- Department of Philosophy, University of Edinburgh, Edinburgh, United Kingdom
The purpose of this article is to show how the comparison or analogy with artifacts (i.e., systems engineered by humans) is foundational for the idea that complex neuro-cognitive systems are amenable to explanation at distinct levels, which is a central simplifying strategy for modeling the brain. The most salient source of analogy is of course the digital computer, but I will discuss how some more general comparisons with the processes of design and engineering also play a significant role. I will show how the analogies, and the subsequent notion of a distinct computational level, have engendered common ideas about how safely to abstract away from the complexity of concrete neural systems, yielding explanations of how neural processes give rise to cognitive functions. I also raise worries about the limitations of these explanations, due to neglected differences between the human-made devices and biological organs.
Introduction
It is worth remembering that the very word organism comes to us via an analogical transfer from the Greek word for tool (organon), and originally meant the property of things comprising heterogenous parts that work together in a coordinated way—a property pretty much captured by the word mechanism today (Cheung, 2006; Illetterati, 2014, p. 89). What this indicates is that even when drawing contrasts between organisms and machines, organs and artifacts, the concepts we are using to theorize living beings have originated through a process of comparison with objects that people have made. As philosopher Martin Heidegger observed, “[p]erhaps it will take a long time to realize that the idea of organism and of organic is a purely modern, mechanical-technical concept, so that what grows naturally by itself is interpreted as an artifact that produces itself” (quoted in Nunziante, 2020, p. 12).
This special issue invites us to weigh up the claim that all metaphors are false but some are useful. Incidentally, our notion of the useful, utility, is shaped by concept of the tool—the tool is the paradigmatic useful thing. This connection is made obvious in the French language, where the words for tool (outil) and useful (utile) are so similar. Thus, we have on the one hand, the root metaphor of the living being (organism) as a system of tool-like components (organs), and on the other, the question of whether metaphors gathered from the making and employment of tools and machines is itself a useful conceptual tool.
This essay will zoom in on one aspect of the comparison between immensely complex nervous systems, and relatively simple information processing machines: the idea that the brain, like the computer, can be explained at distinct and somewhat autonomous levels of analysis. I will account for the utility of this analogy as due to its providing a simplifying strategy for neuroscientists. The assumption that there is a “high level” description of the brain which can be modeled and comprehended in the absence of detailed knowledge of the “low level” components is motivated by consideration of the hardware/software distinction in computing. I will illustrate this strategy via an exposition of David Marr’s well known system of levels of explanation (section “Marr’s levels of explanation”). We will then see how the levels framework is motivated by analogies with machines, primarily computers, but also with the procedures that people undertake when making devices, here analyzing the influential ideas of Herbert Simon on hierarchical complex systems (section “The artifact analogies”). A risk of reliance on such analogies is that it leads to neglect of differences. All analogies are imperfect, but sometimes researchers forget this. The section “Limitations of the analogies” considers the limitations of the analogy between messy “heterarchical” biological systems and man-made designs that have a clearly delineated modularized and leveled structure. To conclude, I ask whether these limitations can be addressed through comparison with more life-like machines—as suggested in this special issue by Bongard and Levin (2021). I argue that their proposal neglects the problem of opacity that comes with the introduction of more complex machine models.
Immauel Kant is one philosopher whose account of biological knowledge recognized that the comparison between the workings of nature, and processes of engineering was indispensable to the conceptualization of living beings: both biology and engineering rely on functional notions, the understanding of certain processes happening for the sake of wider system-level goals. At the same time, he warned against an anthropomorphism that comes with taking this as the literal, ultimate truth about the natural world. He wrote in the Critique of the Power of Judgment that, “we picture to ourselves the possibility of the [biological] object on the analogy of a causality of this kind—a causality such as we experience in ourselves—and so regard nature as possessed of a capacity of its own for acting technically” (Kant, 1790/1952, Part II p.5/§361; Breitenbach, 2014). But as Illetterati (2014, p. 91) explains, “these kinds of notions, even if necessary, seem to maintain a sort of fictional character too: indeed, they have no justification in things themselves, but neither do they have their origin in mere human invention. They rather have their justification in the way subjects necessarily understand living beings.” I think that this is the right way to interpret machine analogies in biology, and engineering metaphors more generally: they are useful precisely because they allow scientists to figure nature in human terms, which is why they are—strictly speaking—false.
Marr’s levels of explanation
As is well known, Marr’s framework is introduced in the first chapter of his book, Vision (Marr, 1982, p. 25). The three levels are:
1. Computational theory
2. Representation and algorithm
3. Hardware implementation
The “top level” computational theory gives an abstract characterization of the performance of a system in terms of its generating a mapping of an input to an output. In addition, characterization at this level shows how that performance is related to environmental constraints and behavioral goals. Thus, the first level is to provide a functional characterization in both senses of the word: explicating a mathematical input-output mapping, and also illuminating the utility of the performance. The middle level involves specification of the format for representation of the inputs and outputs, and of the algorithm that transforms one into the other. The bottom level describes how the representations and algorithm are physically realized, for example in the electronic components of a computer vision system, or in the neurons of an animal’s retina.
In the next section I will say more about how analogies with machines motivate this three-level system, and why they are essential in the interpretation of it. Here we should note that Marr’s proposal carries on from a discussion of the limitations of reductionist approaches to explaining the visual system—attempts to understand how neural activity gives rise to useful perceptions of the environment by way of careful study of the anatomy and physiology of neurons. In effect, the reductionist is restricted to the bottom level of explanation. Marr (1982, p. 27) describes this approach as equivalent in futility with the attempt to understand bird flight just through the examination of feathers. As he asserts in the preamble to the three levels, “[a]lmost never can a complex system of any kind be understood as a simple extrapolation from the properties of its elementary components” (Marr, 1982, p. 19). The basic complaint against reductionism is that this is a strategy that quickly gets the investigator overwhelmed with details whose significance cannot be assessed because she lacks knowledge of the overall functionality of the system, and therefore has no working hypothesis about how the elementary components contribute to global properties and behavior. The shape of the forest is invisible because there are so very many leaves. The introduction of the two additional levels of explanation allows for lines of investigation that prioritize general questions about the system’s functionality and operations independently of investigation into implementational details. The upper two levels are levels of abstraction away from the concrete, complicated material system. Ideally, the results of these upper level investigations provide a map of what to look for in the concrete system, and a guide to interpreting the material details, even though the levels are only “loosely related” (Marr, 1982, p. 25).
One of the virtues of Marr’s framework, highlighted by later researchers, is that it offers this strategy for simplification.1 For example, Ballard (2015, p. 13) writes that it, “opened up thinking about the brain’s computation in abstract algorithmic terms while postponing the reconciliation with biological structures.” Speaking of level schemas more generally, Ballard emphasizes that, “[b]y telescoping through different levels, we can parcellate the brain’s enormous complexity into manageable levels” (2015, p. 18).
The artifact analogies
The general impression given by Marr’s presentation is that he does not care to set a division between engineered and living systems, between those that have (computational) functions, properly speaking, and those for which it is only a heuristic posit. A striking feature of Marr’s presentation is that in the first instance it relies exclusively on examples of information processing machines. Cases from within neuroscience are mentioned only after a complete account of the three levels has been given, without there being any comment on this transition. The primary illustration of the levels comes by way of a cash register, an adding machine. At the computational level, the task is to find out “what the device does and why.” (Marr, 1982, p. 22).2 This means specification of the arithmetical theory of addition, as well as an account of the functional role of the machine for adding up charges in a shop. We learn that the second level characterization involves showing how numbers are represented in the device (e.g., Arabic or Roman notation), and specifying the algorithm used to work out the total bill. The implementation level involves characterization of the “physical substrate” which runs the algorithm. A point Marr (1982, p. 24) emphasizes is that the same algorithm can be realized in very different materials. This also goes for the relationship between the top two levels: one and the same computational task can be achieved by a range of different algorithms. This is why the levels are only “loosely related” (p. 25)—a discovery at one level cannot reliably pre-specify what will be found at the level below.
We might speculate that Marr leans on artifacts for purposes of exposition just because the core concept of each of these levels comes out especially clearly in cases like the cash register. But then we ought to wonder why it is that it is harder to get a grip on how to define these levels in neuroscience, even though the framework is intended for use there. We can discern a deeper reason for the primacy of machines in Marr’s exposition if we consider Dennett’s observation that the three levels actually schematize the stages taken in the engineering of a complex information processing system. Dennett (1995, p. 682) writes,
Marr’s obiter dicta [passing words] on methodology gave compact and influential expression to what were already reigning assumptions in Artificial Intelligence. If AI is considered as primarily an engineering discipline, whose goal is to create intelligent robots or thinking machines, then it is quite obvious that standard engineering principles should guide the research activity:
first you try to describe, as generally as possible, the capacities or competences you want to design,
and then you try to specify, at an abstract level, how you would implement these capacities,
and then, with these design parameters tentatively or defeasibly fixed, you proceed to the nitty-gritty of physical realization.
The point here is that the three levels of explanation are an expression of three broad steps in the forward engineering of a machine with some functionality equivalent to a cognitive capacity in an animal. It is then not surprising that the different levels are more easy to illustrate with an example of reverse engineering some such device.
The issue I am highlighting here is that artifacts are the foundational cases for Marr’s framework, and the application to neuroscience occurs via an analogical transfer to brains, systems which are arguably similar to computing ones. Researchers habitually think of brains, just like the artifacts, as taking in inputs (e.g., from sensory organs), implementing some algorithms, and sending an output (e.g., a motor command).3 The importance of this analogy comes out in Dennett’s characterization of what his own approach has in common with that of Marr and cognitive scientist Allen Newell, namely:
stress on being able (in principle) to specify the function computed …. independently of the other levels. an optimistic assumption of a specific sort of functionalism: one that presupposes that the concept of the function of a particular cognitive system or subsystem can be specified (It is the function which is to be optimally implemented.) A willingness to view psychology or cognitive science as reverse engineering in a rather straightforward way. Reverse engineering is just what the term implies: the interpretation of an already existing artifact by an analysis of the design considerations that must have governed its creation (Dennett, 1995, p. 683).
Dennett’s articulation of the reverse engineering methodology, his design stance, comes with strict assumptions of optimality and adaptationism in evolved systems that we need not attribute to the scientific practice. In my view, the essential point about the reverse engineering methodology is that it treats the biological object by analogy with a man-made thing, and in this way attempts to make it intelligible by showing how it operates according to principles that make sense from the perspective of a person designing things; in other words, by treating it as if it were an artifact, the scientist can explain it in terms of the practical rationality of causal means being used to produce useful effects.
We should appreciate that there are two levels of analogy, so to speak. Superficially, the analogy just holds between certain organs of living bodies and man-made devices that have a rough functional equivalence with them—the brain and a computer, the heart and a pump. But the deeper and more general point—the one spelled out by Kant—is that there is an analogy being invoked between the systematic organization of parts and processes through which organs generate their functional effects, and the parts and processes set in place by a human engineer in order for a device to achieve the desired effect. An artifact is intelligible to the extent that its operations are the manifestations of the instrumental rationality through which its human makers have put components together in order to achieve their goals. A similar kind of intelligibility is tacitly assumed for the biological object. This becomes clearer when we consider functional analysis, which is a general schema for reverse engineering (see Figure 1).
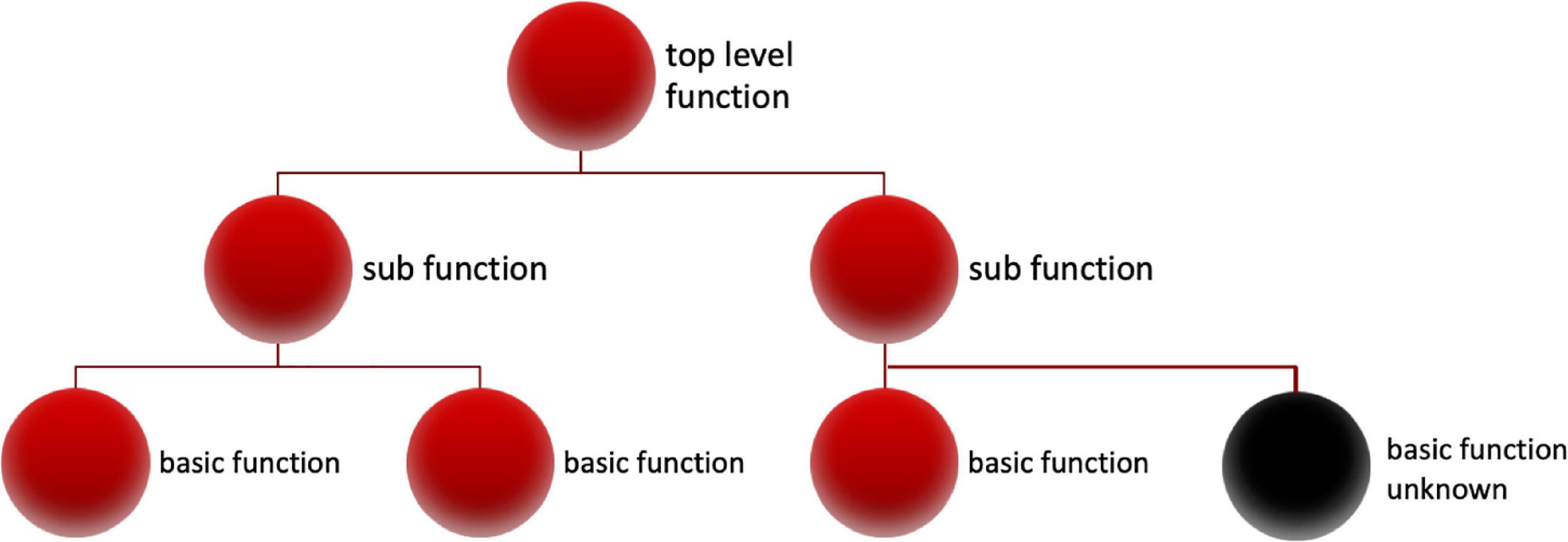
Figure 1. Reverse engineering is expressed schematically as performance of a functional analysis (Cummins, 1975, Cummins, 2000). The top level function of the whole system is decomposed into sub-functions, which can themselves be explained in terms of the interaction of basic functions. See also Bechtel and Richardson (2010) on the research strategy of functional decomposition, employed for investigating modular, hierarchical systems.
The link between this reverse engineering methodology in cognitive and neuroscience, and simplification of the brain becomes apparent if we focus on the importance of encapsulation in functional analysis. When a system is described in this way, the payoff is that at any given level of analysis the component modules can be treated as black boxes whose inner workings are either unknown or ignored, since the only information relevant to the current level of analysis is the input-output profiles of the modules. Descent to a lower level of analysis involves opening the black boxes and seeing how their inner workings can be accounted for in terms of the functional capacities of their components. But for many explanatory purposes, lower level details can safely be kept out of view, which is why this methodology offers a handy simplification.
To illustrate this point, I will make use of an example from computing given by Ballard (2015, p. 14ff.). Most people who program computers only ever use a high level programming language such as Python. But the terms of this high level language are actually black boxes which unpack into more complicated expressions in a lower level assembly language. These lower level terms themselves unpack into instructions in machine code. For a program to be carried out, it needs to be translated down into lower level languages, “closer to machine’s architecture.” But this is all done behind the scenes and the ordinary coder can comfortably stick with description of the computation in the compact, highest level language. The point of Ballard’s example here is to argue that there is a tight analogy between the computer and the brain, which he thinks can be described similarly in terms of “levels of computational abstraction.”
Crucially, the abstraction hierarchy is posited to be there in the brain’s own representations of the extra-cranial world, not just in those imposed upon it by a scientist. The proposal is that the brain is a system that, at the top level of control, ignores its own complexity, like a digital computer where the execution of a piece of code is indifferent to micro-physical fluctuations in the electronic hardware. Just as the programmer, the controller of a computer, can govern the performances of the machine while ignoring and remaining ignorant of its low-level languages and physical workings, it is supposed that the brain systems ultimately responsible for behavior employ an abstract, high level system of representation that is invariant to changes in the complex, low-level workings of the brain and rest of the body. If this assumption holds, there are good prospects for a relatively simple computational theory that explains how the brain governs behavior, by way of these high-level representations.
But why would neuroscientists think that this assumption does hold, that the analogy between computer and brain is tight enough? The intelligible organization of systems as hierarchically arranged, encapsulated modules, or levels of more or less abstract representations, can be found in artifacts designed by humans, but its existence in the natural world should not be taken for granted. As far as I can determine, the foundational argument in support of this assumption comes from another analogy put forward by Herbert Simon in the “Architecture of Complexity” (Simon, 1962, 1969).4 In a tale of two watchmakers, Simon describes how the production of a complex system (a watch) is much more likely to be successful if the production process occurs in stages, where sub-processes in the production result in stable sub-components of the system that are assembled together at a later stage. Simon then draws an analogy between human manufacture and the evolution of complex life forms. His point is that the likelihood of evolution producing organisms of any complexity is vanishingly small unless it is the case that it comes about via the evolution of intermediate, self-standing forms that become the components of more complex organisms. Hence, he argues, it must be the case that evolved, as well as manufactured complex systems are composed, hierarchically, of relatively independent sub-systems. In these near decomposable complex systems, there is only a weak frequency and strength of interaction horizontally between the subsystems at any one level, and vertically across the levels of organization. This means that the subsystems-the modular components-can usefully be studied in isolation from the rest of the system, and that the system can be studied at higher levels of organization (which we can here equate to larger scales) without attention to most of the lower level (i.e., small scale) details. The optimistic upshot is that evolved complex systems are scientifically intelligible through decomposition into levels and components, and that this is an alternative to intractable reductionist methodologies.5
Reductionist methodologies can be successful for relatively simple systems. The task of the research is to acquire sufficient information about the elementary components, and their interaction, to yield an explanation of the behavior of the whole system. This is a “flat,” as opposed to multi-level, approach. Once there is enough complexity that the amount of information about elementary components and the interactions that can feasibly be dealt with (in models or theory) is much less than what is required for explanation of the system’s behavior, then a multi-level approach is needed. The common virtue of all of the multi-level approaches discussed above—from Marr, Ballard, and Simon—is that they offer a guide for how to abstract away from low-level details and how to set about work on top-down explanations when bottom-up, reductionist approaches are intractable, even if possible in principle. These three scientists are all advocates of computational explanations of how the brain gives rise to cognition, and this kind of explanation is favored because, they argue, it does not require that much attention be paid to the details of neurophysiology which would otherwise threaten an overwhelming complexity.
An additional feature of computational explanations is that they assert an equivalence between organic and artificial systems, so long as they are computing the same functions. This is known in philosophy as multiple-realization. A mechanical cash register, an electronic calculator, and a human brain region, can all be said to be doing the same computation when adding up a particular sum, even though the physical substrates are so different. The benefit of this for neuroscientific research is that it justifies the substitution of actual neural tissue with relatively simple computational models, such as artificial neural networks (ANNs), as objects of investigation. A goal of various neuro-computational research projects has been to create models of brain areas in silico that will yield confirmatory or disconfirmatory evidence for theories of cognition and pathology, where traditional experimental approaches are untenable because it is not possible to make the required interventions on actual neurons. Even though large ANNs are themselves rather complicated and hard to interpret, they are at least more accessible to (simulated) experimental interventions, such as lessoning of individual nodes.
Aside from the specifics of computational explanation (explanation via analogy between brains and computers), one of the general implications of the artifact analogy is that the nervous system is composed of relatively encapsulated working parts (modules) or functional components. This also supports the “black-boxing” of neural details. As Haugeland (1978, p. 221) relates,
if neurons are to be functional components in a physiological system, then some specific few of their countless physical, chemical, and biological interactions must encapsulate all that is relevant to understanding whatever ability of that system is being explained.
One way to think about the importance of neuron doctrines in the history of the discipline-theories that posit individual neurons as the basic anatomical and functional units of the nervous system-is that they facilitate this simplifying strategy, even while departing from many of the observable results on the significance of sub-neuronal and non-neuronal structures and interactions.6 Moreover, we should note also that this black-boxing can be employed to achieve abstract representations of functional components other than individual neurons (e.g., Hawkins et al., 2017 model of cortical columns).
Limitations of the analogies
I have argued that the dominant multi-level approaches in neuroscience rest on the assertion of there being a close similarity between the multi-level organization of artifacts such as computers, and the brain, an evolved organ whose organizational “plan” is far less well characterized than that of the machine, and remains a matter of controversy. This prompts consideration of the difficulties that the multi-level approach faces, to the extent that the claim for similarity can be challenged. If the comparison between brain and computer is at best a loose analogy, in which the dissimilarities between the two are of equal importance or even outnumber the similarities, then the leveled approach might sometimes be a hindrance in the project of explaining how brain activity gives rise to cognition.
The first concern to bring up here is that the case for encapsulation in the nervous system is fairly weak. This was pointed out decades ago by Haugeland, in the passage following on from the one quoted above:
[encapsulation] is not at all guaranteed by the fact that cell membranes provide an anatomically conspicuous gerrymandering of the brain. More important, however, even if neurons were components in some system, that still would not guarantee the possibility of “building back up.” Not every contiguous collection of components constitutes a single component in a higher level system; consolidation into a single higher component requires a further encapsulation of what’s relevant into a few specific abilities and interactions—usually different in kind from those of any of the smaller components. Thus the tuner, pre-amp and power amp of a radio have very narrowly specified abilities and interactions, compared to those of some arbitrary connected collection of resistors, capacitors, and transistors. The bare existence of functionally organized neurons would not guarantee that such higher level consolidations were possible. Moreover, this failure of a guarantee would occur again and again at every level on every dimension. There is no way to know whether these explanatory consolidations from below are possible, without already knowing whether the corresponding systematic explanations and reductions from above are possible—which is the original circularity (Haugeland, 1978, p. 221).
It is interesting that Haugeland focuses on the possibility of a strong disanalogy between the organization of the nervous system, and that of a human-designed artifact, a radio. Whereas it is a feature of the design of a radio that higher level sub-components (the tuner, pre-amp and power amp) are made up of careful arrangements of lower level sub-components (resistors, capacitors, and transistors), and themselves have narrowly specified capacities and input-output profiles, it should not be assumed that collections of neurons consolidate into higher level sub-components in this way, and that explanations of the neural basis of cognition can safely be restricted to the higher levels. I will now discuss two reasons to be skeptical that the analogy holds. The first relates to the potential importance of low-level activity, the second brings up the difference between hierarchical, designed systems and evolved ones.
It is an open possibility that cognition is the product of dense interactions across a number of levels or scales, and is not restricted to a high level of computational abstraction, as hypothesized by Ballard. The cognitive properties of the brain may be enmeshed in its material details, in a way not congenial to Marr’s vision of a there being computational and algorithmic/representation levels that are only loosely related to the implementational one. A reason to give credence to these possibilities comes from consideration of the fact that biological signaling, a general feature of living cells, is the omnipresent background to neuronal functionality. The low level details of neuronal activity can themselves be characterized as doing information processing, and are not merely the hardware implementors of the system’s global computations, or bits of infrastructure keeping the system running. This is an argument put forward by Godfrey-Smith (2016, p. 503):
This coarse-grained cognitive profile is part of what a living system has, but it also has fine-grained functional properties—a host of micro-computational activities in each cell, signal-like interactions between cells, self-maintenance and control of boundaries, and so on. Those finer-grained features are not merely ways of realizing the cognitive profile of the system. They matter in ways that can independently be identified as cognitively important.
The point is that in an electronic computer there is a clean separation of the properties of the physical components that are there holding the device together, and the ones involved specifically in information processing. This is how the machine has been designed. Whereas in the brain this is not the case-it is not clear cut which entities within the brain, and which of their properties, are responsible for information processing, and which are the infrastructural background.7 In addition to the “coarse-grained” computations that might be attributed on the basis of the whole animal’s psychology and behavior, Godfrey-Smith argues that there are a countless number of “micro-computational activities” in cells, which are not unrelated to global cognition. If in the brain metabolism, cell-maintenance, and global (i.e., person-level) cognitive functions are enmeshed together, then low level material details about neural tissue, such as the specific chemical structures of the many kinds of neurotransmitter, and the thousands of proteins expressed at synapses (Grant, 2018), probably do matter to the explanation of cognition. They cannot be safely discounted with the same confidence as merited in aeronautics, when air is treated as a continuous fluid and molecular details are left unrepresented.8
We saw that Herbert Simon gives an in principle argument for the existence of hierarchical organization in complex living systems which would, if accepted, justify the exclusion of low level details for the purposes of most explanations of whole system behavior. However, the strict analogy this argument supposes, between human manufacture and the processes of evolution, calls for scrutiny. Bechtel and Bich (2021) argue that hierarchical control structures, with their neat pyramidal arrangement of superordinate and subordinate levels, are less likely to evolve than heterarchical systems, which have a more haphazard arrangement of horizontal and vertical interconnections, meaning that one component of the system is open to significant influence from components at other levels (they are not just “loosely related”), and there is no top-level locus of control, as posited by Ballard (2015, p. 242) in his comparison between control in robots and humans. The reason for the hypothesized predominance of heterarchical systems is that evolution is not like a smooth, linear, process of design and manufacture, but is full of processes comparable with those engineers would call ‘‘tinkering’’ and ‘‘kludging.’’9 A common occurrence in evolution is that a trait that is adaptive because serving one function is co-opted for another, and so it is not obvious what the function of the trait is in the subsequent system. Co-option and functional multi-tasking are reasons why evolved systems have the heterarchical character of interactions ranging across levels. Generally speaking, to the extent that evolution is “inelegant” and divergent from the designs that would be considered rational and perhaps optimal by a human engineer, there is an obstacle to understanding organic systems through reverse engineering. This is a point made by Kitcher (1988) in relation to Marr’s levels, and is reiterated by these biologists more recently:
deep degeneracy at all levels is an integral part of biology, where machineries are developed through evolution to cope with a multiplicity of functions, and are therefore not necessarily optimized to the problem that we choose to reverse engineer. Viewed in this way, our limitation in reverse engineering a biological system might reflect our misconception of what a design principle in biology is. There are good reasons to believe that this conclusion is generally applicable to reverse engineering in a wide range of biological systems (Marom et al., 2009, p. 3).
Of course, Dennett is aware that the strong assumption of optimality cannot be expected to hold in many cases, but he would advocate for it as a first approximation: the initial prediction is that the evolved system conforms to the expectation based on optimality considerations, and then we look for divergences from this prediction. In this way, reverse engineering retains its heuristic value for biology.
However, we might become less sanguine about the value of this strategy as a heuristic, the more we attend to the worry that cases of conformity to the predictions based on human design considerations are likely to be rare—the first approximation is likely to be just too wide off the mark. On signaling networks in living cells, Moss (2012) points to research findings of everything “cross-talking” to everything else. Such networks are nowhere near the ideal of a hierarchical and near decomposable system. Application of a neat, leveled explanatory framework would only be Procrustean. Both Moss (2012) and Nicholson (2019, p. 115) point to a problem with the wiring diagrams commonly used to represent such networks, based on an analogy with electronic networks, because they lead researchers to underestimate the dynamic nature of these signaling pathways, in comparison with a fixed circuit structure.10 There is a felt need for better analogies, but perhaps they will not be available for the very reason that human engineered systems—at least when they are intelligible enough to usefully serve as analogies—are too fundamentally different from the evolved ones.
A somewhat controversial view on what is distinctive about natural systems, such that the assumption of near decomposability does not hold, is that they show emergence, meaning that higher level structures impose downward causation on their component parts (Green, 2018). On this view, living systems do have leveled architectures, though radically different from the ones found in artifacts for which the assumption of near decomposability does hold. It is interesting to note that there are new frameworks for engineering, which allow for machines to assemble themselves rather than be constructed according to a transparent, rational plan. It has been argued that some of these artifacts are not modular and near decomposable, and that they may show emergence (see section “Conclusion”).
To summarize, my considerations about the difference between living systems and artifacts, boil down to a concern about oversimplification. By making the assumption that living systems such as the nervous system have distinct levels of organization (without downward causation), and using this to justify leveled frameworks in neuroscientific explanation, the density and complexity of brain interactions are most likely being vastly under-estimated. Perhaps this does not matter for a range of predictive and technical purposes, but it does undermine more ambitious claims of level-based theories to be unlocking the riddles of information processing in the brain. Potochnik (2021, p. 24) states the general worry in a compelling way:
our adherence to the levels concept in the face of the systematic problems plaguing it amounts to a failure to recognize structure we’re imposing on the world, to instead mistake this as structure we are reading off the world. Attachment to the concept of levels of organization has, I think, contributed to underestimation of the complexity and variability of our world, including the significance of causal interaction across scales. This has also inhibited our ability to see limitations to our heuristic and to imagine other contrasting heuristics, heuristics that may bear more in common with what our world turns out to actually be like.
The prospect of alternative heuristics is the loaded question. Better notions of levels may yet arise from multi-scale modeling in systems biology. But it could well be that the over-simplifications imposed by artifact analogies and traditional level frameworks are indispensable for making such complex biological systems intelligible to human scientists, given our finite cognitive capacities. In which case, there may be no overall improvement in the heuristics, because any attempts to get closer to the actual complexity of the targets result in a loss of tractability and intelligibility. In which case researchers can, without condemnation, settle for the heuristics that they have, but they should uncouple advocacy of their modest explanatory utility from any stronger claims about brains being computers or organisms being machines.
Conclusion
In this special issue, Bongard and Levin (2021) argue, against Nicholson (2019), that twenty first century machines, such as deep convolutional neural networks (DCNN’s), do not have the rigid, modular qualities that, according to Nicholson, make them misleading as models for biological systems. What Bongard and Levin do not consider is that the utility of the analogies is likely to decline once reference is made to self-organizing devices like DCNN’s, which do not have the intelligibility of simpler, explicitly designed machines. While the analogy between organisms and machines may become tighter, with the development of machines that are more life-like—that are not modular, and which lack a clear hardware/software division—the motivation for drawing the analogies in the first place may evaporate. For, I have argued in this essay that the payoff of thinking about brains in terms of machine-based comparisons is that it aids explanation by framing the biological object in terms of transparent principles of human-led design. Self-organizing machines lack this attractive transparency. That machines would 1 day become inscrutable was a situation long ago envisaged by one of the first proponents of artificial intelligence and artificial life, John von Neumann:
At the Hixon Symposium, finding himself taxed by the neurophysiologists … for not stressing enough the difference between natural and artificial automata, he replied that this distinction would grow weaker over time. Soon, he prophesied, the builders of automata would find themselves as helpless before their creations as we ourselves feel in the presence of complex natural phenomena (Dupuy, 2009, p. 142).
That said, we should not be tempted to conclude that self-organizing, twenty first century machines are absolutely life-like. The problem is that given our relative ignorance about how they work, in comparison with classical machines, we risk also being left in the dark about all the ways they too are not like organisms.
Author contributions
The author confirms being the sole contributor of this work and has approved it for publication.
Conflict of interest
The author declares that the research was conducted in the absence of any commercial or financial relationships that could be construed as a potential conflict of interest.
Publisher’s note
All claims expressed in this article are solely those of the authors and do not necessarily represent those of their affiliated organizations, or those of the publisher, the editors and the reviewers. Any product that may be evaluated in this article, or claim that may be made by its manufacturer, is not guaranteed or endorsed by the publisher.
Footnotes
- ^ Of course, the details of Marr’s framework have been criticized by later researchers, such as Love (2021), who argue for a greater number of levels. Gurney (2009) proposes a four-level framework which is incidentally more similar to one proposed by Marr in a 1976 technical report.
- ^ To reinforce this point about the primacy of artifacts, note that Marr does not use the neutral language of “things” or “systems” but refers specifically to a “device” here. We find this also in the legend for the summary table: “The three levels at which any machine carrying out an information-processing task must be understood” (p. 25 emphasis added). Cf. “the different levels at which an information processing device must be understood before one can be said to have understood it completely” (p. 24 emphasis added). Later in the book, when again summarizing the three levels as applied to the visual system, it is interesting that the terms “machine” and “machinery” are still used: “The human system is a working example of a machine that can make such descriptions, and as we have seen, one of our aims is to understand it thoroughly, at all levels: What kind of information does the human visual system represent, what kind of computations does it perform to obtain this information, and why? How does it represent this information, and how are the computations performed and with what algorithms? Once these questions have been answered, we can finally ask, How are these specific representations and algorithms implemented in neural machinery?” (Marr, 1982, p. 99).
- ^ E.g., Marcus and Freeman (2015, p. xiii): “The brain is not a laptop, but presumably it is an information processor of some kind, taking in inputs from the world and transforming them into models of the world and instructions to the motor systems that control our bodies and our voices.” See Chirimuuta (2021; under contract) on why this practice should be interpreted as resting on a loose analogy rather than strict functional similarity between computer and brain.
- ^ It is interesting that Marr (1982, p. 102) also makes the connection between evolvability, intelligibility, and modular organization: “This observation [of isolated visual processing] …. is fundamental to our approach, for it enables us to begin separating the visual process into pieces that can be understood individually. Computer scientists call the separate pieces of a process its modules, and the idea that a large computation can be split up and implemented as a collection of parts that are as nearly independent of one another as the overall task allows, is so important that I was moved to elevate it to a principle, the principle of modular design. This principle is important because if a process is not designed in this way, a small change in one place has consequences in many other places. As a result, the process as a whole is extremely difficult to debug or to improve, whether by a human designer or in the course of natural evolution, because a small change to improve one part has to be accompanied by many simultaneous, compensatory changes elsewhere. The principle of modular design does not forbid weak interactions between different modules in a task, but it does insist that the overall organization must, to a first approximation, be modular.”
- ^ See Bechtel and Richardson (2010) for further discussion of methods for investigating near decomposable systems.
- ^ See Bullock et al. (2005) and Cao (2014) on the empirical inadequacy of the neuron doctrine. Barlow (1972) is a great example of its role in explanatory simplification.
- ^ For example, glial cells—the very numerous kinds of brain cells that do not generate action potentials—were long thought to be providing metabolic support, but not involved in cognition. This does not appear to be the case, but the challenge of integrating glia into computational theory is immense (Kastanenka et al., 2019).
- ^ Lillicrap and Kording (2019) also argue against the comparison between coarse-graining methods in physics and computational explanation in neuroscience.
- ^ We should note here that Ballard’s representation of software systems as neat and pyramidal is itself an idealization, since large programs like Microsoft Word are themselves the result of years of tinkering and kludging of previous versions of the code.
- ^ “Perhaps the most significant barrier to appreciating the dynamic, heterogeneous aspect of signaling complexes is the lack of a good analogy from our daily experience. This contributes to a second related problem, our inability to depict such interactions diagrammatically. Indeed, the typical “cartoon” of signaling pathways, with their reassuring arrows and limited number of states could be the real villain” (Mayer et al., 2009, p. 6, quoted in Moss, 2012, p. 170).
References
Barlow, H. (1972). ‘Single units and sensation: A neuron doctrine for perceptual psychology?’. Perception 1, 371–394. doi: 10.1068/p010371
Bechtel, W., and Bich, L. (2021). ‘Grounding cognition: Heterarchical control mechanisms in biology’. Philos. Trans. R. Soc. B 376:20190751. doi: 10.1098/rstb.2019.0751
Bechtel, W., and Richardson, R. C. (2010). Discovering Complexity. Cambridge, MA: MIT Press. doi: 10.7551/mitpress/8328.001.0001
Bongard, J., and Levin, M. (2021). ‘Living things are not (20th century) machines: Updating mechanism metaphors in light of the modern science of machine behavior’. Front. Ecol. Evol. 9:650726. doi: 10.3389/fevo.2021.650726
Breitenbach, A. (2014). “’Biological purposiveness and analogical reflection,” in Kant’s Theory of Biology, eds I. Goy and E. Watkins (Berlin: Walter De Gruyter). doi: 10.1515/9783110225792.131
Bullock, T. H., Bennett, M. V., Johnston, D., Josephson, R., Marder, E., and Field, R. D. (2005). ‘The neuron doctrine, redux. Science 310, 791–793. doi: 10.1126/science.1114394
Cao, R. (2014). ‘Signaling in the brain: In search of functional units’. Philos. Sci. 81, 891–901. doi: 10.1086/677688
Cheung, T. (2006). ‘From the organism of a body to the body of an organism: Occurrence and meaning of the word ‘oganism’ from the seventeenth to the nineteenth centuries’. Br. J. Hist. Sci. 39, 319–339. doi: 10.1017/S0007087406007953
Chirimuuta, M. (2021). “’Your brain is like a computer: Function, analogy, simplification,” in Neural Mechanisms: New Challenges in the Philosophy of Neuroscience, eds F. Calzavarini and M. Viola (Berlin: Springer). doi: 10.1007/978-3-030-54092-0_11
Chirimuuta, M. (under contract). The Brain Abstracted: Simplification in the History and Philosophy of Neuroscience. Cambridge, MA: MIT Press.
Cummins, R. (2000). “How does it work?” Versus “What are the laws?”: Two conceptions of psychological explanation,” in Explanation and Cognition, eds F. C. Keil and R. A. Wilson (Cambridge, MA: MIT Press).
Dennett, D. C. (1995). “Cognitive science as reverse engineering: Several meanings of “Top-Down” and “Bottom-Up,” in Proceedings of the Ninth International Congress of Logic, Methodology and Philosophy of Science, eds D. Prawitz, B. Skyrms, and D. Westerståhl (Uppsala), 680–689. doi: 10.1016/S0049-237X(06)80069-8
Godfrey-Smith, P. (2016). ‘Mind, matter, and metabolism’. J. Philos. 113, 481–506. doi: 10.5840/jphil20161131034
Grant, S. (2018). ‘Synapse molecular complexity and the plasticity behaviour problem’. Brain Neurosci. Adv. 2, 1–7. doi: 10.1177/2398212818810685
Green, S. (2018). ‘Scale dependency and downward causation in biology’. Philos. Sci. 85, 998–1011. doi: 10.1086/699758
Gurney, K. N. (2009). ‘Reverse engineering the vertebrate brain: Methodological principles for a biologically grounded programme of cognitive modelling’. Cogn. Comput. 1, 29–41. doi: 10.1007/s12559-009-9010-2
Haugeland, J. (1978). ‘The nature and plausibility of cognitivism’. Behav. Brain Sci. 2, 215–226. doi: 10.1017/S0140525X00074148
Hawkins, J., Ahmad, S., and Cui, Y. (2017). ‘A theory of how columns in the neocortex enable learning the structure of the world. Front. Neural Circuits 11:81. doi: 10.3389/fncir.2017.00081
Illetterati, L. (2014). “’Teleological judgment: Between technique and nature,” in Kant’s Theory of Biology, eds I. Goy and E. Watkins (Berlin: Walter De Gruyter). doi: 10.1515/9783110225792.81
Kastanenka, K. V., Moreno-Bote, R., De Pittà, M., Perea, G., Eraso-Pichot, A., Masgrau, R., et al. (2019). ‘A roadmap to integrate astrocytes into systems neuroscience’. Glia 68, 5–26. doi: 10.1002/glia.23632
Kitcher, P. (1988). ‘Marr’s computational theory of vision’. Philos. Sci. 55, 1–24. doi: 10.1086/289413
Lillicrap, T. P., and Kording, K. (2019). ‘What Does it Mean to Understand a Neural Network?. Available online at: https://arxiv.org/abs/1907.06374 (accessed July 10, 2020).
Love, B. C. (2021). ‘Levels of biological plausibility’. Philos. Trans. R. Soc. B 376:20190632. doi: 10.1098/rstb.2019.0632
Marcus, G., and Freeman, J. (2015). “Preface,” in The Future of the Brain, eds G. Marcus and J. Freeman (Princeton, NJ: Princeton University Press). doi: 10.1515/9781400851935
Marom, S., Meir, R., Braun, E., Gal, A., Kermany, E., and Eytan, D. (2009). ‘On the precarious path of reverse neuro-engineering’. Front. Computat. Neurosci. 3:5. doi: 10.3389/neuro.10.005.2009
Mayer, B., Blinov, M., and Loew, L. (2009). ‘Molecular machines or pleiomorphic ensembles: Signaling complexes revisited’. J. Biol. 8:81. doi: 10.1186/jbiol185
Moss, L. (2012). ‘Is the philosophy of mechanism philosophy enough?’. Stud. Hist. Philos. Biol. Biomed. Sci. 43, 164–172. doi: 10.1016/j.shpsc.2011.05.015
Nicholson, D. J. (2019). ‘Is the cell really a machine?’. J. Theor. Biol. 477, 108–126. doi: 10.1016/j.jtbi.2019.06.002
Nunziante, A. M. (2020). “’Between laws and norms. Genesis of the concept of organism in leibniz and in the early modern western philosophy,” in Natural Born Monads, eds A. Altobrando and P. Biasetti (Berlin: Walter de Gruyter). doi: 10.1515/9783110604665-002
Potochnik, A. (2021). “’Our world isn’t organized into levels,” in Levels of Organization in Biology, eds D. Brooks, J. DiFrisco, and W. C. Wimsatt (Cambridge, MA: MIT Press).
Keywords: philosophy of neuroscience, levels of abstraction, levels of explanation, analogy, philosophy of cognitive science
Citation: Chirimuuta M (2022) Artifacts and levels of abstraction. Front. Ecol. Evol. 10:952992. doi: 10.3389/fevo.2022.952992
Received: 25 May 2022; Accepted: 08 July 2022;
Published: 09 August 2022.
Edited by:
Pedro Martinez, University of Barcelona, SpainReviewed by:
Javier Suárez, Jagiellonian University, PolandDenis Walsh, University of Toronto, Canada
Copyright © 2022 Chirimuuta. This is an open-access article distributed under the terms of the Creative Commons Attribution License (CC BY). The use, distribution or reproduction in other forums is permitted, provided the original author(s) and the copyright owner(s) are credited and that the original publication in this journal is cited, in accordance with accepted academic practice. No use, distribution or reproduction is permitted which does not comply with these terms.
*Correspondence: M. Chirimuuta, bS5jaGlyaW11dXRhQGVkLmFjLnVr