- 1Biology Department, University of Regina, Regina, SK, Canada
- 2Institute of Environmental Change and Society, University of Regina, Regina, SK, Canada
- 3School of Environment and Sustainability and Global Institute for Water Security University of Saskatchewan, Saskatoon, SK, Canada
Inland waters are important global sources, and occasional sinks, of CO2, CH4, and N2O to the atmosphere, but relatively little is known about the contribution of GHGs of constructed waterbodies, particularly small sites in agricultural regions that receive large amounts of nutrients (carbon, nitrogen, phosphorus). Here, we quantify the magnitude and controls of diffusive CO2, CH4, and N2O fluxes from 20 agricultural reservoirs on seasonal and diel timescales. All gases exhibited consistent seasonal trends, with CO2 concentrations highest in spring and fall and lowest in mid-summer, CH4 highest in mid-summer, and N2O elevated in spring following ice-off. No discernible diel trends were observed for GHG content. Analyses of GHG covariance with potential regulatory factors were conducted using generalized additive models (GAMs) that revealed CO2 concentrations were affected primarily by factors related to benthic respiration, including dissolved oxygen (DO), dissolved inorganic nitrogen (DIN), dissolved organic carbon (DOC), stratification strength, and water source (as δ18Owater). In contrast, variation in CH4 content was correlated positively with factors that favoured methanogenesis, and so varied inversely with DO, soluble reactive phosphorus (SRP), and conductivity (a proxy for sulfate content), and positively with DIN, DOC, and temperature. Finally, N2O concentrations were driven mainly by variation in reservoir mixing (as buoyancy frequency), and were correlated positively with DO, SRP, and DIN levels and negatively with pH and stratification strength. Estimates of mean CO2-eq flux during the open-water period ranged from 5,520 mmol m−2 year1 (using GAM-predictions) to 10,445 mmol m−2 year−1 (using interpolations of seasonal data) reflecting how extreme values were extrapolated, with true annual flux rates likely falling between these two estimates.
1 Introduction
Inland waters are often sources of greenhouse gases (GHG) to the atmosphere as a result of net emissions of carbon dioxide (CO2), methane (CH4), and nitrous oxide (N2O) (Cole et al., 2007; Tranvik et al., 2009; DelSontro et al., 2018). However, recent research, including that on hard-water prairie ecosystems, suggests that the magnitude, spatial extent, and timing of emissions varies greatly among locales (Finlay et al., 2010; Finlay et al., 2015; Webb et al., 2019a; Webb et al., 2019b; Finlay et al., 2019). Major drivers of GHGs have been shown to be linked to nutrient status (carbon, nitrogen, and phosphorus), primary productivity, surface area, temperature, and latitude (Downing 2010; Deemer et al., 2016; Holgerson and Raymond 2016; DelSontro et al., 2018). Complete accounting of the role of inland waters in the global carbon budget needs to better characterize such temporal variability in different regions and waterbody types, particularly with respect to the cumulative effects of main greenhouse gases.
Waterbodies in agricultural areas are of particular interest in greenhouse gas dynamics as they have elevated nutrient loading reflecting elevated fertilizer application and livestock densities to meet the growing demand of global human populations (Tilman 1999; Earles et al., 2012; Baumann et al., 2017). Small agricultural reservoirs (also referred to as dams, impoundments, or dugouts) appear to vary dramatically in terms of the magnitude and direction of GHG fluxes. For example, Australian farm reservoirs emit large amounts of CO2 and CH4 to the atmosphere, but only small quantities of N2O (Ollivier et al., 2018; Ollivier et al., 2019). In contrast, agricultural reservoirs of the northern Great Plains of North America are often sinks for CO2 (52% of sites) and N2O (67%), but are consistent net sources of CH4 (Webb et al., 2019a; Webb et al., 2019b). Unfortunately, most of these surveys are based on single samples in late-summer (August) samples (Maberly 1996; Dillon and Molot 1997; Baehr and DeGrandpre 2004; Ducharme-Riel et al., 2015; Denfeld et al., 2016; Finlay et al., 2019), raising questions of the role of seasonal variability in influencing net annual carbon sources.
Understanding the mechanistic controls of aquatic CO2, CH4, and N2O concentrations is critical for upscaling efforts, predictions of future climate change effects, and management opportunities to use these systems as carbon offsets (Bastviken et al., 2004; DelSontro et al., 2016). Temporal dynamics of CO2 are usually correlated with changes in either microbial metabolism or the supply of inorganic carbon to freshwaters (Chapin et al., 2006; Dubois et al., 2009; McDonald et al., 2013; Bogard and Giorgio 2016; Wilkinson et al., 2016). In boreal ecosystems, the main pathway of CO2 production and consumption is through photosynthesis (P, consumption) and respiration (R, production), with CO2 evasion occurring when P < R (Chapin et al., 2006; Bogard and Giorgio 2016). However, this metabolic control can be overridden in non-boreal systems due to substantial inputs of inorganic C (e.g., DIC in runoff or groundwater) or changes in pH and carbonate chemistry (Dubois et al., 2009; Finlay et al., 2010; McDonald et al., 2013; Bogard and Giorgio 2016; Wilkinson et al., 2016). For example, in small prairie hardwater systems, CO2 concentration are regulated by a complex interaction between primary production, DIC content, nutrient status, stratification intensity, groundwater fluxes, and regional edaphic conditions (Webb et al., 2019b). Together, these models suggest that seasonal variation in the interaction among control factors is likely to create significant temporal variation in net CO2 fluxes.
Methane is produced by methanogenic bacteria through the anaerobic degradation of organic matter, most often in the anoxic or hypoxic sediments of waterbodies (Glass and Orphan 2012). This CH4 can be released to the atmosphere through diffusion, ebullition, or plant-mediated fluxes. Rates of CH4 production are highly dependent on temperature (Q10 ∼ 4; range 1–35) (Yvon-Durocher et al., 2014; DelSontro et al., 2016), whereas CH4 can be consumed by methane-oxidizing bacteria (“methanotrophy”) under oxic conditions (King 1992; Duc et al., 2010). Gas concentrations are also influenced by other factors including organic carbon availability and quality (Segers 1998; Bridgham et al., 2006), mineral nutrient availability, and productivity (Deemer et al., 2016; DelSontro et al., 2018; Ollivier et al., 2018; Beaulieu et al., 2019) and competitive inhibition by sulfur-reducing bacteria (Lovley and Klug 1983; Webb et al., 2019b). Landscape models of farm reservoirs suggest that lowest CH4 concentrations occur with DO supersaturation, low sediment C:N ratios, reduced dissolved inorganic nitrogen (DIN) concentrations, intermediate water residence time, elevated groundwater inflow, and higher conductivity levels (Webb et al., 2019b). Taken together, these observations suggest that seasonal CH4 production in agricultural ponds will reach a maximum in late summer, when temperature, productivity, thermal stratification and deepwater anoxia are greatest.
Production of N2O arises as an intermediary product during nitrification (Quick et al., 2019), incomplete denitrification (Firestone and Davidson 1989; Glass and Orphan 2012), or dissimilatory nitrate reduction to NH4+ (DNRA; Scott et al., 2008), whereas denitrification consumes N2O (Quick et al., 2019). Previous research suggests that temporal controls of N2O flux include DO saturation, organic carbon availability, nitrogen availability and speciation, pH, and temperature (Baulch et al., 2011; Gooding & Baulch 2017; Quick et al., 2019; Zhang et al., 2021). Spatial analysis of variation in N2O emissions from small agricultural reservoirs reveals N2O concentrations were lowest when thermal stratification was strong, phytoplankton biomass (as Chl-a) was elevated, and DO was supersaturated (Webb et al., 2019a). Although N2O emissions from prairie wetlands are known to be high in spring (Pennock et al., 2010) when DOC and NOx is abundant, there have been few systematic studies of the controls of seasonal variation in the regulation of N2O fluxes.
Thus, while previous research provides some mechanistic predictions for GHG fluxes from small waterbodies, it is difficult to anticipate how all three gases will sum to a CO2-equivalent (CO2-eq) flux over the entire open water season. This study presents a seasonal and diel assessment of the magnitude of CO2, CH4, and N2O concentration and diffusive flux, and the factors controlling the seasonal concentrations of these GHGs across 20 agricultural reservoirs in the northern Great Plains, the largest farming region in Canada. This study builds on previous work which found that some regional lakes sequester CO2 (Finlay et al., 2009; Finlay et al. 2010; Finlay et al. 2015; Finlay et al. 2019), as well as previous spatial surveys of 101 agricultural reservoirs that found highly variable fluxes of CO2 and CH4, but general undersaturation of N2O (Webb et al., 2019a; Webb et al., 2019b). The goals of this study were to: 1) quantify the change in concentrations of CO2, CH4, and N2O on seasonal and diel timescales in agricultural reservoirs; 2) identify the seasonal drivers of CO2, CH4, and N2O concentrations in these agricultural reservoirs, and; 3) estimate the total annual CO2 equivalence contribution of GHG to the atmosphere from these systems. Based on earlier work, we anticipated that the reservoirs would be consistent sinks for CO2 and N2O, with only notable release in spring and late fall (Finlay et al., 2015; Finlay et al., 2019), that GHG release varied inversely with stratification intensity (Webb et al., 2019a), and that CH4 may be the main control of net CO2-eq exchanges in these systems (Pennock et al., 2010; Webb et al., 2019b).
2 Methods
Twenty constructed agricultural reservoirs were chosen for this study (Figure 1). Agricultural reservoirs, known regionally as “dugouts”, typically have a rectangular construction, with steep sloping sides (1.5:1) and a maximum depth of 4–6 m (Webb et al., 2019b). All reservoirs are located in southern Saskatchewan, Canada, within a 150 km radius of the City of Regina (50° 27′ 10.19″ N, −104° 36’ 14.39″ W), as a previous survey of 100 regional reservoirs did not identify significant spatial component to regulation of GHG concentrations (Webb et al., 2019a; Webb et al., 2019b). Sites were selected to encompass a wide range of physical and chemical parameters (Table 1 and Table 2) based on data collected in 2017. Reservoirs were sampled four times during 2018; in late April when 75% of ice cover had melted, mid-May when sites were entirely ice-free, mid-July, and at the end of September prior to October ice formation. Five sites were also sampled in June and August to evaluate the effect of increased temporal resolution on determination of seasonal patterns in GHG content and fluxes.
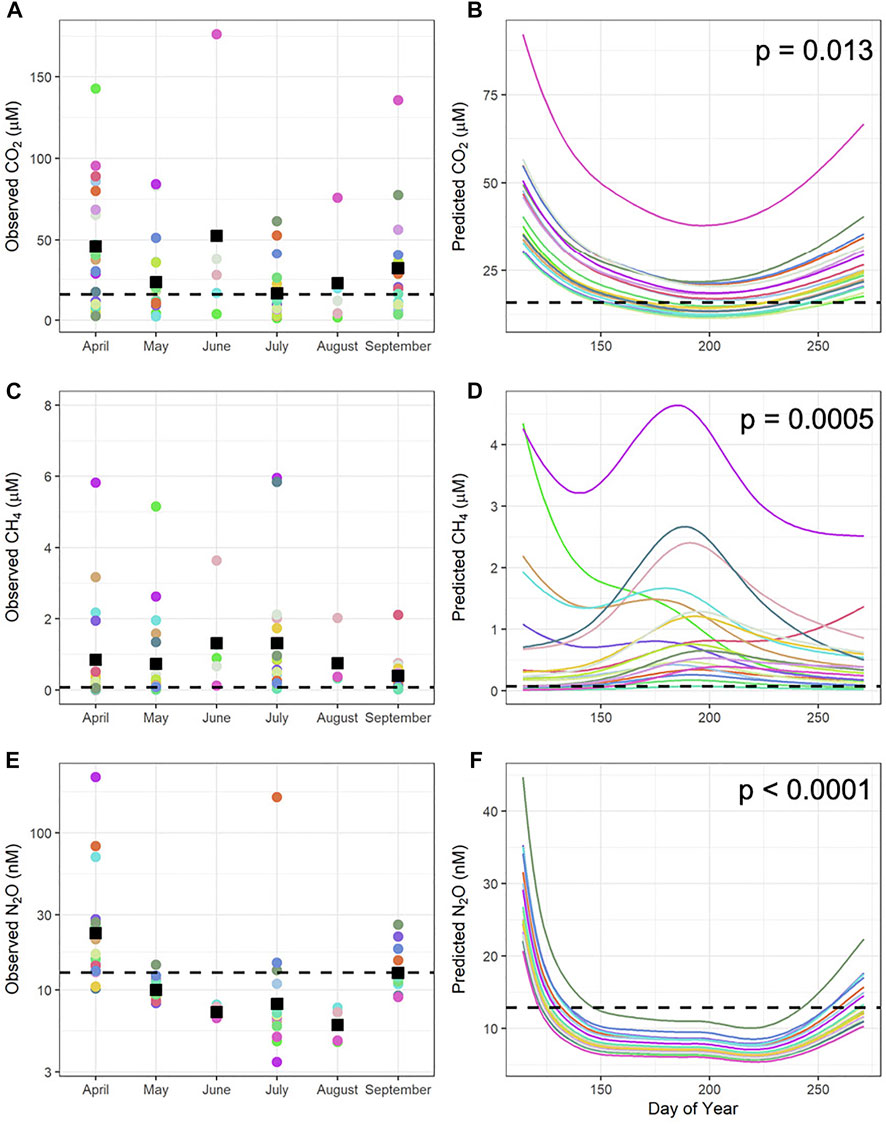
FIGURE 1. Seasonal carbon dioxide (CO2, (A,B)), methane (CH4, (C,D)), and nitrous oxide (N2O, (E,F)) concentrations from 20 reservoirs in 2018. Left column: Raw observed GHG data for all three gases. Each coloured circle is one reservoir. The black squares represent the monthly mean. This data was used to inform the models. Right column: The modelled seasonal gas concentration from a generalized additive model (GAM) of all three gases. Each coloured line represents a single reservoir. p-values for the GAM are located at the top of each plot. Black dashed line in both panels represents the calculated (from ppm) atmospheric concentration of each gas. The colour in the left panel corresponds to the coordinating colour in the right panel. Deviance explained = 36.6% for CO2, 88.7% for CH4, and 90.2% for N2O.
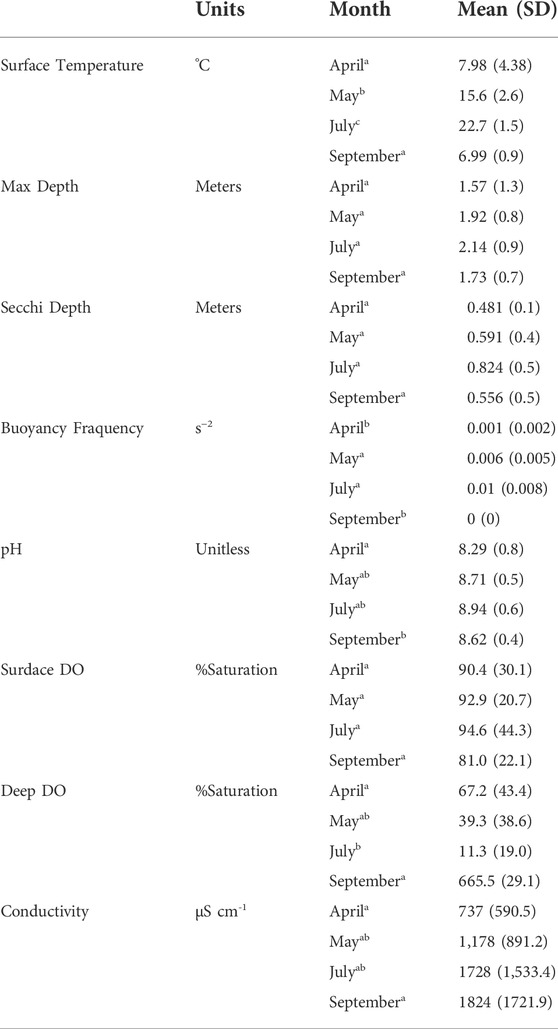
TABLE 1. Physical and environmental parameters measured in the 20 reservoirs monthly in 2018. All parameters, excluding max depth, Secchi depth, and buoyancy frequency were measured using the YSI multi-parameter probe at 0.5 m below the water surface, excluding deep dissolved oxygen which was taken 0.5 m above the sediment. Presented as mean ± standard deviation (SD). Superscript letters denote significant differences between months based on ANOVA and post-hoc Tukey test. DO = Dissolved Oxygen.
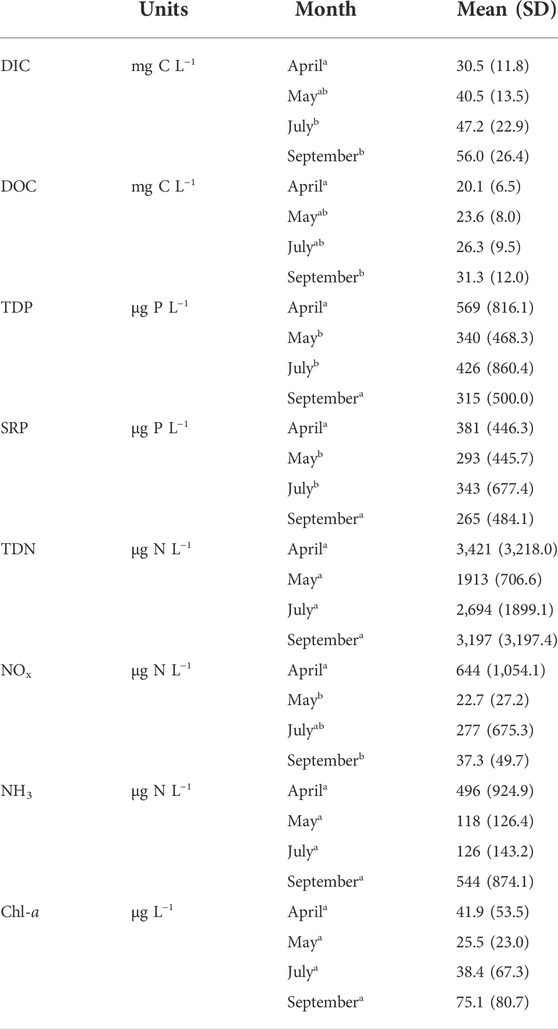
TABLE 2. Carbon and nutrient concentrations for each month in the 20 reservoirs in 2018. Presented as mean ± standard deviation (SD). Superscript letters denote significant differences based on ANOVA and post-hoc Tukey test. DIC = dissolved inorganic carbon. DOC = dissolved organic carbon. TDP = total dissolved phosphorus. SRP = soluble reactive phosphorus. TDN = total dissolved nitrogen. NOx = nitrate and nitrite concentration. NH3 = ammonia concentration. Chl-a = chlorophyll a concentration. Dissolved inorganic nitrogen (DIN) is the sum of NOx and NH3.
2.1 Field collection
2.1.1 Seasonal sampling
During each sampling period, samples were collected within a 10-day interval, and all collections were made during daylight hours. Each site was sampled from a canoe, anchored near the center of the reservoir over the deepest water. Water temperature (°C), DO (mg L−1, % saturation), conductivity (µS cm−1), and pH were measured using a Yellow Springs Instrument (YSI) multi-parameter probe at ∼0.5-m depth intervals from the surface to the bottom. The probe was calibrated monthly using three standard solutions for pH, whereas DO saturation was calibrated at each sampling location. Atmospheric pressure (mm Hg) was estimated at each site using the YSI probe. Depth of the reservoir was taken using a Norcross Hawkeye handheld depth finder. Water clarity was measured using a 20-cm diameter Secchi disk.
Water samples were collected using a submersible pump with an intake deployed at ∼0.5-m depth. Whole water was screened through 80-μm mesh to remove large zooplankton and particulate matter and combined into a pre-rinsed cleaned carboy. Samples were transported back to the laboratory for chemical analyses.
GHG concentration of water was sampled on site using the headspace extraction method of Webb et al. (2019a). This process involves using a submersible pump to fill a 1.2-L glass serum bottle to overflow using water from ∼0.5-m depth, ensuring that there were no bubbles present, and sealing the bottle with a two-way rubber stopper. A 60-ml sample of atmospheric air was added to the bottle while 60-ml of water was removed to maintain constant pressure in the bottle. The bottle was then shaken vigorously for 2 min to equilibrate the air and water. Two replicate gas samples were extracted from the headspace using an air-tight syringe and put into 12-ml pre-evacuated Exetainer vials with double wadded caps. This process was repeated in duplicate to collect four samples per date. A sample of atmospheric air was also collected and placed in a 12-ml pre-evacuated Exetainer vial with double wadded cap. All gas samples were stored at room temperature until analysis within 2 months of collection. While methane ebullition is known to contribute significantly to methane emissions, this analysis only focuses on diffusive flux of greenhouse gases. Methane ebullition is known to be very patchy both spatially and temporally, and seasonal changes in bubble flux is currently not well constrained.
2.1.2 Diel sampling
Four reservoirs were selected for diel monitoring to assess daily variability in CO2, CH4, and N2O concentrations. Samples were collected at 4-h intervals over 24 h (6 total), beginning ∼10:00 h, between 26 June and 01 August 2018. Each diel cycle involved GHG sampling and YSI readings as described above. Water samples were collected at the 0-h, 12-h, and 24-h marks to determine changes in water chemistry throughout the 24-h period. Additionally, a YSI multi-parameter probe was deployed in each site for the entire 24-h period, taking readings for water temperature, DO, pH, and conductivity at 10-min intervals. Sites were selected from a set of previously sampled sites to include a wide range of physical and chemical parameters. Secondarily, sites were selected based on ease of access and availability of services for the field team.
2.2 Laboratory analysis
At the laboratory, water samples were stored at 4°C and filtered within 24 h of collection. Sufficient water to visibly discolour a Whatman 1.2-µm GF/C filter was filtered was recorded, and filtrate was frozen (−10°C) in sealed darkened film canisters until analysis for chlorophyll pigments.
Greenhouse gas samples were analyzed at the Global Institute for Water Security, University of Saskatchewan. Headspace gas samples were analyzed for the dry molar fraction of CO2, CH4, and N2O using a fully calibrated Scion 456 Gas Chromatograph (Bruker Ltd.) with Combipal autosampler (CTC Analytics–PAL System), using argon as the carrier gas. A flame ionization detector was used for methane samples (<100,000 ppmv). A thermal conductivity detector was used for CO2 and high level CH4 concentrations (>100,000 ppmv). N2O was measured using a micro-electron capture detector and argon/methane (90/10) as a makeup gas (injector temperature 60°C, column temperature 60°C, detector temperature 350°C). All gases were calibrated using mixed gas standards (Praxair) with the addition of a single gas N2O standard (0.1 ppmv; Scotty).
Water chemistry and water isotope samples were analyzed at the Institute for Environmental Change and Society at the University of Regina. Water chemistry samples were analyzed for soluble reactive phosphorus (SRP), total dissolved nitrogen (TDN), nitrate and nitrite (NOx), ammonia (NH3), dissolved organic carbon (DOC), and dissolved inorganic carbon (DIC). DOC and DIC were analyzed using standard analytic procedures on a Shimadzu model 5000 A total carbon analyzer (Finlay et al., 2009; Webb et al., 2019b). Dissolved nitrogen (TDN, NOx, NH3) and dissolved phosphorus (TDP, SRP) were measured using standard procedures on a Lachat QuikChem 8,500 (APHA-AWWA/WEF 1998; Bergbusch et al., 2021). Chl-a concentration was standard trichromatic spectrophotometric methods (Jeffrey and Humphrey 1975; Finlay et al., 2009). Water was analyzed for δ18O-H2O using a Picarro L2120-I cavity ring-down spectrometer (CRDS), and δ18O inflow was calculated using coupled isotope tracer methods as an estimate of water source (winter vs. summer precipitation, groundwater, Yi et al., 2008; Webb et al., 2019a; Haig et al., 2020).
2.3 Numerical analyses
The squared Brunt-Väisälä buoyancy frequency (s−2) was calculated as a measure of maximum stratification strength within the water column. This approach uses the greatest density gradient from the vertical water temperature profiles taken at intervals of 0.5 m (0.25 m in shallower waterbodies) using the rLakeAnalyzer package (Read et al., 2011) in R (version 4.0.5; R Core Team, 2021) (Webb et al., 2019b).
The dry molar fractions of CO2, CH4, and N2O were corrected for dilution and converted to concentrations according to the solubility coefficients and atmospheric partial pressure of each gas and the salinity of the water. Four replicate gas concentrations for each site were averaged for CO2, CH4, and N2O to estimate mean concentrations for each sampling period. We removed an extreme CH4 outlier for in one reservoir seen during April (93.5 µM) and assumed an ebullitive event that was captured in the associated water sample.
The concentration of each of the gases were used to estimate the diffusive flux for CO2, CH4, and N2O. The diffusive flux (fC) was calculated using the gas transfer velocity (kc), gas concentration of the water (Cwater), and the ambient air concentration (Cair) using the following equation (Eq. 1):
The ambient air concentration of each gas was taken as the average over the sampling period at the Mauna Loa NOAA station from April to September 2018, inclusive. The average concentrations were 408.94 µatm (or ppm) for CO2, 1.86 µatm (or ppm) for CH4, and 0.33 µatm (or ppm) for N2O, and corrected for the barometric pressure for each sampling effort. The diffusive flux was calculated using the gas transfer velocity as measured on similar agricultural reservoirs in 2017 (Webb et al. 2019a; Webb et al. 2019b). We did not estimate ebullitive CH4 fluxes, although we recognize this will lead to an underestimation of the true total CH4 efflux.
Integrating seasonal GHG flux across all gases is challenging, owing to temporal variability and the assumptions required to extrapolate between sampling dates. Here, we calculated total CO2-eq flux using several methods to bound true values. First, the “July only” procedure used only the daily CO2-eq value observed in July multiplied over the total number of ice-free days to extrapolate over the entire open water period (e.g., metanalysis in DelSontro et al., 2018). Second, the “Seasonal” protocol used the CO2-eq values measured in April, May, July, and September for the respective intervals summed over the entire open water period (e.g., Striegl and Michmerhuizen 1998; Finlay et al., 2019). Third, we calculated a “Predicted” flux using the generalized additive models (GAMs) of the smoothed seasonal splines to interpolate fluxes on each date between sampling periods. For this last method, we took both the mean smooth value and the upper 95% credible interval (“Upper CI”) to estimate mean and maximum seasonal CO2-eq flux. Given the lack of consistent diel trend (see Section 3.4 below), we used measured flux rates from daytime determinations only. In all cases, the CO2-eq flux was calculated by first converting the moles of diffusive flux value to grams of diffusive flux, and then multiplying by the sustained global warming potential (45 and 270 for CH4 and N2O, respectively) if the diffusive flux value was positive, and by the sustained global cooling potential (203 and 349 for CH4 and N2O, respectively) if the diffusive flux value was negative (Neubauer and Megonigal 2015).
Generalized additive models (Wood 2006; Wood et al., 2016) were used to assess the seasonal trends in CO2, CH4, and N2O concentration in the reservoirs, as they are particularly useful for estimating linear, nonlinear, and nonmonotonic relationships between response variables and predictors (Webb et al., 2019a; b; Swarbrick et al., 2019). All modelling was done using the mgcv package (Wood 2011; Wood 2016) for R (version 4.0.5; R Core Team, 2021). GAMs were also used to link CO2, CH4, and N2O concentrations to various biotic and abiotic predictive parameters to identify which factors might regulate changes in GHG concentrations in agricultural reservoirs. Covariates included those thought to control seasonal variation in GHG levels during the open-water period and were based on spatial analysis of these agricultural reservoirs (Webb et al., 2019a; Webb et al., 2019b). Variables included nutrient content (DOC, DIN, SRP), production (Chl-a, DO), and stratification strength (buoyancy frequency). The CO2 model additionally included water sources (as δ18O of inflow), specifically groundwater, rainfall, or snowmelt (Yi et al., 2008; Haig et al., 2020). Conductivity was additionally included in the CH4 model as a proxy for ionic content and, in this region, sulfate concentrations (r2cond-SO4 = 0.64, p < 0.01). Similarly, pH was included in the N2O model because of its known effects on N2O production and consumption processes (Quick et al., 2019). Site identity (ID) was included as a random effect in the model, to account for the differences between individual sites.
A Gamma distribution was used with a log-link function, for positive, continuous responses of CO2 and CH4. We used a gammals distribution for N2O concentrations to model the log mean and log scale parameter (or standard deviation/variance) for the probability density function. The gammals distribution was used because two sites exhibited high, outlying N2O concentrations compared to the rest, so this allowed for grouping (high and low) of sites separately from the rest of the sites to inform a non-normal distribution. Sites were put into the high group if 25% or more of their N2O concentrations were outliers. Basis size, dispersion of residuals, homogeneity of variance, and the relationship between the observed and predicted response were assessed in each model to ensure assumptions were not violated. Residual marginal likelihood (REML) was used for selection of smoothness parameters (Wood, 2011). To help with model selection, the double penalty approach of Marra and Wood (2011) was used. An additional penalty is applied to the perfectly smooth parts of the basis (the functions in the penalty null space) of each smooth function in the model, which allows entire smooths to be effectively penalised out of the model, while accounting for the selection procedure in the statistical tests applied to model terms. Parameters predicting CO2, CH4, and N2O concentrations were considered significant at 95% confidence level (α = 0.05) for each waterbody.
3 Results
3.1 Water quality
Physical and environmental parameters of the 20 reservoirs exhibited a wide range of values among sites and over the season (Table 1). The mean monthly max depth (mean = 1.88 m, standard deviation (SD) = 0.92), Secchi depth (0.61 ± 0.43 m), DO saturation (89.5 ± 31.2%), and Chl-a (44.8 ± 61.1 μg L−1) of the reservoirs did not change significantly between any month (ANOVA, p-value > 0.05). Mean surface water temperature was low in April (mean = 7.98°C, SD = 4.38), reached a maximum in July (mean = 22.7°C, SD = 1.47), and declined again in September (mean = 6.99°C, SD = 0.87, ANOVA post-hoc Tukey test, p-value < 0.001). The pH of the surface water was lowest in April (mean = 8.29, SD = 0.84), reached a maximum in July (mean = 8.94, SD = 0.61), and decreased again in September (mean = 8.62, SD = 0.44, ANOVA post-hoc Tukey test, p-value = 0.014). Conductivity increased from April (mean = 737 μS cm−1, SD = 591) to September (mean = 1824 μS cm−1, SD = 1722, ANOVA post-hoc Tukey test, p-value = 0.029). Salinity also increased from April (mean = 0.53 ppt, SD = 0.42) to September (mean = 1.25 ppt, SD = 1.11, ANOVA post-hoc Tukey test, p-value = 0.033).
Water chemistry parameters were highly variable both between months and among reservoirs (Table 2). Soluble reactive phosphorus (seasonal mean = 320 μg P L−1, SD = 515), TDN (2,793 ± 2,141 μg N L−1) and NH3 (seasonal mean = 316 μg N L−1, SD = 654) concentrations did not have a significant change between monthly means (ANOVA post-hoc Tukey test, p-value = 0.001). DOC concentrations also increased from April (mean = 20.1 mg C L−1, SD = 6.54) to September (mean = 31.3 mg C L−1, SD = 12.0, ANOVA post-hoc Tukey test, p-value = 0.004). The concentration of NOx was highest initially following ice-off in April (mean = 644 μg N L−1, SD = 1,054, ANOVA post-hoc Tukey test, p-value = 0.009).
3.2 Seasonal greenhouse gas concentration and flux
Temporal variation in concentrations of CO2, CH4, and N2O (Figure 1A) was identified as significant seasonal trends when analyzed by GAMs for all gases (Figure 1B). The concentrations of each of the gases was highly variable between reservoirs, yet there were common trends for each gas. For example, concentrations of CO2 were greatest immediately following ice-off in April (mean = 45.8 µM, SD = 39.3), declined during spring and summer (May–mean = 23.5 µM, SD 23.6, July–mean = 16.5 µM, SD 17.0), then increased again in fall (mean = 32.0 µM, SD 31.2, GAM, p-value = 0.013, deviance explained = 49%). In contrast, CH4 concentration were initially low in the spring (April–mean = 0.849 µM, SD = 1.54; May–mean = 0.73 µM, SD = 1.28), but increased into a mid-summer peak (mean = 1.32 µM, SD = 1.70) before decreasing in fall (mean = 0.40 µM, SD = 0.47, GAM, p-value = 0.0005, deviance explained = 91.4%). Finally, concentration of N2O exhibited a strong pulse during ice-off in April (mean = 35.2 nM, SD = 50.3), followed by a sharp decrease to low values in spring and summer (May–mean = 10.1 nM, SD = 1.5; July–mean = 15.4 nM, SD = 36.1), before rising slightly again prior to ice formation (mean = 13.3 nM, SD = 4.31, GAM, p-value < 0.0001, deviance explained = 89.5%).
Estimates of diffusive fluxes of CO2, CH4, N2O were all highly variable among sites (Figure 2). Despite among-site variation, diffusive flux of CO2 varied little between months (ANOVA, p-value = 0.161), with the overall mean (= SD) of 19.7 ± 56.6 mmol m−2 day−1. Similar patterns were recorded for the diffusive flux of CH4, with no seasonal difference between monthly means (ANOVA, p-value = 0.654) and an overall flux of 2.9 ± 10.9 mmol m−2 day−1. Diffusive flux of N2O was variable among sites but not months (ANOVA, p-value = 0.361), with an overall mean diffusive N2O flux of 9.7 ± 52.9 μmol m−2 day−1.
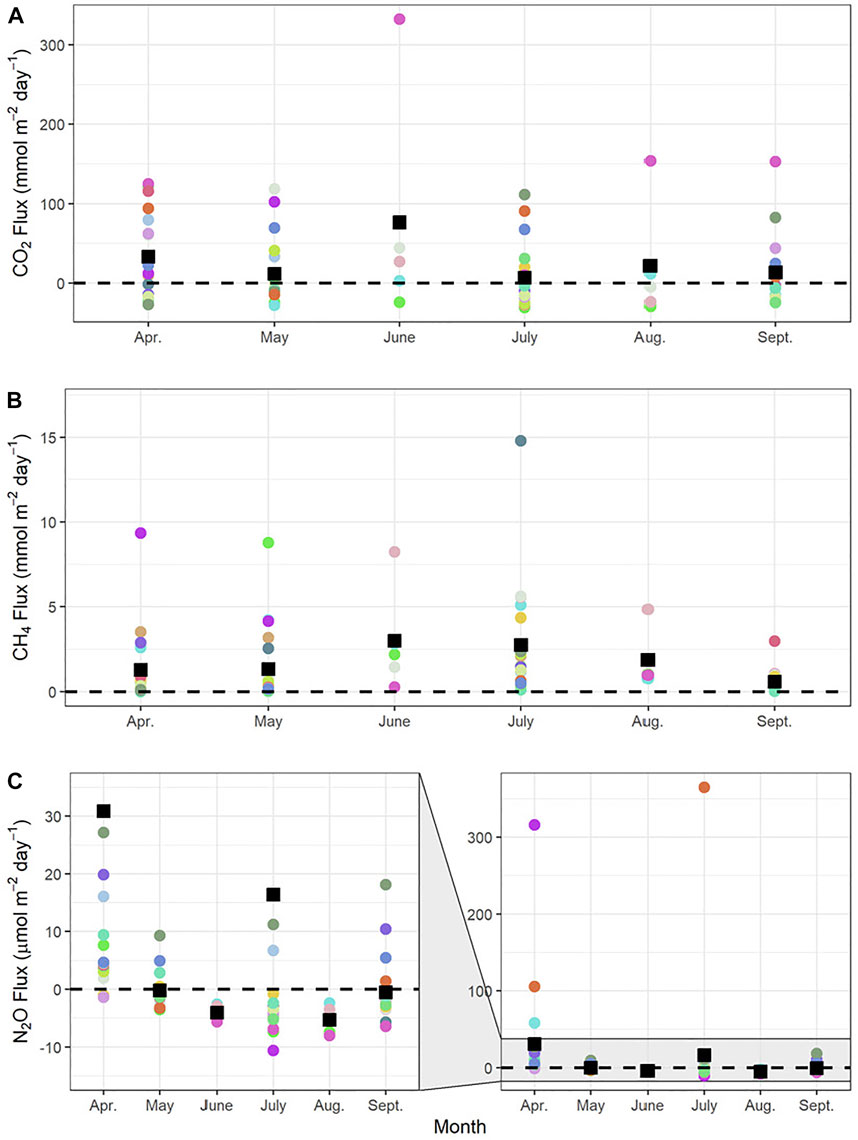
FIGURE 2. (A) Carbon Dioxide (CO2) (B) methane (CH4), and (C) nitrous oxide (N2O) flux for the sampling period. Each coloured circle represents a single reservoir, while the black square represents the monthly mean. Black dashed line atmospheric equilibrium, where values above indicate loss of gas to the atmosphere and values below indicate removal of gas from the atmosphere. One outlying CH4 value in April was omitted (value = 100 mmol m-2 day-1) as it is assumed that an ebullition event was captured.
3.3 Diel water chemistry
Most water quality parameters did not change substantially during the diel cycle (Figure 3). In general, temperature was greater during the day and lower at night, although there were up to 4°C differences among sites (Figure 3D). Similarly, while DO exhibited some high frequency variation, concentrations were generally lower at night than in the day (Figure 3E). In contrast, few trends were observed for conductivity, and pH exhibited diel cycle (elevated in day) at only one site with elevated Chl-a values and high diel changes in DO. Nutrients (TN, TP, SRP, DOC, DIC) and Chl-a did not exhibit any observable changes during the diel cycle in any sites, while NOx concentration and ammonia concentration only exhibited notable variation at one site with high DIN levels (Supplementary Table S1).
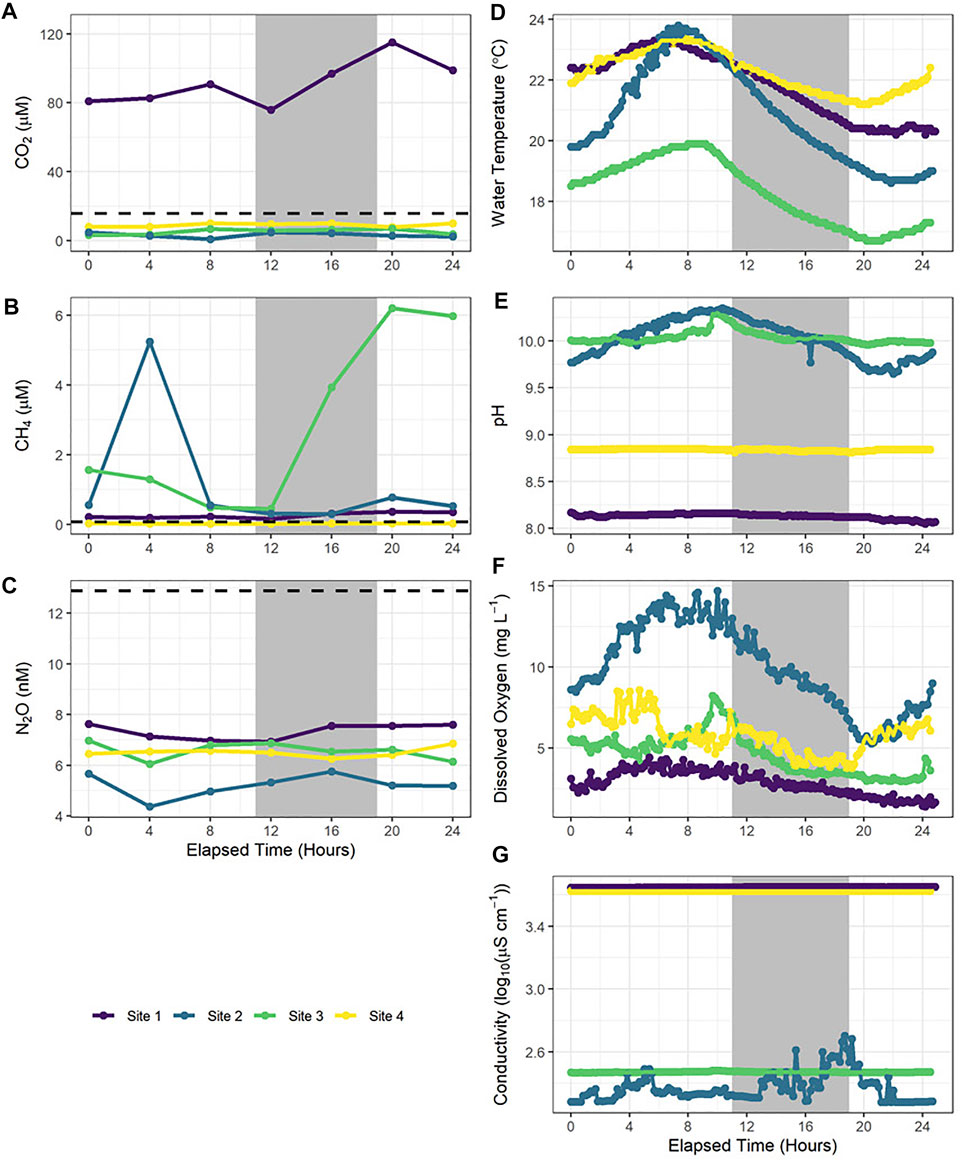
FIGURE 3. Greenhouse gas (A–C) and water quality parameters (D–G) in four reservoirs measured over a 24-h period. Each coloured line is representative of one site. The grey shaded regions are the average night-time period. Elapsed time is time from the initiation of the 24-h period. Earliest start was 09:50, while the latest start was 11:24. Temperature (D), DO (dissolved oxygen (E)), pH (F) and conductivity (G) readings were taken every 10 min, while greenhouse gas concentrations were collected every 4 h. CO2 = carbon dioxide (A). CH4 = methane (B). N2O = nitrous oxide (C). See 24-h averages of nutrient, Chl-a and carbon concentrations in Supplementary Table S1.
3.4 Diel greenhouse gas concentration and flux
Concentrations of CO2, CH4, and N2O showed no consistent trends during a 24-h period in the four reservoirs sampled (Figure 3). At three sites, CO2 concentrations were consistently undersaturated over the diel cycle, while values were supersaturated at the fourth site yet no more variable through time. CH4 concentrations at two sites remained low throughout the diel cycle, while the other two basins showed inconsistent but elevated variation during the 24 h study period. Overall, N2O concentrations were consistently low and undersaturated during the diel cycle.
3.5 Seasonal greenhouse gas controls
Seasonal changes in CO2 concentration in the reservoirs were explained best by a GAM using the pH of surface water as the sole predictor (Supplementary Figure S2). This model explained 89.1% of deviance in CO2 and was characterized by a strong negative relationship with CO2 above pH of 8. When pH was not included in the model, variation in CO2 was explained best by a combination of DO saturation (Figure 4A), DIN concentration (Figure 4B), DOC levels (Figure 4C), buoyancy frequency (Figure 4F), and water source (as δ18O; Figure 4G). Dissolved oxygen, buoyancy frequency, and δ18O exhibited significant negative relationships with variation in CO2 concentration, while DIN and DOC had positive relationships. Overall, this model explained 63.9% of deviance in the CO2 levels.
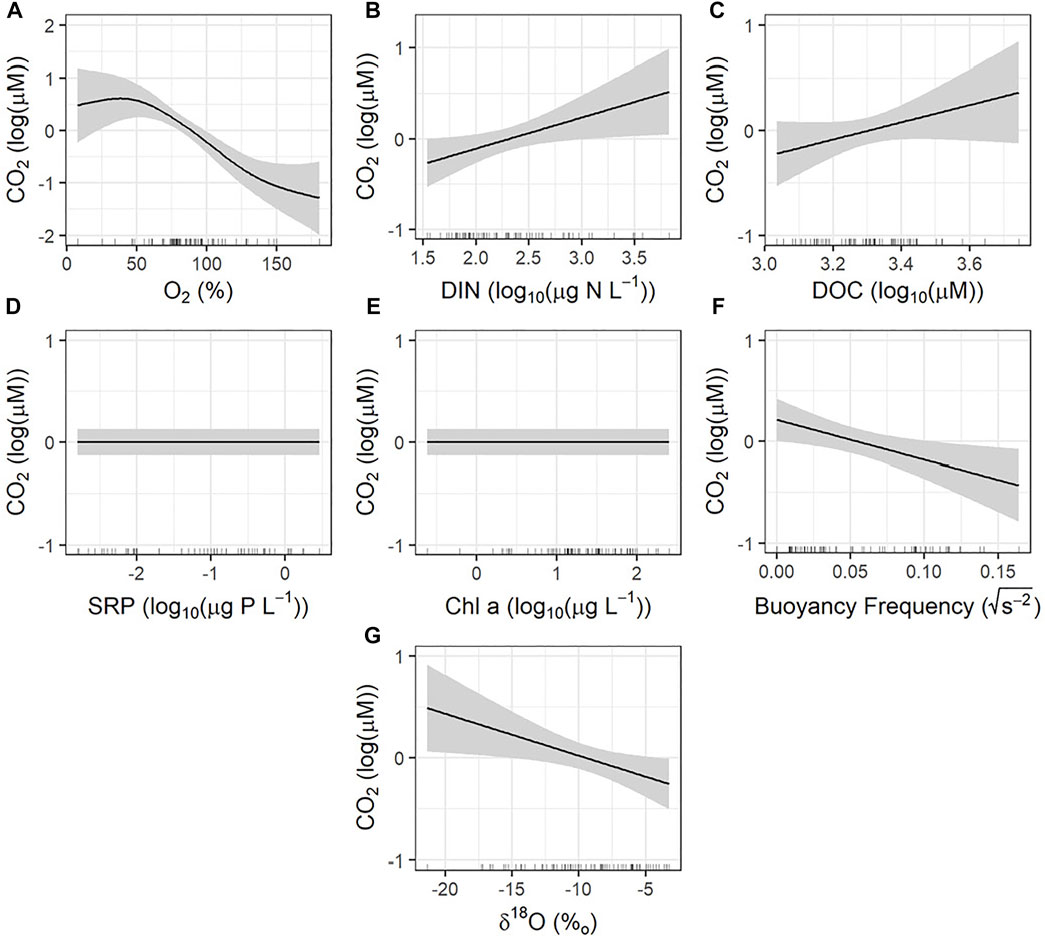
FIGURE 4. Partial effects plots from generalized additive models assessing the seasonal controls of carbon dioxide (CO2). Model deviance explained was 63.9%. Shaded region represents the 95% confidence interval. The rug along the x-axis indicates the distribution of observations. (A) Dissolved oxygen saturations; significant. (B) Dissolved inorganic nitrogen concentration; significant. (C) Dissolved organic carbon concentration; significant. (D) Soluble reactive phosphorus concentration; not significant. (E) Chl-a concentration; not significant. (F) Buoyancy frequency (stratification strength); significant. (G) d18O inflow (measure of water source); significant.
Seasonal changes in CH4 concentrations were explained best by a combination of DO saturation (Figure 5A), concentrations of DIN (Figure 5B), DOC (Figure 5C), and SRP (Figure 5D), as well as surface water temperature (Figure 5F), and conductivity (Figure 5G). Dissolved oxygen, SRP, and conductivity exhibited significant negative relationships with the seasonal CH4 concentration, while DIN, DOC, and surface water temperature exhibited significant positive relationships with methane levels. Model deviance explained was 74.5% of variation in CH4 levels.
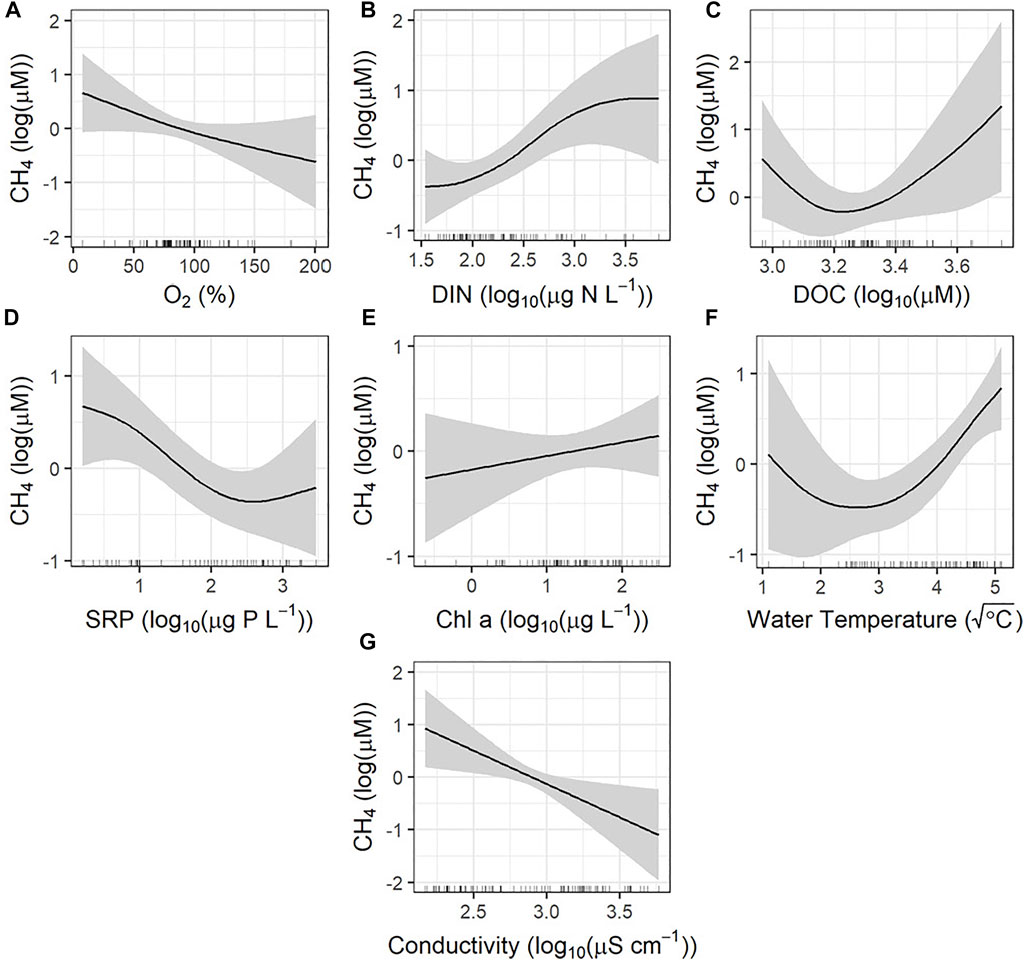
FIGURE 5. Partial effects plots from generalized additive models assessing the seasonal controls of methane (CH4). Model deviance explained was 74.5%. Shaded region represents the 95% confidence interval. The rug along the x-axis indicates the distribution of observations. (A) Dissolved oxygen saturations; significant. (B) Dissolved inorganic nitrogen concentration; significant. (C) Dissolved organic carbon concentration; significant. (D) Soluble reactive phosphorus concentration; significant. (E) Chl-a concentration; not significant. (F) Water temperature; significant. (G) Conductivity; significant.
Regulation of seasonal variation in N2O concentrations was also complex, with significant effects of changes in DO saturation (Figure 6A), DIN concentration (Figure 6B), SRP concentration (Figure 6D), Chl-a concentration (Figure 6E), buoyancy frequency (Figure 6F), and pH of the surface water (Figure 6G). Variation in N2O levels were related positively to changes in DO, DIN, and SRP, and negatively to variation in Chl-a, buoyancy frequency, and pH, while the model explained 87.4% of the deviance in N2O levels.
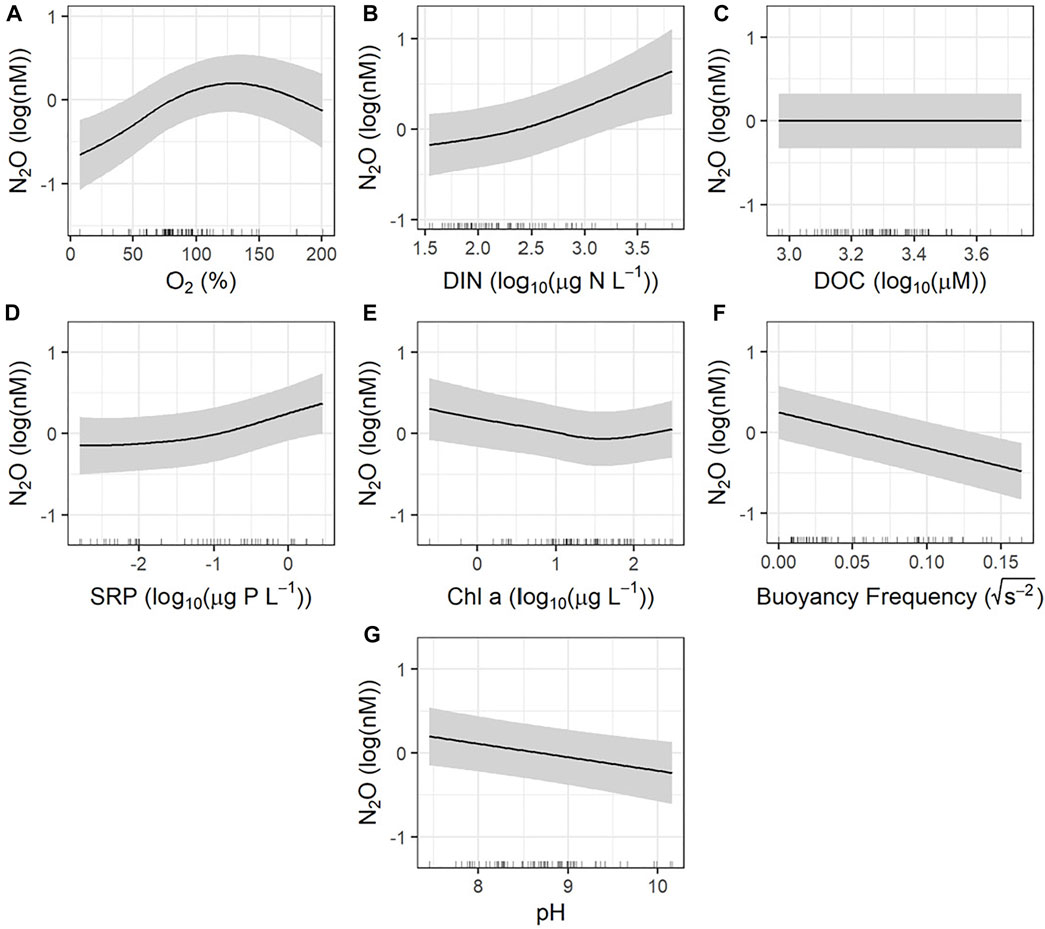
FIGURE 6. Partial effects plots from generalized additive models assessing the seasonal controls of nitrous oxide (N2O). Model deviance explained was 87.4%. Shaded region represents the 95% confidence interval. The rug along the x-axis indicates the distribution of observations. (A) Dissolved oxygen saturations; significant. (B) Dissolved inorganic nitrogen concentration; significant. (C) Dissolved organic carbon concentration; not significant. (D) Soluble reactive phosphorus concentration; significant. (E) Chl-a concentration; significant. (F) Buoyancy frequency (stratification strength); significant. (G) pH; significant.
3.6 Seasonal CO2-eq flux
Estimates of total CO2-eq flux over the open-water period varied dependent on the method used to estimate in situ GHG concentrations (Figure 7), but all were within an order of magnitude of each other. Mean seasonal CO2-eq flux was comparable between the “July-only” (9,811 ± 10,269 mmol m−2, Figure 7A) and “seasonal” methods (10,446 ± 17,893 mmol m−2, Figure 7B), and were roughly twice the fluxes predicted using GAMs (5,027 ± 4,806 mmol m−2, Figure 7C). All methods suggest that methane was the dominant GHG responsible for CO2-eq flux, and contributed more than 50% to total GHG efflux in 16 of 20 sites (Figure 7, yellow bars). While the mean seasonal values were comparable among methods, there were some discrepancies within individual sites. For example, Site 23 A demonstrated extremely high CO2-eq flux, attributable to one very high CH4 measurement in April. This likely reflected an ebullition event at this time that was not captured when the “July-only” method was used, and which was constrained using the GAM-predicted method.
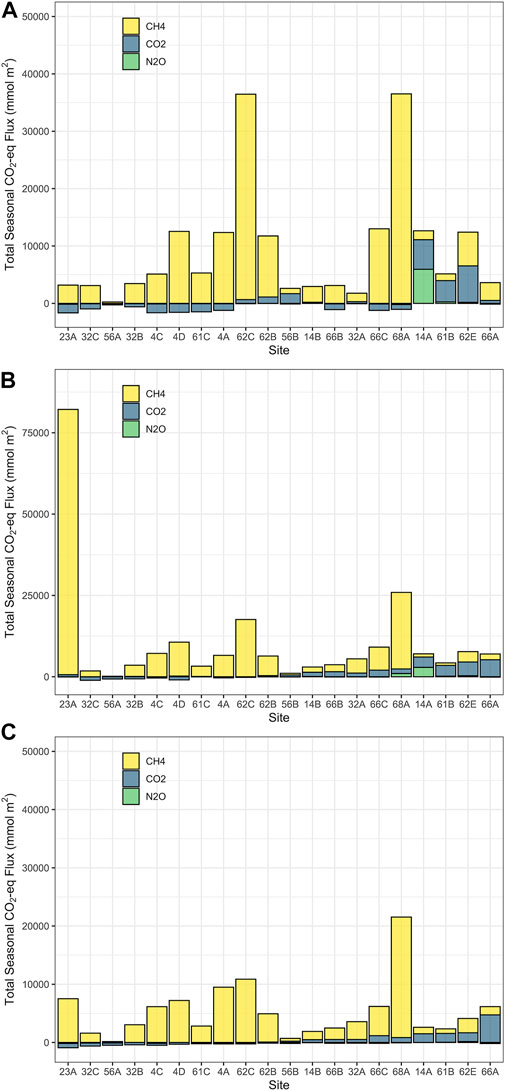
FIGURE 7. Calculated CO2-eq fluxes based on three different estimates: (A) Using GHG fluxes measured in July and extrapolating over the entire open-water season; (B) Using GHG fluxes measured on four dates (April, May, July and Sept) in 2018 and interpolating between sampling dates; (C) Mean predicted flux using GAMs from Figure 1 to interpolate gas flux between sampling dates.
4 Discussion
Evaluation of temporal variability and controls of GHG in 20 agricultural reservoirs in the northern Great Plains indicated that while CO2, CH4, and N2O flux exhibited few diel trends (Figures 3A–C), all GHG exhibited pronounced seasonality during the ice-free period regulated by complex interactions between microbial metabolism, allochthonous carbon inputs, and physico-chemical features (Webb et al., 2019a; Webb et al., 2019b). As predicted, all sites demonstrated peaks of CO2 and N2O in spring, as well as a second, lower peak of CO2 during fall (Pennock et al., 2010; Webb et al., 2019a; Webb et al., 2019b), whereas CH4 content was maximal in mid-summer (Figure 1), consistent with studies of Yvon-Durocher et al. (2014) and DelSontro et al. (2018). Contrary to predominantly planktonic controls identified in earlier regional GHG studies (Finlay et al., 2015; Wiik et al., 2018; Finlay et al., 2019), seasonal variation in CO2 concentrations were correlated with temperature and organic carbon availability, indicating effects of benthic respiration (Figure 4). Similarly, CH4 levels were correlated to changes in temperature, while a negative correlation with conductivity suggests inhibition of methanogenesis by sulfate-reducing bacteria in ion-rich waters (Figure 5). In contrast, N2O concentrations appeared to be controlled mainly by variation in reservoir stratification or mixing (Figure 6). Taken together these findings suggest that the response of small farm reservoirs to future climate change will depend strongly on which climatic parameters (temperature, evaporation, runoff, wind) are most affected by atmospheric heating. In particular, factors that regulate mid-summer CH4 release appear to be paramount in determining whether small reservoirs hold potential as net CO2-eq sources or sinks in the coming decades.
4.1 Carbon dioxide
CO2 concentration in agricultural reservoirs was high in the spring, low throughout the summer, and began to increase again in fall (Figure 1B). This seasonal pattern was consistent with that observed in boreal and other hard-water systems, where CO2 accumulates under the ice in the winter, causing a pulse of CO2 released in the spring following ice off (Baehr and DeGrandpre 2002; Finlay et al., 2015; Denfeld et al., 2016). Depressed CO2 in summer is attributed to isolation of surface waters leading to elevated photosynthetic rates that increase pH and deplete dissolved pCO2 to values below atmospheric values (Huotari et al., 2009; Holgerson 2015). Conversely, elevated fall CO2 concentrations appear to reflect loss of water-column stratification, and release of hypolimnetic CO2 which had been accumulated during the summer period of stratification (Huotari et al., 2009; Holgerson 2015; Webb et al., 2019b; Finlay et al., 2019). Agricultural reservoirs in this study have a high relative depth (5.6 ± 3.1%), and thus exhibit a high resistance to vertical mixing (Wetzel and Likens 1991; Holgerson et al., 2022) during summer. As elevated primary production sinks into the hypolimnion and decomposes during summer, CO2 is released leading to supersaturation of this gas in deep water (Huotari et al., 2009; Finlay et al., 2019).
Seasonally, pronounced negative correlations between CO2 concentration and DO saturation (Figure 4A) and positive correlations between CO2 and both DIN and DOC (Figure 4C) are indicative of metabolic controls of CO2 concentrations (Holgerson 2015; Balmer and Downing 2017; Vachon et al., 2019). The metabolic stoichiometry between CO2 and DO is well documented (Vachon et al., 2019) and represent autotrophic and heterotrophic processes occurring both in the water column or emanating from the sediments (Roulet et al., 1997; Jonsson et al., 2003; Rantakari and Kortelainen 2005; Kortelainen et al., 2006; Holgerson 2015). Here, the significant positive relationship between CO2 concentration and DIN levels (Figure 4B), as well as DOC levels (Figure 4C), and lack of significant relationship with Chl-a (Figure 4E) suggests that elevated nutrient content stimulated microbial respiration rather than primary production, such as seen in other artificial waterbodies and prairie lakes (Ollivier et al., 2018; Wiik et al., 2018; Webb et al., 2019b; Peacock et al., 2019), and natural temporary wetland ponds (Holgerson 2015). As shown in mesocosm fertilization experiments, moderate eutrophication of shallow prairie waters first elevates primary production and DO saturation, then leads to water-column anoxia due to build-up of dissolved and particulate organic matter (Donald et al., 2011; Bogard et al., 2020). Consistent with this mechanism, monitored reservoirs exhibited low ratios of dissolved N:P characteristic of N-limited regional lakes (Donald et al., 2011) (Table 2), while on-going whole-reservoir fertilization experiments show that N fertilization both increases surface water Chl-a levels and causes deep water supersaturation of CO2 (C.A.C. Gushulak and P.R. Leavitt, unpublished data). At present, it is unclear whether the relationships observed in this or other studies reflect water column processes or the disproportionate effect of warm sediments in these shallow water bodies.
The negative correlation between CO2 concentration and buoyancy frequency, a measure of stratification strength (Figure 4F), is consistent with the effects of seasonal thermal stratification in isolating deeper waters and constraining CO2 loss to the atmosphere (Huotari et al., 2009; Holgerson 2015). Here, CO2 concentrations are elevated both in spring after ice melting allows release of the winters’ accumulation of respired CO2 (Baehr and DeGrandpre 2002; Finlay et al., 2015; Denfeld et al., 2016), as well as in fall after when cooling air temperatures erode thermal stratification and release hypolimnetic CO2 (Huotari et al., 2009; Holgerson 2015). Between these intervals, agricultural reservoirs can exhibit thermal stratification that isolates respired CO2 derived from sediments and prevents accumulation in surface waters. In general, this pattern contrasts findings from a spatial survey of prairie farm reservoirs in 2017 that found a significant positive relationship between CO2 concentration and buoyancy frequency (Webb et al., 2019b). We speculate that this difference arose because sampling for Webb et al. (2019b) took place during the summer and would have mainly captured variation in the degree of thermal stratification among sites, rather than the sharp seasonal patterns of water column mixing exhibited in this study.
CO2 concentration and δ18O exhibited a pronounced negative relationship (Figure 4G) suggesting that elevated inflow of snowmelt or groundwater resulted in higher CO2 concentrations in these farm reservoirs, consistent with observations in the 2017 spatial study (Webb et al., 2019b). In general, regional farmers site the reservoirs in existing lowland or wetland areas, often with direct contact to shallow aquifers, or with abundant overland runoff. In this region, snowmelt in spring is both the main source of overland flow (Pomeroy et al., 2007), and the mechanism recharging shallow aquifers (Haig et al., 2020) and, in both cases, exhibited highly depleted δ18O values relative to rainwater sources (Haig et al., 2020; Haig et al., 2021). Further, this groundwater has high concentrations of both DOC and DIC, and is frequently supersaturated with CO2 (Macpherson 2009; Webb et al., 2019b). Seasonal monitoring of piezometers for water isotopes, CO2 levels and dissolved C concentrations will be required to further resolve this mechanism.
We did not observe consistent diel fluctuations of CO2 concentrations in the four reservoirs sampled (Figure 3). Previous studies have shown that CO2 concentrations are typically lower during the daytime when primary production exceeds respiration (P > R), but increase overnight due to continued respiration (Liu et al., 2016; Raymond et al., 2013; Wiik et al., 2019). The magnitude in the shifts of CO2 concentration is often linked to the water-column productivity, with the largest diel changes observed in systems with the highest productivity (Hanson et al., 2003; Morales-Pineda et al., 2014; Shao et al., 2015; Wiik et al., 2018). Here Chl-a concentrations ranged from 2.9 to 296 μg L−1, therefore, a strong diel change in CO2 concentration would be expected in the more productive sites. Lack of pronounced variation through the day may reflect the high pH of these systems which would favour rapid conversion of CO2 to bicarbonate (HCO3−) and carbonate (CO32-). Furthermore, the high total alkalinity (241.3 ± 159.4 mg L−1) of the systems likely resisted diel variation in pH in all but one reservoir (Figure 3D), as diel changes in CO2 were much less than the total reservoir content of DIC (Dodds 2002; Stets et al., 2017).
4.2 Methane
CH4 concentrations showed a marked peak during July-August (Figure 1B) similar to those seen high latitude wetlands (Pickett-Heaps et al., 2011), aquaculture ponds (Yang et al., 2015), shallow urban basins (van Bergen et al., 2019), and prairie wetlands (Bansal et al., 2016). In general, this interval corresponds to the period of greatest water temperatures and is consistent with peak CH4 levels occurring when water temperatures are greatest in this study (Figure 5F) and the observation that metabolism of methanogenic bacteria is greater in warmer waters (Segers 1998). Such a positive relationship of CH4 and temperature has been observed in other shallow systems including prairie wetland ponds (Bansal et al., 2016), urban ponds (van Bergen et al., 2019), natural temporary wetlands (Holgerson 2015), and sites with abundant Phragmites growth (Kim et al., 1998). Methane ebullition was not included in our study; however, we acknowledge that this process can contribute significantly to GHG fluxes from inland water and may also vary seasonally.
Dissolved CH4 is expected to be more abundant under anoxic conditions (Glass and Orphan 2012), therefore, the significant negative relationship with DO saturation was expected (Figure 5A). Such a trend is common in diverse ecosystems, including boreal lakes (Kankaala et al., 2013), natural temporary wetland ponds (Holgerson 2015), and our earlier spatial survey of prairie farm reservoirs (Webb et al., 2019b).
The strong negative relationship between CH4 concentration and conductivity (Figure 5G) was also observed in the 2017 spatial study, which is likely attributed to sulfate limiting methanogenesis (Webb et al., 2019b). Sulfate-reducing bacteria have been shown to outcompete methanogenic bacteria at sulfate concentrations as low as 60 µM in freshwater sediments in oligotrophic lakes (Lovley and Klug 1983), and as low as 200 µM in eutrophic lakes (Winfrey and Zeikus 1977; Lovley et al., 1982). A linear regression showed that conductivity and sulfate are highly related in these agricultural reservoirs (r2 = 0.64, p-value < 0.01). Based on this calculation, the sulfate concentration ranges from 0.33 to 35.9 mM in these agricultural reservoirs. Surface water conductivity significantly increased over the open water season (Table 1) and is likely one of the mechanisms driving down the CH4 concentrations in fall.
Nutrient (DOC, DIN, and SRP) levels were also important predictors of CH4 concentrations in agricultural reservoirs (Figures 5B,C,E), although solute concentrations did not appear to be correlated to primary production as Chl-a (Supplementary Figure S2.1). As noted above, effects of allochthonous nutrients may be felt mainly through changes in the redox potential and dissolved oxygen content of these shallow ecosystems, rather than via augmentation of primary production. In particular, elevated production (Figure 5E) and sedimentation of labile organic matter may have favoured decomposition and anoxia in sediments, thereby improving habitat and substrate for methanogenesis (Smith et al., 1999; Anderson et al., 2002; Ollivier et al., 2019). The pronounced negative relationship between CH4 and SRP may suggest that there is competition for substrate between methanogenesis, and denitrification at high P levels, consistent with the increase in N2O concentration is seen at high SRP concentrations (Figure 6D). Such a mechanism has also been observed in anaerobic wetland sediment slurry incubations (Kim et al., 2015).
CH4 concentrations did not show a consistent diel trend in the four reservoirs sampled, despite obvious variation in both temperature and DO in the surface water (Figure 3). In contrast, distinct diel patterns of CH4 production have been seen in other systems where gas levels increase following sunrise, peak in the early afternoon, then decline quickly a stable low level at night (Neue et al., 1997; Kim et al., 1998; Xing et al., 2004). In general, mid-day maxima were seen here only in ponds with greatly elevated CH4 levels, possibly suggesting that the absence of strong diurnal maxima in some sites reflects a generally low abundance of methanogenic bacteria in some reservoirs. While speculative, we suggest that differences in the influx of groundwater- or sub-surface sulfate (SO4=) may explain the variation in absolute and relative CH4 levels during a 24 h cycle (see below), where the presence of sulfur-reducing bacteria can inhibit methanogenesis (Lovley and Klug 1983; Pennock et al., 2010).
4.3 Nitrous oxide
Although freshwaters are often significant emitters of N2O (Beaulieu et al., 2008; Beaulieu et al., 2011; DelSontro et al., 2018), patterns and controls of the seasonality of N2O concentrations are poorly understood. The seasonal trend observed here shows that following an initial pulse in N2O production immediately following ice melt in the spring, these reservoirs were often undersaturated in N2O for much of the ice-free period (Figure 1B). These low N2O values may be attributed to complete denitrification of dissolved NO3− to di-nitrogen gas (N2), similar to patterns seem in some lakes (Piña-Ochoa and Álvarez-Cobelas 2006), including Lake Kasumiguara, a shallow eutrophic lake that does not have an anaerobic zone (Hashimoto et al., 1993). Widespread undersaturation of N2O was also observed in regional farm reservoirs during 2017 (Webb et al., 2019a); however, this study provides the first evidence that N2O undersaturation last much of the summer, a finding which has not previously been recorded for nitrogen-rich agricultural landscapes. Better understanding of the mechanisms controlling this process may be important to regulating net CO2-eq fluxes from small water bodies in the continental interiors that represent much of the global agricultural regions.
The significant relationships seen between DO saturation, DIN (NOx + NH3), Chl-a, and buoyancy frequency (Figure 6) were similar to those seen spatial surveys of agricultural reservoirs (Webb et al., 2019a). This finding suggests that mechanisms relating to productivity and mixing are controlling N2O concentrations in reservoirs both seasonally and spatially (Webb et al., 2019a). The pulse of N2O in the spring may be explained by the build-up of N2O under ice over the winter season, which is a common observation in prairie and boreal lakes (Soued et al., 2016; Cavaliere and Baulch, 2018). The lack of atmospheric exchange (during ice cover) combined with ongoing N2O producing processes such as nitrification and denitrification (Cavaliere and Baulch, 2018), without the competing factor of primary productivity for DIN (NOx was elevated in April, Table 2), may explain the higher N2O levels. The switch to N2O undersaturation coincides with a decline in NOx concentration owing to elevated algal uptake, along with the onset of temporary water column stratification and a decline in the DO saturation in the deep waters (Table 1) where complete denitrification can proceed. Complete denitrification leads to the consumption of N2O in the process of producing N2 (Quick et al., 2019), which suggests that the conditions in agricultural reservoirs favour complete denitrification during most of the open-water period. Additionally, the significant positive relationship between N2O concentrations and SRP may be driven by direct, or indirect effects, via impacts of SRP on algal biomass and carbon production, or via direct effects on N2O producing microbes.
N2O concentration in the four reservoirs sampled on a diel timescale remained under-saturated throughout the 24 h cycle. This pattern is similar to that seen in an arid zone alpine pond which remained undersaturated in N2O for most of the diel cycle (Molina et al., 2021). Previous studies have diel variation in N2O concentrations, primarily in rivers and streams, although comparisons among these site exhibit little common diel pattern among habitats. For example, some studies show clear diel patterns with greater concentrations observed during nighttime relative to those during the daytime (Rosamond et al., 2011; Wu et al., 2018a; Wu et al., 2018b), while other locations show a peak during the day (Molina et al., 2021) as well as a peak during the nighttime (Rosamond et al., 2011). One study found a sharp decrease in N2O concentration during the nighttime (Harrison et al., 2005) and others did not find a consistent diel trend (Baulch et al., 2012).
4.4 Seasonal CO2 equivalent budget
We calculated areal CO2-eq flux for the farm ponds to range from an average 32.0 mmol m−2 day−1 using the GAM-predicted flux, to 66.5 mmol m−2 day−1 using the seasonal method, over the 157-days open-water season. These values are somewhat lower than the mean (129 mmol m−2 day−1) but well within the range (−10 to 1,462 mmol m−2 day−1) of the daily flux estimates in the 2017 spatial survey (Webb et al., 2019a; Webb et al., 2019b). Similar to findings from previous studies (DelSontro et al., 2018; Ollivier et al., 2019), we observed that CH4 was the major driver of CO2-eq flux (average 91.5% across all methods), while N2O contributed the least (0.73%). The contribution of CH4 to total CO2-eq flux is likely to be even higher when ebullition is also considered (DelSontro et al., 2016; Rosentreter et al., 2021).
Findings from this study show that all three GHGs exhibited significant seasonal trends, thereby suggesting that extrapolation from a single date to the entire open-water period may produce inaccurate estimates of the total CO2 equivalent (CO2-eq) flux from these systems. In our study, the seasonal trends of each GHG (Figure 1), combined with the fact that CH4 is typically the dominant gas contributing to CO2-eq flux (Figure 7) would suggest that extrapolating measurements in mid-summer are likely to overestimate seasonal flux. The GAM-predicted seasonal fluxes were, on average, half of that of the other two methods, likely because the modeled fluxes constrained occasional extreme effluxes GHG and avoided excessive extrapolation through time series. For example, the exceptionally high estimate of seasonal flux at site 23 A in Figure 7B reflects a pulse of CH4 in April (observed CH4 = 90.7 µM) which was not included in the July-only flux calculations, and was constrained in the GAM models. Depending on whether this extreme point was interpolated to the next sampling date or not, estimated CO2-eq flux differed during the spring period by up to 72,800 mmol m−2 depending on whether elevated values were (∼75,600 mmol m−2) or were not (2,800 mmol m−2) used to estimate vernal fluxes. Although ebullition can occur due to changes in production, water depth, or meteorological conditions (DelSontro et al., 2016), observed elevated values are unlikely to persist over the 3-week spring interval. Conversely, GAM predicted values missed the likely-real pulsed efflux, and thereby likely underestimated seasonal fluxes. Overall, the “seasonal” calculation also likely overestimates CO2-eq flux by inflating short-term emissions, while the July-only calculation will miss seasonal trends, and the use of the GAM smooths may dampen any short-term pulses of GHG efflux and lose the contribution of these to the seasonal flux rates. Reconciling these discrepancies to provide the best estimate of total open-water GHG fluxes will require continuous monitoring of GHG with sondes, now possible for CO2, but still limited for CH4 and N2O due to more limited sensor sensitivity.
This study shows that agricultural reservoirs exhibit significant seasonal trends in the concentrations of all major GHGs, and that the concentrations of these gases are regulated by a combination of physical, chemical, and biological mechanisms. Additionally, it was found that inclusion of estimates of this seasonal variability may be essential to accurately total CO2-eq fluxes to the atmosphere. However, reconciling accurate net GHG over the annual scale will only truly be overcome through increased GHG monitoring via in situ sensors to capture extreme changes in water conditions (such as at ice off). The Northern Great Plains, as the largest agricultural area of Canada, is an area where agricultural water management is ongoing. Reservoirs in the landscape mediate substantive fluxes, although more work is required to reduce error in estimation of annual efflux from these often-eutrophic reservoirs. Perhaps more importantly, the Northern Great Plains are a region of extremely high variability in factors known to influence GHG efflux, and upscaling efforts aimed at understanding the role of inland waters in GHG efflux need to account for spatial variability in solutes, including sulfate.
Data availability statement
The datasets presented in this study can be found in online repositories. The names of the repository/repositories and accession number(s) can be found below: https://github.com/finlay4k/Jensen_dugout_seasonality.git.
Author contributions
SJ, JW, GS, PL, HB, and KF designed research; SJ performed research and drafted the paper; HB contributed new reagents/analytic tools; JW, PL, GS, HB, and KF contributed towards ideas and data analysis; SJ developed generalized additive models. All authors revised and approved the final manuscript.
Funding
Financial support for data collection and analyses were provided in part by Government of Saskatchewan (Award 200160015), Natural Sciences and Engineering Research Council of Canada Discovery grants (to KF, GS, HB, and PL), the Canada Foundation for Innovation (Award RGPIN--2018-0490), University of Regina.
Acknowledgments
We thank Shaeya Cluff, Ann King, and Mackenzie Metz for fieldwork assistant and all landowners for their generous cooperation in volunteering their reservoirs for this research. This research took place on Treaty 4 and 6 territories, traditional areas of Cree, Saulteaux, Lakota, Dakota, and Nakota peoples, and homeland of the Métis.
Conflict of interest
The authors declare that the research was conducted in the absence of any commercial or financial relationships that could be construed as a potential conflict of interest.
Publisher’s note
All claims expressed in this article are solely those of the authors and do not necessarily represent those of their affiliated organizations, or those of the publisher, the editors and the reviewers. Any product that may be evaluated in this article, or claim that may be made by its manufacturer, is not guaranteed or endorsed by the publisher.
Supplementary material
The Supplementary Material for this article can be found online at: https://www.frontiersin.org/articles/10.3389/fenvs.2022.895531/full#supplementary-material
References
Anderson, D. M., Glibert, P. M., and Burkholder, J. M. (2002). Harmful algal blooms and eutrophication: Nutrient sources, composition, and consequences. Estuaries 25, 704–726. doi:10.1007/bf02804901
Baehr, M. M., and DeGrandpre, M. D. (2002). Under-ice CO2 and O2 variability in a freshwater lake. Biogeochemistry 61, 95–113. doi:10.1023/a:1020265315833
Baehr, M. M., and DeGrandpre, M. D. (2004). In situ pCO2 and O2 measurements in a lake during turnover and stratification: Observations and modeling. Limnol. Oceanogr. 49, 330–340. doi:10.4319/lo.2004.49.2.0330
Balmer, M. B., and Downing, J. A. (2017). Carbon dioxide concentrations in eutrophic lakes: Undersaturation implies atmospheric uptake. Inland Waters 1, 125–132. doi:10.5268/IW-1.2.366
Bansal, S., Tangen, B., and Finocchiaro, R. (2016). Temperature and hydrology affect methane emissions from prairie pothole wetlands. Wetlands 36, 371–381. doi:10.1007/s13157-016-0826-8
Bastviken, D., Cole, J., Pace, M., and Tranvik, L. (2004). Methane emissions from lakes: Dependence of lake characteristics, two regional assessments, and a global estimate. Glob. Biogeochem. Cycles 18, n/a. doi:10.1029/2004gb002238
Baulch, H. M., Dillon, P. J., Maranger, R., and Schiff, S. L. (2011). Diffusive and ebullitive transport of methane and nitrous oxide from streams: Are bubble-mediated fluxes important? J. Geophys. Res. 116, G04028. doi:10.1029/2011JG001656
Baulch, H. M., Dillon, P. J., Maranger, R., Venkiteswaran, J. J., Wilson, H. F., and Schiff, S. L. (2012). Night and day: Short‐term variation in nitrogen chemistry and nitrous oxide emissions from streams. Freshw. Biol. 57, 509–525. doi:10.1111/j.1365-2427.2011.02720.x
Baumann, M., Gasparri, I., Piquer‐Rodríguez, M., Pizarro, G. G., Griffiths, P., Hostert, P., et al. (2017). Carbon emissions from agricultural expansion and intensification in the Chaco. Glob. Chang. Biol. 23, 1902–1916. doi:10.1111/gcb.13521
Beaulieu, J. J., Arango, C. P., Hamilton, S. K., and Tank, J. L. (2008). The production and emission of nitrous oxide from headwater streams in the Midwestern United States. Glob. Chang. Biol. 14, 878–894. doi:10.1111/j.1365-2486.2007.01485.x
Beaulieu, J. J., Tank, J. L., Hamilton, S. K., Wollheim, W. M., Hall, R. O., Mulholland, P. J., et al. (2011). Nitrous oxide emission from denitrification in stream and river networks. Proc. Natl. Acad. Sci. U. S. A. 108, 214–219. doi:10.1073/pnas.1011464108
Beaulieu, J. J., DelSontro, T., and Downing, J. A. (2019). Eutrophication will increase methane emissions from lakes and impoundments during the 21st century. Nat. Commun. 10, 1375. doi:10.1038/s41467-019-09100-5
Bergbusch, N. T., Hayes, N. M., Simpson, G. L., Swarbrick, V. J., Quiñones-Rivera, Z. J., and Leavitt, P. R. (2021). Effects of nitrogen removal from wastewater on phytoplankton in eutrophic prairie streams. Freshw. Biol. 66, 2283–2300. doi:10.1111/fwb.13833
Bogard, M. J., and Giorgio, P. A. (2016). The role of metabolism in modulating CO2 fluxes in boreal lakes. Glob. Biogeochem. Cycles 30, 1509–1525. doi:10.1002/2016GB005463
Bogard, M. J., Vogt, R. J., Hayes, N. M., and Leavitt, P. R. (2020). Unabated nitrogen pollution favors growth of toxic cyanobacteria over chlorophytes in most hypereutrophic lakes. Environ. Sci. Technol. 54, 3219–3227. doi:10.1021/acs.est.9b06299
Bridgham, S. D., Megonigal, J. P., Keller, J. K., Bliss, N. B., and Trettin, C. (2006). The carbon balance of North American wetlands. Wetlands 26, 889–916. doi:10.1672/0277-5212(2006)26[889:tcbona]2.0.co;2
Cavaliere, E., and Baulch, H. M. (2018). Denitrification under lake ice. Biogeochemistry 137 (3), 285–295. doi:10.1007/s10533-018-0419-0
Chapin, F. S., Woodwell, G. M., Randerson, J. T., Rastetter, E. B., Lovett, G. M., Baldocchi, D. D., et al. (2006). Reconciling carbon-cycle concepts, terminology, and methods. Ecosystems 9, 1041–1050. doi:10.1007/s10021-005-0105-7
Cole, J. J., and Caraco, N. F. (1998). Atmospheric exchange of carbon dioxide in a low‐wind oligotrophic lake measured by the addition of SF6. Limnol. Oceanogr. 43 (4), 647–656. doi:10.4319/lo.1998.43.4.0647
Cole, J., Prairie, Y., Caraco, N., McDowell, W. H., Tranvik, L. J., Striegl, R. G., et al. (2007). Plumbing the global carbon cycle: Integrating inland waters into the terrestrial carbon budget. Ecosystems 10, 172–185. doi:10.1007/s10021-006-9013-8
Deemer, B. R., Harrison, J. A., Li, S., Beaulieu, J. J., DelSontro, T., Barros, N., et al. (2016). Greenhouse gas emissions from reservoir water surfaces: A new global synthesis. BioScience 66, 949–964. doi:10.1093/biosci/biw117
DelSontro, T., Boutet, L., St‐Pierre, A., Giorgio, P., and Prairie, Y. (2016). Methane ebullition and diffusion from northern ponds and lakes regulated by the interaction between temperature and system productivity. Limnol. Oceanogr. 61, S62–S77. doi:10.1002/lno.10335
DelSontro, T., Beaulieu, J. J., and Downing, J. A. (2018). Greenhouse gas emissions from lakes and impoundments: Upscaling in the face of global change. Limnol. Oceanogr. Lett. 3, 64–75. doi:10.1002/lol2.10073
Denfeld, B. A., Kortelainen, P., Rantakari, M., Sobek, S., and Weyhenmeyer, G. A. (2016). Regional variability and drivers of below ice CO2 in boreal and subarctic lakes. Ecosystems 19, 461–476. doi:10.1007/s10021-015-9944-z
Dillon, P. J., and Molot, L. A. (1997). Dissolved organic and inorganic carbon mass balances in central Ontario lakes. Biogeochemistry 36, 29–42. doi:10.1023/a:1005731828660
Dodds, W. K. (2002). “Aquatic chemistry controlling nutrient cycling: Redox and O2,” in Freshwater Ecology. Editors J. H. Thorp, J. A. Stanford, R. Stein, and R. G. Wetzel (Academic Press), 202–228.
Donald, D. B., Bogard, M. J., Finlay, K., and Leavitt, P. R. (2011). Comparative effects of urea, ammonium, and nitrate on phytoplankton abundance, community composition, and toxicity in hypereutrophic freshwaters. Limnol. Oceanogr. 56, 2161–2175. doi:10.4319/lo.2011.56.6.2161
Downing, J. (2010). Emerging global role of small lakes and ponds: Little things mean a lot. Limnetica 29, 9–24. doi:10.23818/limn.29.02
Dubois, K., Carignan, R., and Veizer, J. (2009). Can pelagic net heterotrophy account for carbon fluxes from eastern Canadian lakes? Appl. Geochem. 24, 988–998. doi:10.1016/j.apgeochem.2009.03.001
Duc, N. T., Crill, P., and Bastviken, D. (2010). Implications of temperature and sediment characteristics on methane formation and oxidation in lake sediments. Biogeochemistry 100, 185–196. doi:10.1007/s10533-010-9415-8
Ducharme-Riel, V., Vachon, D., del Giorgio, P. A., and Prairie, Y. T. (2015). The relative contribution of winter under-ice and summer hypolimnetic CO2 accumulation to the annual CO2 emissions from northern lakes. Ecosystems 18 (4), 547–559. doi:10.1007/s10021-015-9846-0
Earles, J. M., Yeh, S., and Skog, K. E. (2012). Timing of carbon emissions from global forest clearance. Nat. Clim. Chang. 2, 682–685. doi:10.1038/nclimate1535
Finlay, K., Leavitt, P., Wissel, B., and Prairie, Y. (2009). Regulation of spatial and temporal variability of carbon flux in six hard‐water lakes of the northern Great Plains. Limnol. Oceanogr. 54, 2553–2564. doi:10.4319/lo.2009.54.6_part_2.2553
Finlay, K., Leavitt, P., Patoine, A., Patoine, A., and Wissel, B. (2010). Magnitudes and controls of organic and inorganic carbon flux through a chain of hard‐water lakes on the northern Great Plains. Limnol. Oceanogr. 55, 1551–1564. doi:10.4319/lo.2010.55.4.1551
Finlay, K., Vogt, R., Bogard, M., Wissel, B., Tutolo, B., Simpson, G., et al. (2015). Decrease in CO2 efflux from northern hardwater lakes with increasing atmospheric warming. Nature 519, 215–218. doi:10.1038/nature14172
Finlay, K., Vogt, R., Simpson, G., and Leavitt, P. (2019). Seasonality of pCO2 in a hard‐water lake of the northern Great Plains: The legacy effects of climate and limnological conditions over 36 years. Limnol. Oceanogr. 64, 11113. doi:10.1002/lno.11113
Firestone, M. K., and Davidson, E. A. (1989). Microbiological basis of NO and N2O production and consumption in soil. Exch. Trace Gases Between Terr. Ecosyst. Atmos. 47, 7–21.
Glass, J. B., and Orphan, V. J. (2012). Trace metal requirements for microbial enzymes involved in the production and consumption of methane and nitrous oxide. Front. Microbiol. 3, 61. doi:10.3389/fmicb.2012.00061
Gooding, R. M., and Baulch, H. M. (2017). Small reservoirs as a beneficial management practice for nitrogen removal. J. Environ. Qual. 46 (1), 96–104. doi:10.2134/jeq2016.07.0252
Haig, H. A., Hayes, N. M., Simpson, G. L., Yi, Y., Wissel, B., Hodder, K. R., et al. (2020). Comparison of isotopic mass balance and instrumental techniques as estimates of basin hydrology in seven connected lakes over 12 years. J. Hydrology X 6, 100046. doi:10.1016/j.hydroa.2019.100046
Haig, H. A., Hayes, N. M., Simpson, G. L., Yi, Y., Wissel, B., Hodder, K. R., et al. (2021). Effects of seasonal and interannual variability in water isotopes (δ2H, δ18O) on estimates of water balance in a chain of seven prairie lakes. J. Hydrology X 10, 100069. doi:10.1016/j.hydroa.2020.100069
Hanson, P. C., Bade, D. L., Carpenter, S. R., and Kratz, T. K. (2003). Lake metabolism: Relationships with dissolved organic carbon and phosphorus. Limnol. Oceanogr. 48 (3), 1112–1119. doi:10.4319/lo.2003.48.3.1112
Harrison, J. A., Matson, P. A., and Fendorf, S. E. (2005). Effects of a diel oxygen cycle on nitrogen transformations and greenhouse gas emissions in a eutrophied subtropical stream. Aquat. Sci. 67, 308–315. doi:10.1007/s00027-005-0776-3
Hashimoto, S., Sun, H. Y., Nakamura, T., Nojiri, Y., and Otsuki, A. (1993). Seasonal variations in dissolved nitrous oxide concentrations in a eutrophic shallow lake without anaerobic layer. Geochem. J. 27, 117–123. doi:10.2343/geochemj.27.117
Holgerson, M. A., Richardson, D. C., Roith, J., Bortolotti, L. E., Finlay, K., Hornback, D. J., et al. (2022). Classifying mixing regimes in ponds and shallow lakes. Water Resour. Res. 58, e2022WR032522. In press. doi:10.1029/2022WR032522
Holgerson, M. (2015). Drivers of carbon dioxide and methane supersaturation in small, temporary ponds. Biogeochemistry 124, 305–318. doi:10.1007/s10533-015-0099-y
Holgerson, M., and Raymond, P. (2016). Large contribution to inland water CO2 and CH4 emissions from very small ponds. Nat. Geosci. 9, 222–226. doi:10.1038/ngeo2654
Huotari, J., Ojala, A., Peltomaa, E., Pumpanen, J., Hari, P., and Vesala, T. (2009). Temporal variations in surface water CO2 concentration in a boreal humic lake basaed on high-frequency measurements. Boreal Environ. Res. 14, 48–60.
Jeffrey, S. W., and Humphrey, G. F. (1975). New spectrophotometric equations for determining chlorophylls a, b, c1 and c2 in higher plants, algae and natural phytoplankton. Biochem. Physiol. Pflanz. 167, 191–194. doi:10.1016/s0015-3796(17)30778-3
Jonsson, A., Karlsson, J., and Jansson, M. (2003). Sources of carbon dioxide supersaturation in clearwater and humic lakes in northern Sweden. Ecosystems 6, 224–235. doi:10.1007/s10021-002-0200-y
Kankaala, P., Huotari, J., Tulonen, T., and Ojala, A. (2013). Lake‐size dependent physical forcing drives carbon dioxide and methane effluxes from lakes in a boreal landscape. Limnol. Oceanogr. 58, 1915–1930. doi:10.4319/lo.2013.58.6.1915
Kim, J., Verma, S. B., Billesbach, D. P., and Clement, R. J. (1998). Diel variation in methane emission from a midlatitude prairie wetland: Significance of convective throughflow in Phragmites australis. J. Geophys. Res. 103, 28029–28039. doi:10.1029/98jd02441
Kim, S. Y., Veraart, A. J., Meima-Franke, M., and Bodelier, P. L. E. (2015). Combined effects of carbon, nitrogen and phosphorus on CH4 production and denitrification in wetland sediments. Geoderma 259, 354–361. doi:10.1016/j.geoderma.2015.03.015
King, G. M. (1992). Ecological aspects of methane oxidation, a key determinant of global methane dynamics. Adv. Microb. Ecol. 12, 431–468. doi:10.1007/978-1-4684-7609-5_9
Kortelainen, P., Rantakari, M., Huttenen, J. T., Mattsson, T., Alm, J., Juutinen, S., et al. (2006). Sediment respiration and lake trophic state are important predictors of large CO2 evasion from small boreal lakes. Glob. Chang. Biol. 12, 1554–1567. doi:10.1111/j.1365-2486.2006.01167.x
Liu, H., Zhang, Q., Katul, G. G., Cole, J. J., Chapin, F. S., and MacIntyre, S. (2016). Large CO2 effluxes at night and during synoptic weather events significantly contribute to CO2 emissions from a reservoir. Environ. Res. Lett. 11 (6), 064001. doi:10.1088/1748-9326/11/6/064001
Lovley, D. R., Dwyer, D. F., and Klug, M. J. (1982). Kinetic analysis of competition between sulfate reducers and methanogens for hydrogen in sediments. Appl. Environ. Microbiol. 43, 1373–1379. doi:10.1128/aem.43.6.1373-1379.1982
Lovley, D. R., and Klug, M. J. (1983). Sulfate reducers can outcompete methanogens at freshwater sulfate concentrations. Appl. Environ. Microbiol. 45, 187–192. doi:10.1128/aem.45.1.187-192.1983
Maberly, S. C. (1996). Diel, episodic and seasonal changes in pH and concentrations of inorganic carbon in a productive lake. Freshw. Biol. 35, 579–598. doi:10.1111/j.1365-2427.1996.tb01770.x
Macpherson, G. L. (2009). CO2 distribution in groundwater and the impact of groundwater extraction on the global C cycle. Chem. Geol. 264, 328–336. doi:10.1016/j.chemgeo.2009.03.018
Marra, G., and Wood, S. N. (2011). Practical variable selection for generalized additive models. Comput. Stat. Data Anal. 55, 2372–2387. doi:10.1016/j.csda.2011.02.004
McDonald, C., Stets, E., Striegl, R., and Butman, D. (2013). Inorganic carbon loading as a primary driver of dissolved carbon dioxide concentrations in the lakes and reservoirs of the contiguous United States. Glob. Biogeochem. Cycles 27, 285–295. doi:10.1002/gbc.20032
Molina, V., Eissler, Y., Fernandez, C., Cornejo-D'Ottone, M., Dorador, C., Bebout, B. M., et al. (2021). Greenhouse gases and biogeochemical diel fluctuations in a high-altitude wetland. Sci. Total Environ. 768, 144370. doi:10.1016/j.scitotenv.2020.144370
Morales-Pineda, M., Cózar, A., Laiz, I., Úbeda, B., and Gálvez, J. A. (2014). Daily, biweekly, and seasonal temporal scales of pCO2 variability in two stratified Mediterranean reservoirs. J. Geophys. Res. Biogeosci. 119, 509–520. doi:10.1002/2013JG002317
Neubauer, S. C., and Megonigal, J. P. (2015). Moving beyond global warming potentials to quantify the climatic role of ecosystems. Ecosystems 18 (6), 1000–1013. doi:10.1007/s10021-015-9879-4
Neue, H. U., Wassmann, R., Kludze, H. K., Bujun, W., and Lantin, R. S. (1997). Factors and processes controlling methane emissions from rice fields. Nutr. Cycl. Agroecosyst. 49, 111–117. doi:10.1023/a:1009714526204
Ollivier, Q. R., Maher, D. T., Pitfield, C., and Macreadie, P. I. (2018). Punching above their weight: Large release of greenhouse gases from small agricultural dams. Glob. Chang. Biol. 25, 721–732. doi:10.1111/gcb.14477
Ollivier, Q. R., Maher, D. T., Pitfield, C., and Macreadie, P. I. (2019). Winter emissions of CO2, CH4, and N2O from temperate agricultural dams: Fluxes, sources, and processes. Ecosphere 10. doi:10.1002/ecs2.2914
Peacock, M., Audet, J., Jordan, S., Smeds, J., and Wallin, M. B. (2019). Greenhouse gas emissions from urban ponds are driven by nutrient status and hydrology. Ecosphere 10, e02643. doi:10.1002/ecs2.2643
Pennock, D., Yates, T. T., Bedard-Haughn, A., Phipps, K., Farrell, R., and McDougal, R. (2010). Landscape controls on N2O and CH4 emissions from freshwater mineral soil wetlands of the Canadian Prairie Pothole Region. Geoderma 155, 308–319. doi:10.1016/j.geoderma.2009.12.015
Pickett-Heaps, C. A., Jacob, D. J., Wecht, K. J., Kort, E. A., Wofsy, S. C., Diskin, G. S., et al. (2011). Magnitude and seasonality of wetland methane emissions from the Hudson Bay Lowlands (Canada). Atmos. Chem. Phys. 11, 3773–3779. doi:10.5194/acp-11-3773-2011
Piña-Ochoa, E., and Álvarez-Cobelas, M. (2006). Denitrification in aquatic environments: A cross-system analysis. Biogeochemistry 81, 111–130. doi:10.1007/s10533-006-9033-7
Pomeroy, J., Gray, D., Brown, T., Hedstrom, N., Quinton, W., Granger, R., et al. (2007). The cold regions hydrological model: A platform for basing process representation and model structure on physical evidence. Hydrol. Process. 21, 2650–2667. doi:10.1002/hyp.6787
Quick, A. M., Reeder, W. J., Farrell, T. B., Tonina, D., Feris, K. P., and Benner, S. G. (2019). Nitrous oxide from streams and rivers: A review of primary biogeochemical pathways and environmental variables. Earth. Sci. Rev. 191, 224–262. doi:10.1016/j.earscirev.2019.02.021
R Core Team (2021). R: A language and environment for statistical computing. Available at: https://www.R-project.org/.
Rantakari, M., and Kortelainen, P. (2005). Interannual variation and climatic regulation of the CO2 emission from large boreal lakes. Glob. Chang. Biol. 11, 1368–1380. doi:10.1111/j.1365-2486.2005.00982.x
Raymond, P. A., Hartmann, J., Lauerwald, R., Sobek, S., McDonald, C., Hoover, M., et al. (2013). Global carbon dioxide emissions from inland waters. Nature 503, 355–359. doi:10.1038/nature12760
Read, J. S., Hamilton, D. P., Jones, I. D., Muraoka, K., Winslow, L. A., Kroiss, R., et al. (2011). Derivation of lake mixing and stratification indices from high-resolution lake buoy data. Environ. Model. Softw. 26, 1325–1336. doi:10.1016/j.envsoft.2011.05.006
Rosamond, M. S., Thuss, S. J., Schiff, S. L., and Elgood, R. J. (2011). Coupled cycles of dissolved oxygen and nitrous oxide in rivers along a trophic gradient in southern ontario, Canada. J. Environ. Qual. 40, 256–270. doi:10.2134/jeq2010.0009
Rosentreter, J. A., Borgest, A. V., Deemer, B. R., Holgerson, M. A., Liu, S., Song, C., et al. (2021). Half of global methane emissions come from highly variable aquatic ecosystem sources. Nat. Geosci. 14, 225–230. doi:10.1038/s41561-021-00715-2
Roulet, N. T., Crill, P., Comer, N., Dove, A., and Boubonniere, R. (1997). CO2 and CH4 flux between a boreal beaver pond and the atmosphere. J. Geophys. Res. 102, 29313–29319. doi:10.1029/97JD01237
Scott, J. T., McCarthy, M. J., Gardner, W. S., and Doyle, R. D. (2008). Denitrification, dissimilatory nitrate reduction to ammonium, and nitrogen fixation along a nitrate concentration gradient in a created freshwater wetland. Biogeochemistry 87, 99–111. doi:10.1007/s10533-007-9171-6
Segers, R. (1998). Methane production and methane consumption: A review of processes underlying wetland methane fluxes. Biogeochemistry 41, 23–51. doi:10.1023/a:1005929032764
Shao, C., Chen, J., Stepien, C. A., Chu, H., Ouyang, Z., Bridgeman, T. B., et al. (2015). Diurnal to annual changes in latent, sensible heat, and CO2 fluxes over a laurentian Great lake: A case study in western Lake Erie. J. Geophys. Res. Biogeosci. 120, 1587–1604. doi:10.1002/2015JG003025
Smith, V. H., Tilman, G. D., and Nekola, J. C. (1999). Eutrophication: Impacts of excess nutrient inputs on freshwater, marine, and terrestrial ecosystems. Environ. Pollut. 100, 179–196. doi:10.1016/s0269-7491(99)00091-3
Soued, C., del Giorgio, P. A., and Maranger, R. (2016). Nitrous oxide sinks and emissions in boreal aquatic networks in Québec. Nat. Geosci. 9, 116–120. doi:10.1038/ngeo2611
Stets, E. G., Butman, D., McDonald, C. P., Stackpoole, S. M., DeGrandpre, M. D., and Striegl, R. G. (2017). Carbonate buffering and metabolic controls on carbon dioxide in rivers. Glob. Biogeochem. Cycles 31, 663–677. doi:10.1002/2016gb005578
Striegl, R. G., and Michmerhuizen, C. M. (1998). Hydrologic influence on methane and carbon dioxide dynamics at two north-Central Minnesota lakes. Limnol. Oceanogr. 43, 1519–1529. doi:10.4319/lo.1998.43.7.1519
Swarbrick, V. J., Simpson, G. L., Glibert, P. M., and Leavitt, P. R. (2019). Differential stimulation and suppression of phytoplankton growth by ammonium enrichment in eutrophic hardwater lakes over 16 years. Limnol. Oceanogr. 64 (S1), S130–S149. doi:10.1002/lno.11093
Tilman, D. (1999). Global environmental impacts of agricultural expansion: The need for sustainable and efficient practices. Proc. Natl. Acad. Sci. U. S. A. 96, 5995–6000. doi:10.1073/pnas.96.11.5995
Tranvik, L. J., Downing, J. A., Cotner, J. B., Loiselle, S. A., Striegl, R. G., Ballatore, T. J., et al. (2009). Lakes and reservoirs as regulators of carbon cycling and climate. Limnol. Oceanogr. 54, 2298–2314. doi:10.4319/lo.2009.54.6_part_2.2298
Vachon, D., Sadro, S., Bogard, M. J., Lapierre, J.-F., Baulch, H. M., Rusak, J. A., et al. (2019). Paired O2-CO2 measurements provide emergent insights into aquatic ecosystem function. Limnol. Oceanogr. Lett. 5, 287–294. doi:10.1002/lol2.10135
van Bergen, T. J., Barros, N., Mendonça, R., Aben, R. C., Althuizen, I. H., Huszar, V., et al. (2019). Seasonal and diel variation in greenhouse gas emissions from an urban pond and its major drivers. Limnol. Oceanogr. 64 (5), 2129–2139. doi:10.1002/lno.11173
Webb, J. R., Hayes, N. M., Simpson, G. L., Leavitt, P. R., Baulch, H. M., and Finlay, K. (2019a). Widespread nitrous oxide undersaturation in farm waterbodies creates an unexpected greenhouse gas sink. Proc. Natl. Acad. Sci. U. S. A. 116, 9814–9819. doi:10.1073/pnas.1820389116
Webb, J. R., Leavitt, P. R., Simpson, G. L., Baulch, H. M., Haig, H. A., Hodder, K. R., et al. (2019b). Regulation of carbon dioxide and methane in small agricultural reservoirs: Optimizing potential for greenhouse gas uptake. Biogeosciences 16, 4211–4227. doi:10.5194/bg-16-4211-2019
Wiik, E., Haig, H., Hayes, N., Finlay, K., Simpson, G., Vogt, R., et al. (2018). Generalized additive models of climatic and metabolic controls of subannual variation in pCO2 in productive hardwater lakes. J. Geophys. Res. Biogeosci. 123, 1940–1959. doi:10.1029/2018JG004506
Wilkinson, G. M., Buelo, C. D., Cole, J. J., and Pace, M. L. (2016). Exogenously produced CO2 doubles the CO2 efflux from three north temperate lakes. Geophys. Res. Lett. 43, 1996–2003. doi:10.1002/2016gl067732
Winfrey, M. R., and Zeikus, J. G. (1977). Effect of sulfate on carbon and electron flow during microbial methanogenesis in freshwater sediments. Appl. Environ. Microbiol. 33, 275–281. doi:10.1128/aem.33.2.275-281.1977
Wood, S. N. (2011). Fast stable restricted maximum likelihood and marginal likelihood estimation of semiparametric generalized linear models. J. Roy. Stat. Soc. B Stat. Methodol. 73, 3–36. doi:10.1111/j.1467-9868.2010.00749.x
Wood, S. (2016). Package ‘mgcv’. Accessed 23 June 2018. Available from: https://cran.r-project.org/web/packages/mgcv/mgcv.pdf.
Wood, S. N., Pya, N., and Säfken, B. (2016). Smoothing parameter and model selection for general smooth models. J. Am. Stat. Assoc. 111, 1548–1563. doi:10.1080/01621459.2016.1180986
Wu, S., Chen, J., Li, C., Kong, D., Yu, K., Liu, S., et al. (2018a). Diel and seasonal nitrous oxide fluxes determined by floating chamber and gas transfer equation methods in agricultural irrigation watersheds in southeast China. Environ. Monit. Assess. 190, 122. doi:10.1007/s10661-018-6502-0
Wu, S., Hu, Z., Hu, T., Chen, J., Yu, K., Zou, J., et al. (2018b). Annual methane and nitrous oxide emissions from rice paddies and inland fish aquaculture wetlands in southeast China. Atmos. Environ. 175, 135–144. doi:10.1016/j.atmosenv.2017.12.008
Xing, Y., Xie, P., Yang, H., Ni, L., Wang, Y., and Tang, W. (2004). Diel variation of methane fluxes in summer in a eutrophic subtropical lake in China. J. Freshw. Ecol. 19, 639–644. doi:10.1080/02705060.2004.9664745
Yang, P., He, Q., Huang, J., and Tong, C. (2015). Fluxes of greenhouse gases at two different aquaculture ponds in the coastal zone of southeastern China. Atmos. Environ. X. 115, 269–277. doi:10.1016/j.atmosenv.2015.05.067
Yi, Y., Brock, B. E., Falcone, M. D., Wolfe, B. B., and Edwards, T. W. D. (2008). A coupled isotope tracer method to characterize input water to lakes. J. Hydrol. X. 350, 1–13. doi:10.1016/j.jhydrol.2007.11.008
Yvon-Durocher, G., Allen, A. P., Bastviken, D., Conrad, R., Gudasz, C., St-Pierre, A., et al. (2014). Methane fluxes show consistent temperature dependence across microbial to ecosystem scales. Nature 507, 488–491. doi:10.1038/nature13164
Keywords: agricultural pond, seasonality, greenhouse gas fluxes, CO2 equivalent (CO2-eq), methane, nitrous oxide, carbon dioxide
Citation: Jensen SA, Webb JR, Simpson GL, Baulch HM, Leavitt PR and Finlay K (2022) Seasonal variability of CO2, CH4, and N2O content and fluxes in small agricultural reservoirs of the northern Great Plains. Front. Environ. Sci. 10:895531. doi: 10.3389/fenvs.2022.895531
Received: 14 March 2022; Accepted: 04 August 2022;
Published: 03 October 2022.
Edited by:
Yves T. Prairie, Université du Québec à Montréal, CanadaReviewed by:
Alistair Grinham, The University of Queensland, AustraliaFrançois Birgand, North Carolina State University, United States
Jean-Francois Lapierre, Université de Montréal, Canada
Sydney Moyo, Rhodes College, United States
Copyright © 2022 Jensen, Webb, Simpson, Baulch, Leavitt and Finlay. This is an open-access article distributed under the terms of the Creative Commons Attribution License (CC BY). The use, distribution or reproduction in other forums is permitted, provided the original author(s) and the copyright owner(s) are credited and that the original publication in this journal is cited, in accordance with accepted academic practice. No use, distribution or reproduction is permitted which does not comply with these terms.
*Correspondence: Kerri Finlay, Kerri.Finlay@uregina.ca
†Present Address: Jackie R. Webb, Centre for Regional and Rural Futures (CeRRF), Faculty of Science, Engineering and Built Environment, Deakin University, NSW, Australia; Gavin L. Simpson, Department of Animal Science, Aarhus University, Aarhus, Denmark
‡ORCID: Sydney A. Jensen, orcid.org/0000-0001-6671-2172; Jackie R. Webb, orcid.org/0000-0003-1274-363X; Gavin L. Simpson, orcid.org/0000-0002-9084-8413; Helen M. Baulch, orcid.org/0000-0001-9018-4998; Peter R. Leavitt, orcid.org/0000-0001-9805-9307; Kerri Finlay, orcid.org/0000-0001-6835-8832