- 1School of Economics and Management, Suqian University, Suqian, China
- 2School of Civil Engineering and Architecture, Suqian University, Suqian, China
Introduction: Measuring the coupling coordination degree between the innovation factor allocation system and the green economy development system, and deeply exploring the changing trends in the coupling coordination between innovation factors allocation, including their subsystems, and green economy development, are essential prerequisites for achieving a high degree of coupling coordination between the two systems.
Methods: This paper, based on panel data from 30 provincial regions in China from 2013 to 2022, measures the coupling coordination degree between innovation factor allocation and green economy development, including their subsystems, and explores the spatial differentiation characteristics of this coupling coordination across the provinces in China and its three major regions. First, an evaluation index system for the innovation factor allocation system and the green economy development system is constructed from both input and output perspectives. The entropy weighted TOPSIS method and the coupling coordination degree model are then employed to explore the temporal characteristics of the coupling coordination between innovation factor allocation and green economy development. Second, Dagum's Gini coefficient is used to reveal the directional distribution of coupling coordination and the sources of spatial disparities. Third, kernel density estimation and spatial Markov chain methods are applied to uncover the dynamic evolution of this coupling coordination.
Results and discussion: The study finds that the overall coupling coordination degree between innovation factor allocation and green economy development in China is relatively low, but it exhibits a continuous upward trend. From the perspective of regional disparities, the coupling level between innovation factor allocation and green economy development across Chinese provinces shows an imbalanced spatial distribution, characterized by higher levels in the east and lower levels in the west, with spatial disparities tending to widen. Inter-regional differences are the primary source of these spatial disparities, followed by intra-regional differences. While internal disparities within Eastern China are narrowing, they are expanding within the Central and Western China. The development processes in these regions are often influenced by neighboring spatial spillover effects. Finally, recommendations are proposed to enhance the coupling coordination degree between innovation factor allocation and green economy development in China.
1 Introduction
As industrial value-added increases, the deterioration of the natural environment also escalates (Mikhno et al., 2021), further impacting long-term economic development (Sun et al., 2024). To achieve sustainable economic development, it is essential to balance growth within the constraints of ecological carrying capacity and resource limitations (Fang et al., 2020). An increasing number of governments in developing countries are now focusing on developing a green economy, particularly in the context of the Paris Agreement on climate change. Many countries have also formulated comprehensive national green growth strategies to establish a green economy (Lutz et al., 2017). Originally, “green growth” was a term applied solely to the growth of ecological industries, but the concept has now been expanded to describe the growth of entire economies (Jänicke, 2012). From an environmental protection perspective, developing a green economy helps guide traditional industrial societies toward an energy revolution in various sectors, including production, distribution, and consumption, transforming a nation’s extensive development model characterized by “high energy consumption, high pollution, and high emissions.” From an economic perspective, the “green transition” can effectively promote the process of “structural adjustment and stable growth” (He et al., 2019). The trend toward green economic development is a key factor in promoting economic and social prosperity (Maria et al., 2015).
The concept of a green economy stems from the principles of sustainable development, with technological innovation serving as a critical pillar (Söderholm, 2020; Abid et al., 2022). Innovation is a key driver of green economic development (Zhang et al., 2024). Technological innovation can promote green economy development through three key pathways: enhancing technological efficiency, reducing pollution emissions, and upgrading industrial structures (Ding et al., 2024). The relationship between innovation factors and green economy development is complex, involving both direct and indirect causal mechanisms. Technological innovation plays a pivotal role in reducing emissions and promoting energy efficiency, thereby positively influencing green economic growth (Khan et al., 2022). Human innovation factors, such as education and R&D personnel, are critical contributors to the adoption of green technologies and the implementation of sustainable industrial practices (Gao et al., 2023). Institutional innovation, including environmental regulations and policy frameworks, has also been shown to promote green development by providing the necessary incentives and structural support (Mu et al., 2024). By optimizing the allocation of human, capital, and institutional innovation factors, regions can significantly enhance their green economic development, as demonstrated by empirical studies conducted in China and other rapidly developing economies. These findings establish a robust causal link between innovation factor allocation and green economy development.
Transitioning to a green economy is a pressing challenge for China as it shifts from rapid economic growth to high-quality development (Liu et al., 2021; Xu and Ding, 2024). While traditional technological innovations have spurred rapid progress in China’s economy, they have also inflicted harm on the shared environment (Ke et al., 2022; Li et al., 2024). Currently, China has entered a new era of high-quality development, but issues of imbalanced and inadequate development persist due to structural imbalances in the allocation of innovation factors (Fan et al., 2021).
Understanding the current alignment between innovation factor allocation and green economy development, optimizing the allocation of innovation factors, and promoting green economic growth are critical issues that need urgent attention. This raises important questions: How well are innovation factor allocation and green economic development aligned? What are the trends in their development? Are there regional disparities? To address these questions, this paper measures the coupling coordination degree between the innovation factor allocation system and the green economy development system, and conducts an in-depth analysis of the trends in the coupling coordination of various subsystems of innovation factors, as well as the overall system with green economic development. These insights will help the government reassess the relationship between innovation factor allocation and green economy development, enabling further optimization of innovation factor allocation, promoting green economic growth, and providing a practical basis for guiding policy direction.
2 Literature review
The distinctions in measuring innovation factor allocation in existing literature primarily lie in the differences in measurement subjects, perspectives, and methods (Zhao, 2024). The level of innovation factor allocation is mainly calculated by the comprehensive method of indicators, such as data envelopment analysis method, entropy weight method, TOPSIS method and random forest method (Xu et al., 2022; Liu et al., 2024; Xu et al., 2024). Chang et al. (2024) constructed a comprehensive index of innovation factor allocation containing four dimensions of human, knowledge, technology and institutional innovation, and used the entropy weight TOPSIS method to calculate the level of innovation factor allocation according to the objective assignment method. Liu et al. (2024) measured the efficiency of innovation factor allocation using the super-efficient SMB-DEA model with non-desired outputs.
Existing studies focusing on the measurement of green economy development have concentrated on two types of literature. The first type of literature is devoted to the study of factors affecting the level of green economy development (Kortelainen, 2008; Fernández et al., 2011; Chi et al., 2014; Chen et al., 2018; Kang et al., 2020). The second type of literature measures the combined level of green development systems and economic development systems by selecting different indicators (Pan et al., 2022; Herman et al., 2023; Liang et al., 2023; Yang et al., 2023a).
Existing studies focusing on the relationship between innovation factor allocation and green economy development can be categorized into three types. The first is the impact of innovation factor allocation on green economy development. Optimizing the allocation of innovation factors promotes economic development (Kogan et al., 2017; Costa Cavalcante, 2024), while mismatch of innovation factors inhibits economic development (Hsieh and Klenow, 2009; Yang et al., 2022b; Zhang et al., 2023; Wang et al., 2023). In this process, the connotation of innovation factors extends from general labor and capital to technology, data, and institutions (McGrattan and Prescott, 2009; Innocenti et al., 2020; Jones and Tonetti, 2020; Peng and Tao, 2022; Yu et al., 2023; Zhao, 2024). The second is the impact of green economy development on innovation factor allocation. Green economy development has a significant impact on the synergistic development of regional green innovation systems (Ying et al., 2021). Regions with higher levels of economic development can provide abundant human capital, foreign investment, R&D investment and other material conditions for innovation (Filippopoulos and Fotopoulos, 2022; Kijek et al., 2023). Economic development can further improve factor market reform and optimize factor allocation (Chang et al., 2024). The third is the interaction between innovation factor allocation and green economy development. Existing literature usually measures the degree of coupled coordination of innovation and economic development systems, with different approaches to indicator selection and measurement regions (Chen and Zhang, 2021; Wang and Tan, 2021; Yang et al., 2022a; Su, 2022; Yin et al., 2022).
According to the findings of existing literature, most studies suggest that optimizing the allocation of innovation factors can promote green economic development, while the misallocation of these factors can hinder it. Additionally, the advancement of the green economy further contributes to the optimization of innovation factor allocation. These studies provide the theoretical foundation for this research. Based on this foundation, the marginal contributions of this paper are as follows.
(1) This paper constructs evaluation indicator systems for both the innovation factor allocation system and the green economy development system, from the perspectives of input and output, systematically assessing the progress China has made in innovation factor allocation and green economy development.
(2) Using the Entropy weight TOPSIS method and the coupling coordination degree model, this paper measures the coupling coordination degree between the innovation factor allocation system and its subsystems and the green economy development system at both the national level and across China’s three major regions. The results indicate an overall upward trend, though significant regional disparities remain, laying a policy foundation for further optimization of innovation factor allocation and the promotion of green economy development in China.
(3) By employing the Dagum Gini coefficient, kernel density estimation, and spatial Markov chain methods, this paper analyzes the regional disparities, sources of differences, and dynamic distribution of the coupling coordination between innovation factor allocation and green economy development. These findings contribute to a better understanding of the diffusion patterns of technological innovation and green economy development, providing practical insights for the government in formulating regionally differentiated policies.
3 Research design
3.1 Indicator system construction
3.1.1 Innovation factor allocation system
Although innovation factor allocation has not been traditionally conceptualized as a systemic phenomenon, this paper approaches it from a systemic perspective due to its complex interrelationships among key sectors such as capital, labor, and R&D, which together shape innovation performance. Recent studies further support this systemic interpretation. Innovation factor allocation encompasses the dynamic interactions between elements such as the institutional environment and regional economic conditions, which reinforce each other and operate in an integrated manner. This, in turn, significantly influences outcomes like industrial structural transformation and economic development (Zhao, 2024; Chang et al., 2024). Therefore, by treating innovation factor allocation as a system, we can gain deeper insights into its role in promoting coordinated regional development and advancing the green economy.
Innovation factors are the productive elements that are involved in the innovation process and contribute positively to innovation outcomes. These factors can be categorized into traditional innovation factors and new innovation factors (Salter & Alexy, 2014). Traditional innovation factors are the essential production elements that provide the foundational support for innovation activities, including human innovation factors and capital innovation factors. New innovation factors, on the other hand, refer to the production elements that facilitate technological application, technology diffusion, and institutional support for innovation activities, encompassing technological innovation factors, data innovation factors, and institutional innovation factors.
Current literature on evaluating the innovation factor allocation has constructed indicator systems from various dimensions. Zhao (2024) constructed an indicator system based on four dimensions: human resources, capital, technology, and information. Chang et al. (2024) developed an indicator system using the dimensions of human resources, knowledge, technology, and institutions. Tao and Xu (2021) and Xu and Zhao (2021) each proposed an indicator system incorporating five dimensions: human resources, knowledge, technology, data, and institutions. To ensure the validity of the indicators, and taking into account the specific economic characteristics of different regions and the actual development status of innovation factors, this paper selects the indicators for the innovation factor allocation system from an input-based perspective. (1) Human innovation factors: Indicators are selected from the perspective of human input according to different innovation subjects. (2) Capital innovation factors: Indicators are selected from the perspective of capital investment according to different innovation agents. (3) Technological innovation factors: Indicators can be categorized into technology R&D and technology introduction based on the source of technological innovation. Indicators were selected from the perspective of technology R&D and technology introduction. (4) Data innovation factors: Infrastructure inputs that support the transfer and sharing of information are selected as indicators. (5) Institutional innovation factors: The environmental regulation intensity, the financial regulation intensity and the intellectual property protection index, which break down institutional barriers and enhance the efficiency of innovation, are selected as indicators. The specific indicators are detailed in Table 1.
3.1.2 Green economy development systems
Current literature assessing the level of green economy development primarily constructs indicator systems from dimensions such as green development, economy, technology, society, resources, environment, and health (Wang et al., 2019; Long et al., 2021; Ma et al., 2023). Drawing on these studies, this paper establishes a comprehensive indicator system for green economy development, focusing on two main aspects: green development and economic development. (1) Green development: Indicators are selected from the perspective of environmental protection. (2) Economic development: Indicators are chosen from economic, social, and health perspectives, including economic growth, innovation output, industrial structure, social employment, foreign trade, per capita consumption, income distribution, and healthcare. The specific details are detailed in Table 2.
3.2 Data sources
This paper takes the panel data of 30 Chinese provinces (except Tibet, Hong Kong, Macao and Taiwan) from 2013 to 2022 as the research sample. The data come from China Statistical Yearbook, China Science and Technology Statistical Yearbook, China High-Tech Industry Statistical Yearbook, China Energy Statistical Yearbook, China Provincial Statistical Yearbook, and Evaluation Report on the Development Status of Intellectual Property Rights in China.
3.3 Methods
3.3.1 Entropy weight TOPSIS method
The level of integrated development of the systems is measured using the entropy weight TOPSIS method. Firstly, the weights of the indicators within the system were determined using the entropy weight method. Secondly, the level of integrated development of the system was represented using the closeness constructed by TOPSIS. The specific steps are as follows.
The raw data matrix for n provinces with m indicators can be represented as Equation 1:
The first step is standardized conversion, as shown in Equations 2, 3.
Positive indicators:
Negative indicators:
The second step is to calculate the information entropy (
The third step is to calculate the weights, as shown in Equation 6.
The fourth step is to calculate the weighted values of the indicators, as shown in Equation 7.
The fifth step is to calculate the Euclidean distance between the evaluation indicator and the maximum value, as shown in Equation 8.
The sixth step is to calculate the level of integrated development for area i, as shown in Equation 9.
3.3.2 Coupled coordination degree model
According to the coupled coordination degree model (Norgaard, 1990), the comprehensive development level of the innovation factor allocation system is set as
The degree of coordination can be expressed by Equation 11.
The innovation factor allocation system and the green economy development system are equally important, so that
Combining the characteristics of the studied data, the type of coupling coordination was categorized into five stages based on the values of the coupling coordination degree. The specific details are detailed in Table 3.
3.3.3 Dagum Gini coefficient
This paper employs the Dagum Gini coefficient to measure and decompose the relative regional disparities in the coupling coordination degree between innovation factor allocation and green economy development in China. Furthermore, it delves into the overall differences and origins of the coupling development between these two systems. This approach effectively addresses the issues of spatial differentiation origins and the cross-overlap between subsamples, and has been widely applied in studies investigating regional disparities (Wen et al., 2023).
According to the decomposition method (Dagum, 1997), the overall Gini coefficient can be decomposed into intra-regional differences (
Equations 14–16 calculate the overall Gini coefficient, the Gini coefficient for region j, and the Gini coefficient between regions j and h. In these equations,
Equations 17–19 calculate the intra-regional differences (
Equations 20–22 calculate the relative impact (
3.3.4 Kernel density estimation
Kernel density estimation is a non-parametric method used to fit the probability density curve of sample data by employing a smooth kernel function as a weight (Wen et al., 2023). In this paper, kernel density estimation is used to analyze the overall distribution, polarization trend and ductility of the coupled and coordinated development of innovation factor allocation and green economic development.
In Equation 23,
3.3.5 Markov chains
The Markov transfer probability matrix reflects the state of each region and its probability of upward or downward transfer. The basic approach is to discretize the regional continuum into k state types and calculate the probability of each state type, thus approximating the regional evolution as a Markov process.
The probabilities of the various state types for each province in year t are represented as a
Considering the spatial spillover effect in the neighboring regions, the traditional Markov transfer probability matrix can be decomposed into k conditional transition probability matrices, each of size
In this matrix,
After prolonged transitions, the coupling coordination degree of each province will eventually reach a stable state, remaining unaffected by any temporal changes. Equation 26 calculates the steady state, which is calculated from the initial state, Markov transfer probability matrix.
In Equation 26, π is the steady state matrix of the Markov process with the conditions shown in Equation 27.
4 Results and analysis
4.1 Temporal characteristics of the coupling coordination between innovation factor allocation and green economy development
4.1.1 Degree of coupling coordination between innovation factor allocation and green economy development
The coupling coordination degree of innovation factor allocation and green economy development is shown in Table 4. Overall data indicate that the mean coupling coordination degree of China’s innovation factor allocation and green economy development is 0.438, which is in the stage of basic coordinated development, indicating that the coupling coordinated development of the two systems still has a large room for improvement. The growth rate data indicate that the average annual growth rate of the coupling coordination degree between innovation factor allocation and green economic development in China is 0.389%. This degree increased from 0.432 in 2013 to 0.448 in 2022, indicating that the mutual promotion between the two systems has achieved some positive results, with potential for further improvement.
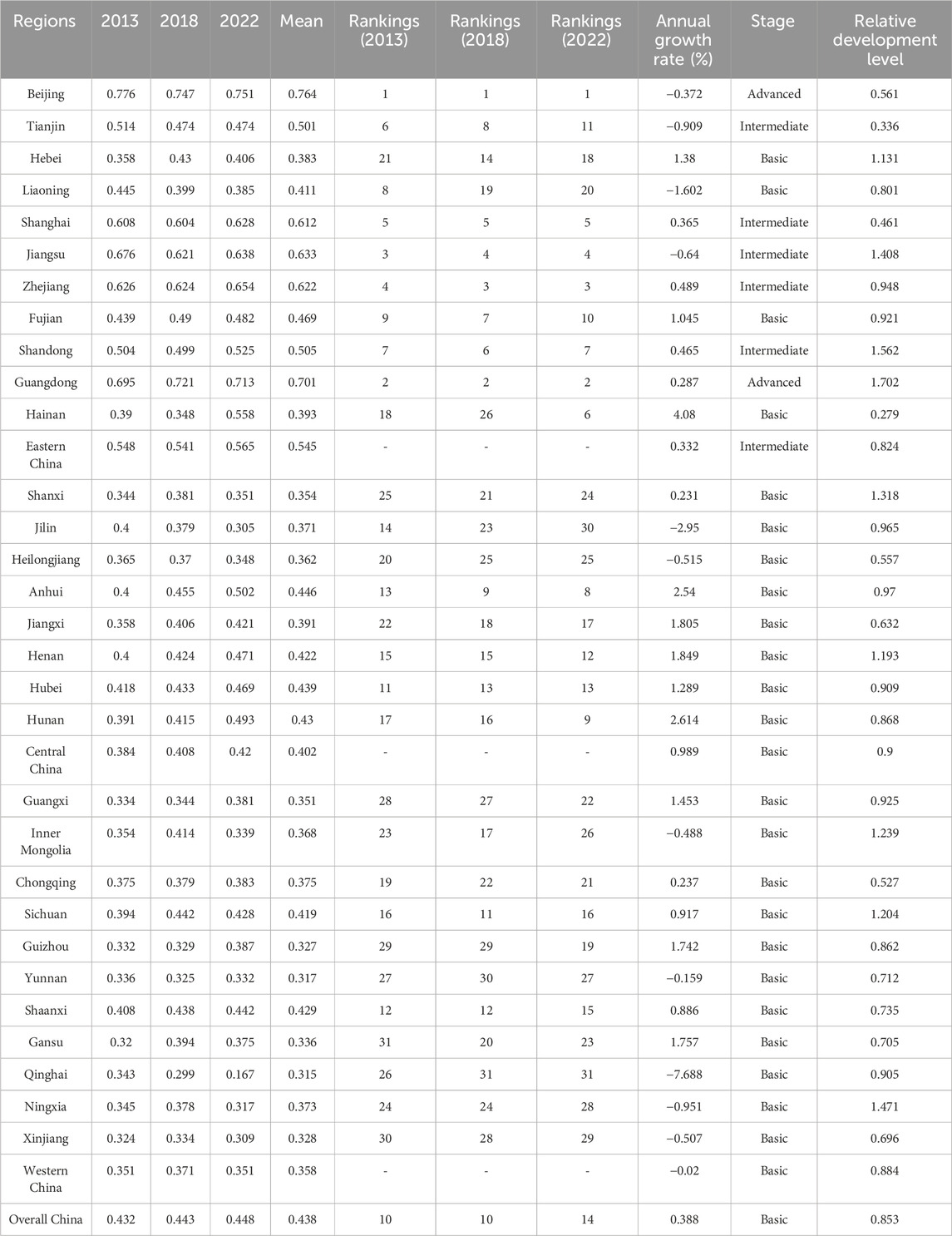
Table 4. Degree of coupling coordination between innovation factor allocation and green economy development in China.
The relative development level of the innovation factor allocation system and the green economy development system is the ratio of these two systems, which is greater than one indicating that the innovation factor allocation system is superior to the green economy development system, otherwise the opposite is true. The relative development level data show that the overall mean is 0.853, while the means for the eastern, central and western regions are all less than 1. Therefore, the green economic development system in China is currently outperforming the innovation factor allocation system. In the short term, improving the development level of the innovation factor allocation system would be beneficial for enhancing the coupling coordination between the two systems. In terms of specific provinces, the relative development level in Hebei, Jiangsu, Shandong, Guangdong, Shanxi, Henan, Inner Mongolia, Sichuan, and Ningxia exceeds 1, indicating that the development of innovation factor allocation in these provinces is relatively advanced.
In order to understand the inter-regional differences, the coupling coordination degree data of innovation factor allocation and green economy development in the eastern, central and western parts of the country are compared. The mean values indicate that the Eastern China has the highest coupling coordination degree at 0.545, followed by the Central China at 0.402, and the Western China at 0.358, displaying a clear pattern of “higher in the East, lower in the West.” This uneven spatial distribution aligns with the broader trends observed in China’s economic development. The annual growth rate data reveal that the Central China has the highest growth rate at 0.989%, followed by the Eastern China at 0.332%, with the Western China showing the lowest rate at −0.020%. This indicates a narrowing gap between the Central and Eastern China, while the disparity between the Western region and the Central and Eastern regions is widening. The uneven spatial distribution characteristics of the coupling coordination degree between China’s innovation factor allocation system and green economy development are further expanding.
At the provincial level, the mean coupling coordination degree is classified as advanced for Beijing (0.764) and Guangdong (0.701). The provinces classified as intermediate include Tianjin (0.501), Shanghai (0.612), Jiangsu (0.633), Zhejiang (0.622), and Shandong (0.505), all of which are located in the Eastern China. This indicates that the overall coupling coordination degree in the Eastern region is higher than that of the Central and Western regions. The ranking data show that the rankings for most provinces remained relatively stable, with significant changes observed only in Liaoning, Jilin, and Hainan. Liaoning dropped from 8th place in 2013 to 20th in 2022, Jilin fell from 14th place in 2013 to 30th in 2022, while Hainan rose from 18th place in 2013 to 6th in 2022. Combined with the analysis of the annual growth rates, it can be inferred that the coupling coordination degree in these three provinces underwent considerable changes during the sample period.
4.1.2 Degree of coupling coordination between the innovation factor allocation subsystem and green economy development
4.1.2.1 Overall situation
Table 5 demonstrates that the coupling coordination degree between the various subsystems of China’s innovation factors and green economic development exhibited an upward trend during the sample period. The mutual promotion and coupling coordination between the various innovation factor inputs and green economic development in China show a positive and expectedly favorable trend. The coupling coordination degrees between the various innovation subsystems and green economic development all fall within the 0.3–0.5 range, indicating that they are in the basic stage of coordinated development, with significant room for improvement. The mean values indicate that the highest coupling coordination degree is observed in the Institution-Economy system (0.496), followed by the Human-Economy system (0.488), with the Technology-Economy system having the lowest degree (0.413). This suggests that during the sample period, the Chinese government effectively promoted the coupling coordination between innovation factor allocation and green economic development through the establishment of appropriate policies. However, the coupling coordination between technological innovation factors and green economy development among various innovation entities still requires improvement.
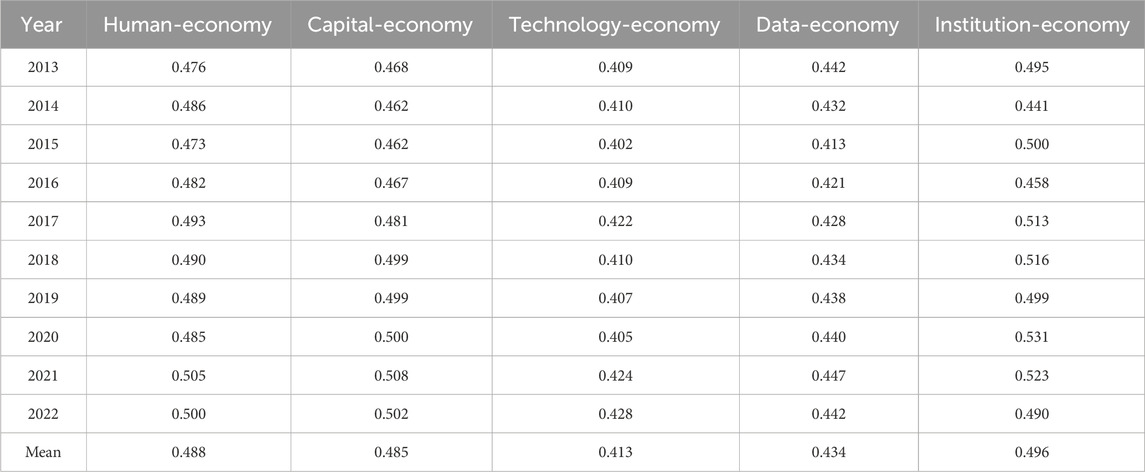
Table 5. Degree of coupling and coordination between China’s innovation factor subsystem and green economy development.
4.1.2.2 Inter-regional comparisons
As shown in Table 6, the coupling coordination degrees between the innovation subsystems and green economic development in the Eastern region are all within the 0.5–0.7 range, indicating that their coupling coordination is at an intermediate stage. In contrast, the Central and Western regions have coupling coordination degrees within the 0.3–0.5 range, placing them at a basic stage of coordination. This indicates that, during the sample period, the coupling coordination between innovation subsystems and green economic development in the Eastern region was significantly higher than in the Central and Western regions, which aligns with the regional characteristics of China’s economic development.
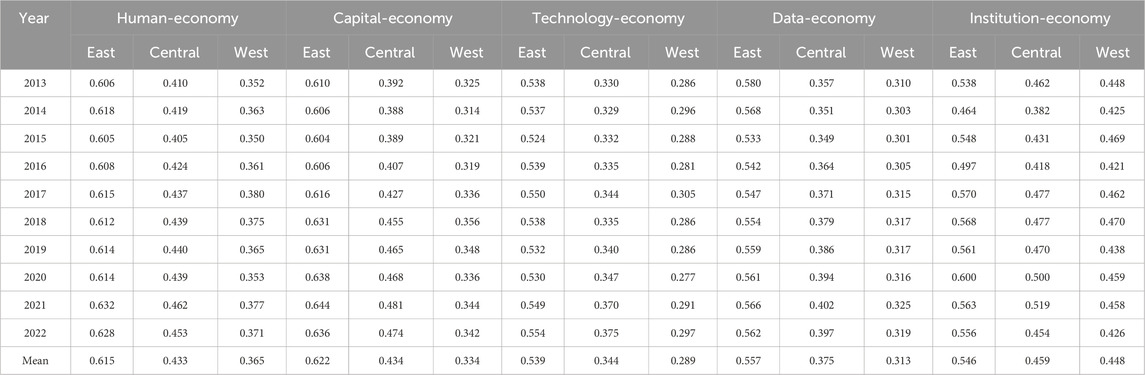
Table 6. Degree of coupling and coordination between innovation factor subsystems and green economy development in China’s regions.
In Eastern China, the highest coupling coordination degree is found in the Capital-Economy system (0.622), indicating a strong mutual promotion between capital innovation factor inputs and green economic development. The Technology-Economy system has the lowest coupling coordination degree (0.539), suggesting that enhancing the allocation of technological innovation factors is a crucial pathway for promoting coordinated development.
For the Central and Western regions, a common characteristic is that the highest coupling coordination degree is found in the Institution-Economy system (0.459 and 0.448, respectively), while the lowest is in the Technology-Economy system (0.370 and 0.291, respectively). This indicates that in economically underdeveloped regions, the allocation level of institutional innovation factors is relatively high, making it easier to promote coupling coordination. Similarly to the Eastern region, enhancing the allocation of technological innovation factors is also a critical pathway for promoting coupling coordination development.
4.2 Spatial differences in the coupling coordination of innovation factor allocation and green economy development
Table 7 presents the overall Gini coefficient (
Figure 1A illustrates the trend in the Gini coefficient of the coupling coordination degree between innovation factor allocation and green economy development for China overall and three major regions during the sample period. As shown in Figure 1A, the Gini coefficient for the overall coupling coordination degree in China increased from 0.1397 to 0.1594 during the sample period, with an average annual growth rate of 1.48%. This result indicates that the spatial disparity in China’s overall coupling coordination degree is widening. Within the three regions, the Eastern region exhibits the highest internal disparity, with an average Gini coefficient of 0.1310, while the Central and Western regions show lower internal disparities, with average Gini coefficients of 0.0553 and 0.0741, respectively. In terms of trends, the Gini coefficient for the coupling coordination degree in the Eastern region decreased from 0.1354 to 0.1310 during the sample period, whereas it increased from 0.0343 to 0.0553 in the Central region and from 0.0423 to 0.0741 in the Western region. This suggests that internal disparities in the Eastern region are gradually narrowing, while those in the Central and Western regions are gradually expanding.
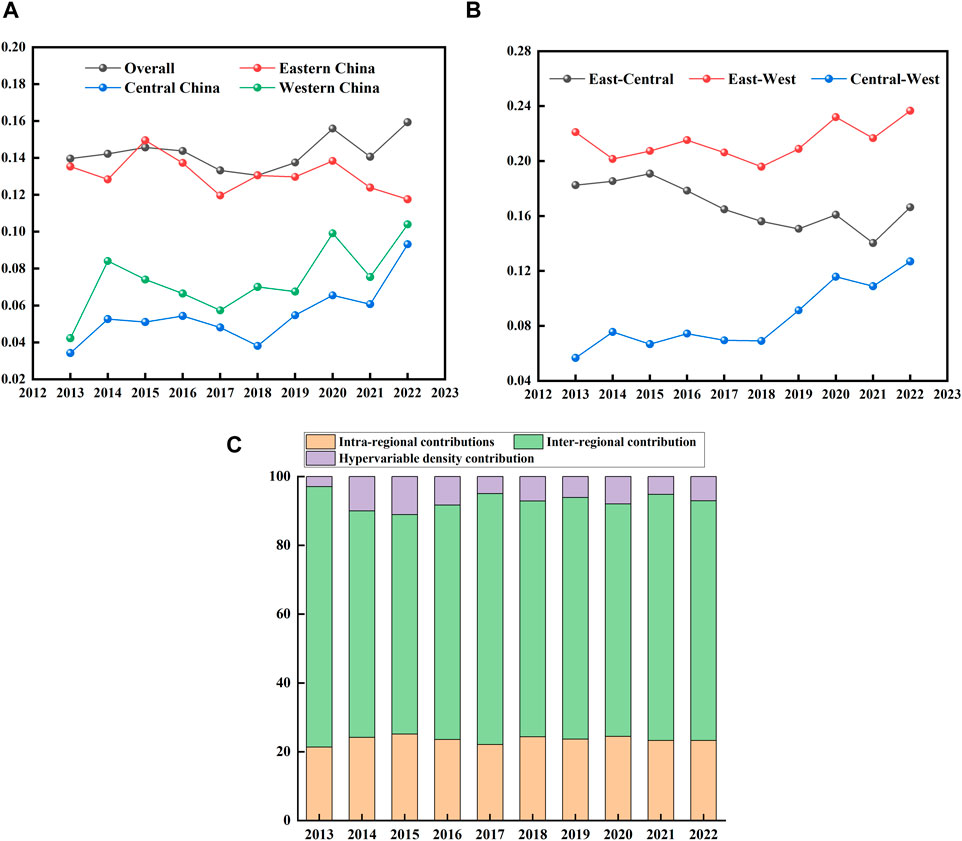
Figure 1. Gini coefficient change and contribution. (A) Overall and intra-regional disparities, (B) Inter-regional disparities, (C) Contribution rates of sources of spatial disparities.
Figure 1B depicts the trend in the inter-regional Gini coefficient for the coupling coordination degree between innovation factor allocation and green economy development in China during the sample period. As shown in Figure 1B, the spatial disparity between the Eastern and Western regions is the largest, with an average Gini coefficient of 0.2142, followed by the disparity between the Eastern and Central regions with an average Gini coefficient of 0.1677. The smallest spatial disparity is observed between the Central and Western regions, with an average Gini coefficient of 0.0855. In terms of trends, the spatial disparity between the Eastern and Central regions is gradually narrowing, with an average annual growth rate of −1.03% during the sample period. In contrast, the spatial disparities between the Eastern and Western regions and between the Central and Western regions are gradually widening, with average annual growth rates of 0.76% and 9.35%, respectively.
Figure 1C illustrates the trend in the contribution rates of various components to the overall Gini coefficient during the sample period. As shown in Figure 1C, the inter-regional disparities contribute the most to the overall disparities, with an average contribution rate of 69.41%, displaying a trend of initial decline followed by a subsequent rise. The second most significant contributor is the intra-regional disparities, with an average contribution rate of 23.53%. Lastly, the contribution rate of the hypervariable density is the smallest, averaging 7.05%. These results indicate that the primary source of overall spatial disparities in China is the inter-regional differences, followed by intra-regional disparities. The relatively minor contribution of hypervariable density, which reflects the overlapping contributions of different regions to the overall disparity, suggests that there is minimal cross-overlap in the coupling and coordination degrees among provinces within China’s three major regions. This indicates that the regional disparities in the coupling coordination degree are primarily driven by inter-regional disparities. The eastern regions benefit from advanced infrastructure and robust policy support, resulting in higher efficiency in innovation factor allocation. In contrast, the western regions, which are more dependent on natural resources and face weaker policy support, exhibit lower levels of coupling coordination. Furthermore, disparities in economic structures, resource distribution, and policy implementation exacerbate the east-west divide.
4.3 Dynamic evolution of the coupling coordination of innovation factor allocation and green economy development
4.3.1 Kernel density analysis of the coupling coordination degree of innovation factor allocation and green economy development
A kernel density estimation plot was created to clarify the overall distribution and dynamic trends of the coupling coordination between innovation factor allocation and green economy development across China and its three major regions. This is illustrated in Figure 2.
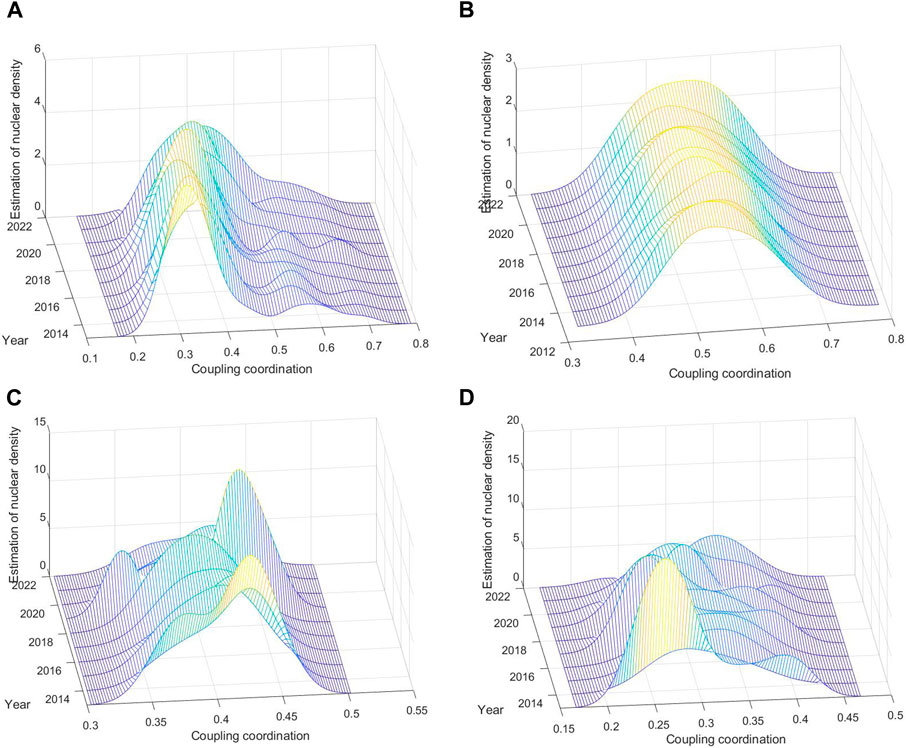
Figure 2. Kernel density estimation. (A) Overall China, (B) Eastern China, (C) Central China, (D) Western China.
Figure 2A illustrates the dynamic distribution of the coupling coordination degree between innovation factor allocation and green economy development across China. The center of the peak of the curve is distributed to the left, indicating that a significant proportion of regions have a relatively low coupling coordination degree. The slow rightward shift of the main peak suggests a gradual, though modest, improvement in the coupling coordination degree over time. The decreasing peak height and increasing width, coupled with a tailing effect, indicate growing disparities among provinces. The presence of secondary peaks further suggests the occurrence of polarization.
Figure 2B illustrates the dynamic distribution of the coupling coordination degree between innovation factor allocation and green economy development in Eastern China. The peak of the curve is centrally located, with no significant tailing effect, and shows a tendency to shift rightward, indicating that a substantial number of provinces have a high level of coupling coordination. The increase in the height of the main peak suggests a reduction in internal disparities. Specifically, provinces with a higher average coupling coordination degree, such as Beijing and Jiangsu, experienced annual growth rates of −0.37% and −0.64%, respectively. In contrast, provinces with a lower average coupling coordination degree, such as Hebei and Hainan, recorded annual growth rates of 1.38% and 4.08%, respectively. This trend has contributed to the narrowing of disparities in coupling coordination degrees among provinces in Eastern China.
Figure 2C illustrates the dynamic distribution of the coupling coordination degree between innovation factor allocation and green economy development in Central China. The peak of the curve is skewed to the left, indicating that a large proportion of regions have relatively low coupling coordination degrees. The main peak shows a tendency to shift rightward, suggesting an overall increase in the coupling coordination degree. Temporary secondary peaks appeared in 2013 and 2019, indicating the presence of polarization, though this was not sustained. The height of the peak underwent a “decline-rise-decline” process, and the width of the main peak consistently broadened, indicating a continuous increase in internal disparities. Specifically, provinces with a higher average coupling coordination degree, such as Anhui and Hubei, exhibited annual growth rates of 2.54% and 1.29%, respectively. In contrast, Jilin and Heilongjiang, with lower average coupling coordination degrees, experienced annual growth rates of −2.95% and −0.52%, respectively. Consequently, the disparities in coupling coordination degrees among provinces in Central China have been widening over time.
Figure 2D illustrates the dynamic distribution of the coupling coordination degree between innovation factor allocation and green economy development in Western China. The peak of the curve is skewed to the left, indicating that a significant proportion of regions have relatively low coupling coordination degrees. The main peak continuously shifts to the right, suggesting sustained growth in the coupling coordination degree. The presence of secondary peaks indicates the occurrence of polarization. The main peak’s height decreases while its width expands, and a tailing effect is observed, all of which suggest increasing disparities among the provinces in the region. Specifically, provinces with higher average coupling coordination degrees, such as Sichuan and Shaanxi, have annual growth rates of 0.92% and 0.89%, respectively. In contrast, provinces with lower average coupling coordination degrees, such as Qinghai and Xinjiang, experience annual growth rates of −7.69% and −0.51%, respectively. As a result, the disparities in coupling coordination degrees among the provinces in Western China have been widening over time.
In summary, the kernel density estimation curves for overall China and its three major regions all exhibit a rightward shift in the main peak, indicating a continuous increase in the coupling coordination degree between innovation factor allocation and green economy development in these regions. The changes in the main peak’s position are consistent with the described characteristics of coupling coordination between innovation factor allocation and green economic development in each region. The narrowing internal disparities in Eastern China, combined with the widening disparities in Central and Western China, contribute to an overall trend of increasing disparities among provinces across China.
This suggests that the pathways for coupled and coordinated innovation factor allocation and green economy development in China exhibit significant regional heterogeneity, underscoring the need to avoid one-size-fits-all policies (Wen et al., 2023). Stronger local governance and more efficient policy implementation have enabled the eastern regions to achieve innovation and green development initiatives more effectively. In contrast, the western regions’ reliance on resource-intensive industries hinders the effective allocation of innovation factors toward greener initiatives, further exacerbating the gap between these regions and the more developed eastern areas. Moreover, Eastern China benefits from a higher concentration of human capital, financial investment, and technological resources—key drivers of innovation and green economy development. Provinces in the East also enjoy greater access to venture capital, highly skilled labor, and advanced technological infrastructure, all of which contribute to the more efficient allocation of innovation factors. Conversely, Central and Western China face significant challenges in attracting these critical resources, primarily due to relatively underdeveloped infrastructure and lower levels of economic development. The uneven distribution of resources across regions restricts the capacity of Central and Western provinces to engage in high-value innovation activities, further widening the gap in their coupling coordination levels. Addressing these disparities will require region-specific policies that strengthen local governance, diversify economic structures, and promote the equitable distribution of innovation resources.
4.3.2 Markov chain analysis of the coupling and coordination between innovation factor allocation and green economy development
4.3.2.1 Traditional Markov chain analysis
The traditional Markov transition probability matrix, along with the spatial Markov transition probability matrix that incorporates spatial spillover effects, has been constructed to facilitate an in-depth analysis of the spatial dynamic evolution characteristics of China’s coupling coordination degree. In this paper, an upward transition is defined as the movement of the coupling coordination degree from a lower to a higher value, while a downward transition is defined as the opposite.
Table 8 presents the traditional Markov transition probability matrix, from which it can be observed that the probabilities along the diagonal are higher than those off the diagonal. This indicates that the states of the coupling coordination degree are relatively stable, with a greater likelihood of maintaining the current state. A clear “club convergence” phenomenon is evident among the coupling coordination degrees of the provinces, with the advanced and superior state types showing the highest probabilities of maintaining their respective states from year t to year t+1, at 92.42% and 71.64%, respectively. The higher the development level of a province, the lower the probability of downward transition in the following year. For example, the intermediate state has a 24% probability of downward transition, the advanced state has a 17.91% probability, and the superior state has only a 7.5% probability of downward transition. This suggests that the coupling coordination degree across China’s provinces generally exhibits a gradient upward development trend.
4.3.2.2 Spatial Markov chain analysis
The state type of neighboring regions may influence the transition path of a region’s state type in the following year. The “0–1″ weight matrix is employed as the spatial weight matrix for the Markov chain to calculate the transition probabilities. This approach is used to examine the steady state distribution of China’s coupling coordination degree levels from 2013 to 2022.
Table 9 presents the spatial Markov transition probability matrix, highlighting the significant role that spatial factors play in the transition of state types. When spatial spillover effects are incorporated, the transition probabilities of provinces’ state types in the following year show notable changes under different neighborhood contexts. The state types of the coupling coordination degree exhibit a pronounced spatial clustering effect. For instance, when the neighborhood type is basic, the number of observations maintaining the basic state from year t to year t+1 is significantly higher than for other states. Similarly, when the neighborhood type is superior, the observations maintaining the superior state across consecutive years are also markedly higher. The spatial Markov transition probability matrix further demonstrates a clear “club convergence” phenomenon in the spatial dimension. Influenced by the neighborhood’s state, the probability of an upward transition is generally higher than that of a downward transition. Moreover, the higher the neighborhood’s state type, the greater the probability of an upward transition in the region’s own state type. This indicates a trend of continuous improvement in the coupling coordination degree between innovation factor allocation and green economy development in China.
4.3.2.3 Steady state forecasting
The steady state distribution of regional state type can be effectively forecasted by calculating the limiting distribution of the Markov transition probability matrix. Table 10 presents the forecasted long-term evolutionary trends of the coupling coordination degree in China.
Under the assumption of no spatial spillover effects, a comparison between the steady-state distribution derived from the traditional Markov transition probability matrix and the initial state reveals a decrease in the number of provinces classified as having basic, intermediate, and advanced state types, while the number of provinces classified as having the superior state type shows a significant increase. This indicates that, over the long term, the state types of China’s coupling coordination degree tend to gradually transition from lower to higher levels, exhibiting an evolutionary trend of sequential progression, with an overall improvement in the coupling coordination level.
Under the consideration of spatial spillover effects, the evolutionary trends of the coupling coordination degree types undergo significant changes. Over the long term, regions adjacent to those basic state type tend to exhibit a higher occurrence of basic state typology (45.45%) and intermediate state type (31.82%), with no instances of the superior state type. Conversely, when neighboring regions have higher levels of coupling coordination, the probability of transitioning to advanced and superior state types is significantly greater than in other scenarios.
Therefore, based on the current development trends, the evolution of China’s coupling coordination degree between innovation factor allocation and green economy development appears to be optimistic. The coupling coordination degree gradually improves over time, exhibiting a trend of high-level regional clustering. The number of provinces in each state type increases progressively from low to high levels. The influence of neighboring state types shows a strong degree of synergy. Proximity to lower-level provinces tends to reduce the extent of improvement in the coupling coordination degree, and may even result in downward transitions. In contrast, proximity to higher-level provinces fosters the clustering of coupling coordination degrees towards advanced and superior state type, leading to an overall upward trend.
5 Conclusions and discussion
5.1 Conclusions
In this paper, based on data from 30 Chinese provinces from 2013 to 2022, an indicator system was developed for China’s innovation factor allocation system and green economy development system. The entropy weight TOPSIS method was applied to measure the comprehensive development level and coupling coordination degree of the two systems. Additionally, Dagum Gini coefficient, kernel density estimation, and spatial Markov chain methods were utilized to uncover the spatiotemporal characteristics and dynamic evolution of the coupling coordination between the innovation factor allocation system and green economy development system. The findings are mainly as follows:
(1) The overall coupling coordination degree between innovation factor allocation and green economy development in China remains relatively low but shows a continuous upward trend. This is consistent with studies that highlight China’s struggle to balance innovation factor allocation with sustainable growth. For instance, Dai et al. (2022) pointed out that while R&D investment is increasing, there is still an imbalance in how these investments are distributed across regions, particularly favoring Eastern China over Central and Western regions. Our study adds to this by showing that although human innovation factors and institutional innovation factors exhibit relatively higher coordination with green economy development at the national level, the overall coupling remains modest.
(2) Regional disparities in the coupling coordination degree exhibit an imbalanced spatial distribution, with higher levels in Eastern China and lower levels in Western China. This aligns with the findings of Shang et al. (2023), who identified that green innovation efficiency is clustered in Eastern regions, while Western regions lag behind due to insufficient economic and policy support for innovation. Our study builds upon this by demonstrating that the widening regional disparity, as revealed by the Dagum Gini coefficient, is driven primarily by the growing gap between Eastern and Western China. This underscores the need for region-specific policies to address these imbalances.
(3) Kernel density estimation indicates a long-term growth trend in the coupling coordination degree, but also evidence of polarization. Similar findings of regional polarization in innovation capacities have been noted by Yang et al. (2023b), who highlighted that Eastern regions are advancing rapidly while Central and Western regions struggle to keep up. Our research contributes new insights by showing that internal disparities within Eastern China are narrowing, while those within Central and Western China are expanding, indicating a need for targeted interventions in these regions.
(4) The spatial Markov chain analysis reveals a clear “club convergence” phenomenon in the coupling coordination degree across provinces. This finding aligns with the work of Jimenez-Moro et al. (2023), who observed similar convergence patterns in regional innovation performance across China. However, our study adds to the literature by showing that provinces adjacent to those with higher coupling coordination degrees are more likely to experience upward transitions due to spatial spillover effects, emphasizing the importance of fostering regional collaborations to enhance innovation and green development.
5.2 Discussion
Based on the above findings, the following recommendations are proposed to assist local governments in adjusting resource allocation policies and promoting green economic development.
(1) Promote the synchronized advancement of innovation factor allocation and green economy development. The government should prioritize the development of institutional and human innovation factors as key subsystems to foster green economy growth. To implement this, targeted policies must be introduced that support education and training in green technologies, especially in regions with a strong industrial base. For instance, setting up specialized training centers focused on green skills development can bridge the gap between workforce capabilities and the needs of the green economy. Moreover, the government should offer tax incentives and subsidies to companies investing in green R&D and the adoption of clean technologies, ensuring that institutional frameworks are conducive to innovation in this field.
(2) Prioritize the coordinated development of innovation factor allocation and green economy growth across regions. In Western China, leveraging natural resources while aligning with the region’s stage of green development requires the establishment of region-specific innovation zones. These zones should focus on clean energy technologies, such as solar and wind energy, given the region’s abundant resources. Additionally, the government could promote cross-regional collaboration through the formation of innovation alliances between Western provinces and more developed Eastern regions. This can be facilitated by improving infrastructure to enhance the mobility of human capital and technology. Feasibility is supported by the existing Belt and Road Initiative, which has already laid some groundwork for infrastructural development in these regions.
(3) Leverage the exemplary role of the high-level regions in Eastern China. The Eastern regions, particularly provinces like Jiangsu and Zhejiang, have developed successful models of coupling innovation with green economy growth. To implement this suggestion, governments in these regions should establish “innovation-driven green development pilot zones.” These zones could serve as experimental areas for testing new policies and technologies related to green economy development, which could then be scaled up in other regions. Additionally, inter-regional workshops and knowledge-sharing platforms could be created, enabling Central and Western regions to adopt best practices from Eastern China. This approach is feasible given the existing provincial-level innovation platforms that have proven effective in fostering technology transfers.
(4) Focus on the neighboring effects in the coupling coordination between innovation factor allocation and green economy development. Regions with low levels of coupling coordination should actively create partnerships with neighboring, highly coordinated regions. A concrete implementation path for this is to establish “green innovation hubs” at the borders of highly coordinated and less coordinated regions. These hubs would act as collaboration centers, where companies and local governments from both sides could work together on joint innovation projects. For regions that neighbor other low-coordination areas, regional governments should introduce joint development agreements that prevent industrial homogenization by promoting complementary industries. This can be achieved by conducting regular joint market assessments to identify areas where regional economies can diversify and avoid competition. The feasibility of this approach is enhanced by leveraging regional development frameworks like the Yangtze River Economic Belt initiative, which already promotes inter-provincial cooperation.
Data availability statement
The original contributions presented in the study are included in the article/supplementary material, further inquiries can be directed to the corresponding author.
Author contributions
KG: Funding acquisition, Methodology, Writing–original draft, Writing–review and editing. XZ: Data curation, Software, Visualization, Writing–original draft. HJ: Methodology, Writing–original draft, Writing–review and editing. ZZ: Investigation, Validation, Writing–original draft.
Funding
The author(s) declare that financial support was received for the research, authorship, and/or publication of this article. This work was supported by the Major Projects of Philosophy and Social Science Research in Jiangsu Universities (2023SJZD060).
Conflict of interest
The authors declare that the research was conducted in the absence of any commercial or financial relationships that could be construed as a potential conflict of interest.
Publisher’s note
All claims expressed in this article are solely those of the authors and do not necessarily represent those of their affiliated organizations, or those of the publisher, the editors and the reviewers. Any product that may be evaluated in this article, or claim that may be made by its manufacturer, is not guaranteed or endorsed by the publisher.
References
Abid, N., Ceci, F., and Ikram, M. (2022). Green growth and sustainable development: dynamic linkage between technological innovation, ISO 14001, and environmental challenges. Environ. Sci. Pollut. Res. 29, 25428–25447. doi:10.1007/s11356-021-17518-y
Chang, X., Yang, Z., and Abdullah, (2024). Digital economy, innovation factor allocation and industrial structure transformation—a case study of the Yangtze River Delta city cluster in China. Plos one 19 (4), e0300788. doi:10.1371/journal.pone.0300788
Chen, C., Lan, Q., Gao, M., and Sun, Y. (2018). Green total factor productivity growth and its determinants in China’s industrial economy. Sustainability 10 (4), 1052. doi:10.3390/su10041052
Chen, Y., and Zhang, D. (2021). Multiscale assessment of the coupling coordination between innovation and economic development in resource-based cities: a case study of Northeast China. J. Clean. Prod. 318, 128597. doi:10.1016/j.jclepro.2021.128597
Chi, Y., Guo, Z., Zheng, Y., and Zhang, X. (2014). Scenarios analysis of the energies’ consumption and carbon emissions in China based on a dynamic CGE Model. Sustainability 6 (2), 487–512. doi:10.3390/su6020487
Costa Cavalcante, P. L. (2024). Innovation performance and its determinants: what does it take to succeed? Innovation and Manag. Rev. 21 (1), 60–76. doi:10.1108/INMR-11-2021-0206
Dagum, C. (1997). A new approach to the decomposition of the gini income inequality ratio. Empir. Econ. 22 (4), 515–531. doi:10.1007/BF01205777
Dai, D., Fan, Y., Wang, G., and Xie, J. (2022). Digital economy, R&D investment, and regional green innovation—analysis based on provincial panel data in China. Sustainability 14 (11), 6508. doi:10.3390/su14116508
Ding, R., Sun, F., and Zhong, T. (2024). Does technological innovation promote green development in the Yangtze River Economic Belt? Based on the spatial econometric analysis. Environ. Dev. Sustain., 1–35. doi:10.1007/s10668-024-04768-2
Fan, F., Dai, S., Zhang, K., and Ke, H. (2021). Innovation agglomeration and urban hierarchy: evidence from Chinese cities. Appl. Econ. 53 (54), 6300–6318. doi:10.1080/00036846.2021.1937507
Fang, G., Wang, Q., and Tian, L. (2020). Green development of Yangtze River delta in China under population-resources-environment-development-satisfaction perspective. Sci. Total Environ. 727, 138710. doi:10.1016/j.scitotenv.2020.138710
Fernández, E., Pérez, R., and Ruiz, J. (2011). Optimal green tax reforms yielding double dividend. Energy Policy 39 (7), 4253–4263. doi:10.1016/j.enpol.2011.04.041
Filippopoulos, N., and Fotopoulos, G. (2022). Innovation in economically developed and lagging European regions: a configurational analysis. Res. Policy 51 (2), 104424. doi:10.1016/j.respol.2021.104424
Gao, C., Cao, M., Wen, Y., and Li, C. (2023). Coupling and interaction between science and technology finance and green development: based on coupling coordination degree model and panel vector autoregression model. Front. Environ. Sci. 11, 1090998. doi:10.3389/fenvs.2023.1090998
He, L., Zhang, L., Zhong, Z., Wang, D., and Wang, F. (2019). Green credit, renewable energy investment and green economy development: empirical analysis based on 150 listed companies of China. J. Clean. Prod. 208, 363–372. doi:10.1016/j.jclepro.2018.10.119
Herman, K. S., Kim, Y. J., Shayegh, S., and Xiang, J. (2023). A critical review of green growth indicators in G7 economies from 1990 to 2019. Sustain. Sci. 18 (6), 2589–2604. doi:10.1007/s11625-023-01397-y
Hsieh, C. T., and Klenow, P. J. (2009). Misallocation and manufacturing TFP in China and India. Q. J. Econ. 124 (4), 1403–1448. doi:10.1162/qjec.2009.124.4.1403
Innocenti, N., Capone, F., and Lazzeretti, L. (2020). Knowledge networks and industrial structure for regional innovation: an analysis of patents collaborations in Italy. Pap. Regional Sci. 99 (1), 55–73. doi:10.1111/pirs.12478
Jänicke, M. (2012). “Green growth”: from a growing eco-industry to economic sustainability. Energy policy 48, 13–21. doi:10.1016/j.enpol.2012.04.045
Jimenez-Moro, E., Piperopoulos, P., Kafouros, M., and Ming, A. A. K. (2023). Innovation dynamics and club convergence in innovation activity in China: a temporal perspective. Pap. Regional Sci. 102 (4), 791–817. doi:10.1111/pirs.12750
Jones, C. I., and Tonetti, C. (2020). Nonrivalry and the economics of data. Am. Econ. Rev. 110 (9), 2819–2858. doi:10.1257/aer.20191330
Kang, W. L., Zou, Y. K., Wang, L., and Liu, X. M. (2020). Measurements and factors of biased technological progress in China's marine economy. Pol. J. Environ. Stud. 29 (6), 4109–4122. doi:10.15244/pjoes/118747
Ke, H., Dai, S., and Yu, H. (2022). Effect of green innovation efficiency on ecological footprint in 283 Chinese Cities from 2008 to 2018. Environ. Dev. Sustain. 24 (2), 2841–2860. doi:10.1007/s10668-021-01556-0
Khan, I., Han, L., BiBi, R., and Khan, H. (2022). The role of technological innovations and renewable energy consumption in reducing environmental degradation: evidence from the belt and road initiative countries. Environ. Sci. Pollut. Res. 29 (48), 73085–73099. doi:10.1007/s11356-022-21006-2
Kijek, T., Kijek, A., and Matras-Bolibok, A. (2023). “Innovation and regional development,” in Innovation and regional technological convergence. SpringerBriefs in regional science (Cham: Springer). doi:10.1007/978-3-031-24531-2_2
Kogan, L., Papanikolaou, D., Seru, A., and Stoffman, N. (2017). Technological innovation, resource allocation, and growth. Q. J. Econ. 132 (2), 665–712. doi:10.1093/qje/qjw040
Kortelainen, M. (2008). Dynamic environmental performance analysis: a malmquist index approach. Ecol. Econ. 64 (4), 701–715. doi:10.1016/j.ecolecon.2007.08.001
Li, J., Zeeshan, M., Rehman, A., and Uktamov, K. (2024). A green revolution in the making: integrating environmental performance and green finance for China’s sustainable development. Front. Environ. Sci. 12, 1388314. doi:10.3389/fenvs.2024.1388314
Liang, K., Li, Z., and Luo, L. (2023). Measurement, dynamic evolution and influencing factors of green development efficiency in western China: based on ecological-economic-social system. Plos one 18 (12), e0290472. doi:10.1371/journal.pone.0290472
Liu, P., Han, G., Yang, H., and Li, X. (2024). A sustainable development study on innovation factor allocation efficiency and spatial correlation based on regions along the belt and road in China. Sustainability 16 (7), 2990. doi:10.3390/su16072990
Liu, Y., Liu, M., Wang, G., Zhao, L., and An, P. (2021). Effect of environmental regulation on high-quality economic development in China—an empirical analysis based on dynamic spatial durbin model. Environ. Sci. Pollut. Res. 28 (39), 54661–54678. doi:10.1007/s11356-021-13780-2
Long, R., Li, H., Wu, M., and Li, W. (2021). Dynamic evaluation of the green development level of China's coal-resource-based cities using the TOPSIS method. Resour. Policy 74, 102415. doi:10.1016/j.resourpol.2021.102415
Lutz, C., Zieschank, R., and Drosdowski, T. (2017). Measuring Germany’s transition to a green economy. Low. Carbon Econ. 8 (1), 1–19. doi:10.4236/lce.2017.81001
Ma, X. F., Zhang, R., and Ruan, Y. F. (2023). How to evaluate the level of green development based on entropy weight TOPSIS: evidence from China. Int. J. Environ. Res. Public Health 20 (3), 1707. doi:10.3390/ijerph20031707
Maria, E., Marina, P., and Pavel, G. (2015). Global trends of «green» economy development as a factor for improvement of economical and social prosperity. Procedia-Social Behav. Sci. 166, 194–198. doi:10.1016/j.sbspro.2014.12.509
McGrattan, E. R., and Prescott, E. C. (2009). Openness, technology capital, and development. J. Econ. Theory 144 (6), 2454–2476. doi:10.1016/j.jet.2008.05.012
Mikhno, I., Koval, V., Shvets, G., Garmatiuk, O., and Tamošiūnienė, R. (2021). Green economy in sustainable development and improvement of resource efficiency. Cent. Eur. Bus. Rev. 2021, 99–113. doi:10.18267/j.cebr.252
Mu, Y., Huang, T., Saleem, N., and Hassan, A. H. (2024). Institutional pressure and low carbon innovation policy: the role of EMS, environmental interpretations and governance heterogeneity. Front. Environ. Sci. 12, 1385062. doi:10.3389/fenvs.2024.1385062
Norgaard, R. B. (1990). Economic indicators of resource scarcity: a critical essay. J. Environ. Econ. Manag. 19 (1), 19–25. doi:10.1016/0095-0696(90)90057-6
Pan, X., Li, J., Wei, J., Yue, Y., and Liu, L. (2022). Measuring green development level at a regional scale: framework, model, and application. Environ. Monit. Assess. 194 (5), 343. doi:10.1007/s10661-022-09953-2
Peng, Y., and Tao, C. (2022). Can digital transformation promote enterprise performance? from the perspective of public policy and innovation. J. Innovation and Knowl. 7 (3), 100198. doi:10.1016/j.jik.2022.100198
Salter, A., and Alexy, O. (2014). The nature of innovation. The Oxford handbook of innovation management. 1, 26–53.
Shang, Y., Niu, Y., and Song, P. (2023). Regional differences and influencing factors of green innovation efficiency in China’s 285 cities. Sustainability 16 (1), 334. doi:10.3390/su16010334
Söderholm, P. (2020). The green economy transition: the challenges of technological change for sustainability. Sustain. Earth 3 (1), 6. doi:10.1186/s42055-020-00029-y
Su, L. (2022). The impact of coordinated development of ecological environment and technological innovation on green economy: evidence from China. Int. J. Environ. Res. Public Health 19 (12), 6994. doi:10.3390/ijerph19126994
Sun, H., Chen, T., and Wang, C. N. (2024). Spatial impact of digital finance on carbon productivity. Geosci. Front. 15 (3), 101674. doi:10.1016/j.gsf.2023.101674
Tao, C. Q., and Xu, M. (2021). Measuring the allocation level of China innovation elements from the perspective of economic high-quality development. J. Quantitative and Tech. Econ. 38 (3), 3–22. (in Chinese). doi:10.13653/j.cnki.jqte.20210201.002
Wang, M., Zhao, X., Gong, Q., and Ji, Z. (2019). Measurement of regional green economy sustainable development ability based on entropy weight-topsis-coupling coordination degree—a case study in Shandong Province, China. Sustainability 11 (1), 280. doi:10.3390/su11010280
Wang, R., and Tan, J. (2021). Exploring the coupling and forecasting of financial development, technological innovation, and economic growth. Technol. Forecast. Soc. Change 163, 120466. doi:10.1016/j.techfore.2020.120466
Wang, Z. C., Xu, Y., and Tao, C. Q. (2023). The impact of innovation-driven policies on innovation factor mismatch: empirical evidence from national innovation-driven city pilot policies. Econ. research-Ekonomska istraživanja 36 (3). doi:10.1080/1331677X.2023.2177181
Wen, H., Liang, W., and Lee, C. C. (2023). China's progress toward sustainable development in pursuit of carbon neutrality: regional differences and dynamic evolution. Environ. Impact Assess. Rev. 98, 106959. doi:10.1016/j.eiar.2022.106959
Xu, M., Qi, Y., Tao, C., and Zhang, S. (2022). A random forest method for identifying the effectiveness of innovation factor allocation. Comput. Intell. Neurosci. 2022 (1), 1–16. doi:10.1155/2022/1135582
Xu, Y., and Ding, Z. (2024). Sustainable growth unveiled: exploring the nexus of green finance and high-quality economic development in China. Front. Environ. Sci. 12, 1414365. doi:10.3389/fenvs.2024.1414365
Xu, Y., Wang, Z. C., and Tao, C. Q. (2024). Can innovative pilot city policies improve the allocation level of innovation factors? Evidence from China. Technol. Forecast. Soc. Change 200, 123135. doi:10.1016/j.techfore.2023.123135
Xu, Y., and Zhao, J. (2021). Measurement of the coupling degree of innovation elements allocation and economic high-quality development in China. J. Quant. Tech. Econ. 38, 46–64. doi:10.13653/j.cnki.jqte.2021.10.003
Yang, G., Cheng, S., Gui, Q., and Chen, X. (2022a). The coupling and coordination characteristics and influencing factors of green innovation efficiency (GIE) and economic development levels in China. Sustainability 14 (21), 14085. doi:10.3390/su142114085
Yang, H., Wang, C., and Wang, G. (2023b). Convergence or dispersion? The impact of China’s innovative city pilot policy on regional innovation differences. Plos one 18 (4), e0281685. doi:10.1371/journal.pone.0281685
Yang, W., Hu, Y., Ding, Q., Gao, H., and Li, L. (2023a). Comprehensive evaluation and comparative analysis of the green development level of provinces in eastern and western China. Sustainability 15 (5), 3965. doi:10.3390/su15053965
Yang, Y., Wu, D., Xu, M., Yang, M., and Zou, W. (2022b). Capital misallocation, technological innovation, and green development efficiency: empirical analysis based on China provincial panel data. Environ. Sci. Pollut. Res. 29 (43), 65535–65548. doi:10.1007/s11356-022-20364-1
Yin, K., Zhang, R., Jin, X., and Yu, L. (2022). Research and optimization of the coupling and coordination of environmental regulation, technological innovation, and green development. Sustainability 14 (1), 501. doi:10.3390/su14010501
Ying, L., Li, M., and Yang, J. (2021). Agglomeration and driving factors of regional innovation space based on intelligent manufacturing and green economy. Environ. Technol. and Innovation 22, 101398. doi:10.1016/j.eti.2021.101398
Yu, H., Ke, H., Ye, Y., and Fan, F. (2023). Agglomeration and flow of innovation elements and the impact on regional innovation efficiency. Int. J. Technol. Manag. 92 (3), 229–254. doi:10.1504/IJTM.2023.128840
Zhang, L., Sun, H., Pu, T., Sun, H., and Chen, Z. (2024). Do green finance and hi-tech innovation facilitate sustainable development? Evidence from the Yangtze River Economic Belt. Econ. Analysis Policy 81, 1430–1442. doi:10.1016/j.eap.2024.02.005
Zhang, S., Luo, J., Huang, D. H., and Xu, J. (2023). Market distortion, factor misallocation, and efficiency loss in manufacturing enterprises. J. Bus. Res. 154, 113290. doi:10.1016/j.jbusres.2022.08.054
Keywords: green economy, innovation factor allocation, coupling and coordinated development, temporal and spatial characteristics, weighted TOPSIS method
Citation: Geng K, Zhang X, Jiang H and Zhuang Z (2024) The spatio-temporal characteristics and dynamic evolution of coupling and coordinated development of innovation factor allocation and green economy in China. Front. Environ. Sci. 12:1475508. doi: 10.3389/fenvs.2024.1475508
Received: 04 August 2024; Accepted: 01 November 2024;
Published: 19 November 2024.
Edited by:
Huaping Sun, Jiangsu University, ChinaCopyright © 2024 Geng, Zhang, Jiang and Zhuang. This is an open-access article distributed under the terms of the Creative Commons Attribution License (CC BY). The use, distribution or reproduction in other forums is permitted, provided the original author(s) and the copyright owner(s) are credited and that the original publication in this journal is cited, in accordance with accepted academic practice. No use, distribution or reproduction is permitted which does not comply with these terms.
*Correspondence: Kangshun Geng, Z2VuZ2thbmdzaHVuQHNxdS5lZHUuY24=