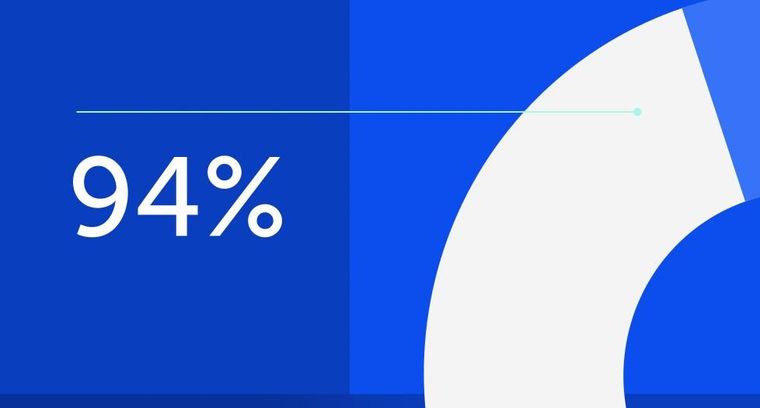
94% of researchers rate our articles as excellent or good
Learn more about the work of our research integrity team to safeguard the quality of each article we publish.
Find out more
ORIGINAL RESEARCH article
Front. For. Glob. Change, 12 February 2025
Sec. Temperate and Boreal Forests
Volume 8 - 2025 | https://doi.org/10.3389/ffgc.2025.1561027
Introduction: The European chestnut (Castanea sativa Mill.) is a historically and ecologically important tree in the Iberian Peninsula. The species now faces challenges related to the abandonment of traditional management practices, and climate change, although the potential impact of the later on chestnut potential range remains unexplored.
Methods: We assessed variations in chestnut potential range under different climate change scenarios in the Iberian Peninsula, by studying the current and projected habitat suitability of the species across distinct biogeographical regions (Atlantic and Mediterranean) and Regions of Provenance (RoPs). Environmental static (topography and soil parameters) and dynamic (bioclimatic variables) factors were used to build Species Distribution Models (SDMs). Future habitat suitability projections were based on an ensemble of five global circulation models (GCMs) for two climate change scenarios included in CMIP6. The SDMs were constructed using the Random Forest algorithm.
Results and Discussion: Our model achieved an accuracy of 86.82%, with high sensitivity (89.91%) and specificity (83.73%). Favourable chestnut habitats were linked to wetter regions, and included factors associated with annual and seasonal precipitations, coldest quarter temperature, soil pH and annual mean temperature. Optimal conditions for chestnut trees include precipitation exceeding 800 mm/year and mean temperature ranging from 10-15°C. Future projections suggest a potential habitat loss for chestnut and slight changes in net primary productivity. The Regions of Provenance exhibit varying levels of resilience, with the Mediterranean regions being particularly vulnerable. We highlight the need to develop mitigation strategies to facilitate chestnut resilience in the face of threats related to climate change.
The European chestnut (Castanea sativa Mill.) is native to the Iberian Peninsula. After the artificial expansion of the species driven by the Greeks and Romans, chestnut cultivation became a common practice throughout the Middle Ages (Conedera et al., 2004; Pereira-Lorenzo et al., 2011; Pereira-Lorenzo et al., 2019), resulting in the species being distributed across extensive areas and subjected to traditional management practices, such as the establishment of grafted trees in orchards (Conedera et al., 2001; Beccaro et al., 2020a; Beccaro et al., 2020b). Hence, past intensive management for agroforestry purposes has led to the current distribution of chestnuts, masking the species’ natural range and autecological preferences (Conedera et al., 2021).
Chestnut trees possess a multifaceted role in biodiversity conservation beyond their productivity potential (Gondard et al., 2006; Guitián et al., 2012). Within chestnut orchards, the trees serve as microhabitats (Kraus et al., 2016) due to their structural characteristics, which include cavities, cracks, water-filled holes, and microsoils. These features host a diverse array of species ranging from fungi and epiphytic plants to insects, birds, and small mammals (Rubio, 2009; Zlatanov et al., 2013). Additionally, chestnut fruits serve as an essential dietary component for various animal species, including the brown bear (Ursus arctos L.; Pérez-Girón et al., 2022), which is threatened in the Iberian Peninsula and constitute an umbrella species for conservation (Gonzalez et al., 2016). When effectively managed (Moretti et al., 2021), chestnut orchards represent significant assets for nature conservation within landscape mosaics (Diaz-Varela et al., 2018).
The European chestnut (or sweet chestnut) is a thermophilic species, and the optimal conditions for growth, phenology and ecological well-being occur in regions where the annual mean temperature ranges from 8°C to 15°C, accompanied by annual rainfall ranging from 600 to 700 mm to 1,500–1,600 mm (Menéndez-Miguélez et al., 2015; Conedera et al., 2016; Pérez-Girón et al., 2020; Freitas et al., 2021). Chestnuts also tolerate maximum temperatures of between 27 and 31°C. However, the species exhibits heightened sensitivity to summer drought and requires moderate chilling accumulation (Conedera et al., 2004; Santos et al., 2019). The growth of chestnuts is closely linked to temperature, precipitation and other climatic factors. As a result, ecosystems relying on this species are vulnerable to climate change (Castellana et al., 2021; Freitas et al., 2022).
The Intergovernmental Science-Policy Platform on Biodiversity and Ecosystem Services (IPBES, 2019) has identified climate change as a primary driver of ecological threats to forest ecosystems, with particular focus on vulnerable areas such as the Mediterranean region, including the Iberian Peninsula (Purvis et al., 2019; European Commission, 2022; Xunta de Galicia, 2022). In this regard, projections for the Mediterranean region, anticipate temperature increases and substantial decreases in precipitation, especially during summer (Giorgi, 2006; Giorgi and Lionello, 2008; Ozturk et al., 2015; IPCC, 2021); leading to more frequent and intense droughts (Böhnisch et al., 2021; García-Valdecasas Ojeda et al., 2021; Soares et al., 2023). Shifts in precipitation patterns (de Luis et al., 2010; Lorenzo and Alvarez, 2020) and an increased frequency of extreme weather events (Mohammed et al., 2018; Cardoso Pereira et al., 2020; Barbosa and Scotto, 2022) are also expected to occur.
The expected potential consequences of climate change, including alterations in the metabolic processes of chestnuts, like phenological changes (Alhinho et al., 2021; Larue et al., 2021; Conedera et al., 2021), physiological stress (Alcaide et al., 2019; Camisón et al., 2020; Pérez-Girón et al., 2020; Camisón et al., 2021; Castellana et al., 2021), shifts in pest and disease dynamics (Bale et al., 2002; Simler-Williamson et al., 2019; Gil-Tapetado et al., 2021; Lombardero et al., 2021; Dorado et al., 2023), impacts on genetic diversity (Pereira-Lorenzo et al., 2011; Míguez-Soto et al., 2019), as well as shifts in environmental variables such as soil composition and water availability, are likely to pose threats to the health, productivity and distribution of chestnuts (Pérez-Girón et al., 2020; Menéndez-Miguélez et al., 2023), with consequences to current chestnut-related ecosystems and of their capacity to provide goods and services (Freitas et al., 2022; Fernandes et al., 2022; Castellana et al., 2021).
Since various climate-related variables can influence on chestnut sensitivity, it is critical to assess the potential impact of climate change on the species, in order to delineate effective and adaptive strategies to maintain the growth, productivity and long-term sustainability of chestnut. In this regard, the application of short- and medium-term adaptation actions may be important to mitigate potential impacts of climate change, such as efficient waterlogging or irrigation management to maintain adequate soil moisture levels (especially in Mediterranean climates; see Mota et al., 2018a; Mota et al., 2018b); the use of protective compounds including symbiotic fungi to improve nutrient uptake, enhance tolerance to drought and contribute to overall plant health, thereby aiding in the defence against environmental challenges (Aryal, 2017; Begum et al., 2019); the use of silicon fertilisation for prophylaxis to prevent the impact of abiotic stress (Carneiro-Carvalho et al., 2023); and the genetic selection of clones with enhanced adaptability and resilience (Fernández-López et al., 2005; Pereira-Lorenzo et al., 2011; Míguez-Soto et al., 2019; Castellana et al., 2021). Better understanding of the potential impacts of climate change on habitat suitability for chestnuts will help to identify the most appropriate actions across space, considering future potential climate scenarios. In this study, we assessed the potential impacts of climate change on chestnut potential range across the Iberian Peninsula, considering both current and projected habitat suitability. We also conducted a comprehensive analysis of the potential impacts of climate change across established chestnut ecological regions (areas where species thrive under uniform conditions).
The study was conducted in mainland Iberian Peninsula (Figure 1), a region characterised by substantial climatic heterogeneity. The climate can be broadly categorised into three main zones: dry climate zones, which dominate the southern and southeastern areas; temperate zones with dry and hot summers, covering approximately 40% of the Peninsula; and temperate zones with dry and mild summers, prevalent in the northeastern regions and along most of the western coast of mainland Portugal (AEMET, 2011). To address the climatic heterogeneity of the area and facilitate specific comparisons and analyses, two types of territorial divisions were used: the biogeographical the Atlantic and Mediterranean regions (where chestnut are present); and the Regions of Provenance (RoPs), regarded as ecologically homogeneous areas for the species, which were used as reference elements.
Figure 1. (A) Map of the Biogeographical Regions in Europe from 2016 onwards (source: European Environment Agency) and (B) Regions of Provenance (RoP) with presence of sweet chestnut. Source: (Instituto da Conservação da Natureza e Florestas (ICNF), 2018; Alía Miranda et al., 2009).
Regions of Provenance (RoPs) are specific areas delineated on the basis of uniform ecological conditions, where stands or seed sources with similar phenotypic or genetic traits are found. RoPs are defined in European Directive EC/1999/105, and they are delineated by EU countries according to their own criteria. We used two types of classification in the present study. For Portugal, we used the RoPs defined for C. sativa by the Instituto da Conservação da Natureza e das Florestas (Instituto da Conservação da Natureza e Florestas (ICNF), 2018). For Spain, we used the RoP method developed by Alía Miranda et al. (2009), which divides the territory into ecologically analogous zones independent of species, as a cumulative RoP system for C. sativa has not yet been developed.
We compiled an extensive database of georeferenced occurrences of sweet chestnut. This compilation draws from data collected in the 3rd and 4th National Forest Inventories in Spain (NFI3 and NFI4), the 6th National Forest Inventory in Portugal (NFI6), the Continuous Forest Inventory of Galicia (IFCG), and the Global Biodiversity Information Facility (GBIF) (GBIF, 2024; MITECO, 2022; Xunta de, 2022; ICNF, 2015). Firstly, we considered only those occurrences with a coordinate accuracy uncertainty <25 m. Then, we eliminated occurrences within artificial surfaces (e.g., urban, industrial, etc.…) by cross-referencing the occurrences with CORINE land cover 2018. In addition, as NFIs follow a systematic statistical sampling system, NFI plots without sweet chestnut were considered as true absences. Finally, to reduce possible spatial bias arising from the use of different data sources, we used random spatial filtering, implemented in the ecoinfo package (Carlisle and Albeke, 2016) in the R 4.3.1 software (R Core Team, 2023). Specifically, we restricted our analysis to occurrences separated from each other by >200 m, while a minimum distance of 1,000 m was selected for absences to match NFI sampling intensity. As a result, we used 4,505 occurrences and 56,498 absences for subsequent analyses. Of the total occurrences, 3,128 georeferenced occurrences were located in the Atlantic biogeographical region, 1,359 in the Mediterranean and 18 in the Alpine region. The latter, due to the small number of samples, has been excluded from the biogeographical regions analysis.
First, we selected a set of environmental variables that are assumed will remain static under the influence of climate change on a short- medium- term, and reflected chestnut habitat preferences. Related to topography, we computed slope and aspect with the 30 m European Digital Elevation Model (EU-DEM). Aspect was further divided into Northness (cosine of aspect) and Eastness (sine of aspect) to mitigate duplication of values for north (0° and 360°). For soil-related parameters, we included soil pH and soil organic carbon content (SOC) within the 5–15 cm layer, sourced from SoilGrids250m version 2.0 (Poggio et al., 2021). On the other hand, we used a set of dynamic bioclimatic variables from the CHELSA V.2.1 dataset, computed as the average for the years 1981–2010, and at a spatial resolution of 30 arc-seconds (approximately 1 km) (Karger et al., 2017). We omitted bioclimatic variables 8, 9, 18, and 19 from the analysis owing to recognised issues of spatial discontinuity (Booth, 2022; see Supplementary Table S1). Finally, to remove multicollinearity we excluded those variables with a Spearman’s pairwise correlation coefficient > |0.7| or a Variance Inflation Factor (VIF) > 5. However, to exclusively assess the importance of predictors in the Atlantic and Mediterranean biogeographical regions, all predictors were considered for modelling without exclusions.
We assumed that estimates of chestnut habitat suitability would reflect the potential range of the species. To approach habitat suitability in the future, we used the bioclimatic variables associated with several climate projections based on the Coupled Model Intercomparison Project Phase 6 (CMIP6) provided by CHELSA V2.1 (Karger et al., 2017). These projections follow the guidelines of the Intersectoral Impact Model Intercomparison Project (ISIMIP) regarding selection of Global Circulation Models (GCMs) and Shared Socioeconomic Pathway scenarios (SSPs). Specifically, we considered five high-performance General Circulation Models (GCMs) (GFDL-ESM4, IPSL-CM6A-LR, MPI-ESM1-2-HR, MRI-ESM2-0, and UKESM1-0-LL) and two Shared Socioeconomic Pathways (SSPs) (SSP3-7.0 and SSP5-8.5) for three distinct periods: 2011–2040, 2041–2070 and 2071–2100 (Lange, 2021). In contrast to the previous Representative Concentration Pathways (RCP4.5 and RCP8.5), they represent possible alternative future scenarios shaped by various political and socio-economic, thus providing a more contemporary basis for assessing the impacts of climate change. SSP3-7.0 portrays a scenario characterised by limited proactive measures to mitigate climate change, resulting in a steady temperature increase of approximately 3.6°C and a doubling of CO2 emissions from current levels by 2,100. Conversely, the SSP5-8.5 scenario presents the most pessimistic outlook, projecting a temperature increase of up to 5°C or more above pre-industrial levels. However, this scenario is often mistakenly perceived as “business as usual” despite being deemed an improbable, high-risk future. Consequently, the focus shifts to the second-highest scenario, SSP3-7.0, emphasising its heightened significance in climate change research. Additionally, to assess variations in primary productivity between the current and potential future ranges, we obtained the projected Net Primary Productivity (NPP) for each GCM, SSP and time period from BIOCLIM+ (Brun et al., 2022). BIOCLIM+ uses the Miami model (Lieth, 1975) and provides a simple but widely applied, empirically derived model that includes valuable information for management considerations.
Both environmental variables as climate change variables were cropped to fit the study area, reprojected to GRS84 (EPSG: 4326) and resampled to a 100 m resolution by bilinear interpolation. These processes were conducted using the terra package (Hijmans et al., 2022) in R 4.3.1 software.
A Random Forest (RF) classifier algorithm was used to develop Species Distribution Models (SDMs) to predict current and future habitat suitability for chestnuts in the Iberian Peninsula. The RF classification and non-parametric regression methodology, proposed by Breiman (2001), includes numerous individual decision trees that work as an ensemble, known as a “forest.” It uses the bagging method (Breiman, 1996), which implies that a random sample is selected with replacement, and therefore the individual sample may be chosen more than once. A class imbalance can therefore lead to the predictive accuracy of the minority class being much lower than that of the majority class. To deal with class-imbalance problems, we used down-sampling technique, in which each tree was trained with a subset of the majority class (absences) equal to minority class (presences) (Liu et al., 2009). This method has proven to be the best when used as the only modelling method (Valavi et al., 2022). Furthermore, RF uses a random subset of predictor variables, controlled by the mtry parameter (Ho, 1998), leading to a randomised and uncorrelated forest. These characteristics lead to the method being less sensitive to parameter tuning (Strobl et al., 2009). Consequently, the presence/absence data were split into two data sets, with 80% allocated for training and 20% for validation purposes. The RF model was trained on a balanced sample achieved through down-sampling, with an mtry value computed as the square root of the number of predictors and including 1,000 trees. The predictive performance of RF models was assessed with the validation data set through sensitivity and specificity (i.e., the numbers of correctly classified presences and absences, respectively), obtained with the confusion matrix.
Spatial predictions were returned in terms of the probability of the target pixel and then converted into binary outcomes using a conservative threshold of 0.4 at a spatial resolution of 100 m. For future projections, a spatial prediction for each GCM, climate change scenario and time interval was generated. Finally, an ensemble of models was generated using both mean and median values for each scenario and time interval and binarized using the above-mentioned threshold. Binary maps were used to assess significant differences in elevation and productivity (NPP) in future scenarios by applying the Wilcoxon-Mann–Whitney test (at α = 0.01) and using the mean of the current scenario as the reference for comparison.
Net Primary Production (NPP), commonly used to evaluate the responses of the terrestrial carbon cycle to climate change, depends on climatic variables (Lieth, 1975). Previous studies by Pérez-Girón et al. (2020) show a negative correlation between NPP and mean temperature variables (MAT, MATmin and MATmax) and a positive correlation between NPP and latitude, while no correlation was found with mean annual precipitation (MAP).
To assess changes in the spatial distribution of environmentally suitable habitats for chestnut under current and future climatic conditions, scenarios and temporal intervals, we used the biomod2 package (Thuiller et al., 2023) to compute the species stability, gains, losses and percent change, the latter of which was calculated as the difference between gains and losses. In order to map the changes on the chestnut distribution, we categorised the species change as stable (change equal to zero), slight (values from (−)1 to (−)24), moderate (values ranging from (−)25 to (−)49) and extreme (values equal to or greater than (−)50).
The key precipitation and temperature variables were chosen to compute significant habitat associations by comparing the occurrences of sweet chestnut within a habitat against a randomly distributed set of points. The comparison was implemented with the shar package (Hesselbarth, 2021) and a significance level of 0.01 was applied. These bioclimatic factors are essential for understanding the dynamic nature of chestnut habitats across the Iberian Peninsula, influencing critical ecological aspects such as vegetation composition, habitat structure and species diversity. Temperature breaks were established every 2.5°C, and 300 mm steps were chosen for precipitation. The resulting positive species-habitat associations were used as a reference framework for future predictions within RoPs.
Within the framework of the source regions, changes in habitat suitability for chestnut were analysed on the basis of future projections. These analyses quantified the percentage differentials between gains and losses for RoPs with a minimum land area of 100 hectares for representation, encompassing a total of 33 distinct regions of origin across the Iberian Peninsula, of which 28 regions are in Spain and 5 in Portugal.
Our RF model demonstrated strong sensitivity (88.86%) and specificity (83.52%), indicating a remarkable capacity to correctly identify presence and absence, respectively. The overall accuracy of the model was 86.19%. The estimated suitable habitat for chestnut currently spans a total area of 69,650 km2 (see Figure 2A), with an optimal distribution observed in the wetter regions influenced by oceanic climates. Mean annual precipitation (Bio12), soil pH, which affects nutrient availability, particularly during critical times when moisture is essential, and annual mean temperature (Bio1) were identified as the most important factor for chestnut growth (Figure 2B). Other important factors such as SOC provides valuable information about soil fertility levels.
Figure 2. Current spatial predictions of habitat suitability for sweet chestnut, expressed as probability (A) and importance of variables (B).
When examining the overall importance of the different variables used concerning the Atlantic and Mediterranean biogeographical regions, notable differences emerged (Figure 3); highlighting the importance of precipitation as one of the most influential variables in habitat suitability and chestnut resilience, particularly in the Mediterranean region. In the Atlantic region, precipitation seasonality (Bio15) was the most important variable, which impacts on soil moisture availability, essential for tree growth. Other important factors were the mean temperature of coldest quarter (Bio11), indicating winter severity and affecting chestnut physiology and survival, isothermality (Bio3), the annual mean temperature (Bio1) (influencing temperature stability and growth conditions), and soil pH, which impact on nutrient availability. By contrast, in the Mediterranean region, annual (Bio12) and wettest quarter (Bio16) precipitation, critical for soil moisture during growth periods, were the most important variables affecting chestnut habitat suitability. Followed by, the precipitation of wettest month (Bio13), essential for chestnut development, temperature conditions (Bio1), and soil pH, which influences nutrient availability, vital for chestnut ecosystem health.
Figure 3. Overall importance of variables in (A) Atlantic and (B) Mediterranean Biogeographical Regions. The importance of variables in the Atlantic and Mediterranean biogeographical regions was assessed exclusively through a RF model that included all predictors without exclusions, and therefore, different from the RF used to predict distributions.
The estimated habitat suitable for chestnut varies significantly across scenarios and time periods according to the median ensemble (Figure 4 and Table 1, see Supplementary Figure S1 for the corresponding maps of mean ensemble). In scenarios SSP3-7.0 and SSP5-8.5, habitat loss increases over time. Between 2011 and 2070, we estimated a substantial and progressive increase in habitat loss, with the percentage of loss exceeding gains. This trend further intensifies between 2071 and 2100, with a significant decrease in stable habitat and a considerable increase in habitat loss. Estimates based on the SSP5-8.5 scenario showed a more pronounced habitat loss than SSP3-7.0 (Table 1), particularly towards the end of the century. Remarkably, while habitat gain is evident in all scenarios and time periods, it is outweighed by the magnitude of habitat loss, resulting in overall negative range changes.
Figure 4. Chestnut habitat suitability for (A) 2011-2040, (C) 2041-2070, and (E) 2071-2100 under the SSP3-7.0 scenario, and for (B) 2011-2040, (D) 2041-2070, and (F) 2071-2100 under the SSP5-8.5 scenario, as determined using a median ensemble approach.
Table 1. Habitat change for sweet chestnut for each scenario and time periods regarding median ensemble.
The projected scenarios also suggest potential changes in the elevational range inhabited by the species and in the productive capacity (NPP) (Figure 5). The median elevation of pixels with suitable habitat undergoes fluctuations across all future scenarios. In the short term, we forecast a median increase of approximately 60 m a.s.l., increasing to over 200 m a.s.l. in the long term. Chestnut demonstrates an ability to colonise higher elevations as conditions shift (Freitas et al., 2021), suggesting the involvement of some elevational-related stress tolerance in its adaptation. Similarly, the median NPP is predicted to undergo a slight but statistically significant increase, particularly between 2041 and 2070 onwards, as might be expected due to the increase in temperatures. The predicted increase is asymmetric, as it is more pronounced at relatively low NPP values.
Figure 5. Distribution and mean comparison in current and future predicted areas of (A) elevation and (B) NPP. Statistical significance: ns: p > 0.05; *p ≤ 0.05; **p ≤ 0.01; ***p ≤ 0.001; ****p ≤ 0.0001. Black dots represent outliers.
The Cantabrian region RoPs (2, 3, 4, 5, 6, and 7) showed resilience and adaptability to changing climatic conditions (facilitated by elevational shifts in mountainous areas: regions 4, 5, and 7). Conversely, RoPs in Mediterranean regions are expected to become more vulnerable, leading to a significant reduction in habitat suitability. This vulnerability is particularly pronounced in RoPs 11, 20, 24, 25, 28, 29, 30, 38, 39, 40, 42, and 46, as well as in the Portuguese RoPs CAS-RP2, CAS-RPA and CAS-RPC. Habitat loss is expected to be particularly high in the CAS-RP2 RoP in Portugal (Figure 6).
Figure 6. Chestnut habitat change in the RoPs for (A) 2011-2040, (C) 2041-2070, and (E) 2071-2100 under the SSP3-7.0 scenario, and for (B) 2011-2040, (D) 2041-2070, and (F) 2071-2100 under the SSP5-8.5 scenario.
Analysing the influence of the two most influential precipitation and temperature variables, annual precipitation (Bio12) and annual mean temperature (Bio1), the presence of chestnut is positively associated with locations receiving precipitation (Bio12) above 800 mm/year (Figure 7). Currently, almost all RoPs with chestnut presence meet these conditions, explaining the presence of chestnut in regions characterised by a pronounced Mediterranean climate, with very dry summers. In relation to the annual mean temperature, we observed a positive association between chestnut presence and mean temperatures ranging from 10 to 15°C (Figure 8). Considering this temperature range as optimal for chestnut, two RoPs, 42 and 46, currently exceed this temperature range. The challenge for chestnut in these RoPs escalates further in the analysed climate change scenarios, SSP3-7.0 and SSP5-8.5, intensifying throughout the periods 2011–2040, 2041–2070 and 2071–2100. Overall, under the projected scenarios, the influence of annual precipitation (Bio12) is less constraining for the species than the annual mean temperature (Bio1). However, during the period 2071 to 2100, annual precipitation is anticipated to fall below acceptable thresholds for the species. This is particularly notable in RoPs 9, 18, 19, 42, 46 and CAS-RP2.
Figure 7. Density function of mean annual precipitation (Bio12) under current environmental conditions and projected future climate change scenarios across RoPs. The red line indicates the threshold above which the presence of the species shows a positive association with mean annual precipitation.
Figure 8. Density function of annual mean temperature (Bio1) under current environmental conditions and projected future climate change scenarios across RoPs. The blue and red lines indicate the thresholds between which there is a positive association between species presence and annual mean temperature.
Genetic diversity, soil health and the capacity to adapt to local climatic conditions have been identified as key factors enabling chestnut to withstand climate challenges (Fernandes et al., 2022). Previous studies suggested that chestnut habitat suitability, and the potential impact of climate change, exhibit a high degree of regional variability within the Iberian Peninsula (Pérez-Girón et al., 2020). Along these lines, our analyses contribute to a better understanding of the potential impacts of climate change on the species, and could enable development of effective adaptation strategies to promote the long-term sustainability of chestnut ecosystems. The widespread distribution of chestnuts, influenced by human activities over hundreds of years across various regions from southern Europe and North Africa to north-western Europe and Western Asia, has enabled the species to flourish at the edges of its ecological limits. This presents difficulties in precisely identifying its initial natural habitat (Conedera et al., 2016).
Chestnuts are thermophilic, but display a remarkable plasticity in response to varying precipitation levels (Bourgeois et al., 2004; Gandullo Gutiérrez et al., 2004; Zhang et al., 2011; Conedera et al., 2016; Freitas et al., 2021; Toujgani et al., 2024). This may illustrate the association of the species with areas affected by oceanic influence (see, for example, Castellana et al., 2021; Cedano Gıraldo and Mumcu Küçüker, 2024; Beridze et al., 2023), which plays an important role in determining suitable habitats for chestnuts, especially in the Mediterranean basin. However, chestnuts also thrive in continental zones with milder climates characterised by warm-temperate and rainy conditions, including France, Italy and Switzerland (Conedera et al., 2016). For instance, chestnuts thrive in the Swiss part of the Ticino river basin, which receives a mean annual precipitation of 1,700 mm and where a mean annual temperature of approximately 12°C is maintained (Krebs et al., 2012). Overall, therefore, available evidence suggest a remarkable capacity of chestnut to thrive across a diverse range of precipitation and temperature conditions, including the Iberian Peninsula (Figures 2, 3, 7, 8).
Our findings are consistent with those of a study conducted by Freitas et al. (2021) in Portugal, where optimal conditions for chestnut tree growth were identified by employing an aggregated Chestnut Suitability Index (CSI), which includes temperature and precipitation variables (growing degree days, annual mean temperature, number of summer days with a maximum temperature below 32°C, and annual accumulated precipitation). They are also highly consistent with the maximal habitat suitability estimations established by Conedera et al. (2016) for Europe. This alignment is particularly marked in the Cantabrian region, which includes Galicia, Asturias and Cantabria. However, there is a clear divergence from the aforementioned study along the Atlantic coast of Galicia and Portugal. By contrast, our projections differ from the optimal areas for chestnut development indicated by the model developed by Castellana et al. (2021) for 2020, where the optimal conditions for chestnut are situated in the central-northern region of Portugal and the Atlantic coastal area of Galicia. Precipitation has also been identified as a key factor influencing site productivity within chestnut plantations and coppice stands in north-western Spain (Atlantic Biogeographical Region), with implications for predicting chestnut growth and subsequent management decisions, especially in areas prioritising timber production (Álvarez-Álvarez et al., 2010; Menéndez-Miguélez et al., 2015).
The results obtained for the two reference scenarios for climate change (SSP3-7.0 and SSP5-8.5) consistently predict a shift in the habitat suitable for chestnut-related ecosystems towards higher elevations. This upward migration illustrates the sensitivity of chestnuts to future changes in climate, particularly water deficit. It is anticipated that humid areas will become restricted to higher elevations in the future, which would have an important impact on the geographical distribution of chestnut ecosystems. The current status of chestnut habitat in the Iberian Peninsula corresponds with approximately 50% of the chestnut habitat suitability distributed within elevations ranging mainly from 250 to 750 m a.s.l. (with some trees present at elevations of up to 1,800 m, exceptionally). However, in future scenarios the species range is expected to move upwards towards higher elevations (see Figures 2, 4, 5; Freitas et al., 2022). This suggests an elevation-related stress tolerance in its adaptation, as evidenced by the 60-m increase above sea level (m a.s.l.) in distribution predicted for the short term. Looking further ahead, a median rise of over 200 m a.s.l. is anticipated in the long term. However, these data must be interpreted with caution because this study does not model the species colonisation capacity in terms of functional traits. The observed increase in NPP values (Figure 5) is probably due to a large reduction in habitat suitability in the southern zones of the Mediterranean region, accentuated by climate scenarios in the latter periods of the century; where Mediterranean climatic conditions are expected to extend northwards into the Iberian Peninsula.
The adaptation of chestnuts to changing environmental conditions would entail complex challenges beyond alterations in the species habitat suitability. Water scarcity, coupled with other anticipated abiotic stressors such as flooding, reduced chilling hours and rainfall deficits, is poised to significantly diminish the production and quality of chestnuts and chestnut wood (Fernandes et al., 2022). This could lead to the abandonment of traditional orchards, thereby increasing the risk of wildfires (Freitas et al., 2021). The prevalence of prolonged drought would damage the integrity of the root systems, rendering them more susceptible to infections during subsequent periods of rainfall, conditions conducive to the development of pathogens (Turchetti and Maresi, 2008; Marzocchi et al., 2024), which may lead to the resurgence of ink disease throughout European chestnut forests (Prospero et al., 2023). Climatic factors also have a proven influence on the spread of pests, as described by Gil-Tapetado et al. (2021) and Lombardero et al. (2021) for Dryocosmus kuriphilus in NW Iberian Peninsula.
The findings of the analysis of the Regions of Provenance (RoPs) show a higher resilience and adaptability of RoPs within the Cantabrian zone to dynamic climatic conditions. By contrast, RoPs situated in Mediterranean sites are increasingly susceptible, leading to marked reductions in their distributional extents—as manifested by significant habitat suitability loss (Figure 6). Thus, under the RoPs delimitation, our findings illustrate the pivotal role of annual precipitation (Bio12) and annual mean temperature variable (Bio1) in shaping the distribution of chestnuts (Santos et al., 2019; Freitas et al., 2021; Cedano Gıraldo and Mumcu Küçüker, 2024). Specifically, locations that receive precipitation exceeding 800 mm/year are positively associated with chestnut presence, while values below this threshold are negatively correlated. Notably, almost all RoPs with chestnut presence are characterised by these favourable precipitation conditions. Furthermore, chestnut presence is closely linked to temperature. Consideration of the annual mean temperature variable (Bio1) revealed a favourable association with chestnut growth within the range 10 to 15°C, which is considered optimal for their development. However, certain specific regions currently exceed the maximum temperature threshold established for chestnut. Cedano Gıraldo and Mumcu Küçüker (2024), for the Black Sea region (Turkey), discussed ecological optima for chestnut and reported optimal temperature ranges of 8 to 15°C and precipitation levels between 600 and 1,600 mm, except in the Black Sea region, and values of annual mean precipitation (Bio12) exceeding 200 mm, which at first glance appears to be very low. Similarly, studies by Cuenca and Majada (2012), conducted in Spain, suggested optimal temperature ranges of 10 to 13°C and precipitation values above 850 mm. In the European Atlas of Forest Tree Species, Conedera et al. (2016) also reported optimal temperature ranges of between 8°C and 15°C and minimum rainfall ranging from 600 to 800 mm for the entire chestnut distribution area.
Our findings contribute to establish an ecological reference framework based on current data for the species and its region of origin in the Iberian Peninsula and provide valuable insights to guide conservation actions in the face of changing climate. These observations are also consistent with previous findings of Pérez-Girón et al. (2020), who evaluated the resilience of sweet chestnut Agroforestry Systems (AFS) under both the RCP2.6 and RCP8.5 scenarios through 2,100. These authors emphasised the need for strategic planning and management of chestnut habitats suited to the diverse RoPs to counteract the detrimental effects of climate change. The comprehensive examination of chestnut RoPs sheds light on the intricate effects of climate change on species distributions, emphasising the urgent need for coordinated regional and international efforts to address these challenges and protect chestnut biodiversity.
The results of this study confirm the preference of chestnut for wetter, oceanic climates and highlight the critical role of precipitation in determining habitat suitability and resilience, particularly in the Mediterranean region. Precipitation levels exceeding 800 mm/year and optimal mean temperatures ranging from 10 to 15°C are key determinants of chestnut habitat suitability. The European chestnut displays a high level of adaptability to varying elevation conditions and adaptive potential in response to productivity-related obstacles. Its stress tolerance and capacity for recovery may be key for its survival in dynamic climate scenarios. Future projections highlight significant potential habitat loss, alterations in elevation and shifts in NPP, with the Mediterranean region facing heightened vulnerability. Escalating temperatures could surpass chestnut tolerance thresholds and lead to alterations in the range of the species. Variations in resilience and vulnerability across different RoPs emphasise the need for strategic habitat management to conserve chestnut diversity amidst climate uncertainties.
Sustainable forest management and biodiversity conservation require understanding how climate change affects chestnut habitats. Climate projections offer valuable insights but must be critically evaluated for limitations and updated with emerging findings. For instance, consideration of the new perspectives on the potential collapse of the Atlantic meridional overturning circulation (AMOC) due to freshwater input (see, e.g., van Westen et al., 2024) could significantly alter the dynamics of the climate change process in the Iberian Peninsula and may necessitate re-evaluation of the effects on species and ecosystems. Despite that, this study aims to serve as a spatial reference point for future conservation and management strategies for the species, laying the groundwork for forthcoming research on chestnut-related socioecological systems.
Publicly available datasets were analysed in this study. This data can be found here: https://zenodo.org/records/14065833.
PÁ-Á: Conceptualization, Data curation, Formal analysis, Funding acquisition, Investigation, Methodology, Project administration, Resources, Software, Supervision, Validation, Visualization, Writing – original draft, Writing – review & editing. AA-A: Data curation, Formal analysis, Investigation, Writing – review & editing, Resources, Validation, Visualization. ED-V: Formal analysis, Investigation, Resources, Writing – review & editing, Methodology, Supervision, Validation, Visualization. JL-B: Formal analysis, Funding acquisition, Investigation, Methodology, Resources, Supervision, Validation, Visualization, Writing – review & editing. JP-G: Conceptualization, Data curation, Formal analysis, Funding acquisition, Investigation, Methodology, Project administration, Resources, Software, Supervision, Validation, Visualization, Writing – original draft, Writing – review & editing.
The author(s) declare financial support was received for the research, authorship, and/or publication of this article. This study was conducted within the framework of the LIFE19 NAT/ES/000913 “Bears with Future” project, funded by the European Union, and coordinated by the Brown Bear Foundation. This research received additional financial support from the Spanish Ministry for the Ecological Transition and the Demographic challenge of the Government of Spain.
We sincerely thank the Instituto da Conservação da Natureza e das Florestas (ICNF) in Portugal for their generous provision and authorization to use the data from the 6th National Forest Inventory (IFN) for our research project.
The authors declare that the research was conducted in the absence of any commercial or financial relationships that could be construed as a potential conflict of interest.
The author(s) declared that they were an editorial board member of Frontiers, at the time of submission. This had no impact on the peer review process and the final decision.
The authors declare that no Generative AI was used in the creation of this manuscript.
All claims expressed in this article are solely those of the authors and do not necessarily represent those of their affiliated organizations, or those of the publisher, the editors and the reviewers. Any product that may be evaluated in this article, or claim that may be made by its manufacturer, is not guaranteed or endorsed by the publisher.
The Supplementary material for this article can be found online at: https://www.frontiersin.org/articles/10.3389/ffgc.2025.1561027/full#supplementary-material
AEMET (2011). Atlas climático ibérico. Temperatura del aire y precipitación (1971–2000), Agencia Estatal de Meteorología, Ministerio de Medio Ambiente y Medio Rural y Marino. Catálogo de Publicaciones de la Administración General del Estado. Edita: Ministerio de Medio Ambiente, y Medio Rural y Marino, Agencia Estatal de Meteorología, Instituto de Meteorologia de Portuga.
Alcaide, F., Solla, A., Mattioni, C., Castellana, S., and Martín, M. Á. (2019). Adaptive diversity and drought tolerance in Castanea sativa assessed through EST-SSR genic markers. Forestry 92, 287–296. doi: 10.1093/forestry/cpz007
Alhinho, A. T., Ramos, M. J. N., Alves, S., Rocheta, M., Morais-Cecílio, L., Gomes-Laranjo, J., et al. (2021). The dynamics of flower development in Castanea sativa mill. Plan. Theory 10:1538. doi: 10.3390/plants10081538
Alía Miranda, R., García del Barrio, J. M., Miguel del Angel, J., De Martín Pérez, F., Iglesias Sauce, S., Mancha Nuñez, J. A., et al. (2009). Regiones de procedencia de especies forestales en España, MARM. Madrid: Organismo Autónomo Parques Nacionales.
Álvarez-Álvarez, P., Díaz-Varela, E., Cámara-Obregón, A., and Afif-Khouri, E. (2010). Relating growth and nutrition to site factors in young chestnut plantations established on agricultural and forest land in northern Spain. Agrofor. Syst. 79, 291–301. doi: 10.1007/s10457-010-9313-z
Aryal, P. (2017). Evaluation of Ecto-mycorrhizae as a determinant of chestnut growth and stress response. Ph.D thesis (3386). Charleston, IL: Eastern Illinois University.
Bale, J. S., Masters, G. J., Hodkinson, I. D., Awmack, C., Bezemer, T. M., Brown, V. K., et al. (2002). Herbivory in global climate change research: direct effects of rising temperature on insect herbivores. Glob. Chang. Biol. 8, 1–16. doi: 10.1046/j.1365-2486.2002.00451.x
Barbosa, S., and Scotto, M. G. (2022). Extreme heat events in the Iberia peninsula from extreme value mixture modeling of ERA5-land air temperature. Weather Clim. Extremes 36:100448. doi: 10.1016/j.wace.2022.100448
Beccaro, G., Bounous, G., Gomes-Laranjo, J., Warmund, M., and Casey, J. (2020b). “Orchard Management” in The chestnut handbook. Crop and Forest management. eds. G. Beccaro, A. Alma, G. Bounous, and J. Gomes-Laranjo (CRC Press). International Standard Book Number-13: 978–1–138-33402-1 (Hardback)
Beccaro, G., Bounous, G., and Tessa, G. (2020a). “European chestnut traditional and high-density orchards” in The chestnut handbook. Crop and Forest management. eds. G. Beccaro, A. Alma, G. Bounous, and J. Gomes-Laranjo (CRC Press). International Standard Book Number-13: 978-1-138-33402-1 (Hardback)
Begum, N., Qin, C., Ahanger, M. A., Raza, S., Khan, M. I., Ashraf, M., et al. (2019). Role of Arbuscular Mycorrhizal Fungi in plant growth regulation: implications in abiotic stress tolerance. Front. Plant Sci. 10:1068. doi: 10.3389/fpls.2019.01068
Beridze, B., Sękiewicz, K., Walas, Ł., Thomas, P. A., Danelia, I., Fazaliyev, V., et al. (2023). Biodiversity protection against anthropogenic climate change: conservation prioritization of Castanea sativa in the South Caucasus based on genetic and ecological metrics. Ecol. Evol. 13:e10068. doi: 10.1002/ece3.10068
Böhnisch, A., Mittermeier, M., Leduc, M., and Ludwig, R. (2021). Hot spots and climate trends of meteorological droughts in Europe–assessing the percent of Normal index in a single-model initial-condition large ensemble. Front. Water 3:716621. doi: 10.3389/frwa.2021.716621
Booth, T. H. (2022). Checking bioclimatic variables that combine temperature and precipitation data before their use in species distribution models. Austral Ecol. 47, 1506–1514. doi: 10.1111/aec.13234
Bourgeois, C., Sevrin, E., and Lemaire, J. (2004). Le Châtaignier, un Arbre, un Bois. 2nd Edn. Institut pour le développement forestier, Lavoisier Ed. 347.
Brun, P., Zimmermann, N. E., Hari, C., Pellisier, L., and Karger, D. N. (2022). Global climate-related predictors at kilometre resolution for the past and future earth system science data. Earth Syst. Sci. Data 14, 5573–5603. doi: 10.5194/essd-14-5573-2022
Camisón, Á., Ángela Martín, M., Dorado, F. J., Moreno, G., and Solla, A. (2020). Changes in carbohydrates induced by drought and waterlogging in Castanea sativa. Trees Struct. Funct. 34, 579–591. doi: 10.1007/s00468-019-01939-x
Camisón, Á., Martín, M. Á., Flors, V., Sánchez-Bel, P., Pinto, G., Vivas, M., et al. (2021). Exploring the use of scions and rootstocks from xeric areas to improve drought tolerance in Castanea sativa miller. Environ. Exp. Bot. 187:104467. doi: 10.1016/j.envexpbot.2021.104467
Cardoso Pereira, S., Marta-Almeida, M., Carvalho, A. C., and Rocha, A. (2020). Extreme precipitation events under climate change in the Iberian Peninsula. Int. J. Climatol. 40, 1255–1278. doi: 10.1002/joc.6269
Carlisle, J., and Albeke, S. (2016). Ecoinfo: assorted tools for the management and analysis of ecological information. R package version 0.9.3.
Carneiro-Carvalho, A., Pinto, T., Gomes-Laranjo, J., and Anjos, R. (2023). The potential of SiK® fertilization in the resilience of chestnut plants to drought - a biochemical study. Front. Plant Sci. 14:1120226. doi: 10.3389/fpls.2023.1120226
Castellana, S., Martin, M. Á., Solla, A., Alcaide, F., Villani, F., Cherubini, M., et al. (2021). Signatures of local adaptation to climate in natural populations of sweet chestnut (Castanea sativa mill.) from southern Europe. Ann. For. Sci. 78:27. doi: 10.1007/s13595-021-01027-6
Cedano Gıraldo, D., and Mumcu Küçüker, D. (2024). Assessing climate change impacts on the spatial distribution of Castanea sativa mill. Using ecological niche modeling. Anadolu Orman Araştırmaları Dergisi 9, 170–177. doi: 10.53516/ajfr.1374398
Conedera, M., Krebs, P., Gehring, E., Wunder, J., Hülsmann, L., Abegg, M., et al. (2021). How future-proof is sweet chestnut (Castanea sativa) in a global change context. For. Ecoly Manag. 494:119320. doi: 10.1016/j.foreco.2021.119320
Conedera, M., Krebs, P., Tinner, W., Pradella, M., and Torriani, D. (2004). The cultivation of Castanea sativa (mill.) in Europe, from its origin to its diffusion on a continental scale. Veg. Hist. Archaeobotany 13, 161–179. doi: 10.1007/s00334-004-0038-7
Conedera, M., Stanga, P., Oester, B., and Bachmann, P. (2001). Different post-culture dynamics in abandoned chestnut orchards and coppices. For Snow Landsc Res 492, 487–492.
Conedera, M., Tinner, W., Krebs, P., de Rigo, D., and Caudullo, G. (2016). “Castanea sativa in Europe: Distribution, habitat, usage and threats” in European atlas of Forest tree species. eds. J. San-Miguel-Ayanz, D. de Rigo, G. Caudullo, T. Houston Durrant, and A. Mauri (Luxembourg: Publ. Off. EU), e0125e0+.
Cuenca, B., and Majada, J. P. (2012). Castanea sativa Mill. In: Pemán García, J., Navarro Cerrillo, R. M., Nicolás Peragón, J. L., Prada Sáez, M. A., Serrada Hierro, R. (Eds.). Producción y manejo de semillas y plantas forestales (Vol. 1, pp. 274–309). Organismo Autónomo Parques Nacionales, Ministerio de Agricultura, Alimentación y Medio Ambiente. Madrid.
de Luis, M., Brunetti, M., Gonzalez-Hidalgo, J. C., Longares, L. A., and Martin-Vide, J. (2010). Changes in seasonal precipitation in the Iberian Peninsula during 1946–2005. Glob. Planet. Chang. 74, 27–33. doi: 10.1016/j.gloplacha.2010.06.006
Diaz-Varela, E. R., Álvarez Álvarez, P., Roces-Diaz, J. V., and Rodríguez-Morales, B. (2018). “The contribution of chestnut orchard recovery projects for effective area-based conservation: two cases in Asturias (North-West Spain). En UNU-IAS & IGES” in Satoyama initiative thematic review vol. 4. Sustainable use of biodiversity in socio-ecological production landscapes and seascapes (SEPLS) and its contribution to effective area-based conservation. Tokyo: United Nations University Institute for the Advanced Study of sustainability.
Dorado, F. J., Alías, J. C., Chaves, N., and Solla, A. (2023). Warming scenarios and Phytophthora cinnamomi infection in chestnut (Castanea sativa mill.). Plants 2023, 12, 556 12. doi: 10.3390/plants12030556
European Commission (2022). Consequences of climate change. Available at: https://ec.europa.eu/clima/climate-change/climate-change-consequences_en (Accessed April 21, 2024).
Fernandes, P., Colavolpe, M. B., Serrazina, S., and Costa, R. L. (2022). European and American chestnuts: an overview of the main threats and control efforts. Front. Plant Sci. 13:951844. doi: 10.3389/fpls.2022.951844
Fernández-López, J., Zas, R., Diaz, R., Villani, F., Cherubini, M., Aravanopoulos, F. A., et al. (2005). Geographic variability among extreme European wild chestnut populations. III International Chestnut Congress. ISHS Acta Horticulturae 693, 21–28. doi: 10.17660/ActaHortic.2005.693.21.
Freitas, T. R., Santos, J. A., Silva, A. P., and Fraga, H. (2021). Influence of climate change on chestnut trees: a review. Plan. Theory 10:1463. doi: 10.3390/plants10071463
Freitas, T. R., Santos, J. A., Silva, A. P., Martins, J., and Fraga, H. (2022). Climate change projections for bioclimatic distribution of Castanea sativa in Portugal. Agronomy 12:1137. doi: 10.3390/agronomy12051137
Gandullo Gutiérrez, J. M., Rubio, A., Sánchez Palomares, O., Blanco, A., Gómez Sanz, V., and Elena, R. (2004). Las estaciones ecológicas de los castañares españoles. Monografıas INIA: Serie Forestal.
García-Valdecasas Ojeda, M., Gámiz-Fortis, S. R., Romero-Jiménez, E., Rosa-Cánovas, J. J., Yeste, P., Castro-Díez, Y., et al. (2021). Projected changes in the Iberian Peninsula drought characteristics. Sci. Total Environ. 757:143702. doi: 10.1016/j.scitotenv.2020.143702
GBIF (2024). GBIF occurrence download. Accessed from R via rgbif: https://github.com/ropensci/rgbif
Gil-Tapetado, D., Castedo-Dorado, F., Lombardero, M. J., Martel, J., and Álvarez-Álvarez, P. (2021). Spatial propagation and patterns of abundance of Dryocosmus kuriphilus throughout an invaded region. J. Appl. Entomol. 145, 10–25. doi: 10.1111/jen.12836
Giorgi, F. (2006). Climate change hot-spots. Geophys. Res. Lett. 33:L08707. doi: 10.1029/2006GL025734
Giorgi, F., and Lionello, P. (2008). Climate change projections for the Mediterranean region. Glob. Planet. Chang. 63, 90–104. doi: 10.1016/j.gloplacha.2007.09.005
Gondard, H., Romane, F., Regina, I. S., and Leonardi, S. (2006). “Forest management and plant species diversity in chestnut stands of three Mediterranean areas” in Forest diversity and management. Topics in biodiversity and conservation. eds. D. L. Hawksworth and A. T. Bull, vol. 2 (Dordrecht: Springer).
Gonzalez, E. G., Blanco, J. C., Ballesteros, F., Alcaraz, L., Palomero, G., and Doadrio, I. (2016). Genetic and demographic recovery of an isolated population of brown bear Ursus arctos L., 1758. PeerJ 4:e1928. doi: 10.7717/peerj.1928
Guitián, J., Guitián, P., Magrach, A., Docampo, C., Domínguez, P., and Guitián, L. (2012). Effect of management and spatial characteristics on plant species richness of Castanea sativa mill. woodlots in the NW Iberian Peninsula. J. For. Res. 17, 98–104. doi: 10.1007/s10310-011-0261-x
Hesselbarth, M. H. K. (2021). Shar: an R package to analyze species-habitat associations using point pattern analysis. J. Open Source Softw. 6:3811. doi: 10.21105/joss.03811
Hijmans, R.J., Bivand, R., Forner, K., Ooms, J., Pebesma, E., and Sumner, M.D. (2022). Package ‘terra’. Available at: https://cran.r-project.org/web/packages/terra/index.html.@
Ho, T. K. (1998). The random subspace method for constructing decision forests. IEEE Trans. Pattern Anal. Mach. Intell. 20, 832–844. doi: 10.1109/34.709601
ICNF (2015). 6° Inventário Florestal Nacional. Available at: https://www.icnf.pt/florestas/flestudosdocumentosestatisticasindicadores.
Instituto da Conservação da Natureza e Florestas (ICNF) (2018). Limites de áreas das Regiões de Proveniência para 24/ou das principais espécies florestais. Metadatos. MFR–R.
IPBES (2019). Global assessment report on biodiversity and ecosystem services of the intergovernmental science-policy platform on biodiversity and ecosystem services (Bonn). Available at: https://ipbes.net/global-assessment-report-biodiversity-ecosystem-services.
IPCC (2021). Climate Change 2021: the physical science basis: working group I contribution to the sixth assessment report of the intergovernmental panel on climate change : Cambridge University Press.
Karger, D. N., Conrad, O., Böhner, J., Kawohl, T., Kreft, H., Soria-Auza, R. W., et al. (2017). Climatologies at high resolution for the earth land surface areas. Sci. Data 4:170122. doi: 10.1038/sdata.2017.122
Kraus, D., Bütler, R., Krumm, F., Lachat, T., Larrieu, L., Mergner, U., et al. (2016). Catalogue of tree microhabitats – Reference field list : Integrate+ Technical Paper, 16p.
Krebs, P., Koutsias, N., and Conedera, M. (2012). Modelling the eco-cultural niche of giant chestnut trees: new insights into land use history in southern Switzerland through distribution analysis of a living heritage. J. Hist. Geogr. 38, 372–386. doi: 10.1016/j.jhg.2012.01.018
Lange, S. (2021). ISIMIP3b bias adjustment fact sheet. Potsdam: Inter-Sectoral Impact Model Intercomparison Project.
Larue, C., Barreneche, T., and Petit, R. J. (2021). Efficient monitoring of phenology in chestnuts. Sci. Hortic. 281:109958. doi: 10.1016/j.scienta.2021.109958
Lieth, H. (1975). “Modeling the primary productivity of the world” in Primary productivity of the biosphere. Ecological studies. eds. H. Lieth and R. H. Whittaker, vol. 14 (Berlin, Heidelberg: Springer).
Liu, X.-Y., Wu, J., and Zhou, Z.-H. (2009). Exploratory Undersampling for class-imbalance learning. IEEE Trans. Syst. Man Cybern. B Cybern. 39, 539–550. doi: 10.1109/TSMCB.2008.2007853
Lombardero, M., Castedo-Dorado, F., and Ayres, M. (2021). Extreme climatic events affect populations of Asian chestnut gall wasps, Dryocosmus kuriphilus, but do not stop the spread. Agric. For. Entomol. 23, 473–488. doi: 10.1111/afe.12448
Lorenzo, M. N., and Alvarez, I. (2020). Climate change patterns in precipitation over Spain using CORDEX projections for 2021–2050. Sci. Total Environ. 723:138024. doi: 10.1016/j.scitotenv.2020.138024
Marzocchi, G., Maresi, G., Luchi, N., Pecori, F., Gionni, A., Longa, C. M. O., et al. (2024). 85 years counteracting an invasion: chestnut ecosystems and landscapes survival against ink disease. Biol. Invasions 26, 2049–2062. doi: 10.1007/s10530-024-03292-8
Menéndez-Miguélez, M., Álvarez-Álvarez, P., Majada, J., and Canga, E. (2015). Effects of soil nutrients and environmental factors on site productivity in Castanea sativa mill. Coppice stands in NW Spain. New For. 46, 217–233. doi: 10.1007/s11056-014-9456-2
Menéndez-Miguélez, M., Álvarez-Álvarez, P., Pardos, M., Madrigal, G., Ruiz-Peinado, R., López-Senespleda, E., et al. (2023). Development of tools to estimate the contribution of young sweet chestnut plantations to climate-change mitigation. For. Ecol. Manag. 530:120761. doi: 10.1016/j.foreco.2022.120761
Míguez-Soto, B., Fernández-Cruz, J., and Fernández-López, J. (2019). Mediterranean and northern Iberian gene pools of wild Castanea sativa mill. Are two differentiated ecotypes originated under natural divergent selection. PLoS One 14:e0211315. doi: 10.1371/journal.pone.0211315
MITECO (2022). Cuarto Inventario Forestal Nacional. Available at: https://www.miteco.gob.es/es/biodiversidad/temas/inventarios-nacionales/inventario-forestal-nacional/cuarto_inventario.aspx.
Mohammed, A. J., Alarcón, M., and Pino, D. (2018). Extreme temperature events on the Iberian Peninsula: statistical trajectory analysis and synoptic patterns. Int. J. Climatol. 38, 5305–5322. doi: 10.1002/joc.5733
Moretti, M., Moretti, G., and Conedera, M. (2021). Le selve castanili della Svizzera italiana. Aspetti storici, paesaggistici, ecologici e gestionali. Memorie della Società ticinese di scienze naturali e del Museo cantonale di storia naturale, vol. 13. Lugano: Società ticinese di scienze naturali; Museo cantonale di storia naturale.
Mota, M., Marques, T., Pinto, T., Raimundo, F., Borges, A., Caço, J., et al. (2018a). Relating plant and soil water content to encourage smart watering in chestnut trees. Agric. Water Manag. 203, 30–36. doi: 10.1016/j.agwat.2018.02.002
Mota, M., Pinto, T., Marques, T., Borges, A., Caço, J., Raimundo, F., et al. (2018b). Study on yield values of two irrigation systems in adult chestnut trees and comparison with non-irrigated chestnut orchard. Rev. Ciências Agrárias 41, 236–248. doi: 10.19084/RCA17233
Ozturk, T., Ceber, Z. P., Türkeş, M., and Kurnaz, M. L. (2015). Projections of climate change in the Mediterranean Basin by using downscaled global climate model outputs. Int. J. Climatol. 35, 4276–4292. doi: 10.1002/joc.4285
Pereira-Lorenzo, S., Costa, R. M. L., Ramos-Cabrer, A. M., Ciordia-Ara, M., Ribeiro, C. A. M., Borges, O., et al. (2011). Chestnut cultivar diversification process in the Iberian Peninsula, Canary Islands, and Azores. Genome 54, 301–315. doi: 10.1139/g10-122
Pereira-Lorenzo, S., Ramos-Cabrer, A. M., Barreneche, T., Mattioni, C., Villani, F., Díaz-Hernández, B., et al. (2019). Instant domestication process of European chestnut cultivars. Ann. Appl. Biol. 174, 74–85. doi: 10.1111/aab.12474
Pérez-Girón, J. C., Álvarez-Álvarez, P., Díaz-Varela, E. R., and Mendes Lopes, D. M. (2020). Influence of climate variations on primary production indicators and on the resilience of forest ecosystems in a future scenario of climate change: application to sweet chestnut agroforestry systems in the Iberian Peninsula. Ecol. Indic. 113:106199. doi: 10.1016/j.ecolind.2020.106199
Pérez-Girón, J. C., Díaz-Varela, E. R., Álvarez-Álvarez, P., Hernández Palacios, O., Ballesteros, F., and López-Bao, J. V. (2022). Linking landscape structure and vegetation productivity with nut consumption by the Cantabrian brown bear during hyperphagia. Sci. Total Environ. 813:152610. doi: 10.1016/j.scitotenv.2021.152610
Poggio, L., de Sousa, L. M., Batjes, N. H., Heuvelink, G. B. M., Kempen, B., Ribeiro, E., et al. (2021). SoilGrids 2.0: producing soil information for the globe with quantified spatial uncertainty. Soil 7, 217–240. doi: 10.5194/soil-7-217-2021
Prospero, S., Heinz, M., Augustiny, E., Chen, Y.-Y., Engelbrecht, J., Fonti, M., et al. (2023). Distribution, causal agents, and infection dynamic of emerging ink disease of sweet chestnut in southern Switzerland. Environ. Microbiol. 25, 2250–2265. doi: 10.1111/1462-2920.16455
Purvis, A., Molnar, Z., Obura, D., Ichii, K., Willis, K., Chettri, N., et al. (2019). “Chapter 2.2. Status and trends – nature” in Global assessment report of the intergovernmental science policy platform on biodiversity and ecosystem services. eds. E. S. Brondízio, J. Settele, S. Díaz, and H. T. Ngo (Bonn, Germany: IPBES Secretariat), 108.
R Core Team (2023). R: a language and environment for statistical computing. Vienna, Austria: R Foundation for Statistical Computing.
Rubio, A. (2009). “‘9260 Bosques de Castanea sativa’” in Bases ecológicas preliminares para la conservación de los tipos de hábitat de interés comunitario en España (Madrid: Ministerio de Medio Ambiente, y Medio Rural y Marino).
Santos, M., Fraga, H., Belo-Pereira, M., and Santos, J. A. (2019). Assessment of growing thermal conditions of Main fruit species in Portugal based on hourly records from a Weather Station network. Appl. Sci. 9:3782. doi: 10.3390/app9183782
Simler-Williamson, A. B., Rizzo, D. M., and Cobb, R. C. (2019). Interacting effects of global change on Forest Pest and pathogen dynamics. Annu. Rev. Ecol. Evol. Syst. 50, 381–403. doi: 10.1146/annurev-ecolsys-110218-024934
Soares, P. M. M., Careto, J. A. M., Russo, A., and Lima, D. C. A. (2023). The future of Iberian droughts: a deeper analysis based on multi-scenario and a multi-model ensemble approach. Nat. Hazards 117, 2001–2028. doi: 10.1007/s11069-023-05938-7
Strobl, C., Malley, J., and Tutz, G. (2009). An introduction to recursive partitioning: rationale, application, and characteristics of classification and regression trees, bagging, and random forests. Psychol. Methods 14, 323–348. doi: 10.1037/a0016973
Thuiller, W., Georges, D., Gueguen, M., Engler, R., Breiner, F., Lafourcade, B., et al. (2023). biomod2: ensemble platform for species distribution modeling. R package version 4.2-4. Available at: https://CRAN.R-project.org/package=biomod2.
Toujgani, I., El Fatehi, S., Ater, M., and Hmimsa, Y. (2024). Variability of the Moroccan sweet chestnut (Castanea sativa Mill.): Contribution of the morphological parameters and qualitative descriptors of the nut. J. Saudi Soc. Agric. Sci. 23, 192–201. doi: 10.1016/j.jssas.2023.11.002
Turchetti, T., and Maresi, G. (2008). “Biological control and management of chestnut diseases” in Integrated management of diseases caused by fungi, phytoplasma and bacteria. eds. A. Ciancio and K. G. Mukerji (New York, NY: Springer), 85–118.
Valavi, R., Guillera-Arroita, G., Lahoz-Monfort, J. J., and Elith, J. (2022). Predictive performance of presence-only species distribution models: a benchmark study with reproducible code. Ecol. Monogr. 92:e01486. doi: 10.1002/ecm.1486
van Westen, R. M., Kliphuis, M., and Dijkstra, H. A. (2024). Physics-based early warning signal shows that AMOC is on tipping course. Sci. Advan. 10:eadk1189. doi: 10.1126/sciadv.adk1189
Xunta de, Galicia (2022). Continuous Forest Inventory of Galicia. Available at: https://invega.xunta.gal/invega/.
Zhang, C., Gomes-Laranjo, J., Correia, C. M., Moutinho-Pereira, J. M., Carvalho Goncalves, B. M., Bacelar, E. L. V. A., et al. (2011). “Response, tolerance and adaptation to abiotic stress of olive, grapevine and chestnut in the Mediterranean region: role of abscisic acid, nitric oxide and microRNAs” in Plants and environment; serie abiotic stress. ed. A. Lazinica (Rijeka, Croatia: InTech), 179–206.
Keywords: Castanea sativa, global change, habitat suitability, Species Distribution Model, resilience
Citation: Álvarez-Álvarez P, Aviñoa-Arias A, Díaz-Varela E, López-Bao JV and Pérez-Girón JC (2025) Impact of climate change over distribution and potential range of chestnut in the Iberian Peninsula. Front. For. Glob. Change. 8:1561027. doi: 10.3389/ffgc.2025.1561027
Received: 15 January 2025; Accepted: 27 January 2025;
Published: 12 February 2025.
Edited by:
Manoj Kumar Jhariya, Sant Gahira Guru Vishwavidyalaya, IndiaReviewed by:
Abhishek Raj, Rajendra Agricultural University, IndiaCopyright © 2025 Álvarez-Álvarez, Aviñoa-Arias, Díaz-Varela, López-Bao and Pérez-Girón. This is an open-access article distributed under the terms of the Creative Commons Attribution License (CC BY). The use, distribution or reproduction in other forums is permitted, provided the original author(s) and the copyright owner(s) are credited and that the original publication in this journal is cited, in accordance with accepted academic practice. No use, distribution or reproduction is permitted which does not comply with these terms.
*Correspondence: Pedro Álvarez-Álvarez, YWx2YXJlenBlZHJvQHVuaW92aS5lcw==
Disclaimer: All claims expressed in this article are solely those of the authors and do not necessarily represent those of their affiliated organizations, or those of the publisher, the editors and the reviewers. Any product that may be evaluated in this article or claim that may be made by its manufacturer is not guaranteed or endorsed by the publisher.
Research integrity at Frontiers
Learn more about the work of our research integrity team to safeguard the quality of each article we publish.