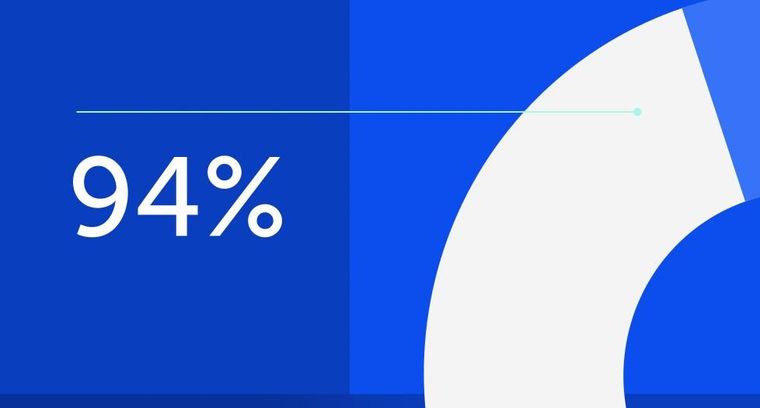
94% of researchers rate our articles as excellent or good
Learn more about the work of our research integrity team to safeguard the quality of each article we publish.
Find out more
CORRECTION article
Front. Endocrinol., 05 March 2025
Sec. Pediatric Endocrinology
Volume 16 - 2025 | https://doi.org/10.3389/fendo.2025.1579425
This article is a correction to:
Meta-analysis of machine learning models for the diagnosis of central precocious puberty based on clinical, hormonal (laboratory) and imaging data
By Chen Y, Huang X and Tian L (2024) Front. Endocrinol. 15:1353023. doi: 10.3389/fendo.2024.1353023
In the published article, there was an error in affiliation(s) 1. Instead of “Chongqing University”, it should be “Chongqing Medical University”.
The authors apologize for this error and state that this does not change the scientific conclusions of the article in any way. The original article has been updated.
All claims expressed in this article are solely those of the authors and do not necessarily represent those of their affiliated organizations, or those of the publisher, the editors and the reviewers. Any product that may be evaluated in this article, or claim that may be made by its manufacturer, is not guaranteed or endorsed by the publisher.
Keywords: machine learning, central precocious puberty, meta-analysis, ML, CPP
Citation: Chen Y, Huang X and Tian L (2025) Corrigendum: Meta-analysis of machine learning models for the diagnosis of central precocious puberty based on clinical, hormonal (laboratory) and imaging data. Front. Endocrinol. 16:1579425. doi: 10.3389/fendo.2025.1579425
Received: 19 February 2025; Accepted: 20 February 2025;
Published: 05 March 2025.
Approved by:
Frontiers Editorial Office, Frontiers Media SA, SwitzerlandCopyright © 2025 Chen, Huang and Tian. This is an open-access article distributed under the terms of the Creative Commons Attribution License (CC BY). The use, distribution or reproduction in other forums is permitted, provided the original author(s) and the copyright owner(s) are credited and that the original publication in this journal is cited, in accordance with accepted academic practice. No use, distribution or reproduction is permitted which does not comply with these terms.
*Correspondence: Lu Tian, MTk1NzkyMTc5QHFxLmNvbQ==
†These authors have contributed equally to this work
Disclaimer: All claims expressed in this article are solely those of the authors and do not necessarily represent those of their affiliated organizations, or those of the publisher, the editors and the reviewers. Any product that may be evaluated in this article or claim that may be made by its manufacturer is not guaranteed or endorsed by the publisher.
Research integrity at Frontiers
Learn more about the work of our research integrity team to safeguard the quality of each article we publish.