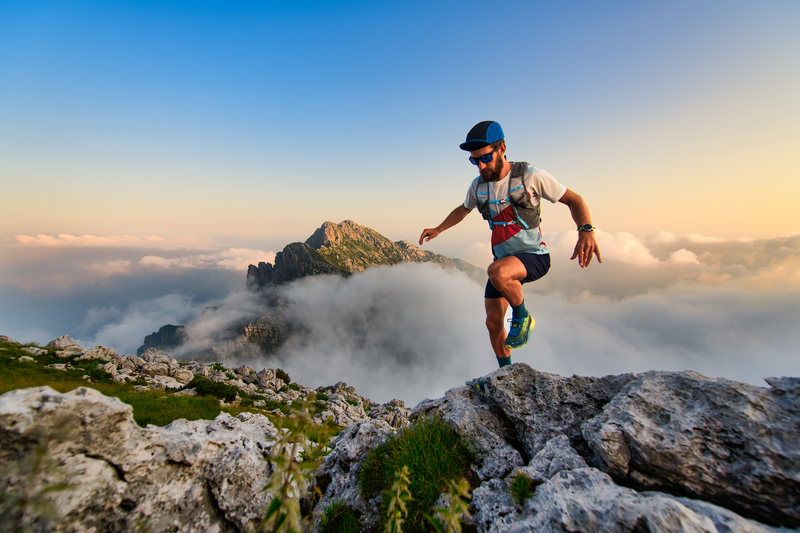
94% of researchers rate our articles as excellent or good
Learn more about the work of our research integrity team to safeguard the quality of each article we publish.
Find out more
REVIEW article
Front. For. Glob. Change , 22 February 2023
Sec. Tropical Forests
Volume 6 - 2023 | https://doi.org/10.3389/ffgc.2023.1106723
This article is part of the Research Topic Dynamics of Asia's and Australasia's forests in a changing world View all 22 articles
Recent advances in satellite-borne optical sensors led to important developments in the monitoring of tropical ecosystems in Asia, which have been strongly affected by recent anthropogenic activities and climate change. Based on our feasibility analyses conducted in Indonesia in Sumatra and Sarawak, Malaysia in Borneo, we discuss the current situation, problems, recent improvements, and future tasks regarding plant phenology observations and land-cover and land-use detection. We found that the Multispectral Instrument (MSI) on board the Sentinel-2A/2B satellites with a 10-m spatial resolution and 5-day observational intervals could be used to monitor phenology among tree species. For the Advanced Himawari Imager (AHI) on board the Himawari-8 geostationary satellite with a 1,000-m spatial resolution and 10-min observational intervals, we found that the time-series in vegetation indices without gaps due to cloud contamination may be used to accurately detect the timing and patterns of phenology among tree species, although the spatial resolution of the sensor requires further improvement. We also found and validated that text and pictures with geolocation information published on the Internet, and historical field notes could be used for ground-truthing land cover and land use in the past and present time. The future development of both high frequency (≤ 10 min) and high spatial resolution (≤ 10 m) optical sensors aboard satellites is expected to dramatically improve our understanding of ecosystems in the tropical Asia.
In the tropics, where biodiversity is the highest (FAO, and UNEP, 2020; Secretariat of the Convention on Biological Diversity, 2020), there is an urgent need to accurately evaluate the spatiotemporal variation of ecosystem functions, ecosystem services which have recently been called “nature’s contributions to people” (Díaz et al., 2018), 1 and biodiversity under the rapid anthropogenic impacts and climate change occurring there (Estoque et al., 2019). Toward this aim, we require accurate and continuous observations of plant phenology (e.g., flowering, leaf flush, leaf coloring, and leaf fall), which serve as proxies of the responses of organisms and ecosystems to the environment (Tang et al., 2016; Piao et al., 2019), and of land-cover and land-use change. Data on plant phenology, and land-cover and land-use change help to explain the spatiotemporal variability of ecosystem properties (e.g., photosynthesis and evapotranspiration, carbon stocks and flows, the land surface’s albedo, and energy balances; Penþuelas et al., 2009; Kumagai et al., 2013; Richardson et al., 2013; Wu et al., 2016), emission of biogenic volatile organic compounds (BVOCs; Penþuelas et al., 2009; Richardson et al., 2013; IPCC, 2021), cultural ecosystem services (e.g., festivals and recreation opportunities; Sakurai et al., 2011; Sparks, 2014; Nagai et al., 2019), regulating ecosystem services (e.g., pollinator abundances and pollination; Lautenbach et al., 2012; Rohde and Pilliod, 2021), environmental changes in various habitats (Muraoka et al., 2012; Gray and Ewers, 2021), and biodiversity conservation (Morisette et al., 2009; Secades et al., 2014; Morellato et al., 2016). Phenological mismatch between plants and their animal pollinators and consumers caused by the changes of the timing of each phenology due to climate change, reduces the biodiversity (Visser and Gienapp, 2019; Secretariat of the Convention on Biological Diversity, 2020). The evaluation of spatial-temporal variability of the interaction between landscape, which is mainly explained by land cover and land use, and anthropogenic activities, also provides fundamental knowledge to deeply understand the spatial-temporal variability of ecosystem functions, ecosystem services and biodiversity under climate and societal changes.
Satellite remote-sensing by optical sensors is useful for evaluating the spatiotemporal variation of plant phenology, land cover, and land use over a broad scale (Muraoka et al., 2012; Nagai et al., 2020a; Shin et al., 2023). Since 1972 when Landsat-1 was launched, 2 satellite optical sensors that observe visible and near-infrared regions of the electro-magnetic spectrum have continuously monitored the state of the ground surface from plot to global scales (Table 1 shows a summary of the specification of optical sensors on board satellites shown in this perspective paper). However, these optical sensors are affected by atmospheric noise and cloud contamination, which is the biggest disadvantage of optical sensors. The opportunity for observation under clear sky conditions in the tropics is much rarer than in other regions (Nagai et al., 2011, 2014a).
In contrast, synthetic aperture radar (SAR) on board satellites is not affected by atmospheric noise and cloud contamination and allows for nighttime observation (e.g., Phased-Array type L-band Synthetic Aperture Radar 2 on board the Advanced Land Observing Satellite [ALOS]-2) and measurement of the spatiotemporal variation of ecosystem structures. SAR transmits microwaves and then actively receives the returned microwave off the ground surface, allowing the detection of forest/non-forest domains, land use and land cover, and aboveground biomass of forests (Miettinen and Liew, 2011; Avtar et al., 2014; Shimada et al., 2014; Kou et al., 2015; Li L. et al., 2015; Stelmaszczuk-Górska et al., 2018). However, SAR cannot observe plant phenology, which is mainly shown as a characteristic of color change of canopy surface on satellite remote-sensing in optical signals. Thus, advancement in SAR technology and/or the integration of SAR and optical sensors will be needed for the accurate detection of land cover and land use (Najib et al., 2020).
To improve the accuracy of phenology observations (e.g., detection of accurate timing of flowering, leaf-flush, and leaf-fall in each ecosystem and/or tree species) and land-cover and land-use detection (e.g., categorization of various land use type and immediate detection of land cover change with a high spatial resolution), we ideally require an optical sensor with high spatial (e.g., 10 m), temporal (e.g., daily interval), and wavelength (many narrow spectral bands) resolutions. However, these three properties have not yet been attained simultaneously with a single sensor due to trade-offs especially between spatial and temporal resolutions (Nagai et al., 2020a). This limitation has made it difficult to accurately monitor the ground surface in the tropics, where the plant phenology and its synchrony among tree species are much less clear than in temperate and boreal vegetation (Harrison, 2001; Nakaji et al., 2014; Nagai et al., 2016a; Osada, 2018; Nakagawa et al., 2019). Higher diversity and heterogeneity of tree species in the Asian tropics (Lee et al., 2002) make satellite-based phenological observations difficult. Marked land-cover and land-use changes have accelerated in the tropics due to anthropogenic activities (e.g., deforestation) and climate change (e.g., forest fires triggered by the El Niño–Southern Oscillation; Ichikawa, 2007; Segah et al., 2010; Wooster et al., 2012; Hansen et al., 2013; Nagai et al., 2014a; Marlier et al., 2015; Spessa et al., 2015).
For phenology observations and detection of the interannual variation of land cover and land use, researchers have frequently used data observed by optical sensors on board public satellites with high frequency but a coarse spatial resolution, such as the Advanced Very High Resolution Radiometer (AVHRR) on board the National Oceanic and Atmospheric Administration satellite (NOAA; 1100-m spatial resolution at a daily interval; e.g., Erasmi et al., 2014; Garonna et al., 2014; Buitenwerf et al., 2015; Gao et al., 2019), the Moderate Resolution Imaging Spectroradiometer (MODIS) on board the Terra and Aqua satellites (250- to 500-m spatial resolution at a daily interval; e.g., Zhang et al., 2003; Miettinen et al., 2011; Pennec et al., 2011; Jin et al., 2019), and the VEGETATION optical sensor on board the Satellite Pour l’Observation de la Terre (SPOT; 1000-m spatial resolution at a daily interval, 3 e.g., Delbart et al., 2006, 2015; Segah et al., 2010; Kobayashi et al., 2016). Also for this purpose, researchers have frequently used data observed by optical sensors on board public satellites with low frequency (16-day intervals) but a moderately high spatial resolution (30 m) such as the Landsat series of satellites (Segah et al., 2010; Kou et al., 2015; Li P. et al., 2015; Ishihara and Tadano, 2017; Morozumi et al., 2019). In contrast, for the detection of land cover and land use with a fine-scale, they have frequently used data observed by optical sensors on board commercial satellites (e.g., the RapidEye: Imukova et al., 2015; Pfeifer et al., 2016; the WorldView series satellites: 4 Nomura and Mitchard, 2018; Rahmandhana et al., 2022) with a high spatial resolution (e.g., 50 cm) but quite low frequency (e.g., 46-day intervals).
Some advantages of optical sensors on board public satellites are the uniformity of observed data coverage, stable long-term continuous observations from the long-term missions (e.g., the Landsat series of satellites: See text footnote 2), and free usage on the Internet. In contrast, the data observed by optical sensors on board commercial satellites tend to be low-frequency data distributed in urban areas, meaning that we cannot easily access satellite data in remote regions. Although researchers could request satellite image acquisitions of remote regions to these companies, it is an impractical idea to request periodic broad-scale satellite observations in remote regions over a long period due to the cost of obtaining such commercial data.
In the latter half of the 2010s, the spatiotemporal resolution of optical sensors on board public satellites remarkably progressed with the launch of the Multispectral Instrument (MSI) on board the Sentinel-2A/2B satellites, with a 10-m spatial resolution at 5-day intervals (; e.g., Nomura and Mitchard, 2018; Persson et al., 2018; Vrieling et al., 2018; Chang et al., 2021), 5 and the Advanced Himawari Imager (AHI) on board the Himawari-8 geostationary satellite, with a 1,000-m spatial resolution at 10-min intervals (at 2.5-min intervals around Japan; Miura et al., 2019; Yan et al., 2019; Miura and Nagai, 2020). 6 Although these optical sensors do not satisfy the need for simultaneous high spatial, temporal, and wavelength resolutions, these optical sensors will be expected to provide much more accurate and precise satellite observations, along with a reduction of uncertainties and systematic noise in land-cover and land-use detection and phenology observations (Shin et al., 2023). Continuous and extensive satellite observations by these optical sensors will be also expected to develop spatiotemporally interpolating and extrapolating in situ observed data on ecosystem functions and biodiversity in each tropical observation field.
In this perspective paper, based on this recent progress in the optical sensors on board satellites and the development of observation systems in the latter half of the 2010s, we focus on satellite optical sensors and discuss the current situation, problems, and recent improvements, as well as future tasks regarding phenology observations and land-cover and land-use detection in the Asian tropics. Here, we focus on island or maritime Southeast Asia. In order to discuss concretely, we review our feasibility analyses in Sarawak, Malaysia in Borneo, where our research group has conducted field studies to validate satellite remote-sensing since the 2010s, and Indonesia in Sumatra by using data from the Sentinel-2A/2B–MSI and the Himawari-8–AHI satellites. In Section 2, we describe how the accuracy of satellite phenology observations can be improved from the viewpoints of advanced resolution sensors and frequency of satellite observations. Next, in Section 3, we describe how to improve the accuracy of land-cover and land-use detection from the viewpoints of detection of year-to-year variability and collection of past and present ground-truth information. Then, in Section 4, we discuss the future tasks to help improve our understanding of Asian tropical ecosystems. Finally, in Section 5, we conclude our discussions in this perspective paper.
Figure 1 shows the time-series of vegetation indices of Lambir Hills National Park (primary tropical rain forest; 4°12’04”N, 114°02’21”E; Nakagawa et al., 2019) and the Lambir oil palm plantation (4°09’07”N, 113°57’58”E) in Sarawak, Malaysia in Borneo observed by the Sentinel-2A/2B–MSI (atmosphere corrected data) and Himawari-8–AHI satellites. Primary tropical rain forests, oil palm plantation forests, and secondary forests are typical landscape features in this area (Ichikawa, 2007). In addition, typical canopy surface images of Lambir Hills National Park are shown in Figure 2. We show only NDVI values for the Himawari-8–AHI satellite because we used the reflectance data at the top of the atmosphere (i.e., atmosphere uncorrected data). For the Sentinel-2A/2B–MSI satellites, we selected observation scenes with cloud cover ≤ 10%, but we plotted all values observed by the Himawari-8–AHI satellites. For the Himawari-8–AHI satellite, we plotted values observed at 3 h around the culmination time because there was no property in the data regarding the cloud contamination. Despite the occurrence of general flowering in Lambir Hills National Park in May 2019, which occurs every 1–4 years, and the color of the canopy surface changing from dark green to whitish green (Sakai et al., 2006; Azmy et al., 2016; Chechina and Hamann, 2019; Ushio et al., 2020), every vegetation index showed no clear seasonal change (low values may be affected by cloud contamination). During the general flowering period, no data were observed by the Sentinel-2A/2B–MSI satellites under clear sky conditions (Figure 1). MSI Green-Red Vegetation Index (GRVI; Motohka et al., 2010) values observed at Lambir Hills National Park were about 0.05 smaller than those at the Lambir oil palm plantation, reflecting the difference in color of the canopy surface between tropical rain forest (dark green) and the oil palm plantation (light green). Nagai et al. (2016a) reported that NDVI and GRVI values observed by the photodiode sensors installed at the top of a crane tower in Lambir Hills National Park were almost constant throughout the year. In contrast, the time-series of the ratio of R, G, and B digital numbers to the total RGB digital numbers extracted from daily canopy surface images taken at Lambir Hills National Park showed characteristics of leaf flush and flowering among individual trees (Nagai et al., 2016a). These results indicate the possibility that long-term continuous observations by future advanced optical sensors on board satellites with a high spatial resolution and high temporal resolution (high frequent) may detect characteristics of phenology for each tree species in a tropical rain forest. In fact, the RGB composite images observed by the PlanetScope constellation of satellites, which consist of approximately 180 microsatellites (as of 16 November 2022; a commercial endeavor),78 with an approximately 3-m spatial resolution at a daily interval could detect the characteristics of flowering phenology among tree species in Lambir Hills National Park during the general flowering event in May 2019 (Miura et al., 2023). The swath range that can be observed at one time by each PlanetScope microsatellite is narrow (25 km), but the entire observation system of approximately 180 microsatellites resolved the trade-off between spatial and temporal resolutions. Some previous studies indicated this advantage of PlanetScope constellation of satellites observations in alpine, temperate, and tropical regions (Leach et al., 2019; John et al., 2020; Wang et al., 2020, 2023; Moon et al., 2021; Wu et al., 2021).
Figure 1. Time-series of vegetation indices observed by (A,B) the Sentinel-2A/2B–MSI and (C,D) the Himawari-8–AHI satellites in Lambir Hills National Park (primitive tropical rain forests; 4°12’04”N, 114°02’21”E) and the Lambir oil palm plantation (4°09’07”N, 113°57’58”E) in northwestern Borneo. The thick vertical solid lines in (A,C) indicate the dates of canopy surface images in Figure 2. Cloud contamination appeared in Lambir Hills National Park and the Lambir oil palm plantation images on 5 March, 10 March (only in the Lambir oil palm plantation), 25 March, 4 April, and 20 November (only in the Lambir oil palm plantation). A cloud shadow appeared in Lambir Hills National Park image on 20 November.
Figure 2. Typical canopy surface images taken at the top of a crane tower in Lambir Hills National Park (http://www.pheno-eye.org/). The day of year (DOY) is shown in the bottom-right corners of each image. General flowering was shown on DOY 123.
How can we detect the characteristics of phenology in tropical rain forests consisting of evergreen broad-leaved trees, with seasonality much less clear than that of deciduous trees, with optical sensors on board satellites? Previous studies reported that the RGB composite images observed by the Sentinel-2A/2B–MSI satellites, with the highest spatial resolution among the optical sensors on board public satellites, detected the color change on the canopy surface of Castanopsis sieboldii, Castanopsis cuspidate, and Lithocarpus edulis (evergreen oak tree species) caused by leaf flush (light green) and successive flowering (cream) in Japan (Nagai et al., 2020b; Shinohara and Nasahara, 2022; Shin et al., 2023). These plants are insect-pollinated flowers of Fagaceae and their flowers bloom across the whole canopy surface. The timing of flowering is different among these tree species (L. edulis is approximately one month later than C. sieboldii and C. cuspidate). In a tropical rainforest in Borneo (Lambir Hills National Park), Miura et al. (2023) reported that the spectral reflectance observed by the PlanetScope constellation satellites detected the characteristic of color change on the canopy surface of Dryobalanops aromatica, Shorea ochracea, Swintonia foxworthyi, and Pentace borneensis during a general flowering in 2019. These results indicate the possibility that satellite-based observations may be used to track the phenological timing and patterns of various tree species in tropical rain forests in tandem with ground-truth information.
Remote-sensing can detect the color of the canopy surface of various tree species, which indicate the characteristics of leaf traits (leaf size, leaf biomass, leaf thickness, amount of pigments in a leaf, and angle of leaves) and structures (tree structure and height) (Sims and Gamon, 2002; Luke et al., 2013; Noda et al., 2014; Asner et al., 2015; Noda et al., 2021; Rahmandhana et al., 2022). The characteristics of leaf longevity, which is explained by leaf-flush and leaf-fall phenology, were correlated with the type of photosynthesis, leaf traits and structures, and climate (Wright et al., 2004; Kikuzawa, 2005; Onoda et al., 2011; Kikuzawa et al., 2013). These facts indicate the importance of discriminating each tree species by referring to the characteristics of phenology and canopy structures and mapping the geographic distributions for each tree species at a broad scale. In Lambir Hills National Park, however, the geographic distribution of each tree species is heterogeneous due to microtopography (Lee et al., 2002). The collection of ground-truth information for various tree species, thus, is both an important and challenging task. Integrating in situ and satellite-based phenological observations should also result in the tree discrimination of forests in the Asian tropics.
As mentioned above, the Sentinel-2A/2B–MSI satellites have the potential to remarkably improve phenology observations in the tropics. The MSI observations occur at 5-day intervals, however, and in 2019, we only obtained seven scenes with cloud cover ≤ 10% (Figure 1). In addition, we confirmed that cloud contamination appeared in Lambir Hills National Park and the Lambir oil palm plantation images on 5 March, 10 March (only in the Lambir oil palm plantation), 25 March, 4 April, and 20 November (only in the Lambir oil palm plantation) by visually checking the RGB composite images. In addition, we confirmed that a cloud shadow appeared in Lambir Hills National Park image on 20 November. Therefore, it appears that the Sentinel-2A/2B–MSI satellites cannot observe the characteristics of phenology for each tree species in the suitable period under clear sky conditions. In contrast, the observation frequency of the Himawari-8–AHI satellite under clear sky conditions is much higher than that of the Sentinel-2A/2B–MSI (Figure 1). In fact, even though the sky image taken at 10:30 (LST) in Lambir Hills National Park, which was the time of the Sentinel-2A/2B–MSI satellites passage, showed cloudy skies, many other images showed clear skies at other times (Nagai et al., 2018).
In previous phenology studies that analyzed time-series of satellite-observed vegetation indices, the researchers used the 8-day, 10-day, and bimonthly composite data in order to eliminate noise and missing data caused by atmospheric noise and cloud contamination and smoothed the composite time-series data by applying some fitting functions (Zhang et al., 2003; Delbart et al., 2006, 2015; Erasmi et al., 2009, 2014; Pennec et al., 2011; Wu et al., 2014; Garonna et al., 2014; Buitenwerf et al., 2015; Kobayashi et al., 2016; Park et al., 2016; Gao et al., 2019; Jin et al., 2019). These analyses were based on the hypothesis that vegetation indices observed under clear sky conditions, which might show the most accurate value, were higher than those under cloudy and rainy conditions. Therefore, the smoothed values are estimated values but not true values. The values smoothed by applying the fitting functions may include two types of systematic errors: (1) an actual value was eventually eliminated, or (2) an actual missing value was eventually misread as a true value. For instance, in the case of an abrupt decrease of vegetation caused by a landslide, vegetation indices after the landslide may be eliminated as noise by applying some fitting functions. In addition, in the case of successive cloudy and rainy conditions in a certain period, smoothed vegetation indices may be misinterpreted as a decrease of vegetation. Thus, from a statistical viewpoint, we should avoid smoothing as much as possible.
The frequency of the observations of vegetation indices in East Asia by the Himawari-8–AHI satellite is 10-min intervals. If we only select the vegetation indices observed during the daytime that were little affected by the solar elevation angle (e.g., 3 h around noon), we can obtain many data observed under clear sky conditions (Miura et al., 2019). Figure 3 shows the relationship between NDVI values observed by the Himawari-8–AHI satellite and state of cloud cover based on in situ observed sky images in Lambir Hills National Park during 8:05 and 16:45 LST (UTC + 8) from 2015 to 2016. The occurrences of higher NDVI values (> 0.8) coincided well with the timings of in situ “very sunny” or “sunny” sky conditions, despite including missing data of sky images. The analysis of Figure 3 indicated that the number of days with clear sky conditions ranged from 57 to 96, which was much more than vegetation index observed by the Terra/Aqua–MODIS satellites (1–5 days per month in the southwest monsoon period [May–October] and 0–2 days per month in the northeast monsoon period [November–April] in Borneo; at a daily interval; Nagai et al., 2014a). In addition, the confidence in the change in vegetation indices, which was detected by the actual change of vegetation or not (i.e., systematic noise caused by atmospheric noise and cloud contamination), may also increase by checking high-frequency continuously observed data under clear sky conditions. So, if we can extract data observed only under clear sky conditions, we scarcely need to smooth by applying fitting functions. Despite a coarse spatial resolution (1,000 m), the vegetation indices observed by the Himawari-8–AHI satellite may indicate fairly accurate values.
Figure 3. Relationship between NDVI values observed by the Himawari-8–AHI satellite and state of cloud cover based on in situ observed sky images in Lambir Hills National Park during 8:05 and 16:45 LST (UTC + 8) from (A) 2015 and (B) 2016 (sky images from Nagai et al., 2018).
At present, however, the Himawari-8–AHI satellite is still not suitable with regard to spatial resolution for phenology observations in tropical rain forests. In fact, despite the high frequency of observations under clear sky conditions, no characteristic change in the time-series of NDVI was shown in May 2019, when the general flowering occurred in Lambir Hills National Park (Figures 1, 2). The time-series in GRVI, which can detect the change of color of the canopy surface (Motohka et al., 2010; Nagai et al., 2014b), might capture some characteristic of temporal change. In Lambir Hills National Park, the time-series in the ratio of RGB digital numbers for each individual tree extracted from daily canopy surface images showed differences among tree species around the general flowering period (Nagai et al., 2016a). In contrast, those for the whole canopy showed almost constant values throughout the year (Nagai et al., 2016a), perhaps because not all individuals and tree species flowered at the same time. In this case, the target region of canopy surface images was within at most 100 m (Nagai et al., 2016a), but in the Lambir Hills National Park, which consists of over 1000 tree species (Lee et al., 2002), vegetation indices observed by the Himawari-8–AHI satellite can detect the average phenology of various tree species within a 1,000-m-by-1,000-m area. Therefore, for accurate phenology observations in tropical rain forests, we require an onboard optical sensor with a high spatial resolution to discriminate each tree individual (≤ 10 m) at high temporal frequencies (on the order of 10-min interval) to eliminate cloud contaminations in satellite data.
Despite the uncertainty caused by the heterogeneity of tree species and microtopography, previous studies indicated that the time-series of vegetation indices could be used to accurately detect the spatiotemporal variation of leaf flush and leaf fall in deciduous forests in Japan by validating the indices against long-term continuous in situ observed data (Miura et al., 2019; Yan et al., 2019). Despite the effect of microtopography (elevation) on phenology and the differences in timing and patterns of leaf flush and leaf fall among tree species (Inoue et al., 2014; Nagai et al., 2014b; Shin et al., 2021a), leaf flush and leaf fall within a narrow region (e.g., 1,000-m square) occur rapidly and nearly simultaneously. The Japanese government is planning to launch a geostationary satellite with an optical sensor with a 3- to 4-m spatial resolution. 9 Such future developments in optical sensors on board satellites with high observation frequency and high spatial resolution will remarkably improve the accuracy of phenology observations in tropical rain forests, where the phenological timing and patterns differ among the numerous and highly diverse tree species (Osada, 2018; Reich et al., 2004).
Figure 4 shows that the interannual variation of deforestation from 2001 to 2020 could be detected by analyzing the time-series of the daily GRVI observed by the Terra/Aqua–MODIS satellites [500-m spatial resolution; adapted from Nagai et al. (2014b)] in Indonesia in Sumatra, where marked land-cover and land-use change has occurred due to deforestation and expansion of oil palm plantations (Ichikawa, 2007; Fitzherbert et al., 2008; GEAS, 2011; Koh et al., 2011; Miettinen et al., 2011; Hansen et al., 2013; Carlson et al., 2014; Nagai et al., 2014a; Estoque et al., 2019; Najib et al., 2020). Here, we defined deforestation as having occurred at points where the ratio of number of days observed GRVI < 0 under clear sky conditions to total observed GRVI under clear sky conditions was above 80% (Nagai et al., 2014a). This hypothesis was based on the fact that GRVI < 0 after leaf fall or when there was no vegetation (Motohka et al., 2010; Nagai et al., 2014b). In the deforested area detected by the Terra/Aqua–MODIS satellites (Figure 4), we also identify that vegetation is sparse by visually inspecting the RGB composite images observed by the Sentinel-2A/2B–MSI satellites with a 10-m spatial resolution (shown in brown; Figure 5). In addition, we identify that GRVI observed by the Sentinel-2A/2B–MSI satellites showed under 0 (Figure 6). From 2001 to 2020, the deforested areas continuously expanded in Riau (central Sumatra; Figure 4C) and Lampung (southern Sumatra; Figure 4B). In Indonesia, the loss rate of primary forests has declined since 2016 according to the Secretariat of the Convention on Biological Diversity (2020); however, our analysis suggests that the deforestation is still ongoing.
Figure 4. Spatiotemporal variation of deforestation in Indonesia in Sumatra from 2001 to 2020 detected by analyzing the time-series of daily GRVI observed by the Terra/Aqua–MODIS satellites (adapted from Nagai et al., 2014b). Each color indicates the latest deforested year. The dashed squares in (A) are enlarged in (B) and (C). Boundary and river data come from the “1:10 m cultural vectors” published by Natural Earth (https://www.naturalearthdata.com).
Figure 5. RGB composite images of Indonesia in Sumatra observed by the Sentinel-2A/2B–MSI satellites in 2020. The RGB composite images for the Sentinel-2A/2B–MSI satellites were composed of 73 days of data observed under clear sky conditions. For the Sentinels-2A/2B–MSI satellites, we estimated the atmospheric corrected ground surface reflectance data by using the SNAP application (Sentinel Toolboxes: https://step.esa.int/main/download/snap-download/). The dashed squares in (A) are enlarged in (B,C). The colored symbols mark the locations of rice paddy, banana, cassava, and rubber recorded in field notes in 1978 (https://fieldnote.archiving.jp/). Boundary and river data come from the “1:10 m cultural vectors” published by Natural Earth (https://www.naturalearthdata.com).
Figure 6. GRVI of Indonesia in Sumatra observed by the Sentinel-2A/2B–MSI satellites in 2020. No algorithm was applied to remove cloud contamination. The black symbols mark the locations of rice paddy, banana, cassava, and rubber recorded in field notes in 1978 (https://fieldnote.archiving.jp/). Boundary and river data come from the “1:10 m cultural vectors” published by Natural Earth (https://www.naturalearthdata.com). The dashed squares in (a) are enlarged in (b) and (c).
Interannual variation of deforestation in the tropics has been detected by analyzing the time-series of vegetation indices observed by the Landsat series (30-m spatial resolution at 16-day intervals; Hansen et al., 2013). However, for those satellites capturing data at 16-day intervals, it is possible that no data are observed under clear sky conditions throughout an entire year especially in the Asian tropics, which is one of regions with active atmospheric water circulation. In addition, in the case of the ETM + sensor on board the Landsat-7 satellite, each observation scene always included partial missing data due to a systematic error in the sensor (malfunction of the scan line corrector; Wang et al., 2021). For the Terra/Aqua–MODIS satellite observations with a 500-m spatial resolution, we could detect large-scale land-cover and land-use change in the tropics, including the establishment of oil palm and acacia plantations after deforestation (Nagai et al., 2014a), but we could not accurately detect local-scale changes such as the loss of vegetation caused by a landslide, which typically occurs at an area smaller than a footprint of satellite data (1 pixel size of satellite data) with a coarse spatial resolution (500-m or 1,000-m; Miura and Nagai, 2020). In contrast, high-frequency observations by the Sentinel-2A/2B–MSI satellites with a 10-m spatial resolution at 5-day intervals may improve the accuracy of detecting interannual variation of land cover and land use in the tropics. Using the time-series of the vegetation index observed by the Sentinel-2A/2B–MSI satellites, whose data will be accumulated over a long period, should help us to more accurately detect the interannual variation of the geographic distribution of deforestation by applying the same analysis as was applied to the Terra/Aqua–MODIS satellites data.
To improve and validate the accuracy of satellite-based land-cover and land-use maps, we must collect ground-truth information at multiple points (Tsutsumida et al., 2019). One way to achieve this is by using digital camera images with the geolocation information, time, and date shot at multiple points that have been uploaded on the Mapillary website, 10 which is a crowdsourcing project. For some reported points, we can use images taken on different dates, thus allowing us to obtain evidence of land-cover and land-use change over a short period. Funada and Tsutsumida (2022) also indicated the usability of the street-level photographs published on the Mapillary to map the geographical distribution of cherry flowering in Fukushima in Japan. The text and images uploaded to the Degree Conference Project (DCP) website11 are also useful ground-truth information regarding land cover and land use. Previous studies reported the suitability of information on the DCP for use in validating satellite-based land-cover and land-use maps (Iwao et al., 2011; Soyama et al., 2017).
Figure 7 shows Mapillary images at four locations that were typical landscapes in Indonesia in Sumatra, according to field notes recorded in 1978 (full details are mentioned later). Mapillary also contains many images taken at intervals along the route of a participant’s trip by using a car-mounted camera. Such uploaded data may have a geographic bias and be concentrated in areas that are strongly affected by anthropogenic activities (e.g., on streets). Compared with Mapillary, there is less systematic bias in the geographic distribution of target points published on the DCP website, which allows users to choose the intersection of latitude and longitude integer values in remote regions that have been little affected by human activities. However, the data volume of Mapillary (more than 1.8 billion street-level images as of 16 November 2022; See text footnote 10) is much larger than that of the DCP (about 1.32 million photographs as of 16 November 2022; See text footnote 11), making the usability of Mapillary superior. By actively uploading captured images with geolocation information on Mapillary, especially in the points and areas where images have not yet been uploaded, field scientists can help to reduce the missing areas of in situ observations.
Figure 7. Example of Mapillary images (https://www.mapillary.com/) taken at four points where the typical landscapes in Indonesia in Sumatra were recorded in field notes in 1978 (https://fieldnote.archiving.jp/): (a) rice paddy (geolocation based on the field note: 0°41’58.9”S, 100°36’02.4”E; geolocation based on the Mapillary image: 0°42’13.0”S, 100°35’56.0”E; location gap of about 500 m), (b) banana (geolocation based on the field note: 3°44’18.5”S, 104°39’33.6”E; geolocation based on the Mapillary image: 3°44’52.2”S, 104°39’28.1”E; location gap of about 1,100 m), (c) cassava (geolocation based on the field note: 5°19’12.9”S, 105°11’59.1”E; geolocation based on the Mapillary image: 5°18’40.0”S, 105°11’21.4”E; location gap of about 1,500 m), and (d) rubber (geolocation based on the field note: 5°19’49.6”S, 105°12’20.5”E; geolocation based on the Mapillary image: 5°19’54.4”S, 105°11’47.2”E; location gap of about 1,100 m). The Mapillary images are provided under the Creative Commons Attribution ShareAlike license (CC-BY-SA; https://www.mapillary.com/).
Another important issue is how to obtain ground-truth information regarding land cover and land use in the past. This solution deepens our understanding of the conversion processes of deforestation and agricultural expansion. We may estimate the historical land cover and land use by examining the present conditions. For instance, we can assume that areas now covered by oil palm and acacia plantations in Sarawak, Malaysia in Borneo were once covered by tropical rain forests (Nagai et al., 2014a). Without ground-truthing, however, those values will always remain as estimates. To solve this issue, landscape descriptions in research field notes may provide useful ground-truth information regarding past land cover and land use. A group of Japanese scientists at Kyoto University launched the “Inheriting field notes” project (Takata et al., 2014; Yamada, 2015)12 and have published 46,281 sets of digitalized field notes on their website. 13 Those field notes include field trips in the Middle East, the Mediterranean, Africa, South Asia, East Asia, Southeast Asia, and Oceania from 1967 to 2016 (mainly the 1980s and 1990s). At the time of the field trips, researchers could not use the tools to capture geolocation information, such as a Global Positioning System (GPS) receiver or a digital camera with this function. However, by thoroughly examining the time-series of objective descriptions in the field notes, we can roughly identify the landscape at a certain point.
For instance, by applying a text mining approach to field notes recorded in Indonesia in Sumatra in 1978 (1802 items) we analyzed the frequency of the words used (Yamamoto et al., 2015). The field notes included typical words regarding landscapes, such as rice paddy (total of 283 cases), banana (164 cases), cassava (136 cases), and rubber (185 cases), which allowed us to identify the landscape at that time. These words are also useful ground-truth information regarding the land cover and land use in Indonesia in Sumatra in 1978. We plotted the locations of rice paddy, banana, cassava, and rubber extracted from the field notes in Figure 5. In addition, by using the Mapillary images (Figure 7), we compared the land cover and land use in 1978 published on field notes with those at the present time. Despite the difficulty in checking precise geolocations, we could validate that there was no land-cover and land-use change at two points, where rice paddy and banana were recorded in the field notes. However, like the Mapillary images, many points recorded on the field notes may be located in areas that were strongly affected by anthropogenic activities. The retirement and decease of owners will accelerate the loss of personal analog data such as field notes. Rescuing and archiving of these analog data and information is an urgent issue (Shin et al., 2020).
To improve our understanding of Asian tropical ecosystems, we propose five issues that need to be addressed: (1) further collecting ground-truth information from multiple locations and various periods; (2) improving the classification of plant functional types (PFTs) on land-cover and land-use maps and detecting the interannual variation of PFTs; (3) studying the interactions between terrestrial and marine ecosystems; (4) investigating the interaction between land-cover and land-use change and anthropogenic activities; and (5) developing integrative analysis and evaluation of in situ and satellite-observed data.
In conjunction with the development of optical sensors on board satellites, researchers also need to gather ground-truth information obtained at multiple locations in various periods. The quality of satellite data depends on the accuracy and precision of atmospheric and geometric corrections of those data. Previous studies used locally collected data such as daily phenology images and spectrum data observed from towers (Nagai et al., 2014a,2020a,b; Nakaji et al., 2014; Lopes et al., 2016). Such ground-truth information provides accurate and precise data collected over a long period. However, the number of locations in the tropics where phenology images and spectrum data are being collected is still limited (Nakaji et al., 2014; Nasahara and Nagai, 2015; Lopes et al., 2016; Alberton et al., 2017; Nagai et al., 2018, 2020a).
Another way to obtain detailed ground-truth information from multiple locations for a broad-scale picture of historical changes in land cover and land use is to examine “social sensing data,” a type of big data. These include videos posted to YouTube and old television programs (De Frenne et al., 2018; Shin et al., 2022b), and text and photographs with geotag information posted to social networking services (e.g., Twitter, Instagram, and Flickr; Fernández-Bellon and Kane, 2020; Silva et al., 2018; Song et al., 2020; Yoshimura and Hiura, 2017). The interests and movement of people at various locations can also be tracked by analyzing the access statistics of Google (Google Trends: Takada, 2012; Proulx et al., 2013), 14 number of visitors at Wikipedia (Fernández-Bellon and Kane, 2020), and geolocation information of mobile phones (Chang et al., 2021; Pintér and Felde, 2021). For instance, the analysis of Twitter posts was useful for evaluating the spatiotemporal variation of the timing of leaf coloring in Japan (Shin et al., 2021b). Kotani et al. (2021) and Shin et al. (2022a) analyzed the time-series of Google Trends and/or Yandex statistics (a major search engine in Russia)15 to assess the spatiotemporal characteristics of people’s interest in the use of berries in Arctic and the Russian Far East regions, which the authors used as proxy data of ripening phenology. Likewise, people’s interests in oil extracted from illipe nuts (Borneo tallow nut), which are seeds of Dipterocarpaceae species (Blicher-Mathiesen, 1994), may be useful as ground-truth information for ripening phenology in Sarawak, Malaysia Borneo.
As an example of a detailed land-cover and land-use map in Asia with a high spatial resolution, the Japan Aerospace Exploration Agency has published the land-cover and land-use maps of Japan and Vietnam with 10-m or 30-m spatial resolutions by integrative analysis of data from multi-satellites such as the Sentinel-2A/2B–MSI, the ALOS–AVNIR2 (Advanced Visible and Near Infrared Radiometer type 2), the Landsat series satellites, and the ALOS2–PALSAR2 (Hirayama et al., 2022; Hoang et al., 2020). 16 However, these maps of Japan did not account for the interannual variation in land cover and land use. In addition, PFTs were classified into broad categories, such as deciduous broad-leaved forest and evergreen coniferous forest. To accurately evaluate the spatiotemporal variation of the heat, water, and carbon cycles and biodiversity in the tropics, and to understand the sensitivity of vegetation to environmental change and succession, accurate classification of PFTs and detection of their interannual variation are needed. For instance, traits of photosynthesis and evapotranspiration differ among ecosystems and tree species in the tropics (Ishida et al., 2005; Kenzo et al., 2004, 2006, 2011, 2015). The improved classification of PFTs and discrimination of tree species based on photosynthesis, leaf traits, and leaf and canopy structures are important tasks because these traits help to account for the sensitivity of the flowering, leaf-flush, and leaf-fall phenology and leaf longevity to environmental changes and succession.
The soil in the tropics is oligotrophic (Fujii et al., 2018), and land-cover and land-use change due to deforestation has strongly affected not only the heat, water, and carbon cycles (Carlson et al., 2014; Kumagai et al., 2013; Takahashi et al., 2017), but also coastal ecosystems due to the outflow of nutrients from the soil surface to rivers (Tanaka et al., 2021). To accurately evaluate the spatiotemporal variation of ecosystem functions, ecosystem services, and biodiversity triggered by anthropogenic activities and climate change, we need to improve our understanding of the interactions between terrestrial and marine ecosystems. The SeaWiFS (1.13-km at a daily interval) 17 and Aqua–MODIS satellites, which were launched around 2000, observe ocean color and allow for estimation of chlorophyll concentration (O’Reilly et al., 1998; Schollaert et al., 2003; Gregg and Casey, 2004; Siswanto and Tanaka, 2014; Groom et al., 2019). In addition, the Second Generation Global Imager (SGLI) on board the Global Change Observation Mission-Climate (GCOM-C) satellite (250-m at 2-day intervals)18 improved the accuracy and precision of ocean color observations (Murakami, 2016; Matsuoka et al., 2021). Further research should examine, for instance, the relationship between the interannual variation of deforestation and the spatiotemporal variation of the chlorophyll concentrations in the coastal areas of some river basins.
To understand the spatiotemporal variation of ecosystem services and biodiversity, we must evaluate the spatiotemporal variation of the interaction between land-cover and land-use change and anthropogenic activities. For this, the nighttime light data observed by the Visible Infrared Imaging Radiometer Suite (VIIRS) on board the Suomi NPP satellite (Day/Night Band [DNB]; 750-m at a daily interval; Elvidge et al., 2017, 2021)19 may be useful, as land-cover and land-use change has caused the spatiotemporal variation of nighttime light. In a study of 46 cities with more than 50,000 inhabitants, Ivan et al. (2020) reported the suitability of the Suomi NPP–VIIRS satellite-observed nighttime light to evaluate the geographic variation of income. Studies of tropical regions could use these satellite data to examine the relationship between the interannual variation of deforestation and spatiotemporal expansion of the nighttime light.
Despite the language barrier (Amano et al., 2016), the collection of in situ observed data and ecophysiological information in each country and region (especially non-English data and information; Nagai et al., 2016b; Takeuchi et al., 2021) will accelerate the development of integrative analysis and evaluation of in situ and satellite-observed data. As noted by Farley et al. (2018), researchers should aim to conduct more big data analyses by integrating citizen science, which has superior veracity; real-time sensor networks, which have superior velocity; in situ observed data collected by scientists, which have superior variety; and remote-sensing, which has a superior volume. The support of international scientific networking communities such as the Asia-Pacific Biodiversity Observation Network (Takeuchi et al., 2021)20, the Asia-Oceania GEO, 21 and the East Asia and Pacific International Long-Term Ecological Research Network (Kim et al., 2018)22 is indispensable for the development of these integrated studies. Takeuchi et al. (2021) emphasized the necessity of satellite observations that provide the academic perspectives and evidence needed to implement natural ecosystem conservation policies. We further encourage the use of in situ observed data to improve the accuracy and precision of analyses of satellite observations and reinforcing the networking of research communities working with in situ and satellite observations in the Asian tropics (Dronova and Taddeo, 2022; Shin et al., 2023).
Our discussions in this perspective paper can be summarized that future advances in the optical sensors on board satellites with high frequency (≤ 10 min) and high spatial resolution (≤ 10 m) are expected to deepen our understanding of ecosystems in the Asian tropics, thus improving our knowledge of phenological changes as well as land-cover and land-use changes due to anthropogenic activities and climate change. Consequently, we could deeply understand the temporal change of the friction between people and ecosystems in the Asian tropics (i.e., degree of the unsustainable circumstances) under societal and climate changes. Despite unclear phenology with a high biodiversity as well as high heterogeneity of land cover and land use, the day is undoubtedly coming when we can monitor tropical ecosystems in Asia even at the individual tree scale. Now, we are in the beginning of a new era of satellite remote-sensing.
NS designed the study. NS, CK, TM, and KI collected and analyzed the data. NS, TM, and YT wrote the manuscript. All authors contributed to critical manuscript revision and read and approved the submitted version.
This research was supported by the Global Change Observation Mission (ER2GCF104) of the Japan Aerospace Exploration Agency and KAKENHI grant (21H02229, 21H05178, and 22K12352) from the Japan Society for the Promotion of Science.
We thank Dr. Eko Siswanto (JAMSTEC) for providing useful information. We thank Ms. Mika Takeuchi (JAMSTEC) for assistance of analysis. We also thank the editor and reviewers for their constructive comments.
The authors declare that the research was conducted in the absence of any commercial or financial relationships that could be construed as a potential conflict of interest.
All claims expressed in this article are solely those of the authors and do not necessarily represent those of their affiliated organizations, or those of the publisher, the editors and the reviewers. Any product that may be evaluated in this article, or claim that may be made by its manufacturer, is not guaranteed or endorsed by the publisher.
Alberton, B., Torres, R. S., Cancian, L. F., Borges, B. D., Almeida, J., Mariano, G. C., et al. (2017). Introducing digital cameras to monitor plant phenology in the tropics: Applications for conservation. Perspect. Ecol. Conserv. 15, 82–90.
Amano, T., González-Varo, J. P., and Sutherland, W. J. (2016). Languages are still a major barrier to global science. PLoS Biol. 14:e2000933. doi: 10.1371/journal.pbio.2000933
Asner, G. P., Martin, R. E., Anderson, C. B., and Knapp, D. E. (2015). Quantifying forest canopy traits: Imaging spectroscopy versus field survey. Remote Sens. Environ. 158, 15–27.
Avtar, R., Suzuki, R., and Sawada, H. (2014). Natural forest biomass estimation based on plantation information using PALSAR data. PLoS One 9:e86121. doi: 10.1371/journal.pone.0086121
Azmy, M. M., Hashim, M., Numata, S., Hosaka, T., Noor, N. S. M., and Fletcher, C. (2016). Satellite-based characterization of climatic conditions before large- scale general flowering events in Peninsular Malaysia. Sci. Rep. 6:32329. doi: 10.1038/srep32329
Blicher-Mathiesen, U. (1994). Borneo Illipe, a fat product from different Shorea spp. (Dipterocarpaceae). Econ. Bot. 48, 231–242. doi: 10.1007/BF02862321
Buitenwerf, R., Rose, L., and Higgins, S. I. (2015). Three decades of multi-dimensional change in global leaf phenology. Nat. Clim. Change 5, 364–368. doi: 10.1038/NCLIMATE2533
Carlson, K. M., Curran, L. M., Ponette-González, A. G., Ratnasari, D., Ruspita, Lisnawati, N., et al. (2014). Influence of watershed-climate interactions on stream temperature, sediment yield, and metabolism along a land use intensity gradient in Indonesian Borneo. J. Geophys. Res. Biogeosci. 119, 1110–1128. doi: 10.1002/2013JG002516
Chang, S., Wang, Z., Mao, D., Liu, F., Lai, L., and Yu, H. (2021). Identifying urban functional areas in China’s Changchun city from Sentinel-2 images and social sensing data. Remote Sens. 13:4512. doi: 10.3390/rs13224512
Chechina, M., and Hamann, A. (2019). Climatic drivers of dipterocarp mass-flowering in South-East Asia. J. Trop. Ecol. 35, 108–117.
De Frenne, P., Van Langenhove, L., Van Driessche, A., Bertrand, C., Verheyen, K., and Vangansbeke, P. (2018). Using archived television video footage to quantify phenology responses to climate change. Methods Ecol. Evol. 9, 1874–1882. doi: 10.1111/2041-210X.13024
Delbart, N., Beaubien, E., Kergoat, L., and Le Toan, T. (2015). Comparing land surface phenology with leafing and flowering observations from the PlantWatch citizen network. Remote Sens. Environ. 160, 273–280. doi: 10.1016/j.rse.2015.01.012
Delbart, N., Le Toan, T., Kergoat, L., and Fedotova, V. (2006). Remote sensing of spring phenology in boreal regions: A free of snow-effect method using NOAA–AVHRR and SPOT–VGT data (1982–2004). Remote Sens. Environ. 101, 52–62.
Díaz, S., Pascual, U., Stenseke, M., Martín-López, B., Watson, R. T., Molnár, Z., et al. (2018). Assessing nature’s contributions to people. Science 359, 270–272.
Dronova, I., and Taddeo, S. (2022). Remote sensing of phenology: Towards the comprehensive indicators of plant community dynamics from species to regional scales. J. Ecol. 110, 1460–1484. doi: 10.1111/1365-2745.138
Elvidge, C. D., Baugh, K., Zhizhin, M., Hsu, F. C., and Ghosh, T. (2017). VIIRS night-time lights. Int. J. Remote Sens. 38, 5860–5879. doi: 10.1080/01431161.2017.1342050
Elvidge, C. D., Zhizhin, M., Ghosh, T., Hsu, F.-C., and Taneja, J. (2021). Annual time series of global VIIRS nighttime lights derived from monthly averages: 2012 to 2019. Remote Sens. 13:922. doi: 10.3390/rs13050922
Erasmi, S., Propastin, P., Kappas, M., and Panferov, O. (2009). Spatial patterns of NDVI variation over Indonesia and their relationship to ENSO warm events during the period 1982–2006. J. Clim. 22, 6612–6623.
Erasmi, S., Schucknecht, A., Barbosa, M. P., and Matschullat, J. (2014). Vegetation greenness in Northeastern Brazil and its relation to ENSO warm events. Remote Sens. 6, 3041–3058. doi: 10.3390/rs6043041
Estoque, R. C., Ooba, M., Avitabile, V., Hijioka, Y., DasGupta, R., Togawa, T., et al. (2019). The future of Southeast Asia’s forests. Nat. Commun. 10:1829. doi: 10.1038/s41467-019-09646-4
FAO, and UNEP (2020). The state of the world’s forests 2020. Forests, biodiversity and people. Rome: FAO. doi: 10.4060/ca8642en
Farley, S. S., Dawson, A., Goring, S. J., and Williams, J. W. (2018). Situating ecology as a big-data science: Current advances, challenges, and solutions. BioScience 68, 563–576. doi: 10.1093/biosci/biy068
Fernández-Bellon, D., and Kane, A. (2020). Natural history films raise species awareness–a big data approach. Conserv. Lett. 13:e12678. doi: 10.1111/conl.12678
Fitzherbert, E. B., Struebig, M. J., Morel, A., Danielsen, F., Brühl, C. A., Donald, P. F., et al. (2008). How will oil palm expansion affect biodiversity? Trends Ecol. Evol. 23, 538–545.
Fujii, K., Shibata, M., Kitajima, K., Ichie, T., Kitayama, K., and Turner, B. L. (2018). Plant–soil interactions maintain biodiversity and functions of tropical forest ecosystems. Ecol. Res. 33, 149–160. doi: 10.1007/s11284-017-1511-y
Funada, S., and Tsutsumida, N. (2022). Mapping cherry blossoms from geotagged street-level photos. bioRxiv [Preprint]. doi: 10.1101/2022.01.18.476550
Gao, M., Piao, S., Chen, A., Yang, H., Liu, Q., Fu, Y. H., et al. (2019). Divergent changes in the elevational gradient of vegetation activities over the last 30 years. Nat. Commun. 10:2970. doi: 10.1038/s41467-019-11035-w
Garonna, I., De Jong, R., De Wit, A. J. W., Mücher, C. A., Schmid, B., and Schaepman, M. E. (2014). Strong contribution of autumn phenology to changes in satellite-derived growing season length estimates across Europe (1982–2011). Glob. Change Biol. 20, 3457–3470. doi: 10.1111/gcb.12625
GEAS (2011). Oil palm plantations: Threats and opportunities for tropical ecosystems. Nairobi: UNEP Global Environmental Alter Service, 8.
Gray, R. E. J., and Ewers, R. M. (2021). Monitoring forest phenology in a changing world. Forests 12:297. doi: 10.3390/f12030297
Gregg, W. W., and Casey, N. M. (2004). Global and regional evaluation of the SeaWiFS chlorophyll data set. Remote Sens. Environ. 93, 463–479. doi: 10.1016/j.rse.2003.12.012
Groom, S., Sathyendranath, S., Ban, Y., Bernard, S., Brewin, R., Brotas, V., et al. (2019). Satellite ocean colour: Current status and future perspective. Front. Mar. Sci. 6:485. doi: 10.3389/fmars.2019.00485
Hansen, M. C., Potapov, P. V., Moore, R., Hancher, M., Turubanova, S. A., Tyukavina, A., et al. (2013). High-resolution global maps of 21st-century forest cover change. Science 342, 850–853.
Harrison, R. D. (2001). Drought and the consequences of El Niño in Borneo: A case study of figs. Popul. Ecol. 43, 63–75.
Hirayama, S., Tadono, T., Ohki, M., Mizukami, Y., Nasahara, K. N., Imamura, K., et al. (2022). Generation of high-resolution land use and land cover maps in JAPAN Version 21.11. J. Remote Sens. Soc. Jpn. 42, 199–216. doi: 10.11440/rssj.42.199
Hoang, T. T., Truong, V. T., Hayashi, M., Tadono, T., and Nasahara, K. N. (2020). New JAXA high-resolution land use/land cover map for Vietnam aiming for natural forest and plantation forest monitoring. Remote Sens. 12:2707.
Ichikawa, M. (2007). Degradation and loss of forest land and land-use changes in Sarawak, East Malaysia: A study of native land use by the Iban. Ecol. Res. 22, 403–413. doi: 10.1007/s11284-007-0365-0
Imukova, K., Ingwersen, J., and Streck, T. (2015). Determining the spatial and temporal dynamics of the green vegetation fraction of croplands using high-resolution RapidEye satellite images. Agric. For. Meteorol. 206, 113–123. doi: 10.1016/j.agrformet.2015.03.003
Inoue, T., Nagai, S., Saitoh, T. M., Muraoka, H., Nasahara, K. N., and Koizumi, H. (2014). Detection of the different characteristics of year-to-year variation in foliage phenology among deciduous broad-leaved tree species by using daily continuous canopy surface images. Ecol. Inform. 22, 58–68.
IPCC (2021). “Summary for policymakers,” in Climate change 2021: The physical science basis. Contribution of working group I to the sixth assessment report of the intergovernmental panel on climate change, eds V. Masson-Delmotte, P. Zhai, A. Pirani, S. L. Connors, C. Péan, S. Berger, et al. (Cambridge: Cambridge University Press). doi: 10.1017/9781009157896
Ishida, A., Toma, T., and Marjenah (2005). A comparison of in situ leaf photosynthetis and chlorophyll fluorescence at the top canopies in rainforest mature trees. JARQ 39, 57–67. doi: 10.6090/jarq.39.57
Ishihara, M., and Tadano, T. (2017). Land cover changes induced by the great east Japan earthquake in 2011. Sci. Rep. 7:45769. doi: 10.1038/srep45769
Ivan, K., Holobâcã, I.-H., Benedek, J., and Török, I. (2020). VIIRS nighttime light data for income estimation at local level. Remote Sens. 12:2950. doi: 10.3390/rs12182950
Iwao, K., Nasahara, K. N., Kinoshita, T., Yamagata, Y., Patton, D., and Tsuchida, S. (2011). Creation of new global land cover map with map integration. J. Geogr. Inf. Syst. 3, 160–165.
Jin, H., Jönsson, A. M., Olsson, C., Lindström, J., Jönsson, P., and Eklundh, L. (2019). New satellite-based estimates show significant trends in spring phenology and complex sensitivities to temperature and precipitation at northern European latitudes. Int. J. Biometeorol. 63, 763–775. doi: 10.1007/s00484-019-01690-5
John, A., Ong, J., Theobald, E. J., Olden, J. D., Tan, A., and HilleRisLambers, J. (2020). Detecting montane flowering phenology with CubeSat imagery. Remote Sens. 12:2894. doi: 10.3390/rs12182894
Kenzo, T., Ichie, T., Watanabe, Y., Yoneda, R., Ninomiya, I., and Koike, T. (2006). Changes in photosynthesis and leaf characteristics with tree height in five dipterocarp species in a tropical rain forest. Tree Physiol. 26, 865–873. doi: 10.1093/treephys/26.7.865
Kenzo, T., Ichie, T., Yoneda, R., Kitahashi, Y., Watanabe, Y., Ninomiya, I., et al. (2004). Interspecific variation of photosynthesis and leaf characteristics in five canopy trees of Dipterocarpaceae in tropical rain forest. Tree Physiol. 24, 1187–1192. doi: 10.1093/treephys/24.10.1187
Kenzo, T., Inoue, Y., Yoshimura, M., Yamashita, M., Tanaka-Oda, A., and Ichie, T. (2015). Height-related changes in leaf photosynthetic traits in diverse Bornean tropical rain forest trees. Oecologia 177, 191–202. doi: 10.1007/s00442-014-3126-0
Kenzo, T., Yoneda, R., Matsumoto, Y., Alias, M. A., and Muhamad, N. M. (2011). Growth and photosynthetic response of four Malaysian indigenous tree species under different light conditions. J. Trop. For. Sci. 23, 271–281.
Kikuzawa, K. (2005). Ecology of leaf longevity: From individual leaves to ecosystems. Tokyo: Kyoritsu Shuppan Co., Ltd, 212.
Kikuzawa, K., Onoda, Y., Wright, I. J., and Reich, P. B. (2013). Mechanisms underlying global temperature-related patterns in leaf longevity. Glob. Ecol. Biogeogr. 22, 982–993.
Kim, E. S., Trisurat, Y., Muraoka, H., Shibata, H., Amoroso, V., Boldgiv, B., et al. (2018). International long-term ecological research east asia-pacific regional network (ILTER-EAP): History, development and perspectives. Ecol. Res. 33, 19–34. doi: 10.1007/s11284-017-1523-7
Kobayashi, H., Yunus, A. P., Nagai, S., Sugiura, K., Kim, Y., Dam, B. V., et al. (2016). Latitudinal gradient of spruce forest understory and tundra phenology in Alaska as observed from satellite and ground-based data. Remote Sens. Environ. 177, 160–170. doi: 10.1016/j.rse.2016.02.020
Koh, L. P., Miettinen, J., Liew, S. C., and Ghazoul, J. (2011). Remotely sensed evidence of tropical peatland conversion to oil palm. PNAS 108, 5127–5132. doi: 10.1073/pnas.1018776108
Kotani, A., Shin, N., Tei, S., Makarov, A., and Gavrilyeva, T. (2021). Seasonality in human interest in berry plants detection by google trends. Front. For. Glob. Change 4:688835. doi: 10.3389/ffgc.2021.688835
Kou, W., Xiao, X., Dong, J., Gan, S., Zhai, D., Zhang, G., et al. (2015). Mapping deciduous rubber plantation areas and stand ages with PALSAR and Landsat images. Remote Sens. 7, 1048–1073. doi: 10.3390/rs70101048
Kumagai, T., Kanamori, H., and Yasunari, T. (2013). Deforestation-induced reduction in rainfall. Hydrol. Process 27, 3811–3814. doi: 10.1002/hyp.10060
Lautenbach, S., Seppelt, R., Liebscher, J., and Dormann, C. F. (2012). Spatial and temporal trends of global pollination benefit. PLoS One 7:e35954. doi: 10.1371/journal.pone.0035954
Leach, N., Coops, N. C., and Obrknezev, N. (2019). Normalization method for multi-sensor high spatial and temporal resolution satellite imagery with radiometric inconsistencies. Comput. Electron. Agric. 164:104893. doi: 10.1016/j.compag.2019.104893
Lee, H. S., Ashton, P. S., Yamakura, T., Tan, S., Davies, S. J., Itoh, A., et al. (2002). The 52-hectare forest research plot at Lambir Hills, Sarawak, Malaysia: Tree distribution maps, diameter tables and species documentation. Forest Department Sarawak and the Arnold arboretum-CTFS Asia program. Kuching: Forest Department Sarawak.
Li, L., Dong, J., Tenku, S. N., and Xiao, X. (2015). Mapping oil palm plantations in Cameroon using PALSAR 50-m orthorectified mosaic images. Remote Sens. 7, 1206–1224. doi: 10.3390/rs70201206
Li, P., Zhang, J., and Feng, Z. (2015). Mapping rubber tree plantations using a Landsat-based phenological algorithm in Xishuangbanna, southwest China. Remote Sens. Lett. 6, 49–58. doi: 10.1080/2150704X.2014.996678
Lopes, A. P., Nelson, B. W., Wu, J., Graça, P. M. L. A., Tavares, J. V., Prohaska, N., et al. (2016). Leaf flush drives dry season green-up of the Central Amazon. Remote Sens. Environ. 182, 90–98. doi: 10.1016/j.rse.2016.05.009
Luke, P., Stenberg, P., Rautiainen, M., Mttus, M., and Vanhatalo, K. M. (2013). Optical properties of leaves and needles for boreal tree species in Europe. Remote Sens. Lett. 4, 667–676. doi: 10.1080/2150704X.2013.782112
Marlier, M. E., DeFries, R. S., Kim, P. S., Gaveau, D. L. A., Koplitz, S. N., Jacob, D. J., et al. (2015). Regional air quality impacts of future fire emissions in Sumatra and Kalimantan. Environ Res. Lett. 10:054010. doi: 10.1046/j.1440-1843.2000.00248.x
Matsuoka, A., Campbell, J. W., Hooker, S. B., Steinmetz, F., Ogata, K., Hirata, T., et al. (2021). Performance of JAXA’s SGLI standard ocean color products for oceanic to coastal waters: Chlorophyll a concentration and light absorption coefficients of colored dissolved organic matter. J. Oceanogr. 93, 187–208. doi: 10.1007/s10872-021-00617-2
Miettinen, J., and Liew, S. C. (2011). Separability of insular Southeast Asian woody plantation species in the 50 m resolution ALOS PALSAR mosaic product. Remote Sens. Lett. 2, 299–307.
Miettinen, J., Shi, C., and Liew, S. C. (2011). Deforestation rates in insular Southeast Asia between 2000 and 2010. Glob. Change Biol. 17, 2261–2270.
Miura, T., and Nagai, S. (2020). Landslide detection with Himawari-8 geostationary satellite data: A case study of a torrential rain event in Kyushu, Japan. Remote Sens. 12:1734. doi: 10.3390/rs12111734
Miura, T., Nagai, S., Takeuchi, M., Ichii, K., and Yoshioka, H. (2019). Improved characterisation of vegetation and land surface seasonal dynamics in central Japan with Himawari-8 hypertemporal data. Sci. Rep. 9:15692. doi: 10.1038/s41598-019-52076-x
Miura, T., Tokumoto, Y., Shin, N., Shimizu, K. K., Pungga, R. A. S., and Ichie, T. (2023). Utility of commercial high-resolution satellite imagery for monitoring general flowering in Sarawak, Borneo. Ecol. Res. doi: 10.1111/1440-1703.12382
Moon, M., Richardson, A. D., and Friedl, M. A. (2021). Multiscale assessment of land surface phenology from harmonized landsat 8 and sentinel-2, PlanetScope, and PhenoCam imagery. Remote Sens. Environ. 266:112716. doi: 10.1016/j.rse.2021.112716
Morellato, L. P. C., Alberton, B., Alvarado, S. T., Borges, B., Buisson, E., Camargo, M. G. G., et al. (2016). Linking plant phenology to conservation biology. Biol. Conserv. 195, 60–72.
Morisette, J. T., Richardson, A. D., Knapp, A. K., Fisher, J. I., Graham, E. A., Abatzoglou, J., et al. (2009). Tracking the rhythm of the seasons in the face of global change: Phenological research in the 21st century. Front. Ecol. Environ. 7:253–260. doi: 10.1890/070217
Morozumi, T., Shingubara, R., Murase, J., Nagai, S., Kobayashi, H., Takano, S., et al. (2019). Usability of water surface reflectance for the determination of riverine dissolved methane during extreme flooding in northeastern Siberia. Polar Sci. 21, 186–194. doi: 10.1016/j.polar.2019.01.005
Motohka, T., Nasahara, K. N., Oguma, H., and Tsuchida, S. (2010). Applicability of green-red vegetation index for remote sensing of vegetation phenology. Remote Sens. 2, 2369–2387.
Muraoka, H., Ishii, R., Nagai, S., Suzuki, R., Motohka, T., Noda, H., et al. (2012). “Linking remote sensing and in situ ecosystem/biodiversity observations by “satellite ecology,” in Biodiversity observation network in Asia-Pacific region, eds S. Nakano, T. Nakashizuka, and T. Yahara (Tokyo: Springer Verlag), 277–308.
Nagai, S., Akitsu, T., Saitoh, T. M., Busey, R. C., Fukuzawa, K., Honda, Y., et al. (2018). 8 million phenological and sky images from 29 ecosystems from the Arctic to the tropics: The phenological eyes network. Ecol. Res. 33, 1091–1092. doi: 10.1007/s11284-018-1633-x
Nagai, S., Nasahara, K. N., Akitsu, T. K., Saitoh, T. M., and Muraoka, H. (2020a). “Importance of the collection of abundant ground-truth data for accurate detection of spatial and temporal variability of vegetation by satellite remote sensing,” in Biogeochemical cycles: Ecological drivers and environmental impact. Geophysical monograph, Vol. 251, eds K. Dontsova, Z. Balogh-Brunstad, and G. Le Roux (New York, NY: John Wiley and Sons, Inc). doi: 10.1002/9781119413332.ch11
Nagai, S., Endo, T., and Nasahara, K. N. (2020b). Phenology observations in Institute for Nature Study by fine spatio-temporal resolution satellite: SENTINEL-2A/B. Miscell. Rep. Inst. Nat. Stud. 52, 19–24.
Nagai, S., Ichie, T., Yoneyama, A., Kobayashi, H., Inoue, T., Ishii, R., et al. (2016a). Usability of time-lapse digital camera images to detect characteristics of tree phenology in a tropical rainforest. Ecol. Inform. 32, 91–106.
Nagai, S., Nasahara, K. N., Inoue, T., Saitoh, T. M., and Suzuki, R. (2016b). Review: Advances in in situ and satellite phenological observations in Japan. Int. J. Biometeorol. 60, 615–627. doi: 10.1007/s00484-015-1053-3
Nagai, S., Ishii, R., Suhaili, A., Kobayashi, H., Matsuoka, M., Ichie, T., et al. (2014a). Usability of noise-free daily satellite-observed green–red vegetation index values for monitoring ecosystem changes in Borneo. Int. J. Remote Sens. 35, 7910–7926.
Nagai, S., Inoue, T., Ohtsuka, T., Kobayashi, H., Kurumado, K., Muraoka, H., et al. (2014b). Relationship between spatio-temporal characteristics of leaf-fall phenology and seasonal variations in near surface–and satellite-observed vegetation indices in a cool-temperate deciduous broad-leaved forest in Japan. Int. J. Remote Sens. 35, 3520–3536.
Nagai, S., Saitoh, T. M., and Yoshitake, S. (2019). Cultural ecosystem services provided by flowering of cherry trees under climate change: A case study of the relationship between the periods of flowering and festivals. Int. J. Biometeorol. 63, 1051–1058. doi: 10.1007/s00484-019-01719-9
Nagai, S., Saitoh, T. M., Suzuki, R., Nasahara, K. N., Lee, W.-K., Son, Y., et al. (2011). The necessity and availability of noise-free daily satellite-observed NDVI during rapid phenological changes in terrestrial ecosystems in East Asia. For. Sci. Tech. 7, 174–183.
Najib, N. E. M., Kanniah, K. D., Cracknell, A. P., and Yu, L. (2020). Synergy of active and passive remote sensing data for effective mapping of oil palm plantation in Malaysia. Forests 11:858. doi: 10.3390/f11080858
Nakagawa, M., Ushio, M., Kume, T., and Nakashizuka, T. (2019). Seasonal and long-term patterns in litterfall in a Bornean tropical rainforest. Ecol. Res. 34, 31–39. doi: 10.1111/1440-1703.1003
Nakaji, T., Kosugi, Y., Takanashi, S., Niiyama, K., Noguchi, S., Tani, M., et al. (2014). Estimation of light-use efficiency through a combinational use of the photochemical reflectance index and vapor pressure deficit in an evergreen tropical rainforest at Pasoh, Peninsular Malaysia. Remote Sens. Environ. 150, 82–92.
Nasahara, K. N., and Nagai, S. (2015). Review: Development of an in situ observation network for terrestrial ecological remote sensing–the phenological eyes network (PEN). Ecol. Res. 30, 211–223.
Noda, H. M., Motohka, T., Murakami, K., Muraoka, H., and Nasahara, K. N. (2014). Reflectance and transmittance spectra of leaves and shoots of 22 vascular plant species and reflectance spectra of trunks and branches of 12 tree species in Japan. Ecol. Res. 29:111. doi: 10.1007/s11284-013-1096-z
Noda, H. M., Muraoka, H., and Nasahara, K. N. (2021). Plant ecophysiological processes in spectral profiles: Perspective from a deciduous broadleaf forest. J. Plant Res. 134, 737–751. doi: 10.1007/s10265-021-01302-7
Nomura, K., and Mitchard, E. T. A. (2018). More than meets the eye: Using sentinel-2 to map small plantations in complex forest landscapes. Remote Sens. 10:1693. doi: 10.3390/rs10111693
O’Reilly, J. E., Maritorena, S., Mitchell, B. G., Siegel, D. A., Carder, K. L., Garver, S. A., et al. (1998). Ocean color chlorophyll algorithms for SeaWiFS. J. Geophys. Res. 103, 24937–24953. doi: 10.1029/98JC02160
Onoda, Y., Westoby, M., Adler, P. B., Choong, A. M. F., Clissold, F. J., Cornelissen, J. H. C., et al. (2011). Global patterns of leaf mechanical properties. Ecol. Lett. 14, 301–312.
Osada, N. (2018). Diversity in leaf phenology of tree species in a tropical rain forest in Malaysia. Sci. Rep. Facul. Agricul. Meijo Univ. 54, 29–35.
Park, T., Ganguly, S., Tømmervik, H., Euskirchen, E. S., Høgda, K.-A., Karlsen, S. R., et al. (2016). Changes in growing season duration and productivity of northern vegetation inferred from long-term remote sensing data. Environ. Res. Lett. 11:084001.
Pennec, A., Gond, V., and Sabatier, D. (2011). Tropical forest phenology in French Guiana from MODIS time series. Remote Sens. Lett. 2, 337–345. doi: 10.1080/01431161.2010.507610
Penþuelas, J., Rutishauser, T., and Filella, I. (2009). Phenology feedbacks on climate change. Science 324, 887–888.
Persson, M., Lindberg, E., and Reese, H. (2018). Tree species classification with multi-temporal Sentinel-2 data. Remote Sens. 10:1794. doi: 10.3390/rs10111794
Pfeifer, M., Kor, L., Nilus, R., Turner, E., Cusack, J., Lysenko, I., et al. (2016). Mapping the structure of Borneo’s tropical forests across a degradation gradient. Remote Sens. Environ. 176, 84–97. doi: 10.1016/j.rse.2016.01.014
Piao, S., Liu, Q., Chen, A., Janssens, I. A., Fu, Y., Dai, J., et al. (2019). Plant phenology and global climate change: Current progresses and challenges. Glob. Change Biol. 25, 1922–1940. doi: 10.1111/gcb.14619
Pintér, G., and Felde, I. (2021). Analyzing the behavior and financial status of soccer fans from a mobile phone network perspective: Euro 2016, a case study. Information 12:468. doi: 10.3390/info12110468
Proulx, R., Massicotte, P., and Pépino, M. (2013). Googling trends in conservation biology. Conserv. Biol. 28, 44–51. doi: 10.1111/cobi.12131
Rahmandhana, A. D., Kamal, M., and Wicaksono, P. (2022). Spectral reflectance-based mangrove species mapping from WorldView-2 imagery of Karimunjawa and Kemujan Island, central Java province, Indonesia. Remote Sens. 14:183. doi: 10.3390/rs14010183
Reich, P. B., Uhl, C., Walters, M. B., Prugh, L., and Ellsworth, D. S. (2004). Leaf demography and phenology in Amazonian rain forest: A census of 40000 leaves of 23 tree species. Ecol. Monogr. 74, 3–23.
Richardson, A. D., Keenan, T. F., Migliavacca, M., Ryu, Y., Sonnentag, O., and Toomey, M. (2013). Climate change, phenology, and phenological control of vegetation feedbacks to the climate system. Agri. For. Meteorol. 169, 156–173.
Rohde, A. T., and Pilliod, D. S. (2021). Spatiotemporal dynamics of insect pollinator communities in sagebrush steppe associated with weather and vegetation. Glob. Ecol. Conserv. 29:e01691. doi: 10.1016/j.gecco.2021.e01691
Sakai, S., Harrison, R. D., Momose, K., Kuraji, K., Nagamasu, H., Yasunari, T., et al. (2006). Irregular droughts trigger mass flowering in aseasonal tropical forests in Asia. Amer. J. Bot. 93, 1134–1139. doi: 10.3732/ajb.93.8.1134
Sakurai, R., Jacobson, S. K., Kobori, H., Primack, R., Oka, K., Komatsu, N., et al. (2011). Culture and climate change: Japanese cherry blossom festivals and stakeholders’ knowledge and attitudes about global climate change. Biol. Conserv. 144, 654–658.
Schollaert, S. E., Yoder, J. A., O’Reilly, J. E., and Westphal, D. L. (2003). Influence of dust and sulfate aerosols on ocean color spectra and chlorophyll a concentrations derived from SeaWiFS off the U.S. east coast. J. Geophys. Res. 108:3191. doi: 10.1029/2000JC000555
Secades, C., O’Connor, B., Brown, C., and Walpole, M. (2014). Earth observation for biodiversity monitoring: A review of current approaches and future opportunities for tracking progress towards the Aichi biodiversity targets. Technical Series No. 72. Montréal, QC: Secretariat of the Convention on Biological Diversity, 183.
Secretariat of the Convention on Biological Diversity (2020). Global biodiversity outlook 5. Montréal, QC: Secretariat of the Convention on Biological Diversity, 208.
Segah, H., Tani, H., and Hirano, T. (2010). Detection of fire impact and vegetation recovery over tropical peat swamp forest by satellite data and ground-based NDVI instrument. Int. J. Remote Sens. 31, 5297–5314.
Shimada, M., Itoh, T., Motohka, T., Watanabe, M., Tomohiro, S., Thapa, R., et al. (2014). New global forest/non-forest maps from ALOS PALSAR data (2007–2010). Remote Sens. Environ. 155, 13–31.
Shin, N., Kotani, A., Maruya, Y., and Oishi, Y. (2022b). Data mining by watching old documentary TV programs to learn about the relationships between people’s lives and the landscape in Sakha in the perestroika era at the end of the 1980s. EarthArXiv [Preprint]. doi: 10.31223/X5KW79
Shin, N., Kotani, A., Maruya, Y., and Gavrilyeva, T. (2022a). Can Yandex statistics and google trends be used to detect people’s interests in berries in the Russian far east? Polar Sci. 33:100871. doi: 10.1016/j.polar.2022.100871
Shin, N., Saitoh, T. M., and Nasahara, K. N. (2021a). How did the characteristics of the growing season change during the past 100 years at a steep river basin in Japan? PLoS One 16:e0255078. doi: 10.1371/journal.pone.0255078
Shin, N., Maruya, Y., Saitoh, T. M., and Tsutsumida, N. (2021b). Usefulness of social sensing using text mining of tweets for detection of autumn phenology. Front. For. Glob. Change 4:659910. doi: 10.3389/ffgc.2021.659910
Shin, N., Saitoh, T. M., Takeuchi, Y., Miura, T., Aiba, M., Kurokawa, H., et al. (2023). Review: Monitoring of land cover changes and plant phenology by remote-sensing in East Asia. Ecol. Res. 38, 111–133. doi: 10.1111/1440-1703.12371
Shin, N., Shibata, H., Osawa, T., Yamakita, T., Nakamura, M., and Kenta, T. (2020). Toward more data publication of long-term ecological observations. Ecol. Res. 35, 700–707. doi: 10.1111/gcb.15123
Shinohara, M., and Nasahara, K. N. (2022). Utility of flowering signals in the detection of evergreen tree species with a mid-resolution optical satellite sensor. J. Remote Sens. Soc. Jpn. 42, 129–134.
Silva, S. J., Barbieri, L. K., and Thomer, A. K. (2018). Observing vegetation phenology through social media. PLoS One 13:e0197325. doi: 10.1371/journal.pone.0197325
Sims, D. A., and Gamon, J. A. (2002). Relationship between leaf pigment content and spectral reflectance across a wide range of species, leaf structures and developmental stages. Remote Sens. Environ. 81, 337–354.
Siswanto, E., and Tanaka, K. (2014). Phytoplankton biomass dynamics in the strait of Malacca within the period of the SeaWiFS full mission: Seasonal cycles, interannual variations and decadal-scale trends. Remote Sens. 6, 2718–2742. doi: 10.3390/rs6042718
Song, X. P., Richards, D. R., He, P., and Tan, P. Y. (2020). Does geo-located social media reflect the visit frequency of urban parks? A city-wide analysis using the count and content of photographs. Landsc. Urban Plan. 203:103908. doi: 10.1016/j.landurbplan.2020.103908
Soyama, N., Akitsu, T., and Temulun, T. (2017). Reference dataset production manual for the accuracy assessment of global land cover products using information from the degree confluence project. Overall Educa. Res. Center Bull. Tenri Univ. 15, 31–39.
Sparks, T. H. (2014). Local-scale adaptation to climate change: The village flower festival. Clim. Res. 60, 87–89.
Spessa, A. C., Field, R. D., Pappenberger, F., Langner, A., Englhart, S., Weber, U., et al. (2015). Seasonal forecasting of fire over Kalimantan, Indonesia. Nat. Hazards Earth Syst. Sci. 15, 429–442.
Stelmaszczuk-Górska, M. A., Urbazaev, M., Schmullius, C., and Thiel, C. (2018). Estimation of above-ground biomass over boreal forests on Siberia using updated in situ, ALOS-2 PALSAR-2, and RADARSAT-2 data. Remote Sens. 10:1550. doi: 10.3390/rs10101550
Takada, K. (2012). Japanese interest in “Hotaru” (Fireflies) and “Kabuto-Mushi” (Japanese rhinoceros beetles) corresponds with seasonality in visible abundance. Insects 3, 424–431. doi: 10.3390/insects3020424
Takahashi, A., Kumagai, T., Kanamori, H., Fujinami, H., and Hiyama, T. (2017). Impact of tropical deforestation and forest degradation on precipitation over Borneo Island. J. Hydrometeorol. 18, 2907–2922. doi: 10.1175/JHM-D-17-0008.1
Takata, Y., Watanabe, H., Yanagisawa, M., and Yamada, T. (2014). A visualization method of field notes based on locations and topic models. Jinmonkon 2014 ronbunsyu. Tokyo: Information Processing Society of Japan, 57–62.
Takeuchi, Y., Muraoka, H., Yamakita, T., Kano, Y., Nagai, S., Bunthang, T., et al. (2021). The Asia-Pacific biodiversity observation network: 10-year achievements and new strategies to 2030. Ecol. Res. 36, 232–257. doi: 10.1111/1440-1703.12212
Tanaka, Y., Minggat, E., and Roseli, W. (2021). The impact of tropical land-use change on downstream riverine and estuarine water properties and biogeochemical cycles: A review. Ecol. Process 10:40. doi: 10.1186/s13717-021-00315-3
Tang, J., Korner, C., Muraoka, H., Piao, S., Shen, M., Thackeray, S., et al. (2016). Emerging opportunities and challenges in phenology: A review. Ecosphere 7:e01436. doi: 10.1002/ecs2.1436
Tsutsumida, N., Nagai, S., Rodríguez-Veiga, P., Katagi, J., Nasahara, K., and Tadono, T. (2019). Mapping spatial accuracy of forest type classification in JAXA’s high-resolution land use and land cover map. ISPRS Ann. Photogr. Remote Sens. Spatial Inf. Sci. IV-3/W1, 57–63. doi: 10.5194/isprs-annals-IV-3-W1-57-2019
Ushio, M., Osada, Y., Kumagai, T., Kume, T., Pungga, R. S., Nakashizuka, T., et al. (2020). Dynamic and synergistic influences of air temperature and rainfall on general flowering in a Bornean lowland tropical forest. Ecol. Res. 35, 17–29. doi: 10.1111/1440-1703.12057
Visser, M. E., and Gienapp, P. (2019). Evolutionary and demographic consequences of phenological mismatches. Nat. Ecol. Evol. 3, 879–885. doi: 10.1038/s41559-019-0880-8
Vrieling, A., Meroni, M., Darvishzadeh, R., Skidmore, A. K., Wang, T., Zurita-Milla, R., et al. (2018). Vegetation phenology from Sentinel-2 and field cameras for a Dutch barrier island. Remote Sens. Environ. 215, 517–529.
Wang, J., Song, G., Liddell, M., Morellato, P., Lee, C. K. F., Yang, D., et al. (2023). An ecologically-constrained deep learning model for tropical leaf phenology monitoring using PlanetScope satellites. Remote Sens. Environ. 286:113429. doi: 10.1016/j.rse.2022.113429
Wang, J., Yang, D., Detto, M., Nelson, B. W., Chen, M., Guan, K., et al. (2020). Multi-scale integration of satellite remote sensing improves characterization of dry-season green-up in an Amazon tropical evergreen forest. Remote Sens. Environ. 246:111865. doi: 10.1016/j.rse.2020.111865
Wang, Q., Wang, L., Li, Z., Tong, X., and Atkinson, P. M. (2021). Spatial–spectral radial basis function-based interpolation for Landsat ETM+ SLC-off image gap filling. IEEE Trans. Geosci. Remote Sens. 59, 7901–7917.
Wooster, M. J., Perry, G. L. W., and Zoumas, A. (2012). Fire, drought and El Niño relationships on Borneo (Southeast Asia) in the pre-MODIS era (1980–2000). Biogeosciences 9, 317–340.
Wright, I. J., Reich, P. B., Westoby, M., Ackerly, D. D., Baruch, Z., Bongers, F., et al. (2004). The worldwide leaf economics spectrum. Nature 428, 821–827.
Wu, C., Gonsamo, A., Gough, C. M., Chen, J. M., and Xu, S. (2014). Modeling growing season phenology in North American forests using seasonal mean vegetation indices from MODIS. Remote Sens. Environ. 147, 79–88. doi: 10.1016/j.rse.2014.03.001
Wu, J., Albert, L. P., Lopes, A. P., Restrepo-Coupe, N., Hayek, M., Wiedemann, K. T., et al. (2016). Leaf development and demography explain photosynthetic seasonality in Amazon evergreen forests. Science 351:972. doi: 10.1126/science.aad5068
Wu, S., Wang, J., Yan, Z., Song, G., Chen, Y., Ma, Q., et al. (2021). Monitoring tree-crown scale autumn leaf phenology in a temperate forest with an integration of PlanetScope and drone remote sensing observations. ISPRS J. Photogr. Remote Sens. 171, 36–48. doi: 10.1016/j.isprsjprs.2020.10.017
Yamada, T. (2015). Characterizing scenes in field note: Knowledge processing using vocabulary. Joho Chishiki Gakkaishi 25, 315–324.
Yamamoto, Y., Fujino, T., and Kubota, T. (2015). First book in data mining using R. Tokyo: Ohmsha, 230.
Yan, D., Zhang, X., Nagai, S., Yu, Y., Akitsu, T., Nasahara, K. N., et al. (2019). Evaluating land surface phenology from the advanced Himawari imager using observations from MODIS and the phenological eyes network. Int. J. Appl. Earth Observ. Geoinf. 79, 71–83.
Yoshimura, N., and Hiura, T. (2017). Demand and supply of cultural ecosystem services: Use of geotagged photos to map the aesthetic value of landscapes in Hokkaido. Ecosyst. Serv. 24, 68–78. doi: 10.1016/j.ecoser.2017.02.009
Keywords: deforestation, general flowering event, geostationary satellite (GEO), optical sensor, cloud contamination, Sarawak (Malaysia), Sumatra
Citation: Shin N, Katsumata C, Miura T, Tsutsumida N, Ichie T, Kotani A, Nakagawa M, Khoon KL, Kobayashi H, Kumagai T, Tei S, Pungga RaS, Yamada T, Kameda A, Yanagisawa M, Nasahara KN, Muraoka H, Ichii K and Tokumoto Y (2023) Perspective: Improving the accuracy of plant phenology observations and land-cover and land-use detection by optical satellite remote-sensing in the Asian tropics. Front. For. Glob. Change 6:1106723. doi: 10.3389/ffgc.2023.1106723
Received: 24 November 2022; Accepted: 08 February 2023;
Published: 22 February 2023.
Edited by:
Nophea Sasaki, Asian Institute of Technology, ThailandReviewed by:
Richard T. Corlett, Xishuangbanna Tropical Botanical Garden (CAS), ChinaCopyright © 2023 Shin, Katsumata, Miura, Tsutsumida, Ichie, Kotani, Nakagawa, Khoon, Kobayashi, Kumagai, Tei, Pungga, Yamada, Kameda, Yanagisawa, Nasahara, Muraoka, Ichii and Tokumoto. This is an open-access article distributed under the terms of the Creative Commons Attribution License (CC BY). The use, distribution or reproduction in other forums is permitted, provided the original author(s) and the copyright owner(s) are credited and that the original publication in this journal is cited, in accordance with accepted academic practice. No use, distribution or reproduction is permitted which does not comply with these terms.
*Correspondence: Nagai Shin, bmFnYWlzQGphbXN0ZWMuZ28uanA=
†These authors have contributed equally to this work
‡ORCID: Narumasa Tsutsumida, orcid.org/0000-0002-6333-0301; Tomo’omi Kumagai, orcid.org/0000-0001-8331-271X; Runi anak Sylvester Pungga, orcid.org/0000-0002-9877-2791; Taizo Yamada, orcid.org/0000-0002-0170-8380; Akihiro Kameda, orcid.org/0000-0002-5439-6456; Kenlo Nishida Nasahara, orcid.org/0000-0003-2646-6805; Yujib Tokumotoorcid.org/0000-0001-5934-026X
Disclaimer: All claims expressed in this article are solely those of the authors and do not necessarily represent those of their affiliated organizations, or those of the publisher, the editors and the reviewers. Any product that may be evaluated in this article or claim that may be made by its manufacturer is not guaranteed or endorsed by the publisher.
Research integrity at Frontiers
Learn more about the work of our research integrity team to safeguard the quality of each article we publish.