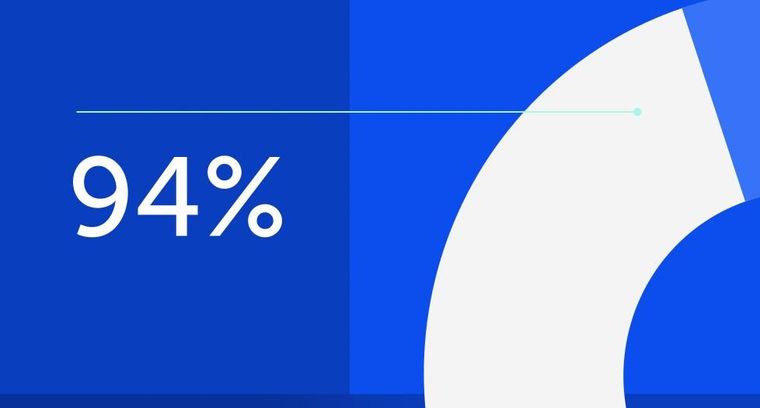
94% of researchers rate our articles as excellent or good
Learn more about the work of our research integrity team to safeguard the quality of each article we publish.
Find out more
REVIEW article
Front. Environ. Sci., 03 March 2025
Sec. Environmental Informatics and Remote Sensing
Volume 13 - 2025 | https://doi.org/10.3389/fenvs.2025.1549301
Monitoring water quality is crucial for sustainable water management and meeting the United Nations Sustainable Development Goals. Urbanisation, agricultural practices, industrial activities, and population growth increase the presence of biological, chemical and physical properties in water bodies. Traditional water quality monitoring methods (laboratory and in situ measurements) are limited spatially, temporarily and are costly. Satellite remote sensing has been shown to provide a systematic, cost-effective, and near-real-time alternative. This paper analysed 142 peer-reviewed articles published between 2002 and 2024 from Web of Science and Scopus databases. The final included articles in the review were achieved through the PRISMA flowchart. The review revealed that low-resolution sensors with long-term records, such as MODIS, were commonly applied to study large lakes. In contrast, sensors such as Landsat-8 and Sentinel-2 were applied for both lakes and dams. These sensors contain necessary spectral regions for monitoring water quality, where it was shown that the 500–600 nm region is critical for chlorophyll assessment, while the 640–670 nm region is used for turbidity. The Secchi disk depth and the total suspended solids were assessed using regions 860–1040 nm and 1570–1650 nm. Water quality research also focused on countries such as China, India, Brazil, and South Africa, with an emphasis on optically active parameters. There is, however, limited research on non-optically active parameters, such as nitrogen, phosphorus, and temperature, especially in small inland water bodies. Therefore, there is a need for more research in these areas, using direct and indirect methods of water quality parameter estimation with the integration of machine learning algorithms.
The global management and protection of water resources is of critical importance due to pressures from population growth, industrial activities, agricultural practices, and climate change (Chathuranika et al., 2023). Sustainable Development Goal (SDG) 6 of the United Nations (UN) prioritises the sustainable management and accessibility of water and sanitation for all, reflecting a worldwide commitment to tackling water-related challenges by 2030. Insufficient sanitation and polluted water pose serious health risks, promoting the spread of diseases like cholera, hepatitis, and typhoid (Zainurin et al., 2022). Therefore, environmental and water quality monitoring of rivers and other water bodies is essential for their sustainable use (Yang and Jin, 2023). Water quality monitoring focuses on the biological, chemical and physical parameters of water and has been completed through laboratory tests and in situ testing (Ahmed et al., 2020). In laboratory tests, water samples are collected at key locations and examined in laboratories to determine the concentration of chemical, physical and biological parameters of water that indicate their quality for a particular purpose (Jaji et al., 2007). In-situ testing involves measuring water quality parameters directly from the water body, leading to a real-time understanding of water quality while preventing potential contamination that may be incurred in transit to the laboratory (Liber et al., 2016).
However, these approaches have limitations in terms of spatial coverage, temporal resolution, cost-efficiency, precision and accuracy (of sampled in situ data) (Ndou, 2023; Zainurin et al., 2022; Adjovu et al., 2023). The availability of multispectral satellite sensors with high temporal and spatial resolution presents an opportunity to monitor water quality regularly and over large geographic areas using spectral sensors onboard satellites (Pizani et al., 2020; Dube et al., 2015). Before the advancements in remote sensing, various studies (Park and Latrubesse, 2014; Shi et al., 2018; Xiong et al., 2019) have used lower-resolution satellite sensors such as the Moderate Resolution Imaging Spectroradiometer (MODIS) multi-spectral instrument to monitor the larger water bodies such as lakes. For instance, MODIS, with its spatial resolution of 250m - 500 m–1,000 m and temporal resolution of 1–2 days has been used by Shi et al. (2018) who combined long-term MODIS-Aqua satellite data and in situ measurements to track changes in water clarity (Secchi disk depth) in Lake Taihu, a shallow, turbid lake, from 1993 to 2015. The findings revealed a decline in water quality linked to increased cyanobacterial blooms driven by eutrophication and decreasing wind speeds. This study demonstrates that low-resolution satellite sensors such as MODIS have had success in regular water quality monitoring especially because of their higher temporal resolutions, however, they cannot provide enough detail to accurately monitor the quality of small inland surface waters due to limitations in spatial resolution (Olmanson et al., 2011).
High-resolution multispectral satellite sensors, such as Sentinel-2, address these limitations by providing finer spatial detail and higher temporal resolution, enabling more precise and regular water quality monitoring (Yang et al., 2022). The Sentinel-2 MSI satellite constellation has a spatial resolution of 10 m–20 m - 60 m, and a temporal resolution of 5 days for each of the 2 sensors. It has been used by Kowe et al. (2023) for monitoring spatial and temporal variations in water quality parameters, such as total nitrogen, turbidity, chlorophyll-a, and total suspended solids, in Lake Manyame, Zimbabwe, from 2017 to 2022. The study revealed Sentinel-2 effectiveness in water quality monitoring by developing empirical models with regression coefficients that reached R2 = 0.63 and R2 = 0.95 for water quality parameter estimation. This study showed that freely available high-resolution remote sensing data can be used for water quality monitoring, especially in resource-constrained areas, offering critical insights for resource management and decision-making. Guo et al. (2023) notes that small water bodies are often ignored in water resource assessments yet they play a critical role in the local ecological environments. Thus the Sentinel-2 MSI with its high spatial and temporal resolutions provides an opportunity to fill this gap and provide a comprehensive understanding of their water quality parameter spatial distribution in the water.
Multispectral sensors such as Landsat-8 Operational Land Imager (OLI) and Sentinel-2 Multispectral Instrument (MSI) collect data in multiple, distinct spectral bands within the electromagnetic spectrum, typically covering the visible and near-infrared region. Data from these satellite sensors facilitates large-scale and consistent water quality monitoring over regular intervals (Li et al., 2022a). Their distinct spectral bands assist in capturing various water quality parameters but these sensors have shown strong potential in monitoring optically active parameters like chlorophyll-a, Secchi disk depth, and turbidity due to their sensitivity to changes in water reflectance characteristics, enabling reliable estimation of these parameters across large spatial scales (Zhang et al., 2022a). For instance, Pizani et al. (2020) assessed a hydroelectric reservoir in Brazil using the Landsat-8 OLI and Sentinel-2 MSI to develop regression models for water quality parameters. The models for optically active parameters showed strong performance, with R2 values exceeding 0.75 for both sensors, demonstrating their ability to complement in situ measurements and reduce operational costs for water agencies. These findings highlight the practicality of using freely available remote sensing data to produce spatially distributed maps of water quality parameters. However, despite the technological advancements in remote sensing, significant gaps remain in its application to water quality monitoring.
Most studies still focus on optically active water quality parameters, while the potential of remote sensing for non-optically active parameters, such as nutrients and dissolved oxygen, is still underexplored (Gao et al., 2024). Moreover, Bangira et al. (2024) indicate that chlorophyll-a and total suspended solids are the most studied optically active water quality parameters in Africa, as studying non-optically active water quality parameters often incurs high costs due to the need for in situ sample collection to validate models for parameters lacking direct spectral signatures. Non-optically active parameters lack clear optical signals, making them difficult to detect directly through remote sensing (Yang et al., 2022). High-resolution multispectral sensors such as the Sentinel-2 MSI have the potential to address this gap by integrating empirical and machine-learning retrieval methods (Yang et al., 2022). For instance, using an empirical regression model, Al-Shaibah et al. (2021) demonstrated that certain sensors from the Landsat series are highly sensitive to non-optically active water quality parameters such as V-phenol, dissolved oxygen (DO), ammonium nitrogen (NH4-N), and nitrate nitrogen (NO3-N) in Erlong Lake, China. Their study achieved good accuracy, with the coefficient of determination R2 reaching up to 0.95 and the Mean Square Error (RMSE) ranging from 1.390 to 0.050 during validation. Meanwhile, Sent et al. (2021) showed that Sentinel-2 data and a multiple linear regression model can be used to indirectly quantify non-optically active water quality parameters in Brazilian lakes with an R2 of 0.85 and 0.83 (MSI_30, MSI_90) for DO and an R2 of 0.89 and 0.79 (MSI_30, MSI_90) for pH.
On the other hand, machine learning algorithms such as Neural Networks, Support Vector Machines, or Random Forests are used to learn complex relationships between input spectral variables and in situ water quality parameter measurements from water bodies (Leggesse et al., 2023). These models have been effectively applied in numerous studies (Guo et al., 2021; Kupssinskü et al., 2020; Mpakairi et al., 2024; Saberioon et al., 2020) for water quality monitoring and retrieval, demonstrating satisfactory results, especially in complex and variable water systems (Zhao et al., 2022). For instance, Guo et al. (2021) attempted to address the challenge of monitoring non-optically active water-quality parameters (total phosphorus, total nitrogen, and chemical oxygen demand) in small urban waterbodies using Sentinel-2 data and machine learning models. By evaluating 255 Sentinel-2 band combinations, they identified optimal bands for parameter retrieval and the models achieved high predictive accuracy (R2 of 0.94, 0.88, and 0.86 for neural networks, random forest, and support vector machine models, respectively). By leveraging machine learning models, the study demonstrates how complex relationships between spectral variables and in situ measurements of non-optically active water-quality parameters (total phosphorus, total nitrogen, and chemical oxygen demand) can be effectively predicted. While advancements in high-resolution multispectral sensors and machine learning models have significantly enhanced water quality monitoring, challenges persist in applying these technologies effectively, particularly in resource-constrained regions like Africa (Bangira et al., 2024).
Remote sensing, combined with machine learning, has demonstrated a potential to address these challenges by enabling the monitoring of water quality parameters with greater spatial and temporal accuracy. However, there remain gaps in the application of these technologies to smaller inland water bodies and non-optically active water quality parameters, which are crucial to local ecological systems but often overlooked in water resource assessments. Thus, this paper provides a comprehensive systematic review of the literature to derive progress in remote sensing of water quality, expose areas requiring further research, and identify opportunities presented by Sentinel-2 sensors for inland water bodies. The specific objectives were to (1) perform a brief bibliometric analysis to identify the trends in publications and distribution of remote sensing-based water quality monitoring studies; (2) review and categorise the types of satellite sensors used in assessing the water quality of inland water bodies, focusing on their advantages, limitations, and technological advancements in assessing optically and non-optically active water quality parameters; (3) review and analyse how machine learning algorithms have been used with remotely-sensed data in water quality parameters and (4) identify existing gaps and trends in current literature and propose areas for future research to enhance remote sensing-based water quality monitoring in small inland water bodies in South Africa.
This review followed the guidelines of the Preferred Reporting Items for Systematic Reviews and Meta-Analyses (PRISMA) framework to identify and extract peer-reviewed articles focused on water quality monitoring through remote sensing. The PRISMA Statement outlines a set of evidence-based items to enhance the quality of reporting in systematic reviews and meta-analyses (Page et al., 2021). Its purpose is to assist authors in enhancing the quality of reporting in systematic reviews and meta-analyses. The literature reviewed in this study, therefore, uses the PRISMA 2020 statement (version 1), which now includes databases and preprint registers. To search for relevant literature, the study used scholarly databases such as SCOPUS and Web of Science. Literature from these databases was accessed based on the following keywords: “Water quality,” “Water pollution,” “Water quality parameters,” “Remote sensing,” “Inland water bodies,” “Machine learning,” “vegetation indices,” “red-edge,” “Sentinel-2,” and “Landsat” (Table 1).
Peer-reviewed international journals with an emphasis on geography, hydrology, ecology, remote sensing, and water resources were some of the publications that were sought for their contributions. To add relevant literature, the search on the above-mentioned databases covered the years 2002–2024. Initially, the literature searches from Scopus and Web of Science retrieved 1695, 281 and 5,702 articles, respectively (Table 1). The retrieved articles underwent further screening as filters were added to further refine the results. This included filtering by the subject area, document type, publishing stage, source type and language, the years of publications, and topic of focus (Figure 1). Full-length articles of the selected abstracts were then obtained and analysed based on their relevance to the study’s objectives and methodology. Subsequently, 142 articles were retained.
Results of publication trends show that there were relatively fewer studies conducted between 2002 and 2015. In 2016 and 2017, studies started to increase. As observed in Figure 2, between 2018 and 2024, there was a steady increase in research on water quality monitoring, as compared to the previous years. Approximately 50 studies focused on lakes, looking at the years 2005, 2008–2009, 2011, 2013, 2015, and 2018–2023. Studies on rivers, particularly large rivers, were conducted between 2013, 2014, 2016, 2017, 2019, 2021, 2022, and 2024, making large rivers the second most studied water body. Some studies also focused on large dams, particularly during 2015, 2017, 2018, 2022, 2023, and 2024. Additionally, a portion of the reviewed studies were systematic and general reviews on various inland water bodies and some ocean waters but ocean waters were not the primary focus. Regarding satellite sensors, the Landsat series was mainly used between 2011 and 2024, with the Landsat-8 OLI being the most utilised sensor.
The Sentinel-2 MSI, known for its high spatial and temporal resolutions, was the second most used sensor, particularly between 2019 and 2024. The MODIS sensor was mainly used before the launch of Landsat-8 OLI and Sentinel-2 MSI, and it continued to be used as recently as 2022. The MERIS sensor was also utilised for water quality monitoring between 2005 and 2023. The SeaWiFS sensor, mainly suited for larger water bodies like oceans, was used in 2002 and 2010. The Sentinel-3 OLCI sensor was employed in studies from 2020, 2022, and 2023.
The table (Figure 3) highlights trends in the distribution of articles across different types of journals. Remote sensing journals take the lead, with a significant number of articles (30), showing just how important spatial technologies are in environmental research. “Remote Sensing of Environment” follows with 21 articles, and “Sensors” follows with 7, both showing the growing role of advanced sensing tools in this field. Water-related journals also have a strong presence. The journal “Water Quality” leads this category with 14 articles, while “Water” has 10, and “Environmental Monitoring and Assessment” contributes 6. These numbers highlight the increasing focus on monitoring and managing water resources. “Water Resources Research” also makes a notable contribution with 9 articles. There’s also a clear move toward interdisciplinary research. Journals like “Science of the Total Environment” (2 articles) and “International Journal of Applied Earth Observation and Geoinformation” (7 articles) show how diverse fields are coming together to address environmental issues. Even niche journals, like “Agriculture (Switzerland)” and “Frontiers in Sustainable Cities,” make an appearance, which suggests that interest in areas like sustainability, agriculture, and urban environments is expanding.
Engineering and technical journals, such as “Alexandria Engineering Journal” and “International Journal of Environmental Impacts,” round out the list. Their inclusion shows a growing emphasis on practical, hands-on solutions to environmental problems. Overall, the trends point to a strong reliance on geospatial technologies (satellite remote sensing), a growing focus on water science, and an increasing recognition of the need for interdisciplinary approaches and practical engineering solutions to tackle complex environmental challenges.
The spatial distribution of studies on water quality monitoring using remote sensing, as shown in the choropleth map (Figure 4), reveals significant global geographic trends in research. Studies were conducted across six continents, excluding Antarctica. In Asia, countries such as India, Malaysia, Bangladesh, and Bhutan were included, with China being the most studied country for monitoring inland water bodies, particularly lakes. In Europe, countries such as Italy, Germany, and Portugal focused primarily on monitoring lakes and rivers for water quality. In Africa, South Africa led studies on dams using the Landsat series and Sentinel-2 MSI, followed by Kenya, Rwanda, and Morocco. North America saw the United States leading research on inland water bodies, with Canada following, while in South America, Brazil and Argentina were prominent. Finally, in Oceania, New Zealand was the primary country engaged in water quality monitoring studies. Overall, the map highlights a need for a more balanced global research landscape, with increased studies on water quality monitoring and remote sensing in currently underrepresented countries.
The development and trajectory of research in water quality monitoring based on primary terms extracted from published paper titles, abstracts, and keywords are analysed in this review (Figure 5). The network map categorises the identified literature into 4 clusters of concepts. The first cluster, represented in red, focuses on keywords related to “water quality,” “remote sensing,” “Landsat,” “Sentinel,” and “optical properties,” among others. This cluster highlights research endeavours in remote sensing applications for water quality monitoring and also highlights connections between these primary keywords and other clusters. The red cluster also features keywords such as “land use,” “pollution,” and “water management,” highlighting investigations into the factors contributing to water quality deterioration and the locations targeted for monitoring efforts. The second cluster, represented in yellow, showcases terms such as “machine learning,” “regression analysis,” and “neural networks.” This cluster indicates the integration of machine learning techniques into water quality monitoring studies, with links to the primary red cluster focusing on how research on water quality has been done using remote sensing and machine learning.
Figure 5. Network map showcasing concepts in water quality monitoring and remote sensing studies derived using data from titles, abstracts, and keywords.
The third cluster, represented in green, focuses on terms such as “lakes”, “China,” and “surface water,” showing that there has been research mostly done on water quality monitoring in China, and the focus has been on lakes. The fourth cluster, represented in blue, focuses on terms such as “chlorophyll-a,” “algae”, “inland”, “reservoirs,” and “sentinel,” emphasising the research focus on chlorophyll-a analysis in water quality monitoring on lakes and reservoirs using remote sensing satellite sensors such as Sentinel-2. These clusters also exhibit connections with other clusters in the network. In summary, the network map offers valuable insights into the trends and connections within water quality monitoring research, highlighting the various approaches and main areas of scholarly interest in this field.
Identifying the main sources and impacts of water quality degradation is fundamental to efficient and effective water quality management (Parris, 2011). Anthropogenic activities such as urbanisation, agricultural practices, industrialisation, and population growth have been identified as significant contributors to the deterioration of water quality worldwide (Gupta and Singh, 2016). For example, industrial activities, including those in the paper, iron and steel, distillery, and nuclear industries, release toxic chemicals and organic and inorganic substances into the environment, leading to increased wastewater production (Gupta and Singh, 2016). Similarly, agricultural activities contribute to water contamination through the use of pesticides, nitrogen fertilisers, and organic farm wastes, which introduce nitrates, phosphorus, pesticides, and soil sediments into water bodies (Parris, 2011; Lin et al., 2022b). Excessive nitrates and phosphorus (nutrient enrichment) in water bodies thus cause eutrophication which Wilkinson and Johnson (2024) describe as the process of enriching aquatic habitats with nutrients, primarily nitrogen and phosphorus, leading to increased rates of organic matter causing harmful algal blooms (HABs) that affect water quality.
This process is a growing concern in the African continent, and in South Africa, the Hartebeespoort Dam serves as a notable example of a reservoir affected by chemical parameters such as nitrogen and phosphorus which cause eutrophication in the water. The dam supplies water for irrigation, industry, residential use, tourism, recreation, and municipal purposes downstream along the Crocodile River (West) (Hobbs, 2017). However, the dam is also impacted by acid mine drainage and bacterially contaminated municipal wastewater effluent, flowing through the Cradle of Humankind World Heritage Site’s karst system (Hobbs, 2017). Matthews and Bernard (2015) highlight eutrophication as a significant issue in South African water bodies, given the country’s limited freshwater resources. This phenomenon, along with cyanobacterial blooms, poses economic burdens due to costs associated with water treatment, tourism losses, and negative human health impacts like cholera and diarrhoea. Eutrophication causes concern about the quality of inland water bodies and needs attention from authorities, scientists and the public (Oliphant et al., 2018).
In countries outside the African continent, Xu et al. (2022) studied the Yangtze and Yellow River Basins in China to identify the primary sources of pollution and their effects on water quality. Their findings revealed that while agricultural activities contribute to water pollution and quality degradation, the impact of industry on river health outweighs that of agriculture. Moreover, Lin et al. (2022) also reported that around 80% of industrial and municipal wastewater is released into the environment, including rivers, without treatment, with higher discharge rates in developing countries. Edokpayi et al. (2017) highlighted poor wastewater infrastructure, design flaws, lack of expertise, and corruption as key issues leading to the failure of wastewater treatment facilities in developing nations. This results in untreated wastewater entering surface water, causing water quality deterioration. Globally, the issue of water pollution continues to raise concern as it affects the environment, public health, and economies. Therefore, monitoring water quality is essential to protect ecosystems, sustain economies, and ensuring access to clean, safe drinking water (Zainurin et al., 2023).
Water quality monitoring focuses on the biological, chemical, and physical parameters of water. These water quality parameters are used to evaluate pollutant levels and determine whether the water is suitable for human use and maintaining ecosystem health (Table 2) (Ahmed et al., 2020; Wen et al., 2020).
Water samples are usually collected from designated stations and water quality monitoring is then completed through traditional methods such as laboratory and in situ tests (Zainurin et al., 2022). In-situ testing involves using sensors that are designed to assess parameters such as pH, dissolved oxygen, conductivity, turbidity, and nutrient concentrations (Osman et al., 2018). These sensors can be used individually or as part of a multisensor monitoring system. This method can prevent artefacts from occurring due to data being collected directly from the water body, leading to an understanding of the conditions and interactions within that environment in real time (Liber et al., 2016). For instance, a study by Mujathel et al. (2022) aimed to evaluate trihalomethane (TTHM) levels of chlorinated drinking water sources in the Aseer region of Saudi Arabia, over four seasons (Summer, Autumn, Winter, and Spring). One of the parameters associated with increasing TTHM was turbidity and the study utilised a turbid meter (HACH 2100 N) to do in situ measurements of turbidity. The in situ results for surface water revealed that the mean turbidity for raw surface water (4.54 NTU) is higher than the mean turbidity for treated surface water (1.458 NTU). This finding revealed that raw surface water with high turbidity contributed to increased TTHM levels.
Another study by Bai et al. (2023) investigated how different ways of controlling river flow affect the movement of dissolved oxygen matter (DOM) in the Yongding River Basin of China. Parameters such as dissolved oxygen, electrical conductivity (EC), temperature, and pH were measured in situ after calibration and stabilisation for 2 min, using the HQ40d, Hach, multiparameter system. The results and values of the parameters were recorded in situ and helped determine the seasonal concentration and patterns of DOM in the river. Randhawa et al. (2016) also used a multiparametric sensor probe for real-time monitoring of water pollution on the Yamuna River in New Delhi, India. The study assessed physio-chemical parameters such as pH, electrical conductivity, dissolved oxygen and turbidity with records of sensor data (e.g., dissolved oxygen recorded as a low 3 mg/L), assisting in creating heatmaps, real-time and on-site monitoring and identification of pollution hotspots. Kalaitzidou et al. (2023) have also used instruments such as the WTW 3310 instrument to measure pH and EC, a Perkin Elmer Analyst 800 Atomic Absorption Spectrophotometer to measure parameters such as Nitrate, Ammonium and Sodium in situ.
These studies (Randhawa et al., 2016; Mujathel et al., 2022; Bai et al., 2023; Kalaitzidou et al., 2023) show how in situ measurements can prevent artefacts from occurring during data capturing in traditional methods as compared to the sampling, transporting, and storing contaminated water and sediment for toxicity testing that would be done in a lab (Liber et al., 2016). However, in situ monitoring can have challenges with reliability of sensors, factors that can ruin their accuracy, environmental conditions (high temperatures, low oxygen concentrations) and being expensive if it involves calibration, upkeep, and periodic replacement of the sensors (Lal et al., 2023). Mujathel et al. (2022), showcased ways of avoiding some of these challenges in their study of evaluating TTHM levels of chlorinated drinking water sources in the Aseer region, where they used a turbid meter (HACH 2100 N) to do in situ measurements of turbidity (as one of the parameters). Water samples were collected and measured for turbidity on-site and the cell of the meter had to be cleaned and dried to prevent false results and ruining accuracy.
Demetillo et al. (2019) also tried to avoid these challenges in their study of developing a low-cost, real-time water quality monitoring system (commercial electrochemical sensors from Atlas Scientific) to monitor temperature, dissolved oxygen and pH in a large aquatic area. For example, the pH sensor in the system was tested and calibrated using a Horiba multi-parameter tester and results showed a 0.23 difference in value readings between the pH sensor and the multi-parameter tester, proving that regular calibration can minimise inaccuracies. The laboratory testing approach has been done through the collection of samples on water bodies, followed by transportation of the samples to a laboratory for chemical and biological analysis (Coraggio et al., 2022). For instance, Barakat et al. (2016) conducted a study to assess water contamination in the Oum Er Rbia River basin in Morocco, focusing on identifying sources and effects on water quality. Samples were collected at different stations and various parameters such as temperature, pH, turbidity, and dissolved oxygen were measured both in situ and in the laboratory.
Additionally, parameters such as total suspended solids, ammonia, nitrate, total phosphorus, and faecal coliforms were analysed in the laboratory. Various analytical methods, including spectrophotometry and pH probes were employed and the findings, analysed using multivariate statistical methods, revealed that water quality at some stations exceeded Moroccan standards, posing potential health risks to consumers. Another study by Zhang et al. (2022a) collected samples and did laboratory testing to calculate the Water Quality Index (WQI), a mathematical tool used to assess and calculate the overall quality of water in a particular water body using a mathematical equation (Equation 1) (Rocha et al., 2015).
Zhang et al. (2022a) used both traditional methods and the Water Quality Index (WQI) to assess water quality in the Nanxi River within the Taihu Watershed, China. The study collected and did a laboratory analysis on 22 water samples from 14 monitoring stations between 2015 and 2020. Results indicated a trend of moderate to low water quality, with certain stations consistently exhibiting good to excellent conditions. Cluster analysis revealed spatial variations in contamination levels, assisting in the identification of pollution sources and the formulation of targeted management strategies. These studies show how in situ and laboratory testing methods have been effective in measuring water quality (Zainurin et al., 2022). They can, however, be time-consuming and costly if there is a need to provide short-term data and they can be affected by weather or other environmental impacts (Coraggio et al., 2022). Additionally, they are criticised for being point-based and limited in temporal and spatial scales (Ndou, 2023). Additionally, water sampling and laboratory testing face limitations, particularly regarding the large number of samples needed for analysis. There are often logistical difficulties in managing and transporting the hundreds of litres of water samples required for laboratory testing to measure various parameters (Altenburger et al., 2019).
Some studies (Barakat et al., 2016; Wu et al., 2018; Zhang et al., 2022b; Kalaitzidou et al., 2023) have reported that water quality needs to be monitored regularly on seasonal periodical variations (i.e., labour will be needed to return to the water bodies and collect samples on a regular basis) for detecting spatiotemporal variations and this may cause issues in relation to the methods being labour-intensive, and time-consuming (Ndou, 2023; Zainurin et al., 2022). To minimise these challenges, the integration of multiple measurement techniques, including laboratory analysis and remote sensing, can help provide a more comprehensive understanding of water quality dynamics (Harmel et al., 2023).
The introduction of satellite remote sensing in water quality monitoring has revolutionised the field by providing a systematic and synoptic perspective on water quality parameters over large water bodies, enabling efficient, cost-effective, and near real-time assessments (Swain and Sahoo, 2017). This technology enables the study of physical and chemical water quality parameters over space and time using remotely sensed data. It is a combination of technology and science to obtain information about an object, area, or phenomenon through the analysis of spectral data collected by technological devices (Jafarbiglu and Pourreza, 2022). These devices collect data by measuring the transmission of electromagnetic energy from surfaces that reflect or emit radiation (Sivakumar et al., 2003). Various surface features reflect various amounts of energy across the electromagnetic spectrum based on their structural, chemical, and biological properties, as well as factors such as surface roughness, angle of incidence, intensity, and wavelength of radiation (Sivakumar et al., 2003). The application of remote sensing in water quality assessment is, thus, premised on the understanding of how the technology measures the physical, chemical, and biological characteristics of water bodies (Bresciani et al., 2019; Sent et al., 2021).
Moreover, it helps to establish the possible sources of water quality contamination with a catchment-level assessment of water quality (Usali and Hasmadi Ismail, 2010). Contamination sources include municipal waste discharges, agricultural chemicals, heavy metals, microorganisms, and sedimentation. These sources of contamination alter the chemical, physical and biological characteristics of water bodies, significantly changing their reflection characteristics and affecting the reflectance signal captured by remote sensing devices. Satellite sensors detect these changes in the spectral signature of reflected light and relate these changes to water quality parameters using empirical or analytical models (Murray et al., 2022). In the visible range of the electromagnetic spectrum, water generally exhibits low reflectivity across most wavelengths, including the blue and green spectral bands and absorbs light in the near-infrared range, further enabling the differentiation of water bodies from other objects in images (Sultana and Dewan, 2021). In contrast, contaminated water has exaggerated concentrations of chemical, physical or biological properties that are detectable using remote sensing by analysing its spectral characteristics and reflectivity (Adjovu et al., 2023).
The contaminants in the water can change how water interacts with light as they display unique spectral characteristics in the visible (400 nm–700 nm) and near-infrared (700 nm–2500 nm) regions of the electromagnetic spectrum (Sultana and Dewan, 2021). As a result, significant advancements have been made in remotely sensing water quality, particularly focusing on optically active and non-optically active water quality parameters. Optically active water quality parameters interact with light, and therefore are detectable in the visible and near-infrared spectral regions (Zhang et al., 2022c). Examples of such parameters are chlorophyll-a, total suspended solids, Secchi Disk Depth, Water temperature, and turbidity. Non-optically active parameters lack clear optical signals, making them difficult to detect directly through remote sensing (Yang et al., 2022). Instead, these parameters have been indirectly estimated using optically active components like chlorophyll-a, total suspended matter, and coloured dissolved organic matter. Examples of non-optically active water quality parameters include electrical conductivity, temperature, pH, dissolved oxygen, nitrogen and phosphorus (among others) (Wang and Yang, 2019). To monitor these parameters, various satellite sensors have been developed and used together with field measurements, empirical, analytical, semi-empirical, and machine learning retrieval methods.
Small inland water bodies, such as rivers, lakes, and reservoirs, play a critical role in local ecosystems by supporting flood control, irrigation, and climate regulation (Sun et al., 2024). These water bodies are often referred to as “sentinels of environmental change,” highlighting the importance of long-term water quality monitoring to track the health of water (Liu et al., 2024b). Traditional methods like sampling, laboratory analysis, and in situ measurements are accurate but time-consuming, costly, point-based, and limited in spatial and temporal coverage (Adjovu et al., 2023). This creates significant challenges for effectively monitoring small, remote, or diverse inland water bodies. Remote sensing has emerged as a cost-effective alternative for large-scale water quality monitoring. For example, Chebud et al. (2012) developed a remote sensing-based approach using Landsat spectral data and a neural network model to predict water quality parameters (chlorophyll-a, turbidity, and phosphorus) in the Kissimmee River basin in South Florida. This approach proved to be highly accurate (R2 > 0.95) between 1998–1999 and 2009–2010 across both wet and dry seasons. The cost-effectiveness of this remote sensing-based approach is largely due to the availability of freely accessible Landsat data, which reduces the need for costly site visits involved in traditional water quality monitoring methods (Chen et al., 2024).
Remote sensing also offers advantages in high spatial and temporal coverage. Satellite sensors with high spatial resolution allow for detailed monitoring of smaller water bodies, capturing fine-scale changes that might not be noticeable (Sigopi et al., 2024). Combined with high temporal resolution, remote sensing enables the detection of dynamic changes and pollution events in near real-time, even in remote or inaccessible locations. For instance, the Geostationary Ocean Colour Imager (GOCI), the world’s first ocean colour satellite sensor, offers a moderate spatial resolution (500 m) and a very high temporal resolution (1 h, eight times a day) for near real-time monitoring of marine environments in northeast Asia (Ryu et al., 2012). On the other hand, the MODIS sensor, which offers a temporal resolution of 1–2 days and spatial resolutions ranging from 250 m to 1000m, is well-suited for monitoring ocean waters and larger inland water bodies and this sensor is one of the most widely used satellite sensors (Shi et al., 2018; Xiong et al., 2019). Recent sensors with even higher spatial and temporal resolutions, like the Sentinel-2 sensor (10 m–20 m - 60 m, spatial resolution and 5 days temporal resolution), have further enhanced the use of remote sensing in monitoring smaller inland water bodies (Caballero et al., 2018). Sentinel-2’s 10 m spatial resolution offers an opportunity for detailed mapping and analysis of smaller inland water bodies, which may be difficult to analyse with coarser-resolution sensors such as MODIS (Li et al., 2022b).
Furthermore, Sentinel-2’s 5-day revisit time can assist with frequent water quality monitoring, making it possible to capture short-term variations and seasonal trends in waterbodies more effectively (Kowe et al., 2023). Satellite sensors such as Sentinel-2 are easily accessible, enabling more frequent, cost-efficient, and accurate monitoring of these smaller bodies (Sigopi et al., 2024). Remote sensing also allows for multi-parameter analysis of water bodies. The advancement of multispectral (and now hyperspectral) sensors has improved the prediction of water quality parameters, as satellite sensors can derive multiple parameters (e.g., turbidity, chlorophyll-a, total suspended solids) from spectral bands across the electromagnetic spectrum (Bangira et al., 2024). Multispectral sensors such as Landsat’s Operational Land Imager (OLI), Thermal Infrared Sensor (TIRS), Sentinel-2’s MultiSpectral Instrument (MSI), and MODIS have been used to derive various water quality parameters simultaneously (Bangira et al., 2024). This is an advantage over traditional methods, which are point-based and require handheld sensors and probes to measure individual parameters (Yang et al., 2022). For instance, Mamun et al. (2024) used Sentinel-2 MSI and Landsat-8 OLI with machine learning to monitor chlorophyll-a, Secchi depth, and total suspended solids in a reservoir.
Key predictor band ratios for chlorophyll-a and total suspended solids included the red/red-edge1 × red-edge2 for Sentinel-2 MSI and the red/blue ratio for Landsat-8 OLI, while Secchi depth was predicted using the red/blue band ratio (Sentinel-2 MSI) and the green band (Landsat-8 OLI). These results demonstrate how specific spectral band combinations can accurately estimate water quality parameters, demonstrating the cost-effectiveness and reduced labour intensity of remote sensing. These results further highlight how the presence of Sentinel-2 red-edge bands (Bands 5, 6, and 7) can enhance the detection of chlorophyll-a, a key indicator of eutrophication (Pizani et al., 2020). Moreover, remote sensing with the Sentinel-2 sensor provides and advantage of suspended sediments and turbidity prediction improvement with its shortwave infrared bands (Bands 11 and 12) (Ndou, 2023). Remote sensing offers significant advantages in monitoring inland water bodies, yet several challenges remain in applying it effectively, particularly for small inland water bodies. One of the most widely studied challenges is related to the resolution of remotely sensed data, which impacts the ability to retrieve both optically and non-optically active water quality parameters (Yang et al., 2022). Spatial resolution constraints of satellite sensors complicate accurate monitoring. For instance, low spatial resolution sensors such as MODIS, MERIS, and SeaWiFS are suitable for monitoring large water bodies like oceans and large lakes but face difficulties in detecting water quality parameters in smaller inland water bodies like small lakes or narrow rivers (Li et al., 2023).
These sensors often fall below the pixel size required for detailed observation of such features, as noted by Frasson et al. (2024). High-resolution sensors such as the Sentinel-2 MSI improve on these limitations with their high spatial resolution, yet they still encounter challenges (Abdelal et al., 2022). According to Bangira et al. (2024), wider spectral bands of multispectral sensors, including those of Sentinel-2, struggle with predicting water quality parameters in water bodies with heterogeneous ecosystems, such as those that contain both green and blue-green algae with comparable spectral profiles. Goyens et al. (2022) note that sensors with narrower spectral bands or hyperspectral sensors (which have much finer spectral resolution) may perform better in identifying and analysing specific water quality parameters in inland water bodies with complex and mixed compositions. Additionally, many existing optical sensors face difficulty in estimating crucial non-optically active water quality parameters like dissolved oxygen, nitrogen, and phosphorus, due to their weak optical characteristics and low signal-to-noise ratios, which complicates measurements (Gholizadeh et al., 2016). To address this challenge, researchers have explored machine learning algorithms as a solution for estimating non-optically active parameters.
For instance, Li et al. (2022b) employed random forest and artificial neural networks to predict water quality parameters such as total nitrogen and phosphorus in large inland rivers, achieving better accuracy (R2 values ranging from 0.44 to 0.67). This approach improves the accuracy of remote sensing models, offering a promising solution for monitoring water quality parameters that cannot be directly measured with optical sensors. Further complicating the use of remote sensing are atmospheric correction errors, which can impact the accuracy of water quality estimations (Jaywant and Arif, 2024). To mitigate this, studies have compared atmospheric correction algorithms like Acolite, C2RCC, FLAASH, iCOR, and L2gen, with varying degrees of effectiveness depending on the band and sensor (Wang et al., 2024). Moreover, Liu et al. (2024a) mentions that finding a suitable algorithm for specific inland water bodies or study areas is essential for reliable satellite-based monitoring of water and environmental conditions due to this varied accuracy and effectiveness of atmospheric correction algorithms. The need for accurate calibration and validation with in situ data is also crucial for ensuring reliable results especially when dealing with the limitations of passive sensors, which rely on sunlight and may be hindered by cloudy conditions (Jaywant and Arif, 2024).
In such cases, integrating in situ measurement networks, Synthetic Aperture Radar (SAR) data, and developing special tools like the WQEI index can enhance model accuracy even in challenging weather conditions (Kumar et al., 2023). While remote sensing continues to show promise for monitoring water quality, especially in smaller and remote inland water bodies, further advancements in sensor technology, data resolution, and algorithm development are necessary to address existing challenges and fully realise the potential of this approach.
Significant advancements have been achieved in assessing the water quality of coastal and inland waters using low-resolution remote sensing sensors that cover larger areas of water bodies and use thermal/optical sensors (Swain and Sahoo, 2017). Ocean colour satellite sensors such as the Moderate-resolution Imaging Spectroradiometer (MODIS), Sea-viewing Wide Field-of-view Sensor (SeaWiFS) and Medium Resolution Imaging Spectrometer (MERIS) have been used for this (McClain et al., 2014). The MODIS sensor, launched on the Terra and Aqua satellites in 1999 and 2002, respectively, provides near-daily coverage with 1–2 days temporal resolution, spatial resolution from 250 m to 1 km, and 36 spectral bands (Olmanson et al., 2011). MODIS data is openly accessible and has proven suitable for monitoring various water bodies, including coastal areas, large lakes, and rivers (Gholizadeh et al., 2016; Chavula et al., 2009; Liu et al., 2022; Abbas et al., 2019). Similarly, the SeaWiFS sensor, launched in 1997, has a 1 km spatial resolution, 8 spectral bands covering visible and near-infrared wavelengths, and a 1–2 days temporal resolution. Although primarily designed for ocean monitoring, it has also been used to monitor water quality in rivers, lakes, and reservoirs (Gholizadeh et al., 2016; Groom et al., 2019).
The MERIS sensor, aboard the Envisat satellite launched in 2002, had 15 spectral bands covering visible and near-infrared wavelengths, a 300 m spatial resolution, and a 3-day repeat cycle, allowing frequent earth observations (Arabi et al., 2020). The sensor was suited for ocean colour monitoring and assessing water quality parameters like chlorophyll-a concentration and suspended sediment load in large lakes and coastal areas (Arabi et al., 2020). Studies (Attila et al., 2018; Odermatt et al., 2008; Reinart, 2005) have used these low resolution sensors to monitor inland water bodies such as lakes. For instance, (Reinart, 2005), compared MERIS data with MODIS and SeaWiFS data to evaluate its efficiency in monitoring the water quality of the European Union’s three largest lakes. Their study found MERIS to be more suitable for estimating water quality parameters, particularly chlorophyll-a concentrations, in larger inland water bodies area >0
SeaWiFS, MODIS, and MERIS sensors can capture data frequently, allowing timely monitoring and analysis of water body changes (Topp et al., 2020). However, these sensors may not provide detailed images in their data for small inland water bodies (area <0.1
The Landsat-9 carries the OLI-2 and the Thermal Infrared Sensor 2 (TIRS-2) launched on the 27th of September 2021 (Lulla et al., 2021). The OLI-2 carries 9 spectral bands and the TIRS has 2 spectral bands, making the Landsat-9 have 11 spectral bands (Lulla et al., 2021). The OLI-2 has spatial resolutions of 15 m (band 8) – 30 m (band 1–7 and band 9) and the TIRS has spatial resolution of 100 m (band 10–11). Niroumand-Jadidi et al. (2022) compared the Landsat-9 OLI 2 to the Sentinel-2 MSI using physics-based and NN-based machine learning algorithms. The study found that Landsat-9 OLI-2 outperformed the Sentinel-2 MSI in retrieving higher accuracies due to its higher signal-to-noise (SNR) and 14-bit radiometric resolution, which is more sensitive to water-leaving reflectance. The Sentinel-3 satellite constellation is another land surface mission, launched in 2016 and 2018 and consists of two satellites, Sentinel-3A and Sentinel-3B. The Ocean and Land Colour Instrument (OLCI) on board these satellites provides 21 band images with high spectral resolution for ocean, land, and atmospheric monitoring (Soomets et al., 2020; Rodrigues et al., 2022). The OLCI can provide data at a temporal resolution of 3 days and a low-medium spatial resolution of 300 m.
A study by Soomets et al. (2020) comparing Sentinel-2’s MSI and Sentinel-3’s OLCI found optimal atmospheric correction led to the best results with the optical water-type guided approach. However, OLCI’s effectiveness was hindered by challenges with atmospheric correction, spatial resolution, and masking, resulting in lower accuracy compared to the MSI. The study highlights that higher spatial resolution sensors like Sentinel-2 MSI are better suited for detailed monitoring, especially for smaller inland water bodies. Multispectral sensors like Sentinel-2 MSI and Landsat-8 OLI offer a chance to regularly monitor water quality, even in smaller inland water bodies (Pizani et al., 2020). Both Landsat-8 OLI and Sentinel-2 MSI are also utilised for monitoring larger water bodies, with Landsat-8 OLI having a spatial resolution of 15 m–30 m and Sentinel-2 MSI offering resolutions of 10 m (4 bands), 20 m (6 bands), and 60 m (3 bands) (Pizani et al., 2020). The MSI on Sentinel-2 provides data across its 13 spectral bands, while Landsat-8 OLI gathers data through its 9 spectral bands (bands 10 and 11 dedicated to Thermal Infrared Sensor (TIRS) (Dube et al., 2015).
Additionally, the IOCCG (2018) highlights Landsat-8 OLI and Sentinel-2 MSI as two of the most important earth-observing sensors for monitoring inland waters, especially small inland lakes (Table 3). For example, the Sent et al. (2021) study on water quality parameters in the Sado Estuary of Portugal found that the Sentinel-2 MSI sensor could be more useful for smaller regions, such as estuaries, due to its ability to reach the smallest water bodies. The sensor offers advantages such as publicly available and well-documented data, facilitating long-term coverage, and its high temporal resolution, compared to Landsat-8 OLI, allowing for more frequent revisits (Sent et al., 2021; Dube et al., 2015). Additionally, its high spatial resolution captures detailed observations of water bodies and facilitates monitoring changes over time, aided by its multispectral capabilities with 13 spectral bands (Table 3). The sensor’s ability to capture the reflectance properties of water is driven by its multispectral capabilities (Soomets et al., 2020; Sent et al., 2021). Pizani et al. (2020) also assessed two satellite sensors’ performance in estimating water quality parameters in a Brazilian reservoir.
The study examined optically active and non-optically active parameters, including chlorophyll-a, Secchi disk depth, turbidity, dissolved oxygen, and temperature, and compared the Landsat-8 OLI with the Sentinel-2 MSI. Employing a multiple regression model, the findings revealed that the Sentinel-2 MSI sensor outperformed the Landsat-8 OLI due to its greater number of spectral bands. The sensor has red-edge, near-infrared, and shortwave infrared bands, along with the finer spectral resolution of bands 3–7 (560–783 nm) and 8a (865 nm), which showed enhanced reflection and retrieval of water quality parameters. Additionally, the sensor was also able to retrieve data related to dissolved oxygen and pH levels (non-optically active parameters) due to the higher concentrations of chlorophyll associated with the photosynthesis presence of algae reflected at bands 2–5 (490–705 nm).
Remote sensing of water quality has made significant progress, particularly in focusing on optically active parameters like chlorophyll-a, total suspended solids, Secchi Disk Depth, water temperature, and turbidity. Additionally, Bangira et al. (2024), mention that chlorophyll-a and total suspended solids are two of the most researched water quality parameters in Africa. However, non-optically active parameters like nitrogen, phosphorus, pH, temperature, and EC are not frequently mentioned in the literature, yet advancements in remote sensing technology and retrieval model techniques have enabled indirect and direct methods for estimating these parameters (Tesfaye, 2024).
The next sections look at the descriptions of some of these optically active and non-optically active water quality parameters and how they have been estimated using the various satellite sensors.
Optically active parameters in water, such as turbidity, total suspended solids (TSS), and chlorophyll-a, interact with light to alter the energy spectrum of reflected solar radiation (Adjovu et al., 2023). Retrieving these parameters requires specific sensors (Table 4) tailored to the task and suitable for the specific environmental conditions.
Out of all these parameters, chlorophyll-a is a commonly used parameter in the assessment of the trophic status of freshwater ecosystems (Bangira et al., 2024; Deng et al., 2019; Mpakairi et al., 2024). Chlorophyll-a, a green pigment found in organisms like land plants and algae, is influenced by factors like light intensity, penetration, turbidity, and nutrient levels. It absorbs light in blue and red wavelengths and reflects light in green wavelengths (Deng et al., 2019). For instance, Arora et al. (2022) focused on assessing optically active water quality parameters in the Punjab region of India across different seasons. The study found that chlorophyll-a concentrations strongly absorbed light in the blue (490 nm) and red (665 nm) wavelengths, while reflecting light in the green (560 nm) and near-infrared (842 nm) wavelengths. Moreover, using the Sentinel-2 MSI, the study estimated maximum, minimum, and average chlorophyll-a concentration accuracies in the Harike wetland area as 36.31, 0.01, and 29.53 mg/
Pizani et al. (2020) used Sentinel-2 (band 2, 3, 4 and 5) and Landsat-8 (band 2, 3 and 4) sensors to estimate chlorophyll-a in Tres Marias Reservoir, Brazil. The Sentinel-2B MSI sensor outperformed the Landsat-8 OLI sensor across all parameters due to its broader range of spectral bands (encompassing red-edge, near-infrared, and shortwave infrared), providing finer spectral resolution and improved water quality parameters reflection and retrieval. Similar studies have compared the Landsat series to Sentinel-2 MSI for chlorophyll-a estimation. For instance, Niroumand-Jadidi et al. (2022) compared Landsat-9 OLI-2 and Sentinel-2 MSI accuracy in estimating water quality parameters in four Italian lakes. Landsat-9 OLI-2 showed superior performance due to its higher signal-to-noise ratio and sensitivity to water-leaving reflectance. However, further research across diverse water types and more in situ data is needed to validate these findings. Sentinel-2 MSI’s coastal blue band with a higher signal-to-noise ratio and spatial resolution could potentially yield better results in estimating water quality parameters, especially in small water bodies.
Various studies (Ali et al., 2022; Munyai et al., 2022; Ndou, 2023; Obaid et al., 2021; Oliphant et al., 2018) in South Africa have assessed chlorophyll-a concentrations in various inland water bodies using high-resolution sensors like the Landsat series and Sentinel-2 MSI. However, it is important to consider seasonal changes that may affect chlorophyll-a concentration and acknowledge potential variations in accuracy among different sensors. For instance, a study by Obaid et al. (2021) employed Sentinel-2 and Landsat-8 OLI to assess water quality and estimate chlorophyll-a in South Africa’s Vaal Dam, finding an average summer concentration of 5.5 μg/L, which dropped to 4.9 μg/L in winter. This seasonal variation is attributed to increased river discharge during the rainy season, which subsequently decreases afterwards. The Sentinel-2 MSI’s red/near-infrared based model performed well, with an R2 of 0.75 and an RMSE of 0.48 μg/L. This is attributed to Sentinel-2 MSI’s higher spatial and spectral resolutions and the additional chlorophyll-a sensitive band in the near-infrared region, which the Landsat-8 OLI lacks. This is also further supported by Oliphant et al. (2018), who used Landsat-8 bands in a stepwise logistic model, revealing fluctuating trends in chlorophyll-a mapping accuracy over time. In April 2014, chlorophyll-a concentration was mapped with 76.8% accuracy, dropping to 35.01% in May 2015.
Such variations in accuracy across seasons and sensors justify the need for further investigation, especially in South African small inland water bodies. The availability of Sentinel-2 sensors with coastal and red-edge bands offers an opportunity to enhance chlorophyll-a estimation, particularly in winter seasons. This potential is also further supported by Mpakairi et al. (2024), who examined chlorophyll-a concentrations in South Africa’s Nandoni Reservoir using Landsat-8 OLI and Sentinel-2 MSI. The study highlighted that Sentinel-2 MSI’s additional red-edge spectral bands effectively captured subtle changes in chlorophyll-a concentrations due to weaker chlorophyll-a absorption, yielding higher reflectance values than other bands (Bramich et al., 2021). Chlorophyll-a is one of the most studied water quality parameters in South African water bodies and Landsat-8 and Sentinel-2 have been utilised for this purpose. However, this research (Bramich et al., 2021; Ali et al., 2022; Munyai et al., 2022) has been done on reservoirs or Dams. The Sentinel-2 MSI, especially with its red-edge band, offers a notable advancement in water quality estimation. Its high spatial resolutions of 10 m, 20 m (for the red-edge band), and 60 m can significantly enhance water quality monitoring and decision-making, particularly for managing small inland rivers in South Africa.
Water turbidity and total suspended solids are other water quality parameters that are frequently studied using various satellite sensors (Bangira et al., 2024). Water turbidity, indicating the degree of light absorption due to suspended particles, is closely linked to total suspended matter levels, with higher concentrations resulting in increased turbidity (Gholizadeh et al., 2016). Therefore, total suspended matter and turbidity are important variables in the remote sensing of water quality due to their interaction with incoming sunlight (Myint and Walker, 2002; Gholizadeh et al., 2016). According to Duan et al. (2024), suspended sediment in surface water exhibits seasonal variations due to climatic factors and reservoir management. The varying concentration of suspended sediments across seasons necessitates algorithms that are sensitive to these variations. In developing such an algorithm, an exploratory analysis of the utility of spectral reflectance from 600 nm to 800 nm may be useful in establishing an algorithm for estimating suspended sediments across seasons. To study suspended sediments across seasons, Sagan et al. (2020) mention that research has been done using Landsat, MODIS, and MERIS sensors on large rivers, lakes and reservoirs.
For instance, a study by Park and Latrubesse (2014) assessed the large Amazon River in South America using MODIS data to estimate regional and seasonal variability of surface sediment concentrations (as one of its aims) with its 250 m–500 m - 1 km spatial resolution and its 1–2 days temporal resolution. Band 1 (620–670 nm) of the MODIS satellite sensor demonstrated a very high and consistent sensitivity to the suspended sediment concentrations in the water, making it well-suited for identifying the spatiotemporal patterns of surface sediment distribution in large rivers. Data from the sensor was then used to develop empirical models to relate field surface sediment concentration to surface reflectance data from MODIS. The results revealed high R2 values (0.79–0.92) and significant slopes at a 99% confidence level, proving that the MODIS-Based model effectively captured the spatial and temporal variability of surface sediments in the large Amazon River Basin. Other studies have also focused on assessing spatiotemporal variability in turbidity and total suspended solids, although they primarily utilised low-resolution satellite sensors and targeted larger water bodies (Hafeez et al., 2019; Hou et al., 2017; Long and Pavelsky, 2013; Sagan et al., 2020).
In the African context, a few studies have looked at the spatiotemporal patterns of suspended sediments and some of these studies, such as Kowe et al. (2023), assessed the spatial and temporal variations of turbidity and total suspended solids in a large lake (between 2017 and 2022) using the Sentinel-2 MSI. Sentinel-2 images were used due to its high spatiotemporal resolution, which makes it suitable for inland water monitoring. The results revealed that the sensor estimated the lowest and highest values of turbidity in 2022 to be 3.01 NTU and 43.96 NTU, respectively with higher turbidity levels concentrated on the lake’s western side. The lowest and highest values of total suspended matter in 2022 were 1.506
These studies show that research about turbidity and total suspended solids has mainly been done on larger water bodies and newer studies focusing on these parameters need to look at their spatiotemporal variability in smaller water bodies.
More optically active water quality parameters that have been studied using satellite sensors include the Secchi disk depth (SDD) which is a parameter that commonly represents water clarity (Yin et al., 2021). Water clarity is affected by parameters such as suspended sediments, coloured dissolved organic matter and chlorophyll-a that change the underwater light field through their concentration in the water and impact water transparency (Maciel et al., 2021). The Secchi Disk, typically 30 cm in diameter and coloured white or black-white, is lowered into the water column until it becomes invisible from the water surface to measure water transparency (Maciel et al., 2021). However, despite how simple and cost-effective the method is, it cannot capture spatial and temporal variations needing a larger sample size to characterise larger areas of statistical significance (Zhang et al., 2021). Due to its advantages, satellite remote sensing emerges as the optimal choice for investigating variations in SDD across various water bodies (including lakes) at various spatiotemporal scales (Zhang et al., 2021).
Various satellite sensors have been used to perform this investigation. For instance, using the MODIS satellite sensor, a study by Shi et al. (2018) aimed to assess the dynamics of Secchi disk depth in the shallow Lake Taihu of China. The study created spectral indices (which were compared with field data) using satellite data to see which one best estimates Secchi disk depth. The index that showed the highest correlation with Secchi disk depth was the MODIS-Aqua reflectance at 645 nm (Reflectance data 645 nm) with a correlation coefficient of 0.76. This created model was able to estimate high Secchi disk concentrations in summer (June-August) and autumn (September-November) compared to the spring (March-May) and winter (December-February). Using high-resolution sensors, another study by Bonansea et al. (2019) aimed to assess the sensitivity of Landsat 8 and Sentinel-2A satellites for estimating and mapping Secchi disk transparency (SDT) in a Cassaffousth Reservoir (Córdoba, Argentina). The study combined ground observation data with datasets from four Landsat-8 OLI and four Sentinel-2A MSI images to develop and validate models for estimating SDT. The results of the study revealed that assessment of the SDT by both sensors was comparable at
The results also revealed that both sensors could estimate SDT using a combination of the blue band and the ratios blue/near-infrared, green/near-infrared for the OLI sensor, and green/near-infrared for the MSI sensor. This capability stems from the way suspended sediments enhance the radiance from the surface water in the visible and near-infrared regions of the electromagnetic spectrum. These studies (Shi et al., 2018; Bonansea et al., 2019) reveal how satellite sensors (low and high-resolution) and retrieval models have been successful in estimating Secchi disk depth in a reservoir or lake. More studies (Liu et al., 2020; Maciel et al., 2021; Yin et al., 2021) have shown that satellite sensors (with high resolutions) can estimate Secchi disk depth but one common aspect that these studies have is how they have looked at Secchi disk depth in lakes (large and shallow lakes). This shows that more studies need to be done in assessing Secchi disk depth concentrations in other water bodies such as small rivers to assess the sensitivity of satellite sensors (particularly high-resolution sensors) in monitoring these parameters.
This section has demonstrated how various satellite sensors have been used in some optically active water quality parameter monitoring. The studies revealed the reflectance abilities of contaminated water using remote sensing technology, demonstrating that optically active water quality parameters are more easily detected compared to non-optically active parameters (Wang and Yang, 2019). However, it is also important to assess how non-optically active water quality parameters have been monitored as they can provide a more comprehensive view of water quality and the overall health of a water body (Zhao et al., 2022).
Assessing non-optically active water quality parameters involves the retrieval of optically active water quality parameters influenced by them (E.g., excessive nitrogen means increased algae and chlorophyll-a concentration). Furthermore, some studies have also detected non-optically active water quality parameters using machine learning algorithms (Leggesse et al., 2023). Using these algorithms with multispectral and high-resolution sensors, such as the MSI on Sentinel-2, in comparison to other low-resolution sensors (Table 4), reveals the potential to provide more detailed and accurate information for detecting water quality parameters. Thus, to detect non-optically active water quality parameters, satellite sensors detect and capture the spectral characteristics of water bodies, and this information is used, along with ground-based measurements and statistical analysis, to identify patterns that correlate with nutrient levels (Guo et al., 2021). Non-optically active components of water quality can also assist in identifying and mapping pollution impacts and sources within water bodies. However, these parameters are difficult to detect directly due to their inability to be influenced by the electromagnetic spectrum but various remote sensing studies have assessed them using indirect methods (Dey and Vijay, 2020).
Some of the most studied non-optically active parameters are nitrogen and phosphorus. Dey and Vijay (2020) mention that nitrogen and phosphorus, nutrients found in algae and cyanobacteria, are strongly correlated with chlorophyll-a, a pigment found in water bodies. Chlorophyll-a serves as an indirect indicator of nutrient presence in water bodies (Fu et al., 2022; Kowe et al., 2023). Thus, satellite sensors can detect the presence of nitrogen and phosphorus nutrients by analysing chlorophyll-a concentrations in water. However, Xiong et al. (2019), dispute this method of indirectly estimating non-optically active water quality parameters. Using MODIS/Aqua data, they developed algorithms for estimating total phosphorus in Lake Hongze in east China, comparing direct and indirect methods. They agree that phosphorus in waters has to be linked to optical substances such as chlorophyll-a as well as the suspended particulate matter (SPM) but point out that phosphorus concentration is influenced by various factors, not just SPM, making indirect estimation less reliable. The direct derivation algorithm based on 645 nm and 1,240 nm MODIS/Aqua provided better results and accuracy (
Another study by Dong et al. (2020) developed an inversion model using the reflectance of various band combinations from Sentinel-2 images, along with water quality monitoring data for total nitrogen (TN) and ammonia nitrogen (NH3-N) at sampling sites in the Danjiangkou Reservoir. Between. 56 band combinations, the correlation coefficient between Band 2 × Band 8 and the measured TN concentration was 0.9301, the highest of all. The inversion model estimated TN and NH3-N concentrations with
Additionally, the Extreme Gradient Boosting (XGBoost) performed fairly well in estimating total nitrogen with an
Monitoring water temperature distribution is crucial to understanding the functioning of water bodies and its influence on the movement and behaviour of contaminants like nutrients (Lamaro et al., 2013). Surface water temperature, in particular, is important because it directly affects the exchange of energy between water and the atmosphere (Lamaro et al., 2013). Temperature can vary due to seasonal shifts, depth, and time of day, which underscores the importance of monitoring it to understand the various physical and biochemical processes in water (Gholizadeh et al., 2016). Ali et al. (2022) assessed water temperature as it influences the productivity of nutrient levels, particularly when total phosphorus levels are below a threshold of approximately 0.4 mg/L highlighting its significance in understanding reservoirs, dams, and lakes. Studies such as Lamaro et al. (2013) have mentioned how the use of satellite sensors such as the Landsat-7 Enhanced Thematic Mapper Plus (ETM+) with its thermal bands has assisted in monitoring water temperatures in a medium-sized reservoir (Argentina). The study applied and compared a single channel generalised method (SCGM) with a Radiative Transfer Method (RTM) to enhance the estimation and it concluded that both methods adequately estimated water temperature with an
Another study by Kramer et al. (2023) aimed to use the Landsat-8 Thermal Infrared Sensor (TIRS) with a developed linear regression model to estimate water surface temperature within the Itaipu Reservoir (Brazil). The study was a success as the model reached an
A study by Zhu and Mao (2021) indirectly assessed temperature concentrations in a freshwater lake in China by incorporating it as an input variable in a model to estimate the trophic state index (TSI) of urban water bodies. By considering temperature alongside other environmental factors and Sentinel-2 data, the study developed a model that accurately predicts TSI levels. This demonstrates how temperature, along with other easily measurable factors, can be utilised in remote sensing-based approaches to assess water quality and trophic state. Non-optically active water quality parameters can, therefore, assist in identifying pollution sources and provide information related to the concentration and retrieval of optically active water quality parameters, displaying how they can vary spatially and seasonally and how this variation affects their optical properties (Sent et al., 2021).
Spatiotemporal monitoring of water quality parameters is crucial to understanding the impacts of urbanisation, intensive land use and land cover (LULC) changes, and population growth on water bodies. Satellite-measured water reflectance plays a critical role in tracking these variations across different water bodies (Chen et al., 2016). Wu et al. (2021) highlight how land use patterns affect water quality differently across spatial and temporal scales and the importance of understanding this. For instance, Umwali et al. (2021) assessed spatial-seasonal variations in water quality across different land use types in Lake Muhazi, Rwanda. They assessed parameters including dissolved oxygen, faecal coliforms (FC), pH, biochemical oxygen demand (BOD), temperature, total phosphorus, nitrate, turbidity, and total solids (TS), finding that turbidity increases due to decaying vegetation and sediment runoff during rainfall events, leading to lake discolouration. Thus, this study displays the relationship between LULC, spatial-seasonal changes, and water quality parameters. It reveals how future studies should further investigate how anthropogenic and natural variables interact with and influence water quality over time and space (Gelsey et al., 2023).
Additionally, Omondi et al. (2023) mapped spatiotemporal patterns of water quality parameters by assessing their distribution and variability in the Chebara Dam of Kenya, using the Sentinel-2 MSI and Landsat-8 OLI. The study focused on parameters such as chlorophyll-a, suspended particulate matter, and turbidity. Results indicated higher reflectance of these parameters at sampling stations T2, T6, T9, and T11, situated at the dam’s inflow edges with shallow depths showing the settling of suspended particulate matter. Chlorophyll-a and suspended particulate matter were effectively retrieved using the visible and Near-Infrared bands, which were recognised as optimal for estimating suspended particulate matter. Thus, analysing spatial and temporal trends helps identify specific locations with good or poor water quality, and this information is valuable for resource allocation and to prioritise water conservation efforts (Behmel et al., 2016). To achieve this, the Sentinel-2 MSI’s high spatial resolution, frequent revisit times, and spectral band configuration present an opportunity to comprehensively assess spatial and seasonal variations in water quality, making it an invaluable tool for monitoring and understanding changes in water bodies over time (Gelsey et al., 2023). This highlights the urgency for stakeholders, including decision-makers, government authorities, mining companies, and water management departments, to update and implement effective water management strategies (Behmel et al., 2016). To update these water management strategies, the use of the Sentinel-2 MSI, with in situ validation and water quality retrieval estimations using machine learning, may be viable.
Machine learning algorithms have become essential for handling spectral data used for assessing water quality parameters (Arias-Rodriguez et al., 2023). These algorithms identify patterns and relationships in complex datasets, capturing spatial and temporal dynamics in satellite images (Tian et al., 2023; Arias-Rodriguez et al., 2023). Machine learning algorithms like Regression Tree, Random Forest, Boosted Regression Tree, Support Vector Regression, and Artificial Neural Networks have been used to monitor water quality parameters in remote sensing data (Li et al., 2022a). For instance, Leggesse et al. (2023) used Landsat-8 imagery and six machine learning algorithms to monitor water quality in Ethiopia’s Lake Tana. The study found that the Extreme Gradient Boosting (XGB) regression model was effective in predicting chlorophyll-a concentration variations in the lake from March to June, while Random Forest regression (RF) performed best in predicting total dissolved solids concentrations during the main rainy season (July to September). This highlights the effectiveness of these models in predicting water quality parameters, even with limited in situ data, enhancing the cost-effectiveness of monitoring efforts.
A study by Kupssinskü et al. (2020) also used Sentinel-2 MSI data, limited field data, the Random Forest model, and Artificial Neural Networks to estimate chlorophyll-a and total suspended solids on a small lake and dam in Brazil. The results revealed that the models achieved
Nevertheless, non-optically active components cannot be directly retrieved using remote sensing due to their lack of influence over the spectral response of water when dissolved (Arias-Rodriguez et al., 2023). Yet, they remain crucial for water quality assessment, and spatiotemporal assessment of water quality parameters and are often detected indirectly from optically active components (Arias-Rodriguez et al., 2023). Guo et al. (2021) also successfully predicted total phosphorus (TP), total nitrogen (TN), and chemical oxygen demand (COD) in a small urban lake using Random Forest Regression and Neural Networks, together with the Sentinel-2 MSI and field data. The study demonstrated a coefficient of determination (
Using these algorithms, Landsat-8 OLI images, and water quality sampling, the study revealed that the Random Forest model and the Neural Networks model both did well in estimating total nitrogen (TN) and total phosphorus (TP) concentrations (with an
Guo et al. (2021), Kupssinskü et al. (2020), Leggesse et al. (2023) and Yang and Jin (2023) have collectively demonstrated the effectiveness of Random Forest models in estimating water quality parameters through remote sensing. Their studies indicate the need for further assessments, particularly in small river ecosystems, and the exploration of additional machine learning algorithms like XGB. Accurate estimation of both optically and non-optically active parameters has the potential to enhance decision-making in managing their sources (Gholizadeh et al., 2016). To enhance water management, it is, therefore, essential to integrate satellite data, field data collection (such as water sampling), and machine learning algorithms (Huang et al., 2020).
The aim of this review was to explore and discuss existing trends in the remote sensing of water quality parameters in inland water bodies while also emphasizing the under exploration of water quality monitoring in smaller water bodies. Publication trends reveal that anthropogenic activities, particularly mining, agriculture, and sewage works, significantly impact water body health. Traditional methods of water quality monitoring have been used to address this challenge; however, they are deemed labor-intensive, point-based, and costly, among other limitations. Increasing publications on remote sensing for water quality monitoring have revealed that satellite sensors offer a better alternative. Remote sensing provides the advantage of large-scale monitoring, cost-effectiveness, and near real-time assessments. With high-resolution sensors, this method can assess both optically active and non-optically active water quality parameters. Publication trends in this review revealed that low-resolution sensors like the widely used MODIS are suitable for large water bodies such as ocean waters and larger inland water bodies like lakes. Although MODIS is a multispectral sensor with a high temporal resolution, it has limitations in monitoring small inland water bodies such as rivers due to larger pixel sizes (lower spatial resolution).
Studies reveal that higher-resolution sensors, like the Sentinel-2 MSI, are more suitable for monitoring water quality parameters in these areas. These sensors can provide better accuracy in estimating water quality parameters in small or narrow rivers due to finer spatial resolution and the added advantage of high temporal resolution. Publication trends also reveal a growing number of studies that utilise the higher-resolution Landsat-8 OLI and the Sentinel-2 MSI to monitor inland water bodies (including smaller ones) between 2022 and 2024. This is attributed not only to their high resolutions but also to their accessibility, even to developing countries, particularly in Africa. Studies in this review, however, highlight the limitation of satellite remote sensing in monitoring non-optically active water quality parameters such as nitrogen, phosphorus, and temperature, among others. Non-optically active water quality parameters are revealed to lack optical signals that can assist in their retrieval, causing their assessment to require solutions that address complex relationships. Publication trends reveal an increasing use of machine learning models to address this challenge (together with in situ data), which has improved the accuracy and efficiency of retrieving not only optically active water quality parameters but also enhanced the retrieval of non-optically active parameters with rigorous machine learning models.
Studies have used popular models such as random forest, neural networks, and eXtreme Gradient Boosting, which is becoming a popular choice for regression, classification, and ranking problems. Future studies need to explore more underutilised machine learning models to assess their accuracy in estimating non-optically active parameters, particularly on lakes and dams. In relation to inversion methods for remote sensing of water quality monitoring, this review focused on empirical methods, particularly machine learning. Publication trends have also shown increasing studies in this topic. Therefore, future studies need to focus on more inversion methods, including semi-empirical models and radiative transfer models, to effectively monitor non-optically active water quality parameters. This review has highlighted the increasing trends and gaps in water quality monitoring of inland water bodies, with the Sentinel-2 MSI revealed to be a widely used satellite sensor for inland water bodies considered small. The fact that it has a high spatial resolution reaching 10 m provides an advantage for extensive water quality monitoring. However, multispectral satellite sensors like the Sentinel-2 MSI still face challenges in predicting water quality parameters in water bodies with heterogeneous ecosystems, such as those containing both green and blue-green algae with comparable spectral profiles. This underscores the need for future studies to further explore the use of hyperspectral sensors, which have much finer spectral resolution, in water quality monitoring. Hyperspectral sensors may be more suitable for assessing the quality of complex small inland water bodies and can enhance remote sensing-based water quality monitoring.
Atmospheric correction errors also still hinder the accuracy of using satellite sensors for water quality monitoring. Studies reveal that it is crucial to find a suitable algorithm for correcting atmospheric errors on satellite sensor images tailored to specific inland water bodies or study areas. For example, the iCOR atmospheric correction algorithm can be used to perform atmospheric correction on the Sentinel-2 MSI when it is used to study inland water bodies. Thus, there is a need for future studies to further explore these satellite remote sensing limitations. This review, therefore, demonstrates that combining high-resolution satellite data, field data, and machine learning algorithms can enhance the accuracy of water quality assessments. Such advancements can significantly improve decision-making processes for South Africa’s water management policies and contribute to the sustainable management of water resources.
SN: Conceptualization, Investigation, Methodology, Writing–original draft. SM: Project administration, Supervision, Writing–review and editing. MK: Funding acquisition, Project administration, Resources, Supervision, Writing–review and editing.
The author(s) declare that financial support was received for the research, authorship, and/or publication of this article. This work was supported by Water Research Commission (WRC) project: Monitoring Surface Water Quality Using Remote Sensing Technology (Project number: C2023/2024-01241).
The publication costs will be funded by the Earth Observation division of the South African National Space Agency (SANSA).
The authors declare that the research was conducted in the absence of any commercial or financial relationships that could be construed as a potential conflict of interest.
The author(s) declare that no Generative AI was used in the creation of this manuscript.
All claims expressed in this article are solely those of the authors and do not necessarily represent those of their affiliated organizations, or those of the publisher, the editors and the reviewers. Any product that may be evaluated in this article, or claim that may be made by its manufacturer, is not guaranteed or endorsed by the publisher.
Abbas, M. M., Melesse, A. M., Scinto, L. J., and Rehage, J. S. (2019). Satellite estimation of chlorophyll-a using moderate resolution imaging spectroradiometer (MODIS) sensor in shallow coastal water bodies: validation and improvement. WaterSwitzerl. 11, 1621. doi:10.3390/w11081621
Abdelal, Q., Assaf, M. N., Al-Rawabdeh, A., Arabasi, S., and Rawashdeh, N. A. (2022). Assessment of sentinel-2 and landsat-8 OLI for small-scale inland water quality modeling and monitoring based on handheld hyperspectral ground truthing. J. Sens. 2022, 1–19. doi:10.1155/2022/4643924
Adjovu, G. E., Stephen, H., James, D., and Ahmad, S. (2023). Overview of the application of remote sensing in effective monitoring of water quality parameters. Remote Sens. (Basel) 15, 1938. doi:10.3390/rs15071938
Ahmed, T., Zounemat-Kermani, M., and Scholz, M. (2020). Climate change, water quality and water-related challenges: a review with focus on Pakistan. Int. J. Environ. Res. Public Health 17, 8518–8522. doi:10.3390/ijerph17228518
Ali, K., Abiye, T., and Adam, E. (2022). Integrating in situ and current generation satellite data for temporal and spatial analysis of harmful algal blooms in the hartbeespoort dam, crocodile River Basin, South Africa. Remote Sens. (Basel) 14, 4277. doi:10.3390/rs14174277
Al-Shaibah, B., Liu, X., Zhang, J., Tong, Z., Zhang, M., El-Zeiny, A., et al. (2021). Modeling water quality parameters using landsat multispectral images: a case study of erlong lake, northeast China. Remote Sens. (Basel) 13, 1603. doi:10.3390/rs13091603
Altenburger, R., Brack, W., Burgess, R. M., Busch, W., Escher, B. I., Focks, A., et al. (2019). Future water quality monitoring: improving the balance between exposure and toxicity assessments of real-world pollutant mixtures. Environ. Sci. Eur. 31, 12. doi:10.1186/s12302-019-0193-1
Arabi, B., Salama, M. S., Pitarch, J., and Verhoef, W. (2020). Integration of in-situ and multi-sensor satellite observations for long-term water quality monitoring in coastal areas. Remote Sens. Environ. 239, 111632. doi:10.1016/j.rse.2020.111632
Arias-Rodriguez, L. F., Tüzün, U. F., Duan, Z., Huang, J., Tuo, Y., and Disse, M. (2023). Global water quality of inland waters with harmonized landsat-8 and sentinel-2 using cloud-computed machine learning. Remote Sens. (Basel) 15, 1390. doi:10.3390/rs15051390
Arora, M., Mudaliar, A., and Pateriya, B. (2022). Assessment and monitoring of optically active water quality parameters on wetland ecosystems based on remote sensing approach: a case study on Harike and keshopur wetland over Punjab region, India. Eng. Proc. 27, 84. doi:10.3390/ecsa-9-13361
Attila, J., Kauppila, P., Kallio, K. Y., Alasalmi, H., Keto, V., Bruun, E., et al. (2018). Applicability of Earth Observation chlorophyll-a data in assessment of water status via MERIS — with implications for the use of OLCI sensors. Remote Sens. Environ. 212, 273–287. doi:10.1016/j.rse.2018.02.043
Bai, Y., Zhang, S., Mu, E., Zhao, Y., Cheng, L., Zhu, Y., et al. (2023). Characterizing the spatiotemporal distribution of dissolved organic matter (DOM) in the Yongding River Basin: insights from flow regulation. J. Environ. Manage 325, 116476. doi:10.1016/j.jenvman.2022.116476
Bangira, T., Matongera, T. N., Mabhaudhi, T., and Mutanga, O. (2024). Remote sensing-based water quality monitoring in African reservoirs, potential and limitations of sensors and algorithms: a systematic review. Phys. Chem. Earth 134, 103536. doi:10.1016/j.pce.2023.103536
Barakat, A., El Baghdadi, M., Rais, J., Aghezzaf, B., and Slassi, M. (2016). Assessment of spatial and seasonal water quality variation of Oum Er Rbia River (Morocco) using multivariate statistical techniques. Int. Soil Water Conservation Res. 4, 284–292. doi:10.1016/j.iswcr.2016.11.002
Behmel, S., Damour, M., Ludwig, R., and Rodriguez, M. J. (2016). Water quality monitoring strategies — a review and future perspectives. Sci. Total Environ. 571, 1312–1329. doi:10.1016/j.scitotenv.2016.06.235
Bonansea, M., Ledesma, M., Rodriguez, C., and Pinotti, L. (2019). Using new remote sensing satellites for assessing water quality in a reservoir. Hydrological Sci. J. 64, 34–44. doi:10.1080/02626667.2018.1552001
Bramich, J., Bolch, C. J. S., and Fischer, A. (2021). Improved red-edge chlorophyll-a detection for Sentinel 2. Ecol. Indic. 120, 106876. doi:10.1016/j.ecolind.2020.106876
Bresciani, M., Giardino, C., Stroppiana, D., Dessena, M. A., Buscarinu, P., Cabras, L., et al. (2019). Monitoring water quality in two dammed reservoirs from multispectral satellite data. Eur. J. Remote Sens. 52, 113–122. doi:10.1080/22797254.2019.1686956
Caballero, I., Steinmetz, F., and Navarro, G. (2018). Evaluation of the first year of operational Sentinel-2A data for retrieval of suspended solids in medium-to high-turbiditywaters. Remote Sens. (Basel) 10, 982. doi:10.3390/rs10070982
Chathuranika, I. M., Sachinthanie, E., Zam, P., Gunathilake, M. B., Denkar, D., Muttil, N., et al. (2023). Assessing the water quality and status of water resources in urban and rural areas of Bhutan. J. Hazard. Mater. Adv. 12, 100377. doi:10.1016/j.hazadv.2023.100377
Chavula, G., Brezonik, P., Thenkabail, P., Johnson, T., and Bauer, M. (2009). Estimating chlorophyll concentration in Lake Malawi from MODIS satellite imagery. Phys. Chem. Earth 34, 755–760. doi:10.1016/j.pce.2009.07.015
Chebud, Y., Naja, G. M., Rivero, R. G., and Melesse, A. M. (2012). Water quality monitoring using remote sensing and an artificial neural network. Water Air Soil Pollut. 223, 4875–4887. doi:10.1007/s11270-012-1243-0
Chen, H., Tan, C., Peng, H., Yang, W., and Li, L. (2024). A qualitative study of water quality using landsat 8 and station water quality-monitoring data to support SDG 6.3.2 evaluations: a case study of deqing, China. WaterSwitzerl. 16, 1319. doi:10.3390/w16101319
Chen, X., Zhou, W., Pickett, S. T. A., Li, W., and Han, L. (2016). Spatial-temporal variations of water quality and its relationship to land use and land cover in Beijing, China. Int. J. Environ. Res. Public Health 13, 449. doi:10.3390/ijerph13050449
Coraggio, E., Han, D., Gronow, C., and Tryfonas, T. (2022). Water quality sampling frequency analysis of surface freshwater: a case study on bristol floating harbour. Front. Sustain. Cities 3. doi:10.3389/frsc.2021.791595
Demetillo, A. T., Japitana, M. V., and Taboada, E. B. (2019). A system for monitoring water quality in a large aquatic area using wireless sensor network technology. Sustain. Environ. Res. 1, 12. doi:10.1186/s42834-019-0009-4
Deng, J., Chen, F., Hu, W., Lu, X., Xu, B., and Hamilton, D. P. (2019). Variations in the distribution of chl-a and simulation using a multiple regression model. Int. J. Environ. Res. Public Health 16, 4553. doi:10.3390/ijerph16224553
Dey, J., and Vijay, R. (2020). A critical and intensive review on assessment of water quality parameters through geospatial techniques. doi:10.1007/s11356-021-14726-4/Published
Dong, G., Hu, Z., Liu, X., Fu, Y., and Zhang, W. (2020). Spatio-temporal variation of total nitrogen and ammonia nitrogen in the water source of the middle route of the south-to-north water diversion project. WaterSwitzerl. 12, 2615. doi:10.3390/W12092615
Duan, M., Qiu, Z., Li, R., Li, K., Yu, S., and Liu, D. (2024). Monitoring suspended sediment transport in the lower Yellow River using landsat observations. Remote Sens. (Basel) 16, 229. doi:10.3390/rs16020229
Dube, T., Mutanga, O., Seutloali, K., Adelabu, S., and Shoko, C. (2015). Water quality monitoring in sub-Saharan African lakes: a review of remote sensing applications. Afr. J. Aquat. Sci. 40, 1–7. doi:10.2989/16085914.2015.1014994
Edokpayi, J. N., Odiyo, J. O., and Durowoju, O. S. (2017). Impact of wastewater on surface water quality in developing countries: a case study of South Africa. Water Qual. (InTech). doi:10.5772/66561
Eon, R., Wenny, B. N., Poole, E., Eftekharzadeh Kay, S., Montanaro, M., Gerace, A., et al. (2024). Landsat 9 thermal infrared sensor-2 (TIRS-2) pre- and post-launch spatial response performance. Remote Sens. (Basel) 16, 1065. doi:10.3390/rs16061065
Flemming, N. C., Vallerga, S., Pinardi, N., Behrens, H. W. A., Manzella, G., Prandle, D., et al. (2002). The use of SeaWiFS for operational monitoring of water quality in the North Sea, 481, 487. doi:10.1016/s0422-9894(02)80054-x
Frasson, R. P. M., Ardila, D. R., Pease, J., Hestir, E., Bright, C., Carter, N., et al. (2024). The impact of spatial resolution on inland water quality monitoring from space. Environ. Res. Commun. 6, 101003. doi:10.1088/2515-7620/ad7dd8
Fu, B., Lao, Z., Liang, Y., Sun, J., He, X., Deng, T., et al. (2022). Evaluating optically and non-optically active water quality and its response relationship to hydro-meteorology using multi-source data in Poyang Lake, China. Ecol. Indic. 145, 109675. doi:10.1016/j.ecolind.2022.109675
Gao, L., Shangguan, Y., Sun, Z., Shen, Q., and Shi, Z. (2024). Estimation of non-optically active water quality parameters in zhejiang province based on machine learning. Remote Sens. (Basel) 16, 514. doi:10.3390/rs16030514
Gelsey, K., Chang, H., and Ramirez, D. (2023). Effects of landscape characteristics, anthropogenic factors, and seasonality on water quality in Portland, Oregon. Environ. Monit. Assess. 195, 219. doi:10.1007/s10661-022-10821-2
Ghasempour, F., Sekertekin, A., and Kutoglu, S. H. (2023). “How Landsat 9 is superior to Landsat 8: Comparative assessment of land use land cover classification and land surface temperature,” ISPRS Annals of the Photogrammetry, Remote Sensing and Spatial Information Sciences, X-4/W1-2022, 221–227. doi:10.5194/isprs-annals-X-4-W1-2022-221-2023
Gholizadeh, M. H., Melesse, A. M., and Reddi, L. (2016). A comprehensive review on water quality parameters estimation using remote sensing techniques. Sensors Switz. 16, 1298. doi:10.3390/s16081298
Goyens, C., Lavigne, H., Dille, A., and Vervaeren, H. (2022). Using hyperspectral remote sensing to monitor water quality in drinking water reservoirs. Remote Sens. (Basel) 14, 5607. doi:10.3390/rs14215607
Groom, S. B., Sathyendranath, S., Ban, Y., Bernard, S., Brewin, B., Brotas, V., et al. (2019). Satellite ocean colour: current status and future perspective. Front. Mar. Sci. 6, 1–30. doi:10.3389/fmars.2019.00485
Guo, H., Huang, J. J., Chen, B., Guo, X., and Singh, V. P. (2021). A machine learning-based strategy for estimating non-optically active water quality parameters using Sentinel-2 imagery. Int. J. Remote Sens. 42, 1841–1866. doi:10.1080/01431161.2020.1846222
Guo, J., Wang, X., Liu, B., Liu, K., Zhang, Y., and Wang, C. (2023). Remote-sensing extraction of small water bodies on the loess plateau, Remote-Sensing Extr. Small Water Bodies Loess Plateau 15. 866. doi:10.3390/w15050866
Gupta, A., and Singh, M. R. (2016). Water pollution-Sources,Effects and control. Available at: https://www.researchgate.net/publication/321289637.
Hafeez, S., Wong, M. S., Ho, H. C., Nazeer, M., Nichol, J., Abbas, S., et al. (2019). Comparison of machine learning algorithms for retrieval of water quality indicators in case-ii waters: a case study of Hong Kong. Remote Sens. (Basel) 11, 617. doi:10.3390/rs11060617
Harmel, R. D., Preisendanz, H. E., King, K. W., Busch, D., Birgand, F., and Sahoo, D. (2023). A review of data quality and cost considerations for water quality monitoring at the field scale and in small watersheds, A Rev. Data Qual. Cost Considerations Water Qual. Monit. A. T. Field Scale Small Watersheds. 15. 3110. doi:10.3390/w15173110
Hobbs, P. J. (2017). TDS load contribution from acid mine drainage to hartbeespoort Dam, South Africa. Water sa. 43, 626–637. doi:10.4314/wsa.v43i4.10
Hou, X., Feng, L., Duan, H., Chen, X., Sun, D., and Shi, K. (2017). Fifteen-year monitoring of the turbidity dynamics in large lakes and reservoirs in the middle and lower basin of the Yangtze River, China. Remote Sens. Environ. 190, 107–121. doi:10.1016/j.rse.2016.12.006
Huang, J. J., Guo, H., Chen, B., Guo, X., and Singh, V. P. (2020). Retrieval of non-optically active parameters for small scale urban waterbodies by a machine learning-based strategy. doi:10.20944/preprints202004.0111.v1
Jafarbiglu, H., and Pourreza, A. (2022). A comprehensive review of remote sensing platforms, sensors, and applications in nut crops. Comput. Electron Agric. 197, 106844. doi:10.1016/j.compag.2022.106844
Jaji, M. O., Bamgbose, O., Odukoya, O. O., and Arowolo, T. A. (2007). Water quality assessment of ogun river, south west Nigeria. Environ. Monit. Assess. 133, 473–482. doi:10.1007/s10661-006-9602-1
Jaywant, S. A., and Arif, K. M. (2024). Remote sensing techniques for water quality monitoring: a review. Sensors 24, 8041. doi:10.3390/s24248041
Jiang, D., Scholze, J., Liu, X., Simis, S. G. H., Stelzer, K., Müller, D., et al. (2023). A data-driven approach to flag land-affected signals in satellite derived water quality from small lakes. Int. J. Appl. Earth Observation Geoinformation 117, 103188. doi:10.1016/j.jag.2023.103188
Johansen, R., Beck, R., Nowosad, J., Nietch, C., Xu, M., Shu, S., et al. (2018). Evaluating the portability of satellite derived chlorophyll-a algorithms for temperate inland lakes using airborne hyperspectral imagery and dense surface observations. Harmful Algae 76, 35–46. doi:10.1016/j.hal.2018.05.001
Kalaitzidou, K., Ntona, M. M., Zavridou, E., Tzeletas, S., Patsialis, T., Kallioras, A., et al. (2023). Water quality evaluation of groundwater and dam reservoir water: application of the water quality index to study sites in Greece. WaterSwitzerl. 15, 4170. doi:10.3390/w15234170
Kowe, P., Ncube, E., Magidi, J., Ndambuki, J. M., Rwasoka, D. T., Gumindoga, W., et al. (2023). Spatial-temporal variability analysis of water quality using remote sensing data: a case study of Lake Manyame. Sci. Afr. 21, e01877. doi:10.1016/j.sciaf.2023.e01877
Kramer, G., Filho, W. P., de Carvalho, L. A. S., Trindade, P. M. P., da Rosa, C. N., and Dezordi, R. (2023). Performance and validation of water surface temperature estimates from landsat 8 of the Itaipu reservoir, state of paraná, Brazil. Environ. Monit. Assess. 195, 137. doi:10.1007/s10661-022-10677-6
Kratzer, S., Vinterhav, C., Kratzer, S., and Vinterhav, C. (2010). Improvement of MERIS level 2 products in Baltic Sea coastal areas by applying the Improved Contrast between Ocean and Land processor (ICOL)-data analysis and validation* MERIS full resolution data Optical case 2 waters Adjacency effect Algorithm development MERIS standard processor FUB processor C2R processor. Available at: http://www.iopan.gda.pl/oceanologia/212.
Kumar, S., Kou, R., Hill, H., Lempges, J., Qian, E., and Jayaram, V. (2023). In-situ Water quality monitoring in Oil and Gas operations. 26. doi:10.1117/12.2663076
Kupssinskü, L. S., Guimarães, T. T., De Souza, E. M., Zanotta, D. C., Veronez, M. R., Gonzaga, L., et al. (2020). A method for chlorophyll-a and suspended solids prediction through remote sensing and machine learning. Sensors Switz. 20, 2125. doi:10.3390/s20072125
Lal, K., Jaywant, S. A., and Arif, K. M. (2023). Electrochemical and optical sensors for real-time detection of nitrate in water. Sensors 23, 7099. doi:10.3390/s23167099
Lamaro, A. A., Mariñelarena, A., Torrusio, S. E., and Sala, S. E. (2013). Water surface temperature estimation from Landsat 7 ETM+ thermal infrared data using the generalized single-channel method: Case study of Embalse del Río Tercero (Córdoba, Argentina). Adv. Space Res. 51, 492–500. doi:10.1016/j.asr.2012.09.032
Leggesse, E. S., Zimale, F. A., Sultan, D., Enku, T., Srinivasan, R., and Tilahun, S. A. (2023). Predicting optical water quality indicators from remote sensing using machine learning algorithms in tropical highlands of Ethiopia. Hydrology 10, 110. doi:10.3390/hydrology10050110
Li, J., Ma, R., Cao, Z., Xue, K., Xiong, J., Hu, M., et al. (2022a). Satellite detection of surface water extent: a review of methodology. WaterSwitzerl. 14, 1148. doi:10.3390/w14071148
Li, L., Gu, M., Gong, C., Hu, Y., Wang, X., Yang, Z., et al. (2023). An advanced remote sensing retrieval method for urban non-optically active water quality parameters: an example from Shanghai. Sci. Total Environ. 880, 163389. doi:10.1016/j.scitotenv.2023.163389
Li, N., Ning, Z., Chen, M., Wu, D., Hao, C., Zhang, D., et al. (2022b). Satellite and machine learning monitoring of optically inactive water quality variability in a tropical river. Remote Sens. (Basel) 14, 5466. doi:10.3390/rs14215466
Liber, K., Goodfellow, W., Den Besten, P., Clements, W., Galloway, T., Gerhardt, A., et al. (2016). in Situ-based effects measures: considerations for improving methods and approaches.
Liu, C., Zhang, F., Jim, C. Y., Oke, S. A., and Adam, E. (2024a). Evaluation of atmospheric correction algorithms for salt lake water assessment: accuracy, band-specific effects, and sensor consistency. PLoS One 19, e0315837. doi:10.1371/journal.pone.0315837
Liu, D., Duan, H., Loiselle, S., Hu, C., Zhang, G., Li, J., et al. (2020). Observations of water transparency in China’s lakes from space. Int. J. Appl. Earth Observation Geoinformation 92, 102187. doi:10.1016/j.jag.2020.102187
Liu, M., Wang, L., and Qiu, F. (2022). Using MODIS data to track the long-term variations of dissolved oxygen in Lake Taihu. Front. Environ. Sci. 10. doi:10.3389/fenvs.2022.1096843
Liu, S., Kim, S., Glamore, W., Tamburic, B., and Johnson, F. (2024b). Remote sensing of water colour in small southeastern Australian waterbodies. J. Environ. Manage 352, 120096. doi:10.1016/j.jenvman.2024.120096
Llodrà-Llabrés, J., Martínez-López, J., Postma, T., Pérez-Martínez, C., and Alcaraz-Segura, D. (2023). Retrieving water chlorophyll-a concentration in inland waters from Sentinel-2 imagery: review of operability, performance and ways forward. Int. J. Appl. Earth Observation Geoinformation 125, 103605. doi:10.1016/j.jag.2023.103605
Long, C. M., and Pavelsky, T. M. (2013). Remote sensing of suspended sediment concentration and hydrologic connectivity in a complex wetland environment. Remote Sens. Environ. 129, 197–209. doi:10.1016/j.rse.2012.10.019
Lulla, K., Nellis, M. D., Rundquist, B., Srivastava, P. K., and Szabo, S. (2021). Mission to earth: LANDSAT 9 will continue to view the world. Geocarto Int. 36, 2261–2263. doi:10.1080/10106049.2021.1991634
Maciel, D. A., Barbosa, C. C. F., Novo, E. M. L. de M., Flores Júnior, R., and Begliomini, F. N. (2021). Water clarity in Brazilian water assessed using Sentinel-2 and machine learning methods. ISPRS J. Photogrammetry Remote Sens. 182, 134–152. doi:10.1016/j.isprsjprs.2021.10.009
Majozi, N. P., Salama, M. S., Bernard, S., Harper, D. M., and Habte, M. G. (2014). Remote sensing of euphotic depth in shallow tropical inland waters of Lake Naivasha using MERIS data. Remote Sens. Environ. 148, 178–189. doi:10.1016/j.rse.2014.03.025
Mamun, M., Hasan, M., and An, K. G. (2024). Advancing reservoirs water quality parameters estimation using Sentinel-2 and Landsat-8 satellite data with machine learning approaches. Ecol. Inf. 81, 102608. doi:10.1016/j.ecoinf.2024.102608
Matthews, M. W., and Bernard, S. (2015). Eutrophication and cyanobacteria in South Africa’s standing water bodies: a view from space. S Afr. J. Sci. 111, 1–8. doi:10.17159/sajs.2015/20140193
McClain, C. R., Meister, G., and Monosmith, B. (2014). “Satellite ocean color sensor design concepts and performance requirements,” in Experimental methods in the physical sciences (Academic Press), 73–119. doi:10.1016/B978-0-12-417011-7.00005-2
Mpakairi, K. S., Muthivhi, F. F., Dondofema, F., Munyai, L. F., and Dalu, T. (2024). Chlorophyll-a unveiled: unlocking reservoir insights through remote sensing in a subtropical reservoir. Environ. Monit. Assess. 196, 401. doi:10.1007/s10661-024-12554-w
Mujathel, A. M., El-Barky, W., Fayed, M., and Aly, S. A. (2022). Trihalomethane evaluation in chlorinated treated drinking water sources in Saudi Arabia (Aseer region a case study). Alexandria Eng. J. 61, 12699–12711. doi:10.1016/j.aej.2022.06.043
Munyai, L. F., Dondofema, F., Banda, K., Mutoti, M. I., and Gumbo, J. R. (2022). Satellite derived estimation of chlorophyll-a on harmful algal blooms (HABs) in selected dams of vhembe district, limpopo province. Int. J. Environ. Impacts 5, 362–374. doi:10.2495/EI-V5-N4-362-374
Murray, C., Larson, A., Goodwill, J., Wang, Y., Cardace, D., and Akanda, A. S. (2022). Water quality observations from space: a review of critical issues and challenges. Environ. - MDPI 9 9, 125. doi:10.3390/environments9100125
Mushore, T. D., Odindi, J., Slotow, R., and Mutanga, O. (2023). Remote sensing-based outdoor thermal comfort assessment in local climate zones in the rural–urban continuum of eThekwini municipality, South Africa. Remote Sens. (Basel) 15, 5461. doi:10.3390/rs15235461
Myint, S. W., and Walker, N. D. (2002). Quantification of surface suspended sediments along a river dominated coast with NOAA AVHRR and Sea WiFS measurements: Louisiana, USA. Int. J. Remote Sens. 23, 3229–3249. doi:10.1080/01431160110104700
Ndou, N. (2023). Geostatistical inference of Sentinel-2 spectral reflectance patterns to water quality indicators in the Setumo dam, South Africa. Remote Sens. Appl. 30, 100945. doi:10.1016/j.rsase.2023.100945
Niroumand-Jadidi, M., Bovolo, F., Bresciani, M., Gege, P., and Giardino, C. (2022). Water quality retrieval from landsat-9 (OLI-2) imagery and comparison to sentinel-2. Remote Sens. (Basel) 14, 4596. doi:10.3390/rs14184596
Obaid, A. A., Ali, K. A., Abiye, T. A., and Adam, E. M. (2021). Assessing the utility of using current generation high-resolution satellites (Sentinel 2 and Landsat 8) to monitor large water supply dam in South Africa. Remote Sens. Appl. 22, 100521. doi:10.1016/j.rsase.2021.100521
Odermatt, D., Heege, T., Nieke, J., Kneubühler, M., and Itten, K. (2008). Water quality monitoring for lake constance with a physically based algorithm for MERIS data. Sensors 8, 4582–4599. doi:10.3390/s8084582
Ogashawara, I., and Moreno-Madriñán, M. J. (2014). Improving inland water quality monitoring through remote sensing techniques. ISPRS Int. J. Geoinf 3, 1234–1255. doi:10.3390/ijgi3041234
Oliphant, T., Tsoeleng, L. T., Mhangara, P., and Malahlela, O. E. (2018). Mapping chlorophyll-a concentrations in a cyanobacteria- and algae-impacted Vaal Dam using Landsat 8 OLI data. S Afr. J. Sci. 114. doi:10.17159/sajs.2018/4841
Olmanson, L. G., Brezonik, P. L., and Bauer, M. E. (2011). Evaluation of medium to low resolution satellite imagery for regional lake water quality assessments. Water Resour. Res. 47. doi:10.1029/2011WR011005
Omondi, A. N., Ouma, Y., Kosgei, J. R., Kongo, V., Kemboi, E. J., Njoroge, S. M., et al. (2023). Estimation and mapping of water quality parameters using satellite images: a case study of Two Rivers Dam, Kenya. Water Pract. Technol. 18, 428–443. doi:10.2166/wpt.2023.010
Osman, S. O., Mohamed, M. Z., Suliman, A. M., and Mohammed, A. A. (2018). “Design and implementation of a low-cost real-time in-situ drinking water quality monitoring system using Arduino,” in 2018 International Conference on Computer, Control, Electrical, and Electronics Engineering (ICCCEEE 2018), Khartoum, Sudan. Institute of Electrical and Electronics Engineers (IEEE). doi:10.1109/ICCCEEE.2018.8515886
Page, M. J., Moher, D., Bossuyt, P. M., Boutron, I., Hoffmann, T. C., Mulrow, C. D., et al. (2021). PRISMA 2020 explanation and elaboration: updated guidance and exemplars for reporting systematic reviews. BMJ 372, n160. doi:10.1136/bmj.n160
Park, E., and Latrubesse, E. M. (2014). Modeling suspended sediment distribution patterns of the Amazon River using MODIS data. Remote Sens. Environ. 147, 232–242. doi:10.1016/j.rse.2014.03.013
Parris, K. (2011). Impact of agriculture on water pollution in OECD countries: recent trends and future prospects. Int. J. Water Resour. Dev. 27, 33–52. doi:10.1080/07900627.2010.531898
Pizani, F. M. C., Maillard, P., Ferreira, A. F. F., and De Amorim, C. C. (2020). “Estimation of water quality in a reservoir from sentinel-2 msi and landsat-8 oli sensors ISPRS Annals of the Photogrammetry,” in Remote Sensing and spatial information sciences, (copernicus GmbH), 401–408. doi:10.5194/isprs-Annals-V-3-2020-401-2020
Randhawa, S., Sandha, S. S., and Srivastava, B. (2016). A multi-sensor process for in-situ monitoring of water pollution in rivers or lakes for high-resolution quantitative and qualitative water quality data. , 122, 129. doi:10.1109/cse-euc-dcabes.2016.171
Reinart, A. (2005). Water quality monitoring of large european lakes using meris full resolution data. Available at: http://envisat.esa.int/services/beam/.
Reinart, A., and Pierson, D. (2014). Water quality monitoring of large european lakes using meris full resolution data. water quality monitoring of large european lakes using meris full resolution data. Available at: http://envisat.esa.int/services/beam/.
Rocha, F. C., Andrade, E. M., and Lopes, F. B. (2015). Water quality index calculated from biological, physical and chemical attributes. Environ. Monit. Assess. 187, 4163. doi:10.1007/s10661-014-4163-1
Rodrigues, G., Potes, M., Penha, A. M., Costa, M. J., and Morais, M. M. (2022). The use of sentinel-3/OLCI for monitoring the water quality and optical water types in the largest Portuguese reservoir. Remote Sens. (Basel) 14, 2172. doi:10.3390/rs14092172
Ryu, J. H., Han, H. J., Cho, S., Park, Y. J., and Ahn, Y. H. (2012). Overview of geostationary ocean color imager (GOCI) and GOCI data processing system (GDPS). Ocean Sci. J. 47, 223–233. doi:10.1007/s12601-012-0024-4
Saberioon, M., Brom, J., Nedbal, V., Souc̆ek, P., and Císar̆, P. (2020). Chlorophyll-a and total suspended solids retrieval and mapping using Sentinel-2A and machine learning for inland waters. Ecol. Indic. 113, 106236. doi:10.1016/j.ecolind.2020.106236
Sagan, V., Peterson, K. T., Maimaitijiang, M., Sidike, P., Sloan, J., Greeling, B. A., et al. (2020). Monitoring inland water quality using remote sensing: potential and limitations of spectral indices, bio-optical simulations, machine learning, and cloud computing. Earth Sci. Rev. 205, 103187. doi:10.1016/j.earscirev.2020.103187
Sent, G., Biguino, B., Favareto, L., Cruz, J., Sá, C., Dogliotti, A. I., et al. (2021). Deriving water quality parameters using sentinel-2 imagery: a case study in the Sado Estuary, Portugal. Remote Sens. (Basel) 13, 1043–1130. doi:10.3390/rs13051043
Shi, K., Zhang, Y., Zhu, G., Qin, B., and Pan, D. (2018). Deteriorating water clarity in shallow waters: evidence from long term MODIS and in-situ observations. Int. J. Appl. Earth Observation Geoinformation 68, 287–297. doi:10.1016/j.jag.2017.12.015
Sigopi, M., Shoko, C., and Dube, T. (2024). Advancements in remote sensing technologies for accurate monitoring and management of surface water resources in Africa: an overview, limitations, and future directions. Geocarto Int. 39. doi:10.1080/10106049.2024.2347935
Sivakumar, M. V. K., Roy, P. S., Harmsen, K., and Saha, S. K. (Editors) (2004). “Satellite remote sensing and GIS applications in agricultural meteorology,” in Proceedings of the training workshop, Dehra Dun, India, July 7–11, 2003. (WMO/TD No. 1182) (World Meteorological Organization). Available at: https://www.unisdr.org/files/1682_9970.pdf.
Soomets, T., Uudeberg, K., Jakovels, D., Brauns, A., Zagars, M., and Kutser, T. (2020). Validation and comparison of water quality products in baltic lakes using sentinel-2 msi and sentinel-3 OLCI data. Sensors Switz. 20, 742. doi:10.3390/s20030742
Sultana, M. S., and Dewan, A. (2021). A reflectance-based water quality index and its application to examine degradation of river water quality in a rapidly urbanising megacity. Environ. Adv. 5, 100097. doi:10.1016/j.envadv.2021.100097
Sun, Y., Wang, D., Li, L., Ning, R., Yu, S., and Gao, N. (2024). Application of remote sensing technology in water quality monitoring: from traditional approaches to artificial intelligence. Water Res. 267, 122546. doi:10.1016/j.watres.2024.122546
Swain, R., and Sahoo, B. (2017). Improving river water quality monitoring using satellite data products and a genetic algorithm processing approach. Sustain. Water Qual. Ecol. 9–10, 88–114. doi:10.1016/j.swaqe.2017.09.001
Tesfaye, A. (2024). Remote sensing-based water quality parameters retrieval methods: a review. East Afr. J. Environ. Nat. Resour. 7, 80–97. doi:10.37284/eajenr.7.1.1753
Tian, S., Guo, H., Xu, W., Zhu, X., Wang, B., Zeng, Q., et al. (2023). Remote sensing retrieval of inland water quality parameters using Sentinel-2 and multiple machine learning algorithms. Environ. Sci. Pollut. Res. 30, 18617–18630. doi:10.1007/s11356-022-23431-9
Topp, S. N., Pavelsky, T. M., Jensen, D., Simard, M., and Ross, M. R. V. (2020). Research trends in the use of remote sensing for inland water quality science: moving towards multidisciplinary applications. WaterSwitzerl. 12, 169. doi:10.3390/w12010169
Trevisiol, F., Mandanici, E., Pagliarani, A., and Bitelli, G. (2024). Evaluation of Landsat-9 interoperability with Sentinel-2 and Landsat-8 over Europe and local comparison with field surveys. ISPRS J. Photogrammetry Remote Sens. 210, 55–68. doi:10.1016/j.isprsjprs.2024.02.021
Umwali, E. D., Kurban, A., Isabwe, A., Mind’je, R., Azadi, H., Guo, Z., et al. (2021). Spatio-seasonal variation of water quality influenced by land use and land cover in Lake Muhazi. Sci. Rep. 11, 17376. doi:10.1038/s41598-021-96633-9
Usali, N., and Hasmadi Ismail, M. (2010). Use of remote sensing and GIS in monitoring water quality. J. Sustain Dev. 3. doi:10.5539/jsd.v3n3p228
Wang, D., Tang, B. H., and Li, Z. L. (2024). Evaluation of five atmospheric correction algorithms for multispectral remote sensing data over plateau lake. Ecol. Inf. 82, 102666. doi:10.1016/j.ecoinf.2024.102666
Wang, M., Li, M., Zhang, Z., Hu, T., He, G., Zhang, Z., et al. (2023). Land surface temperature retrieval from landsat 9 TIRS-2 data using radiance-based split-window algorithm. IEEE J. Sel. Top. Appl. Earth Obs. Remote Sens. 16, 1100–1112. doi:10.1109/JSTARS.2022.3232621
Wang, X., and Yang, W. (2019). Water quality monitoring and evaluation using remote-sensing techniques in China: a systematic review. Ecosyst. Health Sustain. 5, 47–56. doi:10.1080/20964129.2019.1571443
Wen, X., Chen, F., Lin, Y., Zhu, H., Yuan, F., Kuang, D., et al. (2020). Microbial indicators and their use for monitoring drinkingwater quality-A review. Sustain. Switz. 12, 2249. doi:10.3390/su12062249
Wilkinson, G. M., and Johnson, R. A. (2024). “Eutrophication of freshwater and coastal ecosystems,” in Encyclopedia of sustainable technologies (Elsevier), 710–721. doi:10.1016/b978-0-323-90386-8.00099-1
Wu, J., Zeng, S., Yang, L., Ren, Y., and Xia, J. (2021). Spatiotemporal characteristics of the water quality and its multiscale relationship with land use in the yangtze river basin. Remote Sens. (Basel) 13, 3309. doi:10.3390/rs13163309
Wu, Z., Wang, X., Chen, Y., Cai, Y., and Deng, J. (2018). Assessing river water quality using water quality index in Lake Taihu Basin, China. Sci. Total Environ. 612, 914–922. doi:10.1016/j.scitotenv.2017.08.293
Xiong, J., Lin, C., Ma, R., and Cao, Z. (2019). Remote sensing estimation of lake total phosphorus concentration based on MODIS: a case study of Lake Hongze. Remote Sens. (Basel) 11, 2068. doi:10.3390/rs11172068
Xu, H., Gao, Q., and Yuan, B. (2022). Analysis and identification of pollution sources of comprehensive river water quality: evidence from two river basins in China. Ecol. Indic. 135, 108561. doi:10.1016/j.ecolind.2022.108561
Yang, H., Kong, J., Hu, H., Du, Y., Gao, M., and Chen, F. (2022). A review of remote sensing for water quality retrieval: progress and challenges. Remote Sens. (Basel) 14, 1770. doi:10.3390/rs14081770
Yang, Y., and Jin, S. (2023). Long-time water quality variations in the Yangtze River from landsat-8 and sentinel-2 images based on neural networks. WaterSwitzerl. 15, 3802. doi:10.3390/w15213802
Yin, Z., Li, J., Liu, Y., Xie, Y., Zhang, F., Wang, S., et al. (2021). Water clarity changes in Lake Taihu over 36 years based on Landsat TM and OLI observations. Int. J. Appl. Earth Observation Geoinformation 102, 102457. doi:10.1016/j.jag.2021.102457
Zainurin, S. N., Wan Ismail, W. Z., Mahamud, S. N. I., Ismail, I., Jamaludin, J., Ariffin, K. N. Z., et al. (2022). Advancements in monitoring water quality based on various sensing methods: a systematic review. Int. J. Environ. Res. Public Health 19, 14080. doi:10.3390/ijerph192114080
Zainurin, S. N., Wan Ismail, W. Z., Wan Azlan, W. A. N., Zainul Ariffin, K. N., and Wan Ahmad Kamil, W. M. (2023). Developing a portable spectrometer to detect chemical contaminants in irrigation water. Agric. Switz. 13, 1202. doi:10.3390/agriculture13061202
Zhang, H., Yan, D., Zhang, B., Fu, Z., Li, B., and Zhang, S. (2022a). An operational atmospheric correction framework for multi-source medium-high-resolution remote sensing data of China. Remote Sens. (Basel) 14, 5590. doi:10.3390/rs14215590
Zhang, H., Yao, B., Wang, S., and Huang, Y. (2022b). Understanding the changes of optically active substances (OACs) in Hulun Lake in the past 35 years and its indication to the degradation of aquatic ecology. J. Clean. Prod. 377, 134286. doi:10.1016/j.jclepro.2022.134286
Zhang, Y., Zhang, Y., Shi, K., Zhou, Y., and Li, N. (2021). Remote sensing estimation of water clarity for various lakes in China. Water Res. 192, 116844. doi:10.1016/j.watres.2021.116844
Zhang, Z. M., Zhang, F., Du, J. L., and Chen, D. C. (2022c). Surface water quality assessment and contamination source identification using multivariate statistical techniques: a case study of the Nanxi River in the Taihu watershed, China. WaterSwitzerl. 14, 778. doi:10.3390/w14050778
Zhao, Y., Yu, T., Hu, B., Zhang, Z., Liu, Y., Liu, X., et al. (2022). Retrieval of water quality parameters based on near-surface remote sensing and machine learning algorithm. Remote Sens. (Basel) 14, 5305. doi:10.3390/rs14215305
Zhu, S., and Mao, J. (2021). A machine learning approach for estimating the trophic state of urban waters based on remote sensing and environmental factors. Remote Sens. (Basel) 13, 2498. doi:10.3390/rs13132498
Keywords: sustainable development goals, chlorophyll-a, total suspended solids, nitrogen, temperature, water contamination, biochemical and biophysical properties, machine learning algorithms
Citation: Ngamile S, Madonsela S and Kganyago M (2025) Trends in remote sensing of water quality parameters in inland water bodies: a systematic review. Front. Environ. Sci. 13:1549301. doi: 10.3389/fenvs.2025.1549301
Received: 20 December 2024; Accepted: 10 February 2025;
Published: 03 March 2025.
Edited by:
Yong Q. Tian, Central Michigan University, United StatesReviewed by:
Pedzisai Kowe, Midlands State University, ZimbabweCopyright © 2025 Ngamile, Madonsela and Kganyago. This is an open-access article distributed under the terms of the Creative Commons Attribution License (CC BY). The use, distribution or reproduction in other forums is permitted, provided the original author(s) and the copyright owner(s) are credited and that the original publication in this journal is cited, in accordance with accepted academic practice. No use, distribution or reproduction is permitted which does not comply with these terms.
*Correspondence: Mahlatse Kganyago, bWFobGF0c2VrQHVqLmFjLnph
Disclaimer: All claims expressed in this article are solely those of the authors and do not necessarily represent those of their affiliated organizations, or those of the publisher, the editors and the reviewers. Any product that may be evaluated in this article or claim that may be made by its manufacturer is not guaranteed or endorsed by the publisher.
Research integrity at Frontiers
Learn more about the work of our research integrity team to safeguard the quality of each article we publish.