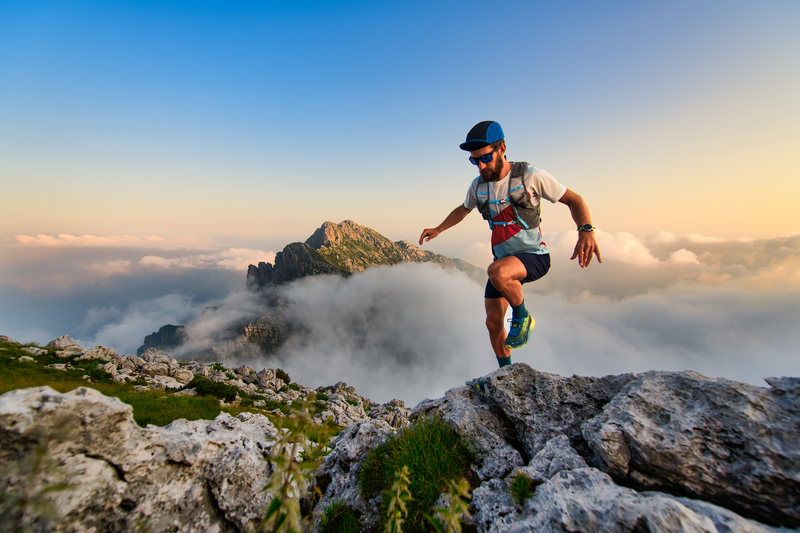
95% of researchers rate our articles as excellent or good
Learn more about the work of our research integrity team to safeguard the quality of each article we publish.
Find out more
ORIGINAL RESEARCH article
Front. Energy Res. , 19 March 2025
Sec. Sustainable Energy Systems
Volume 13 - 2025 | https://doi.org/10.3389/fenrg.2025.1563828
This article is part of the Research Topic Advanced Technologies for High-quality Development of Distribution Systems with Distributed Energy Resources View all 4 articles
To enhance methane utilization in coal-rich regions and integrate methane power generation with distributed renewable energy systems, this study proposes a coordinated optimization dispatch model with adjustable methane-fired generation. The methodology first establishes a methane transmission model incorporating virtual storage characteristics based on coal mine power supply topology and extraction processes, then develops a multi-resource optimization framework integrating wind, solar, hydropower, and methane under distribution network constraints, equipment operation limits, and coal mine safety requirements. Through case studies in a Shanxi coal mine under multiple scenarios, the results validate the model's effectiveness in improving methane utilization and coordinating hybrid energy resources, with the proposed fuzzy-enhanced IGDT (F-EIGDT) method demonstrating enhanced robustness against source-load uncertainties compared to conventional approaches. The study confirms methane's dual role as fuel and virtual storage medium in mining-area power systems, providing a safety-constrained coordination paradigm for fossil-renewable integration, while suggesting the need for further optimization of long-term storage strategies.
Methane is a flammable gas, typically referring to coal mine methane or natural gas. In this paper, the methane discussed is coal mine methane, primarily composed of methane (CH4), which is a high-energy, clean fuel. Its production is generally associated with coal mining (Bai et al., 2024). The total amount of coal mine methane resources in China can reach 30.05 × 1012 m3, with 41% of this being recoverable, making it an important source of natural gas in the country (Chen et al., 2024a). With the development of clean energy technologies, methane, as an essential low-carbon energy source, has gradually gained more attention for its development and utilization (Kang et al., 2024). The utilization methods of methane vary depending on its concentration, with CH4 volume fractions greater than 6% being suitable for power generation (Akbari et al., 2024). Methane power generation typically uses gas turbines, internal combustion engines, or fuel cells. Gas turbines are suitable for medium to high-concentration methane and offer rapid startup and response capabilities. Internal combustion engines are highly adaptable and can adjust output based on methane supply, making them suitable for lower-concentration methane. Fuel cells, on the other hand, generate electricity through electrochemical reactions using the hydrogen in methane, making them ideal for small-scale or distributed energy systems (Wang et al., 2024). As a crucial component of China’s energy structure adjustment, methane power generation plays an essential role in improving the utilization of coal-associated resources, reducing greenhouse gas emissions, and promoting clean energy development. However, in the context of the rapid development of new energy sources, fully leveraging the regulatory role of methane power generation to accommodate fluctuations in wind and solar energy, while coordinating various renewable energy sources to achieve power balance in the grid and enhance grid stability, has become a hot topic in the energy sector.
With the increasing share of renewable energy, methane power generation, with its flexible regulatory capabilities, has become an important means of balancing grid load and ensuring the stability of electricity supply, especially in integrated energy systems and microgrids. Nadaraju et al. (2019) utilizes ventilation air methane emission reduction heat recovery to provide flexible regulation and self-sustaining operation, supporting grid stability and energy system integration. The vam oxidation device was used to convert low concentration coal mine methane into thermal energy for use in coal mines (Hu et al., 2022), which to some extent improved the utilization rate of methane. Similarly, a regenerative thermal oxidizer device is used to convert low concentration coal mine methane into thermal energy through catalytic oxidation for recovery and utilization (Wang et al., 2022). Huang et al. (2021) integrates various renewable energy sources such as coal mine methane, and utilizes abandoned mines in mining areas to transform into pumped storage power stations to participate in system coordination and operation, improving the utilization level of coal mine methane. These studies have made significant theoretical contributions to methane power generation and multi-energy collaborative operation, improving the efficiency of coal mine methane participation in the power system’s operation. However, most of the studies have rough methane power generation modeling processes, insufficient consideration of safety constraints, and little research on optimizing scheduling in the context of coal mine production and the actual operation of coal mine distribution networks.
The power output of methane power generation is mainly determined by the methane gas source and the characteristics of the equipment. During coal mine production, factors such as methane content, geological conditions, and extraction techniques can all impact the methane gas source, introducing a certain level of uncertainty into methane power generation. How to address this uncertainty is an important issue in system optimization scheduling. Existing studies often use methods such as scenario generation, robust optimization, stochastic optimization, and interval optimization to handle uncertainty. Zhang et al. (2024) proposed a novel scenario generation method based on filtering scenarios with environmental wind conditions, enhancing scenario deployment while using minimal resources to meet the required risk levels. This method provides an accurate and effective solution for opportunity-constrained economic scheduling problems. Lin et al. (2023) transformed a fuzzy set-based distributed robust optimization model into a scenario-oriented model with probabilistic uncertainty, effectively avoiding complex mathematical transformations. Cao et al. (2024) considered source-load uncertainty and established an uncertain islanding model using correlated random variables, generating islanding scenarios with scenario trees to enhance energy supply reliability under islanding conditions. Kou and Li (2020) used interval optimization to decompose the potential transmission capacity uncertainty of wind power into lower and upper boundary models, transforming the problem into a single-level maximization problem, thus achieving efficient solutions. While the above studies improve the system’s adaptability to uncertainty, these methods are computationally complex, and the models often rely on historical information or probability functions of uncertainty variables. In practice, however, methane power plants have limited access to detailed information about the methane gas source, as they typically only record partial information about the gas injected into the power generation units (i.e., the modulated methane gas information), making the information on the methane power source constrained.
Information Gap Decision Theory (IGDT) is a method for handling uncertainty and information insufficiency in decision-making processes. It aims to make robust decisions by assessing and addressing information gaps, especially in situations where decisions must be made under incomplete information or in response to uncertainty challenges (Ben-Haim, 2006). IGDT has been widely applied in the field of power systems. Zhang et al. (2023) combined IGDT with opportunity constraints to handle forecast deviations in load demand and distributed generation, as well as associated power fluctuations in distribution networks, to obtain proactive management strategies for operators. Yazdaninejad et al. (2020) specifically modeled uncertainty sources and used multi-level IGDT to establish a framework for non-deterministic and non-probabilistic uncertainty, where each uncertainty parameter is targeted at a specific range around its expected value. Chen et al. (2024b) applied the IGDT method to provide optimal bidding strategies for load aggregators, considering time-shifted loads and distributed renewable energy under uncertainty. Khaloie et al. (2022) used IGDT to address the uncertainty of photovoltaic output by combining conditional risk values to handle both stochastic uncertainty and the information gap in IGDT. However, there has been little research applying IGDT to quantify the uncertainty in methane power generation, and the methods in the aforementioned studies lack probabilistic information, which limits the decision-making process. Most of these studies focus on maximizing the region or range of uncertainty variables under the condition of meeting pre-set objectives, rather than incorporating a more comprehensive uncertainty analysis.
Therefore, to address the issues of rough methane power generation modeling, insufficient exploration of its regulatory potential, and the uncertainty arising from limited source-side information, this paper proposes a coordinated optimization scheduling method for wind, solar, and hydropower systems with adjustable methane power generation. The main contributions of this paper are as follows:
1) Developing a detailed model for methane power generation and virtual storage based on coal mine production realities. In this paper, a detailed modeling approach is applied to methane extraction and power generation within the actual coal mine energy supply network. Key factors influencing methane extraction are extracted based on current coal mining production standards. Considering the energy storage characteristics of methane gas, the model integrates methane storage and utilization closely, establishing a virtual storage model for methane that focuses on dynamic regulation. Through detailed modeling, the flexibility and rapid response capability of the methane power generation units are quantitatively assessed, providing a theoretical foundation for fully exploiting the regulatory potential of methane power generation.
2) Promoting coordinated optimization scheduling of wind, solar, hydropower, and methane generation using the dynamic regulation characteristics of methane storage tanks. By introducing coal mine safety constraints and the operating mechanism of methane storage tanks, while accounting for network flow and equipment operating constraints, an optimization scheduling model for wind, solar, hydropower, and adjustable methane power generation is developed, with a focus on economic and environmental objectives. The dynamic regulation of methane injection and extraction processes enables the time-shifting and flexible utilization of methane resources. This effectively addresses the randomness and intermittency of wind and solar generation, enhances the overall regulation capability of the system, and achieves the efficient integration of methane power generation with wind, solar, and hydropower.
3) Enhancing the uncertainty handling capability of IGDT with fuzzy logic. This paper considers the uncertainty in wind, solar, and hydropower output, load forecasting, and the time-varying characteristics of coal mine methane supply. Using IGDT, these uncertainties are integrated into the optimization decision-making process. On this basis, fuzzy logic is introduced to handle both uncertainty and fuzziness, leading to the development of a F-EIGDT. By combining fuzzy logic and fuzzy set theory, this method addresses the lack of probabilistic information in IGDT, ensuring that the decision-making process remains robust and feasible when facing fluctuations in wind and solar output and methane supply.
The rest of the paper is organized as follows: Section 2 introduces the coal mine-grid system topology with adjustable methane power generation. Section 3 discusses the coordinated optimization scheduling model for wind, solar, hydropower, and methane power generation. Section 4 addresses the coordinated optimization scheduling model for wind, solar, hydro, and methane under source-load fluctuations based on F-EIGDT. Section 5 presents case studies. Finally, Section 6 provides the conclusions.
The system topology with adjustable methane power generation is shown in Figure 1, consisting of four components: the coal mine distribution network, methane extraction system, methane power generation system, and urban distribution network. The methane extraction and power generation system serves as an intermediate link, where methane extracted from the coal mine is used for power generation, supplying the urban distribution network. This system also forms the physical connection between the coal mine distribution network and the urban distribution network. The characteristics of each system component are as follows:
Coal Mine Distribution Network: Based on the actual power supply system of a coal mine in Shanxi, this paper simplifies the topology to a 34-node coal mine distribution system. Detailed load information for each node can be found in (Liang et al., 2024b), with node 9 representing the methane extraction station, where methane is extracted from the coal mine.
Methane Extraction System: This system consists of two main parts: methane extraction and methane storage. Methane extraction involves drawing methane from underground coal seams, adjacent layers, and mined-out areas through a pipeline system, which includes control valves, water-ring vacuum pumps, and auxiliary equipment (Zhou et al., 2024). Methane storage involves temporarily storing the extracted methane in surface methane storage tanks.
Methane Power Generation System: The methane power generation system consists of methane storage tanks, a mixer, and a methane generator set. The mixer adjusts the air intake based on the methane concentration to ensure the optimal combustion mixture of methane and air. The intake valve controls the flow of combustible methane-air mixture into the generator. The mixture burns in the generator, driving pistons in a reciprocating motion, converting the chemical energy of the gas into electrical and thermal energy output.
Urban Distribution Network: The urban distribution network consists of 33 nodes and 32 branches. Node 5 is connected to a photovoltaic generation unit, node 21 to a wind turbine, node 14 to a hydropower unit, and node 4 to the methane power generation unit.
Through the above-mentioned coal mine methane power generation and coal mine-grid coordinated operation, the system ensures the safety of both coal mine and grid operations while improving methane resource utilization efficiency. Methane storage tanks are configured based on coal mine production conditions and the demand of the distribution network, allowing for flexible adjustment of methane power generation. This helps to mitigate the fluctuations of wind and solar generation while achieving efficient power distribution.
Assuming that there are no tunneling tasks scheduled for the day and only mining tasks are carried out, based on the current coal mine industry standards in China (China National Coal Mine Construction Association, 2018), the key characteristics of coal mine production are abstracted. The critical factors influencing methane yield in coal mines, such as coal production and geological conditions, are identified. A methane extraction model considering coal mine production characteristics is then constructed, as shown in Equations 1–4.
Where Gmine, Gadja, Ggoaf represent the methane extraction rates from the mined coal seam, adjacent seam, and gob area, respectively. Ggoaf1 is the total methane that can be extracted from the coal mine. K1, Kc are the methane extraction imbalance coefficient and the ratio of pre-extraction methane to extraction during the mining process for the specific coal seam. L1, L2, L3, L4 represent the width of the mining face, length of the mining face, width of the mining face, and the annual advancing length of the mining face, respectively. M is the average thickness of the pre-extracted coal seam. γ is the apparent density of coal. W0, Wc, W1 are the original methane content, residual methane content, and pre-extraction qualified methane content of the coal seam, respectively. mj, ηj represent the coal thickness and gas emission rate of the j-th adjacent seam. Ky is the methane extraction rate from the adjacent seam due to pressure relief. W0j, Wcj are the original methane content and residual methane content of the j-th adjacent coal seam, respectively. MC is the coal reserve in the mined coal seam. k1 is the negative pressure extraction coefficient, k2 is the expected methane extraction rate for the coal seam, and k3 is the methane emission rate in the mine.
The relationship between the coal reserve in the mined coal seam and the coal mining amount, as shown in Equation 5.
Where
In addition, since K1, Kc, mj, and ηj are all geological parameters of the coal mine, Equations 1–4 can be transformed into a function of coal mining amount and geological conditions, as shown in Equations 6–9.
Therefore, based on the coal mining process, the mathematical model for gas extraction can be simplified as Equations 10, 11.
Where
In Equation 11, it can be seen that the gas extraction amount from the coal mine at time t is related to the coal production and geological conditions at previous time t.
In this article, “virtual storage” specifically refers to simulating the physical storage behavior of methane (CH4) resources through mathematical models and dynamic regulation mechanisms, rather than relying on traditional physical energy storage facilities such as batteries and pumped storage. The core idea is to utilize the dynamic characteristics of methane in pipelines, storage tanks, and production consumption processes to achieve time translation and flexible scheduling of energy.
When establishing the methane virtual storage model, the following assumptions or limitations need to be made:
(1) Methane is considered an ideal gas.
(2) The temperature and pressure distribution inside the methane storage tank are uniform.
(3) The compression process of methane gas is considered isothermal.
This paper focuses on the process of methane virtual storage, including the injection of extracted methane gas into the storage tank via the pressurization pump station, storage within the tank, and the release process. The ‘gas loading status’ of the storage tank is defined to represent the current remaining capacity of methane in the storage tank. The methane virtual storage model is shown in Equations 12–18.
Where
Methane can balance fluctuations in other resources (Hu et al., 2022; Huang et al., 2021). Methane and filtered air are mixed in the mixer, and after reaching the preset concentration, they are injected into the methane power generation unit through the intercooler and intake valve. The combustion generates electrical energy and waste heat. The waste heat is recovered by a waste heat recovery device, while the electrical energy is transmitted through the city power grid. The model is shown in Equations 19–25.
Where
The output power of the photovoltaic system is mainly determined by the solar radiation, the area of the photovoltaic array, the system efficiency, and the temperature correction factor. Its simplified model is shown in Equations 26–28.
Where
The output power of the wind power generation system has a nonlinear relationship with wind speed, which specifically depends on whether the wind speed is between the cut-in wind speed, rated wind speed, and cut-out wind speed. The model is shown in Equation 29.
Where
The output power of hydropower generation is typically determined by the head height, flow rate, and equipment efficiency (Qiu et al., 2020). The calculation formula is shown in Equations 30, 31.
Where
The coordinated optimization scheduling model for wind, solar, hydro, and methane aims to minimize the total daily operating cost Ctot, which includes the operating cost Cope, the investment cost of the methane storage tank Cgas, and the environmental management cost Cpoll. The objective function is shown in Equations 32–35.
Where T is the total number of time periods in the scheduling cycle.
The system constraints mainly include distribution network power flow constraints (Equations 36-41), equipment operation constraints (Equations 42, 43), and coal mine production constraints (Equations 44, 45).
Where Pt,ij and Qt,ij are the active and reactive power from node i to node j at time t, respectively. rij and xij are the resistance and reactance between node i and node j, respectively. It,ij is the current from node i to node j at time t; Pt,j and Qt,j are the active and reactive power injected at node j at time t, respectively. Ωbc is the set of boundary nodes; Ut,i and Ut,j are the voltage magnitudes at nodes i and j at time t, respectively; Umin and Umax are the lower and upper limits of node voltage magnitudes, respectively.
Where
To ensure the safe operation of the coal mine, under the three-shift system, one shift must be arranged for the maintenance of important equipment. The constraint is as follows:
Where κt is the operating status of the mining machine at time t, where 0 indicates maintenance and 1 indicates operation; t1 and t2 are the start and end times of the maintenance period, respectively.
Decision theory aims to provide decision-makers with methods to make optimal choices in uncertain environments. Traditional decision-making methods, such as Bayesian decision theory, rely on probabilistic information. However, in practical applications, decision-makers often face situations with missing or incomplete information, making it difficult to accurately estimate probability distributions. IGDT addresses this by quantifying the decision-maker’s cognitive gap regarding uncertainty, offering a method for assessing the robustness of decision alternatives under extreme uncertainty. However, the conservatism of IGDT may limit its effectiveness in practical applications. Therefore, this paper proposes the introduction of fuzzy logic and fuzzy set theory into IGDT, forming F-EIGDT, to enhance its performance in dealing with fuzzy uncertainty.
IGDT was proposed by Yakov Ben-Haim in the late 1990s, aiming to provide robust decision alternatives in highly uncertain environments. IGDT evaluates the performance of different decision alternatives under varying levels of information gaps (ϕ), which measure the degree of deviation of decision parameters from reference values (Ben-Haim, 2006). The core of IGDT is to find solutions that can still meet the decision objectives within the maximum information gap range, incorporating both risk-averse and risk-seeking strategies. This paper involves the safe scheduling of coal mines, thus constructing an IGDT robust model to maximize the avoidance of uncertainty’s impact on the solution results. The general form of the IGDT robust model (Peng et al., 2022; Lv et al., 2023) is shown in Equation 46.
Where φ represents uncertainty, indicating the fluctuation range of uncertain variables. F is the objective function. s is the decision variable; w is the actual value of the uncertain variable.
F-EIGDT enhances flexibility and accuracy in handling fuzzy uncertainty by combining fuzzy logic with IGDT. In F-EIGDT, fuzzy set theory defines a membership function that relates elements to their degree of membership in a fuzzy set (Nikoobakht et al., 2020), allowing the expression of partial membership, which can be represented as Equation 47.
Where H is the domain of the fuzzy variable.
Common membership functions include linear, Gaussian membership functions, etc. Taking the linear function as an example, the membership function μ(β) of the uncertainty β in the IGDT model and the membership function μ(F) of the scheduling cost F are represented as Equations 48, 49.
Where βmin and βmax are the minimum and maximum values of uncertainty β. Fmin and Fmax are the minimum and maximum values of the scheduling cost F.
The satisfaction of the robust strategy can be expressed in Equation 50.
Where λ is the satisfaction of the robust strategy.
Fuzzy set theory, by introducing membership functions and fuzzy operations, provides a flexible and powerful tool for dealing with and analyzing complex systems with fuzziness and uncertainty. When combined with IGDT, fuzzy set theory can further enhance the expressiveness of the decision model and the accuracy of the robustness evaluation, enabling decision-makers to make more scientific and reasonable decisions in highly uncertain and fuzzy information environments.
The uncertainty fluctuation range of source-load demand under the F-EIGDT model can be expressed in Equations 51–53.
Where αi is the uncertainty of the ith power generation unit on the source side.
The F-EIGDT model is constructed by combining IGDT with fuzzy set theory. Equation 54 is the IGDT robust model, and Equations 55–57 represent the fuzzy set theory model.
Where X is the decision variable. P is the uncertainty variable. C0 is the optimal solution of the deterministic model. CC is the maximum expected value acceptable to the decision-maker. βR is the deviation factor. G(X,P) = 0 represents other equality constraints; K(X,P) ≤ 0 represents other inequality constraints. L is the domain of the fuzzy variable.
Based on the above models, the following can be derived, as shown in Equation 58.
Where λR is the satisfaction level of the F-EIGDT robust strategy.
Equation 58 is a two-level optimization model, where the lower level model calculates the maximum operating cost of the system based on the system operation scheduling model when uncertain variables fluctuate; The upper level is to solve the maximum satisfaction value when the operating cost of the system meets the predetermined objective of robust optimization; In the lower level model, when the resource output decreases, the system will purchase energy from external sources to compensate for this shortfall, and the lower the output, the higher the cost of external energy purchase; When the system demand increases, the system energy consumption increases, and the greater the load demand, the higher the system scheduling cost. Therefore, for a given range of uncertainty, the maximum operating cost of the lower level model occurs at the lowest resource output and the highest load demand. Therefore, when the resource output takes the lower boundary and the load demand takes the upper boundary value, the lower level scheduling cost is the highest. At this point, the bi-level optimization model in Equation 58 can be equivalently transformed into a single-level optimization model (Dolatabadi et al., 2019), that is Equation 59.
At this point, for any objective function C (X,P), it is less than the maximum expected value CC set by the decision-maker.
Since there are nonlinear terms in the modeling process, which make it difficult for solvers to solve directly, this paper adopts piecewise linearization (Liang et al., 2024a) and second-order cone relaxation (Lv et al., 2022) based on the characteristics of the nonlinear terms for linearization.
The formulas used for piecewise linearization include Equations 14, 15. The linearization process can be represented as Equations 60–64.
Where ak is the segmentation point of the piecewise function f (x). L (f(x)) is the linearized function of f (x). k is the index of the segmentation point. n is the number of segmentation points. tk and z are 0/1 variables.
The above expression contains n newly added binary variables z0, z1, … , zn-1, where the number of these variables is the same as the number of segmentation intervals in the linearized function L (f(x)).
For the quadratic terms present in the distribution network power flow constraints, we introduce slack variables αt,i and βt,ij, and apply second-order cone relaxation for convexification, which can be expressed as Equations 65, 66.
Therefore, Equations 36–39 can be transformed into Equations 67–70.
The standard second-order cone form is Equation 71.
The relaxation error Et,ij can be expressed as Equation 72.
The solution process for the wind-solar-hydro-methane coordinated optimization scheduling model based on F-EIGDT is shown in Figure 2.
1) Input the device parameters, the output forecast values of wind, solar, hydro, and methane power generation units, and the forecast values of electrical load demand.
2) Minimize the total cost as the objective and solve for the optimal solution C0 of the deterministic model.
3) Set C0 as the baseline value for the F-EIGDT model, and replace the forecast values with the actual values of wind, solar, hydro, and methane generation units, and load demand.
4) Set the cost deviation factor βR, uncertainty αi, αL, and their weight coefficients εi.
5) Introduce fuzzy membership functions μ(ψ), μ(C), and solve for the objective function λR.
6) Dispatch the unit scheduling plan.
In this paper, a case study is conducted on a coal mine in Shanxi and its adjacent distribution system. The computer configuration used in this study is an Intel Core i7-14700F processor with a 64-bit Windows 11 operating system. The proposed model is simulated using MATLAB_R2024b software, with the YALMIP toolbox calling the GUROBI solver (version 11.0.3).
This study focuses on a typical summer day. The wind speed, solar radiation, temperature, and water flow data for the typical day are clustered using the K-means algorithm. The predicted output of the photovoltaic, wind turbine, and hydroelectric power generation for the typical day are shown in Figure 3. The mine needs to conduct maintenance on underground production equipment between 11:00 and 16:00, during which no coal mining occurs. The time-of-use electricity prices are shown in Table 1. The equipment parameters are listed in Table 2, and other parameter information is in Table 3. For the renewable energy forecasts, many methods can achieve good prediction accuracy, so the forecast error range for wind, solar, hydro, and methane generation is set to [-10%, +10%]. The coal mine has a strong production schedule with minimal load fluctuations, so the error range for the overall load forecast of the mine is set to [-3%, +3%]. The forecast deviation for the overall load of the urban distribution network is set to [-5%, +5%].
In the deterministic scenarios, two cases are set to study the impact of the gas regulation characteristics on the system’s optimal scheduling:
Scenario 1: No consideration of the virtual regulation characteristics of methane (no methane storage tank installed).
Scenario 2: Consideration of the virtual regulation characteristics of methane (methane storage tank installed).
The system relaxation error using the second-order cone relaxation method adopted in this study is shown in Figure 4. The maximum magnitude of branch power relaxation error is on the order of 10–6, indicating that second-order cone relaxation is feasible without loss of accuracy.
The system scheduling costs under different scenarios are shown in Table 4.
From Table 4, it can be seen that in Scenario 2, where a methane storage tank is configured, the system operating cost decreases from 75,258.6 RMB in Scenario 1 to 69,651.5 RMB, a reduction of approximately 7.45%. This indicates that the introduction of the methane storage tank significantly improved the system’s operational efficiency and regulation capability. At the same time, the environmental management cost slightly increased from 322.5 RMB to 366.7 RMB. Although the investment cost for the methane storage tank in Scenario 2 reached 6,584.1 RMB (the investment cost here is the equivalent annual cost of the total investment in the methane storage tank), the total cost of Scenario 2 is only 1,021.2 RMB higher than that of Scenario 1. Therefore, equipping the system with a methane storage tank helps to enhance the flexibility of methane storage and supply, while also improving the economic performance of the system to some extent.
Figure 5 shows the variation in methane power generation under different scenarios. The power generation is determined by the methane quality input to the methane power generation units. Specifically, Scenario 1 is significantly affected by the coal mine maintenance between 11:00 and 16:00, leading to a shortage of methane supply and resulting in notably lower power generation. In contrast, Scenario 2 introduces the methane storage tank, which enables the reserve and scheduling of methane resources, thereby providing supplementation when methane supply is insufficient. In this way, Scenario 2 effectively mitigates the limitations of power generation caused by methane supply fluctuations, significantly improving the flexibility and responsiveness of the power generation system.
Scenario 2 is selected to analyze the virtual energy storage state of the methane storage tank. The variation in the methane gas state in the storage tank is shown in Figure 6.
From Figure 6, it can be seen that the State of Charge (SOC) of the methane storage tank changes with methane injection and release. Between 01:00 and 07:00, and 17:00 and 19:00, the amount of methane injected into the storage tank is less than the amount released, causing the SOC to decrease. From 07:00 to 12:00 and 14:00 to 17:00, the SOC changes more steadily. At 12:00 and 21:00, the amount of methane injected into the storage tank is significantly greater than the release amount, resulting in a noticeable increase in SOC at those times. Additionally, between 19:00 and 22:00, the injection efficiency of methane increases, and the SOC of the storage tank gradually rises. Through dynamic regulation of methane injection and release, the methane storage tank promotes the efficient utilization of methane.
As shown in Figure 7, the system’s electric power mainly consists of wind, solar, hydro, and purchased power from the grid. Methane power generation acts as a supplementary unit to traditional wind, solar, and hydro generation, compensating for the shortfalls in renewable generation. During the 22:00–05:00 period, when the output of wind, solar, and hydro generation is low, there is a power shortage. At the same time, due to the impact of peak and valley electricity prices, most of the power deficit is made up by purchasing electricity from the grid. By adjusting methane power generation, the system can effectively address the randomness and intermittency of wind and solar power, enhancing the overall regulation capability of the system and promoting the efficient coupling and coordinated operation of methane generation with wind, solar, and hydro power.
In summary, although the methane storage tank slightly increases the total operational cost of the system, it can dynamically adjust methane resources, enabling efficient storage and scheduling of methane, effectively mitigating the impact of methane supply fluctuations on power generation capability, and promoting the efficient utilization of methane. At the same time, methane power generation serves as an effective supplement to traditional wind, solar, and hydro generation, working in synergy to meet the operational demands of the system.
Scene 2 is selected for the study of wind, solar, hydro, and methane uncertainty. To explore the impact of uncertainty in wind, solar, hydro, and methane on system scheduling, the following five combinations are set:
Combination 1: Only consider the uncertainty of wind turbines.
Combination 2: Only consider the uncertainty of photovoltaics.
Combination 3: Only consider the uncertainty of hydropower.
Combination 4: Only consider the uncertainty of methane.
Combination 5: Consider the combined uncertainty of wind, solar, hydro, and methane.
Under the IGDT strategy, the variations of each uncertainty and scheduling cost with the cost deviation factor under different combinations are shown in Figure 8. The uncertainties of wind, solar, hydro, methane, and the combined uncertainty correspond to combinations 1–5, respectively.
Figure 8 illustrates the total scheduling cost of the system and the uncertainty variations of each distributed energy source under different cost deviation factors based on the IGDT strategy. As the cost deviation factor increases, the total scheduling cost rises non-linearly, and the uncertainty of each distributed energy source gradually increases, eventually reaching the maximum robustness level (uncertainty of 0.1). Methane and hydropower have relatively low uncertainties due to their stable power generation capability and flexible regulation. In contrast, photovoltaic and wind turbines are significantly affected by weather and climate, resulting in higher cost uncertainties and greater sensitivity to the deviation factor. Additionally, once the system reaches maximum robustness, further increasing the scheduling cost offers limited improvement in robustness and significantly reduces the economic performance. Therefore, the optimal scheduling strategy should choose a moderate deviation factor that enables the system to achieve a reasonable level of robustness at a lower cost.
Furthermore, for each target cost deviation set by the decision-maker, each distributed energy source has its corresponding maximum uncertainty fluctuation range. This relationship provides key reference for the expansion planning and coordinated scheduling of multi-energy systems, helping to formulate more robust power scheduling schemes in highly heterogeneous and stochastic environments. It enables efficient energy management that balances both system robustness and economic performance, further promoting the efficient integration and optimized utilization of wind, solar, hydro, and methane resources.
Under the F-EIGDT strategy, the uncertainty and scheduling cost variations with cost deviation factors under different combinations are shown in Figure 9.
Figure 9 illustrates the trend of uncertainty and total system scheduling cost of different distributed energy sources under the F-EIGDT strategy as the cost deviation factor changes. The trend is similar to that in Figure 8, except that under the F-EIGDT strategy, the increase in cost is lower than that under the traditional IGDT. This indicates that the introduction of fuzzy logic enhances the system’s ability to handle uncertainty. In particular, when the cost deviation factor is 0.092, the system reaches its strongest robustness level, and the total scheduling cost is reduced by 4.3% compared to IGDT. When the actual output of wind, solar, and hydro power fluctuates within 10% of the forecasted values, the system can effectively mitigate the fluctuations in the output of wind, solar, and hydro, while ensuring that the total scheduling cost does not exceed 83,649.71 yuan, significantly improving operational economics. This shows that F-EIGDT, through fuzzy logic and fuzzy set theory, addresses various uncertainties, overcoming the limitations of traditional IGDT in the absence of probabilistic information. It enables the system to achieve higher robustness and economic performance in complex scenarios such as wind and solar output deviations and methane supply fluctuations.
The methane power generation and methane storage tank SOC (State of Charge) under the IGDT and F-EIGDT strategies are shown in Figure 10.
As shown in Figure 10, under the IGDT strategy, the methane power generation fluctuates significantly, with frequent adjustments causing notable peaks and valleys. For example, power changes are sharp at 03:00, 12:00, and 23:00, and the methane storage tank SOC (State of Charge) also experiences rapid fluctuations, indicating poor operational stability. This is because IGDT lacks the precise handling of uncertainty information and relies solely on worst-case scenarios for scheduling, resulting in frequent changes in gas power generation and storage tank charging/discharging operations. In contrast, the F-EIGDT strategy introduces fuzzy logic and fuzzy set theory, effectively smoothing the adjustment process of gas power generation, reducing the amplitude of SOC fluctuations, and keeping it within a relatively stable range. This strategy significantly enhances the system’s robustness and economic performance when facing fluctuations in wind and solar output, load forecasting errors, and methane supply uncertainties. It improves the stability of gas storage tank operations and reduces the economic cost caused by frequent adjustments, providing a better solution for the stable operation of multi-energy systems.
In conclusion, F-EIGDT can effectively smooth the fluctuations of gas power generation, reducing the amplitude of power fluctuations and enhancing the stability of system scheduling. By optimizing the trend of gas storage tank SOC changes, it reduces the dramatic fluctuations in gas injection and release, ensuring the stability and reliability of gas storage tank operations. Furthermore, F-EIGDT comprehensively considers various uncertainties, such as wind and solar output deviations, load forecasting errors, and methane supply fluctuations, enabling the scheduling scheme to achieve high robustness while maintaining superior economic performance.
To verify the superiority of the proposed F-EIGDT in handling the uncertainty of wind, solar, hydro, and gas power, this section compares the F-EIGDT, IGDT, and stochastic optimization methods. The cost deviation factor of 0.092 from F-EIGDT is selected as the fluctuation range for the companion resources in the stochastic optimization method. Monte Carlo scenario generation and K-means clustering methods are used to generate five typical scenarios, and the maximum prediction deviation in different scenarios is represented using the definition of integrated uncertainty in the IGDT model. The comparison results of the three strategies are shown in Table 5.
From Table 5, it can be seen that stochastic optimization has a lower computational efficiency. Due to its insufficient consideration of system uncertainty, it results in the highest operating costs and the worst robustness, with a maximum prediction deviation of only 4.6%. IGDT, through worst-case-based optimization, significantly reduces operating costs and improves robustness, with a maximum prediction deviation of 9.2%, and the highest solving efficiency. In comparison, F-EIGDT, after introducing fuzzy logic to handle uncertainty, not only keeps operating costs consistent with IGDT but also increases the maximum uncertainty to 10.0%, demonstrating stronger robustness and risk resistance. Although the solving time is slightly higher, it remains within a reasonable range. Overall, the F-EIGDT strategy achieves a better balance between robustness, economy, and computational efficiency, making it a superior choice for addressing complex uncertainty scenarios in the system.
This paper proposes a wind-solar-hydro-gas coordinated optimal scheduling method with adjustable methane power generation. By analyzing the actual energy supply network of coal mines in depth, we construct a refined methane power generation and virtual methane storage model, and propose an optimized scheduling framework that incorporates the dynamic regulation characteristics of gas resources. In addition, to address the multiple uncertainties of wind-solar power generation and gas supply, this paper introduces the F-EIGDT, which significantly improves the system’s scheduling robustness and economic performance in complex uncertain environments. The analysis and verification are conducted through a case study of a coal mine in Shanxi. The results show that:
1) A refined model for gas power generation and virtual gas storage is developed, closely aligned with the actual coal mine production. This model fully considers the key influencing factors in the gas extraction process and integrates gas storage and utilization, forming a virtual storage mechanism focused on dynamic gas regulation. Through detailed modeling, the flexibility and fast response capability of the gas power generation units are quantitatively evaluated.
2) The proposed wind-solar-hydro-gas coordinated optimization scheduling model makes full use of the dynamic regulation characteristics of the gas storage tank, combining coal mine safety constraints and network operation requirements to achieve time-shifting and flexible utilization of gas resources. The case study results show that this method effectively mitigates the challenges caused by the randomness and intermittency of wind-solar power generation, enhances the system’s comprehensive regulation capability, optimizes energy utilization efficiency, and reduces operational costs to some extent.
3) The F-EIGDT method with fuzzy logic significantly improves the decision-making robustness under multiple uncertainty factors. By applying fuzzy logic, the stability and economy of the decision-making plan are enhanced when facing actual operational fluctuations. Experimental results show that F-EIGDT outperforms traditional stochastic optimization methods in smoothing gas power generation, reducing gas storage tank fluctuations, and optimizing system operation costs.
In summary, the wind-solar-hydro-methane coordinated optimization scheduling method proposed in this paper, combined with virtual gas storage and F-EIGDT, provides an innovative solution for addressing the challenges of complex system uncertainties. Future research will focus on expanding this method’s application to larger-scale multi-gas power generation systems, exploring the integration of more uncertainty handling techniques, and continuously optimizing model parameters with actual operational data to promote the wide application and sustainable development of coordinated optimization scheduling methods in energy systems.
The original contributions presented in the study are included in the article/supplementary material, further inquiries can be directed to the corresponding author.
WC: Writing–original draft, Conceptualization, Investigation, Methodology. XW: Conceptualization, Supervision, Writing–review and editing. RT: Formal Analysis, Methodology, Software, Writing–original draft. YJ: Methodology, Software, Writing–review and editing. HL: Formal Analysis, Writing–review and editing. YY: Investigation, Writing–review and editing.
The author(s) declare that financial support was received for the research and/or publication of this article. This study received funding from the State Grid Shanxi Electric Power Co., Ltd. Science and Technology Project “Key Technology Research on Coordinated Operation and Optimization Scheduling of Wind-Solar-Hydro-Methane Multi-resource Considering Methane Power Generation” (5205E0240002). The funder was not involved in the study design, collection, analysis, interpretation of data, the writing of this article, or the decision to submit it for publication.
Authors WC, XW, RT, YJ, HL, and YY were employed by State Grid Jincheng Power Supply Co., Ltd.
The author(s) declare that no Generative AI was used in the creation of this manuscript.
All claims expressed in this article are solely those of the authors and do not necessarily represent those of their affiliated organizations, or those of the publisher, the editors and the reviewers. Any product that may be evaluated in this article, or claim that may be made by its manufacturer, is not guaranteed or endorsed by the publisher.
Akbari, B., Garrison, J., Raycheva, E., and Sansavini, G. (2024). Flexibility provision in the Swiss integrated power, hydrogen, and methane infrastructure. Energy Convers. Manag. 319, 118911. doi:10.1016/j.enconman.2024.118911
Bai, S., Zhang, Y., Li, F., Yan, Y., Chen, H., Feng, S., et al. (2024). High-resolution satellite estimates of coal mine methane emissions from local to regional scales in Shanxi, China. Sci. Total Environ. 950, 175446. doi:10.1016/j.scitotenv.2024.175446
Ben-Haim, Y. (2006). Info-gap decision theory: decisions under severe uncertainty. 2nd ed. London: Academic Press.
Cao, Y., Mu, Y., Jia, H., Yu, X., Hou, K., and Wang, H. (2024). A multi-objective stochastic optimization approach for planning a multi-energy microgrid considering unscheduled islanded operation. IEEE Trans. Sustain. Energy. 15 (2), 1300–1314. doi:10.1109/TSTE.2023.3341898
Chen, D., Ma, M., Hu, L., Du, Q., Li, B., Yang, Y., et al. (2024a). Characteristics of China's coal mine methane emission sources at national and provincial levels. Environ. Res. 259, 119549. doi:10.1016/j.envres.2024.119549
Chen, L., Xu, J., Sun, Y., Liao, S., Ke, D., Yao, L., et al. (2024b). Bidding strategies of load aggregators for day-ahead market with multiple uncertainties. IEEE Trans. Power Syst. 39 (2), 2786–2800. doi:10.1109/TPWRS.2023.3296273
China National Coal Mine Construction Association (2018). GB/T 50471-2018, Design standard for coal mine gas drainage engineering. Beijing: China Planning Press.
Dolatabadi, A., Jadidbonab, M., and Mohammadi-ivatloo, B. (2019). Short-term scheduling strategy for wind-based energy hub: a hybrid stochastic/IGDT approach. IEEE Trans. Sustain. Energy 10 (1), 438–448. doi:10.1109/TSTE.2017.2788086
Hu, H., Sun, X., Zeng, B., Gong, D., and Zhang, Y. (2022). Enhanced evolutionary multi-objective optimization-based dispatch of coal mine integrated energy system with flexible load. Appl. Energy. 307, 118130. doi:10.1016/j.apenergy.2021.118130
Huang, H., Liang, R., Lv, C., Lu, M., Gong, D., and Yin, S. (2021). Two-stage robust stochastic scheduling for energy recovery in coal mine integrated energy system. Appl. Energy. 290, 116759. doi:10.1016/j.apenergy.2021.116759
Kang, Y., Tian, P., Li, J., Wang, H., and Feng, K. (2024). Methane mitigation potentials and related costs of China's coal mines. Fundam. Res. 4 (6), 1688–1695. doi:10.1016/j.fmre.2023.09.012
Khaloie, H., Vallée, F., Lai, C. S., Toubeau, J.-F., and Hatziargyriou, N. D. (2022). Day-ahead and intraday dispatch of an integrated biomass-concentrated solar system: a multi-objective risk-controlling approach. IEEE Trans. Power Syst. 37 (1), 701–714. doi:10.1109/TPWRS.2021.3096815
Kou, X., and Li, F. (2020). Interval optimization for available transfer capability evaluation considering wind power uncertainty. IEEE Trans. Sustain. Energy. 11 (1), 250–259. doi:10.1109/TSTE.2018.2890125
Liang, R., Li, J., Gong, D., Huang, H., Liang, K., Liu, H., et al. (2024a). Optimal planning method for the high-quality coal mine energy system with complete clean energy supply. J. China Coal Soc. 49 (3), 1669–1679. doi:10.13225/j.cnki.jccs.2023.1511
Liang, R., Zhang, G., Yuan, L., Huang, H., Zhang, X., Lv, C., et al. (2024b). Coordinated operation of coal flow and transportation in coal mine power supply systems for low-carbon optimization. Proc. CSEE. 44 (6), 2245–2257. doi:10.13334/j.0258-8013.pcsee.221690
Lin, Z., Wu, Q., Chen, H., Ji, T., Xu, Y., and Sun, H. (2023). Scenarios-oriented distributionally robust optimization for energy and reserve scheduling. IEEE Trans. Power Syst. 38 (3), 2943–2946. doi:10.1109/TPWRS.2023.3244018
Lv, C., Liang, R., Jin, W., Chai, Y., and Yang, T. (2022). Multi-stage resilience scheduling of electricity-gas integrated energy system with multi-level decentralized reserve. Appl. Energy. 317, 119165. doi:10.1016/j.apenergy.2022.119165
Lv, C., Sun, W., Liang, R., Luo, G., Lin, S., and Cheng, Y. (2023). Information gap decision theory-based robust scheduling of coal mine integrated energy systems with power-to-gas. High. Volt. Technol. 49 (10), 4203–4212. doi:10.13336/j.1003-6520.hve.20230358
Nadaraju, F. J., Maddocks, A. R., Zanganeh, J., and Moghtaderi, B. (2019). Simulation of power and cooling generation via heat recovery from a ventilation air methane abatement unit. Fuel 249, 27–35. doi:10.1016/j.fuel.2019.03.077
Nikoobakht, A., Aghaei, J., Shafie-Khah, M., and Catalão, J. P. S. (2020). Continuous-time co-operation of integrated electricity and natural gas systems with responsive demands under wind power generation uncertainty. IEEE Trans. Smart Grid. 11 (4), 3156–3170. doi:10.1109/TSG.2020.2968152
Peng, C., Xiong, Z., Zhang, Y., and Zheng, C. (2022). Multi-objective robust optimization allocation for energy storage using a novel confidence gap decision method. Int. J. Electr. Power Energy Syst. 138, 107902. doi:10.1016/j.ijepes.2021.107902
Qiu, Y., Lin, J., Liu, F., Song, Y., Chen, G., and Ding, L. (2020). Stochastic online generation control of cascaded run-of-the-river hydropower for mitigating solar power volatility. IEEE Trans. Power Syst. 35 (6), 4709–4722. doi:10.1109/TPWRS.2020.2991229
Wang, D., Jasim, D. J., Zoghi, M., and Habibi, H. (2024). Optimized multi-criteria performance of a poly-generation layout including a Stirling engine and a supercritical Brayton cycle using biogas and methane as two potential fuels of a topping gas turbine cycle. Energy 310, 133172. doi:10.1016/j.energy.2024.133172
Wang, Y., Hu, H., Sun, X., Zhang, Y., and Gong, D. (2022). Unified operation optimization model of integrated coal mine energy systems and its solutions based on autonomous intelligence. Appl. Energy. 328, 120106. doi:10.1016/j.apenergy.2022.120106
Yazdaninejad, M., Amjady, N., and Dehghan, S. (2020). VPP self-scheduling strategy using multi-horizon IGDT, enhanced normalized normal constraint, and bi-directional decision-making approach. IEEE Trans. Smart Grid. 11 (4), 3632–3645. doi:10.1109/TSG.2019.2962968
Zhang, Q., Shukla, A., and Xie, L. (2024). Efficient scenario generation for chance-constrained economic dispatch considering ambient wind conditions. IEEE Trans. Power Syst. 39 (4), 5969–5980. doi:10.1109/TPWRS.2023.3349237
Zhang, S., Ge, S., Liu, H., Li, J., Gu, C., and Wang, C. (2023). A multi-objective chance-constrained information-gap decision model for active management to accommodate multiple uncertainties in distribution networks. J. Mod. Power Syst. Clean. Energy. 11 (1), 17–34. doi:10.35833/MPCE.2022.000193
Keywords: methane power generation, virtual storage characteristics of methane, wind-solarhydro-methane multi-resource coordination, fuzzy-enhanced information gap decision theory (F-EIGDT), economic dispatch
Citation: Chen W, Wang X, Tian R, Ji Y, Liu H and Yuan Y (2025) Coordinated optimization scheduling of wind, solar, hydro and methane power generation with adjustable methane. Front. Energy Res. 13:1563828. doi: 10.3389/fenrg.2025.1563828
Received: 20 January 2025; Accepted: 28 February 2025;
Published: 19 March 2025.
Edited by:
Quan Sui, Zhengzhou University, ChinaReviewed by:
Junjun Xu, Nanjing University of Posts and Telecommunications, ChinaCopyright © 2025 Chen, Wang, Tian, Ji, Liu and Yuan. This is an open-access article distributed under the terms of the Creative Commons Attribution License (CC BY). The use, distribution or reproduction in other forums is permitted, provided the original author(s) and the copyright owner(s) are credited and that the original publication in this journal is cited, in accordance with accepted academic practice. No use, distribution or reproduction is permitted which does not comply with these terms.
*Correspondence: Wengang Chen, amNjaGVud2VuZ2FuZ0AxNjMuY29t
Disclaimer: All claims expressed in this article are solely those of the authors and do not necessarily represent those of their affiliated organizations, or those of the publisher, the editors and the reviewers. Any product that may be evaluated in this article or claim that may be made by its manufacturer is not guaranteed or endorsed by the publisher.
Research integrity at Frontiers
Learn more about the work of our research integrity team to safeguard the quality of each article we publish.