- 1School of Resource and Environmental Sciences, Wuhan University, Wuhan, China
- 2Collaborative Innovation Center of Geospatial Information Technology, Wuhan University, Wuhan, China
- 3College of Economics and Management, Northwest A&F University, Xianyang, China
Soil erosion is a land degradation process that may threaten the sustainability of ecosystem as well as cause severe social and economic problems. The studies on the effect of ecological restoration policies on soil erosion have been well documented, while the spatial relationships and spillover effects of the policies on soil erosion have been rarely scrutinized, though it is of great policy implications in soil erosion control. Based on the analysis of the spatio-temporal evolution of soil erosion in 107 counties of Shaanxi Province with Revised Universal Soil Loss Equation, this study employs a panel spatial Durbin model (SDM) with multi-source data for 107 counties from 2000 to 2015 to investigate the spatial effects of the Sloping Land Conversion Program (SLCP) on soil erosion control with investment data of SLCP. The results show that during 2000–2015, the average amount of soil erosion exhibited a significant downtrend contributed by the continuous investment of SLCP. The spatial economic results demonstrate that the own effect, as well as the spillover effect of investment on soil erosion control, is significant and positive. A 0.1 billion CNY increase in investment in a county will result in a reduction of the total soil erosion by 288.69 thousand t/a, of which 63% is contributed to the own direct effect on local soil erosion control and the 37% of it is contributed to the spillover effect on the neighbors. Moreover, rainfall has a significant and positive moderating effect on the mitigation effect of SLCP on soil erosion at county scale; the marginal contribution of investment of SLCP on soil erosion control in the county with less rainfall is greater than that with more rainfall. These findings contribute to further our understanding of the long-term effects of ecological restoration policies and transboundary ecological governance.
Introduction
Soil erosion is a widespread form of land degradation that severely threatens the ecosystem services, especially the terrestrial ecosystems globally (Pimentel, 2006; Amundson et al., 2015). The total amount of soil erosion caused by land use changes in the world increased by 2.5% from 2001 to 2012 (Borrelli et al., 2017). Similarly, soil erosion has been one of the most urgent environmental problems in China, with an estimated 2–4 billion tons of silt released into the Yangtze River and the Yellow River annually, of which 65% came from the sloping cropland (Bennett, 2008). This phenomenon was particularly obvious in the Loess Plateau, which was once regarded as the most heavily eroded area in the world (Wen and Deng, 2020). Therefore, there are many different forms of strategies including soil management (Cerdà et al., 2017), nature-based solutions (Keesstra et al., 2018), and landscape restoration programs (Kong et al., 2018) conducted to mitigate regional soil erosion around the world. Among them, the Sloping Land Conversion Program (SLCP) is a typical ecological restoration program initiated by the Chinese central government in 1999 with the stated environmental goals of reducing water and soil erosion (Bennett, 2008), also known as the Grain for Green Project in the form of payments for ecosystem services (PES). SLCP had encompassed more than 2000 counties in 25 provinces, benefiting over 41 million rural households and invested $55 billion to increase the forest cover, converting sloping cropland and degraded rangeland back to forest and grassland in the past 20 years (NFGA (National Forestry and Grassland Administration, 2018). Hence, it is significant to evaluate the effect of SLCP on soil erosion with such a huge investment for formulating scientific and reasonable soil erosion management policies and the sustainable development of regional ecosystems.
Revised Universal Soil Loss Equation (RUSLE) is by far one of the most widely applied soil erosion prediction models at a large regional scale around the world due to its simple form and parsimonious parameterization (Renard et al., 1997; Farhan and Nawaiseh, 2015). Besides, a variety of long-term satellite-derived remote sensing data and the development of the integrated geographic information system (GIS) have made it possible to assess the spatio-temporal characteristics of soil erosion with RUSLE at a regional scale more effectively and accurately (Vrieling, 2006; Poesen, 2018; Alewell et al., 2019). Some of the studies evaluated the dynamic spatio-temporal changes of soil erosion and indicated that the policies improved the ecosystem services by increasing vegetation cover and land use conversion (Xu et al., 2018). Although there are several environmental factors including topography, mean annual rainfall, land use and cover, and the soil conservation methods that have significant effects on soil erosion (Nearing, 1997; Zhao et al., 2022), land use still dominates the variation of soil loss and runoff, especially at the large regional scale (Zhao et al., 2022). Wen and Deng indicated that the change in land use and land cover induced by SLCP is the most critical factor that affects the soil erosion in the Loess Plateau after a literature review (Wen and Deng, 2020). The study in southern China’s red soil hilly region indicated that the soil loss was largest for cropland, while grassland and natural shrub have less soil loss and lower sediment values (Chen et al., 2021). Satellite-derived observations used in the studies mentioned above can capture the objective changes of soil erosion at the regional scale, but it is difficult to demonstrate that these changes are only caused by SLCP due to the contributions from the other ecological projects such as National Forest Conservation Program and Soil and Water Conservation Program (Li et al., 2020). While this problem could be solved partly in technic with econometric methods in which the missing variables are represented by the random error term, the research exploring the effect of ecological restoration policies on soil erosion as well as the impact of socio-economic factors with econometric methods is well documented (Rao et al., 2016; Li et al., 2020; Zhou et al., 2021). For instance, the increasing grain yield could mitigate soil erosion by diminishing the substantial demand for farmland and the excessive deprivation of ecological lands (Zhou et al., 2021); however, the area of forest and grassland converted from cropland or the vegetation cover (Kong et al., 2018; Zhou et al., 2021) was used to characterize the SCLP in many studies, which would lead to biases in the evaluation of policy effectiveness. To compensate for this deficiency, the investment of SLCP is used in this study to examine the effect of SLCP on soil erosion from the perspective of cost-effectiveness of investment, which is important for the sustainability and efficiency of the policy (Börner et al., 2017).
Soil erosion has a strong geographical dimension in the formation process whether at the watershed scale or at the regional scale (Ganasri and Ramesh, 2016; Rao et al., 2016); so, it tends to fall into dilemmas when evaluating the impact of the ecological restoration policy on soil erosion with administrative divisions. One of the dilemmas is that the ecological effects of SLCP such as mitigating soil erosion may not only remain in the implementation area but also diffuse into the neighboring area (Lin et al., 2020). Hence, we propose a hypothesis: the marginal effects of SLCP on soil erosion control may be the combination of mitigation effects of the local region with specific characteristics and spatial spillover effects of neighboring regions. However, regional policy and practice often completely ignore external beneficiaries like the adjacent administrative districts, which leave afforestation and management burdens on the local area when the spatial effects exist (Wolch et al., 2014). The classical econometric models are used in the studies mentioned above (Rao et al., 2016; Kong et al., 2018; Li et al., 2020) to examine the influencing factors of soil erosion in China with the omission of the spatial spillover effects of the afforestation, which could result in biased estimations and even undermine the scientific foundations (Jiang et al., 2020). Hence, it is necessary to examine the spillover effects of SLCP on soil erosion and separate the own and spatial spillover effects of afforestation at the regional scale with spatial econometric models for the effective transboundary ecological governance, which improves the cost-effectiveness of ecological governance and the sustainability of green development. Besides, the effect of rainfall on soil erosion is significant for all land use types (Zhao et al., 2022), and the rainfall has a significant threshold effect on the fiscal efficiency of SLCP (Liu and Yao, 2021). However, detailed mechanisms of the interaction role of rainfall and SLCP in soil erosion control are still unclear at the county scale.
Shaanxi Province, in inland northwest China, a pilot and demonstration province for SLCP since 1999 due to serious soil erosion problems in the 20th century, is selected as the study area. Due to the rapid economic development and the large-scale implementation of SCLP, it provides an ideal sample to examine the effect of SLCP on soil erosion. In this study, we employed RUSLE to evaluate the spatio-temporal variations of soil erosion over Shaanxi Province from 2000 to 2015 and then we construct a panel data set that spans yearly data on soil erosion estimated by RUSLE and investment data on SLCP as well as a range of natural and socio-economic variables for 107 counties in Shaanxi Province from 2000 to 2015. Different from most related studies, data that characterize SLCP are the investment of SLCP instead of the area of forest converted from cropland or other satellite-derived observations of vegetation cover. This shift from satellite-derived observations to investment data benefits improves the validity and reliability of the estimation of the marginal contribution of SLCP to soil erosion control. To fill up the knowledge gap mentioned above, the study manages to make two major contributions to the literature with a spatial panel model framework proposed by us with both socio-economic and natural factors concerned. Based on the spatial panel models, we first derive the possibly unbiased estimates for the marginal contribution of SLCP to soil erosion control with the investment variable after accounting for the existence of spatially autocorrelated disturbances. Secondly, we construct first-time empirical evidence regarding the own and spillover effects of SLCP on soil erosion and the direct and indirect paths of how SLCP has a spillover effect on neighboring counties.
Materials and Methods
Study Area
Shaanxi Province is located in the inland northwest of China (Figure 1), which spans 105° 29′–111° 15′ E and 31° 42′ –39°35′ N with a total area of 20.58 × 104 km2. There are obvious differences in climate and topography across this area, which divides this region into three sub-regions (Shaanbei, Guanzhong, Shannan). Shaanbei is the typical ecologically fragile area of the Loess Plateau. Guanzhong is a plain with a concentrated population and developed agriculture. Shannan is a mountain area with sufficient rainfall and dense vegetation.
SLCP started in Shaanxi Province as a pilot in 1999 to especially mitigate severe soil erosion in the 20th century, since 1999 the project has invested over 400 billion CNY for afforestation in this area, which improved the ecological environment—the statistical data from Shaanxi Forestry Bureau showed that the forest coverage increased from 30.92% in 1999 to 43.06% in 2015 and the sediment transport in the Loess Plateau decreased from 800 million tons in 2000 to nearly 400 million tons at present. Meanwhile, Shaanxi has experienced rapid urbanization and booming socio-economic development. During the period 2000–2015, Shaanxi’s GDP and proportion of the urban population had increased from 180.40 billion CNY to 1789.88 billion CNY and 27.80–53.92%, respectively. Therefore, it is an ideal region to explore the effect of SLCP on soil erosion under the interaction of socio-economic factors, policy, and natural factors.
As shown in Figure 2, we present the analysis procedure of the study for readers’ better understanding.
Revised Universal Soil Loss Equation
RUSLE is used to estimate the potential annual average soil erosion loss. In this study, all the input factors of RUSLE including rainfall, soil, topography, and land use and cover are transformed and calculated on ArcGIS10.5 software in GIS raster form (30 m resolution), with the annual soil erosion loss raster map produced by the tool of raster calculator on the GIS platform. The formula of RUSLE is as follows (Renard et al., 1997):
where A is the estimated average annual soil loss (t∙ha−1∙a−1), R is the rainfall erosivity factor (MJ∙mm∙ha−1 h−1∙a−1), K is the soil erodibility factor (t∙h∙MJ−1∙mm−1), LS is the terrain (slope length and steepness) factor (dimensionless), C is the cover and management factor (dimensionless), and p is the conservation practice factor (dimensionless).
The Rainfall Erosivity (R) Factor
The R reflects the power of soil separation and transportation caused by rainfall, which is the main driving force of soil erosion (Wischmeier and Smith, 1978). Based on the availability of data and the actual situation in the study area, this study calculates the R factor from Eq. 2, which is verified and applied by many similar studies in China (Yu and Rosewell, 1996; Zhang and Fu, 2003):
where p is the average annual rainfall (mm), R is the average rainfall erosivity (MJ∙mm∙hm−2∙h−1∙a−1) in many years, and α1 (α1 = 0.0668) and β1 (β1 = 1.6266) are model parameters.
The Soil Erodibility (K) Factor
The soil erodibility (K) factor is an index that represents the sensitivity of soil texture to soil erosion, determined by the soil physical properties like soil texture, soil structure, organic content, and permeability (Wischmeier and Smith, 1978). The most common equation is as follows:
where K (t∙h∙MJ−1∙mm−1) represents soil erodibility, N1 (0.002–0.100 mm) represents the percentage of silt (0.002–0.050 mm) plus very fine sand (0.050–0.100 mm), N2 (<0.002 mm) is the percentage of clay fraction, OM is the soil organic matter content (%), Sc and Pc are the codes of soil structure and permeability. Provided by the National Earth System Science Data Center of China, the K factor used in this study is calculated by the above equations, which are modified by the experimental observation data of the runoff plot.
The Slope Length and Steepness (LS) Factor
Numerous soil erosion studies on slopes or watershed scales show that terrain factors are the direct factors that induce soil erosion, and the LS factor reflects the impact of terrain factors on soil erosion, and its value is between 0 and 1. The calculation of LS in this study is based on the DEM data, using the modified soil erosion model terrain factor calculation tool proposed by Fu et al. (2015) to generate the LS factor map of the study area. The formulas are as follows:
where S is the slope gradient factor, θ is the slope of the grid cell (in degree), Li is the slope length factor of the grid cell i, λout and λin are the slope lengths of the outlet and entrance of the grid, and m is the slope length index determined by slope θ.
The Cover Management (C) factor
In RUSLE, the C factor reflects the impact of vegetation coverage and management measures on soil erosion, considered to be the most sensitive factor affecting soil erosion, ranging from 0 to 1. Based on the related research (Zhou et al., 2006; Yan et al., 2020), this study calculates the C factor with the Normalized Difference Vegetation Index (NDVI) as follows:
where C is the cover management factor, f is the vegetation coverage determined by NDVI, and NDVIs and NDVIv are the NDVI values for pure bare soil grid cell and pure vegetation grid cell, respectively.
The Conservation Practice (P) Factor
The p factor reflects the impact of conservation practice in different land types on reducing the erosion potential of the runoff; the value of p ranges from 0 to 1.0 means areas where no soil erosion occurs and one means areas where no conservation practice has been taken. This study assigns p values to different land use types according to land use maps and slope gradients based on research in similar areas in China (Li and Zheng, 2012): the p values of built-up land, water land, unused land, forest land, and grassland are 0, 0, 1, 1, and 1, respectively. The value of cultivated land is assigned according to different slope grades as shown in Table 1.
In order to validate the model’s regional applicability, we validated RUSLE with the soil erosion area in Shaanxi gathered from the First China Census for Water (Ministry of Water Resources of China (MWR), 2013). The validation results showed that our estimation of soil erosion area in Shaanxi was consistent with the results of the census (the consistency of results reaches 85%). Besides, we further compared the total amount of soil erosion in Shaanbei between our results and the study using the high-precision terrace data (Liu et al., 2019), and the simulations of RUSLE were significantly similar to the corresponding results in the study (the consistency of results reaches 78%). Therefore, we could confirm that RUSLE and its parameters were reasonable for the simulation of soil erosion in the study area based on the two validations above.
Spatial Econometric Methods
Spatial Autocorrelation
In this study, the spatial autocorrelation of soil erosion of counties in Shaanxi is measured by Moran’s I statistics, and then the spatial econometric analysis is employed to avoid biased and inconsistent estimation rather than the traditional econometric analysis (Anselin, 2013). Moran’s I can be expressed as:
where I is the value of Moran’s I, which ranges from -1 to 1. A positive value of Moran’s I indicates a positive spatial autocorrelation, a negative value implies a negative autocorrelation, and 0 means random spatial distribution. Y represents the variable of interest (i≠j), and
Spatial Econometric Models
We specify a generic spatial panel model as follows:
where TSEit denotes the total weight of soil erosion in county i in year t, INVit is the investment of SLCP in county i in year t, Xit is a vector of covariates, ci presents the unobserved county fixed effects (FEs) accounting for all time-invariant space-fixed variables whose omission in a typical cross-sectional analysis could lead to biased estimation, dt presents the unobserved time FEs accounting for all time-related effects whose omission could bias the estimates in time-series analysis, uit is a composite error term, and vit is an independently and identically distributed disturbance with zero mean and heteroscedastic variance. The (vectors of) parameters to be estimated in this model are ρ, α, λk, and δ. W is the spatial weight matrix, which is composed of the corresponding element Wij (the squared inverse geographic distance between two counties i and j with row standardized as suggested). Besides, to ensure the robustness of the estimation results in this study, a queen contiguity spatial weight matrix is used as an alternative spatial matrix in the model, in which the elements Wij equal one if there are common boundaries or points between county i and county j, 0 otherwise.
Besides, two interaction terms are added, respectively, to the following new type of soil erosion model:
where (RAINit * INVit) and (RAINit * NDVIit) are the interaction terms, while the coefficients β1 and β2 for the terms indicate how rainfall moderates the effect of INV and NDVI on soil erosion. The marginal effects of INV and NDVI on soil erosion control are as follows:
It is difficult to interpret the effect of investment of SLCP on soil erosion by its coefficient estimates (α) when explained variable lag exists. It is obvious that changes in investment in any county will affect the soil erosion in the county as well as the soil erosion in neighboring regions through the spatial lag effect. Therefore, partial differentiation needs to be used to decompose the model results to obtain direct and indirect effects if the coefficient ρ is not 0 (Lesage and Pace, 2010). The direct effect (own effect) refers to the average value of the explanatory variable’s influence on the explained variable in the local county, and the indirect effect refers to the average value of the explanatory variable’s influence on the explained variable in the neighboring counties, that is, the spatial spillover effect. The total effect is the sum of the two items. Hence, the generic spatial panel model can be transformed as follows to get the direct and indirect effects:
Let
and the spillover effects are:
Diagnostic Evaluations for Model Selection
Generally, there are several commonly used spatial econometric models: (17) spatial lag mode (SLM), (18) spatial error model (SEM), and (19) spatial Durbin model (SDM) (Hao & Liu, 2016). Their basic form can be written as:
where Y denotes the dependent variables, X is a matrix of explanatory variables
In this study, we choose SDM with spatial and time-period FEs to conduct the spatial econometric analysis after conducting the diagnostic evaluations like the likelihood ratio (LR), Lagrange multiplier (LM) test, and Hausman test (Khezri et al., 2021).
As shown in Table 2, the LM test’s null hypothesis is dismissed, and this can affirm the SLM and SEM. The Hausman test is used to explore which effects to choose in the model: FEs or random effects (REs), and the result indicates that the presence of FEs is verified at a 1% significance level in the SDM. In order to figure out whether the SDM can be simplified as the SLM or SEM, LR tests are conducted. The results show that the SDM cannot be simplified as the SLM; either the SEM or the SDM is the basis model to explore the effects of SLCP on soil erosion. Then, LR tests are conducted to examine the possibility of the spatial FEs and the time-period FEs in the model, and the results reject all the null hypothesis; the spatial and time-period FEs are chosen to continue with the estimation process.
Data Definitions and Sources
Variable Design
Explained variables are the average soil erosion modulus (SE) and the total soil erosion (TSE) in the county. The calculation result of the integration of RUSLE and GIS is a raster map of the soil erosion modulus, and SE is the average soil erosion modulus of the county obtained by the regional statistical function of the ArcGIS10.5 platform. The total soil erosion represents the total weight of soil erosion in the county, with the area of the region taken into consideration. What’s more, SE is used as the alternative explained variable to enhance the robustness of the model.
Explanatory variable is the total investment (INV) of SLCP. The initial and fundamental goal of SLCP is to alleviate soil erosion by afforestation; so, this study chooses the INV as the explanatory variable to verify the effects of SLCP on soil erosion, especially with the spatial spillover effect of variables. Since rainfall has an extremely important impact on soil erosion, this study chooses rainfall (RAIN) as the natural factor to test the regulating effects in the prevention and mitigation of soil erosion by SLCP.
According to the previous studies (Kong et al., 2018), we account for socio-economic factors on soil erosion by including the following variables as control variables: the urbanization rate (URBR) is the proportion of the built-up land of the total land, the industrial structure (IS) is the ratio of the gross industrial product to the gross domestic product as an indicator for industrialization, the GDP per capita (PGDP) represents the change of economic condition, grain production (CROP) is the total production of wheat, corn, and rice, and the rural per capita annual income (RPI) is the index reflecting the condition of the rural economic development. In addition, reflecting the growth status of vegetation with significant impact on soil erosion, the Normalized Vegetation Index (NDVI) is selected as the natural control variable accounting for regional natural resource endowments.
Data Source
There are 107 counties in Shaanxi as samples on the cross-sectional dimension of the panel data set used in the spatial econometric analysis in this study, and the time period is from 2000 to 2015 with 5 years gap in its time dimension with multiple sources of sample data. Soil erosion data are obtained from the result of RUSLE. The investment data of counties in Shaanxi come from the Central South Investigation and Planning Institute of State Forestry and Grassland Administration; the detailed calculation of these data is shown in the study of Liu et al. (Liu and Yao, 2021). The raster data of rainfall with a resolution of 1 km are from the Resources and Environmental Sciences Data Center of the Chinese Academy of Sciences (http://www.resdc.cn/). Land use and cover data with a resolution of 30 m are from the Resources and Environmental Sciences Data Center of the Chinese Academy of Sciences (http://www.resdc.cn/). The accuracy of land use classification is 94.3% with field verification and error correction (Liu et al., 2010). The soil erodibility factor raster data with a resolution of 30 m are from the National Earth System Science Data Center of China (http://www.geodata.cn/). Administrative boundaries of the counties are obtained from the national 1:1,000,000 database of China Geographic Information Monitoring Platform (http://www.dsac.cn/). The digital elevation model (DEM) data are SRTM data with a resolution of 30 m (http://www.resdc.cn/). The NDVI data are derived from the Chinese Geospatial Data Cloud (http://www.gscloud.cn/) with a resolution of 500 m. The socio-economic data such as GDP, population, and grain yield are all from the “Shaanxi Regional Statistical Yearbook.” The spatial data used in this study are uniformly resampled at a resolution of 30 m with the same spatial coordinate system (Krasovsky_1940_Albers). The design of each variable and the descriptive statistical analysis are reported in Table 3.
Results
Spatio-Temporal Characteristics of Soil Erosion
The temporal variations and the spatial distribution of soil erosion over Shaanxi from 2000 to 2015 are shown in Figure 3 and Figure 4. As shown in Figure 3, the total amount of the soil erosion in Shaanxi showed a downward trend from 2000 to 2015. Specifically, the total amount of soil erosion in 2000 was 341.66 million t, of which the largest proportion in Shaanbei was 54.21%, exceeding the sum of the proportions of Guanzhong and Shannan. It decreased to 282.61 million t in 2015, indicating that 17.29% of the soil erosion (341.66 million t in 2000) was mitigated over the 15-year period. And Shaanbei contributed 87.14% to this total mitigation (a reduction of 51.47 million t of soil erosion). Besides, we visualized the spatial distribution of soil erosion changes over Shaanxi from 2000 to 2015, as shown in Figure 3. The soil erosion in Shaanbei was more serious than in other sub-regions; however, the decrease in soil erosion in Shaanbei was also the most significant. Especially in Yan’an City, the soil erosion modulus of most counties was basically reduced by more than 5 t/ha/a. The soil erosion modulus was even reduced by more than 10 t/(ha∙a) in some specific areas with a dark green color in Figure 3. It is obvious that soil erosion had been effectively controlled during the period of SLCP, which is also consistent with similar studies (Liu, et al., 2019). At the same time, the soil erosion modulus of Changwu County, Xunyi County, Chunhua County, and Long County in the northern part of Guanzhong also decreased significantly, while the soil erosion reductions in Shannan were mainly scattered in some areas such as Langao County, Mian County, and Shiquan County. Besides, the spatial distribution of the total investment of SLCP in Shaanxi from 2000 to 2015 is visualized for a more intuitive understanding of the relationship between the total investment of SLCP and soil erosion changes at the county scale (Figure 5).
As shown in Figure 5, counties in Shaanbei like Ansai, Zhidan, Wuqi, and Zichang were the key area of SLCP, which got more investment than other counties, ranging from 0.87 to 1.79 billion CNY. This also coincided with the good performance in mitigating soil erosion in these counties. The investment of Shannan counties like Zhenba, Ningqiang, and Ziyang also reached a high level, more than 0.51 billion CNY, while most counties in Guanzhong had less investment in SLCP than other regions, and the decline of soil erosion modulus in these areas was not obvious either.
To test whether the distributions of soil erosion in Shaanxi counties exist, we conducted Moran’s I statistics on the ArcGIS (Table 4). As shown in Table 4, Moran’s I values are all positive in the study period and are basically greater than 0.6 at a 1% significance level, indicating that there is a strong positive spatial autocorrelation, which means that soil erosion in counties appears to be spatially dependent. Hence, it is necessary to use the spatial econometric methods in the next analysis.
Results of Spatial Econometric Models
The main results of estimating the factors influencing soil erosion with Eq. 11 are reported in Table 5. Besides, we also report results on estimations of non-spatial economic models adapted from Eq. 11 with conventional panel FEs and pooled OLS. As shown in Table 5, most of the model variables have a significant effect on soil erosion except for RAIN and IS; these results confirm our hypothesis that the heterogeneity in the investment of SLCP (INV), socio-economic, and natural characteristics can systematically explain differences in soil erosion. A highly positive significant coefficient for the spatial lag parameter of soil erosion (W*Y) indicates that a county’s total weight of soil erosion (TSE) is systematically affected by its geographical neighbors’ soil erosion status and the spatial spillover effect of soil erosion is significant; hence, there is an indirect spillover effect of INV in a county on TSE of its adjacent counties through the transboundary spillover of soil erosion. The coefficient of the investment of SLCP indicates a negative link between the investment of SLCP and soil erosion at a 1% significance level, which means that the increase of a county’s investment of SLCP will significantly reduce the TSE in that area. While the coefficient of INV (−23.256) in the panel fixed estimator is also significant but the absolute value of it is larger than that in SDM, which indicates the panel fixed model would overestimate the effect of INV on TSE with spatial effects ignored. The coefficient of INV is significantly negative in the pooled OLS, which contradicts our hypothesis that SLCP has alleviated soil erosion, so the pooled OLS is not suitable for carrying out the regression estimation in this study. As shown in the table, we used SE as the alternative explained variable to enhance the robustness of the SDM model (column 3 of Table 5), and the estimation results show consistent conclusion with the estimation in column 2 of Table 5.
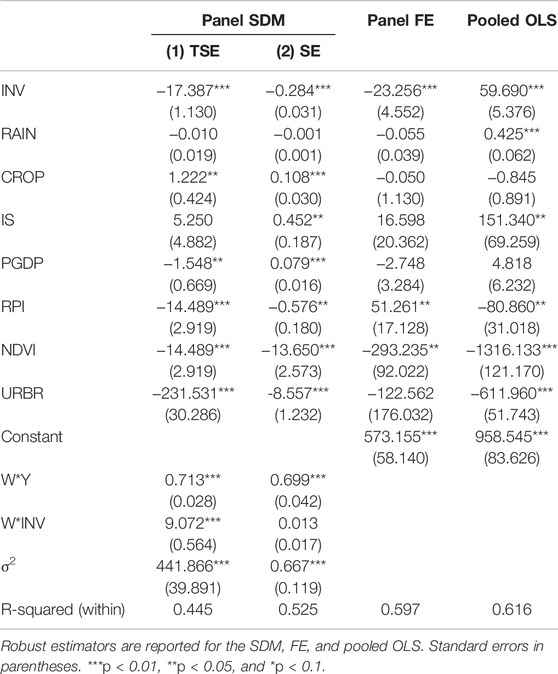
TABLE 5. Estimation results of the model specified in Eq. 11 with different specifications: panel SDM, panel FE, and pooled OLS.
The coefficient estimation for the spatial lag of INV is found to have a significant but positive impact on TSE, but it is difficult to interpret the effect of variables based on the coefficient estimate when the dependent variable lag exists because the coefficient is part of the recursive computation of marginal effects just as Equation 14 shows. Hence, we report the own and spillover effects as well as the average total impacts for the SDM in Table 61.
The own effect of INV on TSE is significant and negative, indicating that a county’s expected conditional mean of TSE would be reduced by 183.15 thousand t/a if the investment of SLCP in this county increases 0.1 billion CNY compared with other conditions remaining unchanged. In addition, the coefficient estimate for the spillover effects of INV is also negative and significant at the 1% level, which means the reduction of TSE in a county can be attributed to the afforestation of SLCP in its neighbors even if there is no spatial spillover effect dependence in TSE. Specifically, the conditional mean of TSE in a county would have depreciated by 105.54 thousand t/a less on average if the investment of SLCP in all other counties each increases 0.1 billion CNY. The total effect of INV in a county i represents the overall effect on TSE of county i from changing TSE by the same amount across all 107 counties. Every 0.1 billion CNY increase in the investment of SLCP in every county would, on average, imply a 288.69 thousand t/a lower in TSE.
In line with our expectations, the impact of NDVI on TSE is found to be negative and significant, indicating that the increase in vegetation coverage will slow down the soil erosion in the area. Hence, SLCP can prevent soil erosion by increasing vegetation coverage. Besides, the spillover effect of NDVI on TSE is also significant at the 1% level, which implies that the ecological effects of vegetation can benefit the neighboring areas through transboundary ways. However, we find a negative but insignificant effect of RAIN on TSE in all models mentioned in Table 5, which is contrary to RUSLE (Eq. 1), in which the rainfall has a positive and significant effect on soil erosion. This difference could be explained by the fact that the changes in rainfall are not significant for specific regions, especially in SDM with county FEs (while a significant and positive effect of RAIN on TSE is found in the pooled OLS specification).
The own effect of urbanization on TSE is negative and significant, indicating that urbanization has a positive effect on soil erosion prevention; indeed, other socio-economic factors like RPI and PGDP also have negative and significant coefficient estimates in the model, which means that the development of the regional economy will benefit the ecological environment, especially for the soil erosion. Besides, the coefficient of CROP indicates a positive link between CROP and TSE, implying that although high-intensity farming activities will increase food production, the disturbance to soil will also aggravate soil erosion. We argue that the estimation results of TSE are robust according to the estimation results of the alternative explained variable (SE) [as shown in columns (2), (4), and (6) of Table 6].
To check whether the RAIN moderates the effect of INV and NDVI on soil erosion, we report the estimation results with two interaction terms added in SDM in Table 7. The result of a positive coefficient on the interaction term between rainfall and investment of SLCP indicates that there is a negative moderating effect of RAIN on the alleviating effect of SLCP on soil erosion. As the RAIN increases, in magnitude, the marginal alleviating effect of INV on soil erosion decreases. Specifically, the increase in rainfall will increase the rainfall erosivity, thereby aggravating regional soil erosion, and the mitigation effect of INV on soil erosion will decrease. We also find that RAIN has a negative and significant moderating effect on the alleviating effect of NDVI on soil erosion, which means with the increase in rainfall, the role of NDVI in preventing soil erosion will be weakened. To sum up, the marginal contribution of the investment of SLCP is smaller in the area with more rainfall, like counties in Shannan than those in Shaanbei. The estimation results of the model with SE as the alternative explained variable indicate the robustness of our conclusion [as shown in columns (2) and (4) in Table 7].
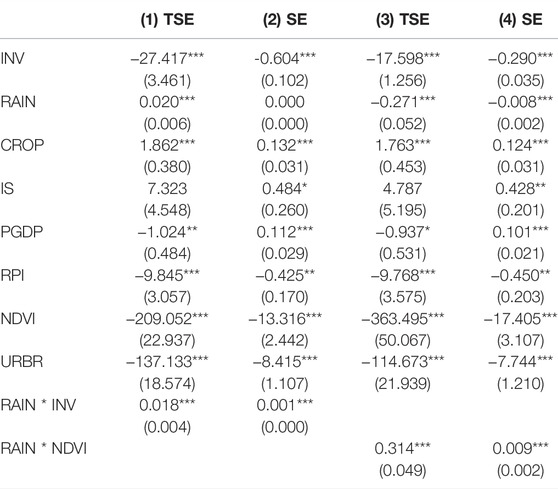
TABLE 7. The estimation results of model specified in Eq. 12.
Discussion
Marginal Contribution of SLCP to Soil Erosion Control and the Transboundary Path
The crucial role of SLCP in mitigating soil erosion has been widely recognized worldwide (Borrelli et al., 2017; Chen et al., 2021), but what cannot be ignored is to explore how soil erosion is controlled by SLCP from the perspective of marginal cost and income for the sustainability of the policy. In this study, we use the data of investment of SLCP to characterize the intensity of policy implementation and some indirect observation indicators like vegetation cover or the area of land use change (Rao et al., 2016; Zhou et al., 2021), which can maximally strip out the marginal contribution of the investment of SLCP to soil erosion with a specific SDM. It is of critical relevance for policymakers to figure out the cost-effectiveness of the investment on soil erosion control and make the next decision with reference to the result. Indeed, in research design, we draw upon the study by Kong et al. (2018), who explored the relationships among SLCP, the soil conservation service, and the socio-economic factors using structural equation modeling (SEM) with a cross section of 779 counties. However, their estimation result bias may arise from county heterogeneity. It is widely recognized that soil erosion is local by nature, influenced by both geographical and socio-economic factors (Ganasri and Ramesh, 2016; Rao et al., 2016), which makes it difficult to properly capture by the analysis of cross-sectional data. Hence, the spatial SDM with FEs proposed in this study is conducted to derive possibly unbiased estimates of the investment of SCLP in soil erosion control.
There are many studies exploring the factors affecting soil erosion at the regional scale (Alewell, et al., 2019; Zhou et al., 2021; Zhao et al., 2022), but the results of this article demonstrate that the spatial spillover effect of soil erosion is significant at the county scale, which has so far been overlooked by the literature mentioned above. It is significant to figure out how soil erosion in one region affects soil erosion in the neighboring regions for the effective transboundary ecological governance. According to the results of the panel SDM in this study, the spillover effect, as well as the own effect of investment in SLCP, is significant and positive on soil erosion control, which means soil erosion control in a county benefits not only from SLCP locally but also from the greening efforts of SLCP in its neighbors, and a joint effort in all counties has a much larger marginal effect on soil erosion control than unilateral ecological restoration effort in one county. Obviously, the separation of own from spillover impacts of SLCP on soil erosion provides solid evidence of the necessity of promoting “beneficiary pay” taxes and afforestation subsidies (Schomers and Matzdorf, 2013) in transboundary ecological governance, especially when it comes to this case that the soil erosion of a county has been mitigated by the green intervention efforts of SLCP in its neighboring counties without investing in these efforts by itself. Therefore, we argue that a well-functioning transboundary soil conservation system with collaborative green efforts from all stakeholders in the region is much more crucial and effective than the efforts only focusing on the local interests.
Impact of Socio-Economic and Natural Factors on Soil Erosion Control
Previous studies have highlighted the positive and significant link between urbanization and soil erosion control (Wang et al., 2020; Kong et al., 2018), which is consistent with our estimate results in Table 5. Due to rural–urban migration and the land use change in urbanization, the negative impacts of population on environmental degradation will be mitigated by alleviating the extent of disturbance to soils from farming (Grau and Aide, 2007; Deshingkar 2012), ultimately facilitating the mitigation of soil erosion. However, urbanization might aggravate other ecological problems like water pollution, carbon emission, and air pollution (Peng et al., 2017; Ke et al., 2020); so, it is necessary to balance ecosystem protection and urbanization. In addition, we also found that the economic growth, as well as the growth of rural per capita income, has a significant and positive effect on soil erosion control. The underlying reason may be that the growth of the economy provides enough funds for the government to take action on soil erosion control (Wang et al., 2020), while the growth of the rural economy will stimulate the farmers to depend less on the vulnerable ecosystem and adopt environment friendly farming methods to avoid damage to the environment. These results emphasized that policymakers should attach importance to the social and economic effects and the related interactions on ecosystem services and functions besides the direct goal of the ecological restoration to achieve a win–win situation for ecology and economy.
The rainfall has a significant impact on the cost-effectiveness of the investment in SLCP; for instance, Liu and Yao (Liu and Yao, 2021) pointed out that there is a distinct threshold effect of rainfall on the ecological effects (characterized by NDVI), while from the perspective of soil erosion control, rainfall has a significant moderating effect on the alleviating effect of investment of SLCP on soil erosion. The complexity of the interaction mechanisms lies in the different roles of rainfall in the process of soil erosion. On the one hand, the rainfall benefits the growth of plants, and then the increased vegetation cover will mitigate soil erosion (Chen et al., 2019). On the other hand, the rainfall also increases the erosivity of the soil, especially in Shaanbei and the typical arid and semi-arid areas in the Loess Plateau (Zhou et al., 2006). The results in our specific SDM indicate that the rainfall will weaken the effect of the investment of SLCP on soil erosion control at the county scale. Moreover, when the investment of SLCP is the same, the county with lower rainfall has a greater marginal contribution to soil erosion control than that with higher rainfall. Indeed, the investment of SLCP for soil erosion control should give priority to counties with intense soil erosion and lower rainfall considering its non-negligible direct spillover effects of soil erosion to neighbors and a much greater marginal effect of SLCP on soil erosion control. Therefore, it is necessary to scientifically identify the complex impact mechanism of climatic conditions on ecosystem services to improve the effectiveness and sustainability of ecological restoration policies.
Limitations of This Research
Admittedly, the green interventions of SLCP have a considerable contribution to soil erosion control, while the scale effect of the distribution of soil erosion and its evolution over time may exist due to the spatial heterogeneity within the region. In this study, to ensure the consistency of all multi-source data, we have to use the soil erosion data at the county scale in a spatial panel model framework, which may neglect the scale effect. Indeed, the small watersheds usually have the same physical–geographical features like climatic type, rainfall pattern, soil type, and vegetation (Chen et al., 2019); hence, further research could take our findings as a basic point to investigate the possibilities of integrating county-level statistics and watershed data. Besides, we only examined the effectiveness of SLCP on soil erosion; a question open to further investigation is how to comprehensively evaluate the efficiency of investment in ecological restoration projects with the existence of trade-offs and synergy among various ecosystem services.
Conclusion
In this study, we evaluated the effect of SLCP on soil erosion based on the specific spatial panel approach after the diagnostic evaluations in the selection process using the data of investment of SLCP. The conclusions were as follows: (1) the erosion intensity in Shaanxi province, especially in Shaanbei, exhibited a significant decreasing trend from 2000 to 2015 contributed by the continuous and comprehensive investment of SLCP. (2) The spatial econometric results demonstrate evidence of positive and economically meaningful effects of the investment of SLCP on soil erosion control, both locally and spatially in the study area. On the one hand, the investment in a county has a significant own effect on the soil erosion control locally. A 0.1 billion CNY increase in the investment in the county will diminish the expected conditional mean of TSE of it by 183.15 thousand t/a, with other conditions remaining unchanged. On the other hand, the soil erosion will be mitigated by the green efforts of SLCP from the neighboring counties due to the significant spillover effect of investment of SLCP on the soil erosion control and the spatial lag of soil erosion. (3) We confirm the moderating effect of rainfall on the mitigation effect of SLCP on soil erosion empirically at the county scale. The county with less rainfall performs better in soil erosion control with the same investment than that with more rainfall. These results indicate that it is necessary to establish a transboundary collaborative ecological governance system based on the long-term monitoring of both natural and socio-economic factors for improving the effectiveness of ecological restoration policy and the sustainability of the ecosystem.
Data Availability Statement
The original contributions presented in this study are included in the article/supplementary material; further inquiries can be directed to the corresponding author.
Author Contributions
YX: conceptualization, methodology, writing—original draft, and software; LW: writing—reviewing and editing and visualization; RA: writing—reviewing and editing and validation; XL: writing—reviewing and editing; YL: writing—reviewing; YLo: writing—reviewing and editing, conceptualization, and supervision; SY: conceptualization; and YLn: software and writing—reviewing.
Funding
This research was financially supported by the National Natural Science Foundation of China (Grant Numbers 41771432 and 71473195).
Conflict of Interest
The authors declare that the research was conducted in the absence of any commercial or financial relationships that could be construed as a potential conflict of interest.
Publisher’s Note
All claims expressed in this article are solely those of the authors and do not necessarily represent those of their affiliated organizations, or those of the publisher, the editors, and the reviewers. Any product that may be evaluated in this article, or claim that may be made by its manufacturer, is not guaranteed or endorsed by the publisher.
Footnotes
1Due to the magnitude of spatial effects, it depends critically on the spatial weight matrix W, which is defined a priori; we also report the estimation results of SDM with a queen contiguity matrix (provided in Appendix Table A1 in the Appendix), which did not lead to a qualitative change on the estimates for the own, spillover, and total effects.
Appendix A
References
Alewell, C., Borrelli, P., Meusburger, K., and Panagos, P. (2019). Using the USLE: Chances, Challenges and Limitations of Soil Erosion Modelling. Int. Soil Water Conservation Res. 7, 203–225. doi:10.1016/j.iswcr.2019.05.004
Amundson, R., Berhe, A. A., Hopmans, J. W., Olson, C., Sztein, A. E., and Sparks, D. L. (2015). Soil and Human Security in the 21st Century. Science 348 (6235), 1261071. doi:10.1126/science.1261071
Bennett, M. T. (2008). China's Sloping Land Conversion Program: Institutional Innovation or Business as Usual? Ecol. Econ. 65 (4), 699–711. doi:10.1016/j.ecolecon.2007.09.017
Börner, J., Baylis, K., Corbera, E., Ezzine-De-Blas, D., Honey-Rosés, J., Persson, U. M., et al. (2017). The Effectiveness of Payments for Environmental Services. World Dev. 96, 359–374. doi:10.1016/j.worlddev.2017.03.020
Borrelli, P., Robinson, D. A., Fleischer, L. R., Lugato, E., Ballabio, C., Alewell, C., et al. (2017). An Assessment of the Global Impact of 21st Century Land Use Change on Soil Erosion. Nat. Commun. 8, 2013. doi:10.1038/s41467-017-02142-7
B. Yu, B., and C. J. Rosewell, C. J. (1996). Technical Notes: A Robust Estimator of the R-Factor for the Universal Soil Loss Equation. Trans. ASAE 39 (2), 559–561. doi:10.13031/2013.27535
Cerdà, A., Rodrigo-Comino, J., Giménez-Morera, A., and Keesstra, S. D. (2017). An Economic, Perception and Biophysical Approach to the Use of Oat Straw as Mulch in Mediterranean Rainfed Agriculture Land. Ecol. Eng. 108, 162. doi:10.1016/j.ecoleng.2017.08.028
Chen, J., Li, Z., Xiao, H., Ning, K., and Tang, C. (2021). Effects of Land Use and Land Cover on Soil Erosion Control in Southern China: Implications from a Systematic Quantitative Review. J. Environ. Manag. 282, 111924. doi:10.1016/j.jenvman.2020.111924
Chen, J., Xiao, H., Li, Z., Liu, C., Wang, D., Wang, L., et al. (2019). Threshold Effects of Vegetation Coverage on Soil Erosion Control in Small Watersheds of the Red Soil Hilly Region in China. Ecol. Eng. 132, 109–114. doi:10.1016/j.ecoleng.2019.04.010
Deshingkar, P. (2012). Environmental Risk, Resilience and Migration: Implications for Natural Resource Management and Agriculture. Environ. Res. Lett. 7, 015603. doi:10.1088/1748-9326/7/1/015603
Farhan, Y., and Nawaiseh, S. (2015). Spatial Assessment of Soil Erosion Risk Using RUSLE and GIS Techniques. Environ. Earth Sci. 74 (6), 4649–4669. doi:10.1007/s12665-015-4430-7
Fu, S., Liu, B., Zhou, G., Sun, Z., and Zhu, X. (2015). Calculation Tool of Topographic Factors. Sci. Soil Water Conservation 13 (5), 105doi:10.16843/j.sswc.2015.05.018
Ganasri, B. P., and Ramesh, H. (2016). Assessment of Soil Erosion by RUSLE Model Using Remote Sensing and GIS - A Case Study of Nethravathi Basin. Geosci. Front. 7 (6), 953–961. doi:10.1016/j.gsf.2015.10.007
Grau, H. R., and Aide, T. M. (2007). Are Rural-Urban Migration and Sustainable Development Compatible in Mountain Systems? Mt. Res. Dev. 27 (2), 119–123. doi:10.1659/mrd.0906
Hao, Y., and Liu, Y.-M. (2016). The Influential Factors of Urban PM2.5 Concentrations in China: a Spatial Econometric Analysis. J. Clean. Prod. 112, 1443–1453. doi:10.1016/j.jclepro.2015.05.005
Jiang, L., He, S., Cui, Y., Zhou, H., and Kong, H. (2020). Effects of the Socio-Economic Influencing Factors on SO2 Pollution in Chinese Cities: A Spatial Econometric Analysis Based on Satellite Observed Data. J. Environ. Manag. 268, 110667. doi:10.1016/j.jenvman.2020.110667
Ke, X., Zhou, Q., Zuo, C., Tang, L., and Turner, A. (2020). Spatial Impact of Cropland Supplement Policy on Regional Ecosystem Services under Urban Expansion Circumstance: a Case Study of Hubei Province, China. J. Land Use Sci. 15 (5), 673–689. doi:10.1080/1747423X.2020.1817166
Keesstra, S., Nunes, J., Novara, A., Finger, D., Avelar, D., Kalantari, Z., et al. (2018). The Superior Effect of Nature Based Solutions in Land Management for Enhancing Ecosystem Services. Sci. Total Environ. 610-611, 997–1009. doi:10.1016/j.scitotenv.2017.08.077
Khezri, M., Karimi, M. S., Khan, Y. A., and Abbas, S. Z. (2021). The Spillover of Financial Development on CO2 Emission: A Spatial Econometric Analysis of Asia-Pacific Countries. Renew. Sustain. Energy Rev. 145, 111110. doi:10.1016/j.rser.2021.111110
Kong, L., Zheng, H., Rao, E., Xiao, Y., Ouyang, Z., and Li, C. (2018). Evaluating Indirect and Direct Effects of Eco-Restoration Policy on Soil Conservation Service in Yangtze River Basin. Sci. Total Environ. 631-632, 887–894. doi:10.1016/j.scitotenv.2018.03.117
Lesage, J. P., and Pace, R. K. (2010). “Spatial Econometric Models,” in Handbook of Applied Spatial Analysis: Software Tools, Methods and Applications. Editors M. M. Fischer, and A. Getis (Berlin, Heidelberg: Springer Berlin Heidelberg). doi:10.1007/978-3-642-03647-7_18
Li, T., and Zheng, L. (2012). Soil Erosion Changes in the Yanhe Watershed from 2001 to 2010 Based on RUSLE Model. J. Nat. Resour. 27 (7), 1164. Available at: https://kns.cnki.net/kcms/detail/detail.aspx?FileName=ZRZX201207008andDbName=CJFQ2012
Li, Z., Ning, K., Chen, J., Liu, C., Wang, D., Nie, X., et al. (2020). Soil and Water Conservation Effects Driven by the Implementation of Ecological Restoration Projects: Evidence from the Red Soil Hilly Region of China in the Last Three Decades. J. Clean. Prod. 260, 121109. doi:10.1016/j.jclepro.2020.121109
Lin, Y., Yang, X., Li, Y., and Yao, S. (2020). The Effect of Forest on PM2.5 Concentrations: A Spatial Panel Approach. For. Policy Econ. 118, 102261. doi:10.1016/j.forpol.2020.102261
Liu, J., Zhang, Z., Xu, X., Kuang, W., Zhou, W., Zhang, S., et al. (2010). Spatial Patterns and Driving Forces of Land Use Change in China during the Early 21st Century. J. Geogr. Sci. 20 (4), 483–494. doi:10.1007/s11442-010-0483-4
Liu, S., and Yao, S. (2021). The Effect of Precipitation on the Cost-Effectiveness of Sloping Land Conversion Program: A Case Study of Shaanxi Province, China. Ecol. Indic. 132, 108251. doi:10.1016/j.ecolind.2021.108251
Liu, W., Liu, J., and Kuang, W. (2019). Spatio-temporal Patterns of Soil Protection Effect of the Grain for Green Project in Northern Shaanxi. Acta Geogr. Sin. 74 (09), 1835. Available at: https://kns.cnki.net/kcms/detail/11.1856.P.20190920.1554.022.html.
Ministry of Water Resources of China (MWR) (2013). Bulletin of Soil and Water Conservation in First China Census for Water. Soil Water Conserv. 10, 2. Available at: http://www.mwr.gov.cn/sj/tjgb/zgstbcgb/201612/t20161222_776093.html.
Nearing, M. A. (1997). A Single, Continuous Function for Slope Steepness Influence on Soil Loss. Soil Sci. Soc. Am. J. 61 (3), 917–919. doi:10.2136/sssaj1997.03615995006100030029x
NFGA (National Forestry and Grassland Administration (2018). China Forestry Statistical Yearbook 2017. Beijing: China Forestry Press.
Peng, J., Tian, L., Liu, Y., Zhao, M., Hu, Y. n., and Wu, J. (2017). Ecosystem Services Response to Urbanization in Metropolitan Areas: Thresholds Identification. Sci. Total Environ. 607-608, 706–714. doi:10.1016/j.scitotenv.2017.06.218
Pimentel, D. (2006). Soil Erosion: A Food and Environmental Threat. Environ. Dev. Sustain 8 (1), 119–137. doi:10.1007/s10668-005-1262-8
Poesen, J. (2018). Soil Erosion in the Anthropocene: Research Needs. Earth Surf. Process. Landforms 43, 64–84. doi:10.1002/esp.4250
Rao, E., Xiao, Y., Ouyang, Z., and Zheng, H. (2016). Changes in Ecosystem Service of Soil Conservation between 2000 and 2010 and its Driving Factors in Southwestern China. Chin. Geogr. Sci. 26 (2), 165–173. doi:10.1007/s11769-015-0759-9
Renard, K. G., Foster, G. R., Weesies, G. A., Mccool, D. K., and Yoder, D. C. (1997). “Predicting Soil Erosion by Water: A Guide to Conservation Planning with the Revised Universal Soil Loss Equation (RUSLE),” in Agriculture Handbook 703 (Washington: United States Department of Agriculture), 404.
Schomers, S., and Matzdorf, B. (2013). Payments for Ecosystem Services: A Review and Comparison of Developing and Industrialized Countries. Ecosyst. Serv. 6, 16–30. doi:10.1016/j.ecoser.2013.01.002
Vrieling, A. (2006). Satellite Remote Sensing for Water Erosion Assessment: A Review. Catena 65 (1), 2–18. doi:10.1016/j.catena.2005.10.005
Wang, L., Yan, H., Wang, X. W., Wang, Z., Yu, S. X., Wang, T. W., et al. (2020). The Potential for Soil Erosion Control Associated with Socio-Economic Development in the Hilly Red Soil Region, Southern China. Catena 194, 104678. doi:10.1016/j.catena.2020.104678
Wen, X., and Deng, X. (2020). Current Soil Erosion Assessment in the Loess Plateau of China: A Mini-Review. J. Clean. Prod. 276, 123091. doi:10.1016/j.jclepro.2020.123091
Wischmeier, W. H., and Smith, D. D. (1978). Predicting Rainfall Erosion Losses - a Guide to Conservation Planning, Agriculture Handbook No. 537. Washington D.C: USDA/Science and Education Administration, US. Govt. Printing Office.
Wolch, J. R., Byrne, J., and Newell, J. P. (2014). Urban Green Space, Public Health, and Environmental Justice: The Challenge of Making Cities 'just Green Enough'. Landsc. Urban Plan. 125, 234–244. doi:10.1016/j.landurbplan.2014.01.017
Xu, G., Zhang, J., Li, P., Li, Z., Lu, K., Wang, X., et al. (2018). Vegetation Restoration Projects and Their Influence on Runoff and Sediment in China. Ecol. Indic. 95, 233–241. doi:10.1016/j.ecolind.2018.07.047
Yan, H., Wang, L., Wang, T. W., Wang, Z., and Shi, Z. H. (2020). A Synthesized Approach for Estimating the C-Factor of RUSLE for a Mixed-Landscape Watershed: A Case Study in the Gongshui Watershed, Southern China. Agric. Ecosyst. Environ. 301, 107009. doi:10.1016/j.agee.2020.107009
Zhang, W., and Fu, J. (2003). Rainfall Erosivity Estimation under Different Rainfall Amount. Resour. Sci. 25 (1), 35. Available at: https://kns.cnki.net/kcms/detail/detail.aspx?FileName=ZRZY200301005andDbName=CJFQ2003.
Zhao, J., Wang, Z., Dong, Y., Yang, Z., and Govers, G. (2022). How Soil Erosion and Runoff Are Related to Land Use, Topography and Annual Precipitation: Insights from a Meta-Analysis of Erosion Plots in China. Sci. Total Environ. 802, 149665. doi:10.1016/j.scitotenv.2021.149665
Zhou, M., Deng, J., Lin, Y., Zhang, L., He, S., and Yang, W. (2021). Evaluating Combined Effects of Socio-Economic Development and Ecological Conservation Policies on Sediment Retention Service in the Qiantang River Basin, China. J. Clean. Prod. 286, 124961. doi:10.1016/j.jclepro.2020.124961
Keywords: soil erosion, Sloping Land Conversion Program, spillover effect, spatial panel model, RUSLE
Citation: Xie Y, Wang L, An R, Luo X, Lu Y, Liu Y, Yao S and Liu Y (2022) The Effect of Sloping Land Conversion Program on Soil Erosion in Shaanxi Province, China: A Spatial Panel Approach. Front. Environ. Sci. 10:913712. doi: 10.3389/fenvs.2022.913712
Received: 06 April 2022; Accepted: 19 April 2022;
Published: 30 May 2022.
Edited by:
Qingsong He, Huazhong University of Science and Technology, ChinaReviewed by:
Penglai Ran, China University of Geosciences Wuhan, ChinaQiushi Zhou, Zhejiang University, China
Copyright © 2022 Xie, Wang, An, Luo, Lu, Liu, Yao and Liu. This is an open-access article distributed under the terms of the Creative Commons Attribution License (CC BY). The use, distribution or reproduction in other forums is permitted, provided the original author(s) and the copyright owner(s) are credited and that the original publication in this journal is cited, in accordance with accepted academic practice. No use, distribution or reproduction is permitted which does not comply with these terms.
*Correspondence: Yaolin Liu, eWFvbGluNjEwQDE2My5jb20=