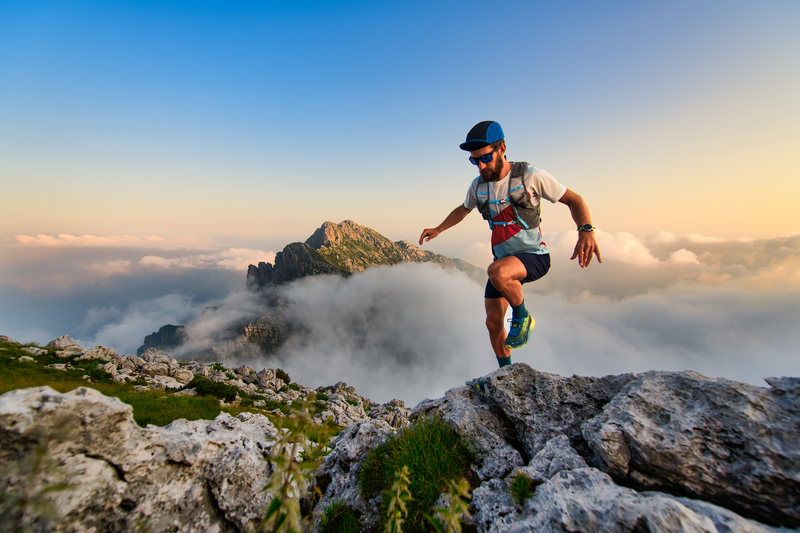
94% of researchers rate our articles as excellent or good
Learn more about the work of our research integrity team to safeguard the quality of each article we publish.
Find out more
ORIGINAL RESEARCH article
Front. Environ. Sci. , 29 January 2025
Sec. Environmental Economics and Management
Volume 13 - 2025 | https://doi.org/10.3389/fenvs.2025.1497121
This article is part of the Research Topic Advancing Carbon Reduction and Pollution Control Policies Management: Theoretical, Application, and Future Impacts View all 31 articles
Introduction: As a major source of pollutant and CO2 emissions, the industrial sector faces the dual challenge of pollution control and carbon reduction. Accurately identifying the synergy between pollutant and carbon emissions in different regions’ industrial sectors is crucial for developing regional policies for coordinated pollution reduction and carbon abatement.
Methods: This study takes Guangzhou as a case study to quantitatively assess the synergistic effect of NOx and CO2 emissions reduction in its industrial sector. First, the LMDI decomposition method was applied to analyze the factors influencing the change in NOx emissions in Guangzhou’s industrial sector. Next, the CFGLS model was used to quantify the synergistic effect between NOx and CO2 emissions. Finally, a robustness check was conducted on the results.
Results and discussion: The findings indicate that the synergistic effect in carbon reduction is the most significant driver of NOx reduction in Guangzhou’s industrial sector, with a 10,000-ton reduction in CO2 emissions leading to a 0.4-ton decrease in NOx emissions. The interaction effect analysis shows that increasing the use of natural gas and reducing energy intensity do not amplify this synergy. The results could provide valuable insights for coordinated pollution reduction and carbon abatement policies designing in Guangzhou’s industrial sector.
The control of pollutants has long been a focal point in environmental concerns. Human energy activities have generated numerous pollutants such as SO2, NOx, PM2.5, etc., causing significant damage to the ecological environment and posing risks to human health (Burnett et al., 2018; Turner et al., 2016). Concurrently, the substantial emission of greenhouse gases has led to global warming, with climate risks becoming increasingly imminent. Thus, measures to control carbon emissions and gradually achieve net-zero emissions are emerging as an international consensus. China is confronted with the dual tasks of pollutant control and carbon reduction. In terms of pollutant control, the State Council of China has issued the “New Action Plan for Pollution Control,” outlining phased targets and implementation roadmaps. Regarding carbon reduction, China made significant commitments during the 75th session of the United Nations General Assembly: China aims to peak its carbon emissions by 2030 and strive for carbon neutrality by 2060. Environmental protection policies and climate actions are crucial topics for realizing China’s green development, with the Chinese government actively seeking pathways to synergistically advance both processes.
There is potential for synergistic emission reductions between CO2 and pollutants, as highlighted in studies such as (He et al., 2010), which quantified the synergistic effects of multiple pollutants. Synergistic governance is considered the most cost-effective approach to reduce both CO2 and pollutant emissions (Xue et al., 2023). Effective synergistic governance requires policies tailored to the specific characteristics of different regions and industries. Previous research has mainly focused on regional or urban levels (Chen et al., 2023; Jia et al., 2023; Shi et al., 2023), with limited studies on specific industries, leading to a lack of localized empirical research for industry-specific emission reduction policies. The industrial sector, as a major source of both pollutants and CO2 emissions, plays a critical role in achieving carbon neutrality. Therefore, quantifying and comprehensively analyzing the synergy between CO2 and pollutant emissions in the industrial sector is essential for formulating effective emission reduction policies.
According to data from the Ministry of Ecology and Environment of China, the NOx emissions from China’s industrial sector in 2022 amounted to 3.333 million tons, accounting for 32.7% of total industrial air pollutant emissions. Research indicates that from 2013 to 2019, the concentrations of PM2.5 and SO2 in 74 key Chinese cities decreased by 47% and 75%, respectively, while NOx concentrations only declined by 23%, indicating limited progress in NOx control (Chu et al., 2022). CO2 and NOx reduction in the industrial sector are key to achieving decarbonization and cleaner production. This paper uses Guangzhou’s industrial sector as a case study, decomposing the factors influencing NOx emissions with an extended Kaya-LMDI model and quantifying the synergistic effect of CO2 and NOx reductions using robust econometric models. It also examines the factors affecting the synergy through an interaction effect model, followed by a robustness check of the results.
This study aims to answer the following questions: 1) What are the factors influencing NOx emissions in Guangzhou’s industrial sector? 2) How can the synergistic effect of CO2 and NOx emission reductions in Guangzhou’s industrial sector be quantified? 3) How do changes in energy structure and energy intensity impact the synergy between CO2 and NOx reductions? The findings of this study will provide localized empirical evidence for coordinated emission reduction policies in Guangzhou’s industrial sector and offer insights for broader policy development in other industrial sectors.
The structure of this paper is outlined as follows: Section 2 reviews relevant studies; Section 3 introduces the research methods and data sources used; Section 4 presents the decomposition results of LMDI, followed by analysis and discussion of the results; Sections 5, 6 discuss the estimation results of synergistic effects and conduct robustness checks; finally, the last section summarizes the research findings and presents corresponding policy suggestions.
The concept of carbon emission reduction synergistic effects was first proposed by the IPCC in 2001. It refers to that GHG emission reductions can simultaneously lead to other socio-economic benefits, the most important part of which is to significantly contribute to synergistic emission reductions of pollutants (Dong et al., 2019). Research by Yi et al. (2023) supports the existence of synergistic effects, as they found that over the past decade, China has achieved the majority of pollutant reduction through coordinated governance efforts. Jia et al. (2024) evaluated the synergistic effects of PM2.5 and CO2 using a synergistic coordinate system and emission reduction elasticity coefficients, finding that synergistic effects exist across all major sectors. Jiao et al. (2020) analyzed the synergistic benefits of CO2 and atmospheric pollutant reduction measures in Guangzhou’s transportation sector using the LEAP model, revealing that promoting the electrification of the transportation sector can achieve the maximum synergistic benefits. Yu et al. (2020) decomposed the factors influencing the synergistic effects of CO2 and pollutant reduction in China’s power sector, indicating that the key to achieving coordinated emission reduction in the power industry lies in adjusting the energy structure and upgrading technologies. Zeng and He (2023) quantified the synergistic emission reduction effects of China’s transportation sector, and based on provincial data, their research showed that every 10,000 tons of CO2 emissions reduced in the transportation sector could lead to a reduction of 11,950 tons of pollutants.
Research on NOx synergistic effects is relatively scarce. Existing studies on NOx synergistic effects are mostly based on model simulations and scenario analyses (Feng et al., 2018; Shi et al., 2024; Yang et al., 2023). These models rely on a series of assumptions and are difficult to comprehensively explain the real world, with results serving only as trend references. Econometric methods and the LMDI method are widely used in NOx emission studies. Wang et al. (2019) used a geographically weighted regression model to analyze the driving factors of NOx emissions from energy consumption in 30 provinces of China, revealing significant north-south differences influenced by economic development and energy intensity. Ding et al. (2017) employed the LMDI method to analyze the driving factors of NOx emissions in provinces of China and regional challenges in emission reduction, finding that improvements in energy efficiency and technological advancements are the main drivers of emission reductions and regional controls on NOx would be more effective. In addition to national-level studies, some scholars have conducted in-depth studies on NOx emissions in individual regions (Xu et al., 2020; Zhang et al., 2015), while research on NOx emissions in more finely segmented sectors and industries is relatively scarce.
Research also indicates that appropriate policies can enhance the synergistic effect of CO2 and pollutant emission reductions. Bollen et al. (2009) used the integrated assessment model MERGE to simulate the synergistic effects of air pollutant and greenhouse gas emissions reductions, finding that some long-term climate change strategies simultaneously improve air quality in the short term. Plachinski et al. (2014) found that low-carbon policies in the U.S. power sector have synergistic effects in reducing PM2.5 emissions. Also, similar results were obtained in studies by Bollen and Brink (2014) and Alam et al. (2018), which used local data from the EU and Ireland, respectively. In terms of research of China, Yang et al. (2017) pointed out that, under China’s carbon peaking and carbon neutrality scenarios, pollutant emissions will be significantly reduced. Yang et al. (2017), highlighted the significant potential for CO2 and pollutant co-reduction in China’s industrial sector based on large-scale industrial enterprise data. However, the key to formulating appropriate synergistic emission reduction policies for the industrial sector lies in the rigorous quantification and empirical analysis of the synergistic effects. As specific targeted research is still limited this paper aims to expand existing insights in this area.
After summarizing existing literature, it is found that most studies focus on the synergistic effects between PM2.5 and SO2, few studies focus on the synergistic effects of NOx and CO2 reduction. Second, studies on synergistic effects are mainly conducted at the provincial or national level, lacking research on individual sectors. Third, research on synergistic effects of NOx and CO2 reduction in the industrial sector is limited, while the industrial sector serves as a major source of CO2 and NOx emissions. Fourth, research methods for synergistic effects are mostly based on model simulations and scenario analyses, while empirical and quantitative analyses are scarce.
Apart from previous studies, the main contributions of this paper are as follows: 1) Based on extended Kaya model and LMDI method, this paper decomposes the influencing factors of NOx emissions variation in the industrial sector of Guangzhou City and identifies the presence of synergistic effects; 2) Employing rigorous econometric models, this paper quantifies the synergistic effects of carbon reduction and NOx, deriving empirically-based conclusions and circumventing the drawbacks associated with excessive assumption; 3) This paper identifies the interaction between synergistic effects and factors such as energy structure and energy intensity, which can offer policy insights for effectively leveraging synergistic effects of carbon and NOx reduction.
LMDI method is a technique used to decompose changes in energy or carbon emissions (Ang, 2005). It decomposes overall changes into contributions from individual factors based on the logarithmic mean of the Divisia index. The advantage of this method lies in its ability to handle zero values and absence of residuals. Building upon the Kaya identity, we extend the relationship between NOx emissions and CO2 emissions, energy structure, industrial output, etc. In this expanded framework, the LMDI method can be meaningfully applied to decompose NOx emission factors.
The NOx emissions from the industrial sector of Guangzhou City can be decomposed into the following factors in Equation 1:
Here, i represents the i-th industry in industrial sector, and t represents the t-th year.
Let
Through further decomposition using the logarithmic index method, we can obtain the following Equations 3–6.
As derived above, the NOx emissions from the industrial sector of Guangzhou City can be decomposed into four parts:
To further quantify the synergistic effects between CO2 and NOx, we established an econometric model for NOx reduction. Building upon the decomposition results of the LMDI, this model takes the NOx reduction quantity as the dependent variable and the CO2 reduction quantity as the core explanatory variable, with the estimated coefficient
where i represents the i-th industry and t represents the year.
The fixed effects model employed in this study is based on the following statistical assumptions regarding the properties of the error term.
1) The error term has a zero mean and is uncorrelated with the explanatory variables, individual fixed effects, and time fixed effects:
The basic data used in this study primarily come from the Guangzhou Statistical Yearbook from 2011 to 2022. Based on the national economic industry classification of China, we subdivide Guangzhou’s industrial sector into ten main industries: Power (PWR), Petrochemicals (PET), Textiles (TEX), Paper and Printing (P&P), Biopharmaceuticals (BioPhar), Iron, Equipment Manufacturing (EM), Information Technology (IT), Building Materials (BM), Other Industries. The CO2 emissions of each industry were calculated according to the following Equation 8.
where: E represents the total CO2 emissions of the industry,
The fuel consumption data is sourced from the energy consumption data provided in the Guangzhou Statistical Yearbook, while the carbon emission factors are sourced from the “Guidelines for Compilation of Greenhouse Gas Inventories for Cities and Counties (Districts) in Guangdong Province (Trial),” respectively.
The formula for calculating the dependent variable, NOx reduction, in the econometric model is as follows in Equation 9.
Here,
The formula for the core explanatory variable, CO2 reduction, is as follows in Equation 10:
where
The summary of all variables is presented in Table 2. The descriptive statistics of variables are presented in Table 3.
Since the “12th Five-Year Plan” period, Guangzhou has actively engaged in pollution reduction and carbon reduction efforts, improving its energy structure and optimizing industrial layout through measures such as technological improvement, energy substitution, and eliminating outdated production capacity. By 2022, the energy intensity of Guangzhou’s industrial sector had decreased by 55% compared to 2011, while the CO2 and NOx emissions reductions reached 26.063 million tons and 63,949 tons, respectively, representing a decrease of 29.5% and 85.4% compared to 2011.
With LMDI method, we decomposed the annual changes in NOx emissions in Guangzhou’s industrial sector from 2011 to 2022 into four influencing factors: CO2 synergistic reduction effect (CSE), energy structure effect (ES), energy intensity effect (EI), and industrial output effect (IVA), with “Total” representing the total change in NOx emissions for that year. The specific decomposition results are shown in Figure 1.
As shown in Figure 1, the NOx emissions from Guangzhou’s industrial sector exhibits an overall downward trend from 2011 to 2019, with the most significant decrease in 2016. There is a slight increase in 2021, followed by a continued decrease in 2022. As the main factor contributing to the increase in NOx emission, the industrial output effect has a consistent positive impact on NOx emissions, indicating that the expansion of production scale leads to a corresponding increase in emissions of pollutants such as NOx.
The main contribution to NOx reduction comes from the synergistic effect of carbon reduction, with an average annual carbon reduction synergy effect of 4,650 tons from 2011 to 2022, indicating significant potential for NOx reduction through carbon synergy. The energy intensity effect contributes an average of 3,025 tons per year to NOx reduction, second only to the carbon reduction synergy effect. Years with negative energy intensity effects are accompanied by a decrease in energy intensity, indicating that improvements in energy efficiency could effectively reduce fossil fuel consumption so that promote NOx reduction. Compared to other effects, the energy structure effect is not significant, suggesting that overall changes in energy carbon emission factors in Guangzhou’s industrial sector are not pronounced, indicating significant potential for the industrial sector’s energy clean-up.
In summary, the synergistic effect of carbon reduction, energy intensity effect, and industrial output effect have a significant impact on NOx reduction in Guangzhou’s industrial sector, while the energy structure effect has a weaker influence. The industrial output effect has a positive impact, leading to a noticeable increase in NOx emissions with an increase in industrial output. The synergistic effect of carbon reduction and the energy intensity effect contribute mainly to negative effects. Among all influencing factors, the contribution of the synergistic effect of carbon reduction to NOx reduction is the largest, making it the most important pathway for NOx reduction.
Figure 2 illustrates the decomposition results of NOx emission changes in the 10 industry categories of Guangzhou’s industrial sector. The total emission change is obtained by subtracting the NOx emissions in 2022 from those in 2011 for each industry, representing the total emission change from 2011 to 2022. Due to the significant disparity in emission levels between the electricity industry and other industries, the decomposition results are presented as the contribution of each influencing factor to the absolute value of the total effect. The specific calculation method is described in Equation 11.
Consistent with the analysis in Section 4.1, we decomposed the NOx emission changes in each industry into the sum of four influencing factors. They are the CO2 synergistic emission reduction effect (CSE), energy structure effect (ES), energy intensity effect (EI), and industrial output effect (IVA).
From Figure 2, it is evident that the synergistic effect of carbon emissions contributes the most to NOx reduction in each industry, indicating that the synergistic effect of carbon reduction is an important pathway for NOx reduction in the industrial sector. Additionally, the contribution of the CO2 reduction synergistic effect to NOx reduction varies significantly across different industries. The power industry exhibits the highest one, with the synergic effect accounting for a reduction of 41,111 tons of NO, and followed by the building materials and petrochemical industries, with contributions of 5,373.3 tons and 3,972.5 tons, respectively. The contribution of energy intensity effect to NOx reduction is second only to the synergistic effect of carbon reduction, and technological progress and improved energy efficiency in each industry significantly promote NOx reduction. The energy intensity effect in the iron industry is positive, which is related to the increase in energy intensity in the iron industry from 2011 to 2022. The iron industry should actively conduct technological research and development, optimize production processes, and reduce energy intensity to improve economic benefits while reducing emissions of pollutants such as NOx. The industrial output effect has a significant positive impact on NOx emissions, indicating that the expansion of production scale leads to a corresponding increase in emissions of pollutants such as NOx. The industrial sector should further transform its current production methods towards sustainable production, introducing negative carbon technologies and pollution control technologies to address environmental externalities during the production process.
Compared to other effects, the energy structure effect is not significant. Except for power and iron, the energy structure effect in other industries is positive, indicating that these industries need to quickly transition their energy structure to increase the proportion of clean fuels, achieving the goal of synergistic carbon reduction and pollution reduction.
In summary, carbon emission synergistic effect and energy intensity effect serve as significant means and pathways for NOx synergistic reduction in Guangzhou’s industrial sectors. All industries should take proactive measures to reduce CO2 emissions while enhancing technological innovation to improve energy efficiency, thereby effectively leveraging energy intensity effects to facilitate the reduction of pollutants such as NOx. There is ample room for optimizing the energy structure across various industrial sectors in Guangzhou. Continuous efforts should be made to increase the proportion of high-quality clean energy usage and explore potential fuel substitutions to lower energy emission intensity factors, transitioning towards a sustainable energy structure.
The variables included in the econometric model (7) may be non-stationary sequences, which can lead to “pseudo-regression.” Therefore, before conducting regression analysis, we perform unit root tests on the variables involved in the econometric model. Considering the relatively small panel sample size, we employ the IPS test. The results of the IPS test are presented in Table 4.
The results indicate that some variables are non-stationary at the level, but the first-difference series of all variables reject the null hypothesis of having a unit root at least at the 0.05 significance level. Therefore, all variables are first-order integrated. To determine the potential cointegration relationships, we conduct the Kao cointegration test between the explanatory and dependent variables. The results of the Kao cointegration test are presented in Table 5.
The results of the Kao cointegration test strongly reject the null hypothesis that there is no cointegration relationship between the dependent and explanatory variables. There exists a “long-term equilibrium” relationship among the variables, allowing for regression analysis using the original variable sequences. Furthermore, to address the possibility of multicollinearity among the variables, we computed the VIF statistic. The results in Table 6 show that all VIF, values are below 10, indicating that multicollinearity is not a concern among the variables (Hair et al., 2009).
Moreover, to ensure the effectiveness of the fixed effects model we employed, a Hausman test is conducted to select the appropriate estimation model. The results of the Hausman test are presented in Table 7. The test results strongly reject the null hypothesis, indicating that in this study, the fixed effects model is more suitable than the random effects model.
To employ the most appropriate estimation methods, we conduct tests for between-group heteroskedasticity, within-group autocorrelation, and between-group contemporaneous correlation on the panel data. The test results are presented in Table 8. The Modified Wald test results strongly reject the null hypothesis of “homoskedasticity,” indicating the presence of between-group heteroskedasticity in the panel data.
We perform the Wooldridge test for within-group autocorrelation, and the results indicate that the null hypothesis of “no first-order within-group autocorrelation” cannot be rejected, suggesting no issue of within-group autocorrelation in the panel data. Additionally, we conduct Pesaran’s test and Friedman’s test for between-group contemporaneous correlation, and both tests strongly reject the null hypothesis of “no between-group contemporaneous correlation,” indicating the need to consider the issue of between-group contemporaneous correlation.
Table 8 results indicate that there is between-group heteroskedasticity and between-group contemporaneous correlation in the panel data of Guangzhou’s industrial sectors. Thus, appropriate estimation methods are required to estimate the fixed-effects model. In this case, the OLS method cannot provide consistent estimates, and instead, the FGLS method is needed. Specifically, the FGLS can only handle within-group autocorrelation, whereas the comprehensive FGLS (CFGLS) estimation can simultaneously address issues of between-group heteroskedasticity, within-group autocorrelation, and between-group contemporaneous correlation. We employ the CFGLS model to estimate the synergistic effects between CO2 and NOx emission. Additionally, for comparison, we will report the results of both FE, FGLS and CFGLS methods.
The estimation results of the CFGLS model, which effectively addresses between-group heteroskedasticity and between-group contemporaneous correlation, indicate significant synergistic effects between CO2 and NOx emission reduction. As shown in Model (3) of Table 9, the estimated coefficient of the core explanatory variable CMR (CO2 emission reduction) on the dependent variable NMR is 0.0004, significant at the 0.001 level. It is noteworthy that the variables used in the model are measured in CMR (in ten thousand tons) and NMR (in thousand tons), implying that each ten thousand tons of CO2 reduction results in a 0.4-ton reduction in NOx emissions. The coefficient of ES is significantly positive, which indicates that actively improving the energy structure and increasing the proportion of natural gas usage is one of the ways to reduce NOx emissions. Furthermore, the estimated coefficient of the variable EI, representing energy intensity, is significantly negative, indicating that a reduction in energy intensity significantly promotes NOx reduction, consistent with the decomposition results of LMDI. The improvement in energy efficiency brought about by technological progress is an important way to promote NOx reduction. The coefficient of the first-order term of industrial value-added is significantly positive, while the coefficient of the second-order term is significantly negative, indicating a reverse U-shaped relationship between industrial value-added and NOx reduction, with the potential for NOx reduction increasing first and then decreasing with the development of industrial economy.
To explore the differences in synergistic effects among different industries, we introduced interaction terms between industry dummy variables and CMR, as shown in Model (4) of Table 9. The interaction term between the dummy variable for the Power industry and CMR is significantly negative, indicating that the synergistic emission reduction potential of the Power industry is significantly greater than that of other industries.
To further investigate the factors influencing the synergistic effects of CO2 and NOx reduction, the interaction effects between CMR and other variables are analyzed. In Model (5), the interaction term between variable ES and CMR is added. It is found that the coefficient of the interaction term is positive but close to zero. This suggests that adjusting the energy structure to increase the proportion of natural gas consumption does not significantly promote synergistic effects.
In Model (6), the interaction term between variable EI and CO2redu is added. The results show that the coefficient of the interaction term is significantly positive, indicating that a decrease in energy intensity does not promote synergistic effects; instead, it weakens them. According to the energy rebound effect, a decrease in energy intensity improves energy efficiency, leading to a decrease in the effective price of energy services. The lower price stimulates more energy demand, which may result in increased energy consumption and emissions of pollutants such as NOx.
The core explanatory variable CMR may suffer from endogeneity issues. To address potential endogeneity concerns, we lagged the core explanatory variable by one period and conduct the regression again. The lagged variable LCMR represents the CO2 emission reduction from the previous period, which cannot affect the current period’s NOx emission reduction and is uncorrelated with the disturbances of the current period. The regression results, as shown in Model (1) of Table 10, still exhibit significantly positive coefficient estimates for the core explanatory variable, indicating a significant synergistic effect between CO2 and NOx reduction. The regression results for other variables are also similar to the baseline results, which confirm the robustness of the baseline results.
Due to the impact of COVID-19, the Chinese government implemented strict measures to restrict outdoor activities and industrial production in 2020, leading to a sharp decrease in CO2 emissions (Liang et al., 2023). Research from Nanchang, China, also indicates that compared to the same period in 2019, the pandemic in 2020 resulted in a 2% reduction in CO2 emissions, with reductions of 54.5% and 18.9% in the power and manufacturing industries, respectively. Furthermore, CO2 emissions in 2021 increased by 14.3%–14.9% compared to 2019, indicating a rapid recovery of economic activities to pre-pandemic levels (Hu et al., 2022). The “unexpected” decline in emissions from industrial activities in 2020 due to the pandemic may interfere with the research results. To address this, we exclude the data from 2020 and conduct the regression again to verify the robustness of the results. Since industrial activities and emission levels in 2021 have essentially returned to pre-pandemic levels, data from that year are not excluded. The regression results, as shown in Model (2) of Table 10, indicate that the estimated coefficient for the core explanatory variable CMR remains significantly positive, and other coefficients are similar to the baseline regression results, demonstrating the robustness of our baseline regression results.
Pollutant emissions and carbon emissions share the same origin, promoting synergies in reducing pollution and carbon emission has become an inevitable choice to facilitate the comprehensive green transformation of China’s economic and social development. This paper, focusing on the industrial sector of Guangzhou City, employs LMDI method to decompose the change in NOx emissions into the effects of four influencing factors. Additionally, a two-way fixed effects model is employed to quantify the synergistic reduction effects between CO2 and NOx.
The LMDI decomposition results indicate the following: 1) The largest driver of NOx reduction in Guangzhou’s industrial sector is the synergistic reduction effect resulting from CO2 mitigation, followed by the emission reduction effect due to decreased energy intensity. 2) Economic growth in the industrial sector is the primary driver of increased NOx emissions. 3) All industries within the industrial sector exhibit significant synergistic reduction effects between CO2 and NOx, with the power industry having the highest synergistic reduction effect.
Based on the LMDI decomposition results, the CFGLS model was used to quantify the synergistic reduction effects between CO2 and NOx in Guangzhou’s industrial sector. The key conclusions are as follows: 1) The synergistic reduction effect between CO2 and NOx is significant at the 0.01 level, with a reduction of 10,000 tons of CO2 leading to a reduction of 0.4 tons of NOx. 2) Increasing the proportion of natural gas usage can effectively promote NOx reduction. 3) There is an inverted U-shaped curve between NOx reduction and industrial added value, indicating that NOx reduction potential first increases and then decreases with industrial economic development. 4) The study of interaction effects shows that the synergistic carbon reduction effect in the power industry is higher than in other industries, highlighting the need to prioritize synergistic reduction in power industry.
This paper identifies the influencing factors of NOx emissions in Guangzhou’s industrial sector and quantifies the synergistic reduction effects between NOx and CO2. It can provide valuable insights into achieving joint NOx and CO2 reductions through synergistic effects in the industrial sector.
This study decomposes the factors influencing NOx emissions in Guangzhou’s industrial sector, quantifies the synergistic emission reduction effects between NOx and CO2, and analyzes the impact of changes in energy intensity and energy structure on this synergy, providing comprehensive and robust conclusions. However, several limitations should be acknowledged. Due to data availability, the panel data used in this study covers the period from 2011 to 2022. Future research could expand the time span by incorporating additional data sources, thereby providing results based on a larger sample. This study focuses only on Guangzhou’s industrial sector, future research could integrate data from multiple regions to provide more generalizable empirical conclusions. While this study offers a thorough analysis of the synergistic effects, it does not delve into specific synergistic emission reduction measures, and future studies could explore this aspect further.
The original contributions presented in the study are included in the article/Supplementary Material, further inquiries can be directed to the corresponding author.
XQ: Data curation, Formal Analysis, Investigation, Methodology, Software, Validation, Visualization, Writing–original draft. PX: Conceptualization, Funding acquisition, Investigation, Methodology, Project administration, Resources, Supervision, Writing–review and editing. CL: Conceptualization, Resources, Supervision, Writing–review and editing.
The author(s) declare that financial support was received for the research, authorship, and/or publication of this article. This study was supported by funding from the Rockefeller Brother Foundation (Grant No. 23-246).
The authors declare that the research was conducted in the absence of any commercial or financial relationships that could be construed as a potential conflict of interest.
All claims expressed in this article are solely those of the authors and do not necessarily represent those of their affiliated organizations, or those of the publisher, the editors and the reviewers. Any product that may be evaluated in this article, or claim that may be made by its manufacturer, is not guaranteed or endorsed by the publisher.
The Supplementary Material for this article can be found online at: https://www.frontiersin.org/articles/10.3389/fenvs.2025.1497121/full#supplementary-material
Alam, Md.S., Hyde, B., Duffy, P., and McNabola, A. (2018). Analysing the Co-Benefits of transport fleet and fuel policies in reducing PM2.5 and CO2 emissions. J. Clean. Prod. 172, 623–634. doi:10.1016/j.jclepro.2017.10.169
Ang, B. W. (2005). The LMDI approach to decomposition analysis: a practical guide. Energy Policy 33, 867–871. doi:10.1016/j.enpol.2003.10.010
Bollen, J., and Brink, C. (2014). Air pollution policy in Europe: quantifying the interaction with greenhouse gases and climate change policies. Energy Econ. 46, 202–215. doi:10.1016/j.eneco.2014.08.028
Bollen, J., van der Zwaan, B., Brink, C., and Eerens, H. (2009). Local air pollution and global climate change: a combined cost-benefit analysis. Resour. Energy Econ. 31, 161–181. doi:10.1016/j.reseneeco.2009.03.001
Burnett, R., Chen, H., Szyszkowicz, M., Fann, N., Hubbell, B., Pope, C. A., et al. (2018). Global estimates of mortality associated with long-term exposure to outdoor fine particulate matter. Proc. Natl. Acad. Sci. 115, 9592–9597. doi:10.1073/pnas.1803222115
Chen, S., Tan, Z., Wang, J., Zhang, L., He, X., and Mu, S. (2023). Spatial and temporal evolution of synergizing the reduction of pollution and carbon emissions and examination on comprehensive pilot effects–evidence from the national eco-industrial demonstration parks in China. Environ. Impact Assess. Rev. 101, 107147. doi:10.1016/j.eiar.2023.107147
Chu, B., Ding, Y., Gao, X., Li, J., Zhu, T., Yu, Y., et al. (2022). Coordinated control of fine-Particle and Ozone pollution by the substantial reduction of nitrogen oxides. Engineering 15, 13–16. doi:10.1016/j.eng.2021.09.006
Ding, L., Liu, C., Chen, K., Huang, Y., and Diao, B. (2017). Atmospheric pollution reduction effect and regional predicament: an empirical analysis based on the Chinese provincial NO x emissions. J. Environ. Manage. 196, 178–187. doi:10.1016/j.jenvman.2017.03.016
Dong, F., Yu, B., and Pan, Y. (2019). Examining the synergistic effect of CO2 emissions on PM2.5 emissions reduction: evidence from China. J. Clean. Prod. 223, 759–771. doi:10.1016/j.jclepro.2019.03.152
Feng, X. Z., Lugovoy, O., and Qin, H. (2018). Co-controlling CO2 and NOx emission in China’s cement industry: An optimaldevelopment pathway study. Adv. Clim. Change Res. 9, 34–42. doi:10.1016/j.accre.2018.02.004
Hair, J., Black, W. C., Babin, B. J., and Anderson, R. E. (2009). Multivariate data analysis. 7th Edn. Prentice-Hall.
He, K., Lei, Y., Pan, X., Zhang, Y., Zhang, Q., and Chen, D. (2010). Co-benefits from energy policies in China. Energy, Energy Sustain. Dev. China 35, 4265–4272. doi:10.1016/j.energy.2008.07.021
Hu, C., Griffis, T. J., Xia, L., Xiao, W., Liu, C., Xiao, Q., et al. (2022). Anthropogenic CO2 emission reduction during the COVID-19 pandemic in Nanchang City, China. Environ. Pollut. 309, 119767. doi:10.1016/j.envpol.2022.119767
Jia, W., Li, L., Lei, Y., and Wu, S. (2023). Synergistic effect of CO2 and PM2.5 emissions from coal consumption and the impacts on health effects. J. Environ. Manage. 325, 116535. doi:10.1016/j.jenvman.2022.116535
Jia, W., Li, L., Zhu, L., Lei, Y., Wu, S., and Dong, Z. (2024). The synergistic effects of PM2.5 and CO2 from China’s energy consumption. Sci. Total Environ. 908, 168121. doi:10.1016/j.scitotenv.2023.168121
Jiao, J., Huang, Y., and Liao, C. (2020). Co-benefits of reducing CO2 and air pollutant emissions in the urban transport sector: a case of Guangzhou. Energy Sustain. Dev. 59, 131–143. doi:10.1016/j.esd.2020.10.005
Liang, M., Zhang, Y., Ma, Q., Yu, D., Chen, X., and Cohen, J. B. (2023). Dramatic decline of observed atmospheric CO2 and CH4 during the COVID-19 lockdown over the Yangtze River Delta of China. J. Environ. Sci. 124, 712–722. doi:10.1016/j.jes.2021.09.034
Plachinski, S. D., Holloway, T., Meier, P. J., Nemet, G. F., Rrushaj, A., Oberman, J. T., et al. (2014). Quantifying the emissions and air quality co-benefits of lower-carbon electricity production. Atmos. Environ. 94, 180–191. doi:10.1016/j.atmosenv.2014.03.028
Shi, Q., Liang, Q., Huo, T., You, K., and Cai, W. (2023). Evaluation of CO2 and SO2 synergistic emission reduction: the case of China. J. Clean. Prod. 433, 139784. doi:10.1016/j.jclepro.2023.139784
Shi, X., Huang, Z., Dai, Y., Du, W., and Cheng, J. (2024). Evaluating emission reduction potential and co-benefits of CO2 and air pollutants from mobile sources: a case study in Shanghai, China. Resour. Conserv. Recycl. 202, 107347
Turner, M. C., Jerrett, M., Pope, C. A., Krewski, D., Gapstur, S. M., Diver, W. R., et al. (2016). Long-term ozone exposure and mortality in a large prospective study. Am. J. Respir. Crit. Care Med. 193, 1134–1142. doi:10.1164/rccm.201508-1633OC
Wang, J., Ma, Y., Qiu, Y., Liu, L., and Dong, Z. (2019). Spatially differentiated effects of socioeconomic factors on China’s NOx generation from energy consumption: implications for mitigation policy. J. Environ. Manage. 250, 109417. doi:10.1016/j.jenvman.2019.109417
Xu, X., Huang, G., Liu, L., Guan, Y., Zhai, M., and Li, Y. (2020). Revealing dynamic impacts of socioeconomic factors on air pollution changes in Guangdong Province, China. Sci. Total Environ. 699, 134178. doi:10.1016/j.scitotenv.2019.134178
Xue, W., Lei, Y., Liu, X., Shi, X., Liu, Z., Xu, Y., et al. (2023). Synergistic assessment of air pollution and carbon emissions from the economic perspective in China. Sci. Total Environ. 858, 159736. doi:10.1016/j.scitotenv.2022.159736
Yang, X., Teng, F., Wang, X., and Zhang, Q. (2017). “System optimization and Co-benefit analysis of China’s deep de-carbonization effort towards its INDC target,” in Energy Procedia, 8th Int. Conf. Appl. Energy, ICAE2016, Beijing, China, October 8–11, 2016, 105, 3314–3319. doi:10.1016/j.egypro.2017.03.754
Yi, M., Guan, Y., Wu, T., Wen, L., and Sheng, M. S. (2023). Assessing China’s synergistic governance of emission reduction between pollutants and CO2. Environ. Impact Assess. Rev. 102, 107196. doi:10.1016/j.eiar.2023.107196
Yu, Y., Jin, Z., Li, J., and Jia, L. (2020). Low-carbon development path research on China’s power industry based on synergistic emission reduction between CO2 and air pollutants. J. Clean. Prod. 275, 123097. doi:10.1016/j.jclepro.2020.123097
Zeng, Q.-H., and He, L.-Y. (2023). Study on the synergistic effect of air pollution prevention and carbon emission reduction in the context of “dual carbon”: evidence from China’s transport sector. Energy Policy 173, 113370. doi:10.1016/j.enpol.2022.113370
Zhang, Y., Wang, H., Liang, S., Xu, M., Zhang, Q., Zhao, H., et al. (2015). A dual strategy for controlling energy consumption and air pollution in China’s metropolis of Beijing. Energy 81, 294–303. doi:10.1016/j.energy.2014.12.041
Keywords: synergistic emission reduction, CO2 emission, NOx emission, industrial sector, CFGLS model
Citation: Qin X, Xie P and Liao C (2025) Study on the synergistic effect of NOx and CO2 emission reduction in the industrial sector of Guangzhou. Front. Environ. Sci. 13:1497121. doi: 10.3389/fenvs.2025.1497121
Received: 16 September 2024; Accepted: 02 January 2025;
Published: 29 January 2025.
Edited by:
Jiachao Peng, Wuhan Institute of Technology, ChinaCopyright © 2025 Qin, Xie and Liao. This is an open-access article distributed under the terms of the Creative Commons Attribution License (CC BY). The use, distribution or reproduction in other forums is permitted, provided the original author(s) and the copyright owner(s) are credited and that the original publication in this journal is cited, in accordance with accepted academic practice. No use, distribution or reproduction is permitted which does not comply with these terms.
*Correspondence: Pengcheng Xie, eGllcGNAbXMuZ2llYy5hYy5jbg==
Disclaimer: All claims expressed in this article are solely those of the authors and do not necessarily represent those of their affiliated organizations, or those of the publisher, the editors and the reviewers. Any product that may be evaluated in this article or claim that may be made by its manufacturer is not guaranteed or endorsed by the publisher.
Research integrity at Frontiers
Learn more about the work of our research integrity team to safeguard the quality of each article we publish.