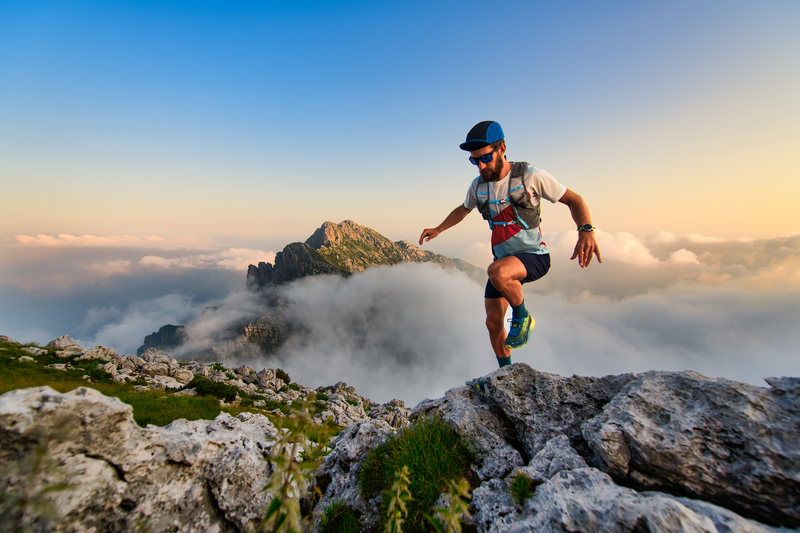
95% of researchers rate our articles as excellent or good
Learn more about the work of our research integrity team to safeguard the quality of each article we publish.
Find out more
ORIGINAL RESEARCH article
Front. Ecol. Evol. , 09 October 2023
Sec. Biogeography and Macroecology
Volume 11 - 2023 | https://doi.org/10.3389/fevo.2023.1229123
This article is part of the Research Topic Interpretation and Implications of Variability in Ecological Systems View all 13 articles
A correction has been applied to this article in:
Corrigendum: Spatially explicit models of seed availability improve predictions of conifer regeneration following the 2018 Carr Fire in northern California
For many conifer species in dry conifer forests of North America, seeds must be present for postfire regeneration to occur, suggesting that seed dispersal from surviving trees plays a critical role in postfire forest recovery. However, the application of tree fecundity and spatial arrangement to postfire conifer recovery predictions have only recently become more common, and is often included at relatively coarse scales (i.e., 30 meters). In this study, we mapped surviving trees using lidar and created a spatially explicit estimate of seed density (seed shadows) with 10 m, 50 m, and 100 m median dispersal distances. We estimated the number of seeds produced by each tree using allometric relationships between tree size and fecundity. Along with the seed shadows, we used a suite of topographic variables as inputs to negative binomial hurdle models to predict conifer seedling abundance in 131 plots following the 2018 Carr Fire in northern California, USA. We compared models using each of the seed shadows to each other as well as to a model using the distance to the nearest surviving tree, which served as a baseline. All model formulations indicated that estimated seed availability was positively associated with conifer regeneration. Despite the importance of seed availability plays in regeneration and the substantial differences in seed availability represented by the different seed shadows in our analysis, we found surprisingly little difference in model performance regardless of which seed shadow was used. However, the models employing seed shadows outperformed the models with distance to the nearest live tree. Although we have demonstrated a modest improvement in predicting postfire conifer regeneration, the uncertainty in our results highlights the importance of tree detection and classification in future studies of this kind. Future studies may find it useful to consider other factors such as predation, site suitability, and seed mortality as potential drivers of discrepancies between total and realized dispersal kernels.
In recent years, wildfires in the western region of the U.S. have become more frequent, larger, and more severe (Stevens et al., 2017; Williams et al., 2019; Goss et al., 2020), and long-term warming and prolonged droughts due to climate change are projected to increase wildfire severity and length of season over the coming decades (Wehner et al., 2017). As fire size and severity has increased, so has the scale of tree mortality, with large, high severity patches becoming increasingly common (Collins et al., 2017; Williams et al., 2023). Therefore, there is an urgent need to understand the mechanisms of forest regeneration. Nearly all conifers in the western U.S. are obligate seeders, implying that natural reforestation following fire requires a locally available seed source, either from surviving trees or via adaptations such as serotinous cones or soil seed banks (Turner et al., 1998; Stevens-Rumann and Morgan, 2019). Many of the dominant species in the mixed conifer forests of northern California are wind dispersed and lack either serotinous cones or soil seed banks (Burns and Honkala, 1990), though serotinous knobcone pine Pinus attenuata (Lemmon) is found in the region (Reilly et al., 2019). In species lacking these adaptations, the probability of postfire seedling establishment for non-serotinous species typically declines with increasing distance from the nearest surviving tree because wind dispersed seeds are less likely to fall further from the source (Chambers et al., 2016; Coop et al., 2019). Most conifer seed dispersal occurs over relatively short distances, with most wind dispersed seeds falling within 100 m of the parent tree, though distances may vary by tree height (Greene and Johnson, 1989; Katul et al., 2005; Bullock et al., 2017). If seeds rarely travel more than 100m, this suggests that the time required for forests to naturally regenerate in the interior of large patches of high tree mortality may be substantially more than areas closer to the patch edge, as seed dispersal into areas further from the edge relies on infrequent long-distance dispersal or the maturation and subsequent reproduction from initial colonizers (Turner et al., 1998). This timeline may be extended further by competition from shrubs and hardwoods, which can more rapidly recolonize severely burned areas via resprouting or persistent soil seed banks (Knapp et al., 2012; Welch et al., 2016) and may compete with regenerating conifers (Collins and Roller, 2013; Crotteau et al., 2013; Tepley et al., 2017).
Seed availability is a critical component of the postfire recovery process (Gill et al., 2022). One common approach is to approximate seed availability with simple metrics such as distance to the nearest surviving tree or unburned forest edge, which are easy to measure and have a proven utility in predicting conifer regeneration (Chambers et al., 2016; Korb et al., 2019). However, these methods do not capture fine scale variation in seed availability, which may be especially important in areas that experienced high tree mortality but where there are multiple locations with surviving trees within the range of most seed dispersal. For example, the center of a circular patch of high tree mortality may receive substantially more seed than would be suggested by simply determining the nearest surviving tree, since the area would be receiving seed from all trees within dispersal range (Peeler and Smithwick, 2020). Simple distance models may also be less optimal when seed production capability varies across the landscape due to variations in tree size, density, or both. While recent efforts have been made to incorporate the fecundity and spatial arrangement of trees into predictions of postfire regeneration (Tepley et al., 2017; Shive et al., 2018; Downing et al., 2019; Peeler and Smithwick, 2020; Stewart et al., 2021), few studies have attempted to do so at the scale of individual trees (but see Landesmann and Morales, 2018).
Seed availability can be modeled using estimates for fecundity and dispersal. Dispersal functions (kernels) use the distance from the parent tree to create a spatially uniform (i.e., isotropic) distribution describing the probability a seed will fall to the ground a given distance from the source (Greene et al., 2004; Nathan et al., 2008; Bullock et al., 2017). When combined with allometrically derived estimates of annual seed production (fecundity) (Greene and Johnson, 1994), these distributions can be used to estimate seed availability at a given distance from a surviving tree or group of trees for a given time after a fire event. The combination of dispersal probability and fecundity is known as a seed shadow (Clark et al., 1999a). Seed shadows have been used to approximate seed availability across geographic space when modeling the probability of postfire conifer regeneration (Shive et al., 2018; Stewart et al., 2021). However, these recent modeling efforts have relied on moderate resolution data such as 30 m burn severity maps from Monitoring Trends in Burn Severity (MTBS, https://www.mtbs.gov/), which may obscure fine-scale variation in seed availability. Fine scale maps of surviving trees, such as those created using fine scale imagery or lidar data, may more accurately reflect the variation in seed source availability on the landscape, and thus more closely represent true seed availability (Barber et al., 2022).
In this study, we used data from one to three years after a severe wildlife event (the 2018 Carr Fire) at Whiskeytown National Recreation Area (WHIS) to assess the ability of seed shadows derived from high-resolution maps of individual trees to predict short-term postfire conifer regeneration. We also tested whether the models using seed shadows outperformed models using distance to the nearest live tree, assuming that the high performance and simplicity of the distance models makes them an appropriate baseline. In addition to estimates of seed availability, our models also included variables for topography and shrub cover to assess the influence of site characteristics on postfire conifer regeneration.
Whiskeytown National Recreation Area is located at the southeastern edge of the Klamath bioregion (Skinner et al., 2006) in Shasta and Trinity Counties, just west of the city of Redding in northern California. WHIS is over 17,000 hectares in size, and is characterized by steep topography and high biodiversity, with a wide variety of forest types including oak woodlands, knobcone pine woodlands, mixed conifer and yellow pine forests, with true fir (red and white) at high elevations (Fry and Stephens, 2006). Elevation in WHIS ranges from 250 to 1,890 meters above sea level. The park also includes large areas of shrubland and sparsely-treed woodlands, especially on the south-facing slopes north of Whiskeytown Lake.
Common conifer species include ponderosa pine (Pinus ponderosa), Jeffrey pine (Pinus jeffreyi), incense cedar (Calocedrus decurrens (Torr.) Floren.), Douglas fir, sugar pine (Pinus lambertiana Dougl.), and white fir (Abies concolor (Gord. & Glend.) Lindl.). Knobcone and gray pine (Pinus sabiniana) are common at low elevations, and some red fir (Abies magnifica var. shastensis) is found in the high elevations of the park. A variety of hardwood species are also found throughout the park, including California black oak (Quercus kelloggii Newb.), canyon live oak (Quercus chrysolepis Liebm.), tanoak (Notholithocarpus densiflorus), golden chinquapin (Chrysolepis chrysophylla), and dogwood (Cornus nuttallii Audubon). See Smith et al. (2021) for a detailed description of vegetation found in the park.
Fires were historically common in the area but decreased in frequency after 1850, with an almost complete absence of fire in the latter half of the 20th century following the widespread adoption of fire suppression (Fry and Stephens, 2006). However, fire has not been completely absent in the last few decades. Starting in the mid-1990s, the National Park Service introduced fuels reduction and other restoration projects, often involving the use of prescribed fire. In addition to these treatments, wildfire has also occurred within the park boundary. Most notably, the Shasta-Trinity Unit Lightning Complex Fire burned through the park in 2008, which burned 4% of the park area at high severity (MTBS, 2018).
The Carr Fire started on July 23rd, 2018, and actively burned for 38 days before containment on August 30th the same year (https://www.fire.ca.gov/incidents/2018/7/23/carr-fire). The fire burned nearly 93,000 hectares, including nearly the entire area of WHIS, destroyed over 1,000 homes in nearby communities, resulted in 8 human deaths, and had an estimated damage cost of >$1.6 billion (USD). The fire occurred under abnormally hot, dry, and windy conditions, and made significant runs when terrain and wind aligned, burning a large portion of WHIS in a single 24-hour period.
Our study focused on the yellow pine and mixed conifer forests of WHIS, which covered over 5,200 ha within the park prior to the Carr Fire (Figure 1). According to data produced by MTBS, over 65% of the conifer forests (3,450 ha) burned at high severity (Figure 1, MTBS, 2020). High severity patches were extensive, especially on the steep slopes of the Brandy, Boulder, and Mill Creek drainages.
Figure 1 (A) Location of forest plots, old growth, and conifer forests at Whiskeytown National Recreation Area. Forest types and old growth polygons were derived from park data products circa 2006. (B) Monitoring Trends in Burn Severity (MTBS) burn severity map with previous fire perimeters (pattern fill) overlayed. The inset shows the location of WHIS and the Klamath bioregion (in green). Klamath bioregion data downloaded from https://databasin.org/datasets/4996c7e61a0e48f2bef646903f51b82b.
We leveraged field data from two separate sources for this analysis. We used data from a preexisting network of 0.1 ha fire monitoring (FMH) plots in WHIS, which are designed to monitor changes in fuels and forest structure at random locations following fire (USDI National Park Service, 2003). We selected all plots where seedling density and overstory trees (>15 cm diameter at breast height or 1.37 m, hereafter DBH) were sampled after the Carr Fire, for a total of 23 plots. The FMH plot data captured species, DBH, and status for all overstory trees in the plot based on a 15 cm DBH threshold. The same information was collected for pole size trees (stems 15 cm DBH) in 0.025 ha subplots. The FMH plots also captured seedling tallies (stems 2.5 cm DBH) by size class, species, and status in a 50 m2 subplot (0.005 ha). The FMH plots also captured vegetation cover using two 50 m point intercept transects, where species and height of vegetation was recorded at 30 cm intervals for a total of 166 points per transect. We averaged the shrub cover estimates from both transects to get a plot level estimate of shrub cover.
In addition to the FMH plots, we established a total of 108 0.1 ha plots in the summers of 2020 and 2021 (Figure 1). We sampled plots over most of the elevation range of the park, with plot elevations ranging from 330 to 1,870 m. For most of these plots (n = 76) we used GIS to randomly select sampling locations within conifer forests according to the following criteria: no slopes > 50%, > 50 m and < 500 m from a road, 100 m from non-vegetated areas (e.g., Whiskeytown Lake), and at least 50 m from one another. The sampling locations were stratified across burn severity and predicted regeneration. Regeneration was predicted using the models developed in (Stewart et al., 2021) using the poscrptR R package (Wright et al., 2020). We used vegetation alliance polygons created for the National Park Service (Fox et al., 2006) to identify conifer forests by selecting polygons with Douglas fir, mixed conifer, ponderosa pine, or red fir forest alliance types. We established 12 of the plots specifically in random locations within unoccupied aerial survey (UAS) sampling areas to assist with future vegetation mapping projects using the same criteria described above, with the exception that plot locations were stratified by burn severity and whether the forest alliance was conifer (described above) or oak, including black oak, blue oak, canyon live oak, interior live oak, Oregon white oak, and tanoak forest and woodland alliances. We established an additional 20 plots to match earlier randomly sampled plot surveys of old-growth conifer forests (Leonzo and Keyes, 2010). Three of the original plots from Leonzo and Keyes (2010) could not be reached due to unsafe conditions, so we sampled three replacement plots using the same random sampling criteria outlined in Leonzo and Keyes (2010).
At each plot, we recorded DBH, species identity, and live and dead status for all standing trees > 15 cm DBH over a 0.1 ha circular plot. We measured sapling (stems 0.1 to 15.0 cm DBH) stem diameter, species location and status in a 0.011 ha subplot at the plot center. We also collected tallies of live seedlings (stems < 1.37 m in height) in the subplot, recording species and height class (0.1–5, 5–10, 11–25, 26–50, 51–75, 76–100, and 100–136 cm). On nine occasions the seedling subplot was reduced in size to 0.001 ha or 0.005 ha because the large numbers of seedlings present made larger subplot sizes impractical. We also visually estimated the cover of shrubs, forbs, and grasses within the plot area. Cover estimates were binned into the following classes: 0–1%, 2–5%, 6–25%, 26–50%, 51–75%, 76–95%, and 96–100%.
We normalized the data for each plot type (FMH and 0.1 ha field plots) by putting basal area and seedlings on a common per hectare scale and binning shrub cover into 25% class bins. Seedlings were not always identifiable beyond the genus level, and we could not determine the species of the lidar mapped trees from aerial imagery. Therefore, we report results for our analyses on seedling density for all conifers combined. We initially performed the analysis at the genus level, with broadly similar results. Plot sizes were similar between plot types, so we expected that any difference in seedling detection probability between plot types due to sampled area is likely to be small.
We mapped surviving tree locations using a combination of lidar and high-resolution imagery. The detection of individual trees from lidar works best on dominant trees whose crowns are clearly visible from above (Maltamo et al., 2004). WHIS is structurally diverse, with a substantial understory component (Leonzo and Keyes, 2010). Therefore, we assumed that trees detected using the lidar data are in reality “tree approximate objects” (North et al., 2017; Jeronimo et al., 2018) and may represent more than one individual tree, though we refer to them as individual trees for simplicity. Given the forest structure at WHIS, we likely underestimated surviving trees and their associated seed production. However, larger trees are of local interest (Leonzo and Keyes, 2010) and generally produce more seed (Greene and Johnson, 1994), so we expect that we have captured the overall spatial trends in tree survival and seed production, even where the absolute values deviate.
We developed a model for mapping individual tree mortality status across the park. We used the lidR R package (Roussel et al., 2020; Roussel and Auty, 2021) to create a 0.5 m resolution canopy height model for the Whiskeytown footprint (grid_canopy and p2r functions) from lidar collected in 2019 (8 points/m2, U.S. Geological Survey, 2021). We further processed the lidar to find individual tree points (locate_trees and lmf functions, 5 m moving window and 7 m height threshold) and crowns (dalponte2016 function, Dalponte and Coomes, 2016). We derived point cloud-based metrics for each crown following methods outlined in Marrs and Ni-Meister (2019). We resampled high resolution (12 inch) multispectral orthomosaic imagery (collected November 2018 for the National Park Service by Eagle Digital Imaging, Inc.) to 1 m resolution, which we then used to calculate the green normalized difference vegetation index (GNDVI; [NIR-green]/[NIR+green]). GNDVI was less sensitive to shadows than NDVI in our exploration of potential model predictors. We then extracted summary statistics of GNDVI for each crown. We generated points for live (n=609) and dead (n=758) trees from manual interpretation of the color infrared imagery and 2020 NAIP imagery (https://www.usgs.gov/centers/eros/science/usgs-eros-archive-aerial-photography-national-agriculture-imagery-program-naip). We used a parameter selection algorithm model (rf.modelSel from the rfUtilities package, parsimony=0.025, Murphy et al., 2010) to reduce the number of predictor variables for the final random forest model (randomForest R package, Breiman, 2001; Liaw et al., 2002). Seven GNDVI metrics and four lidar metrics were included in the final model (Table 1). We partitioned the data into 80/20% training/testing data sets to assess model performance. We used a random forest model with default parameters to classify trees by their mortality status.
The tree mortality status model performed well, with 93.4% overall accuracy, a Cohens’s Kappa of 0.87, sensitivity of 0.96, and specificity of 0.91. The model correctly identified live trees with an accuracy of 90.3% when we compared it to a separate validation data set of live and dead trees generated using UAS imagery (Thorne et al., 2023). Of the 2.23 million individual trees detected across WHIS, 77.1% were classified as dead. However, we noticed that there were occasional trees incorrectly classified as live within large high severity patches, likely due to the presence of shrubs or herbaceous vegetation within the tree crown footprint. Though these trees were likely to have little to no influence on many of our analyses, they would have a strong influence on calculating metrics such as distance to the nearest surviving tree. Based on this assumption, we used 2020 NAIP aerial imagery to manually check the mortality classification on all trees classified as alive that were greater than 70 m from the nearest other surviving tree (n=374); of these, 186 were manually reclassified as dead for further analyses, often because of the presence of resprouting shrubs underneath the dead tree. Of course, manually correcting these trees did not address incorrectly classified dead trees in these areas or any incorrectly classified trees in areas of greater tree density. Regardless, due to the outsize influence an isolated tree can have on the availability of seed, we felt that correcting the misclassified isolated trees was the more conservative approach.
Rather than misclassification, we assumed that the omission of smaller surviving trees would be the most likely error in areas of high tree density and stand complexity because tree detection is more difficult under these conditions (Jeronimo et al., 2018). Omitting small trees likely lead to a subsequent underestimation of available seed in these locations. However, large trees account for the most basal area and produce the most seed (Greene and Johnson, 1994), so we assume that the overall pattern of seed dispersal is maintained.
We estimated diameter for each mapped tree using a height-diameter allometric equation from (Parker and Evans, 2004), which we parameterized using data from the Klamath Inventory and Monitoring Network plots in WHIS (I&M, Odion et al., 2011). Some sampling variability has been observed in the I&M tree height measurements, so we averaged heights and diameters by tree from the 2012 and 2015 sample dates. We fit the models using the brms package (Bürkner, 2017) in R using a gamma likelihood with a log link. It has been shown that including other predictors such as crown diameter improves the predictive accuracy of height-diameter equations (Jucker et al., 2017). However, since we did not have access to field measurements of crown diameter, we elected to use the univariate model. Model predictions are shown in Supplementary Figure 1.
We produced isotropic kernels at 1m resolution for each of the mapped surviving trees (described above) using a lognormal dispersal function (Greene et al., 2004), shown below.
The values from the lognormal dispersal function represent an estimate of the probability of seed dispersal at a given distance ( in the equation above) from the parent tree on a two-dimensional plane. The parameters and determine the median dispersal distance and the standard deviation of the log distances, respectively (Greene et al., 2004).
We were unable to estimate the parameters in the kernel functions directly from our field data. Therefore, we calculated seed dispersal probability for three parameterizations for each kernel: short, medium, and long-distance, with the shape parameter held constant at one following recommendations in Greene et al. (2004) and scale parameter (median dispersal distance) at 10, 50, and 100m respectively (Figure 2). During preliminary analysis, we tried several other dispersal functions including the WALD, log-hyperbolic secant, exponential power, and inverse Gaussian, all of which had similar performance to the lognormal function. We selected these functions because they received good support in the literature (Katul et al., 2005; Bullock et al., 2017; but see Cousens et al., 2018).
Figure 2 Estimated seed density for each of the kernel function parameterizations for a hypothetical tree producing 10,000 seeds.
Larger trees are likely to produce more seeds (Greene and Johnson, 1994; Krannitz and Duralia, 2004), so we calculated the expected annual seed production of each tree using equations from Greene and Johnson (1994). We estimated the individual seed mass using average seeds per kilogram by species from Bonner and Karrfalt (2008), using regionally specific values where possible. Since we did not know the species for the lidar mapped trees, we created a weighted seed weight coefficient for each field plot based on the proportion of each species by basal area in the plot. We then used the median of the plot-level values as the seed coefficient for the fire. The equations in Greene and Johnson (1994) derive leaf mass from basal area, which we estimated from the modeled tree diameters described above. We converted the annual seed estimates for each tree location to a raster surface with 1m resolution and applied the lognormal dispersal function using a moving window function from the terra package in R (Hijmans, 2022) to create the seed shadows for each of the different kernel parameterizations. Finally, we extracted the estimated seed rain for each seedling subplot and converted it to the estimated total expected seed availability at the plot in seedlings per hectare by multiplying the predicted seed rain by the number of seed-producing seasons between when the fire was contained and when the plot was sampled. We assumed that the seed producing season was from September to December.
To visually demonstrate the variation in different kernel parameterizations, we plotted the seed shadows for the area 200 m surrounding a plot that burned at high severity with complete basal area mortality (Figure 3). The plot is >70 m from the nearest surviving tree, but with many surviving trees in the surrounding area, especially to the north and west. We subjectively selected the area surrounding this plot because there were few surviving trees in the area immediately surrounding the plot but many surviving trees nearby. This image shows the potential effect of different kernel parameterizations on estimated seed availability.
Figure 3 Orthophoto and maps of seed shadows for a 200 m area surrounding a plot in a high severity patch with extensive tree mortality. The plot area is shown as a white circle, plot area is to scale. Orthophoto shown in false color infrared. (A) 10 m median dispersal distance. (B) 50 m median dispersal distance. (C) 100 m median dispersal distance. (D) False color infrared orthophoto (National Park Service, unpublished data).
We calculated the distance to the nearest surviving tree to use as a comparison with expected seed availability at each plot. This estimate is not a seed shadow, though we assumed that it functioned as a proxy for the amount of seed available at the plot location.
We used whitebox tools (Wu, 2021) to estimate topographic wetness index from a 1 m-resolution lidar digital elevation model (DEM). First, we breached depressions to remove sinks. We then calculated D-infinity flow direction and flow accumulation for each cell, which we used to calculate topographic wetness index:
We calculated heat load index following methods outlined in McCune and Keon (2002) using the spatialEco package (Evans, 2021). We extracted the average heat load index, topographic wetness index, slope, and elevation from the 1 m DEM for the area of each seedling subplot.
We used the brms package (Bürkner, 2017) in R (R Core Team, 2021) to fit Bayesian generalized linear models estimating the influence of estimated seed availability, shrub cover, topographic wetness index, elevation, and heat load index on postfire conifer regeneration. There were several plots without seedlings, so we used negative binomial hurdle models to estimate both the influence of covariates on the probability of encountering no conifer seedlings as well as the effects on conifer seedling abundance, conditional on their being present (Steel et al., 2013). The model takes the following form:
Where is the negative binomial distribution, is the intercept, and are the regression coefficients for the predictor. The count portion of the model is a zero truncated negative binomial with a log link and shape parameter :
We used the same predictors in the hurdle and count portions of the model.
We fit separate models with different seed availability variables: three models with seed shadows derived using each of the three dispersal function parameterizations, and an additional model that used the distance to the nearest surviving tree. We standardized all continuous predictors by subtracting the mean and dividing by two standard deviations, which puts continuous and binary predictors on a common scale (Gelman, 2008). We used weakly informative priors in all models, where is the intercept, are the regression coefficients, and is the shape parameter of the negative binomial distribution:
We ran all models for 2,000 iterations and ensured that all values did not exceed 1.01. We checked the model fit using posterior predictive checks and leave-one-out cross-validation (LOO, Vehtari et al., 2017). We compared models using the expected log pointwise predictive density (), which estimates the predictive accuracy of the model for each data point held out during LOO (Vehtari et al., 2017). Models can be compared by differencing estimates (), and the standard error of can characterize the uncertainty in the model comparison. Generally, models with of less than four have similar predictive performance (Sivula et al., 2020). Here, we report the on the parameters for the model with the highest . We calculated mean absolute error (MAE) for each model as an additional measure of model performance. Along with conditional effects for the hurdle and count portions of the model, we report the probability of direction, which describes the probability that the parameter is the same sign as the median of the posterior distribution (Makowski et al., 2019).
Several plots were located in areas that burned multiple times, including 26 in the 2008 Shasta-Trinity Unit Lightning Complex fire. Reburned areas may have lower conifer regeneration than similar areas that have not experienced recent burns if surviving seed trees are killed (Tepley et al., 2017; McCord et al., 2020). We assumed that multiple burns would largely effect any regeneration we observed by removing potential parent trees. We modeled seed availability from the mapped surviving trees, so we elected not to include whether a plot was reburned in any of the models as this was unlikely to add additional information. The data can be found at Wright et al. (2023).
Conifer recruitment was found at most plots throughout the park. Out of the 131 plots, 35 (27%) did not have any conifer seedlings. Average seedling density for all conifers combined was 2,265 stems per hectare (Figure 4). Abies, Pinus and Calocedrus had the similar average seedling densities, with 703, 635, and 737 stems per hectare, respectively. Pseudotsuga seedlings were rare, found in only 29 plots, with the lowest average seedling density of 189 stems per hectare.
Oak regeneration was also widespread throughout the fire; 101 plots had either Quercus or Notholithocarpus regenerating in the plot, usually resprouting from topkilled trees.
There were considerable differences in the seed shadows we produced, depending on parameterizations (Figure 3). Unsurprisingly, seed shadows parameterized with short distance kernels (10m median dispersal distance) were much more concentrated around the source trees, with no predicted seed presence in the plot. In contrast, the estimates created with medium and long-distance parameterizations (50 and 100 m median dispersal distance, respectively) showed more widespread seed availability but at relatively low densities, especially for the long-distance parameterization.
All plots were within 321 meters of at least one surviving tree (Figure 5), with a median distance of 21 meters and 119 plots within 100 meters of a surviving tree.
LOO suggested that the models using seed availability extracted from seed shadows modestly outperformed the model using simple distance to live trees (Supplementary Table 1, Figure 6), with greater than four at least one standard error from zero. The medium distance model had the highest , though there was little evidence for major differences between the models using seed availability extracted from seed shadows with less than four in all cases. The short distance dispersal model had the lowest MAE, but MAE was similar across the seed shadow models. As with LOO, MAE suggested that the distance to live tree model had the worst performance, with MAE of 186 then the next best model. As with LOO, MAE for the models using dispersal kernels were very similar, although the model using the short-distance dispersal had lower MAE than the medium-distance model.
Figure 6 and mean absolute error (MAE, ± SE) for all models. is a relative measure against the model with the best in the set, so the models with the greatest predictive power have estimates of zero.
The medium distance model selected by LOO suggested that increased seed availability was associated with increased conifer seedling presence and density (Figures 7, 8), with probability of direction approaching 1 for both the hurdle and count components of the model. The effects of elevation were more uncertain. The best performing model indicated that increasing elevation resulted in fewer seedling observations (probability of direction 0.96), though there was little strength of evidence for a similar effect on seedling abundance (probability of direction 0.58). There was also little evidence for a consistent effect of topographic wetness index on either seedling presence or abundance, with probability of direction 0.78 and 0.56, respectively). There was some evidence that conifer seedlings were less likely to be found in areas with a high heat load index (probability of direction 0.95). The effect of heat load index on seedling density was more uncertain, but also suggested a positive relationship (probability of direction 0.87).
Figure 7 Model predictions of probability of observing zero conifer seedlings (± 95% credible intervals) for each predictor in the model with the highest . Predictions are made holding all other variables constant. Note the different axes scales.
Figure 8 Model predictions of conifer seedling density in stems per hectare (± 95% credible intervals) for each predictor in the model with the highest . Predictions are made holding all other variables constant. Note the different axes scales.
The effects of shrub cover were mixed. The model suggested that conifer seedlings were more likely to be observed (hurdle portion of the model) with shrub cover in the 26–50% range than in 0–25% (probability of direction 0.91), though the model also suggested that seedling abundance (density portion of the model) was lower in the 26–50% shrub cover class than in lower shrub densities (probability of direction 0.94). Evidence was much weaker for effects in the higher shrub cover classes (probability of direction below 85% in all cases), though it should be noted that the highest two shrub cover classes had very few observations (five and six plots, respectively).
For the bulk of obligate seeding conifers lacking serotinous cones, spatial variability in fire-related tree mortality will determine the availability of seeds and thus the spatial variability in regeneration following the fire event (Gill et al., 2022). There is a vast literature demonstrating this using a variety of methodologies at a variety of scales (Korb et al., 2019; Peeler and Smithwick, 2020; Gill et al., 2022). However, relatively few studies have incorporated variation in the spatial arrangement and fecundity of surviving trees at fine spatial scales. Our results suggest that incorporating fine scale estimates of seed availability can improve estimates of variability in postfire conifer regeneration.
Though the models with seed availability derived from seed shadows generally outperformed the models using the distance to the nearest surviving tree, the performance gain from the simple distance model was less than expected. This may be explained in part by species-specific variation in postfire dispersal capability. For example, the presence of serotinous knobcone pine in 15 of the low-elevation plots might have muted the effects of any dispersal model since the parent tree does not need to survive the fire for seeds to disperse. In addition to species-level differences, we were unable to precisely determine the species of the lidar mapped trees, including whether each mapped tree was indeed a conifer. Though our study was largely performed in conifer dominated areas and all but two plots had conifers present, tree misclassification as either conifer or live almost certainly drove a large part of the uncertainty in our results.
As expected, seed availability was associated with increased seedling presence and density. However, we did not observe the highest seedling densities in the places with the greatest estimated seed availability. Instead, the highest seedling densities were observed in plots with estimates of between 50 and 100 seeds per square meter (Supplementary Figure 2). High seed availability was most often observed in areas with a high density of surviving trees. This may be due to competition and shading from surviving trees, which can inhibit seed germination (Kroiss and HilleRisLambers, 2015). This pattern is also reminiscent of the Janzen-Connel effect (Janzen, 1970; Connell, 1971), which suggests that seeds and seedlings face higher predation levels where they are most abundant, that is near the parent tree. These effects have been demonstrated in some forest types (Steinitz et al., 2011; but see Hyatt et al., 2003). While an investigation of the Janzen-Connel effect is beyond the scope of this work, we mention it here as another possible mechanism contributing the uncertainty in our results.
Elevation had the most influence on conifer regeneration of all the topographic variables in the hurdle portions of the model. This is likely due in part to the prefire distribution of conifer trees throughout the park. Conifers are more prevalent with increasing elevation and are typically only dominant above ~1,500 m at WHIS (Smith et al., 2021). The effect of elevation may also be attributed to increased moisture availability and lower temperatures (Dodson and Root, 2013). The lack of a notable effect of elevation on seedling abundance may have been affected by the distribution of species along the elevation gradient, especially due to the presence of serotinous knobcone pine at lower elevations which reproduce in great numbers following fire events (Keeley et al., 1999). However, without reliable species identification for the potential parent trees, we were unable to disentangle the effects of species distribution from other potential mechanisms.
Our model indicated that the probability of seedling establishment was lower in areas with higher heat load index, though the strength of evidence for this effect was relatively low. This is similar to the findings of Boag et al. (2020), who found that greater heat load index resulted in a reduced probability of conifer regeneration, though the strength of the effect varied by species. As with elevation, species distributions may explain some of the uncertainty in our results. There was also little evidence for a consistent effect of topographic wetness index. We expected that conifers would be more likely to regenerate in more mesic areas, so the reason for the uncertainty in our results is unclear. Harvey et al. (2016) did not find a substantial relationship between Pseudotsuga regeneration and drought severity, noting that it is relatively drought tolerant. However, the variation in drought tolerant species in our study area suggests that this explanation may be insufficient at WHIS.
We found considerable uncertainty in the relationship between shrub cover and conifer regeneration, though there was some evidence for a facilitative effect for up to 50% shrub cover on seedling establishment but not density. These results are consistent with previous work. Observations from the nearby Sierra Nevada have shown shrub cover to have a complex association with postfire conifer recruitment, with both facilitative and competitive effects (Gray et al., 2005; Collins and Roller, 2013). Within the Klamath bioregion, the prevalence of shade tolerant conifers (Donato et al., 2009) and the facilitative effects of shrubs on local microsite conditions (Irvine et al., 2009) may enhance postfire recruitment. Shatford et al. (2007) found that while shrubs did seem to influence seedling density, the effect varied by species and competition from shrub cover did not seem to meaningfully affect the presence of conifer regeneration. We suspect the uncertainty in our results was likely influenced at least in part by relatively few observations we had in high (i.e., >50%) shrub cover. Additionally, our study occurred relatively soon after the fire, when the initial pulse of regenerating conifers established at a similar time to the shrubs, giving these conifers a competitive advantage relative to conifers that may establish later (Tepley et al., 2017).
Approaches such as we have presented here can be used to help improve postfire conifer regeneration tools such as poscrptR (Wright et al., 2020), particularly by incorporating fine scale seed dispersal and tree mortality information. Incorporating finer scale (i.e., less than 30 m resolution) mortality and regeneration data into future modeling efforts may be particularly important given the projections of a warmer and dryer climate in dry conifer forest regions, which is linked to more severe and frequent wildfires (Abatzoglou et al., 2017; Williams et al., 2019). Furthering the understanding of fine-scale variation in regeneration potential will help inform management intervention and future modeling efforts.
We were unable to directly parameterize the dispersal kernels we used to create the seed shadows, so we chose to limit our analysis to isotropic kernels, even though anisotropic kernels generally outperform simple isotropic kernels where they have been applied (Savage et al., 2011). Because we selected the parameterizations a priori, we were necessarily limited in the possible number of parameterizations that could reasonably be assessed, as well as the number of potential variables that may influence the seed shadows. For example, we elected to ignore the influence of terrain and wind. The effect of wind on seed dispersal is well documented (Greene and Johnson, 1996; Sánchez et al., 2011; van Putten et al., 2012), though the results for the influence of terrain have been more mixed and are likely scale- and species-dependent (Donato et al., 2009; Katul and Poggi, 2012; Peeler and Smithwick, 2020). There is little doubt the rugged, steep terrain in WHIS had some influence on seed dispersal, not the least of which is the effect terrain would have on local wind patterns. We also ignored the effect neighboring trees and other obstacles might have had on seed dispersal, which likely contributed to model error because seeds can disperse further when the parent tree is in or near an open area than when it is surrounded by neighbors (Greene and Johnson, 1996). Our models also attempted to associate established seedlings with seed shadows that were modeled assuming a single dispersal agent (i.e., wind), ignoring any potential additional dispersal agents that may have changed the distribution of seeds, such as the movement of cones or seeds by animals (Rogers et al., 2019).
Additionally, seed availability does not necessarily directly translate to seed establishment and survival. Millerón et al. (2013) found large disparities between seed dispersal kernels and kernels from established saplings, suggesting that dispersal alone is insufficient to capture patterns in recruitment and survival. Although we attempted to include variables that might distinguish between site quality and thus the probability of seedling establishment, we simply could not precisely determine the effects of site quality (i.e., soil moisture or temperature, see Wooten et al., 2022) with our data. For example, the presence of downed logs and shrubs can provide protection and increase soil moisture for regenerating seedlings (Tappeiner and Helms, 1971; Landesmann and Morales, 2018; Marcolin et al., 2019). Furthermore, many conifer seeds are consumed by small mammals and birds before germination (Gashwiler, 1970; Zwolak et al., 2010), which would change the distribution of available seed if the predation pressure was not spatially uniform (Janzen, 1970; Connell, 1971). Beyond predation, the factors influencing seed germination and seedling survival are complex, and include climate, competition, and abiotic factors (Irvine et al., 2009; Tepley et al., 2017; Stewart et al., 2021). We expect that the influence of climatic variables on postfire recruitment will become more apparent in coming years, especially since temperature has been unusually high in the postfire years (data not shown).
We tried to account for variation in fecundity with tree size when calculating estimates of seed availability, and to consider the amount of time available for seeds to disperse by including the number of seasons between the fire and when the plot was sampled. However, there is temporal variation in seed production in most conifer species (Clark et al., 1999b). Masting, the synchronization of periodic seed production between plants (Kelly, 1994), may also have influenced conifer regeneration after the Carr Fire. Masting has been demonstrated in many conifer species, including many of the species included in this study (Wright et al., 2021). Whether or not we sampled following a mast year undoubtedly influenced the total number of seedlings available, and thus the error between real and estimated seed availability. Finally, there was likely substantial model error arising from our inability to identify the species or even survival status of trees using aerial imagery or lidar, which may have obscured the advantages of modeling seed dispersal using individual tree locations.
Understanding the spatial variation in tree survival and seed availability is fundamental to understanding variability in postfire conifer regeneration, and therefore ecosystem recovery. However, simple metrics such as distance to surviving tree may not adequately capture the potential variation in seed dispersal or establishment. Indeed, our analysis demonstrates that models of dispersal at the tree level may also be inadequate to fully describe regeneration if they do not accurately capture variation in fecundity and dispersal (i.e., masting and anisotropy) or in establishment and survival (i.e., variation in microsite and predation). Spatial variation in conifer regeneration will drive forest recovery and structure in the years to come, including determining future fires through their effects on fuel availability (Tepley et al., 2018).
Our analysis suggest that successful postfire conifer regeneration is most likely to occur in areas where seed sources are available within a relatively short distance, at least in the years immediately after fire. These results highlight the importance of isolated surviving trees, which may serve as the only locally available seed source in areas of extensive tree mortality. The spatial arrangement, seed production, and seed dispersal characteristics of these surviving trees control both the rate and the possibility of forest recovery at WHIS. These results also highlight the importance of future disturbances such as drought and wildfire to forest recovery trajectories, since the death of isolated trees and those in small refugia may have an outsize influence on forest structure for years to come.
The datasets presented in this study can be found on sciencebase, the U.S. Geological Survey data repository. A full citation for the data releases is as follows: Wright, M.C., Smith, S.B., Engber, E., Thorne, K.M., Buffington, K.J., and van Mantgem, P.J., 2023, Data describing site characteristics including conifer regeneration following the 2018 Carr Fire in Whiskeytown National Recreation Area: U.S. Geological Survey data release, https://doi.org/10.5066/P95G11FE.
MW combined the data, performed the Bayesian analysis, and wrote the manuscript. KB performed the tree location and mortality analyses and assisted in the manuscript writing and editing. SS provided the inventory and monitoring data, EE provided the FMH data, and SS, EE, PM, and KT secured the funding for the project, organized the remaining data collection efforts, and assisted in the manuscript writing and editing. All authors contributed to the article and approved the submitted version.
This work was supported by H.R. 2157 Additional Supplemental Appropriations for Disaster Relief Act 2019 (Public Law 116-20), the National Park Service, and the U.S. Geological Survey Ecosystems Mission Area.
We thank the NPS and USGS crews who collected the field data. Thanks to C. Freeman and A. Colley for spatial data and processing, and to Laura Shaskey for logistical support.
The authors declare that the research was conducted in the absence of any commercial or financial relationships that could be construed as a potential conflict of interest.
All claims expressed in this article are solely those of the authors and do not necessarily represent those of their affiliated organizations, or those of the publisher, the editors and the reviewers. Any product that may be evaluated in this article, or claim that may be made by its manufacturer, is not guaranteed or endorsed by the publisher.
Any use of trade, firm, or product names is for descriptive purposes only and does not imply endorsement by the U.S. Government.
The Supplementary Material for this article can be found online at: https://www.frontiersin.org/articles/10.3389/fevo.2023.1229123/full#supplementary-material
Abatzoglou J. T., Kolden C. A., Williams A. P., Lutz J. A., Smith A. M. (2017). Climatic influences on interannual variability in regional burn severity across western US forests. Int. J. Wildland Fire 26, 269–275. doi: 10.1071/WF16165
Barber C., Graves S. J., Hall J. S., Zuidema P. A., Brandt J., Bohlman S. A., et al. (2022). Species-level tree crown maps improve predictions of tree recruit abundance in a tropical landscape. Ecol. Appl. 32, e2585. doi: 10.1002/eap.2585
Boag A. E., Ducey M. J., Palace M. W., Hartter J. (2020). Topography and fire legacies drive variable post-fire juvenile conifer regeneration in eastern Oregon, USA. For. Ecol. Manage. 474, 118312. doi: 10.1016/j.foreco.2020.118312
Bonner F. T., Karrfalt R. P. (Eds.) (2008). The woody plant seed manual (U.S. Department of Agriculture, Forest Service, Washington, DC).
Bullock J. M., Mallada González L., Tamme R., Götzenberger L., White S. M., Pärtel M., et al. (2017). A synthesis of empirical plant dispersal kernels. J. Ecol. 105, 6–19. doi: 10.1111/1365-2745.12666
Bürkner P.-C. (2017). brms: An R package for Bayesian multilevel models using Stan. J. Stat. Software 80, 1–28. doi: 10.18637/jss.v080.i01
Burns R. M., Honkala B. H. (1990). Silvics of North America. Volume 1. Conifers. United States Department of Agriculture (USDA), Forest Service. Available at: https://www.fs.usda.gov/research/treesearch/1547.
Chambers M. E., Fornwalt P. J., Malone S. L., Battaglia M. A. (2016). Patterns of conifer regeneration following high severity wildfire in ponderosa pine–dominated forests of the Colorado Front Range. For. Ecol. Manage. 378, 57–67. doi: 10.1016/j.foreco.2016.07.001
Clark J., Beckage B., Camill P., Cleveland B., HilleRisLambers J., Lichter J., et al. (1999b). Interpreting recruitment limitation in forests. Am. J. Bot. 86, 1–16. doi: 10.2307/2656950
Clark J. S., Silman M., Kern R., Macklin E., HilleRisLambers J. (1999a). Seed dispersal near and far: Patterns across temperate and tropical forests. Ecology 80, 1475–1494. doi: 10.1890/0012-9658(1999)080[1475:SDNAFP]2.0.CO;2
Collins B. M., Roller G. B. (2013). Early forest dynamics in stand-replacing fire patches in the northern Sierra Nevada, California, USA. Landscape Ecol. 28, 1801–1813. doi: 10.1007/s10980-013-9923-8
Collins B. M., Stevens J. T., Miller J. D., Stephens S. L., Brown P. M., North M. P. (2017). Alternative characterization of forest fire regimes: Incorporating spatial patterns. Landscape Ecol. 32, 1543–1552. doi: 10.1007/s10980-017-0528-5
Connell J. H. (1971). On the role of natural enemies in preventing competitive exclusion in some marine animals and in rain forest trees. Dynamics populations 298, 298–310.
Coop J. D., DeLory T. J., Downing W. M., Haire S. L., Krawchuk M. A., Miller C., et al. (2019). Contributions of fire refugia to resilient ponderosa pine and dry mixed-conifer forest landscapes. Ecosphere 10, 1–24. doi: 10.1002/ecs2.2809
Cousens R. D., Hughes B. D., Mesgaran M. B. (2018). Why we do not expect dispersal probability density functions based on a single mechanism to fit real seed shadows. J. Ecol. 106, 903–906. doi: 10.1111/1365-2745.12891
Crotteau J. S., Varner J. M. III, Ritchie M. W. (2013). Post-fire regeneration across a fire severity gradient in the southern Cascades. For. Ecol. Manage. 287, 103–112. doi: 10.1016/j.foreco.2012.09.022
Dalponte M., Coomes D. A. (2016). Tree-centric mapping of forest carbon density from airborne laser scanning and hyperspectral data. Methods Ecol. Evol. 7, 1236–1245. doi: 10.1111/2041-210X.12575
Dodson E. K., Root H. T. (2013). Conifer regeneration following stand-replacing wildfire varies along an elevation gradient in a ponderosa pine forest, Oregon, USA. For. Ecol. Manage. 302, 163–170. doi: 10.1016/j.foreco.2013.03.050
Donato D. C., Fontaine J. B., Campbell J. L., Robinson W. D., Kauffman J. B., Law B. E. (2009). Conifer regeneration in stand-replacement portions of a large mixed-severity wildfire in the Klamath–Siskiyou Mountains. Can. J. For. Res. 39, 823–838. doi: 10.1139/X09-016
Downing W. M., Krawchuk M. A., Meigs G. W., Haire S. L., Coop J. D., Walker R. B., et al. (2019). Influence of fire refugia spatial pattern on post-fire forest recovery in Oregon’s Blue Mountains. Landscape Ecol. 34, 771–792. doi: 10.1007/s10980-019-00802-1
Evans J. S. (2021) spatialEco. Available at: https://github.com/jeffreyevans/spatialEco.
Fox L. I., Stuart J. D., Steinberg S. J. (2006). Using high resolution satellite imagery and feature extraction software for vegetation mapping at the Whiskeytown National Recreation Area, Redding, California.
Fry D. L., Stephens S. L. (2006). Influence of humans and climate on the fire history of a ponderosa pine-mixed conifer forest in the southeastern Klamath Mountains, California. For. Ecol. Manage. 223, 428–438. doi: 10.1016/j.foreco.2005.12.021
Gashwiler J. S. (1970). Further study of conifer seed survival in a western Oregon clearcut. Ecology 51, 849–854. doi: 10.2307/1933977
Gelman A. (2008). Scaling regression inputs by dividing by two standard deviations. Stat Med. 27, 2865–2873. doi: 10.1002/sim.3107
Gill N. S., Turner M. G., Brown C. D., Glassman S. I., Haire S. L., Hansen W. D., et al (2022). Limitations to propagule dispersal will constrain postfire recovery of plants and fungi in western coniferous forests. BioScience. 7, 347–364 doi: 10.1093/biosci/biab139
Goss M., Swain D. L., Abatzoglou J. T., Sarhadi A., Kolden C. A., Williams A. P., et al. (2020). Climate change is increasing the likelihood of extreme autumn wildfire conditions across California. Environ. Res. Lett. 15, 094016. doi: 10.1088/1748-9326/ab83a7
Gray A. N., Zald H. S., Kern R. A., North M. (2005). Stand conditions associated with tree regeneration in Sierran mixed-conifer forests. For. Sci. 51, 198–210. doi: 10.1093/forestscience/51.3.198
Greene D. F., Canham C. D., Coates K. D., Lepage P. T. (2004). An evaluation of alternative dispersal functions for trees. J. Ecol. 92, 758–766. doi: 10.1111/j.0022-0477.2004.00921.x
Greene D., Johnson E. (1989). A model of wind dispersal of winged or plumed seeds. Ecology 70, 339–347. doi: 10.2307/1937538
Greene D., Johnson E. (1994). Estimating the mean annual seed production of trees. Ecology 75, 642–647. doi: 10.2307/1941722
Greene D., Johnson E. (1996). Wind dispersal of seeds from a forest into a clearing. Ecology 77, 595–609. doi: 10.2307/2265633
Harvey B. J., Donato D. C., Turner M. G. (2016). High and dry: Post-fire tree seedling establishment in subalpine forests decreases with post-fire drought and large stand-replacing burn patches. Global Ecol. Biogeography 25, 655–669. doi: 10.1111/geb.12443
Hijmans R. J. (2022) terra: Spatial Data Analysis. Available at: https://CRAN.R-project.org/package=terra.
Hyatt L. A., Rosenberg M. S., Howard T. G., Bole G., Fang W., Anastasia J., et al. (2003). The distance dependence prediction of the Janzen-Connell hypothesis: a meta-analysis. Oikos 103, 590–602. doi: 10.1034/j.1600-0706.2003.12235.x
Irvine D. R., Hibbs D. E., Shatford J. P. (2009). The relative importance of biotic and abiotic controls on young conifer growth after fire in the Klamath-Siskiyou region. Northwest Sci. 83, 334–347. doi: 10.3955/046.083.0405
Janzen D. H. (1970). Herbivores and the number of tree species in tropical forests. Am. Nat. 104, 501–528. doi: 10.1086/282687
Jeronimo S. M., Kane V. R., Churchill D. J., McGaughey R. J., Franklin J. F. (2018). Applying LiDAR individual tree detection to management of structurally diverse forest landscapes. J. Forestry 116, 336–346. doi: 10.1093/jofore/fvy023
Jucker T., Caspersen J., Chave J., Antin C., Barbier N., Bongers F., et al. (2017). Allometric equations for integrating remote sensing imagery into forest monitoring programmes. Global Change Biol. 23, 177–190. doi: 10.1111/gcb.13388
Katul G., Poggi D. (2012). The effects of gentle topographic variation on dispersal kernels of inertial particles. Geophysical Res. Lett. 39. doi: 10.1029/2011GL050811
Katul G., Porporato A., Nathan R., Siqueira M., Soons M., Poggi D., et al. (2005). Mechanistic analytical models for long-distance seed dispersal by wind. Am. Nat. 166, 368–381. doi: 10.1086/432589
Keeley J. E., Ne’eman G., Fotheringham C. (1999). Immaturity risk in a fire-dependent pine. J. Mediterr. Ecol. 1, 41–48.
Kelly D. (1994). The evolutionary ecology of mast seeding. Trends Ecol. Evol. 9, 465–470. doi: 10.1016/0169-5347(94)90310-7
Knapp E. E., Weatherspoon C. P., Skinner C. N. (2012). Shrub seed banks in mixed conifer forests of northern California and the role of fire in regulating abundance. Fire Ecol. 8, 32–48. doi: 10.4996/fireecology.0801032
Korb J. E., Fornwalt P. J., Stevens-Rumann C. S. (2019). What drives ponderosa pine regeneration following wildfire in the western United States? For. Ecol. Manage. 454, 117663. doi: 10.1016/j.foreco.2019.117663
Krannitz P. G., Duralia T. E. (2004). Cone and seed production in Pinus ponderosa: a review. Western North Am. Nat. 64, 208–218.
Kroiss S. J., HilleRisLambers J. (2015). Recruitment limitation of long-lived conifers: Implications for climate change responses. Ecology 96, 1286–1297. doi: 10.1890/14-0595.1
Landesmann J. B., Morales J. M. (2018). The importance of fire refugia in the recolonization of a fire-sensitive conifer in northern Patagonia. Plant Ecol. 219, 455–466. doi: 10.1007/s11258-018-0808-4
Leonzo C. M., Keyes C. R. (2010). Fire-excluded relict forests in the southeastern Klamath Mountains, California, USA. Fire Ecol. 6, 62–76. doi: 10.4996/fireecology.0603062
Makowski D., Ben-Shachar M. S., Lüdecke D. (2019). bayestestR: Describing effects and their uncertainty, existence and significance within the Bayesian framework. J. Open Source Software 4, 1541. doi: 10.21105/joss.01541
Maltamo M., Eerikäinen K., Pitkänen J., Hyyppä J., Vehmas M. (2004). Estimation of timber volume and stem density based on scanning laser altimetry and expected tree size distribution functions. Remote Sens. Environ. 90, 319–330. doi: 10.1016/j.rse.2004.01.006
Marcolin E., Marzano R., Vitali A., Garbarino M., Lingua E. (2019). Post-fire management impact on natural forest regeneration through altered microsite conditions. Forests 10 1014. doi: 10.3390/f10111014
Marrs J., Ni-Meister W. (2019). Machine learning techniques for tree species classification using co-registered LiDAR and hyperspectral data. Remote Sens. 11, 819. doi: 10.3390/rs11070819
McCord M., Reilly M. J., Butz R. J., Jules E. S. (2020). Early seral pathways of vegetation change following repeated short-interval, high-severity wildfire in a low-elevation, mixed conifer–hardwood forest landscape of the Klamath Mountains, California. Can. J. For. Res. 50, 13–23. doi: 10.1139/cjfr-2019-0161
McCune B., Keon D. (2002). Equations for potential annual direct incident radiation and heat load. J. Vegetation Sci. 13, 603–606. doi: 10.1111/j.1654-1103.2002.tb02087.x
Millerón M., López de Heredia U., Lorenzo Z., Alonso J., Dounavi A., Gil L., et al. (2013). Assessment of spatial discordance of primary and effective seed dispersal of European beech (Fagus sylvatica L.) by ecological and genetic methods. Mol. Ecol. 22, 1531–1545. doi: 10.1111/mec.12200
MTBS (2018) MTBS Data Access: Fire Level Geospatial Data. MTBS Project (USDA Forest Service/U.S. Geological Survey) (Accessed 2022-07-18).
MTBS (2020). Monitoring trends in burn severity assessment of event ID - ca4069812251020080622 (U.S. Geological Survey and USDA Forest Service). doi: 10.5066/P9IED7RZ
Murphy M. A., Evans J. S., Storfer A. (2010). Quantifying Bufo boreas connectivity in Yellowstone National Park with landscape genetics. Ecology 91, 252–261. doi: 10.1890/08-0879.1
Nathan R., Schurr F. M., Spiegel O., Steinitz O., Trakhtenbrot A., Tsoar A. (2008). Mechanisms of long-distance seed dispersal. Trends Ecol. Evol. 23, 638–647. doi: 10.1016/j.tree.2008.08.003
North M. P., Kane J. T., Kane V. R., Asner G. P., Berigan W., Churchill D. J., et al. (2017). Cover of tall trees best predicts California spotted owl habitat. For. Ecol. Manage. 405, 166–178. doi: 10.1016/j.foreco.2017.09.019
Odion D., Sarr D., Mohren S., Smith S. (2011). Monitoring vegetation composition, structure and function in the parks of the Klamath Network Parks (Fort Collins, Colorado: National Park Service).
Parker R. C., Evans D. L. (2004). An application of LiDAR in a double-sample forest inventory. Western J. Appl. Forestry 19, 95–101. doi: 10.1093/wjaf/19.2.95
Peeler J. L., Smithwick E. A. (2020). Seed source pattern and terrain have scale-dependent effects on post-fire tree recovery. Landscape Ecol. 35, 1945–1959. doi: 10.1007/s10980-020-01071-z
R Core Team (2021). R: A Language and Environment for Statistical Computing (Vienna, Austria: R Foundation for Statistical Computing). Available at: https://www.R-project.org/.
Reilly M. J., Monleon V. J., Jules E. S., Butz R. J. (2019). Range-wide population structure and dynamics of a serotinous conifer, knobcone pine (Pinus attenuata L.), under an anthropogenically-altered disturbance regime. For. Ecol. Manage. 441, 182–191. doi: 10.1016/j.foreco.2019.03.017
Rogers H. S., Beckman N. G., Hartig F., Johnson J. S., Pufal G., Shea K., et al. (2019). The total dispersal kernel: A review and future directions. AoB Plants 11, plz042. doi: 10.1093/aobpla/plz042
Roussel J., Auty D. (2021) Airborne LiDAR data manipulation and visualization for forestry applications. Available at: https://cran.r-project.org/package=lidR.
Roussel J.-R., Auty D., Coops N. C., Tompalski P., Goodbody T. R., Meador A. S., et al. (2020). lidR: An R package for analysis of Airborne Laser Scanning (ALS) data. Remote Sens. Environ. 251, 112061. doi: 10.1016/j.rse.2020.112061
Sánchez J. M. C., Greene D. F., Quesada M. (2011). A field test of inverse modeling of seed dispersal. Am. J. Bot. 98, 698–703. doi: 10.3732/ajb.1000152
Savage D., Barbetti M. J., MacLeod W. J., Salam M. U., Renton M. (2011). Can mechanistically parameterised, anisotropic dispersal kernels provide a reliable estimate of wind-assisted dispersal? Ecol. Model. 222, 1673–1682. doi: 10.1016/j.ecolmodel.2011.03.003
Shatford J., Hibbs D., Puettmann K. (2007). Conifer regeneration after forest fire in the Klamath-Siskiyous: how much, how soon? J. Forestry 105, 139–146. doi: 10.1093/jof/105.3.139
Shive K. L., Preisler H. K., Welch K. R., Safford H. D., Butz R. J., O’Hara K. L., et al. (2018). From the stand scale to the landscape scale: Predicting the spatial patterns of forest regeneration after disturbance. Ecol. Appl. 28, 1626–1639. doi: 10.1002/eap.1756
Sivula T., Magnusson M., Vehtari A. (2020). Uncertainty in Bayesian leave-one-out cross-validation based model comparison. arXiv preprint arXiv:2008.10296. 1–88. doi: 10.48550/arXiv.2008.10296
Skinner C. N., Taylor A. H., Agee J. K. (2006). “Klamath mountains bioregion,” in Fire in California’s ecosystems. Eds. Sugihara N. G., Wagtendonk J. W. v., Fites-Kaufmann J., Shaffer K. E., Thode A. E. (Berkeley: University of California Press), 170–194.
Smith S. B., van Mantgem P. J., Odion D. (2021). Vegetation community monitoring: Species composition and biophysical gradients in Klamath Network parks (National Park Service, Fort Collins, Colorado).
Steel E. A., Kennedy M. C., Cunningham P. G., Stanovick J. S. (2013). Applied statistics in ecology: Common pitfalls and simple solutions. Ecosphere 4, 1–13. doi: 10.1890/ES13-00160.1
Steinitz O., Troupin D., Vendramin G., Nathan R. (2011). Genetic evidence for a Janzen–Connell recruitment pattern in reproductive offspring of Pinus halepensis trees. Mol. Ecol. 20, 4152–4164. doi: 10.1111/j.1365-294X.2011.05203.x
Stevens J. T., Collins B. M., Miller J. D., North M. P., Stephens S. L. (2017). Changing spatial patterns of stand-replacing fire in California conifer forests. For. Ecol. Manage. 406, 28–36. doi: 10.1016/j.foreco.2017.08.051
Stevens-Rumann C. S., Morgan P. (2019). Tree regeneration following wildfires in the western US: a review. Fire Ecol. 15, 1–17. doi: 10.1186/s42408-019-0032-1
Stewart J. A., van Mantgem P. J., Young D. J., Shive K. L., Preisler H. K., Das A. J., et al. (2021). Effects of postfire climate and seed availability on postfire conifer regeneration. Ecol. Appl. 31, e02280. doi: 10.1002/eap.2280
Tappeiner J. C., Helms J. A. (1971). Natural regeneration of Douglas fir and white fir on exposed sites in the Sierra Nevada of California. Am. Midland Nat. 86, 358–370. doi: 10.2307/2423629
Tepley A. J., Thomann E., Veblen T. T., Perry G. L., Holz A., Paritsis J., et al. (2018). Influences of fire–vegetation feedbacks and post-fire recovery rates on forest landscape vulnerability to altered fire regimes. J. Ecol. 106, 1925–1940. doi: 10.1111/1365-2745.12950
Tepley A. J., Thompson J. R., Epstein H. E., Anderson-Teixeira K. J. (2017). Vulnerability to forest loss through altered postfire recovery dynamics in a warming climate in the Klamath Mountains. Global Change Biol. 23, 4117–4132. doi: 10.1111/gcb.13704
Thorne K. M., Freeman C. M., Rankin L. L. (2023). UAS Imagery at whiskeytown national recreation area in 2018 and 2019 following the carr fire. U.S. geological survey data release. ScienceBase. https://doi.org/10.5066/P9GS9V1J
Turner M. G., Baker W. L., Peterson C. J., Peet R. K. (1998). Factors influencing succession: Lessons from large, infrequent natural disturbances. Ecosystems 1, 511–523. doi: 10.1007/s100219900047
USDI National Park Service (2003). Fire monitoring handbook (Boise (ID: Fire Management Program Center, National Interagency Fire Center).
van Putten B., Visser M. D., Muller-Landau H. C., Jansen P. A. (2012). Distorted-distance models for directional dispersal: A general framework with application to a wind-dispersed tree. Methods Ecol. Evol. 3, 642–652. doi: 10.1111/j.2041-210X.2012.00208.x
Vehtari A., Gelman A., Gabry J. (2017). Practical Bayesian model evaluation using leave-one-out cross-validation and WAIC. Stat Computing 27, 1413–1432. doi: 10.1007/s11222-016-9696-4
Wehner M. F., Arnold J. R., Knutson T., Kunkel K. E., LeGrande A. N. (2017). “Droughts, floods, and wildfires,” In Climate Science Special Report: Fourth National Climate Assessment, Volume I [Wuebbles D. J., Fahey D. W., Hibbard K. A., Dokken D. J., Stewart B. C., Maycock T. K. (Eds.)]. U.S. Global Change Research Program, Washington, DC, USA, pp. 231–256.doi: 10.7930/J0CJ8BNN
Welch K. R., Safford H. D., Young T. P. (2016). Predicting conifer establishment post wildfire in mixed conifer forests of the North American Mediterranean-climate zone. Ecosphere 7, e01609. doi: 10.1002/ecs2.1609
Williams A. P., Abatzoglou J. T., Gershunov A., Guzman-Morales J., Bishop D. A., Balch J. K., et al. (2019). Observed impacts of anthropogenic climate change on wildfire in California. Earth’s Future 7, 892–910. doi: 10.1029/2019EF001210
Williams J., Safford H., Enstice N., Steel Z., Paulson A. (2023). High-severity burned area and proportion exceed historic conditions in Sierra Nevada, California, and adjacent ranges. Ecosphere 14, e4397. doi: 10.1002/ecs2.4397
Wooten J. T., Stevens-Rumann C. S., Schapira Z. H., Rocca M. E. (2022). Microenvironment characteristics and early regeneration after the 2018 Spring Creek Wildfire and post-fire logging in Colorado, USA. Fire Ecol. 18, 10. doi: 10.1186/s42408-022-00133-8
Wright M. C., Mantgem P., Stephenson N. L., Das A. J., Keeley J. E. (2021). Seed production patterns of surviving Sierra Nevada conifers show minimal change following drought. For. Ecol. Manage. 480, 118598. doi: 10.1016/j.foreco.2020.118598
Wright M. C., Stewart J. A. E., van Mantgem P. J., Young D. J. N., Shive K. L., Preisler H. K., et al. (2020). poscrptR. R package and U.S. Geological Survey software release. U.S. Geological Survey software release, https://code.usgs.gov/
Wright M. C., Smith S. B., Engber E., Thorne K. M., Buffington K. J., van Mantgem P. J., et al (2023) Data describing site characteristics including conifer regeneration following the 2018 Carr Fire in Whiskeytown National Recreation Area: U.S. Geological Survey data release. doi: 10.5066/P95G11FE
Wu Q. (2021) whitebox: ‘WhiteboxTools’ R Frontend. R package version 2.0.0. https://CRAN.R-project.org/package=whitebox. doi: 10.5066/P95G11FE.
Keywords: wildland fire, conifer regeneration, dispersal kernel, Bayesian modeling, lidar
Citation: Wright M, van Mantgem P, Buffington K, Thorne K, Engber E and Smith S (2023) Spatially explicit models of seed availability improve predictions of conifer regeneration following the 2018 Carr Fire in northern California. Front. Ecol. Evol. 11:1229123. doi: 10.3389/fevo.2023.1229123
Received: 25 May 2023; Accepted: 18 September 2023;
Published: 09 October 2023.
Edited by:
Matteo Marcantonio, Université catholique de Louvain, BelgiumReviewed by:
Kristen Shive, University of California, Berkeley, United StatesCopyright © 2023 Wright, van Mantgem, Buffington, Thorne, Engber and Smith. This is an open-access article distributed under the terms of the Creative Commons Attribution License (CC BY). The use, distribution or reproduction in other forums is permitted, provided the original author(s) and the copyright owner(s) are credited and that the original publication in this journal is cited, in accordance with accepted academic practice. No use, distribution or reproduction is permitted which does not comply with these terms.
*Correspondence: Micah Wright, bWN3cmlnaHRAdXNncy5nb3Y=
Disclaimer: All claims expressed in this article are solely those of the authors and do not necessarily represent those of their affiliated organizations, or those of the publisher, the editors and the reviewers. Any product that may be evaluated in this article or claim that may be made by its manufacturer is not guaranteed or endorsed by the publisher.
Research integrity at Frontiers
Learn more about the work of our research integrity team to safeguard the quality of each article we publish.