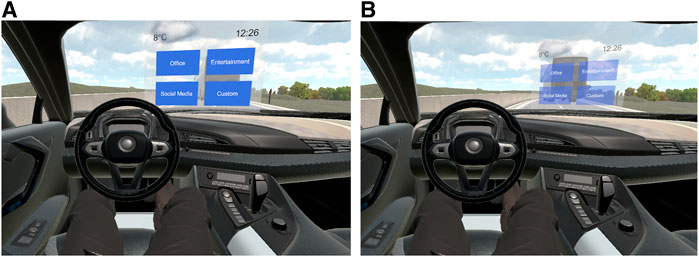
FIGURE 2. Condition S: Single main window displayed on a 3D WSD for SAE L3 AD. (A) Baseline, screen-fixed 2D WSD content proposed by Riegler et al. (2018) for SAE L3. (B) Example personalization using 3D WSD content for SAE L3.
In case a vehicle in the front was closer than a WSD content window, the window would “snap” to the back of the front vehicle, in order to avoid appearing occluded by the front vehicle (see Figure 3). In a recent study, Riegler et al. (2020c) explored anchoring WSD content to real-world objects in SAE L3 AD and found that task performance and situation awareness were increased compared to screen-fixed content. To this end, we dynamically snap the content window to the front vehicle to 1) decrease visual clutter between the real and virtual objects, and 2) improve the driver’s awareness of the front vehicle’s movements by adjusting the content window correspondingly. The snapping functionality only pertains to other moving road objects, such as cars, busses, and trucks, in order to facilitate situation awareness, as in SAE L3 AD the driver must be prepared to intervene within limited time (SAE On-Road Automated Vehicle Standards Committee, 2021). Especially for platooning situations, and traffic jams, where the conditionally automated vehicle would operate as a “traffic jam chauffeur” (Luca et al., 2018), the snap functionality may be useful Riegler et al. (2020c).
5 Driving Simulator Study
We designed our user study as 2 × 2 within-subject design, where each study participant would try both content presentation modes (i.e., multiple windows, condition M, and one single main window, condition S) for both the baseline and personalized content presentation. Additionally, we used a counterbalanced design to reduce the bias caused by the order in the variables.
5.1 Procedure
First, the subjects were given an introduction to the purpose of the study. This included the explanation of the virtual reality driving simulator (see Figure 4), automated driving at SAE level 3. We further explained the concept of a windshield display and emphasized the precedence of a safe but also comfortable drive. Additionally, we gave an overview of the scenario procedure. Next, participants were asked to answer a demographic questionnaire, as well as the Affinity for Technology Interaction (ATI) questionnaire which is used to determine subjects’ attitudes and engagements towards technology interplay (Attig et al., 2018), and finally the pre-study Simulator Sickness Questionnaire (SSQ) (Kennedy et al., 1993) to determine the initial level of nausea before proceeding. After each of the two scenario in the virtual reality environment, participants had to fill in the Technology Acceptance Model (TAM) (Davis, 1993), NASA Raw Task Load Index (NASA RTLX) (Hart and Staveland, 1988), and User Experience (UEQ-S) (Schrepp et al., 2017) questionnaires. Finally, subjects were asked to complete the post-study Simulator Sickness Questionnaire (SSQ) (Kennedy et al., 1993) and to describe their experience in an interview. The interview also included questions about WSD user preferences for fully automated vehicles. The entire procedure took approximately 75 min per participant. Subjects did not receive any compensation for participating in the study.
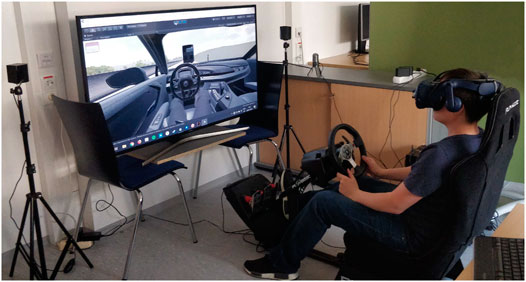
FIGURE 4. The study setting. The study participant is wearing the HTC Vive Pro Eye Virtual Reality headset, which includes speakers for audio output. The Logitech steering wheel and pedals are used for take-overs and manual driving. The microphone next to the steering wheel is used for speech input. The monitor is used by the study organizer to follow along.
5.1.1 Windshield Display Personalization
For both conditions M and S, participants were given the opportunity to adjust the initial baseline condition (content windows on a flat 2D WSD). With assistance of our experimenters, participants were asked to position multiple content windows (condition M, “multiple windows”) on the WSD according to their personal preferences, and afterwards associate each content window with further attributes such as their transparency and size. We set the initial WSD layout and content windows according to the qualitative findings by Riegler et al. (2018).
For each window, participants had to specify the desired content type (by drag and drop). Therefore, we offered a list containing potential media types:
• Warnings (W), such as a potentially short headway, mechanical failures, etc.
• Vehicle information (V), such as the current speed, or distance/time to destination
• Work/office related information (O), such as emails or calendar
• Entertainment (E), such as music playlists, videos, etc.
• Social Media (S), such as Facebook, Twitter, etc.
• Custom/Other (C), such as notifications, weather information, smarthome control, etc.
Consequently, the participants started with the personalization of the provided content windows (i.g., warnings, vehicle-related information, entertainment, social media, work-related information, etc.) on the 3D WSD on a large monitor, using a computer mouse, and subsequently refining the layout in the virtual environment, using the directional-pad buttons on the steering wheel. The reason we chose to let subjects first generate a coarse layout on a monitor was because of its ease to move the content windows, and quickly modify the layout to one’s preference. Additionally, we instructed participants that speech commands to adjust the content windows were possible, such as “place the social media window more to the right,” in which case the study organizer would perform the changes with the Wizard-of-Oz technique. For condition S (“single window”), the participants could only place that one-fits-all window on the WSD. We have chosen to use only rectangles as content windows, since this shape is most commonly used in applications in many areas, such as desktop computers, tablets, or smartphones. Following the initial positioning and sizing of the windows, participants were then asked to put on the HTC Vive Pro Eye and the study organizer started the VR application with the subject’s initial WSD layout. Participants could familiarize themselves with the VR environment and their WSD personalization on a short warm-up track, and fine-tune the WSD layout further. Subsequently, the instructions for the current task were given, and the scenario began with the vehicle starting to drive automatically. During the ride, subjects were also allowed to adjust their WSD personalization, however, we noted that this could negatively affect their task and take-over performance.
5.1.2 Non-Driving Related Task
For the cognitive task engagement, we chose a reading comprehension task, which is based on the reading-span task by Daneman and Carpenter (1980), in which subjects had to determine whether a given sentence is semantically correct or incorrect. We used this particular task as NDRT as it is commonly used in office and social media tasks, such as proofreading, and reading text messages, and can be compared between conditions and users. Participants were requested to rate the sentences as quickly but also as correct as possible on their semantic correctness.
For condition S, the text was displayed on the one main window on the WSD, and for condition M, the text was displayed in the work window. Only one sentence was shown to the participant at once. In case the sentence was semantically correct, participants were asked to say “Yes,” otherwise “No.” Upon the affirmative or negative answer, correct or not, the next sentence would be displayed. We recorded the task completion time as well as correctness of the answer. If the participant took more than 10 s to answer the given sentence, it was counted as incorrectly answered, and the next sentence was displayed.
5.1.3 Take-Over Task
Participants drove on a two-lane highway road, and always started with activated AD mode. As we used SAE L3 scenarios, we designed take-over requests (TORs) where the driver would need to take control of the vehicle and steer it manually away from the danger zone. In case a TOR occurred, the warning was displayed in the “warning” window in condition M, and in the single main window in condition S. In that case, all other WSD contents were faded out to emphasize the importance of the TOR warning. Additionally, the TOR display message was accompanied by an auditory “beep.” A TOR was displayed when an accident occurred in front of the vehicle, representing an emergency TOR as the time to collision, or TOR lead time, was approx. 5 s (Eriksson and Stanton, 2017; Wintersberger et al., 2019). At this time, participants had to take over control of the vehicle, either by pressing a pedal for >5% or a steering angle change of >2° (Wintersberger et al., 2017a), to activate the manual driving mode and subsequently to avoid a collision. After steering the vehicle away from the danger zone, the vehicle resumed automated driving, and the WSD content was faded back in. Since SAE L3 conditional automated driving was studied, and therefore not all potential traffic situations and road maneuvers could be performed by the vehicle, we instructed participants to both carry out the NDRT and to keep in mind that take-over requests could occur at any time during the drive.
5.1.4 Measurements for Safety, Usability, User Experience, Workload, and Acceptance
To assess safety, we determine TOR reaction times. For usability, we assessed drivers’ NDRT performance. For evaluating the workload, we used self-ratings using the NASA RTLX questionnaire. Acceptance of the investigated technologies was measured with self-ratings based on the Technology Acceptance Model questionnaire. Usability and user experience was determined using the self-rated User Experience Questionnaire.
5.2 Participants
We recruited 24 participants (14 male, 10 female) aged between 21 and 52 (Mean = 27.1, SD = 7.1) years with no knowledge of the project from the general population of our university, using mailing lists and posted flyers. All subjects were in possession of a valid driver’s license. In terms of annual mileage, nine participants drove less than 2,000 km, and (36%) 10 participants between 2,000 and 10,000 km (40%). The remaining five respondents reported a higher mileage (24%). Furthermore, 17 participants were already familiar with virtual reality. Participants had normal or corrected to normal vision. Participants with glasses were able to keep them on while wearing the HTC Vive Pro Eye HMD. The questionnaire on interaction-related technical affinity (ATI scale) provided a mean value of 4.24 (SD = 1.19) for the sample, which means that the participants assessed themselves as having a high affinity for technology (Attig et al., 2018).
6 Results
In the following, we present a detailed analysis of the collected data with respect to our research questions. To evaluate differences in task and take-over performance for conditions M and S, we performed analyses of variance (ANOVAs) with Bonferroni correction considering a 5% threshold for significance. In case of multiple comparisons, we adjusted the significance level from α = 0.05 to the Bonferroni-corrected α′. For multi-item self-rating scores, we computed Cronbach’s α, resulting in satisfying reliability scores for all NASA RTLX, UEQ-S, and TAM dimensions. Throughout this paper, we use the abbreviations Mdn for median, SD for standard deviation, and SE for standard error.
6.1 Windshield Display Characteristics
For both conditions, we determined the window properties position, width, height, tilt, distance, and transparency. For condition M, we additionally counted the number of utilized windows for each content type. This was not the case for condition S, as the single WSD window contained all content types. The overview of the personalized WSD window properties for both conditions is provided in Table 1, where both the mean values and standard deviations are provided for each metric.
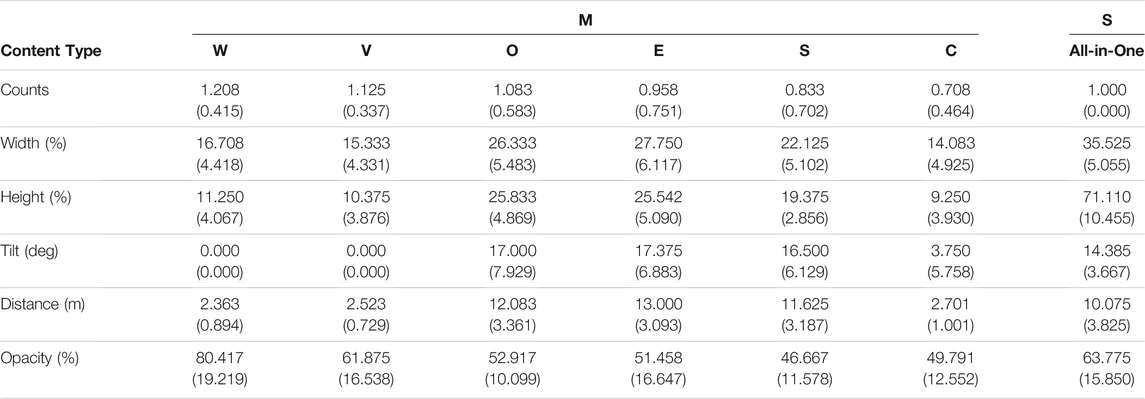
TABLE 1. Overview of the different content types and their window properties as specified by the study participants. The SD are given in brackets.
6.1.1 Condition M: Multiple Content-Specific Windows
6.1.1.1 Counts
The Counts metric is only relevant for condition M, as condition S always consists of a single main window. For condition M, the number of utilized windows differs with its associated content type. Participants seem to be well aware of the limitations of conditionally automated vehicles (SAE L3), as they provided a mean number of 1.208 warning windows (W) on the WSD. This signifies the importance of receiving feedback from the AD system, especially in safety-relevant situations. Also, vehicle-related information windows (V) were utilized commonly, and work (O), entertainment (E) as well as social media (S) windows were not used as often. Finally, custom content windows (C) that do not include any of the aforementioned information, for example smarthome information, were used more sparingly.
6.1.1.2 Position
We also looked at the prominent positions for each content-specific window. While warning and vehicle-related windows were mostly placed on the left section of the WSD, work, entertainment and social media windows were positioned in the center location of the WSD. Warnings were prominently placed at the bottom of the WSD, similar to currently available HUDs, vehicle-related information were placed both at the bottom and top left side of the WSD. Further, custom-information windows were mostly placed in peripheral sections of the WSD.
6.1.1.3 Width and Height
We calculated the width and height of each content window as a ratio of the entire windscreen dimensions. Work, entertainment and social media windows received the largest area of the windshield for their content. Warnings and vehicle-related information, and particularly custom-content windows, did not receive as much screen space from the participants.
6.1.1.4 Tilt
As study subjects had the option to rotate/tilt the content windows, we also measured these individual preferences. Interestingly, warnings and vehicle-related information were not rotated at all, which makes sense as these windows were prominently placed on the left side of the vehicle where the driver is situated. Additionally, custom-content windows were rarely tilted as subjects did not suppose to look at these windows often or for longer periods of time. In contrast, the remaining content windows, as they were located in the center of the WSD, as opposed to the driver’s side, were tilted towards the driver with a mean angle of approx. 17°.
6.1.1.5 Distance
The distance parameter shows significant differences between the content windows, as warnings and vehicle-related information, as well as custom-content windows, were placed more closely to the driver, similar to currently available HUDs, with approx. 2 m distance from the driver. Contrariwise, work, entertainment, and social media windows were placed at larger distances of approx. 12 m.
6.1.1.6 Opacity
We also investigated differences regarding the provided opacity for each content window, and found that warnings received the highest opacity (approx. 80%), while other content types showed more transparency. These findings indicate that warnings are considered important to the users, hence the low transparency, and other media types, where immediate actions might be perceived as not as important, were designed to be more transparent.
6.1.2 Condition S: Single Main Window
6.1.2.1 Position
The single main window was prominently placed in the horizontal and vertical center of the windshield. While some participants started to position it either on the driver’s side, or on the passenger’s side, they realized during the drive that both these setups were not ideal in terms of see-through capabilities, and visual attention in case of take-over requests.
6.1.2.2 Width and Height
The main window was designed to be larger than any single content window in condition M, spanning approx. 35% of the windscreen’s width, and 70% of the windscreen’s height. We found that some participants had troubles finding the “right” dimensions to accommodate the media types, and would therefore design the window larger.
6.1.2.3 Tilt
As the window was mainly positioned in the center of the windscreen, participants chose to tilt it towards the driver’s direction for better readability and reduced perspective distortion of the content.
6.1.2.4 Distance
Distance-wise, we found user-specific differences as participants on the one hand intended to use the main window as a replacement for nowadays common tablet interfaces located at the center stack of the vehicle. On the other hand, similar to condition M, where users placed work, entertainment, and social media windows farther away, the single main window was placed such that all potential content types would be appropriately visible, according to the participants. To this end, most participants opted to place the window at a distance of approx. 10 m, which puts it farther away and today’s HUDs, but not as distant as some content windows in condition M.
6.1.2.5 Opacity
The mean opacity of the single main window was set to approx. 63%, which, again, similar to the above metrics, is an intermediate value compared to the content windows in condition M. As the main window is quite large, and placed in the center of the WSD, on average, the transparency reflects this circumstance. Participants seem to be aware that in SAE L3 AD, drivers should still monitor the outside environment from time to time, and take-over requests can occur at any time, and hence designed a semi-transparent user interface.
6.2 Quantitative Measures
As quantitative measures, we assessed the error rates, task completion times, as well as take-over request (TOR) times for both conditions, for both the baseline and personalized designs. Table 2 gives an overview of the overall task performances and take-over times. Since the results are normally distributed, we applied a paired two-samples t-test for our further analysis.

TABLE 2. Paired two samples t-test for NDRT and take-over performance for the baseline and personalized variants of conditions M and S.
6.2.1 Task Performance and Error Rate
The mean task completion time (i.e., giving a reply regarding the semantic correctness of a given sentence) for condition M (baseline) was 6.784s (SD = 0.955) and for condition S (baseline) 6.204s (SD = 0.988) (see Table 2). The respective personalized user interfaces led to a mean task completion time of 6.168s (SD = 1.079) for condition M, and 5.810s (SD = 1.266) for condition S. As expected, the user-defined personalized UIs achieved better results than their pre-defined baseline counterparts. Regarding statistical significance, the personalized single-window UI achieved a significantly better task performance than the baseline multi-window solution (p = 0.01, Cohen’s d = 0.98).
The mean error rate for condition M (baseline) was 0.225 (SD = 0.184) and for condition S (baseline) 0.277 (SD = 0.158). Again, the personalized WSD UIs resulted in lower error rates than the baseline layouts. However, we did not find a statistically significant differences between all conditions.
6.2.2 Take-Over Performance
Regarding the mean take-over time, we identified a statistically significant difference between the multi-window baseline and personalized UIs (baseline: Mean = 3.358s, SD = 1.426, personalized: Mean = 2.451s, SD = 1.040, p = 0.025, Cohen’s d = 1.0). Additionally, we found a statistically significant difference between the single-window personalized UI and the multi-window baseline UI (p = 0.021, Cohen’s d = 1.05). Therefore, the personalized window modes resulted in faster take-over times when compared to their baseline counterparts.
6.3 Qualitative Measures
To validate the VR driving simulator setup, we report on simulator sickness based on the Simulator Sickness Questionnaire (SSQ) used before and after the VR drive. We further assessed the Technology Acceptance Model (TAM), the User Experience Questionnaire (UEQ-S), as well as the NASA Raw Task Load Index (NASA RTLX). Additionally, we report interview results based on semi-structured interviews conducted after the experiment.
6.3.1 Technology Acceptance Model
Considering the TAM, we evaluated the subscales Perceived Usefulness (PU), Perceived Ease of Use (PEOU), Attitude towards Use (ATT), Intent of the system, and Trust in the system. Considering the means of the evaluated TAM subscales we can see that condition M (multi-window UI) received higher ratings than condition S (single-window UI) in the scales PU, PEOU, ATT, and Trust when comparing the personalized variants. The comparative analysis of the inter-condition variants (baseline vs. personalized UIs) reveals that the personalized variants received equal or better ratings by the subjects (see Table 3). Overall, the highest subjective ratings were given to the personalized multi-window user interface. Friedman ANOVA reported significant differences between PU (p = 0.030), PEOU (p = 0.022), and ATT (p = 0.034) between both personalized UIs.
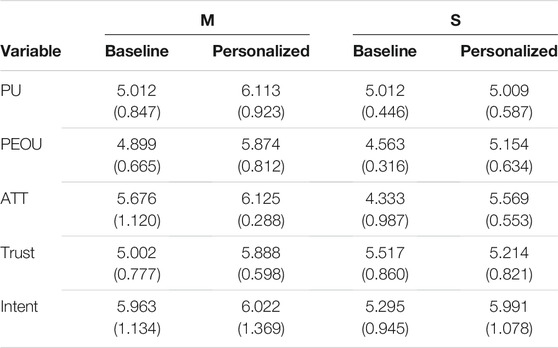
TABLE 3. Overview of the mean values of the TAM scores for the multi-window (M) and single-window (S) conditions. The SD are given in brackets.
6.3.2 User Experience Questionnaire
The User Experience Questionnaire Short (UEQ-S) allowed us to calculate the pragmatic quality (usability) and hedonic quality (user experience) of the two WSD conditions. The UEQ-S has a range from −3 to 3. Table 4 shows the mean values depending on the two WSD conditions M and S in both the baseline and customized variants. Values greater than 0.8 indicate a positive evaluation.
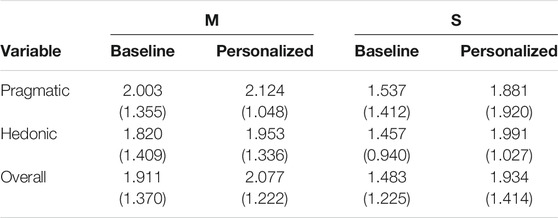
TABLE 4. UEQ-S scores for both WSD conditions, in baseline and personalized configurations. The SD are given in brackets.
Table 4 gives an overview of the UEQ-S results. For the pragmatic quality a mean value of 2.003 (SD = 1.355) was achieved for condition M (baseline) and 2.124 (SD = 1.048) for condition M (personalized). For conditions S, we calculated for the pragmatic quality a mean value of 1.537 (SD = 1.412) (baseline) and 1.881 (SD = 1.920) (personalized), respectively. Accordingly, a mean value of 1.820 (SD = 1.409) for condition M (baseline) and a value of 1.953 (SD = 1.336) for condition M (personalized) was determined for the hedonic quality, and 1.457 (SD = 0.940) for condition S (baseline) and 1.991 (SD = 1.027) for condition S (personalized). Friedman ANOVA revealed significant effects for the overall UEQ-S scores of the baseline and personalized single-window conditions (p = 0.009). The comparative analysis of both multi-window variants resulted in no statistically significant differences.
6.3.3 NASA Raw Task Load Index
Figure 5 shows the aggregated NASA RTLX results for the WSD conditions in both baseline and personalized variants. The scales physical and temporal demand were on average rated higher as the other categories. As expected, the default baseline configurations received a higher subjective workload than the custom user-defined setups. However, comparing the two personalized variants provides interesting insights. While mental demand and frustration were rated lower for condition M, the metrics performance and effort received more positive ratings for condition S. We also calculated the total mean score for the WSD conditions. For condition M (baseline), we determined a total mean score of 25.00 (SD = 5.58), and for the personalized counterpart 20.14 (SD = 9.64). In contrast, the total mean score for condition S (baseline) is 24.51 (SD = 4.82), and for the personalized variant 19.23 (SD = 5.67). They represent the subjectively experienced stress and were calculated from the mean values of the six subscales. Using the t-test for independent samples, we did not find a statistically significant difference between the two personalized variants. However, we calculated a statistically significant difference between the baseline and personalized variant in condition S (p = .007).
6.3.4 Simulator Sickness Questionnaire
In order to validate the VR driving simulation, we assessed the participants’ simulator sickness using the standardized Simulator Sickness Questionnaire (SSQ). The SSQ consists of 16 questions and allows the analysis and measurement of simulator sickness from various perspectives. Each question was derived from the results of various experiments on the effects of simulators and interaction methods used in VR applications, and the symptoms of users. Participants selected one of four answers (none, slight, moderate, and severe) on a Likert scale for each of the 16 questions. None, slight, moderate, and severe are converted to 0, 1, 2, and 3 points, respectively. We used the SSQ before and after the VR scenarios for each subject. Results show that nausea (items 1, 6, 7, 8, 9, 15, and 16) among all participants received a mean value of 0.147 (SD = 0.233); oculomotor sickness (items 1, 2, 3, 4, 5, 9, and 11) amounted to a mean value of 0.245 (SD = 0.515); and disorientation sickness (items 5, 8, 10, 11, 12, 13, and 14) received a mean score of 0.265 (SD = 0.410) before the VR exposure. After the VR scenarios, SSQ results revealed that nausea among all participants received a mean value of .385 (SD = 0.350); oculomotor sickness amounted to a mean value of 0.390 (SD = 0.625); and disorientation sickness received a mean score of 0.344 (SD = 0.500) When interpreting a given score of zero as no VR sickness, and 1 as slight VR sickness, these results suggest that the VR driving simulator caused very little VR sickness. The items that were most graded as moderate or severe were “Eye strain” (Mean = 0.890, SD = 0.855) and “Difficulty focusing” (Mean = 0.769, SD = 0.896), and “Blurred vision” (Mean = 0.713, SD = .800). Since no participant felt moderate or even severe overall VR sickness, we were able to use all participant data for analysis.
6.3.5 Interviews
By interviewing participants after the experiment, we aimed to gain further insight into the subjects’ experiences. They were asked to share their general experience, attitude, and preference towards the system and the individual display modes.
Participants preferred condition M over condition S in terms of customization and personalization. Because participants had the autonomy to move individual content windows for different tasks/needs, it was possible to “design the windshield display as large command center.” Five participants also indicated that collaborative aspects were more supported by the multi-window UI, as passenger-specific content could easily be placed on the passenger side of the vehicle, rather than using the single window interface in the center of the WSD (condition S). In addition, the single-window UI was found to require appropriate navigation concepts for efficient navigation between content types, such as work tasks, and entertainment activities. For example, it was stated that media consumption should be accessible as soon as it is required by the driver/user and that no cumbersome navigation should be necessary to view that content. In this respect, traditional menu navigation should be avoided, and speech interfaces, such as conversational UI could also be considered (Braun et al., 2017). It was also brought up that the single-window interface was poorly suited for micro-tasks, such as quickly checking notifications, or skimming a few emails, while longer tasks, such as reading the news or watching videos could be better accomplished by directing attention towards only one main window on the WSD. This insight by half the participants was also reflected in the better task performance of condition S compared to condition M, which required subjects to focus on the cognitive non-driving related task. Participants indicated that one way to get the “best of both worlds” would be to provide the ability to adjust multiple windows (condition M), and to provide a type of “focus/performance mode” that emphasizes visualization of the desired task, while the other tasks fade into the background. In terms of initial setup time, most participants stated that the multi-window setup was more tedious to fine-tune, while the single-window UI provided a faster initial customization. In addition, safety aspects were also discussed. When asked whether they felt safer in condition M or S, most subjects indicated that condition M conveyed a greater overall sense of safety (“better overview of the outside road environment”), because most content windows were smaller and more transparent as well as located in more peripheral areas of the WSD, compared to the single-window UI. Participants also noted that a potential large window intended for elaborate work or entertainment tasks might be more appropriate for fully automated vehicles to create a more immersive environment, while simple, quick tasks might be better visualized using smaller, scattered windows as envisioned in condition M.
Since we wanted to gain insights into WSD user preferences for fully automated vehicles (SAE L5), we concluded the interview by asking participants how they would adjust their preferred presentation mode (multi-window or single-window layout) based on their experiences in the SAE L3 driving scenarios. Therefore, we gave participants the opportunity to modify all WSD parameters (e.g., size, distance, transparency etc.), and received similar results to Riegler et al. (2019e) in terms of increased utilization of the WSD space. More than two third of participants chose the multi-window WSD setup stating “the ability to have all information at a glance” and “using the vehicle as a personal command center for many tasks.” Users who preferred a single main window for content display noted that this setup would allow for a “full immersion in the digital content.” The “ability to engage in playful activities or work collaboration with other passengers” was also mentioned. Since SAE L5 AVs require no human driving interactions, subjects updated their WSD preferences by making the work and entertainment windows larger as well as moving them to more user-centric locations, while warning and vehicle-related information windows were either removed or placed in peripheral areas of the WSD, consistent with prior work by Riegler et al. (2019e). Additionally, distance and tilt parameters were only slightly changed, however, windows were made more opaque for “increased visibility and immersion of the WSD content”. For SAE L3 conditionally AVs, drivers seemed to be aware that occluding the driving scene with many or large digital overlays would impair their ability to stay in the loop and take over control of the AV (Riener et al., 2017), however, SAE L5 AVs eliminate this constraint. Concerns were expressed about motion sickness in fully automated vehicles, especially when the outside environment is not visible because of opaque WSD content (“not knowing when sharp turns or emergency brakes occur would diminish the in-vehicle experience”).
7 Discussion
Three-dimensional augmented reality windshield displays are an interesting concept in the context of highly automated driving. Answering RQ1, and as an extension of the initial research by Riegler et al. (2018) on two-dimensional WSDs for semi-automated vehicles, our results indicate that the additional distance parameters for displaying continuous-depth content on the WSD were used by our participants. While there are some common areas for certain content types in both configurations, we found that more peripheral windows were not only placed at a greater distance, but also tilted towards the driver, which was not considered in screen-fixed two-dimensional layouts. Overall, the personalized user interfaces differed from the baseline concepts in some aspects such as placement on the WSD, mainly influenced by the distance parameter. While current HUDs are screen-fixed, recent concepts showcased by automobile manufacturers use AR with continuous depth, which, combined with higher levels of vehicle automation, introduces a new design and research scope for immersive experiences in the vehicle (Wiegand et al., 2019; Yontem et al., 2020).
As for RQ2, our study subjects appeared to be aware of the boundaries of conditionally automated driving and, in case of the multi-window user interface, placed warning messages in the driver’s side of the WSD, and less critical content types in the peripheral areas of the WSD. As Topliss et al. (2019) found, manual driving performance deteriorates when WSD is displayed further from the driver’s forward view. For the single-window presentation mode, participants had to balance an acceptable intermediate solution between being able to observe roadside activities, and performing the non-driving related task. Accordingly, window size, distance, and transparency parameters were adjusted. We examined a number of self-rated usability, user experience, and workload metrics to assess user preferences. While the personalized creations received better ratings than their baseline screen-fixed counterparts, comparison of the two personalized interfaces showed mixed results. The TAM results indicated that the multi-window customization received a higher acceptance, however, subjective user experience and workload findings revealed no significant differences. Post-experiment interviews provided further insights into potential use cases for both WSD presentation modes, such as multi-window setups with peripheral content windows for conditionally automated driving for an increased situational awareness. However, larger, more immersive and interactive content windows may be better suited for fully automated driving scenarios, which Riegler et al. (2019e) supports. For this reason, future vehicle user interfaces need to consider context awareness, such as the vehicle’s capabilities, and the ability for users to personalize in-vehicle interfaces, such as WSD content (RQ3). While warning and vehicle-related information windows were primarily placed similarly to current screen-fixed HUDs, other content windows, such as work, entertainment and social media windows, were positioned with adjusted distance and tilt parameters so that subjects accounted for the benefits and limitations of a larger UI design space in the forward viewing direction. Research (e.g., Schroeter and Steinberger, 2016; Schartmüller et al., 2018; Gerber et al., 2020) is already considering the use of WSDs to facilitate situational awareness while performing work or entertainment activities.
Regarding task performance (RQ4), we discovered that the utilization of a single main window encompassing all media types significantly improved task performance for the text comprehension task. As we found in post-study interviews, many subjects indicated that they were better able to focus on the task than when they had multiple potentially distracting windows in their field of view. Single-window UIs (condition S) might therefore be useful for focussing on specific tasks that require more and undivided attention from the user, while multi-window UIs (condition M) could be helpful for activities requiring lower attention, such as browsing the web, skimming over notifications, consuming media etc. In addition, we found that take-overs were performed faster for the single-window mode. One possible explanation for the better TOR performance could be the warning message was displayed directly in the driver’s field of view, as contrasted with the dedicated warning window in condition M, which may have required a change in the driver’s attention. Feedback design should therefore consider the driver’s viewing direction, maybe regardless of the driver’s personal preference towards a specified area of the WSD to display notifications/warnings. These findings are consistent with Abdi et al. (2015). Another way to reduce take-over times with AR interfaces could be to not only use multimodal alerts (e.g., Yun and Yang, 2020), but also highlighting outside information that could cause a takeover (e.g., inattentive pedestrians) (Kim et al., 2016). From a research viewpoint, understanding (semi-)automated vehicle behavior, and leveraging novel interfaces to enrich in-vehicle experiences for drivers and passengers is an ongoing process in which we believe AR WSDs serve as an integral catalyst.
With respect to WSD user preferences for SAE L5 fully automated vehicles (RQ5), we found that our study participants are aware of the automation capabilities of SAE L3 conditionally automated vehicles, and considered these aspects with their respective WSD designs. In our previous study on 2D WSDs (Riegler et al., 2019e), users also adjusted content windows for the different levels of vehicle automation. It is important that the general public is made aware of these automation levels and their technological capabilities and advancements. As Dixon (2020) state, the misunderstanding of automation capabilities can lead to misuse of AVs, and affect trust in automation technology as well road safety. Häuslschmid et al. (2019) investigated personalized WSD layouts in the context of manual driving, and comparison between one-fits-all and customized designs revealed safety issues for personalized WSDs. Häuslschmid et al. (2019) therefore recommended restricting areas for safety-critical information presentation. However, in higher levels of vehicle automation (i.e., SAE L3 and higher), the primary driving task may be performed by the system, and keeping the driver in the loop while they engage in NDRTs is important. Comparison of personalized WSD designs between manual (Häuslschmid et al., 2019), conditional, and fully automated driving show stark differences in user preferences, and awareness of drivers for each automation level. Our studies indicate that as vehicle automation progresses, drivers prefer engagement in work/entertainment activities, maybe even with other passengers, and vehicle-related or safety information become less critical to the driver. Motion sickness must be addressed for highly automated vehicles in conjunction with (opaque) WSD content. We believe that our “snap” functionality (Riegler et al., 2020c) could alleviate this issue, as the AR WSD could help driver/passengers anticipate future vehicle motion and reduce sensory conflicts using visual indicators (Diels et al., 2016; Wintersberger et al., 2017b).
7.1 Limitations and Future Work
We are aware of limitations in our experiment. First, the study was carried out in a VR driving simulator. Although it has been shown that VR can adequately simulate AR content (e.g., Kim and Dey, 2009; Langlois et al., 2016), user preferences and perceptions might differ in a real driving scene. In addition, our study involved only a limited population, mainly young college students and staff in Europe. Other users might behave and perform differently (Barkhuus and Rode, 2007). We would also like to point out that participants’ preferences and feedback might very over time as new technologies, and people’s attitude and acceptance towards them are introduced, such as AR HUDS and large vehicular displays. We therefore suggest that future studies should explore our proposed approach with individuals of different ages, education, technophilia, or cultural background. Additionally, we evaluated simulator sickness before and after the VR exposure to determine the internal validity of the user study (Hock et al., 2018), as we intended to design the VR scenarios in a way that would minimize simulator sickness, and thereby focus our evaluation on the WSD conditions. We did not measure simulator sickness for each WSD condition. While we are confident that AR WSDs could facilitate driver/passengers to anticipate road maneuvers by visually indicating vehicle intentions, we propose to further explore the role of simulator and motion sickness in AR WSD content presentation for highly automated vehicles.
In future studies, based on the results of this research, we intend to incorporate and evaluate an interaction management system for multimodal user interactions with windshield displays. Specifically, we aim to unify gaze, voice and gesture input for interacting with WSDs in order to establish context-aware cooperative interfaces (Walch et al., 2017). Although there is existing research on separate input modalities [e.g., head gaze (Riegler et al., 2020a), speech (Lo and Green, 2013), gestures (May et al., 2017), and olfactory (Dmitrenko et al., 2016)], we encourage researchers to exploit the large design space of AR in the automotive domain (Haeuslschmid et al., 2016a; Wiegand et al., 2019).
8 Conclusion
In this paper, we let drivers/passengers of conditionally and fully automated vehicles draft their own 3D WSD layout in a virtual reality driving simulator. We also explored both subjective preferences and objective task and take-over performance for utilizing such WSDs. Our aim was to examine how WSDs can be used to improve productivity and user experience for future drivers and passengers. To this end, we identified an initial design space for 3D WSD window parameters (such as distance, content types, and transparency) by comparing two display modalities (multiple windows for specific content types vs. one main window) in conditionally and fully automated driving settings. Our results yield a number of insights and recommendations for the use of 3D AR windshield displays in AVs:
• Customization and personalization of in-vehicle interfaces requires more research attention, and conventional one-fits-all solutions should be questioned. Depending on the level of automation of the vehicle, certain levels of in-vehicle user interface customizations should be addressed.
• The added dimensionality of variable distance by the means of continuous-depth AR WSDs shows promises in terms of objective task performance and subjective user experience in the evolution of screen-fixed HUDs towards world-relative AR interfaces for future in-vehicle activities, both for driver-related tasks and passenger experiences.
• The provision of a focus/performance mode for certain visually demanding tasks displayed on AR WSDs should be looked into. Quantitative and qualitative findings show that 3D AR interfaces have the potential to increase productivity and user experience in conditionally automated vehicles, and further context-specific experiments should explore their feasibility for additional automotive use cases.
• For fully automated vehicles, users prefer customizable, multi-window setups that visualize content-type specific applications, and facilitate interactions and collaborations with other passengers.
Data Availability Statement
The original contributions presented in the study are included in the article/supplementary material, further inquiries can be directed to the corresponding author.
Ethics Statement
Ethical review and approval was not required for the study on human participants in accordance with the local legislation and institutional requirements. The patients/participants provided their written informed consent to participate in this study.
Author Contributions
AR (1st author) had the initial idea, implemented the prototype, conducted the user study, and took the lead in writing the paper. AR (2nd author) and CH assisted with user study specific feedback, helped in writing the paper, and proof-reading.
Funding
This work was supported by the University of Applied Sciences PhD program and research subsidies granted by the government of Upper Austria.
Conflict of Interest
The authors declare that the research was conducted in the absence of any commercial or financial relationships that could be construed as a potential conflict of interest.
Publisher’s Note
All claims expressed in this article are solely those of the authors and do not necessarily represent those of their affiliated organizations, or those of the publisher, the editors, and the reviewers. Any product that may be evaluated in this article, or claim that may be made by its manufacturer, is not guaranteed or endorsed by the publisher.
References
Abdi, L., Abdallah, F. B., and Meddeb, A. (2015). In-vehicle Augmented Reality Traffic Information System: a New Type of Communication between Driver and Vehicle. Proced. Comput. Sci. 73, 242–249. doi:10.1016/j.procs.2015.12.024
Attig, C., Mach, S., Wessel, D., Franke, T., Schmalfuß, F., and Krems, J. (2018). “Technikaffinität als ressource für die arbeit in industrie 4.0,” in Innteract 2018.
Bark, K., Tran, C., Fujimura, K., and Ng-Thow-Hing, V. (2014). “Personal Navi,” in AutomotiveUI '14: Proceedings of the 6th International Conference on Automotive User Interfaces and Interactive Vehicular Applications, Seattle, WA, USA, 1–8. doi:10.1145/2667317.2667329
Barkhuus, L., and Rode, J. A. (2007). “From Mice to Men - 24 Years of Evaluation in Chi,” in Proceedings of the SIGCHI Conference on Human Factors in Computing Systems-CHI ’07, San Jose, California, USA (New York, NY, USA: ACM). doi:10.1145/1240624.2180963
Bazilinskyy, P., Petermeijer, S. M., Petrovych, V., Dodou, D., and de Winter, J. C. F. (2018). Take-over Requests in Highly Automated Driving: A Crowdsourcing Survey on Auditory, Vibrotactile, and Visual Displays. Transport. Res. F Traffic Psychol. Behav. 56, 82–98. doi:10.1016/j.trf.2018.04.001
Braun, M., Broy, N., Pfleging, B., and Alt, F. (2017). “A Design Space for Conversational In-Vehicle Information Systems,” in Proceedings of the 19th International Conference on Human-Computer Interaction with Mobile Devices and Services, Vienna, Austria, 1–8. doi:10.1145/3098279.3122122
Calvi, A., D’Amico, F., Ferrante, C., and Bianchini Ciampoli, L. (2020). Effectiveness of Augmented Reality Warnings on Driving Behaviour whilst Approaching Pedestrian Crossings: A Driving Simulator Study. Accid. Anal. Prev. 147, 105760. doi:10.1016/j.aap.2020.105760
Charissis, V., Papanastasiou, S., Mackenzie, L., and Arafat, S. (2011). “Evaluation of Collision Avoidance Prototype Head-Up Display Interface for Older Drivers,” in International Conference on Human-Computer Interaction, Berlin, Heidelberg (Springer), 367–375. doi:10.1007/978-3-642-21616-9_41
Colley, A., Häkkilä, J., Forsman, M.-T., Pfleging, B., and Alt, F. (2018). “Car Exterior Surface Displays,” in PerDis '18: Proceedings of the 7th ACM International Symposium on Pervasive Displays, Munich, Germany, 1–8. doi:10.1145/3205873.3205880
Damböck, D., Weißgerber, T., Kienle, M., and Bengler, K. (2012). “Evaluation of a Contact Analog Head-Up Display for Highly Automated Driving,” in 4th International Conference on Applied Human Factors and Ergonomics, Garching, Germany (San Francisco. USA: Citeseer).
Daneman, M., and Carpenter, P. A. (1980). Individual Differences in Working Memory and reading. J. Verbal Learn. Verbal Behav. 19, 450–466. doi:10.1016/S0022-5371(80)90312-6
Davis, F. D. (1993). User Acceptance of Information Technology: System Characteristics, User Perceptions and Behavioral Impacts. Int. J. Man-Machine Stud. 38, 475–487. doi:10.1006/imms.1993.1022
De Winter, J., van Leeuwen, P. M., and Happee, R. (2012). “Advantages and Disadvantages of Driving Simulators: A Discussion,” in Proceedings of Measuring Behavior, Utrecht, The Netherlands.
Diels, C., Bos, J. E., Hottelart, K., and Reilhac, P. (2016). “The Impact of Display Position on Motion Sickness in Automated Vehicles: an On-Road Study,” in Automated vehicle symposium, San Francisco, US (San Francisco), 19–21.
Dillmann, J., den Hartigh, R. J. R., Kurpiers, C. M., Raisch, F. K., de Waard, D., and Cox, R. F. A. (2021). Keeping the Driver in the Loop in Conditionally Automated Driving: A Perception-Action Theory Approach. Transport. Res. F Traffic Psychol. Behav. 79, 49–62. doi:10.1016/j.trf.2021.03.003
Dixon, L. (2020). Autonowashing: the Greenwashing of Vehicle Automation. Transport. Res. Interdiscip. Perspect. 5, 100113. doi:10.1016/j.trip.2020.100113
Dmitrenko, D., Vi, C. T., and Obrist, M. (2016). “A Comparison of Scent-Delivery Devices and Their Meaningful Use for In-Car Olfactory Interaction,” in Proceedings of the 8th International Conference on Automotive User Interfaces and Interactive Vehicular Applications, Ann Arbor, MI, 23–26. doi:10.1145/3003715.3005464
Dmitrenko, D., Maggioni, E., and Obrist, M. (2019). “Towards a Framework for Validating the Matching between Notifications and Scents in Olfactory In-Car Interaction,” in Extended Abstracts of the 2019 CHI Conference on Human Factors in Computing Systems, Glasgow, Scotland, 1–6. doi:10.1145/3290607.3313001
Eriksson, A., and Stanton, N. A. (2017). Takeover Time in Highly Automated Vehicles: Noncritical Transitions to and from Manual Control. Hum. Factors 59, 689–705. doi:10.1177/0018720816685832
Frison, A.-K., Wintersberger, P., Schartmüller, C., and Riener, A. (2019). “The Real T (H) or: Evaluation of Emergency Take-Over on a Test Track,” in Proceedings of the 11th International Conference on Automotive User Interfaces and Interactive Vehicular Applications: Adjunct Proceedings, Utrecht, Netherlands, 478–482.
Fu, W.-T., Gasper, J., and Kim, S.-W. (2013). “Effects of an In-Car Augmented Reality System on Improving Safety of Younger and Older Drivers,” in 2013 IEEE International Symposium on Mixed and Augmented Reality (ISMAR), Australia: Adelaide, SA, 59–66. doi:10.1109/ismar.2013.6671764
Gabbard, J. L., Fitch, G. M., and Kim, H. (2014). Behind the Glass: Driver Challenges and Opportunities for AR Automotive Applications. Proc. IEEE 102, 124–136. doi:10.1109/jproc.2013.2294642
Gerber, M. A., Schroeter, R., Xiaomeng, L., and Elhenawy, M. (2020). “Self-Interruptions of Non-driving Related Tasks in Automated Vehicles: Mobile vs Head-Up Display,” in Proceedings of the 2020 CHI Conference on Human Factors in Computing Systems, CHI ’20, New York, NY, 1–9. doi:10.1145/3313831.3376751
Häuslschmid, R., Osterwald, S., Lang, M., and Butz, A. (2015). “Augmenting the Driver’s View with Peripheral Information on a Windshield Display,” in Intelligent User Interfaces 2015, Atlanta, Georgia (New York, USA: ACM).
Häuslschmid, R., Ren, D., Alt, F., Butz, A., and Höllerer, T. (2019). Personalizing Content Presentation on Large 3D Head-Up Displays. PRESENCE: Virtual Augmented Reality 27, 80–106. doi:10.1162/pres_a_00315
Haeuslschmid, R., Pfleging, B., and Alt, F. (2016a). “A Design Space to Support the Development of Windshield Applications for the Car,” in The 2016 CHI Conference, San Jose, California (New York, USA: ACM Press), 5076–5091. doi:10.1145/2858036.2858336
Haeuslschmid, R., Shou, Y., O’Donovan, J., Burnett, G., and Butz, A. (2016b). “First Steps towards a View Management Concept for Large-Sized Head-Up Displays with Continuous Depth,” in The 8th International Conference, Ann Arbor, MI (New York, USA: ACM Press), 1–8. doi:10.1145/3003715.3005418
Han, J.-H., and Ju, D.-Y. (2021). Advanced Alarm Method Based on Driver's State in Autonomous Vehicles. Electronics 10, 2796. doi:10.3390/electronics10222796
Hart, S. G., and Staveland, L. E. (1988). Development of Nasa-Tlx (Task Load index): Results of Empirical and Theoretical Research. Adv. Psychol. 52, 139–183. doi:10.1016/s0166-4115(08)62386-9
Hock, P., Kraus, J., Babel, F., Walch, M., Rukzio, E., and Baumann, M. (2018). “How to Design Valid Simulator Studies for Investigating User Experience in Automated Driving: Review and Hands-On Considerations,” in Proceedings of the 10th International Conference on Automotive User Interfaces and Interactive Vehicular Applications, Toronto, ON, 105–117.
Horswill, M. S., and Plooy, A. M. (2008). Auditory Feedback Influences Perceived Driving Speeds. Perception 37, 1037–1043. doi:10.1068/p5736
Hwang, Y., Park, B.-J., and Kim, K.-H. (2016). Effects of Augmented-Reality Head-Up Display System Use on Risk Perception and Psychological Changes of Drivers. ETRI J. 38, 757–766. doi:10.4218/etrij.16.0115.0770
Kennedy, R. S., Lane, N. E., Berbaum, K. S., and Lilienthal, M. G. (1993). Simulator Sickness Questionnaire: An Enhanced Method for Quantifying Simulator Sickness. Int. J. Aviation Psychol. 3, 203–220. doi:10.1207/s15327108ijap0303_3
Kern, D., and Schmidt, A. (2009). “Design Space for Driver-Based Automotive User Interfaces,” in Proceedings of 1st International Conference on Automotive User Interfaces and Interactive Vehicular Applications, Automotive UI 2009, Essen, Germany (New York, USA: ACM). doi:10.1145/1620509.1620511
Kim, S., and Dey, A. K. (2009). “Simulated Augmented Reality Windshield Display as a Cognitive Mapping Aid for Elder Driver Navigation,” in Proceedings of the 27th International Conference on Human Factors in Computing Systems, CHI 2009, Boston, MA, 133. doi:10.1145/1518701.1518724
Kim, H., Miranda Anon, A., Misu, T., Li, N., Tawari, A., and Fujimura, K. (2016). “Look at Me: Augmented Reality Pedestrian Warning System Using an In-Vehicle Volumetric Head up Display,” in Proceedings of the 21st International Conference on Intelligent User Interfaces, Sonoma, California, 294–298.
Kun, A. L., Tscheligi, M., Riener, A., and van der Meulen, H. (2017). “Arv 2017: Workshop on Augmented Reality for Intelligent Vehicles,” in Proceedings of the 9th International Conference on Automotive User Interfaces and Interactive Vehicular Applications Adjunct-AutomotiveUI ’17, Oldenburg, Germany (New York, NY, USA: ACM), 47–51.
Langlois, S., and Soualmi, B. (2016). “Augmented Reality versus Classical Hud to Take over from Automated Driving: An Aid to Smooth Reactions and to Anticipate Maneuvers,” in 2016 IEEE 19th International Conference on Intelligent Transportation Systems (ITSC), Rio de Janeiro, Brazil (IEEE), 1571–1578. doi:10.1109/itsc.2016.7795767
Langlois, S., That, T. N., and Mermillod, P. (2016). “Virtual Head-Up Displays for Augmented Reality in Cars,” in Proceedings of the European Conference on Cognitive Ergonomics, Nottingham, UK, 1–8. doi:10.1145/2970930.2970946
Lee, J.-h., Yanusik, I., Choi, Y., Kang, B., Hwang, C., Park, J., et al. (2020). Automotive Augmented Reality 3d Head-Up Display Based on Light-Field Rendering with Eye-Tracking. Opt. Express 28, 29788–29804. doi:10.1364/oe.404318
Lo, V. E.-W., and Green, P. A. (2013). Development and Evaluation of Automotive Speech Interfaces: Useful Information from the Human Factors and the Related Literature. Int. J. Vehicular Techn 2013. doi:10.1155/2013/924170
Luca, S., Serio, A., Paolo, G., Giovanna, M., Marco, P., and Bresciani, C. (2018). “Highway Chauffeur: State of the Art and Future Evaluations: Implementation Scenarios and Impact Assessment,” in 2018 International Conference of Electrical and Electronic Technologies for Automotive, Milan, Italy (IEEE), 1–6. doi:10.23919/eeta.2018.8493230
May, K. R., Gable, T. M., and Walker, B. N. (2017). “Designing an In-Vehicle Air Gesture Set Using Elicitation Methods,” in Proceedings of the 9th International Conference on Automotive User Interfaces and Interactive Vehicular Applications-AutomotiveUI ’17, Oldenburg, Germany (New York, NY, USA: Association for Computing Machinery), 74–83. doi:10.1145/3122986.3123015
Medenica, Z., Kun, A. L., Paek, T., and Palinko, O. (2011). “Augmented Reality vs. Street Views: a Driving Simulator Study Comparing Two Emerging Navigation Aids,” in Proceedings of the 13th International Conference on Human Computer Interaction with Mobile Devices and Services, Stockholm, Sweden, 265–274.
Meixner, G., Häcker, C., Decker, B., Gerlach, S., Hess, A., Holl, K., et al. (2017). “Retrospective and Future Automotive Infotainment Systems-100 Years of User Interface Evolution,” in Automotive User Interfaces (Springer), 3–53. doi:10.1007/978-3-319-49448-7_1
Naujoks, F., Forster, Y., Wiedemann, K., and Neukum, A. (2016). “Speech Improves Human-Automation Cooperation in Automated Driving,” in Mensch und Computer 2016 – Workshop Band, Aachen, Germany. Editors B. Weyers, and A. Dittmar (Aachen: Gesellschaft für Informatik e.V.).
On-Road Automated Driving (ORAD) Committee (2021). Taxonomy and Definitions for Terms Related to On-Road Motor Vehicle Automated Driving Systems. [Dataset].
Pettersson, I., Karlsson, M., and Ghiurau, F. T. (2019). “Virtually the Same Experience? Learning from User Experience Evaluation of In-Vehicle Systems in Vr and in the Field,” in Proceedings of the 2019 on Designing Interactive Systems Conference, San Diego, CA, 463–473.
Pfleging, B., Schneegass, S., and Schmidt, A. (2012). “Multimodal Interaction in the Car: Combining Speech and Gestures on the Steering Wheel,” in Proceedings of the 4th International Conference on Automotive User Interfaces and Interactive Vehicular Applications, Portsmouth, New Hampshire, 155–162.
Pfleging, B., Rang, M., and Broy, N. (2016). “Investigating User Needs for Non-driving-related Activities during Automated Driving,” in Proceedings of the 15th International Conference on Mobile and Ubiquitous Multimedia-MUM ’16 (New York, NY, USA: ACM), 91–99. doi:10.1145/3012709.3012735
Rane, P., Kim, H., Marcano, J. L., and Gabbard, J. L. (2016). Virtual Road Signs. Proc. Hum. Factors Ergon. Soc. Annu. Meet. 60, 1750–1754. doi:10.1177/1541931213601401
Riegler, A., Wintersberger, P., Riener, A., and Holzmann, C. (2018). “Investigating User Preferences for Windshield Displays in Automated Vehicles,” in Proceedings of the 7th ACM International Symposium on Pervasive Displays, Munich, Germany (New York, USA: ACM Press), 1–7. doi:10.1145/3205873.3205885
Riegler, A., Riener, A., and Holzmann, C. (2019a). “Adaptive Dark Mode: Investigating Text and Transparency of Windshield Display Content for Automated Driving,” in Mensch und Computer 2019-Workshop Band, Hamburg, Germany.
Riegler, A., Riener, A., and Holzmann, C. (2019b). “AutoWSD,” in Mensch und Computer 2019, Hamburg, Germany (New York, NY, USA: ACM). doi:10.1145/3340764.3345366
Riegler, A., Riener, A., and Holzmann, C. (2019c). “Towards Dynamic Positioning of Text Content on a Windshield Display for Automated Driving,” in 25th ACM Symposium on Virtual Reality Software and Technology-VRST ’19, Parramatta, NSW (New York, NY, USA: Association for Computing Machinery). doi:10.1145/3359996.3364757
Riegler, A., Riener, A., and Holzmann, C. (2019d). “Virtual Reality Driving Simulator for User Studies on Automated Driving,” in Proceedings of the 11th International Conference on Automotive User Interfaces and Interactive Vehicular Applications: Adjunct Proceedings-AutomotiveUI ’19, Utrecht, Netherlands (New York, NY, USA: Association for Computing Machinery), 502–507. doi:10.1145/3349263.3349595
Riegler, A., Wintersberger, P., Riener, A., and Holzmann, C. (2019e). Augmented Reality Windshield Displays and Their Potential to Enhance User Experience in Automated Driving. I-COM 18, 127–149. doi:10.1515/icom-2018-0033
Riegler, A., Aksoy, B., Riener, A., and Holzmann, C. (2020a). “Gaze-based Interaction with Windshield Displays for Automated Driving: Impact of Dwell Time and Feedback Design on Task Performance and Subjective Workload,” in 12th International Conference on Automotive User Interfaces and Interactive Vehicular Applications, AutomotiveUI ’20, Virtual Event, DC, 151–160. doi:10.1145/3409120.3410654
Riegler, A., Riener, A., and Holzmann, C. (2020b). “A Research Agenda for Mixed Reality in Automated Vehicles,” in 19th International Conference on Mobile and Ubiquitous Multimedia, MUM 2020, Essen, Germany, 119–131. doi:10.1145/3428361.3428390
Riegler, A., Weigl, K., Riener, A., and Holzmann, C. (2020c). “StickyWSD: Investigating Content Positioning on a Windshield Display for Automated Driving,” in 19th International Conference on Mobile and Ubiquitous Multimedia, MUM 2020, Essen, Germany, 143–151. doi:10.1145/3428361.3428405
Riegler, A., Riener, A., and Holzmann, C. (2021a). A Systematic Review of Augmented Reality Applications for Automated Driving: 2009–2020. PRESENCE: Virtual Augmented Reality 28, 1–80. doi:10.1162/pres_a_00343
Riegler, A., Riener, A., and Holzmann, C. (2021b). A Systematic Review of Virtual Reality Applications for Automated Driving: 2009–2020. Front. Hum. Dyn. 48. doi:10.3389/fhumd.2021.689856
Riener, A., Boll, S., and Kun, A. L. (2016). Automotive User Interfaces in the Age of Automation (Dagstuhl Seminar 16262). Dagstuhl Rep. 6, 111–159. doi:10.4230/DagRep.6.6.111
Riener, A., Jeon, M., Alvarez, I., and Frison, A. K. (2017). “Driver in the Loop: Best Practices in Automotive Sensing and Feedback Mechanisms,” in Automotive User Interfaces (Springer), 295–323. doi:10.1007/978-3-319-49448-7_11
SAE On-Road Automated Vehicle Standards Committee (2021). Taxonomy and Definitions for Terms Related to On-Road Motor Vehicle Automated Driving Systems. Technical Report. [Dataset].
Schartmüller, C., Riener, A., Wintersberger, P., and Frison, A.-K. (2018). “Workaholistic,” in MobileHCI '18: Proceedings of the 20th International Conference on Human-Computer Interaction with Mobile Devices and Services, Barcelona, Spain, 1–12. doi:10.1145/3229434.3229459
Schrepp, M., Hinderks, A., and Thomaschewski, J. (2017). Design and Evaluation of a Short Version of the User Experience Questionnaire (Ueq-s). IJIMAI 4, 103. doi:10.9781/ijimai.2017.09.001
Schroeter, R., and Steinberger, F. (2016). “Pokémon Drive: towards Increased Situational Awareness in Semi-automated Driving,” in Proceedings of the 28th Australian Conference on Computer-Human Interaction, Launceston, Tasmania, 25–29. doi:10.1145/3010915.3010973
Slater, M., Antley, A., Davison, A., Swapp, D., Guger, C., Barker, C., et al. (2006). A Virtual Reprise of the stanley Milgram Obedience Experiments. PloS one 1, e39. doi:10.1371/journal.pone.0000039
Smith, M., Streeter, J., Burnett, G., and Gabbard, J. L. (2015). “Visual Search Tasks,” in The 7th International Conference, Nottingham, UK (New York, USA: ACM Press), 80–87. doi:10.1145/2799250.2799291
Smith, M., Gabbard, J. L., and Conley, C. (2016). “Head-up vs. Head-Down Displays: Examining Traditional Methods of Display Assessment while Driving,” in Proceedings of the 8th International Conference on Automotive User Interfaces and Interactive Vehicular Applications, Ann Arbor, MI (ACM), 185–192.
Topliss, B. H., Pampel, S. M., Burnett, G., and Gabbard, J. L. (2019). “Evaluating Head-Up Displays across Windshield Locations,” in Proceedings of the 11th International Conference on Automotive User Interfaces and Interactive Vehicular Applications-AutomotiveUI ’19, Utrecht, Netherlands (New York, USA: Association for Computing Machinery), 244–253. doi:10.1145/3342197.3344524
Walch, M., Mühl, K., Kraus, J., Stoll, T., Baumann, M., and Weber, M. (2017). “From Car-Driver-Handovers to Cooperative Interfaces: Visions for Driver-Vehicle Interaction in Automated Driving,” in Automotive User Interfaces (Springer), 273–294. doi:10.1007/978-3-319-49448-7_10
Wiegand, G., Mai, C., Holländer, K., and Hussmann, H. (2019). “InCarAR: A Design Space towards 3D Augmented Reality Applications in Vehicles,” in AutomotiveUI '19: Proceedings of the 11th International Conference on Automotive User Interfaces and Interactive Vehicular Applications, Utrecht, Netherlands, 1–13. doi:10.1145/3342197.3344539
Wintersberger, P., Green, P., and Riener, A. (2017a). “Am I Driving or Are You or Are We Both? a Taxonomy for Handover and Handback in Automated Driving,” in 9th International Driving Symposium on Human Factors in Driver Assessment, Training and Vehicle Design, Manchester, VT.
Wintersberger, P., von Sawitzky, T., Frison, A.-K., and Riener, A. (2017b). “Traffic Augmentation as a Means to Increase Trust in Automated Driving Systems,” in The 12th Biannual Conference, Cagliari, Italy (New York, USA: ACM Press), 1–7. doi:10.1145/3125571.3125600
Wintersberger, P., Riener, A., Schartmüller, C., Frison, A.-K., and Weigl, K. (2018). “Let Me Finish before I Take over,” in Proceedings of the 10th International Conference on Automotive User Interfaces and Interactive Vehicular Applications-AutomotiveUI ’18, Toronto, ON (New York, NY, USA: ACM), 53–65. doi:10.1145/3239060.3239085
Wintersberger, P., Schartmüller, C., and Riener, A. (2019). Attentive User Interfaces to Improve Multitasking and Take-Over Performance in Automated Driving. Int. J. Mobile Hum. Comput. Interaction 11, 40–58. doi:10.4018/ijmhci.2019070103
Yontem, A. O., Li, K., Chu, D., Meijering, V., and Skrypchuk, L. (2020). Prospective Immersive Human-Machine Interface for Future Vehicles: Multiple Zones Turn the Full Windscreen into a Head-Up Display. IEEE Vehicular Techn. Mag. 16, 83–92. doi:10.1109/MVT.2020.3013832
Yun, H., and Yang, J. H. (2020). Multimodal Warning Design for Take-Over Request in Conditionally Automated Driving. Eur. Transport Res. Rev. 12, 1–11. doi:10.1186/s12544-020-00427-5
Keywords: augmented reality, windshield display, automated driving, user study, user preferences
Citation: Riegler A, Riener A and Holzmann C (2022) Towards Personalized 3D Augmented Reality Windshield Displays in the Context of Automated Driving. Front. Future Transp. 3:810698. doi: 10.3389/ffutr.2022.810698
Received: 07 November 2021; Accepted: 06 January 2022;
Published: 03 February 2022.
Edited by:
Andry Rakotonirainy, Queensland University of Technology, AustraliaReviewed by:
Peter Burns, Transport Canada, CanadaAlexandra Kondyli, University of Kansas, United States
Copyright © 2022 Riegler, Riener and Holzmann. This is an open-access article distributed under the terms of the Creative Commons Attribution License (CC BY). The use, distribution or reproduction in other forums is permitted, provided the original author(s) and the copyright owner(s) are credited and that the original publication in this journal is cited, in accordance with accepted academic practice. No use, distribution or reproduction is permitted which does not comply with these terms.
*Correspondence: Andreas Riegler, andreas.riegler@fh-hagenberg.at