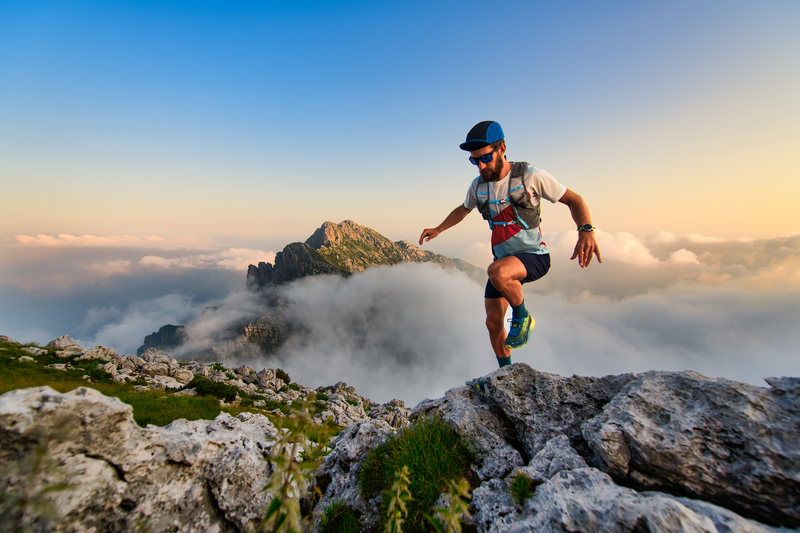
95% of researchers rate our articles as excellent or good
Learn more about the work of our research integrity team to safeguard the quality of each article we publish.
Find out more
ORIGINAL RESEARCH article
Front. Ecol. Evol. , 14 September 2022
Sec. Interdisciplinary Climate Studies
Volume 10 - 2022 | https://doi.org/10.3389/fevo.2022.977598
Beijing-Tianjin-Hebei urban agglomeration (BTH), Yangtze River Delta (YRD), and Pearl River Delta (PRD) are the most important economic hinterlands in China, offering high levels of economic development. In 2020, their proportion of China’s total GDP reached 39.28%. Over the 5 years of 2014–2018, the annual maximum air quality index (AQI) of the three major urban agglomerations was greater than 100, thus maintaining a grade III light pollution (100 < AQI < 200) in Chinese air standards. This research thus uses a two-stage empirical analysis method to explore the spatial-temporal dispersal physiognomies and spillover effects of air quality in these three major urban agglomerations. In the first stage, the Kriging interpolation method regionally estimates and displays the air quality monitoring sampling data. The results show that the air quality of these three major urban agglomerations is generally good from 2014 to 2018, the area of good air is gradually expanding, the AQI value is constantly decreasing, the air pollution of YRD is shifting from southeast to northwest, and the air pollution of PRD is increasing. The dyeing industry shows a trend of concentration from northwest to south-central. In the second stage, Moran’s I and Spatial Durbin Model (SDM) explore the spatial autocorrelation and spillover effects of air quality related variables. The results show that Moran’s I values in the spatial autocorrelation analysis all pass the significance test. Moreover, public transport, per capita GDP, science and technology expenditure, and the vegetation index all have a significant influence on the spatial dispersal of air quality in the three urban agglomerations, among which the direct effect of public transport and the indirect effect and total effect of the vegetation index are the most significant. Therefore, the China’s three major urban agglomerations (TMUA) ought to adjust the industrial structure, regional coordinated development, and clean technology innovation.
The air pollution has been brought to the fore by data as the rapid progress of society. The United Nations mentioned1, “If the world does not act now, and forcefully, the catastrophic effects of climate change will be far greater than the current pandemic.” Climate change continues to exacerbate the frequency and severity of natural disasters, which affected more than 39 million people in 2018, resulting in deaths, disrupted livelihoods and economic losses (The United Nations, 2020). A joint report published by the World Bank and the Institute for Health Metrics and Evaluation pointed out that air pollution has become the deadliest type of pollution and the fourth largest risk factor for premature death in the world (World Bank Group, 2016). Data provided by the World Health Organization reveals that nine-tenths of global people breathe air with high pollutant concentration. In Ten threats to global health in 2019, WHO ranked air pollution and climate change in first place.
In recent years, China’s air quality has been gradually improving, but the situation is still grim. Among the 161 prefectural and above cities that promoted new air quality standard monitoring in 2014, only 16 cities reached the air quality standard, while 90% cities exceeded the standard.2 The China government has also formulated corresponding measures, promulgating Air Pollution Prevention and Control Action Plan, Law of the People’s Republic of China on the Prevention and Control of Atmospheric Pollution, and Three-year action plan to fight air pollution from 2013 to 2018. In 2019, among 337 prefectural and above cities in China, only 157 cities met ambient air quality standard, accounting for 46.6% of the total cities, while the ambient air quality of 180 cities went off the standard, accounting for 53.4%.3 Due to the large amounts of CO2 and SO2 emissions, acid rain frequently appears in the east of the Yunnan Guizhou Plateau to the south of the Yangtze River,4 seriously polluting the soil, causing the land to be acid and saline, reducing agricultural output, and threatening the safety of water sources.
The three major urban agglomerations in China (BTH, YRD, and PRD) are seated in the northern, eastern coastal, and southern parts of China, respectively, covering the nation’s political, economic, and cultural centers and 4 of its first-tier cities. The regional GDP of BTH, YRD, and PRD, respectively, hit 8.6 trillion, 21.5 trillion, and 9.7 trillion Yuan, accounting for 39.28% of Chinese GDP in 2020. The three have become large city groups with world-class influence in China and even in the world.
As a result of to the mobility of air, both air quality and air pollution in various regions are undergoing dynamic changes. Air pollutant spills from some heavily polluted cities lead to a decrease of air quality of surrounding cities, and geographical and climate factors also promote or inhibit the dispersion of air pollutants. BTH in this study is bounded by Bohai Sea in the east, Taihang Mountains in the west, and Yanshan in the north. The terrain is low in the southeast and high in the northwest, which is not conducive to air pollution diffusion of cities in the northwest. YRD is an alluvial plain formed before the Yangtze River enters the sea. It is adjacent to the East China Sea and the Yellow Sea, and is located at the intersection of its named river and ocean. Flat topography facilitates the spread of air pollution. The urban agglomerations of Pearl River Delta are surrounded by hills, mountains, and islands, and the central part is a plain. The large fluctuation of the ground terrain limits the spread of air pollution.
Any study of regional air quality problems therefore must take into account the differences in their time and space distribution in order to obtain more real and effective information. Some Chinese researchers conducted on the time-space distribution characteristics of air quality for urban agglomerations via multiple indicators. Guo et al. (2019) summarized the spatial and temporal evolution characteristics of air quality in 20 urban agglomerations in China from 2015 to 2017. Chen et al. (2017) used spatial interpolation technique, correlation analysis and GIS to study the spatial-temporal distribution characteristics of the concentrations of air pollution factor and AQI in YRD. Apart from China, most existing international research studies on the time and space differences of air pollution take a single city or region or most prefecture level-cities as their object or study a single indicator.
Different from these studies above, this research breaks through the above limitations and makes the following innovations. (1) Two-stage empirical analysis is adopted to fully consider the spatial and temporal distribution differences of air quality among the three major urban agglomerations. In the first stage, Kriging interpolation method shows the time-space changes of the air quality index (AQI) of Chinese three major urban agglomerations spanning 2014–2018. In the second stage, Moran’s I and SDM models assess the spatial correlation and spillover effect of air quality-related factors. (2) The selection of air quality indicators is more comprehensive and rigorous. In the first stage, specific indicators of air quality such as AQI, SO2, PM2.5, and PM10 are selected for analysis one by one. In the second stage, industrialization level (Indus), foreign direct investment (FDI), technology expenditure (Tech), population density (population), built-up area (area), public transport (transport), and a normalized differential presence index (NDVI) are selected along with other influencing factors to evaluate the spatial spillover effect of air quality. (3) We select Chinese three most representative urban agglomerations to study the time and space differences and spillover effects of air pollution and thus break through the limitations of existing research on a provincial administrative region or single city level. This runs in line with the current rapid progress of urbanization process in China as well as its long-term plan to integrate into the global urbanization process in the future. This research provides a reference for other regions in China to control air pollution and also offers a representative case for the air pollution literature covering the world’s urban agglomerations. Figure 1 presents the structure of this study.
The existing research on air quality is mainly divided into single index and multiple indexes. In terms of single index, most scholars (Huang et al., 2015; Wen et al., 2018; Zhao et al., 2019; Chen X. et al., 2020; Dai et al., 2020; Zhang et al., 2020; Yu et al., 2021) selected PM2.5 as the research index. A few scholars (Jiang et al., 2014; Wu et al., 2019) studied from the perspective of API Pu et al. (2017) selected AQI for research. In terms of multiple indicators, there are few researchers and different perspectives. Some scholars (Xie et al., 2015; Mao et al., 2020) selected PM2.5, PM10, SO2, NO2, CO and O3 for research. Shi et al. (2020) selected PM2 5 and meteorological parameters. Zhao et al. (2020) selected PM2.5 and O3 for analysis. There are certain limitations in the selection of indicators of these results, which fail to comprehensively study the regional and temporal and spatial differences of air quality in combination with the indicators of influencing factors.
In terms of the selection of research objects, there are more achievements in single cities and multiple cities, but less in urban agglomerations. Some scholars (Huang et al., 2015; Mao et al., 2020; Zhang et al., 2020; Yu et al., 2021) have studied single cities or provinces in China. The research of most scholars (Xie et al., 2015; Jassim and Coskuner, 2017; Fan et al., 2019; Chen X. et al., 2020; Dai et al., 2020; Wang et al., 2020; Zhao et al., 2020) is based on the overall region. Yang et al. (2020) studied the Yangtze River Delta and found that the degree of urbanization was positively correlated with the concentration of PM2.5, while negatively correlated with the change trend of PM2.5 concentration. Xue et al. (2020) found that the environmental governance of eight urban agglomerations in China has a response lag of at least 1 year, and it takes a long time for regional internal governance and external collaborative governance to fully affect the concentration of PM2.5. Wang and Fang studied the urban agglomeration around the Bohai Sea and found that the concentration of PM2.5 showed obvious spatial variation and aggregation, and the per capita GDP, urbanization rate and urban construction were closely related to the concentration of PM2.5 (Wang and Fang, 2016). Li X. L. et al. (2019) studied the central Liaoning Urban Agglomeration and Harbin Changchun urban agglomeration, and found that the regional transportation of air pollutants between urban agglomerations in Northeast China is common.
At present, there are few studies on the air quality spillover effect in the existing studies, and the research directions and perspectives are different.
Part of the research is to show the empirical results of the spillover effects of various air pollution indicators in different regions. For example, Hoti et al. (2005) for the research of atmospheric carbon dioxide concentrations between Asia and South West Pacific shows that the spatial correlation between the two is almost zero; The research of Zheng et al. (2018), Fang et al. (2019), Li H. et al. (2019), and Ma et al. (2019) shows the spillover effects of PM2.5 concentration, CO2 emission, SO2 emission and other air indicators in different degrees within and between regions.
To summarize, the above research shows the spillover effect of various atmospheric indicators on a regional scope, but they only stop at displaying the data. There is no further research on the deep-seated reasons behind the phenomenon.
Most other studies have further sunk, focusing on the factors that affect the air quality spillover effect. Zhang et al. (2018) studied the spillover effect of supply chain transmission on SO2 emissions; Chen and Ye (2019) studied the influence of wind direction on PM2.5 concentration and its spillover effect; studied the spillover effect of cement production on air pollution; Du et al. (2018); Xiong et al. (2018), and Song et al. (2020) all studied the spillover effect from the perspective of economic development or urbanization level. Chen W. et al. (2020) considered the impact of agricultural industry factors on this basis, and obtained research results similar to those of the above scholars: the improvement of economic development or urbanization level has increased the spillover effect of air pollution; Jeffrey (2017), Huang et al. (2018), and Tong et al. (2020) studied the spillover effect of environmental regulations and policies on air quality governance; Among them, the conclusions of Fang et al. (2019) and Tong et al. (2020) are similar, that is, the reduction of pollution emissions in the target area is at the cost of the increase of pollution emissions in surrounding areas. Liu et al. (2020) studied the air quality changes and spillover effects during the Covid-19 epidemic blockade. The results showed that human flow, economic activities and other factors were closely related to air pollution and its spillover effects.
To summarize, human mobility, economic activities, and other factors closely relate to air pollution and its spillover effects. The above studies have explored the influencing factors of the spillover effect phenomenon and put forward relevant improvement suggestions, but index selection is only single or external influencing factors, thus having a certain degree of singleness. In contrast, this study involves an exploration of the internal influence of multiple air pollution indicators, in order to uncover the mutual influence relationship between various pollutants. The conclusion of Tong et al. (2020) is similar to that of Fang et al. (2019)—that is, the reduction of pollution emissions in the target area comes at the cost of increasing pollution emissions in the surrounding area.
Various studies on air quality spillover effect have achieved different results, but few of them take urban agglomeration as the research object. In addition to Xiong et al. (2018) taking 29 prefecture level cities in the Central Plains urban agglomeration as the research object and Song et al. (2020) taking the Bohai economic circle as the research object, Du et al. (2018), Li H. et al. (2019), and Tong et al. (2020) selected some cities in China’s Representative Beijing Tianjin Hebei, Yangtze River Delta and Pearl River Delta as the research object.
To summarize, various aspects of the literature on the spillover effect of air quality have achieved different results, but there are few studies on the spillover effect of urban agglomeration. The development scale and air pollution problems of TMUA are quite typical for other global urban agglomerations. Taking TMUA as the research object, this paper analyzes the air quality spillover effect, in order to obtain conclusions and suggestions that have guiding significance for global air pollution control.
Kriging Method is a linear unbiased optimal estimation method for variables in a limited region. Based on the variogram, this method takes into account not only the positional relationship between the estimation points and the observation data points, but also their spatial relationship.
In the existing research on air quality, scholars mostly use three Kriging models: Ordinary Kriging (OK), Universal Kriging (UK) and Bayesian Kriging (BK), in which Universal Kriging (UK) also includes Kriging with an external drift (KED). The use of ordinary Kriging is simple and convenient, with significant flexibility. For example, Bayraktar and Turalioglu (2005), and Pinto et al. (2020) all use ordinary Kriging. When the trend value in the model is expressed as a linear function of spatial coordinates and the regression coefficient is unknown, original Kriging evolves into Universal Kriging, such as the research of Wolterbeek and Verburg (2004) and Mercer et al. (2011). Kriging with an external drift method allows the inclusion of exogenous variables on the basis of Universal Kriging, such as Kassteele and Velders (2005), Kassteele and Stein (2006), Ignaccolo et al. (2014), Maxime et al. (2017, 2018) methods used in research. The Bayesian Kriging method divides the data used for spatial estimation into observation data and guess data according to accuracy and quantity, such as the method used by Kassteele et al. (2009) and Vicedo-Cabrera et al. (2013) in the study.
Due to the spatial correlation and spillover effect of air pollution, SDM model is widely used in the related research of air quality assessment. In addition, SDM model contains the spatial lag term of explanatory variables, which helps to reduce the bias caused by missing variables in empirical analysis.
For example, Feng et al. (2018) used SDM model to study the impact of air pollution control on urban environment; Feng and Wang (2019), Jiang et al. (2019) and Lv et al. (2019) used SDM to study the correlation between urbanization level and haze pollution and energy consumption; Long et al. (2020) and Xie et al. (2020) studied green finance in the Yangtze River economic belt; In addition to spatial autocorrelation, more SDM models have been used to study the spillover effect of air quality, such as the study of PM2.5 spillover effect on air quality improvement by Tong et al. (2020), the study of the impact of air pollution spillover effect on public health by Chen et al. (2016), and the study of carbon emission spillover effect of Bohai rim economic circle by combining Moran’s I with SDM by Song et al. (2020). Wu and Pu (2020) and Yang and Xu (2020) studied the impact of air pollution on income level through SDM.
Although the Kriging interpolation method and SDM are becoming more and more mature in the current research, there are few results from combining them. In the first stage, the Kriging interpolation method shows the temporal and spatial changes of AQI of TMUA from 2014 to 2018. In the second stage, SDM helps study the spillover effects of air quality-related factors, and Moran’s model analyzes the spillover effects. We show its spatial autocorrelation, which has a breakthrough in research object and research method, in order to contribute new results and conclusions to the research of air quality for world-class urban agglomerations.
Kriging interpolation is a common statistical analysis method in spatial statistics, which is widely used in Environmental Science, atmospheric science and other observation data. Kriging interpolation method, also known as spatial local interpolation approach, is an impartial optimum estimation of regionalized variables in a partial area based on variogram theory and structural analysis. If the results of variogram and structure analysis show that there is spatial correlation between regionalized variables, Kriging interpolation can be used for interpolation or extrapolation. Its core is to use the original data of regionalized variables and the structural characteristics of variogram to make linear impartial optimum estimation of unknown sample points. Impartial means that the mathematical expectation of deviation is 0, and optimum means that the sum of squares of the difference between the estimated value and the actual value is the minimum. Therefore, Kriging interpolation method is known sample points in the limited unknown sample field. By considering the shape, size, spatial direction of the sample points and the spatial relationship with the unknown sample points, the linear unbiased optimal estimation of the unknown sample points is carried out, and the structure information provided by the variogram is obtained.
The specific calculation formula runs as follows:
In formula (1), Z(xi) is the value of the ith meteorological datapoint; n is the total number of interpolation points; λi is the weight value of the ith meteorological datapoint; and Z(x0) is a datapoint.
This paper shall explain the possible spatial lag, spatial error, and SDM. Before setting a specific spatial econometric model for estimation, we need to confirm the existence of spatial correlation and construct the Moran index statistics for verification. If the test based on LM statistics rejects the non-spatial model and accepts the spatial lag model or spatial error model, then Lesage and Pace (2009) recommend to use SDM and extend the spatial lag to the model with spatial lag explanatory variables.
In formula (2), yit is the explained variable of observation unit I in time t, is the ith line of explanatory variable, Wij is the pre-set non-zero n*n spatial matrix, λ is the autocorrelation coefficient of the spatial disturbance term, and μi and ξt correspond to spatial and temporal effects, respectively. In formula (3), is the spatial interaction term between yit and its adjacent unit yit, and the spatial effect controls all undetermined non-time-varying variables of spatial units. In formula (4), SDM can be used to test the hypothesis: H:θ = 0 and H:θ = θ + ρβ = 0. The first hypothesis test examines whether SDM can be simplified as a spatial lag model, while the second hypothesis test examines whether it can be simplified as a spatial error model. If both hypotheses are rejected, then SDM can better describe the spatial spillover effect of air pollution.
Table 1 lists the predicted variable and explanatory variables we use in this paper.
Specifically, the choices of FDI indicator and Built-up area indicator in this paper are explained here:
1) Copeland and Taylor (1994) and Javorcik and Wei (2004) theoretically verified the existence of the “Pollution Haven Hypothesis” by studying the relationship between foreign trade and environmental pollution. Their results show that FDI has a significant impact on environmental pollution.
2) This paper chooses built-up area to represent the urbanization level of a certain region. As urbanization will lead to the increase of industrial pollution and the destruction of vegetation, there is no doubt that urbanization will worsen the air quality.
The research samples are collected from 60 cities in TMUA. The relationships between urban agglomerations and the cities appear in Table 2.
Figures 2A–D shows a bar graph of statistical indicators for 4 variables: GDP, industrialization level, technology expenditure, and NDVI. As shown in Figure 2A, the mean value of GDP shows a gradual upward trend at an average annual increase rate of 6.68% in 2014–2018. The maximum value steadily increases, with an average annual increment of more than RMB 10,000. The minimum value slightly increases, and the standard deviation gradually increases. This illustrates that the overall level of GDP is rising, but the three major urban agglomerations show obvious polarization in GDP.
As is demonstrated in Figure 2B, the mean value of indus shows a slow upward trend at an average annual increase rate of 4.03% in 2014–2018. The maximum value decreases slightly in 2015–2016, by 2.84% and 0.60%, respectively, and continues to increase in 2017–2018. The minimum value remains basically unchanged and at a low level. The rising trend of standard deviation is distinct. This explains that cities with a higher level of industrialization are improving, while the development of cities with a lower level is stagnant. The gap in industrialization level between cities has a trend of further widening.
As can be seen from Figure 2C, tech on average increase steadily in 2014–2018 at an average annual growth of 5.83%. The maximum value has an upward trend of fluctuation, or 0.0321 higher in 2018 than in 2014. The minimum value is always at a low level. With the increase of the maximum value, the standard deviation also increases. This shows that scientific expenditure has an upward trend.
As is exhibited in Figure 2D, the average value of NDVI shows slight increase during 2014–2018, with the maximum value unchanged. The minimum shows a steady upward trend. The standard deviation basically remains unchanged. It shows that the NDVI level is relatively high at the current stage, and backward cities have steadily improved.
In addition to the above indicators, we also selected FDI, population, area and transport. indicators for analysis (Table 3). The overall FDI level is low, showing a straight line decline in 2017–2018. The population and the area are similar, showing a slow development trend as a whole. The gap between cities in terms of public transport is widening.
Figures 3A–D help provide an overall analysis of the four air pollution indicators of AQI, PM2.5, PM10, and SO2 in the three major urban agglomerations.
From Figure 3A the average AQI shows a downward trend in 2014–2018. Except for the flat part in 2016–2017, BTH has a downward trend and the largest annual decrease of 8.28%. There are fluctuations in both YRD and PRD, with a small decrease. From a numerical perspective, the AQI values of YRD and PRD have been maintained at level two standard (51–100), of which PRD’s is lower. The AQI value of BTH is at level three standard (101–150) in 2014–2017, but improved to level two in 2018.
Figure 3B depicts that the average PM2.5 emissions show a downward trend in 2014–2018. The PM2.5 emissions of BTH are declining for 5 years, with an average annual decrease of 13.48%. The PM2.5 emissions of YRD shows a downward trend, with a decrease of 10 over 5 years, except for 2015. The PM2.5 emissions of PRD decreased significantly in 2015 and 2018 and remained the same in 2016 and 2017. In terms of value, the lowest PM2.5 in PRD decreased to below 30 in 2018, followed by YRD to 41 in 2018, and PM2.5 in BTH fell to 51 by 2018, nearly approaching that of YRD.
From Figure 3C we see that the average PM10 emissions decrease steadily over 2014–2018. The PM10 emissions of BTH continue to decline for the 5 years, with a significant annual drop of 12.11%. The PM10 emissions of YRD and PRD decrease slightly, staying the same in 2016 and 2017, with an annual decrease of 7.83% and 6.92%, respectively. From the numerical perspective, the concentration of PM10 in PRD is the lowest, followed by YRD, and the highest is in BTH. Although the decrease is significant, there is still a certain gap between BTH and YRD.
Figure 3D illustrates that the average SO2 emissions decreased significantly during 2014–2018. The SO2 emissions of BTH decreased in a straight line, with an average annual decrease of 24.08%. YRD and PRD also decreased for 5 years, but at a relatively slow decrease, reaching 17.85% and 16.02%, respectively. In terms of value, in 2014 BTH was above 50, while YRD and PRD were both around 20. In 2018, YRD and PRD were around 10, and BTH dropped to 17.0897.
Combining Figures 3A–D, we note that BTH has the most serious pollution, but the highest governance efficiency. The efficiency of air pollution control in YRD and PRD is similar, and the air quality in PRD is the best. In terms of the four air pollution indicators, SO2 treatment is the best, followed by PM2.5 and PM10. Although AQI is decreasing, the drops in YRD and PRD are not significant.
AQI is a dimensionless index to quantitatively describe air quality issued in 2012. The index includes hourly measurements of 6 pollutants: PM10, PM2.5, O3, CO, SO2, and NO2. As the most authoritative and commonly used air quality monitoring indicator in China, the analysis of the time-space evolution trend of AQI values is one of the most significant measures to evaluate the air pollution status of the three major urban agglomerations. Figures 4A–I show the AQI spatial distribution of Kriging interpolation in 2014, 2016, and 2018, respectively, with the help of Arcgis10.3 software.
Figure 4. AQI spatial distribution by Kriging interpolation. (A–C) The BTH urban agglomeration. (D–F) YRD urban agglomeration. (G–I) The PRD urban agglomeration.
From the angle of spatial distribution, the AQI value of BTH gradually increased from the north to the south in 2014, but nearly one-third of the areas were excellent. The AQI value of the southernmost city was the highest, and its air pollution was relatively serious. From the perspective of time evolution, the excellent areas in the northern part of BTH continued to extend in 2014–2018. Although air pollution in the southern area is still grave, most serious areas have gradually decreased.
Spatially, in 2014 the AQI value of YRD in the central cities was the highest, while the AQI values of the western and eastern coastal areas were better. The overall air pollution is relatively serious. Temporally, the most severe areas of YRD gradually transferred to the northwest in 2014–2018, the air pollution of southeast cities and some southern cities gradually became better. The most severe areas are shrinking, and overall urban agglomeration turned better.
From the spatial distribution, in 2014 the highest AQI values of the central cities and the northern cities in PRD were on the high side, while the values of the southern and eastern cities was at a low level, and the overall air pollution was relatively low. From the perspective of time evolution, the central and southern cities with more serious air pollution in PRD are increasing and have been on the high side in 2014–2018. The air conditions in other areas of PRD have improved and performed well.
As a whole, the pollution in YRD and BTH is relatively serious. Moreover, areas with serious pollution in BTH are relatively fixed, and there is a trend of gradual reduction. The air pollution in most areas of the YRD city group was serious in 2014, and the number of cities with serious pollution was reducing in 2016–2018, mainly reflected in the transfer of pollution from the east to the northwest. The pollution of the whole city group is still severe. PRD performed the best among the three. Except for the most polluted cities with pollution, other cities have gradually improved and presented a good trend.
In this section we use two-stage data analysis on spatial correlation and spillover effects using the spatial econometric model.
Before using a spatial econometric model to analyze the data, we need to study the spatial connection test between AQI and explanatory variables to prove the requirement of using the spatial econometric model. This study uses Moran’s I to explore the spatial model of regional air quality so as to show the spatial correlation and spatial differentiation of air quality in three major urban agglomerations and to show the resemblance of specific feature values in adjoining regions.
Moran’s I is a description of the correlation degree of attribute values of units with similar or adjacent spatial distance. The test is to evaluate the existence of associations that have known certain attributes and must have spatial attribute data arrays, and finally determine whether there is clustering, discrete or random pattern data distribution in space.
Moran’s I can show the spatial correlations between variables and infer the overall distribution of values in space. The adjacency space weight matrix is adopted here. For example, adjacency is 1 and non-adjacency is 0. Its specific calculation is shown in formula (5). N means the spatial samples in here, subscripts i and j shows different regions, x is the average value of the observed characters of the spatial unit, s is its standard deviation, and Wij represents the elements in row i and column j of the n*n-dimensional space weight matrix.
The results of Moran’s I are in Table 4. We see from Table 4 that Moran’s I of AQI and each explanatory variable are greater than 0, and there is a significantly positive correlation between 2014 and 2018. Among them, Moran’s I of AQI and per capita GDP fluctuate at a high level, indicating that AQI and GDP per capita present a high spatial autocorrelation, while population density, built-up area, and public transport fluctuate at a low level, indicating that they present weak spatial autocorrelation. Moran’s I of science and technology expenditure from 2014 to 2015 shows a high spatial autocorrelation. However, according to the original data, the proportion of science and technology expenditure does not increase significantly, but per capita GDP does show significantly positive growth, considering that the rise in per capita GDP led to a significant increase in the spatial autocorrelation of science and technology expenditure. Moran’s I of other variables show a trend of shock from 2014 to 2018. Therefore, we cannot use the traditional OLS regression estimation, but instead should use the spatial econometric model for analysis.
Table 5 presents the results of selecting the fixed effect model and random effect model by the Hausman test. The results of the LR test show that the P value of double fixed and regional fixed comparison is 0.5667, which supports the hypothesis of adopting the regional fixed effect model at the 1% significance level. The P value of double fixed and time fixed comparison is 0.0000, which rejects the hypothesis of adopting the time fixed effect model at the 1% significance level. Due to the opposite conclusions, this paper adopts the double fixed effect model.
Through the Wald and lratio tests, we select the SDM, SAR and SEM spatial econometric models. Table 6 tests whether the SDM model can degenerate into the SAR model and SEM model. The findings show that the two test results reject the original hypothesis that can degenerate at the 1% significance level, and so the SDM model should be used.
Table 7 presents the spatial spillover effect of air quality in three urban agglomerations. The results of spatial spillover effect analysis have three kinds of results: direct effect, indirect effect, and overall effect. The direct effect shows the influence of explanatory variables on the air quality of the three urban agglomerations, the indirect effect shows the influence of explanatory variables on the surrounding areas, and the overall effect reflects the influence of all areas of the three urban agglomerations.
From the above table we see that the direct, indirect, and overall effects of FDI, population density, and built-up area are not significant. This indicates that although FDI, population density, and built-up area have an impact on the air quality of the three urban agglomerations, their impact is not distinct.
The direct effect, indirect effect, and overall effect of the industrialization level are negative, but not significant, meaning that improvement of the industrialization level can inhibit the deterioration of air quality in TMUA to a certain extent, but this effect is not obvious. It indicates that TMUA are paying attention to the protection of air quality while developing industrialization and have made great progress at improving air quality. Some achievements have been realized, but the improvement results are not obvious. It can be seen that China still does not put air quality protection in the most important position of its industrial development. At the same time, although China has continuously strengthened the policy stimulus for green economy in industrial development since 2014,5 more and more industrial enterprises have begun to apply energy-saving and emission reduction technology to industrial development and production, but the technology has not had a great positive influence.
The direct effect of per capita GDP and science and technology expenditure on air quality is positive, but not significant. Considering that there are many factors affecting local air quality, the impact of economic development and science and technology expenditure on local air quality is not obvious, while the indirect effect and total effect of the two are significantly positive, which shows that the development of the regional economy and science and technology expenditure on the surrounding areas and the three areas increase air pollution in the three urban agglomerations and have a significant aggravating effect. It can be seen that the economic development of the three urban agglomerations comes ultimately at the cost of the aggravation of air pollution, and the positive correlation between science and technology expenditure and air pollution also shows that the increased science and technology expenditure by the three urban agglomerations is not only applied to the development of environmental protection technology, but also raises air pollution. Therefore, the three urban agglomerations should consider increasing the proportion of environmental protection technology innovation in the next science and technology expenditure.
Public transport shows a weak and significantly negative correlation direct effect, which indicates that the development of public transport can improve the air quality of its own areas to a certain extent. The reason is that the proportion of new energy use in public transport is further increased. From 2013 to 2018, a number of ministries and commissions in China issued a series of policies such as Notice on Continuing the Promotion and Application of New Energy Vehicles.6 The policies have effectively promoted the use of clean energy by public transport. Moreover, the indirect and total effects of public transport on air quality are not significant, showing that the impact of public transport is more limited to local areas, and its impact on the surrounding external areas is not obvious. Considering that the development of public transport is limited by a city’s own scale, only the direct effect is significant, which is reasonable.
We also see at the same time that the indirect and total effects of vegetation index on the air quality of the three urban agglomerations have a significantly negative impact on the air quality. This shows that vegetation, as a natural air purifier, plays a great role in improving the air quality of the three urban agglomerations. In addition, we should face up to the impact of vegetation’s increase on the improvement of air quality. This has a certain guiding significance for the next development of China’s green economy.
Through the spatial econometric analysis of air quality in TMUA, this paper finds that some variables have a significant spillover effect. This further shows that the governance of air quality needs to pay close attention to the impact of the spillover effect, which has certain guiding significance for the governance of air quality in TMUA.
Comparing our results with previous studies, we find some similarities and differences as follows.
1. In terms of research findings, Zheng et al. (2018) offer similar conclusions with us. Zheng et al. (2018) took PM2.5 as the basic of research and analyzed the haze pollution in Beijing and surrounding areas. The results show that PM2.5 between the above cities has obvious spillover effect, and the pollution in the south is more serious than that in the north. In this paper, there is also a spillover effect of air pollution in neighboring areas in TMUA. Their same empirical results as this paper are not only an auxiliary proof of the research conclusion on the air quality of BTH, but also a detailed indication of the PM2.5 pollution spillover effect of BTH, which can be regarded as a supplement to the research object of this paper.
Xiong et al. (2018) show that science and technology expenditure has a certain inhibitory effect on industrial sulfur dioxide emissions of Central Plains urban agglomeration, which is different from the conclusion of this paper about the deterioration of air quality caused by science and technology expenditure. Considering that the spillover effect research in this paper is the empirical result obtained by taking TMUA as a whole as well as the selections of indicators in Xiong et al. (2018)’s research are different from this paper. Therefore, the empirical results herein are different from those of Xiong et al. (2018).
2. In the existing research on air quality through Moran’s I and SDM, Song et al. (2020) has a similar research structure and purpose with this paper, they explores the spatiotemporal regularity, the spatial correlation, and the spillover effect in carbon emission intensity in The Bohai Economic Rim (BER) employing the Moran index and the spatial Durbin model. But their research object is BER, which is different from the three urban agglomerations studied in this paper in terms of magnitude and structure. It is worth noting that the above research also includes Beijing City and Tianjin City, two cities belonging to BTH. The research shows that the carbon emissions between the two cities also have a certain spatial correlation, which also strengthens the confirmation of the conclusion of this paper. However, taking their urban agglomerations as the research object, we arrive at a constructive recommendation in a larger agglomeration range.
The research of spatial-temporal characteristics and determinants of PM2.5 in BER by Wang and Fang (2016) is literally similar with the research by Song et al. (2020). However, the difference between Wang and Fang (2016) and this paper also lies in the different research objects.
Du et al. (2018) take TMUA as the research objects and draw the conclusion that there is a positive correlation between the urbanization level and the concentration of PM2.5 in cities. Moreover, their empirical methods used and research objects selected are exactly the same as those in this paper. However, their research is only limited to the thinking of PM2.5, and this paper examines the spillover effect of more influencing factors. Totally, this paper further expands the scope of the topic by considering the impact of multiple factors on air quality.
3. The deficiency of this paper is that it does not separate the three urban agglomerations and discuss them independently. The end result is that it is impossible for future research to further study individual urban agglomerations.
Based on the data of TMUA from 2014 to 2018, this paper analyzes the spatial and temporal differences and spatial autocorrelation of air quality in urban agglomerations and studies the spatiotemporal distribution characteristics of air quality in TMUA and the spillover effects among its own region, surrounding region, and the whole region.
1. From 2014 to 2018, the air quality of TMUA had a great performance, and the AQIs and other various pollutant indicators of them were steadily declining. The 5-year average of AQI decreased from 89.40 to 75.06, the 5-year average of PM2.5 concentration decreased from 57.92 to 40.35, the 5-year average of PM10 concentration decreased from 85.22 to 66.16, the 5-year average of SO2 emissions decreased from 27.75 to 11.46, and the AQI level of the three urban agglomerations gradually improved from the third level of air quality standard to the second level.
2. The internal individual air quality levels of each urban agglomeration are different, and the gaps are large. The air quality in the north of BTH is improving, while the air pollution in the south is still serious, but the area of heavy air pollution is gradually decreasing. The air pollution areas in YRD are gradually transferring to the northwest, while the air pollution in the southeast and some southern cities is gradually improving, and the overall air pollution in the whole YRD is better. The air pollution in PRD urban agglomeration is more serious: air pollution in the east and the west of PRD has improved. In general, the pollution problems of BTH and YRD have decreased, but are still serious. PRD performs the best among the three urban agglomerations, and most cities in PRD are in a good situation.
3. There are spatial autocorrelation and spillover effects of air pollution in TMUA. The direct effect of public transport is the most significant, and the indirect effect and overall effect of the vegetation index are the most significant. The development of public transport has a weak effect on the improvement of air quality, while the increase of per capita GDP and science and technology expenditure has led to the deterioration of air pollution, and the improvement of the vegetation index has a significantly positive effect on the improvement of air quality.
According to the different characteristics of air quality and economic development of TMUA, it is necessary to formulate differentiated environmental governance policies.
1. From the results of spatiotemporal variation analysis, TMUA have different priorities for improvement. The air pollution area of BTH is relatively fixed and shows signs of slow transfer to the south. Tianjin and Tangshan in the south of BTH are the largest comprehensive industrial bases in the north of China, with a strong foundation of a secondary industry, which not only brings economic development to BTH, but also brings serious air quality problems. Therefore, the government should strengthen the adjustment of industrial structure, eliminate backward production capacity, and increase investment in the development of environmental protection technology in BTH.
YRD shows obvious characteristics of air pollution transferring from coastal cities to the northwest inland, because the pollution transfer is brought about via industrial transfer. Industrial transfer is a double-edged sword for inland cities, which not only helps develop the urban economy, but also damages it with air pollution. Therefore, coastal cities in YRD should continue to protect the environment. With good air quality control effect, inland cities cannot blindly pursue economic development and accept industrial transfer regardless of air pollution. At the same time, YRD as a whole needs the government to promote coordinated development, and coastal cities can help inland cities to promote innovative development, environmental protection, and clean technology.
The air quality performance of PRD is the best, which relates to the industrial structure of PRD—the degree of PRD’s tertiary industry development degree is much higher than that of BTH’s and YRD’s. However, air pollution in PRD from 2014 to 2018 shows a trend of clustering to the middle. Considering that PRD is vigorously promoting the integration of Guangzhou, Foshan, and Zhaoqing in recent years,7 PRD presents a trend of industrial park gathering and transferring to Foshan, which explains the reason why air pollution is concentrated in the central region. Therefore, Guangzhou, Foshan, and Zhaoqing should make great efforts to use technological innovation to improve energy efficiency and reduce energy consumption, and PRD should continue to give full play to the advantages of the development of the tertiary industry and maintain the existing achievements in economic development and air quality control.
2. The spillover effect should also be a focus of attention. Due to the significant spillover effect of spatial autocorrelation, the government must attach importance to the impact of the spillover effect during air quality governance. When dealing with air quality problems in TMUA, the influence that the spillover effect brings to air quality should be noticed, so as to promote the spatial linkage of policies among urban agglomerations. According to the results of spatial autocorrelation, policies can further focus on increasing the proportion of clean energy use in public transport and the proportion of environmental protection technology innovation and development in science and technology expenditure. As a variable factor that plays a significantly positive role in improving air quality, NDVI needs to attract more attention, and each urban agglomeration needs to further increase the content of vegetation area.
The original contributions presented in this study are included in the article/supplementary material, further inquiries can be directed to the corresponding author.
ZC: conceptualization, investigation, and visualization. F-rR: methodology, software, resources, writing—review and editing, and project administration. QW and ZX: formal analysis and writing—original draft preparation. QW: data curation. All authors read and contributed to the manuscript.
This study was supported by Jiangsu Province Social Science Foundation Project (22GLD019), Major Project of Philosophy and Social Science Research in Universities of Jiangsu Province (2022SJZDA053), and Soft Science Project of Nanjing Municipal Science and Technology Bureau (202206005).
The authors declare that the research was conducted in the absence of any commercial or financial relationships that could be construed as a potential conflict of interest.
All claims expressed in this article are solely those of the authors and do not necessarily represent those of their affiliated organizations, or those of the publisher, the editors and the reviewers. Any product that may be evaluated in this article, or claim that may be made by its manufacturer, is not guaranteed or endorsed by the publisher.
Bayraktar, H., and Turalioglu, F. S. (2005). A kriging-based approach for locating a sampling site—in the assessment of air quality. Stoch. Environ. Res. Risk Assess. 19, 301–305.
Chen, W., Peng, Y., and Yu, G. (2020). The influencing factors and spillover effects of interprovincial agricultural carbon emissions in China. PLoS One 15:e0240800. doi: 10.1371/journal.pone.0240800
Chen, X., Li, F., Zhang, J. D., Zhou, W., Wang, X. Y., and Fu, H. J. (2020). Spatiotemporal mapping and multiple driving forces identifying of PM2.5 variation and its joint management strategies across China. J. Clean. Prod. 250:119534.
Chen, X., Shuai, S., Tian, Z., Xie, Z., and Yin, P. (2016). Impacts of air pollution and its spatial spillover effect on public health based on China’s big data sample. J. Clean. Prod. 142, 915–925.
Chen, X., and Ye, J. (2019). When the wind blows: Spatial spillover effects of urban air pollution in China. J. Environ. Plan. Manag. 62, 1359–1376.
Chen, Y. L., Tao, T. H., and Ding, P. (2017). Spatial-temporal distribution characteristics of air quality in the urban agglomeration of the Yangtze river delta. Resour. Environ. Yangtze Basin 26, 687–697.
Copeland, B. R., and Taylor, M. S. (1994). North-South trade and the environment. Q. J. Econ. 109, 755–787.
Dai, F., Chen, M., and Yang, B. (2020). Spatiotemporal variations of PM2.5 concentration at the neighborhood level in five Chinese megacities. Atmos. Pollut. Res. 11, 190–202.
Du, Y., Sun, T., Peng, J., Fang, K., Liu, Y., Yang, Y., et al. (2018). Direct and spillover effects of urbanization on PM2.5 concentrations in China’s top three urban agglomerations. J. Clean. Prod. 190, 72–83.
Fan, Q. Y., Yang, S., and Liu, S. B. (2019). Asymmetrically spatial effects of urban scale and agglomeration on haze pollution in China. Int. J. Environ. Res. Public Health 16:4936. doi: 10.3390/ijerph16244936
Fang, D., Chen, B., Hubacek, K., Ni, R., Chen, L., Feng, K., et al. (2019). Clean air for some: Unintended spillover effects of regional air pollution policies. Sci. Adv. 5:eaav4707.
Feng, Y., Wang, X., Du, W., and Liu, J. (2018). Effects of air pollution control on urban development quality in Chinese cities based on spatial Durbin model. Int. J. Environ. Res. Public Health 15:2822. doi: 10.3390/ijerph15122822
Feng, Y., and Wang, X. (2019). Effects of urban sprawl on haze pollution in China based on dynamic SDM during 2003–2016. J. Clean. Prod. 242:118368.
Guo, Y. M., Lin, X. Q., and Bian, Y. (2019). The spatial-temporal characteristics and influencing factors of air quality in China’s Urban agglomerations. Ecol. Econ. 35, 167–175.
Hoti, S., Mcaleer, M., and Chan, F. (2005). Modelling the spillover effects in the volatility of atmospheric carbon dioxide concentrations. Math. Comput. Simul. 69, 46–56.
Huang, C., Santibanez-Gonzalez, E. D. R., and Song, M. (2018). Interstate pollution spillover and setting environmental standards. J. Clean. Prod. 170, 1544–1553.
Huang, F. F., Li, X., Wang, C., Xu, Q., Wang, W., Luo, Y. X., et al. (2015). PM2.5 spatiotemporal variations and the relationship with meteorological factors during 2013-2014 in Beijing, China. PLoS One 10:e0141642. doi: 10.1371/journal.pone.0141642
Ignaccolo, R., Mateu, J., and Giraldo, R. (2014). Kriging with external drift for functional data for air quality monitoring. Stoch. Environ. Res. Risk Assess. 28, 1171–1186.
Jassim, M. S., and Coskuner, G. (2017). Assessment of spatial variations of particulate matter (PM10 and PM2.5) in Bahrain identified by air quality index (AQI). Arab. J. Geosci. 10:19.
Javorcik, B. S., and Wei, S. J. (2004). Pollution havens and foreign direct investment: Dirty secret or popular myth? B.E. J. Econ. Anal. Policy 3:1244.
Jeffrey, C. P. (2017). Natural gas and spillover from the US clean power plan into the Paris agreement. Energy Policy 106, 41–47.
Jiang, H. Y., Li, H. R., Yang, L. S., Li, Y. H., Wang, W. Y., and Yan, Y. C. (2014). Spatial and seasonal variations of the air pollution index and a driving factors analysis in China. J. Environ. Qual. 43, 1853–1863. doi: 10.2134/jeq2014.06.0254
Jiang, S., Wang, J., Lei, S., and Mong, Z. (2019). Impact of energy consumption and air pollution on economic growth an empirical study based on dynamic spatial Durbin model. Energy Proc. 158, 4011–4016.
Kassteele, J., and Stein, A. (2006). A model for external drift kriging with uncertain covariates applied to air quality measurements and dispersion model output. Environmetrics 17, 309–322.
Kassteele, J., Stein, A., Dekkers, A., and Velders, G. J. M. (2009). External drift kriging of NOx concentrations with dispersion model output in a reduced air quality monitoring network. Environ. Ecol. Stat. 16, 321–339.
Kassteele, J., and Velders, G. (2005). Uncertainty assessment of local NO2 concentrations derived from error-in-variable external drift kriging and its relationship to the 2010 air quality standard. Atmos. Environ. 40, 2583–2595.
Lesage, J. P., and Pace, R. K. (2009). Introduction to spatial econometric. Boca Rato, FL: CRC Press.
Li, H., Qi, Y., and Li, C. (2019). Routes and clustering features of PM2.5 spillover within the Jing-Jin-Ji region at multiple timescales identified using complex network-based methods. J. Cleaner Prod. 209, 1195–1205.
Li, X. L., Hu, X. M., Shi, S. Y., Shen, L. D., Luan, L., and Ma, Y. J. (2019). Spatiotemporal variations and regional transport of air pollutants in two urban agglomerations in Northeast China plain. Chin. Geogr. Sci. 29, 917–933.
Liu, S., Kong, G., and Kong, D. (2020). Effects of the covid-19 on air quality: Human mobility, spillover effects, and city connections. Soc. Sci. Electron. Publ. 76, 635–653. doi: 10.1007/s10640-020-00492-3
Long, R., Guo, H., Zheng, D., Chang, R., Na, S., et al. (2020). Research on the measurement, evolution, and driving factors of green innovation efficiency in Yangtze river economic belt: A super-SBM and spatial Durbin model. Complexity 2020:8094247.
Lv, Y., Chen, W., and Cheng, J. (2019). Modelling dynamic impacts of urbanization on disaggregated energy consumption in China: A spatial Durbin modelling and decomposition approach. Energy Policy 133:110841.
Ma, Q., Zhang, T., and Li, J. (2019). Estimate on spillover effect of environmental pollution between peripheral areas. Chin. J. Popul. Resour. Environ. 17, 254–266.
Mao, M., Zhang, X. L., Shao, Y. M., and Yin, Y. (2020). Spatiotemporal variations and factors of air quality in urban central China during 2013-2015. Int. J. Environ. Res. Public Health 17:229. doi: 10.3390/ijerph17010229
Maxime, B., Chantal, F., and Laure, M. (2017). Dealing with non-stationarity through explanatory variables in kriging-based air quality maps. Spat. Stat. 22, 18–46.
Maxime, B., Laure, M., Chantal, F., Laurent, L., and Frédéric, T. (2018). A polynomial approximation of the traffic contributions for kriging-based interpolation of urban air quality model. Environ. Model. Softw. 105, 132–152.
Mercer, L. D., Szpiro, A. A., Sheppard, L., Lindström, J., Adar, S. D., Allen, R. W., et al. (2011). Comparing universal kriging and land-use regression for predicting concentrations of gaseous oxides of nitrogen (NOx) for the multi-ethnic study of atherosclerosis and air pollution (MESA Air). Atmos. Environ. 45, 4412–4420. doi: 10.1016/j.atmosenv.2011.05.043
Pinto, J. A., Kumar, P., Alonso, M. F., Andreão, W. L., Pedruzzi, R., Espinosa, S. I., et al. (2020). Kriging method application and traffic behavior profiles from local radar network database: A proposal to support traffic solutions and air pollution control strategies. Sustain. Cities Soc. 56:102062.
Pu, H. X., Luo, K. L., Wang, P., Wang, S. B., and Kang, S. (2017). Spatial variation of air quality index and urban driving factors linkages, evidence from Chinese cities. Environ. Sci. Pollut. Res. 24, 4457–4468. doi: 10.1007/s11356-016-8181-0
Shi, Y., Liu, R. M., Luo, Y., and Yang, K. (2020). Spatiotemporal variations of PM2.5 pollution evolution in China in recent 20 years. Environ. Sci. 41, 1–13. doi: 10.13227/j.hjkx.201905109
Song, M., Wu, J., Song, M., Zhang, L., and Zhu, Y. (2020). Spatiotemporal regularity and spillover effects of carbon emission intensity in China’s bohai economic rim. Sci. Total Environ. 740:140184. doi: 10.1016/j.scitotenv.2020.140184
The United Nations (2020). The sustainable development goals report 2020. New York, NY: The United Nations.
Tong, F., Huibin, D., Zhongguo, L., and Jian, Z. (2020). Spatial spillover effects of environmental regulations on air pollution: Evidence from urban agglomerations in China. J. Environ. Manag. 272:110998.
Vicedo-Cabrera, A. M., Annibale, B., Laura, G., Fabio, B., and Dolores, C. (2013). A Bayesian kriging model for estimating residential exposure to air pollution of children living in a high-risk area in Italy. Geospat. Health 8, 87–95. doi: 10.4081/gh.2013.57
Wang, F., Fan, W. N., Liu, J., Wang, G., and Chai, W. (2020). The effect of urbanization and spatial agglomeration on carbon emissions in urban agglomeration. Environ. Sci. Pollut. Res. 27, 24329–24341.
Wang, Z. B., and Fang, C. L. (2016). Spatial-temporal characteristics and determinants of PM2.5 in the Bohai Rim Urban agglomeration. Chemosphere 148, 148–162. doi: 10.1016/j.chemosphere.2015.12.118
Wen, X., Zhang, P. Y., and Liu, D. Q. (2018). Spatiotemporal variations and influencing factors analysis of PM2.5 concentrations in Jilin Province, Northeast China. Chin. Geogr. Sci. 28, 810–822.
Wolterbeek, H. T., and Verburg, T. G. (2004). A kriging-based estimate of the survey quality in biomonitoring of trace element air pollution. J. Atmos. Chem. 49, 15–22.
World Bank Group (2016). The Cost of Air Pollution: Strengthening the Economic Case for Action. Washington, DC: World Bank.
Wu, J., and Pu, Y. (2020). Air pollution, general government public-health expenditures and income inequality: Empirical analysis based on the SDM. PLoS One 15:e0240053. doi: 10.1371/journal.pone.0240053
Wu, X. P., Gao, M., Guo, S. H., and Li, W. (2019). Effects of environmental regulation on air pollution control in China, a spatial Durbin econometric analysis. J. Regul. Econ. 55, 307–333.
Xie, H., Ouyang, Z., and Choi, Y. (2020). Characteristics and influencing factors of green finance development in the Yangtze river delta of China: Analysis based on the spatial Durbin model. Sustainability 12:9753.
Xie, Y. Y., Zhao, B., Zhang, L., and Luo, R. (2015). Spatiotemporal variations of PM2.5 and PM10 concentrations between 31 Chinese cities and their relationships with SO2, NO2, CO and O-3. Particuology 20, 141–149.
Xiong, L., DeJong, M., Wang, F., Cheng, B., and Yu, C. (2018). Spatial spillover effects of environmental pollution in china’s central plains urban agglomeration. Sustainability 10:994. doi: 10.1016/j.ecoenv.2021.112772
Xue, W. H., Zhang, J., Zhong, C., Ji, D. Y., and Huang, W. (2020). Satellite-derived spatiotemporal PM2.5 concentrations and variations from 2006 to 2017 in China. Sci. Total Environ. 712:134577. doi: 10.1016/j.scitotenv.2019.134577
Yang, D. Y., Chen, Y. L., Miao, C. H., and Liu, D. X. (2020). Spatiotemporal variation of PM2.5 concentrations and its relationship to urbanization in the Yangtze river delta region, China. Atmos. Pollut. Res. 11, 491–498. doi: 10.3389/fpubh.2022.843862
Yang, J., and Xu, L. (2020). How does China’s air pollution influence its labor wage distortions? Theoretical and empirical analysis from the perspective of spatial spillover effects. Sci. Total Environ. 745:140843. doi: 10.1016/j.scitotenv.2020.140843
Yu, M. Y., Xu, Y., Li, J. Q., Lu, X. C., Xing, H. Q., and Ma, M. L. (2021). Geographic detector-based spatiotemporal variation and influence factors analysis of PM2.5 in Shandong, China. Pol. J. Environ. Stud. 30, 463–475.
Zhang, L. C., An, J., Liu, M., Li, Z. W., Liu, Y., Tao, L. X., et al. (2020). Spatiotemporal variations and influencing factors of PM2.5 concentrations in Beijing, China. Environ. Pollut. 262:144276.
Zhang, Q., Nakatani, J., Shan, Y., and Moriguchi, Y. (2018). Inter-regional spillover of China’s sulfur dioxide (SO2) pollution across the supply chains. J. Clean. Prod. 207, 418–431.
Zhao, S. P., Yin, D. Y., Yu, Y., Kang, S. C., Qin, D. H., and Dong, L. X. (2020). PM2.5 and O-3 pollution during 2015-2019 over 367 Chinese cities, Spatiotemporal variations, meteorological and topographical impacts. Environ. Pollut. 264:114694. doi: 10.1016/j.envpol.2020.114694
Zhao, X. L., Zhou, W. Q., Han, L. J., and Locke, D. (2019). Spatiotemporal variation in PM2.5 concentrations and their relationship with socioeconomic factors in China’s major cities. Environ. Int. 133(Part A):105145. doi: 10.1016/j.envint.2019.105145
Keywords: urban agglomeration, air quality, spillover effect, spatiotemporal variation, Kriging interpolation
Citation: Cui Z, Ren F-r, Wei Q and Xi Z (2022) What drives the spatio-temporal distribution and spillover of air quality in China’s three urban agglomerations? Evidence from a two-stage approach. Front. Ecol. Evol. 10:977598. doi: 10.3389/fevo.2022.977598
Received: 24 June 2022; Accepted: 03 August 2022;
Published: 14 September 2022.
Edited by:
Farrukh Chishtie, University of British Columbia, CanadaReviewed by:
Shaohua Zhao, Ministry of Ecology and Environment Center for Satellite Application on Ecology and Environment, ChinaCopyright © 2022 Cui, Ren, Wei and Xi. This is an open-access article distributed under the terms of the Creative Commons Attribution License (CC BY). The use, distribution or reproduction in other forums is permitted, provided the original author(s) and the copyright owner(s) are credited and that the original publication in this journal is cited, in accordance with accepted academic practice. No use, distribution or reproduction is permitted which does not comply with these terms.
*Correspondence: Fang-rong Ren, MTgwMjEzMTIwMDA4QGhodS5lZHUuY24=
†These authors have contributed equally to this work and share first authorship
Disclaimer: All claims expressed in this article are solely those of the authors and do not necessarily represent those of their affiliated organizations, or those of the publisher, the editors and the reviewers. Any product that may be evaluated in this article or claim that may be made by its manufacturer is not guaranteed or endorsed by the publisher.
Research integrity at Frontiers
Learn more about the work of our research integrity team to safeguard the quality of each article we publish.