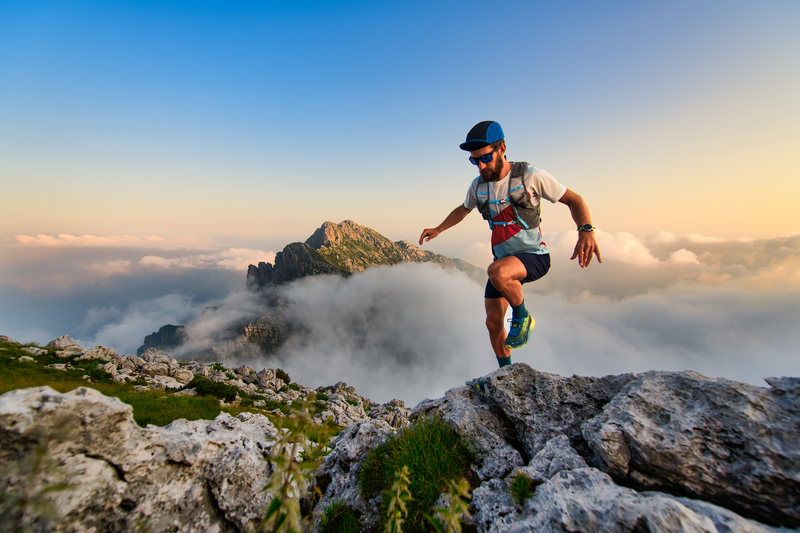
95% of researchers rate our articles as excellent or good
Learn more about the work of our research integrity team to safeguard the quality of each article we publish.
Find out more
BRIEF RESEARCH REPORT article
Front. Energy Res. , 14 January 2022
Sec. Smart Grids
Volume 9 - 2021 | https://doi.org/10.3389/fenrg.2021.835440
This article is part of the Research Topic Advanced Anomaly Detection Technologies and Applications in Energy Systems View all 64 articles
The sooner the system instability is predicted and the unstable branches are screened, the timelier emergency control can be implemented for a wind power system. In this paper, aiming at the problem that the existing unstable branch screening methods are lack prejudgment, an unstable branch screening method for power system with high-proportion wind power is proposed. Firstly, the equivalent external characteristics model of the wind farm was deduced. And based on this, the out-of-step oscillation characteristics of the power system with high proportion wind power was analyzed. Secondly, based on the oscillation characteristics, line weak-connection index (LWcI) was proposed to quantify the stability margin of a branch. Then an instability prediction method and an unstable branch screening method were proposed based on LWcI and voltage phase angle difference. Finally, the rapidity and effectiveness of the proposed method are verified through the simulation analysis of IEEE-118 system.
With the proposal of “carbon peaks” and “carbon neutralization” goals, the penetration of renewable energy is increasing rapidly. However, different from synchronous generator, wind power and other renewable energy units have the disadvantages of low inertia and weak poor disturbance immunity, which have a profound impact on the stability of the grid (Liu et al., 2019; Shen and Raksincharoensak, 2021a). Power outage accidents (Nagpal et al., 2018; Alhelou et al., 2019; Liu, 2019; Yang et al., 2021c) in recent years indicate that due to the replacement of synchronous machines by renewable energy sources, the risk of power angle instability is greatly increased, and the development rate of chain fault is greatly accelerated. Therefore, how to prejudge the instability of the system and screen unstable branches as early as possible, so as to reserve more time to guarantee the implementation of emergency control, has become a research hotspot.
Currently, research results about instability criteria are mainly from traditional power systems. For example, Yang (Yang et al., 2006) proposed to use the extended equal area criterion (EEAC) to analyze the transient stability. Yan (Yan et al., 2011) used the maximal Lyapunov exponent (MLE) to prejudge the rotor angle stability. Saunders (Saunders et al., 2014) analyzed transient stability by constructing transient potential energy functions. Yang (Yang et al., 2021b) adopted data-driven method to study power system security and stability problem. This paper was the first study for SCUC problems, it can accommodate the mapping samples of SCUC, and consider the various input factors that affect SCUC decision-making, possessing strong generality, high solution accuracy, and efficiency over traditional methods. The above methods can prejudge the transient stability of the grid quickly using generator information such as rotor angle, but the further application in the emergency control such as the splitting control is limited because the splitting section cannot be determined directly. Therefore, some scholars designed some new instability criterion using the branch response information, such as ucosφ criterion (Yang et al., 2013; Zhang et al., 2021), phase angle criterion (Regulski et al., 2018; Shen et al., 2021a), bus voltage frequency criterion (Zhang et al., 2019; Yang et al., 2022), apparent impedance differential method (Sreenivasachar, 2021; Shen and Raksincharoensak, 2021b) and so on. Compared with methods using generator response information, these methods can not only prejudge the unstable state of the system, but also accurately capture the unstable branches and the splitting section. However, the disadvantage is that these methods do not start until the instability accident has occurred (or approach to instability), thus it is difficult to reserve sufficient time for emergency control.
Research on the instability criterion of the high proportion renewable energy power system is still in the preliminary stage, and relevant work is mainly focused on the influence of wind power integration on transient stability (Ma et al., 2017; Liu et al., 2017; Zheng et al., 2019; Shen et al., 2021b). As for the research on the instability criterion, Wei (Wei et al., 2021; Yang et al., 2021a) and Qin (Li et al., 2021; Qin et al., 2021) respectively analyzed the influence of wind and photovoltaic power integration on oscillation center migration, but failed to propose oscillation center identification method and instability criterion. Chen (Chen et al., 2020; Li et al., 2021) proposed a new transient stability prediction method by defining a new stability index, but still failed to capture the unstable branch. Liu (Liu et al., 2020) proposed to use the slow coherency theory to study the coherency group of wind power system, but the impact of actual faults cannot be fully considered.
Therefore, aiming at the problem that the existing instability criterion lacks prejudgment, a system instability prediction method and an unstable branches screening method are proposed in this paper. which can predict power angle instability earlier and screen unstable branches accurately.
The rest of this paper is organized as follows. In Section 2, the out-of-step oscillation characteristics of the high proportion wind power system is analyzed. In Section 3, the line weak-connection index (LWcI) is proposed to quantify the stability margin, and the unstable branches screening method is proposed. Case study and discussion are shown in Section 4. Section 5 concludes this study by summarizing key findings and contributions of this paper.
Taking doubly-fed induction generator (DFIG) as an example, the control strategy is shown in Figure 1A (Wang et al., 2015). In steady state, DFIG adopts Maximum Power Point Tracking (MPPT) control strategy. When the system is disturbed, DFIG limits the active power output under the fault ride-through control, and outputs reactive power to support the grid. After the fault is cleared, DFIG switches back to MPPT control.
FIGURE 1. Out-of-step oscillation characteristics of wind power system (A) is the control strategy of DFIG (B) is the equivalent two-machine system with DFIG integrated (C) is the distribution law of Um in δ-λ space (D) is the variation law between Um and λ (E) is the variation law between Um and δ (F) is the distribution law of θm in δ-λ space (G) is the variation law of θm with δ at different position (H) is the variation law between Δθm and δ of different branches (I) is the variation law between Um at point n4 and δ when wind farm output changes (J) is the variation law between Δθm of B5-6 and δ when wind farm output changes.
Obviously, without additional control, DFIG cannot actively respond to the disturbance of the system. When the system is disturbed, the output power of DFIG do not oscillate like a synchronous generator, but quickly return to the original state. Therefore, there is no power angle swing between DFIG and synchronous generator in electromechanical time scale. Thus, DFIG can be regarded as a power source without inertia, and its equivalent external characteristics can be equivalent to negative resistance and negative reactance in parallel:
Where the negative sign indicates the power injected into the bus.
In addition, if the system is still transient stable after the fault is cleared, DFIG can quickly recover to the pre-fault state and the output power also quickly resumes stability. So the equivalent impedance can be considered constant. If the system is already unstable, the output power of DFIG will oscillate due to the oscillation of the grid voltage. And the equivalent impedance also oscillates. Considering that there is a certain inertia in the system, it takes a certain amount of time from fault removal to complete instability, during which the output power of DFIG does not change dramatically, so it can be simply considered that the equivalent impedance during this period is constant.
Taking an equivalent two-machine system with a DFIG-based wind farm integrated as an example to analyze the out-of-step oscillation characteristics of wind power system. As shown in Figure 1B, bus O is the common coupling point of the wind farm, and the voltage vector can be expressed as Uo∠θo. r and x are equivalent resistance and reactance of the wind farm respectively. E1 and E2 are the equivalent potentials of generator G1 and G2 respectively, and δ is the rotor angle difference. X1 and X2 are equivalent resistance between G1 and bus O, bus O and G2, respectively. For convenience analysis, the X2 is divided into 10 equivalents by points n1∼n11, and each branch section is represented by B1-2∼B10-11. The voltage vector of any point M between the bus O and G2 is expressed as Um∠θm, and its position function is λ = Xm/X2, λ∈[0,1].
Based on the node voltage method, the voltage vector of bus O can be expressed as:
Where
Considering that the active power output of DFIG is limited under low voltage ride-through (LVRT) control, so the
As shown in Eq. 3, the integration of wind farm changes the amplitude of
In addition, according to Yang (Yang et al., 2013), the unequal voltage on both sides of the branch is one of the reasons for the migration of out-of-step center. When the voltage amplitude on both sides of the branch is unequal, the out-of-step center migrates to the side where the voltage amplitude is smaller. Therefore, the integration of wind power increases the voltage amplitude of the common coupling point, resulting in the unequal voltage amplitude on both sides of the branch, which in turn leads to the migration of the out-of-step center to the side where the voltage amplitude is smaller.
Then, according to Ohm’s law, the following equation exists:
Therefore, based on Equation 2 and Equation 4, the voltage vector of point M can be expressed as:
Ignoring the real part of
According to Eq. 6, the Um and θm are related to the position function λ, the output power of wind farm, the power angle difference and so on. Thus, assuming that E1 = E2 = 1.0, X2 = 3X1 = 0.3, and the output power of the wind farm under LVRT control is 0.1 + j0.5, the temporal and spatial distribution law of voltage amplitude Um and θm are shown in Figures 1C–H.
Figures 1C–E show that with the increase of δ, the voltage Um at any position on the branch decreases and then increases, getting the minimum value when δ = 180°. Meanwhile, the variation amplitude of Um varies from location to location, so there is always a point where the variation is the most drastic, called the out-of-step center. According to Figure 1E, the out-of-step center is located between n4 and n5.
According to Figures 1F–H, when δ increases from 0° to 180°, for the node between the out-of-step center and G1, θm changes monotonically in (0°, 180°). While for the node between the out-of-step center and G2, θm firstly increases and then decreases, and the maximum value is no more than 90°. Therefore, only for the branch where the out-of-step center is located, the voltage phase difference Δθm variation rule is consistent with the power angle difference δ.
According to Figures 1I,J, as the output power of wind farm increases, the Um at point n4 increases, and the maximum value of Δθm at B5-6 increases until the Δθm changes monotonically between (0°, 180°), indicating that the out-of-step center migrates from point n4 to point n5, until it completely enters into B5-6.
In summary, the output power of wind farm changes the flow distribution and voltage level of the system, ultimately affecting the system transient stability and the out-of-step center position. However, the phenomenon that voltage amplitude drops sharply, and the voltage phase angle difference of the branch where the out-of-step center located increases monotonously are still exist, which can be used to predict the transient stability of the system and capture the unstable branch.
According to the conclusion in Section 2, when the out-of-step oscillation occurs, one or more branches loses stability, resulting in a sharp drop of voltage at each point of the branches. Considering that the voltage reduction at the oscillation center is the most severe (Yang et al., 2013), so the voltage of the oscillation center can be used to measure the stability margin of a branch. However, if the oscillation center is not on the branch due to the oscillation center migration, the voltage at one end of the branch is the minimum voltage, which is much greater than the oscillation center voltage. And the result can be conservative and misjudgment can occur. Therefore, in order to prevent misjudgment as the oscillation center migrates outside the branch, the terminal voltage can be used to correct the out-of-step center voltage. Thus, Line weak-connection Index (LWcI) is defined to reflect the stability margin of a branch:
Where
According to Eq. 7, there is a negative correlation between LWCI and Δθ. The larger the Δθ, the smaller the branch stability margin, the smaller the LWcI. Therefore, LWcI can be used to prejudge the transient stability of the wind power system.
Further, according to Section 2.2, only the Δθ of the unstable branch varies monotonously in (−180°, 180°). Thus, there are significant differences in the trajectories between the unstable branches and others in the plane with Δθ as the horizontal axis and LWcI as the vertical axis.
Taking the wind power system in Figure 1B as an example, the trajectory of each branch in LWcI-Δθ plane when the system is stable and unstable is shown in Figure 2.
FIGURE 2. Analysis of branch trajectory characteristics (A) is the Δθ-t curve of different branches when the system is stable (B) is the LWcI-Δθ curve of different branches when the system is stable (C) is the Δθ-t curve of different branches when the system is unstable (D) is the LWcI-Δθ curve of different branches when the system is unstable.
As shown in Figures 2A,B, the Δθ of all branches constantly oscillate and decays when the power angle is stable. Taking one period as an example, during t1∼t2, the Δθ increases, and the stability margin of all branches decreases, so the trajectories in LWcI-Δθ plane shows a monotonically decreasing trend, that is:
During t2∼t3, the Δθ of each branch decreases and the stability margin increases, as shown in Eq. 9. Therefore, an “upward inflection point” appears on the trajectory in LWcI-Δθ plane, indicating that there is no risk of instability in this cycle.
As shown in Figure 2C, only the Δθ of B5-7 exceeds 180°, which is the unstable branch. Corresponding to Figure 2D, only the trajectory of B5-7 monotonically decreases, satisfying Eq. 8. While for a branch which is still stable, because the extreme value of Δθ exists, a “downward inflection point” appears. After crossing the “downward inflection point”, as the system is already unstable, the voltage of each node is still decreasing, so the LWcI and Δθ both decrease, as shown in.
Therefore, the “unstable branch capture area”, which is shown in Figure 2D, can be used to prejudge the system instability and screen the unstable branch. If the trajectory of a branch always satisfies Eq. 8 and enters into the “unstable branch capture area”, which can be expressed as in Eq. 11, the stability margin of the branch is significantly reduced and the system is at risk of instability.
Where
The system instability prediction and unstable branch screening process proposed in this paper is shown in Figure 3. After the fault is cleared, the voltage phase angle difference of each branch is firstly calculated. As shown in Eq. 12, if it is greater than the threshold, the stability margin of the branch needs further analysis.
Secondly, calculate LWcI of the branch which satisfies Eq. 12. If the value is lower than the threshold as shown in Eq. 13, the stability margin is already small, and it is necessary to monitor the trajectory in the LWcI-Δθ plane.
Thirdly, if the trajectory of the branch satisfying Equation 12 and Equation 13 in the LWcI-Δθ plane always satisfies Eq. 8, the stability margin of the branch keeps decreasing and the risk of instability keeps increasing. When the trajectory satisfies Eq. 11, that is, it enters into the “unstable branch capture area”, the branch can be considered as an unstable branch.
Finally, traverse all branches in turn, and then repeat the steps above based on the data at the next sampling moment until all unstable branches constitute a splitting section.
In order to verify the effectiveness of the method proposed in this paper, the IEEE-118 system with wind farm integrated is built on the PSS/E platform. As shown in Figure 4A, the synchronous generators on the bus 89, 100 and 103 are replaced by wind farms, the capacity of which is 450, 300 and 300 MVA, respectively. Meanwhile, another wind farm with capacity of 75MVA is integrated at the bus 25. Assume that the output power of wind farms equals to the rated power, and the load power is 4252 MW, so the penetration rate of wind power is 26.46%.
FIGURE 4. The transient response of the IEEE-118 wind power system (A) is the diagram of IEEE-118 system with wind farms (B) is power angle curves of generators (C) is the voltage phase angle difference of branches (D) is the LWcI-t curve of different branches (E) is the LWcI-Δθ curve of different branches during the period of 0.39 and 0.51 s.
According to Eq. 7, when the voltage on both sides is equal and the Δθ is equal to 130°, LWcI = 0.41. Considering that the system is still stable at that time, so set LWcIth = 0.4,θth = 20°. According to the variation rule of Δθ of an unstable branch, set
Assume that a three-phase short circuit failure occurs on branch 8–30 at 0.1 s, and lasts 0.2 s. The transient response of the system is shown in Figures 4B–E. Considering that there are too many branches, only the branches whose voltage phase angle difference is greater than 20° are displayed. According to Figures 4B,C, the power angle of G10 and G12 increases rapidly after the fault is cleared, and the voltage phase angle difference of branch 8–30 13–15 14–15 and 16–17 continues to increase, indicating that G10 and G12 lose stability compared with other generators, and the out-of-step center is located on branch 8–30 13–15 14–15 and 16–17.
As shown in Figure 4D, the LWcI of each branch is monotonically decreasing when the fault is removed, indicating that the stability margin of each branch declines continuously. Around 0.39s, the LWcI of branch 8–30 firstly decreases to 0.4, satisfying Eq. 13. And the program begins to monitor the trajectory of each branch in the LWcI-Δθ plane to screen the unstable branches. Corresponding to Figure 4E, the trajectories of the branch 8–30 13–15 14–15 and 16–17 in the LWcI-Δθ plane are always monotonously decreasing, and enter into the " unstable branch capture area” at 0.45, 0.47, 0.48 and 0.49 s respectively. It indicates that the above branches are all unstable and constitute a splitting section, as shown by the red dashed line in Figure 4A. By contrast, at 0.49 s, the trajectories of the branch 9–8, 10–9, 11–13 are located at points B, C and D respectively. Obviously, there have been inflection points in the trajectories of branch 9–8 and 10–9, indicating that there is no out-of-step center on branch 9–8 and 10–9. For branch 11–13, the trajectory still drops monotonically, so it still needs to be monitored. But according to “upward inflection point” in the trajectory during the period of 0.49–0.51 s, it can be judged that branch 11–13 are not unstable branch, either.
In summary, the unstable status of the system can be prejudged and all unstable branches can be accurately screened at 0.49 s using the method in this paper. If the traditional method (Tang et al., 2015) is adopted, the complete splitting section cannot be obtained until the system is already unstable at 0.57 s. Therefore, compared with other methods, the method proposed in this paper can prejudge unstable status of the system and screen all the unstable branches earlier under the premise of ensuring correctness, and thus reserve more time for emergency control such as splitting control.
An instability prediction method and an unstable branch screening method are proposed in this paper. Some conclusions are summed up as follows. Firstly, when the wind power system loses stability, the bus voltage amplitude drops, and the voltage phase angle difference of the unstable branch increases, which are the key response information mapped on the branch. Secondly, according to the response information, an indicator called line weak-connection index (LWcI) is built to assess the stability margin of a branch. The smaller the LWcI, the smaller the stability margin of the branch. Thirdly, the trajectories of different branches are different in the plane composed of LWcI and voltage phase angle difference. Only the trajectory of the unstable branch shows a monotonically decreasing trend, while there are “upward inflection points” or “downward inflection points” in the trajectories of other branches. Depending on the difference of trajectory characteristics, the unstable branch can be accurately screened.
The original contributions presented in the study are included in the article/supplementary material, further inquiries can be directed to the corresponding author.
FT was responsible for methodology and revision of the manuscript. XW was responsible for writing the original draft. YG and JQ were responsible for data processing and supervision. JX and XL were responsible for visualization and supervision. All authors contributed, read, and approved the submitted version.
This work was supported by the National Natural Science Foundation of China under Grant 51977157.
The authors declare that the research was conducted in the absence of any commercial or financial relationships that could be construed as a potential conflict of interest.
All claims expressed in this article are solely those of the authors and do not necessarily represent those of their affiliated organizations, or those of the publisher, the editors and the reviewers. Any product that may be evaluated in this article, or claim that may be made by its manufacturer, is not guaranteed or endorsed by the publisher.
Alhelou, H., Hamedani-Golshan, M., Njenda, T., and Siano, P. (2019). A Survey on Power System Blackout and Cascading Events: Research Motivations and Challenges. Energies 12 (4), 682. doi:10.3390/en12040682
Chen, Y., Mazhari, S. M., Chung, C. Y., Faried, S. O., and Pal, B. C. (2020). Rotor Angle Stability Prediction of Power Systems with High Wind Power Penetration Using a Stability Index Vector. IEEE Trans. Power Syst. 35 (6), 4632–4643. doi:10.1109/TPWRS.2020.2989725
Li, Z., Jiang, W., Abu-Siada, A., Li, Z., Xu, Y., and Liu, S. (2021). Research on a Composite Voltage and Current Measurement Device for HVDC Networks. IEEE Trans. Ind. Electron. 68 (9), 8930–8941. doi:10.1109/TIE.2020.3013772
Liu, J., Tang, F., Zhao, J., Liu, D., and Kamwa, I. (2020). Coherency Identification for Wind-Integrated Power System Using Virtual Synchronous Motion Equation. IEEE Trans. Power Syst. 35 (4), 2619–2630. doi:10.1109/TPWRS.2020.2968294
Liu, J., Yang, D., Yao, W., Fang, R., Zhao, H., and Wang, B. (2017). PV-based Virtual Synchronous Generator with Variable Inertia to Enhance Power System Transient Stability Utilizing the Energy Storage System. Prot. Control. Mod. Power Syst. 2 (1), 39. doi:10.1186/s41601-017-0070-0
Liu, Y. (2019). “Analysis of Brazilian Blackout on March 21st,2018 and Revelations to Security for Hunan Grid,” in 2019 4th International Conference on Intelligent Green Building and Smart Grid (IGBSG), Yichang, China, 06-09 Sep. 2019, 422–426. doi:10.1109/IGBSG.2019.8886219
Mosi, L., Zhiyuan, S., Guangshi, L., Mingpo, L., and Xiaoyin, Q. (2019). “Study on the Influence of Large-Scale Wind Power Integration on Transient Stability of Power System,” in 2019 IEEE 8th International Conference on Advanced Power System Automation and Protection (APAP), Xi'an, China, 21-24 Oct. 2019, 1156–1159. doi:10.1109/APAP47170.2019.9224650
Ma, Z., Chen, H., and Chai, Y. (2017). Analysis of Voltage Stability Uncertainty Using Stochastic Response Surface Method Related to Wind Farm Correlation. Prot. Control. Mod. Power Syst. 2 (1), 20. doi:10.1186/s41601-017-0051-3
Nagpal, M., Martinich, T. G., Jiao, Z., Manuel, S.-H., Zhang, H. A., and Alimardani, A. (2018). Lessons Learned from a Regional System Blackout and Restoration in BC Hydro. IEEE Trans. Power Deliv. 33 (4), 1954–1961. doi:10.1109/TPWRD.2017.2768046
Qin, F., Tang, F., Zhang, T., Wei, X., and Li, Y. (2021). “Impact of Photovoltaic Grid-Connected on Distribution of Power System Disturbed Oscillation Center,” in 2021 IEEE 4th International Electrical and Energy Conference (CIEEC), Wuhan, China, 28-30 May 2021. doi:10.1109/CIEEC50170.2021.9510601
Regulski, P., Rebizant, W., Kereit, M., and Herrmann, H.-J. (2018). “PMU-based Generator Out-of-step Protection,” in 2018 10th IFAC Symposium on Control of Power and Energy Systems (CPES), Tokyo, Japan, 04-06 Sep. 2018, 79–84. IFAC-PapersOnLine51. doi:10.1016/j.ifacol.2018.11.681
Saunders, C. S., Alamuti, M. M., and Taylor, G. A. (2014). “Transient Stability Analysis Using Potential Energy Indices for Determining Critical Generator Sets,” in 2014 IEEE PES General Meeting - Conference & Exposition, National Harbor, MD, USA, 27-31 July. 2014, 1–5. doi:10.1109/PESGM.2014.6939235
Shen, X., Ouyang, T., Khajorntraidet, C., Li, Y., Li, S., and Zhuang, J. (2021a). Mixture Density Networks-Based Knock Simulator. Ieee/asme Trans. Mechatron., 1. doi:10.1109/TMECH.2021.3059775
Shen, X., Ouyang, T., Yang, N., and Zhuang, J. (2021b). Sample-Based Neural Approximation Approach for Probabilistic Constrained Programs. IEEE Trans. Neural Netw. Learn. Syst., 1–8. doi:10.1109/TNNLS.2021.3102323
Shen, X., and Raksincharoensak, P. (2021a). Pedestrian-Aware Statistical Risk Assessment. IEEE Trans. Intell. Transport. Syst., 1–9. doi:10.1109/TITS.2021.3074522
Shen, X., and Raksincharoensak, P. (2021b). Statistical Models of Near-Accident Event and Pedestrian Behavior at Non-signalized Intersections. J. Appl. Stat., 1–21. doi:10.1080/02664763.2021.1962263
Sreenivasachar, K. (2021). Out-Of-Step Detection on Transmission Lines Using Apparent Impedance Differential Method. IEEE Trans. Power Deliv., 1. doi:10.1109/TPWRD.2021.3125525
Tang, F., Yang, J., Liao, Q., Wang, Y., and Jia, J. (2015). Out-of-step Oscillation Splitting Criterion Based on Bus Voltage Frequency. J. Mod. Power Syst. Clean. Energ. 3 (3), 341–352. doi:10.1007/s40565-015-0140-0
Wang, Y., Hu, J., Zhang, D., Ye, C., and Li, Q. (2015). “DFIG WT Electromechanical Transient Behaviour Influenced by PLL: Modelling and Analysis,” in International Conference on Renewable Power Generation (RPG 2015), Beijing, China, 17-18 Oct. 2015. doi:10.1049/cp.2015.0331
Wei, X., Tang, F., Li, Y., Qin, F., and Zhang, T. (2021). “Study on the Oscillation Center of DFIG-Based Wind Farm Power System,” in 2021 IEEE 4th International Electrical and Energy Conference (CIEEC), Wuhan, China, 28-30 May 2021. doi:10.1109/CIEEC50170.2021.9510498
Xiaoming, Y., and Dichen, L. (2013). “Research on Dynamic Migration Mechanism of Out-of-step Oscillation center Based on Wide Area Measurement,” in 3rd International Conference on Intelligent System Design and Engineering Applications (ISDEA), Hong Kong, China, 16-18 Jan. 2013. doi:10.1109/ISDEA.2012.275
Yan, J., Liu, C.-C., and Vaidya, U. (2011). PMU-based Monitoring of Rotor Angle Dynamics. IEEE Trans. Power Syst. 26 (4), 2125–2133. doi:10.1109/TPWRS.2011.2111465
Yang, J., Liao, Q. F., Tang, F., Wang, Y. F., Zhou, Y. T., and Tu, L. (2013). Study on Splitting Criterion Based on Improvement Ucosφ. Amm 433-435, 1313–1319. doi:10.4028/www.scientific.net/AMM.433-435.1313
Yang, N., Liu, S., Deng, Y., and Xing, C. (2021a). An Improved Robust SCUC Approach Considering Multiple Uncertainty and Correlation. IEEJ Trans. Elec Electron. Eng. 16 (1), 21–34. doi:10.1002/tee.23265
Yang, N., Qin, T., Wu, L., Huang, Y., Huang, Y., Xing, C., et al. (2022). A Multi-Agent Game Based Joint Planning Approach for Electricity-Gas Integrated Energy Systems Considering Wind Power Uncertainty. Electric Power Syst. Res. 204, 107673. doi:10.1016/j.epsr.2021.107673
Yang, N., Yang, C., Wu, L., Shen, X., Jia, J., Li, Z., et al. (2021b). Intelligent Data-Driven Decision-Making Method for Dynamic Multi-Sequence: An E-Seq2Seq Based SCUC Expert System. IEEE Trans. Ind. Inf., 1. doi:10.1109/TII.2021.3107406
Yang, N., Yang, C., Xing, C., Ye, D., Jia, J., Chen, D., et al. (2021c). Deep Learning‐based SCUC Decision‐making: An Intelligent Data‐driven Approach with Self‐learning Capabilities. IET Gener. Transm. Distrib. doi:10.1049/gtd2.12315
Yang, W., Xue, Y., Song, X., Wu, D., Ge, F., and Xie, D. (2006). “Study on Stability Mechanism for a Typical Fault with EEAC Theory,” in 2006 International Conference on Power System Technology, Chongqing, China, 22-26 Oct. 2006, 1–4. doi:10.1109/ICPST.2006.321621
Zhang, L., Xie, Y., Ye, J., Xue, T., Cheng, J., Li, Z., et al. (2021). Intelligent Frequency Control Strategy Based on Reinforcement Learning of Multi-Objective Collaborative Reward Function. Front. Energ. Res. 9, 587. doi:10.3389/fenrg.2021.760525
Zhang, S., and Zhang, Y. (2019). Characteristic Analysis and Calculation of Frequencies of Voltages in Out-of-step Oscillation Power System and a Frequency-Based Out-of-step Protection. IEEE Trans. Power Syst. 34 (1), 205–214. doi:10.1109/TPWRS.2018.2866022
Keywords: unstable branch, transient stability, line weak-connection index, wind power, out-of-step oscillation
Citation: Tang F, Wei X, Guo Y, Qi J, Xie J and Li X (2022) Research on the Unstable Branch Screening Method for Power System With High-Proportion Wind Power. Front. Energy Res. 9:835440. doi: 10.3389/fenrg.2021.835440
Received: 14 December 2021; Accepted: 24 December 2021;
Published: 14 January 2022.
Edited by:
Xun Shen, Tokyo Institute of Technology, JapanReviewed by:
Yingbiao Li, Huazhong University of Science and Technology, ChinaCopyright © 2022 Tang, Wei, Guo, Qi, Xie and Li. This is an open-access article distributed under the terms of the Creative Commons Attribution License (CC BY). The use, distribution or reproduction in other forums is permitted, provided the original author(s) and the copyright owner(s) are credited and that the original publication in this journal is cited, in accordance with accepted academic practice. No use, distribution or reproduction is permitted which does not comply with these terms.
*Correspondence: Xiaoqing Wei, MTcxODIwMTc1QHFxLmNvbQ==
Disclaimer: All claims expressed in this article are solely those of the authors and do not necessarily represent those of their affiliated organizations, or those of the publisher, the editors and the reviewers. Any product that may be evaluated in this article or claim that may be made by its manufacturer is not guaranteed or endorsed by the publisher.
Research integrity at Frontiers
Learn more about the work of our research integrity team to safeguard the quality of each article we publish.