- 1Institute of Geographic Sciences and Natural Resources Research, Chinese Academy of Sciences, Beijing, China
- 2School of Geoscience and Technology, Southwest Petroleum University, Chengdu, China
- 3HEOA Group, West China School of Public Health and West China Fourth Hospital, Sichuan University, Chengdu, China
- 4Institute for Healthy Cities and West China Research Centre for Rural Health Development, Sichuan University, Chengdu, China
Malaria occurrence is highly related to the geographical distribution of Anopheles dirus (An. dirus) in the South-East Asia Region and Western Pacific Region (SEAR/WPR). Future climate change has been shown to alter the geographical distribution of malaria vectors. However, few studies have investigated the impact of climate change on the potential distribution of An. dirus in the SEAR/WPR. We considered future climate and land-use data under two climate change scenarios for Representative Concentration Pathways (RCP 4.5 and RCP 8.5) and population data from five Shared Socioeconomic Pathways (SSPs), by using three machine learning models, namely, Random Forest (RF), Boosted Regression Trees (BRT), and Maximum entropy (Maxent) to project the geographical distribution of An. Dirus and to estimate the exposed population. A pseudo-absence dataset was generated based on the relationships between model performance and the distance from the pseudo-absence point to the occurrence point in order to improve model accuracy for projection of the Environmentally Suitable Area (ESA) and exposed human population. The results show that the pseudo-absence data corresponding to the distance of 250 km are appropriate for modeling. The RF method ultimately proved to have the highest accuracy. The predicted ESA of An. dirus would mainly be distributed across Myanmar, Thailand, the southern and eastern part of India, Vietnam, the northern part of Cambodia, and the southern part of Laos. The future ESA is estimated to be reduced under the RCP 4.5 climate change scenario. In the 2070s under RCP 8.5, the reduction of ESA is even greater, especially in Thailand (loss of 35.49 10,000 square kilometers), Myanmar (26.24), Vietnam (17.52), and India (15), which may prevent around 282.6 million people from the risk of malaria under the SSP3 scenarios in the SEAR/WPR. Our predicted areas and potential impact groups for An. dirus under future climate change may provide new insights into regional malaria transmission mechanisms and deployment of malaria control measures based on local conditions in the SEAR/WPR’s.
1 Introduction
Malaria is a severe vector-borne disease caused by the transmission of malaria parasites to humans through mosquito bites, and this has caused a tremendous disease burden in sub-Saharan Africa, Southeast Asia, the Western Pacific, and Latin America (Kogan, 2020). It is estimated that 229 million cases and 409,000 malaria deaths were reported worldwide in 2019 (WHO, 2019). The occurrence of malaria depends on the interaction of parasites, malaria vectors, the ambient environment, and human beings. Among these factors, the vector plays the most important role because malaria’s global geographical distribution is highly consistent with that of its vectors (Hay et al., 2004; Sinka et al., 2012). The prediction of changes in the geographic distribution of malaria vectors could provide valuable knowledge in assessing the potential threat of malaria transmission.
Anopheles dirus (An. dirus) is one of the most efficient and geographically extensive malaria vectors (Rosenberg et al., 1990; Meek, 1995). This effectiveness is demonstrated by the fact that An. dirus is a long-lived and anthropophilic malaria vector. This vector species is usually active in the early evening when people are enjoying various indoor and outdoor activities, which thus increases the bite rate and makes humans susceptible to malaria. In addition, An. dirus has been found in more than ten countries in the South-East Asia Region and Western Pacific Region (SEAR/WPR), especially in Myanmar and Thailand, where malaria prevalence is high (Gould et al., 1961; Obsomer et al., 2013; Sriwichai et al., 2016). So, it is necessary to focus on the geographical distribution of An. dirus.
It is worth noting that the geographical distribution of malaria vectors is linked to climate change (Bueno-Marí and Jiménez-Peydró, 2013). Many studies have shown that the spatial distribution patterns of malaria mosquito vectors may change in the context of climate change (Ren et al., 2016; Akpan et al., 2019; Karypidou et al., 2020). For example, Akpan et al. (2019) found that the distribution of An. gambiae s.l. may have large range expansions toward the north under future climate change scenarios (Akpan et al., 2019). Valderrama et al. (2021) found that the geographical area of An. pseudopunctipennis may shift in longitude and latitude in Chile under projected climate scenarios (Valderrama et al., 2021), while Hertig (2019) found that the potential distributions of dominant vectors of human malaria may reduce in some Mediterranean areas (Hertig, 2019). These studies have demonstrated the complexity of the impacts of climate change on the spatial distribution of malaria vectors. At present, only a few studies have been conducted concerning the distribution projection of An. dirus under climate change scenarios (Ren et al., 2016). Ren et al. used the current and future climate data to project the environmentally suitable area for An. dirus in China and found that the environmentally suitable area would increase in the 2030s but decrease in the 2050s (Ren et al., 2016). Ren’s research has given us an insight into the potential distribution of An. dirus in China under climate change conditions. However, existing evidence over the impacts of climate change on An. dirus’s potential distribution in the SEAR/WPR is insufficient, although the SEAR/WPR is more informative on understanding the geographical distribution of malaria vectors.
Machine learning models (for example, Boosted Regression Trees, Random Forest, and Maxent) have been widely used in predicting the geographical distribution of malaria vectors under future climate change scenarios (Hertig, 2019; Karypidou et al., 2020; Valderrama et al., 2021). These models have demonstrated outstanding model performance and prediction results. It is worth noting that such predictive models rely on both presence and absence data, but the absence data are usually replaced by background data or artificial absence data, which are called “pseudo-absence data” (Zaniewski et al., 2002; Engler et al., 2004; Phillips et al., 2009). Many studies have agreed that the choice of pseudo-absence data affects the accuracy of model predictions (VanDerWal et al., 2009; Wisz and Guisan, 2009; Lobo et al., 2010; Barbet-Massin et al., 2012; Senay et al., 2013). These studies focused on the number of pseudo-absence, the geographical distance from presence sites, and the environmental features between pseudo-absence and occurrence points. Several pseudo-absence data-generation approaches have been proposed in species modeling, such as an ENFA-weighted pseudo-absence generation method or an AUC-driven method (Engler et al., 2004; VanDerWal et al., 2009; Wisz and Guisan, 2009; Iturbide et al., 2015). For example, VanDerWal developed an exploratory analysis method of obtaining pseudo-absence data (VanDerWal et al., 2009), who considered the relationships between pseudo-absence data and model performance, finding that the model AUC value was related to pseudo-absence data generated by different distance to occurrence points. However, these methods of pseudo-absence data selection are rarely considered in the distribution projection of malaria vectors.
This study first used the model performance–based approach to generate the pseudo-absence data by considering the various distances to An. dirus occurrence points in the study area (VanDerWal et al., 2009). Then, we collected the geographic raster data on climate change and land-use change and compared three machine learning–based models in predicting An. dirus distributions. Finally, we selected the best model to predict the geographical distribution of An. dirus and examined the changes in the ESA of An. dirus and the exposed human population under current and future climate scenarios. Our study may provide decision-making evidence for regional malaria control in the SEAR/WPR and a streamlined approach reference for modeling the impacts of climate change on malaria vectors.
2 Data
2.1 Study Area
The study area of the South-East Asia Region and Western Pacific Region (SEAR/WPR) has a considerable latitude span, from 47°S to 53°N, including the two most populous countries, China and India, containing about half the world’s population. The SEAR/WPR experiences a high diversity of malaria vector species, had an estimated 8 million malaria cases in 2019, and the deaths reached 12,200 cases (WHO, 2019). Imbalanced environmental sustainability and socio-economic growth problems, coupled with population, geographical locations, and intricate climate types, make this region highly vulnerable to climate change (Yang et al., 2020). In the SEAR/WPR, the temperature may increase by 0.5–2°C by 2030 and 1–7°C by 2070, especially in the arid regions of India and Western China (Preston, 2006). Rainfall may increase in most areas in the future, but the winter rainfall may decline in South and Southeast Asia. There are 25 countries included in the study area (Figure 1), with 10 countries in Southeast Asia and 15 in the Western Pacific, as defined by WHO regions; this excludes small island countries.
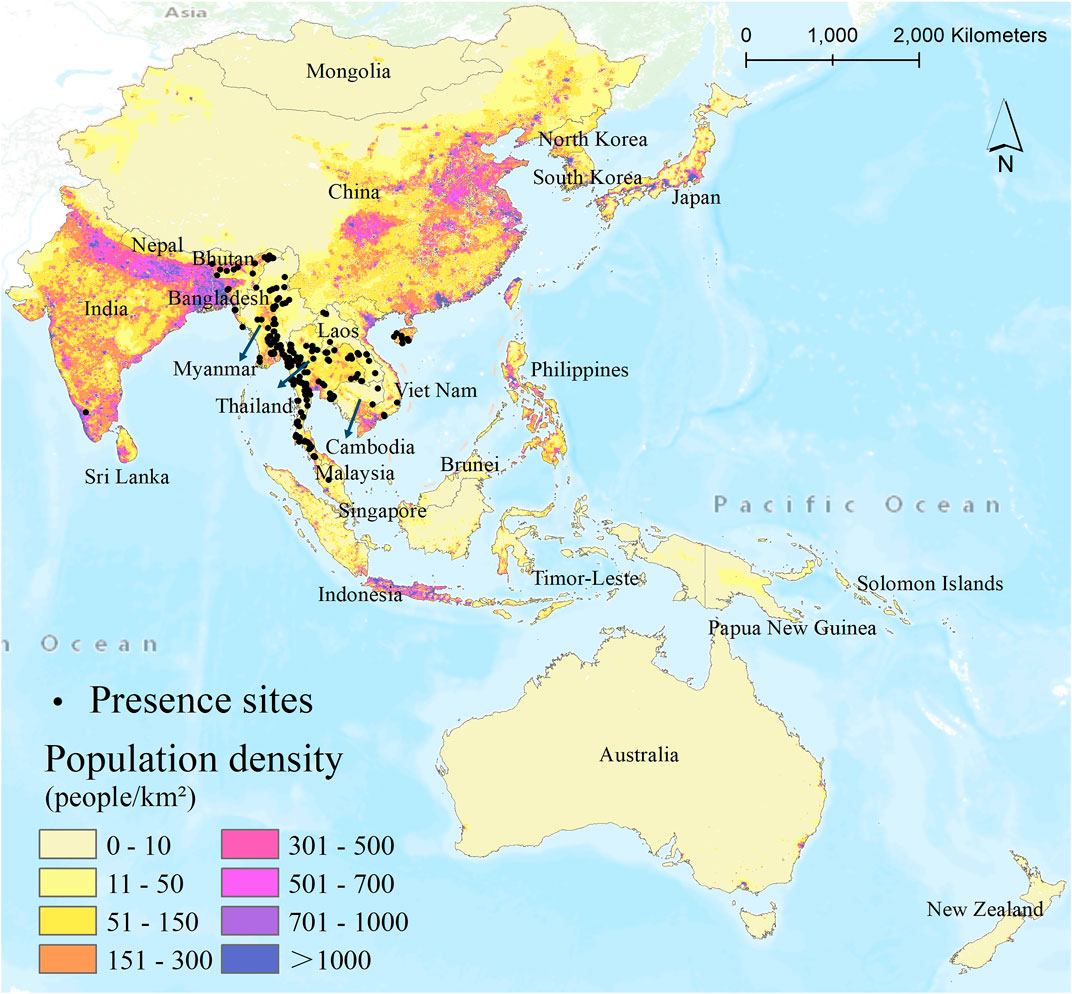
FIGURE 1. Map of the present sites of An. dirus during the baseline period (1983–2000) in the study area of the South-East Asia Region and Western Pacific Region (SEAR/WPR). The base map shows the population distribution at a 1 km2 resolution in 2000.
2.2 An. dirus Presence Data
The An. dirus presence data, with 234 records, were collected from the Malaria Atlas Project (MAP) (https://www.malariaatlas.org/). The data were generated via a systematic literature search of Anopheles distribution and biology and combined expert opinions (Hay et al., 2010; Sinka et al., 2011). In addition, in this study, we combined another An. dirus presence database (Massey et al., 2016) and obtained 77 records. The time range of the An. dirus presence data is filtered from the earliest presence records (1983 to 2000) to represent the baseline/current period. When aggregating the data from the two databases, we deleted those records that did not contain coordinate information or were identified as duplicate records. In addition, only one unique record was retained if multiple records appeared within a 1-kilometer grid. Finally, a total of 228 An. dirus occurrence data were retained for modeling and predicting (Figure 1). We summarized the presence of An. dirus in each country over the study area, as shown in Supplementary Table S1.
2.3 Environmental and Population Data
We utilized bioclimatic environmental data from the Worldclim database (http://www.worldclim.org/), which was processed from global weather station data, widely used, and of good quality (Hijmans et al., 2005; Fick and Hijmans, 2017). The bioclimatic data include 19 variables related to temperature and precipitation, and they can be used to describe environmental variations and climate changes. We chose the bioclimatic data from the 1970–2000 period representing the baseline climate, and their spatial resolution was 30 arc-s (∼1 km2 at the Equator).
Furthermore, we obtained future bioclimatic data from the Climate Change, Agriculture, and Food Security (CCAFS) climate data portal (http://www.ccafs-climate.org/) (Navarro-Racines et al., 2020) under two scenarios regarding RCPs (RCP 4.5 and RCP 8.5) and three-time slices (30-year means): 2030s (2020–2049), 2050s (2040–2069), and 2070s (2060–2089). CCAFS-Climate data corresponds to output data of General Circulation Models (GCMs) from the Coupled Model Intercomparison Project Phase 5 (CMIP5). We chose five bias-correcting GCM output data (GFDL-ESM2M, IPSL-CM5A-LR, MIROC-ESM-CHEM, MOHC-HadGEM2-ES, and NCC-NorESM1-M) to reduce the uncertainty of a single GCM result.
The RCPs are a series of future potential development trajectories that can be used to denote the magnitude of emissions and concentrations of future greenhouse gases (Moss et al., 2010; van Vuuren et al., 2011). We chose two RCPs (RCP4.5 and RCP8.5) to represent potential future climate change scenarios. In RCP4.5, radiative forcing increases to 4.5 W m−2 by 2,100, representing a low, stable emission scenario, while RCP8.5 represents an extreme scenario, with the radiative forcing reaching over 8.5 W m−2 corresponding to significant energy and industry CO2 emissions. These future bioclimatic data meet the spatial resolution requirements for baseline climate data and have been adopted to investigate the effects of future climate change on the vectors of infectious diseases, such as malaria (Laporta et al., 2015; Ryan et al., 2020) and dengue (Messina et al., 2019).
Land-use data were obtained from the Global Ecology Laboratory, University of Maryland (https://luh.umd.edu/) (Hurtt et al., 2011). These data provide an advantage in species distribution research as it is temporally consistent with the bioclimatic data, including the baseline and future scenarios. Because the land-use data were made up of half-degree grids, a bilinear interpolation resample method was applied to ensure that spatial resolution corresponds with the bioclimatic data in R.
Population data on a future spatial population projection under new explicit population scenarios based on Shared Socioeconomic Pathways (SSPs) were obtained from the Climate and Global Dynamics Laboratory (https://www.cgd.ucar.edu/) and further improved from the original resolution of 1/8° to 1 km (Jones and O’Neill, 2016; Gao, 2017). Five SSP pathways represent different development patterns regarding demographics, economics, lifestyles and technological development, governance, and other societal factors (Jones and O’Neill, 2016). In short, SSP1 is a sustainable development path. SSP2 represents the center regarding population development and economic growth. SSP3 and SSP4 denote the regional rivalry and inequality pathways under a relatively low development trajectory. In addition, SSP5 is a fossil fuel–dependent development trajectory. The five SSPs were combined with two RCPs to represent population distribution under future scenarios in our research.
3 Methods
3.1 Machine Learning Models
We adopted three machine learning models (i.e., RF, BRT, and Maxent) to predict the future geographical distribution of An. dirus. The Random Forest (RF) model is an ensemble classifier that combines the results of multiple trees to determine the final predictions using the bootstrapping method (Breiman, 2001). The RF model can also identify essential variables and produce current and future species distribution predictions (Evans et al., 2011).
The Boosted Regression Trees (BRT) model combines two algorithms (Elith et al., 2008). One is the regression tree, the goal of which is to determine the response to predictor factors based on a recursive binary splits method. Another one is boosting, an ensemble learning method similar to RF, but the process is different in terms of generating results (Svetnik et al., 2003; De’ath, 2007). The BRT model can generate highly reliable results and significantly improve predictive power (Sugawara and Nikaido, 2014).
The concept behind the maximum entropy method (Maxent) in SDMs is based on using environmental information on known species presence sites to find the species probability distribution of maximum entropy (closest to uniform) and estimate the probability distribution (Phillips et al., 2006). The prediction result is generated by establishing functional relationships between species presence and environmental information. The Maxent model has more condensed mathematical formulations than other machine learning technologies, such as RF and BRT (Phillips et al., 2006). The implementation of the three abovementioned models was performed in R (Version 4.0.3) with the “dismo,” “gbm,” and “randomForest” packages and the Maxent (Version 3.4.1) interface.
3.2 Model Evaluation
Among RF, BRT, and Maxent, we aimed to select the model with the best performance to conduct the distribution prediction for An. dirus. First, we randomly split the dataset into 75% and 25% subsets for model training and testing. The process was repeated 30 times, producing 30 groups of training and testing datasets to increase the robustness of model prediction. Second, in order to evaluate model performance, we used two threshold-independent metrics based on threshold dependence (Liu et al., 2011), namely, the area under the receiver operating characteristic curve (AUC-ROC) and the area under the precision-recall curve (AUC-PRC), and two threshold-dependent metrics (accuracy and precision). To be specific, AUC-ROC is a standard indicator representing the discrimination ability for species distribution models (Fielding and Bell, 1997; West et al., 2016). However, AUC-ROC could be misleading in terms of model evaluation when facing an imbalanced dataset with rare occurrence points and a large number of pseudo-absence points (Saito and Rehmsmeier, 2015). In this case, AUC-PRC is more suitable for use in evaluating the predictive model performance when the model data are imbalanced (Saito and Rehmsmeier, 2015; Sofaer et al., 2019). Regarding threshold-dependent metrics, the index of accuracy is the proportion of all correct predictions, and the index of precision is the proportion of positive observations that are predicted to be positive (Liu et al., 2005). These are often used to evaluate the performance of machine learning technologies (Ren et al., 2018; June 2021). The 10th percentile training presence value was used to calculate the threshold-dependent metrics because it provided high correct classification rates for validation (Vale et al., 2014).
3.3 Pseudo-Absence Data Generation
A species distribution model requires using the values of 0 and 1, where 0 represents the absence and 1 represents the presence. Absence data are often artificially generated (called “pseudo-absence data”), and there are various approaches to generating pseudo-absence data. In this study, according to VanDerWal’s proposed exploratory approach to pseudo-absence data generation (VanDerWal et al., 2009), we conducted a four-step procedure to generate our pseudo-absence data. First, we obtained 10,000 random points in the study area (Urbani et al., 2017). Second, we calculated the distance from each random point to the nearest presence point and excluded those points within a given distance to provide different datasets based on varying different distances. Third, we used the pseudo-absence and presence data to compose the training and testing datasets for modeling and then evaluated the model performance. Finally, trend curves based on the distance and metrics values were plotted. We determined the optimal pseudo-absence data when the model metric values were stable with increased distances (VanDerWal et al., 2009).
4 Results
4.1 Variable Screening
In choosing the final variables for modeling, we took three separate steps. First, we utilized the Maxent model for univariate variables, in which variables did not contribute to the model if their AUC values were below 0.5 and were, therefore, excluded. Second, we screened for variables with biological importance for An. dirus survival (Ren et al., 2016). Third, Pearson’s correlation coefficients were calculated to identify the potential collinearity between the variables. We then excluded the variable with the lower AUC value when the correlation coefficient between the two variables was greater than 0.75 (Tuanmu et al., 2013). The land use variables were chosen as they provided essential information regarding An. dirus occurrence.
As a result, we selected seven variables as predictors for the projection of An. dirus distribution (Supplementary Table S2). Specifically, three bioclimatic variables were selected: annual temperature range, mean temperature of the warmest quarter, and precipitation during the wettest quarter. Four land use variables were selected: pasture, cropland, primary vegetation land, and urban land. In addition, the temporal change of climatic variables based on five GCMs and two climate change scenarios are plotted in Supplementary Figure S1. We found that the annual temperature range may increase by 0–0.5°C in the occurrence area of An. dirus. The mean temperature of the warmest quarter may rise by more than 4°C in the 2070s under RCP 8.5, while the precipitation of the wettest quarter shows only a slight increase in the future.
4.2 Selection of Pseudo-Absence Data
Figure 2 depicts the relationships between model performance (AUC-ROC, AUC-PRC, accuracy, and precision) and the pseudo-absence datasets obtained by using distances to occurrence points. All four metrics are increased with an increase in the distance until the values approach 1. The result of the three models shows a consistent trend. It is worth noting that the AUC-ROC and accuracy values are always high. In contrast, AUC-PRC and precision values vary widely, showing an obvious increase with distance. Here, in line with a previous study (VanDerWal et al., 2009), we selected the pseudo-absence data corresponding to a distance value of 250 km.
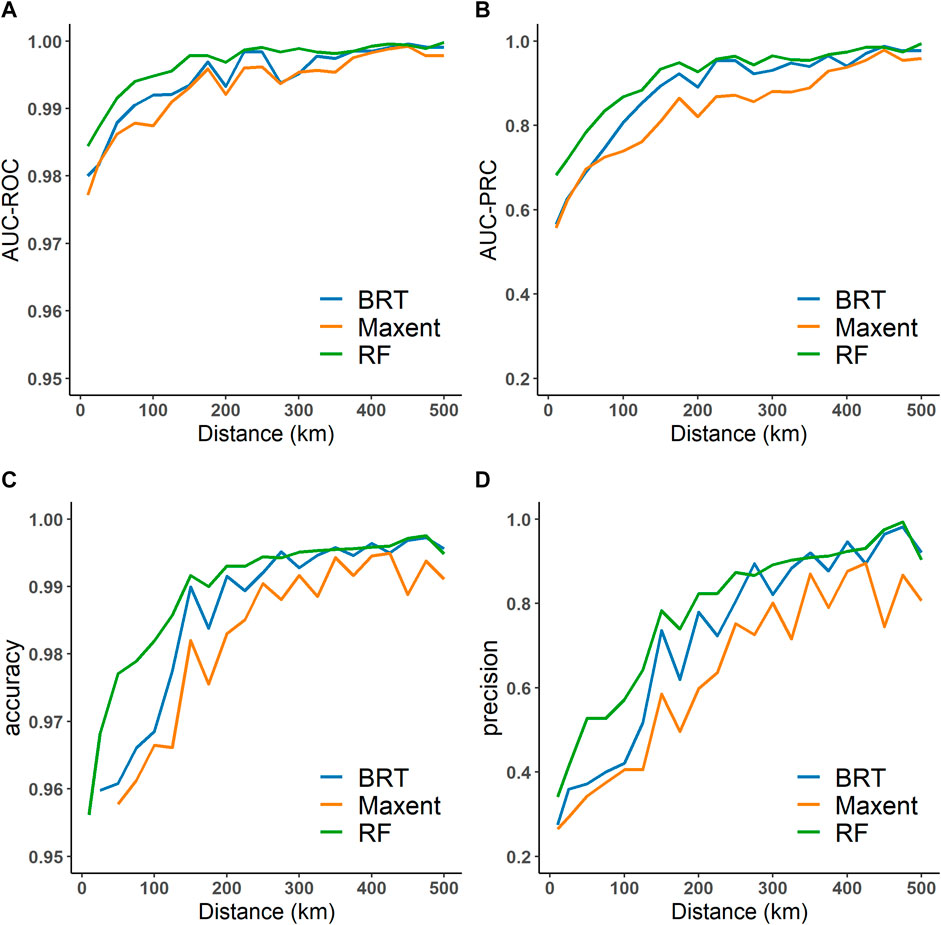
FIGURE 2. Relationships between model performance and the pseudo-absence datasets selected based on various distances: (A) AUC-ROC, (B) AUC-PRC, (C) accuracy, and (D) precision. The distance refers to the radius from An. dirus occurrence points. The models include Boosted Regression Trees (BRT), Random Forest (RF), and the Maximum entropy method (Maxent).
4.3 Model Performance
Table 1 shows that there is not much difference between the three models regarding the AUC-ROC and accuracy metrics. These two metric estimates are all above 0.9. In contrast, AUC-PRC and precision show apparent differences between the three models. The AUC-PRC value of the BRT model is close to the RF (both are greater than 0.9), but the BRT model (0.779) shows poorer performance than RF (0.867) for precision. The Maxent model performed the worst of the three models by all measures. Based on the quality of the output, we chose the RF model to conduct the distribution prediction for An.dirus.
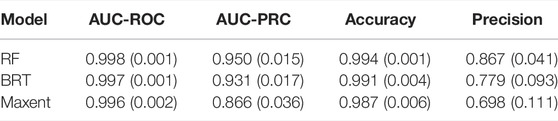
TABLE 1. Evaluation of three alternative machine learning models (RF, BRT, and Maxent). Values in parenthesis are the average standard deviation calculated from 30 iterations.
The relative importance levels of environmental variables for An. dirus based on the RF model are summarized in Supplementary Figure S2 (Song et al., 2020; Zhang et al., 2021). On the one hand, temperature variables, including the annual temperature range and the mean temperature of the warmest quarter, make an essential contribution to predicting the distribution of An. dirus. On the other hand, two land-use variables, the fraction of each grid cell in pasture and the fraction of each grid cell in primary vegetation land, are identified as the primary factors for the occurrence probability of An. dirus. The results also show that the fraction of each grid cell in cropland, the fraction of each grid cell in urban land, and the precipitation during the wettest quarter may have relatively low importance.
4.4 Prediction of Current and Future Environmentally Suitable Area
We divided the continuous probability of RF prediction into suitable/unsuitable using the 10th percentile training presence value as a threshold (Vale et al., 2014). The ESA represents the area where the predictive An. dirus occurrence probability is greater than the threshold. Conversely, we supposed that the area with a smaller probability value than the threshold is not suitable for An. dirus. The predicted current ESA for An. dirus is mainly distributed in Myanmar, Thailand, the southern and eastern parts of India, Vietnam, the northern part of Cambodia, and the southern part of Laos. Bangladesh, the Hainan province of China, and the northern part of Malaysia are also predicted in a few ESAs (Figure 3A). The detailed statistics are summarized in Supplementary Table S3. Furthermore, the predicted results based on five GCMs show that under both future climate scenarios, there is considerable reduction of An. dirus ESA (Supplementary Figure S3), especially in Thailand (loss of 35.49 ten thousand square kilometers under RCP8.5 in the 2070s), Myanmar (26.24), Vietnam (17.52), and India (15) under the RCP 8.5 scenario (Figure 4). In addition, the loss of ESA is larger than the increase in ESA for An.dirus in Thailand, Myanmar, Vietnam, and Cambodia under both scenarios. The lost ESA has 76.66 ten thousand square kilometers across the entire study in the 2070s under RCP 4.5, while the ESA gained is only 12.97. The predicted potential increase is mainly in India, Laos, and Malaysia (Figure 4). The ESA of the southern part of India has a notable tendency to expand northward under RCP 8.5. Moreover, the ESAs of Laos and Malaysia exhibit a slight tendency to move to the north and south, respectively, under RCP 4.5.
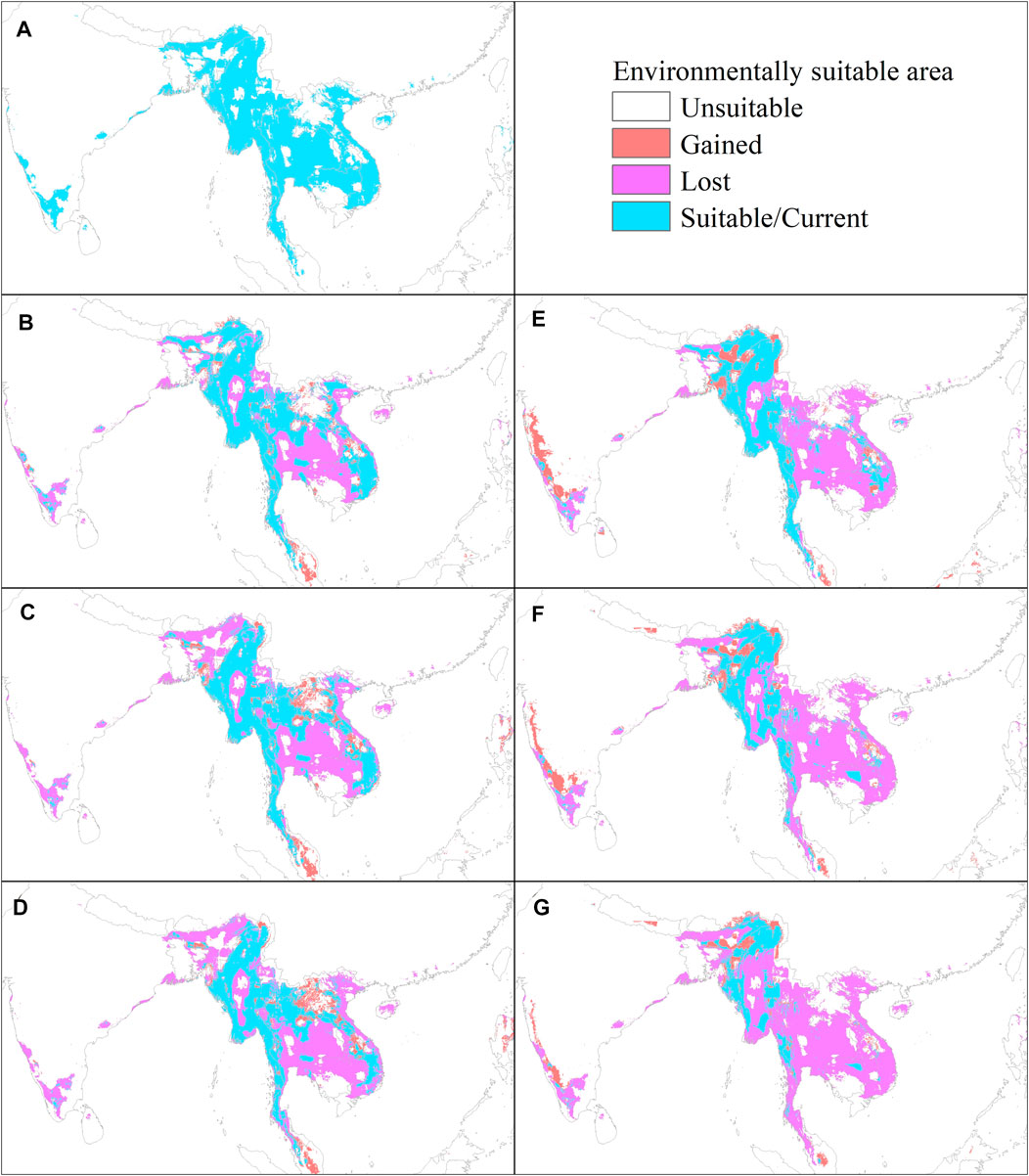
FIGURE 3. Geographical distribution of the projected Environmentally Suitable Area (ESA) of An. dirus in the current (1983–2000) and in the future under scenarios of RCP 4.5 and RCP 8.5 in SEAR/WPR. (A) Current and (B–D) future conditions of the 2030s, 2050s, and 2070s under RCP 4.5, and (E–G) future conditions of the 2030s, 2050s, and 2070s under RCP 8.5. The unsuitable class in the legend represents that the predicted probability is lower than the threshold. The suitable class represents a higher predicted probability than the threshold. The gained and lost classes represent the gained ESA and lost ESA, respectively, calculated based on the current distribution as the reference. Predictions are based on an ensemble of predictions from five general circulation models (GFDL-ESM2M, IPSL-CM5A-LR, MIROC-ESM-CHEM, MOHC-HadGEM2-ES, and NCC-NorESM1-M).
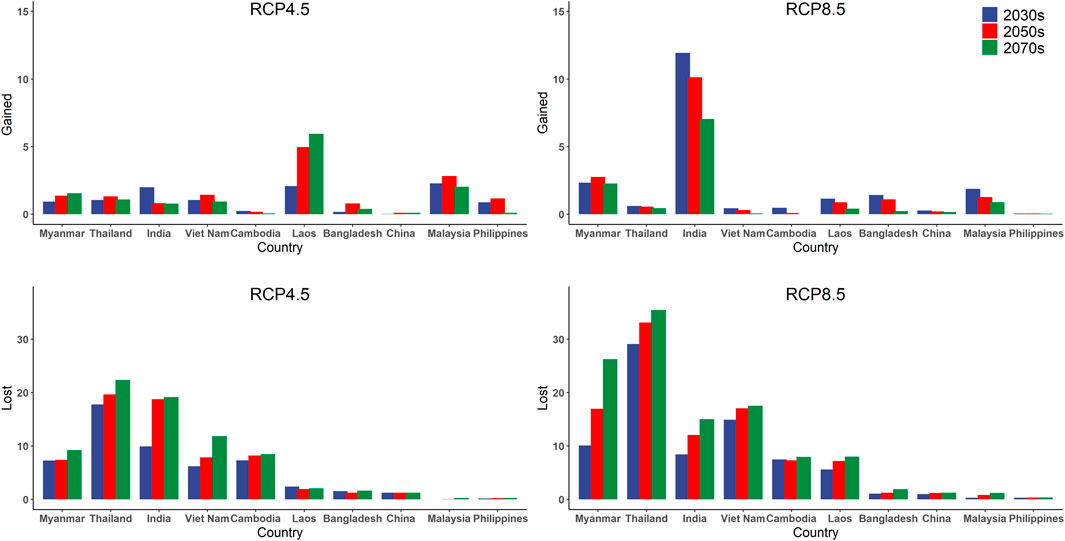
FIGURE 4. Gained and lost sizes of the projected Environmentally Suitable Area (ESA) of An. dirus by the country under scenarios of RCP 4.5 and RCP 8.5 in the 2030s, 2050s, and 2070s. The results are based on an ensemble of predictions from five general circulation models (GFDL-ESM2M, IPSL-CM5A-LR, MIROC-ESM-CHEM, MOHC-HadGEM2-ES, and NCC-NorESM1-M). The x-axis shows the top 10 countries with the most remarkable change in ESA. Countries are sorted from left to right based on the current ESA. Countries not shown indicate that no ESA is predicted. The gained and lost values of ESA are calculated by comparing the ESA of the baseline period. The y-axis unit is ten thousand square kilometers.
4.5 Estimation of Exposed Population
The changes in the estimated exposed population based on the gained and lost ESA under five SSP scenarios and two RCP scenarios in the 2030s, 2050s, and 2070s are summarized in Figure 5. The detailed statistics are listed in Supplementary Table S4. Overall, the human population exposed to the lost ESA is significantly larger than those exposed to the gained ESA. Temporally, the population exposed to the lost ESA may gradually increase from the 2030s to the 2070s under both scenarios. The population exposed to the lost ESA may reach 164.5 million people in the 2030s, 220.5 million in the 2050s, and 257.7 million in the 2070s under the RCP 4.5–SSP3 scenario. However, the population exposed to the gained ESA would be small and will gradually decrease in the future under RCP 8.5 (Figure 5). The population exposed to the gained ESA is expected to be 47.5 million people in the 2030s, 43.7 in the 2050s, and 19.1 in the 2070s under the RCP 8.5–SSP3 scenario. Comparing the two climate change scenarios, we find that the change in the population exposed to the gained and lost ESA of An. dirus in the future under the RCP 8.5 scenarios may be greater than that under the RCP 4.5 scenario. Comparing the five SSPs, we find that the relationship between population exposure changes is mostly consistent with each SSP scenario. The only difference is that it may have the highest population exposure under the SSP3 scenario rather than other SSPs. It is estimated that there may be 282.6 million people exposed to the lost ESA of An. dirus in the 2070s under the RCP 8.5-SSP3 scenario.
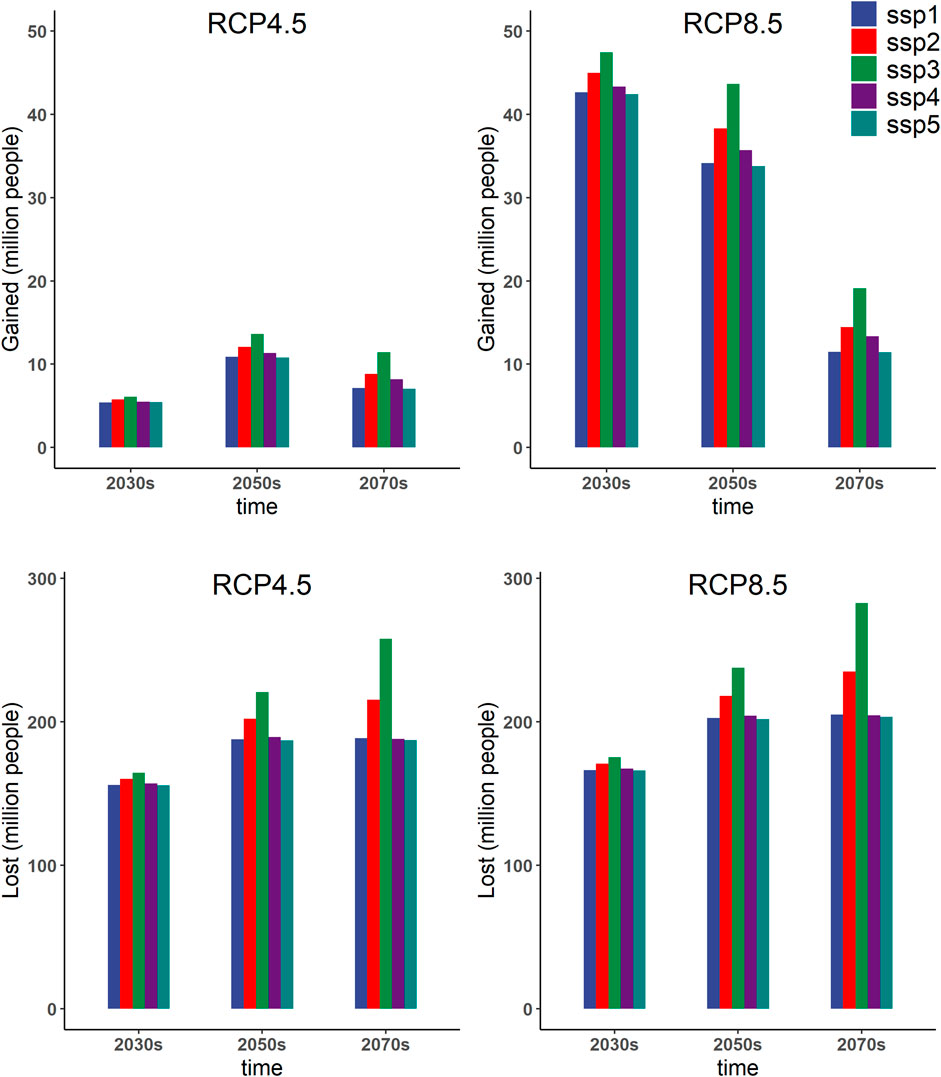
FIGURE 5.Statistics of projected gained and lost exposed human populations based on the variation of ESA of An. dirus under scenarios of RCP 4.5 and RCP 8.5 in the 2030s, 2050s, and 2070s. The results are based on an ensemble of predictions from five general circulation models (GFDL-ESM2M, IPSL-CM5A-LR, MIROC-ESM-CHEM, MOHC-HadGEM2-ES, and NCC-NorESM1-M). The x-axis shows different future times. The y-axis represents the human population exposed to gained and lost ESA of An.dirus.
5 Discussion
Understanding the current and future geographical distribution of malaria vectors may provide critical clues in identifying potential malaria transmission areas. In this study, we assessed the current and future effects of climate and land-use changes on the geographical distribution of An. dirus under scenarios RCP 4.5 and RCP 8.5 in the SEAR/WPR based on the RF model. The current predicted results show that the ESA for An. dirus is mainly distributed across Myanmar, Thailand, the southern and eastern parts of India, Vietnam, the northern part of Cambodia, and the southern part of Laos. Our predicted current distribution of An. dirus is largely consistent with other research (Sinka et al., 2011; Obsomer et al., 2012; Moyes et al., 2016), and the advantage of this study is to identify the potential change of distribution An. dirus in the noted regions.
The geographical distribution of An. dirus may be significantly reduced under future climate change in the SEAR/WPR, providing new insights into future malaria transmission patterns in these regions. Furthermore, we assessed how many exposed humans might benefit from future climate change in these regions against the background of the malaria-associated Anopheles dirus. Fine-scale mapping with 1-kilometer resolution can ensure the accurate implementation of regional malaria control measures. Moreover, we considered the environmental factors related to the future land-use change to provide more accurate geographical predictions than other studies (Obsomer et al., 2012).
Regarding variable importance, we found that temperature plays a major role in predicting the distribution of An. dirus. Previous studies have shown that the annual temperature range is a key environmental variable and a negative near-linear relationship exists between the annual temperature range and the probability of occurrence for An. dirus (Ren et al., 2016). The mean temperature of the warmest quarter will affect the entire An. dirus life process, including egg, larva, pupa, and adult (Beck-Johnson et al., 2017). Higher mean temperatures of the warmest quarter in the future may be the main reason for the reduction of An. dirus ESA (Supplementary Figure S1). In addition, the land-use variables regarding the fraction of each grid cell in the pasture and the fraction of each grid cell in primary vegetation land are identified as important factors, which could be related to the living environment of An. dirus, such as breeding sites and larval survival (Htay-Aung et al., 1999; Obsomer et al., 2007).
Our study found a staggering loss of the ESA for An. dirus under future climate and land-use changes in the SEAR/WPR. It is estimated that a large amount of ESA loss for An. dirus may occur in Thailand (35.5 ten thousand square kilometers), Myanmar (26.2), Vietnam (17.5), and India (19.2) in the 2070s under the RCP 8.5 scenario. This may be related to rising temperature and land-use transformation in the future. For instance, in countries with previously suitable environmental conditions, such as Thailand, deforestation would lead to the destruction of An. Dirus’s habitat (Guerra et al., 2006; Zeng et al., 2018). In addition, the excessive temperature and erratic precipitation would further reduce the survival rate of the mosquito populations (Paaijmans et al., 2007; Murdock et al., 2016). Subsequent studies should be conducted to investigate the response relationship between correlated variables such as temperature and the ESA of An. dirus in order to explain the changes of An. dirus ESA under the context of climate change.
We predicted that the future ESA of An. dirus may increase in India, Laos, and Malaysia, but this differs between climate change scenarios. For example, the ESA for An. dirus expands from the southern part of India to the further north under the RCP 8.5 scenario. Such an explanation may be due to the rising temperature under RCP 8.5 scenarios, and the existing forest areas are making the environmental conditions more suitable for An. dirus’s survival (Palanisami et al., 2019; Hinz et al., 2020). Meanwhile, in Laos and Malaysia, the newly gained ESA for An. dirus is more likely to appear under the RCP 4.5 scenario rather than the RCP 8.5 scenario. This could be due to the temperature and vegetation there meeting the survival conditions of An. dirus under a stable emission scenario.
Climate change has an uncertain impact on the distribution of malaria vectors. On the one hand, climate change could have a negative impact, such as expanding the distribution area of malaria vectors and increasing the population at risk (Karypidou et al., 2020; Ryan et al., 2020). However, climate change could also be an opportunity for malaria eradication in some regions, such as the Mediterranean area (Hertig, 2019) or some overheated areas (Murdock et al., 2016). We used the predicted demographic data from five SSP scenarios to calculate the exposed population and found that the number of people exposed to the reduced ESA may gradually increase. Humans may benefit more substantially in the context of regional rivalry (SSP3) than other SSPs. It is estimated that in the 2070s, under the scenarios of RCP 8.5–SSP3, climate change may potentially prevent approximately 282.6 million people from living in environmentally suitable areas of An. dirus. Moreover, this information on the decrease of the exposed population may help further understand the burden of malaria for countries in the SEAR/WPR to make a reasonable investment in terms of economic and social resources.
This study concludes that humans may benefit from the shrinking of the potential spatial distribution area of An. dirus under the future climate change, but this is only a result of the potential impact of climate change on the malaria vectors in the SEAR/WPR. Neither a single species nor a region is entirely representative. Therefore, our findings do not indicate that we encourage countries to maintain or potentially increase emissions to improve malaria control in the future. In fact, many studies have shown an increase in the number of people at risk of malaria worldwide (Caminade et al., 2014). Thus, we still suggest that countries should actively take measures to reduce carbon emissions in order to protect human beings from the threat of climate change and promote human development.
This study has several limitations. First, we assumed that the current relationships between the environmental variables and the presence probability of An. dirus are applicable under future environmental conditions. However, such relationships may change when the mosquitoes have evolved in a changing environment (Tonnang et al., 2010; Ren et al., 2016). Second, we did not consider anthropogenic factors, such as urbanization, human migration, and other human behaviors, that may also influence the suitable habitat for An. dirus (Hume et al., 2003; Qi et al., 2012). Third, other dominant malaria vectors should be further investigated in SEAR/WPR to systematically assess the impact of climate change on the risk of malaria transmission in the region (Sinka et al., 2011).
6 Conclusion
Focusing on the SEAR/WPR, we used the future climate and land-use data to project the geographical distribution of An. dirus under two climate change scenarios, RCP 4.5 and RCP 8.5. Among three alternative machine-learning technologies, RF had better overall performance than the BRT and Maxent technologies. We adopted a practical approach of pseudo-absence generation to improve the models’ predictive accuracies. We utilized the ESA of An. dirus and the population exposed to the ESA of An. dirus to measure the potential impacts of climate change and future population changes. We detected significant reduction in the ESA of An. dirus and a considerable decrease in the exposed population based on the lost ESA under future climate change scenarios in the study area, indicating that climate change may positively affect potential malaria transmission. Among the potential influencing variables of climate and land use, pastures, primary vegetation land, annual temperature range, and mean temperature of the warmest quarter are relatively more essential in the geographical prediction of An. dirus distribution. Our findings are expected to help country-specific departments develop regional malaria prevention and control strategies based on local conditions and provide a reference method for similar species distribution modeling research.
Data Availability Statement
The original contributions presented in the study are included in the article/Supplementary Material; further inquiries can be directed to the corresponding author.
Author Contributions
XL: Data curation, Methodology, Formal analysis, Software, Validation, Visualization, Writing—Original draft, and Writing—Review and editing. CS: Data curation, Formal analysis, Writing—Review and editing, Funding acquisition, and Project administration. ZR: Conceptualization, Writing—original draft, Writing—Review and editing, Funding acquisition, Project administration, and Supervision. SW: Writing—review and editing and Visualization.
Funding
This study was supported by the National Natural Science Foundation of China (Nos. 42071377 and 42071379), the Strategic Priority Research Program of the Chinese Academy of Sciences (No. XDA20030302), the Medical Science and Technology Project of Sichuan Provincial Health Commission (No. 21PJ067), and the Fund for Introducing Talents of Sichuan University (YJ202157).
Conflict of Interest
The authors declare that the research was conducted in the absence of any commercial or financial relationships that could be construed as a potential conflict of interest.
Publisher’s Note
All claims expressed in this article are solely those of the authors and do not necessarily represent those of their affiliated organizations, or those of the publisher, the editors, and the reviewers. Any product that may be evaluated in this article, or claim that may be made by its manufacturer, is not guaranteed or endorsed by the publisher.
Supplementary Material
The Supplementary Material for this article can be found online at: https://www.frontiersin.org/articles/10.3389/fenvs.2022.841966/full#supplementary-material
References
Akpan, G. E., Adepoju, K. A., and Oladosu, O. R. (2019). Potential Distribution of Dominant Malaria Vector Species in Tropical Region under Climate Change Scenarios. PLoS One 14, e0218523–21. doi:10.1371/journal.pone.0218523
Barbet-Massin, M., Jiguet, F., Albert, C. H., and Thuiller, W. (2012). Selecting Pseudo-absences for Species Distribution Models: How, where and How Many? Methods Ecol. Evol. 3, 327–338. doi:10.1111/j.2041-210X.2011.00172.x
Beck-Johnson, L. M., Nelson, W. A., Paaijmans, K. P., Read, A. F., Thomas, M. B., and Bjørnstad, O. N. (2017). The Importance of Temperature Fluctuations in Understanding Mosquito Population Dynamics and Malaria Risk. R. Soc. open Sci. 4, 160969. doi:10.1098/rsos.160969
Bueno-Marí, R., and Jiménez-Peydró, R. (2013). Global Change and Human Vulnerability to Vector-Borne Diseases. Front. Physiol. 4, 136–173. doi:10.3389/fphys.2013.00158
Caminade, C., Kovats, S., Rocklov, J., Tompkins, A. M., Morse, A. P., Colón-González, F. J., et al. (2014). Impact of Climate Change on Global Malaria Distribution. Proc. Natl. Acad. Sci. U.S.A. 111, 3286–3291. doi:10.1073/pnas.1302089111
De’ath, G. (2007). Boosted Trees for Ecological Modeling and Prediction. Ecology 88, 243–251. doi:10.1890/0012-9658(2007)88[243:BTFEMA]2.0.CO;2
Elith, J., Leathwick, J. R., and Hastie, T. (2008). A Working Guide to Boosted Regression Trees. J. Anim. Ecol. 77, 802–813. doi:10.1111/j.1365-2656.2008.01390.x
Engler, R., Guisan, A., and Rechsteiner, L. (2004). An Improved Approach for Predicting the Distribution of Rare and Endangered Species from Occurrence and Pseudo-absence Data. J. Appl. Ecol. 41, 263–274. doi:10.1111/j.0021-8901.2004.00881.x
Evans, J. S., Murphy, M. A., Holden, Z. A., and Cushman, S. A. (2011). Modeling Species Distribution and Change Using Random Forest. New York, NY: Springer, 139–159. doi:10.1007/978-1-4419-7390-0
Fick, S. E., and Hijmans, R. J. (2017). WorldClim 2: New 1‐km Spatial Resolution Climate Surfaces for Global Land Areas. Int. J. Climatol. 37, 4302–4315. doi:10.1002/joc.5086
Fielding, A. H., and Bell, J. F. (1997). A Review of Methods for the Assessment of Prediction Errors in Conservation Presence/absence Models. Envir. Conserv. 24, 38–49. doi:10.1017/S0376892997000088
Gao, J. (2017). Downscaling Global Spatial Population Projections from 1/8-degree to 1-km Grid Cells. NCAR Technical Note (No. NCAR/TN-537+STR), 9. doi:10.5065/D60Z721H
Gould, D. J., Scanlon, J. E., and Ward, R. A. (1961). Anopheles Vectors of Malaria in Southeast Asia. Medicine, 361–373.
Guerra, C. A., Snow, R. W., Hay, S. I., and Hay, S. I. (2006). A Global Assessment of Closed Forests, Deforestation and Malaria Risk. Ann. Trop. Med. Parasitol. 100, 189–204. doi:10.1179/136485906X91512
Hay, S. I., Guerra, C. A., Tatem, A. J., Noor, A. M., and Snow, R. W. (2004). The Global Distribution and Population at Risk of Malaria: Past, Present, and Future. Lancet Infect. Dis. 4, 327–336. doi:10.1016/S1473-3099(04)01043-6
Hay, S. I., Sinka, M. E., Okara, R. M., Kabaria, C. W., Mbithi, P. M., Tago, C. C., et al. (2010). Developing Global Maps of the Dominant anopheles Vectors of Human Malaria. PLoS Med. 7, e1000209. doi:10.1371/journal.pmed.1000209
Hertig, E. (2019). Distribution of Anopheles Vectors and Potential Malaria Transmission Stability in Europe and the Mediterranean Area under Future Climate Change. Parasites Vectors 12, 18. doi:10.1186/s13071-018-3278-6
Hijmans, R. J., Cameron, S. E., Parra, J. L., Jones, P. G., and Jarvis, A. (2005). Very High Resolution Interpolated Climate Surfaces for Global Land Areas. Int. J. Climatol. 25, 1965–1978. doi:10.1002/joc.1276
Hinz, R., Sulser, T. B., Huefner, R., Mason‐D’Croz, D., Dunston, S., Nautiyal, S., et al. (2020). Agricultural Development and Land Use Change in India: A Scenario Analysis of Trade‐Offs between UN Sustainable Development Goals (SDGs). Earths Future 8, e2019EF001287. doi:10.1029/2019EF001287
Htay-Aung, , , Minn, S., Thaung, S., Mya, M. M., Than, S. M., Hlaing, T., et al. (1999). Well-breeding Anopheles Dirus and Their Role in Malaria Transmission in Myanmar. Southeast Asian J. Trop. Med. Public Health 30, 447–453.
Hume, J. C. C., Lyons, E. J., and Day, K. P. (2003). Human Migration, Mosquitoes and the Evolution of Plasmodium Falciparum. Trends Parasitol. 19, 144–149. doi:10.1016/S1471-4922(03)00008-4
Hurtt, G. C., Chini, L. P., Frolking, S., Betts, R. A., Feddema, J., Fischer, G., et al. (2011). Harmonization of Land-Use Scenarios for the Period 1500-2100: 600 Years of Global Gridded Annual Land-Use Transitions, Wood Harvest, and Resulting Secondary Lands. Clim. Change 109, 117–161. doi:10.1007/s10584-011-0153-2
Iturbide, M., Bedia, J., Herrera, S., del Hierro, O., Pinto, M., and Gutiérrez, J. M. (2015). A Framework for Species Distribution Modelling with Improved Pseudo-absence Generation. Ecol. Model. 312, 166–174. doi:10.1016/j.ecolmodel.2015.05.018
Jones, B., and O’Neill, B. C. (2016). Spatially Explicit Global Population Scenarios Consistent with the Shared Socioeconomic Pathways. Environ. Res. Lett. 11, 084003. doi:10.1088/1748-9326/11/8/084003
Jun, M.-J. (2021). A Comparison of a Gradient Boosting Decision Tree, Random Forests, and Artificial Neural Networks to Model Urban Land Use Changes: the Case of the Seoul Metropolitan Area. Int. J. Geogr. Inf. Sci. 35, 2149–2167. doi:10.1080/13658816.2021.1887490
Karypidou, M. C., Almpanidou, V., Tompkins, A. M., Mazaris, A. D., Gewehr, S., Mourelatos, S., et al. (2020). Projected Shifts in the Distribution of Malaria Vectors Due to Climate Change. Clim. Change 163, 2117–2133. doi:10.1007/s10584-020-02926-9
Kogan, F. (2020). “Malaria Burden,” in Remote Sensing for Malaria: Monitoring and Predicting Malaria from Operational Satellites (Cham: Springer International Publishing), 15–41. doi:10.1007/978-3-030-46020-4_2
Laporta, G. Z., Linton, Y.-M., Wilkerson, R. C., Bergo, E. S., Nagaki, S. S., Sant’Ana, D. C., et al. (2015). Malaria Vectors in South America: Current and Future Scenarios. Parasites Vectors 8, 426. doi:10.1186/s13071-015-1038-4
Liu, C., Berry, P. M., Dawson, T. P., and Pearson, R. G. (2005). Selecting Thresholds of Occurrence in the Prediction of Species Distributions. Ecography 28, 385–393. doi:10.1111/j.0906-7590.2005.03957.x
Liu, C., White, M., and Newell, G. (2011). Measuring and Comparing the Accuracy of Species Distribution Models with Presence-Absence Data. Ecography 34, 232–243. doi:10.1111/j.1600-0587.2010.06354.x
Lobo, J. M., Jiménez-Valverde, A., and Hortal, J. (2010). The Uncertain Nature of Absences and Their Importance in Species Distribution Modelling. Ecography 33, 103–114. doi:10.1111/j.1600-0587.2009.06039.x
Massey, N. C., Garrod, G., Wiebe, A., Henry, A. J., Huang, Z., Moyes, C. L., et al. (2016). A Global Bionomic Database for the Dominant Vectors of Human Malaria. Sci. Data 3, 1–13. doi:10.1038/sdata.2016.14
Meek, S. R. (1995). Vector Control in Some Countries of Southeast Asia: Comparing the Vectors and the Strategies. Ann. Trop. Med. Parasitol. 89, 135–147. doi:10.1080/00034983.1995.11812944
Messina, J. P., Brady, O. J., Golding, N., Kraemer, M. U. G., Wint, G. R. W., Ray, S. E., et al. (2019). The Current and Future Global Distribution and Population at Risk of Dengue. Nat. Microbiol. 4, 1508–1515. doi:10.1038/s41564-019-0476-8
Moss, R. H., Edmonds, J. A., Hibbard, K. A., Manning, M. R., Rose, S. K., Van Vuuren, D. P., et al. (2010). The Next Generation of Scenarios for Climate Change Research and Assessment. Nature 463, 747–756. doi:10.1038/nature08823
Moyes, C. L., Shearer, F. M., Huang, Z., Wiebe, A., Gibson, H. S., Nijman, V., et al. (2016). Predicting the Geographical Distributions of the Macaque Hosts and Mosquito Vectors of Plasmodium Knowlesi Malaria in Forested and Non-forested Areas. Parasites Vectors 9, 242. doi:10.1186/s13071-016-1527-0
Murdock, C. C., Sternberg, E. D., and Thomas, M. B. (2016). Malaria Transmission Potential Could be Reduced with Current and Future Climate Change. Sci. Rep. 6, 27771. doi:10.1038/srep27771
Navarro-Racines, C., Tarapues, J., Thornton, P., Jarvis, A., and Ramirez-Villegas, J. (2020). High-resolution and Bias-Corrected CMIP5 Projections for Climate Change Impact Assessments. Sci. Data 7, 1–14. doi:10.1038/s41597-019-0343-8
Obsomer, V., Defourny, P., and Coosemans, M. (2007). The Anopheles Dirus Complex: Spatial Distribution and Environmental Drivers. Malar. J. 6, 26. doi:10.1186/1475-2875-6-26
Obsomer, V., Defourny, P., and Coosemans, M. (2012). Predicted Distribution of Major Malaria Vectors Belonging to the Anopheles Dirus Complex in Asia: Ecological Niche and Environmental Influences. PLoS ONE 7, e50475. doi:10.1371/journal.pone.0050475
Obsomer, V., Dufrene, M., Defourny, P., and Coosemans, M. (2013). Anopheles Species Associations in Southeast Asia: Indicator Species and Environmental Influences. Parasites Vectors 6, 136. doi:10.1186/1756-3305-6-136
Paaijmans, K. P., Wandago, M. O., Githeko, A. K., and Takken, W. (2007). Unexpected High Losses of Anopheles gambiae Larvae Due to Rainfall. PLoS One 2, e1146. doi:10.1371/journal.pone.0001146
Palanisami, K., Kakumanu, K. R., Nagothu, U. S., and Ranganathan, C. R. (2019). Climate Change and Agriculture in India. India Studies in Business and Economics, 1–6. doi:10.1007/978-981-13-8363-2_1
Phillips, S. J., Anderson, R. P., and Schapire, R. E. (2006). Maximum Entropy Modeling of Species Geographic Distributions. Ecol. Model. 190, 231–259. doi:10.1016/j.ecolmodel.2005.03.026
Phillips, S. J., Dudík, M., Elith, J., Graham, C. H., Lehmann, A., Leathwick, J., et al. (2009). Sample Selection Bias and Presence-Only Distribution Models: Implications for Background and Pseudo-absence Data. Ecol. Appl. 19, 181–197. doi:10.1890/07-2153.1
Preston, B. (2006). Climate Change in the Asia/Pacific Region. Australia: Commonwealth Scientific and Industrial Research Organisation (‘CSIRO’). doi:10.13140/RG.2.1.3530.4084
Qi, Q., Guerra, C. A., Moyes, C. L., Elyazar, I. A. F., Gething, P. W., Hay, S. I., et al. (2012). The Effects of Urbanization on Global Plasmodium Vivax Malaria Transmission. Malar. J. 11, 403. doi:10.1186/1475-2875-11-403
Ren, Z., Wang, D., Ma, A., Hwang, J., Bennett, A., Sturrock, H. J. W., et al. (2016). Predicting Malaria Vector Distribution under Climate Change Scenarios in China: Challenges for Malaria Elimination. Sci. Rep. 6, 1–13. doi:10.1038/srep20604
Ren, Z., Zhu, J., Gao, Y., Yin, Q., Hu, M., Dai, L., et al. (2018). Maternal Exposure to Ambient PM10 during Pregnancy Increases the Risk of Congenital Heart Defects: Evidence from Machine Learning Models. Sci. Total Environ. 630, 1–10. doi:10.1016/j.scitotenv.2018.02.181
Rosenberg, R., Andre, R. G., and Somchit, L. (1990). Highly Efficient Dry Season Transmission of Malaria in Thailand. Trans. R. Soc. Trop. Med. Hyg. 84, 22–28. doi:10.1016/0035-9203(90)90367-N
Ryan, S. J., Lippi, C. A., and Zermoglio, F. (2020). Shifting Transmission Risk for Malaria in Africa with Climate Change: A Framework for Planning and Intervention. Malar. J. 19, 170. doi:10.1186/s12936-020-03224-6
Saito, T., and Rehmsmeier, M. (2015). The Precision-Recall Plot is More Informative than the ROC Plot when Evaluating Binary Classifiers on Imbalanced Datasets. PLoS ONE 10, e0118432–21. doi:10.1371/journal.pone.0118432
Senay, S. D., Worner, S. P., and Ikeda, T. (2013). Novel Three-step Pseudo-absence Selection Technique for Improved Species Distribution Modelling. PLoS ONE 8, e71218. doi:10.1371/journal.pone.0071218
Sinka, M. E., Bangs, M. J., Manguin, S., Chareonviriyaphap, T., Patil, A. P., Temperley, W. H., et al. (2011). The Dominant Anopheles Vectors of Human Malaria in the Asia-Pacific Region: Occurrence Data, Distribution Maps and Bionomic Précis. Parasites Vectors 4, 89. doi:10.1186/1756-3305-4-89
Sinka, M. E., Bangs, M. J., Manguin, S., Rubio-Palis, Y., Chareonviriyaphap, T., Coetzee, M., et al. (2012). A Global Map of Dominant Malaria Vectors. Parasites Vectors 5, 69. doi:10.1186/1756-3305-5-69
Sofaer, H. R., Hoeting, J. A., and Jarnevich, C. S. (2019). The Area under the Precision‐recall Curve as a Performance Metric for Rare Binary Events. Methods Ecol. Evol. 10, 565–577. doi:10.1111/2041-210X.13140
Song, C., Wang, Y., Yang, X., Yang, Y., Tang, Z., Wang, X., et al. (2020). Spatial and Temporal Impacts of Socioeconomic and Environmental Factors on Healthcare Resources: A County-Level Bayesian Local Spatiotemporal Regression Modeling Study of Hospital Beds in Southwest China. Int. J. Environ. Res. Public Health 17, 5890. doi:10.3390/ijerph17165890
Sriwichai, P., Longley, R., and Sattabongkot, J. (2016). “Ecology of Malaria Vectors and Current (Nongenetic) Methods of Control in the Asia Region,” in Genetic Control of Malaria and Dengue. Editor Z. N. Adelman (Boston: Academic Press), 69–80. doi:10.1016/B978-0-12-800246-9.00004-1
Sugawara, E., and Nikaido, H. (2014). Properties of AdeABC and AdeIJK Efflux Systems of Acinetobacter Baumannii Compared with Those of the AcrAB-TolC System of Escherichia coli. Antimicrob. Agents Chemother. 58, 7250–7257. doi:10.1128/AAC.03728-14
Svetnik, V., Liaw, A., Tong, C., Culberson, J. C., Sheridan, R. P., and Feuston, B. P. (2003). Random Forest: A Classification and Regression Tool for Compound Classification and QSAR Modeling. J. Chem. Inf. Comput. Sci. 43, 1947–1958. doi:10.1021/ci034160g
Tonnang, H. E., Kangalawe, R. Y., and Yanda, P. Z. (2010). Predicting and Mapping Malaria under Climate Change Scenarios: The Potential Redistribution of Malaria Vectors in Africa. Malar. J. 9, 111. doi:10.1186/1475-2875-9-111
Tuanmu, M.-N., Viña, A., Winkler, J. A., Li, Y., Xu, W., Ouyang, Z., et al. (2013). Climate-change Impacts on Understorey Bamboo Species and Giant Pandas in China's Qinling Mountains. Nat. Clim. Change 3, 249–253. doi:10.1038/nclimate1727
Urbani, F., D’Alessandro, P., and Biondi, M. (2017). Using Maximum Entropy Modeling (MaxEnt) to Predict Future Trends in the Distribution of High Altitude Endemic Insects in Response to Climate Change. Bull. Insectol. 70, 189–200.
Valderrama, L., Ayala, S., Reyes, C., and González, C. R. (2021). Modeling the Potential Distribution of the Malaria Vector Anopheles (Ano.) Pseudopunctipennis Theobald (Diptera: Culicidae) in Arid Regions of Northern Chile. Front. Public Health 9, 350. doi:10.3389/fpubh.2021.611152
Vale, C. G., Tarroso, P., and Brito, J. C. (2014). Predicting Species Distribution at Range Margins: Testing the Effects of Study Area Extent, Resolution and Threshold Selection in the Sahara-Sahel Transition Zone. Divers. Distrib. 20, 20–33. doi:10.1111/ddi.12115
van Vuuren, D. P., Edmonds, J., Kainuma, M., Riahi, K., Thomson, A., Hibbard, K., et al. (2011). The Representative Concentration Pathways: An Overview. Clim. Change 109, 5–31. doi:10.1007/s10584-011-0148-z
VanDerWal, J., Shoo, L. P., Graham, C., and Williams, S. E. (2009). Selecting Pseudo-absence Data for Presence-Only Distribution Modeling: How Far Should You Stray from what You Know? Ecol. Model. 220, 589–594. doi:10.1016/j.ecolmodel.2008.11.010
West, A. M., Kumar, S., Brown, C. S., Stohlgren, T. J., and Bromberg, J. (2016). Field Validation of an Invasive Species Maxent Model. Ecol. Inf. 36, 126–134. doi:10.1016/j.ecoinf.2016.11.001
WHO (2019). World Malaria Report 2019. Available at: https://www.who.int/news-room/fact-sheets/detail/malaria (December 4, 2020).
Wisz, M. S., and Guisan, A. (2009). Do pseudo-absence Selection Strategies Influence Species Distribution Models and Their Predictions? An Information-Theoretic Approach Based on Simulated Data. BMC Ecol. 9, 8. doi:10.1186/1472-6785-9-8
Yang, L., Wang, Y., Wang, R., Klemeš, J. J., Almeida, C. M. V. B. d., Jin, M., et al. (2020). Environmental-social-economic Footprints of Consumption and Trade in the Asia-Pacific Region. Nat. Commun. 11, 4490. doi:10.1038/s41467-020-18338-3
Zaniewski, A. E., Lehmann, A., and Overton, J. M. (2002). Predicting Species Spatial Distributions Using Presence-Only Data: A Case Study of Native New Zealand Ferns. Ecol. Model. 157, 261–280. doi:10.1016/S0304-3800(02)00199-0
Zeng, Z., Estes, L., Ziegler, A. D., Chen, A., Searchinger, T., Hua, F., et al. (2018). Highland Cropland Expansion and Forest Loss in Southeast Asia in the Twenty-First Century. Nat. Geosci. 11, 556–562. doi:10.1038/s41561-018-0166-9
Keywords: climate change, land-use change, geographical distribution, random forest, South-East Asia, Western Pacific, An. dirus
Citation: Liu X, Song C, Ren Z and Wang S (2022) Predicting the Geographical Distribution of Malaria-Associated Anopheles dirus in the South-East Asia and Western Pacific Regions Under Climate Change Scenarios. Front. Environ. Sci. 10:841966. doi: 10.3389/fenvs.2022.841966
Received: 23 December 2021; Accepted: 09 May 2022;
Published: 16 June 2022.
Edited by:
Argaw Ambelu, Jimma University, EthiopiaReviewed by:
Maquins Odhiambo Sewe, Umeå University, SwedenNirmal Kumar Ganguly, Indraprastha Apollo Hospitals, India
Copyright © 2022 Liu, Song, Ren and Wang. This is an open-access article distributed under the terms of the Creative Commons Attribution License (CC BY). The use, distribution or reproduction in other forums is permitted, provided the original author(s) and the copyright owner(s) are credited and that the original publication in this journal is cited, in accordance with accepted academic practice. No use, distribution or reproduction is permitted which does not comply with these terms.
*Correspondence: Zhoupeng Ren, cmVuenBAbHJlaXMuYWMuY24=
†These authors have contributed equally to this work