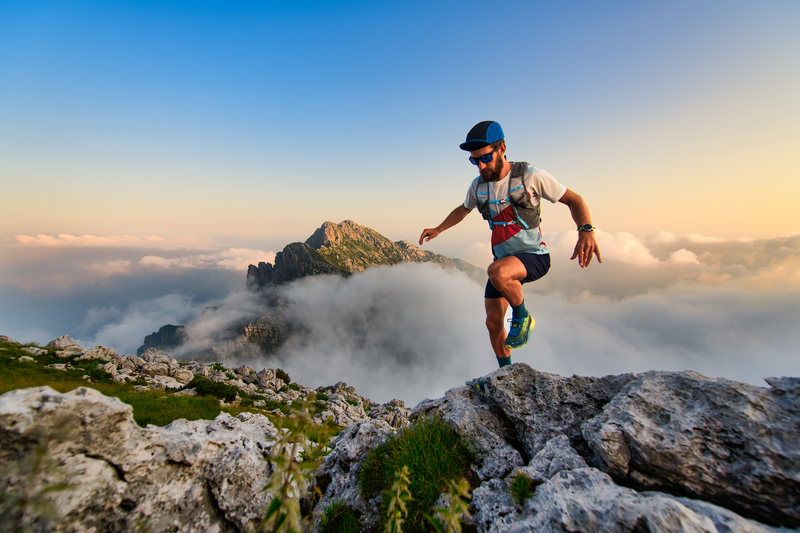
94% of researchers rate our articles as excellent or good
Learn more about the work of our research integrity team to safeguard the quality of each article we publish.
Find out more
SYSTEMATIC REVIEW article
Front. Endocrinol. , 25 March 2024
Sec. Pediatric Endocrinology
Volume 15 - 2024 | https://doi.org/10.3389/fendo.2024.1353023
This article is part of the Research Topic Challenges in pediatric endocrinology regarding alterations in glucose metabolism, growth disorders, disorders of sex development, and puberty in adolescents. View all 8 articles
Background: Central precocious puberty (CPP) is a common endocrine disorder in children, and its diagnosis primarily relies on the gonadotropin-releasing hormone (GnRH) stimulation test, which is expensive and time-consuming. With the widespread application of artificial intelligence in medicine, some studies have utilized clinical, hormonal (laboratory) and imaging data-based machine learning (ML) models to identify CPP. However, the results of these studies varied widely and were challenging to directly compare, mainly due to diverse ML methods. Therefore, the diagnostic value of clinical, hormonal (laboratory) and imaging data-based ML models for CPP remains elusive. The aim of this study was to investigate the diagnostic value of ML models based on clinical, hormonal (laboratory) and imaging data for CPP through a meta-analysis of existing studies.
Methods: We conducted a comprehensive search for relevant English articles on clinical, hormonal (laboratory) and imaging data-based ML models for diagnosing CPP, covering the period from the database creation date to December 2023. Pooled sensitivity, specificity, positive likelihood ratio (LR+), negative likelihood ratio (LR-), summary receiver operating characteristic (SROC) curve, and area under the curve (AUC) were calculated to assess the diagnostic value of clinical, hormonal (laboratory) and imaging data-based ML models for diagnosing CPP. The I2 test was employed to evaluate heterogeneity, and the source of heterogeneity was investigated through meta-regression analysis. Publication bias was assessed using the Deeks funnel plot asymmetry test.
Results: Six studies met the eligibility criteria. The pooled sensitivity and specificity were 0.82 (95% confidence interval (CI) 0.62-0.93) and 0.85 (95% CI 0.80-0.90), respectively. The LR+ was 6.00, and the LR- was 0.21, indicating that clinical, hormonal (laboratory) and imaging data-based ML models exhibited an excellent ability to confirm or exclude CPP. Additionally, the SROC curve showed that the AUC of the clinical, hormonal (laboratory) and imaging data-based ML models in the diagnosis of CPP was 0.90 (95% CI 0.87-0.92), demonstrating good diagnostic value for CPP.
Conclusion: Based on the outcomes of our meta-analysis, clinical and imaging data-based ML models are excellent diagnostic tools with high sensitivity, specificity, and AUC in the diagnosis of CPP. Despite the geographical limitations of the study findings, future research endeavors will strive to address these issues to enhance their applicability and reliability, providing more precise guidance for the differentiation and treatment of CPP.
Central precocious puberty (CPP), also known as true precocious puberty, is characterized by the premature activation of the hypothalamic-pituitary-gonadal (HPG) axis, resulting in clinical pubertal symptoms in girls under 8 years old and boys under 9 years old (1–3). Several countries have conducted research on the prevalence of precocious puberty within their own borders, with findings indicating an increasing trend in childhood precocious puberty. However, consensus regarding the underlying reasons for this rise remains elusive (4–6). Previous studies have indicated a much higher likelihood of girls developing idiopathic central precocious puberty compared to boys. However, a recent nationwide multicenter study from Italy suggests that the percentage of idiopathic forms of CPP in boys may be much higher than previously reported (7). CPP can potentially impact adult height and may even lead to social and psychological disturbances. Notably, girls with CPP face an elevated risk of developing breast or cervical cancer (8, 9). Consequently, timely diagnosis and treatment are crucial for girls with CPP. Meanwhile, peripheral precocious puberty (PPP), clinically known as pseudoprecocious puberty, exhibits clinical features similar to CPP but without activation of the hypothalamic-pituitary-gonadal (HPG) axis (1). In routine clinical diagnosis, diagnosing CPP is challenging without the gonadotropin-releasing hormone (GnRH) stimulation test. However, this test is not only expensive and time-consuming but also often causes anxiety in patients due to the need for establishing vascular access and collecting multiple blood samples at various intervals (10, 11). Furthermore, in non-tertiary or community hospitals with limited resources, this laborious test is not consistently accessible. Therefore, some studies have attempted to explore other convenient clinical, hormonal (laboratory) and imaging-related markers, such as basal sex hormone levels, pelvic ultrasound, or bone age, to identify patients with idiopathic CPP (12–15). However, the cut-off values of these factors varied widely, and their efficiency remains unclear. In recent years, with the advancement of artificial intelligence (AI) in the medical field, clinical, hormonal (laboratory) and imaging data-based machine learning (ML) models have utilized clinical, hormonal (laboratory) and imaging data as inputs to create classifiers, enabling the rapid identification of CPP. This approach offers a new perspective for an objective, swift, and intelligent diagnosis of CPP. However, the results of these studies varied widely and were difficult to directly compare, mainly due to diverse ML methods (16–21). Therefore, the diagnostic value of clinical, hormonal (laboratory) and imaging data-based ML models for CPP remains elusive. The aim of this study was to investigate the diagnostic value of ML models based on clinical, hormonal (laboratory) and imaging data for CPP through a meta-analysis of existing studies.
This meta-analysis conformed to the recommendations provided in the Cochrane Handbook for Systematic Reviews of Diagnostic Test Accuracy (22) and adhered to the guidelines set by the Preferred Reporting Items for Systematic Reviews and Meta-Analyses (PRISMA) (23).
A comprehensive search was performed using PubMed, EMBASE, The Cochrane Library, Wiley Online Library, and the Web of Science to identify relevant articles in English (database creation dated to December 2023).The search strategy adhered to the Population, Intervention, Comparison, Outcome, and Study Design (PICOS) principle (24) (P: “CPP”, I: “ML, DL”, S: “diagnostic test”). The search employed a blend of Medical Subject Heading (MeSH) terms and free-text terms, as follows:(“central precocious puberty” [MeSH] or “CPP” [text]) and (“machine learning” [MeSH] or “ML” [text] or “deep learning” [text] or “DL” [text]) and (“sensitivity and specificity” [MeSH] or predict* [text] or diagnos* [text] or accura* [text]).
Included studies were those that employed machine learning models utilizing clinical, hormonal (laboratory) and imaging data for the diagnosis of CPP, with the capability to formulate a 2×2 table encompassing true positive (TP), false positive (FP), true negative (TN), and false negative (FN) values. Studies lacking a clearly defined reference standard or providing insufficient data for the computation of study outcomes were excluded. Animal experiments, case reports, meta-analyses, and reviews were also excluded.
The initial screening involved evaluating study titles and abstracts based on the inclusion criteria before proceeding to a comprehensive review of the full text. Two researchers independently conducted data extraction, and any disagreements were resolved through mutual consultation or discussion with a third expert. Extracted data encompassed details such as authorship, publication year, gender, CPP and non-CPP group size and age, features utilized, classifier employed, optimal classifier, and results (specificity, sensitivity, and AUC). In cases where additional information was required, corresponding authors were contacted.
The evaluation of the risk of bias in individual studies was conducted employing the Quality Assessment of Diagnostic Accuracy Studies-2 (QUADAS-2) checklist (25). Independent reviewers assessed each article according to these criteria, and any disparities were resolved through discussion.
Stata software (version 23; Stata Corporation, College Station, Texas, USA) was utilized for both graphical representation and calculations. Pooled sensitivity (SEN), pooled specificity (SPE), positive likelihood ratio (LR+), negative likelihood ratio (LR-), diagnostic odds ratio (DOR) with a 95% confidence interval (CI), summary receiver operating characteristic (SROC) curve, and area under the curve (AUC) were computed to evaluate the accuracy of clinical, hormonal (laboratory) and imaging data-based ML models in diagnosing CPP. A binary generalized linear mixture model was employed for pooling. Heterogeneity was assessed using chi-square and Cochran Q tests, with I2 > 50% indicating substantial heterogeneity. Subgroup analyses and meta-regression analyses were carried out to investigate potential sources of heterogeneity. Publication bias was assessed using Deeks’ funnel plot asymmetry test, with significance set at P < 0.05.
Through database searches, a total of 179 articles were initially identified. Subsequently, 95 duplicate articles were excluded, followed by the exclusion of 43 articles after reviewing titles and abstracts. After a thorough full-text review, 35 additional studies were excluded, resulting in the inclusion of 6 studies for our analysis (refer to Figure 1).
Figure 1 Flowchart summarizing the study selection process. Note-Some studies were excluded for more than one reason. Irrelevant: Did not investigate the diagnostic value of ML models based on clinical, hormonal (laboratory) and imaging data for CPP. Improper design: Did not meet the selection criteria.
Table 1 presents the characteristics of the included studies. Notably, all analyzed articles were recently published, with the earliest study dating back to 2019, highlighting the relatively recent introduction of machine learning models in the context of CPP diagnosis. Examining Table 1 reveals a singular focus on girls across all the studies. The CPP group exhibited case numbers ranging from 137 to 1153, while the non-CPP group ranged from 24 to 1370 cases. It is noteworthy that among the included studies, only Zou et al. (21) reported subjects with PPP as controls. In the other studies, the non-PPP group was represented by subjects who tested normal on diagnostic tests. In Pan et al. (19), reference was made to a self-administered questionnaire administered in schools. The average age of most cases hovered around 7 years old. Furthermore, there was substantial variation in ML methods among the studies, particularly in terms of feature selection and classifier choice. The data sources, extracted from clinical, hormonal (laboratory) and imaging data, encompassed four main aspects: general features (e.g., age, height, weight, body mass index), clinical features (e.g., disease duration, breast Tanner stage, vaginal bleeding), laboratory features (e.g., 17α-hydroxyprogesterone (17α-hydroxy), adrenocorticotropic hormone (ACTH), cortisol, human chorionic gonadotropin (HCG), follicle-stimulating hormone (FSH), luteinizing hormone (LH), prolactin, estradiol (E2), total testosterone (TT)), and imaging features (e.g., bone age X-rays, pelvic ultrasound, pituitary MRI imaging features). Additionally, the classifiers used in the studies were diverse, including logistic regression (LR), support vector machine (SVM), Gaussian naive Bayes (GaussianNB), extreme gradient boosting (XGBoost), random forest (RF), and k-nearest neighbor algorithm (kNN), among others. Finally, the levels of AUC (ranging from 0.79 to 0.97), sensitivity (ranging from 0.34 to 0.96), and specificity (ranging from 0.77 to 0.93) varied across ML models, indicating the need for further pooled analysis to comprehensively assess the diagnostic value of clinical, hormonal (laboratory) and imaging data-based ML models for CPP.
Table 2 provides an overview of the risk of bias and applicability concerns identified in the included studies. The information is categorized into 14 items, distributed across four sections: patient selection, index test, reference standard, and flow and timing. While none of the studies met all the items, each study fulfilled a minimum of 10 items. Notably, high-risk items were mainly reflected in the patient selection part because the included studies were case-control studies rather than randomized controlled trials, and it is not clear whether the sample of patients enrolled is a continuous case. whereas the remaining sections indicated a low risk of bias.
In our study, the pooled sensitivity and specificity of clinical, hormonal (laboratory) and imaging data-based ML models for diagnosing for CPP were 0.82 (95% CI 0.62-0.93) and 0.85 (95% CI 0.80–0.90), respectively (Figure 2). SROC curves showed that the accuracy of the AUC was 0.90 (Figure 3). The closer the AUC is to 1, the better the diagnosis test. Based on these findings, clinical, hormonal (laboratory) and imaging data-based ML models has good diagnostic value for CPP and exhibits high sensitivity and specificity. Theoretically, the higher the positive likelihood ratio, the better the diagnostic test is in correctly identifying the true disease. The lower the negative likelihood ratio, the better the ability of the diagnostic test to exclude a disease. As noted in Figure 4, clinical, hormonal (laboratory) and imaging data-based ML models had a high positive likelihood ratio (6) and a low negative likelihood ratio (0.21), revealing that clinical, hormonal (laboratory) and imaging data-based ML models exhibited an excellent ability to confirm or exclude CPP.
Figure 2 Forest plots of the sensitivity (A) and specificity (B) of ML models based on clinical, hormonal (laboratory) and imaging data for the diagnosis of CPP. Note-The dots correspond to the individual studies included in this analysis, and both sides of the line represent the 95% confidence interval. The narrower the line is, the greater the accuracy of the study and the greater the weight. The diamond corresponds to the pooled result. The intermediate vertical line represents an invalid line. Q statistic test card square value (chi-square), degree of freedom (df), p-values and I2 statistic test results (Inconsistency (I- square)) correspond to heterogeneity test results. The Q test was used to assess heterogeneity, while the I2 test was used to measure the size of heterogeneity. Heterogeneity was considered when p was less than 0.01. If I2<25%, no heterogeneity was noted. If the value of I2 was between 25% and 50%, the degree of heterogeneity was considered to be small. If the value of I2 was between 50% and 75%, heterogeneity was noted. If I2>75%, large heterogeneity was noted.
Figure 3 Hierarchical summary SROC plots of ML models based on clinical, hormonal (laboratory) and imaging data for the diagnosis of CPP. The ellipse represents 95% CI for this estimate. Numbers correspond to enrolled studies.
Figure 4 Fagan nomogram of ML models based on clinical, hormonal (laboratory) and imaging data for the diagnosis of CPP.
We assessed publication bias using Deeks’ regression test of asymmetry (t = 1.17; P = 0.31) (refer to Figure 5). Examination of Deeks’ funnel plots for clinical, hormonal (laboratory) and imaging data-based ML models indicated the absence of publication bias (P > 0.05).
Figure 5 Deeks’ funnel plot asymmetry test for publication bias Note-Numbers correspond to enrolled studies.
Significant heterogeneity was observed among the studies (I² = 99.37%, 95% CI 99.20-99.54). To identify the source of heterogeneity, subgroup analyses and meta-regression analysis were conducted (refer to Table 3). The primary contributor to heterogeneity appeared to be the variations in features and classifiers. Meta-regression analysis further emphasized that differences in features and classifiers were the key sources of heterogeneity across these studies. Results from the subgroup analysis revealed that: studies incorporating image features exhibited higher sensitivity and specificity compared to those that did not include image features (P < 0.05). Studies employing the LR classifier demonstrated higher sensitivity and specificity in diagnosing CPP compared to those using the XGBoost classifier (P < 0.05). However, studies utilizing the RF classifier displayed higher sensitivity and specificity in diagnosing CPP compared to those opting for the LR model (P < 0.05).
Table 3 Univariate and multivariate meta-regression analyses for identifying covariates to explain heterogeneity among studies on clinical, hormonal (laboratory) and imaging data-based ML models for the diagnosis of CPP.
To the best of our knowledge, this is the first meta-analysis in existing research on ML models for diagnosing CPP based on clinical, hormonal (laboratory) and imaging data. Our meta-analysis reveals that ML models utilizing clinical, hormonal (laboratory) and imaging data for CPP diagnosis demonstrate high AUC, sensitivity, and specificity, indicating significant diagnostic value. This suggests that the application of ML models in diagnosing CPP holds promising potential. The development of this technology is attributed to the advancements in AI in the medical field. ML models based on clinical, hormonal (laboratory) and imaging data utilize clinical, hormonal (laboratory) and imaging data as inputs for ML algorithms, creating classifiers that rapidly identify CPP. This provides a new perspective for an objective, swift, and intelligent diagnosis of CPP.
In the studies we included, despite the use of different ML models for diagnosing CPP, the steps involved in ML technology were similar, primarily encompassing three stages: feature selection, feature extraction, and decision-making through a classifier. On the one hand, feature selection is a critical component of ML technology. The selection of features primarily derives from clinical, hormonal (laboratory) and imaging data, such as age, gender, height, weight, breast development, vaginal bleeding, LH, FSH, E2, TT, bone age, pelvic ultrasound, pituitary MRI, etc. In the studies we included, while the types and number of features varied, all studies incorporated LH and FSH as laboratory indicators, indicating their significant discriminatory value for CPP, consistent with previous research (26–28). Certainly, we aim to include as few features as possible to establish a machine learning model that can accurately diagnose CPP. Pan et al. (19) employed ML algorithms, incorporating 19 features such as age, baseline LH, baseline FSH, insulin-like growth factor-1 (IGF-1), growth hormone (GH), etc., to construct a predictive model for CPP diagnosis. They achieved an AUC range of 0.88 to 0.90, with sensitivity ranging from 77.91% to 77.94% and specificity from 84.32% to 87.66%. Huynh et al. (17) also developed six classical ML diagnostic models for girls suspected of having CPP, including 14 clinical indicators such as baseline LH, baseline FSH, uterine volume, etc. The best-performing model, the RF model, demonstrated an AUC of 0.972, sensitivity of 96.6%, and specificity of 89.3%. It can be observed that compared to the study by Pan et al., Huynh et al. achieved improved diagnostic efficiency while reducing the number of included features. Our meta-analysis does not provide definitive recommendations for the development of an optimal feature combination. In high-dimensional spaces, there is typically no method superior to others. However, our subgroup analysis results indicate that studies incorporating imaging features tended to have higher sensitivity and specificity in diagnosing CPP compared to studies that did not include imaging features. Imaging features primarily include BA, pelvic ultrasound, pituitary MRI, etc. Pan et al. (19) demonstrated that using only laboratory data is challenging to effectively differentiate between CPP and non-CPP (sensitivity of 66.23%). However, once combined with pelvic ultrasound, the model showed a significant improvement in the area under the ROC curve, increasing from 0.42 to 0.63. Therefore, it is suggested that in the development of machine learning diagnostic models for CPP, efforts should be made to include imaging features whenever possible. On the other hand, classifier selection is another crucial aspect of machine learning technology. The included studies encompassed various types of classifiers, including XGBoost, RF, GBM, LR, SVM, naïve Bayesian, kNN, etc. In the studies we incorporated, apart from Chen et al. (20) and Zou et al. (21), where LR was the optimal model, the optimal models in other studies varied, including XGBoost, RF, GBM, etc. Our subgroup analysis results indicate that studies opting for the RF classifier tended to have higher specificity and sensitivity compared to studies choosing other types of classifiers. Therefore, it is recommended that in the development of machine learning diagnostic models for CPP, efforts should be made to include the RF classifier whenever possible.
We hope that the results of the aforementioned meta-analysis will catalyze further advancements in this field. Despite the challenging nature of diagnosing CPP using ML models based on clinical, hormonal (laboratory) and imaging data, and being in its early stages, our meta-analysis suggests that these technologies have shown promising results. Future research should focus on developing ML models that incorporate fewer features, provide accurate diagnoses, and are interpretable. We further observed that Zou et al. (21) employed the SHAP global interpretability technique, and Huynh et al. (17) utilized the LIME analysis method to interpret their machine learning models. This interpretability is crucial for clinical decision-making as it enhances healthcare professionals’ trust in the model outputs and helps them better understand the reasons behind specific diagnostic decisions. This breaks the curse of machine learning models being perceived as black boxes. Additionally, in clinical practice, these machine learning models can be developed into a small software tool to facilitate their application in healthcare settings. In resource-limited medical environments, primary care physicians can use the model’s results to make preliminary management decisions. If, based on the model’s assessment, a patient is classified into the CPP group, it suggests a reason to suspect early development, warranting appropriate medical intervention.
We assessed the quality of the included studies using the following four components: patient selection, index test, reference standard, and flow and timing, among which the high risk was mainly reflected in the patient selection component. A potential explanation for this finding is that the inclusion criteria for diagnostic trials are often based on case-control trials rather than randomized controlled trials, and patients included in the study only reported the time period without specifying whether they were consecutive cases. In the included studies, ML methods and the gold standard method were performed without knowing the results of each other, and the GnRH stimulation test was used as the gold standard. Therefore, selection bias was minimal, and the results were reliable, indicating that these factors were associated with a low risk of bias. Additionally, the Deeks funnel plot showed no publication bias in these studies.
In the included studies, a significant amount of heterogeneity was observed, and the causes of this heterogeneity were multifaceted. Differences in feature selection, imaging methods, and classifiers were the main contributors to this heterogeneity. However, in another sense, this heterogeneity might be valuable when developing clinically deployable ML models. To accurately reflect the actual performance of ML models in diagnosing CPP, these models must be tested on different features, imaging methods, and classifiers to identify the model with the best performance. In addition, differences in control groups also serve as a primary source of heterogeneity. Among the included studies, only Zou et al. (21) reported subjects with PPP as controls. In the other studies, the non-PPP group was represented by subjects who tested normal on diagnostic tests. In Pan et al. (19), reference was made to a self-administered questionnaire administered in schools. These factors may all contribute to the heterogeneity.
Our study has some limitations. Our study has several limitations. Firstly, all participants were recruited from China and Taiwan, which may restrict the generalizability of our findings as environmental factors, ethnicity, and medical conditions can vary significantly across different regions. Therefore, caution should be exercised when extrapolating our results to other populations, and future research should consider geographical, ethnic, and medical variations to enhance the applicability of our findings. Secondly, distinguishing between rapidly progressing and slowly progressing forms of central precocious puberty (CPP) poses a significant challenge for pediatricians, and our meta-analysis failed to provide clear guidance in this regard. Thus, future research should prioritize addressing this issue by exploring methods to differentiate between different presentations of CPP and guiding personalized treatment strategies. Lastly, the substantial heterogeneity observed among the included studies may impact the evaluation of the diagnostic performance of machine learning (ML) models. Given the limited number of studies and the ongoing development of ML technology, conducting further research in this field is crucial for accurately assessing the utility of ML in diagnosing CPP.
In summary, our meta-analysis findings demonstrate promisingly high accuracy of machine learning models based on clinical, hormonal (laboratory), and imaging data in diagnosing CPP, exhibiting elevated levels of sensitivity, specificity, and AUC values. Nevertheless, we acknowledge certain limitations regarding the generalizability of our results due to the exclusive inclusion of participants from China and Taiwan. Given the disparities in environmental factors, ethnicity, and healthcare conditions across different regions, caution should be exercised when extrapolating these findings to other populations. Additionally, while distinguishing between rapid and slow progression forms of CPP remains a challenge for pediatricians, we are optimistic about future research prospects. Future studies will continue to address these challenges to further enhance the applicability and reliability of the results, and explore more effective means of differentiating between various types of CPP, thereby providing more precise guidance for appropriate treatment strategies.
The original contributions presented in the study are included in the article/Supplementary Material. Further inquiries can be directed to the corresponding author.
YC: Data curation, Writing – original draft. XH: Writing – original draft, Methodology. LT: Writing – review & editing, Funding acquisition.
The author(s) declare that financial support was received for the research, authorship, and/or publication of this article. Chongqing Municipal Education Commission. The study is supported by the Chongqing Municipal Education Commission(No.KJQN202300428).
The authors declare that the research was conducted in the absence of any commercial or financial relationships that could be construed as a potential conflict of interest.
All claims expressed in this article are solely those of the authors and do not necessarily represent those of their affiliated organizations, or those of the publisher, the editors and the reviewers. Any product that may be evaluated in this article, or claim that may be made by its manufacturer, is not guaranteed or endorsed by the publisher.
The Supplementary Material for this article can be found online at: https://www.frontiersin.org/articles/10.3389/fendo.2024.1353023/full#supplementary-material
1. Cleemann Wang A, Hagen CP, Johannsen TH, Madsen AG, Hartvig Cleemann L, Christiansen P, et al. Differentiation of idiopathic central precocious puberty from premature thelarche using principal component analysis. J Clin Endocrinol Metab. (2024) 109(2):370–9. doi: 10.1210/clinem/dgad535
2. Arcari AJ, Freire AV, Ballerini MG, Escobar ME, Diaz Marsiglia YM, Bergada I, et al. Prevalence of polycystic ovarian syndrome in girls with a history of idiopathic central precocious puberty. Horm Res Paediatr. (2023), 1–6. doi: 10.1159/000531264
3. Xie LL, Yang Y, Xiong XY, Yang L, Wu X, Zhang DG. A clinical study of girls with idiopathic central precocious puberty and psychological behavior problems. Clin Pediatr (Phila). (2023) 62:914–8. doi: 10.1177/00099228221149551
4. Soriano-Guillén L, Corripio R, Labarta JI, Cañete R, Castro-Feijóo L, Espino R, et al. Central precocious puberty in children living in Spain: incidence, prevalence, and influence of adoption and immigration. J Clin Endocrinol Metab. (2010) 95:4305–13. doi: 10.1210/jc.2010-1025
5. Bräuner EV, Busch AS, Eckert-Lind C, Koch T, Hickey M, Juul A. Trends in the incidence of central precocious puberty and normal variant puberty among children in Denmark, 1998 to 2017. JAMA Netw Open. (2020) 3:e2015665. doi: 10.1001/jamanetworkopen.2020.15665
6. Kang S, Park MJ, Kim JM, Yuk JS, Kim SH. Ongoing increasing trends in central precocious puberty incidence among Korean boys and girls from 2008 to 2020. PloS One. (2023) 18:e0283510. doi: 10.1371/journal.pone.0283510
7. Cassio A, Marescotti G, Aversa T, Salerno M, Tornese G, Stancampiano M, et al. Central precocious puberty in italian boys: data from a large nationwide cohort. J Clin Endocrinol Metab. (2024). doi: 10.1210/clinem/dgae035
8. Knific T, Lazarevic M, Zibert J, Obolnar N, Aleksovska N, Suput Omladic J, et al. Final adult height in children with central precocious puberty - a retrospective study. Front Endocrinol (Lausanne). (2022) 13:1008474. doi: 10.3389/fendo.2022.1008474
9. Soriano-Guillen L, Argente J. Central precocious puberty, functional and tumor-related. Best Pract Res Clin Endocrinol Metab. (2019) 33:101262. doi: 10.1016/j.beem.2019.01.003
10. Ab Rahim SN, Omar J, Tuan Ismail TS. Gonadotropin-releasing hormone stimulation test and diagnostic cutoff in precocious puberty: a mini review. Ann Pediatr Endocrinol Metab. (2020) 25:152–5. doi: 10.6065/apem.2040004.002
11. Ozalkak S, Cetinkaya S, Budak FC, Erdeve SS, Aycan Z. Evaluation of gonadotropin responses and response times according to two different cut-off values in luteinizing hormone releasing hormone stimulation test in girls. Indian J Endocrinol Metab. (2020) 24:410–5. doi: 10.4103/ijem.IJEM_314_20
12. Dura-Trave T, Gallinas-Victoriano F, Malumbres-Chacon M, Ahmed-Mohamed L, Guindulain MJC, Berrade-Zubiri S. Clinical data and basal gonadotropins in the diagnosis of central precocious puberty in girls. Endocr Connect. (2021) 10:164–70. doi: 10.1530/EC-20-0651
13. Yuan B, Pi YL, Zhang YN, Xing P, Chong HM, Zhang HF. A diagnostic model of idiopathic central precocious puberty based on transrectal pelvic ultrasound and basal gonadotropin levels. J Int Med Res. (2020) 48:300060520935278. doi: 10.1177/0300060520935278
14. Calcaterra V, Klersy C, Vinci F, Regalbuto C, Dobbiani G, Montalbano C, et al. Rapid progressive central precocious puberty: diagnostic and predictive value of basal sex hormone levels and pelvic ultrasound. J Pediatr Endocrinol Metab. (2020) 33:785–91. doi: 10.1515/jpem-2019-0577
15. Martin DD, Meister K, Schweizer R, Ranke MB, Thodberg HH, Binder G. Validation of automatic bone age rating in children with precocious and early puberty. J Pediatr Endocrinol Metab. (2011) 24:1009–14. doi: 10.1515/JPEM.2011.420
16. Pan L, Liu G, Mao X, Liang H. Machine learning identifies girls with central precocious puberty based on multisource data. JAMIA Open. (2020) 3:567–75. doi: 10.1093/jamiaopen/ooaa063
17. Huynh QTV, Le NQK, Huang SY, Ho BT, Vu TH, Pham HTM, et al. Development and validation of clinical diagnostic model for girls with central precocious puberty: machine-learning approaches. PloS One. (2022) 17:e0261965. doi: 10.1371/journal.pone.0261965
18. Pang B, Wang Q, Yang M, Xue M, Zhang Y, Deng X, et al. Identification and optimization of contributing factors for precocious puberty by machine/deep learning methods in Chinese girls. Front Endocrinol (Lausanne). (2022) 13:892005. doi: 10.3389/fendo.2022.892005
19. Pan L, Liu G, Mao X, Li H, Zhang J, Liang H, et al. Development of prediction models using machine learning algorithms for girls with suspected central precocious puberty: retrospective study. JMIR Med Inform. (2019) 7:e11728. doi: 10.2196/11728
20. Chen YS, Liu CF, Sung MI, Lin SJ, Tsai WH. Machine learning approach for prediction of the test results of gonadotropin-releasing hormone stimulation: model building and implementation. Diagnostics (Basel). (2023) 13. doi: 10.3390/diagnostics13091550
21. Zou P, Zhang L, Zhang R, Wang C, Lin X, Lai C, et al. Development and validation of a combined MRI radiomics, imaging and clinical parameter-based machine learning model for identifying idiopathic central precocious puberty in girls. J magnetic resonance Imaging JMRI. (2023) 58:1977–87. doi: 10.1002/jmri.28709
22. Cumpston M, Li T, Page MJ, Chandler J, Welch VA, Higgins JP, et al. Updated guidance for trusted systematic reviews: a new edition of the Cochrane Handbook for Systematic Reviews of Interventions. Cochrane Database Syst Rev. (2019) 10:ED000142. doi: 10.1002/14651858
23. McInnes MDF, Moher D, Thombs BD, McGrath TA, Bossuyt PM, P-DTAG, et al. Preferred reporting items for a systematic review and meta-analysis of diagnostic test accuracy studies: the PRISMA-DTA statement. JAMA. (2018) 319:388–96. doi: 10.1001/jama.2017.19163
24. Methley AM, Campbell S, Chew-Graham C, McNally R, Cheraghi-Sohi S. PICO, PICOS and SPIDER: a comparison study of specificity and sensitivity in three search tools for qualitative systematic reviews. BMC Health Serv Res. (2014) 14:579. doi: 10.1186/s12913-014-0579-0
25. Qu YJ, Yang ZR, Sun F, Zhan SY. [Risk on bias assessment: (6) A Revised Tool for the Quality Assessment on Diagnostic Accuracy Studies (QUADAS-2)]. Zhonghua Liu Xing Bing Xue Za Zhi. (2018) 39:524–31.
26. Cao R, Liu J, Fu P, Zhou Y, Li Z, Liu P. The diagnostic utility of the basal luteinizing hormone level and single 60-minute post gnRH agonist stimulation test for idiopathic central precocious puberty in girls. Front Endocrinol (Lausanne). (2021) 12:713880. doi: 10.3389/fendo.2021.713880
27. Chotipakornkul N, Onsoi W, Numsriskulrat N, Aroonparkmongkol S, Supornsilchai V, Srilanchakon K. The utilization of basal luteinizing hormone in combination with the basal luteinizing hormone and follicle-stimulating hormone ratio as a diagnostic tool for central precocious puberty in girls. Ann Pediatr Endocrinol Metab. (2023) 28:138–43. doi: 10.6065/apem.2346072.036
Keywords: machine learning, central precocious puberty, meta-analysis, ML, CPP
Citation: Chen Y, Huang X and Tian L (2024) Meta-analysis of machine learning models for the diagnosis of central precocious puberty based on clinical, hormonal (laboratory) and imaging data. Front. Endocrinol. 15:1353023. doi: 10.3389/fendo.2024.1353023
Received: 09 December 2023; Accepted: 12 March 2024;
Published: 25 March 2024.
Edited by:
Gianluca Tornese, Institute for Maternal and Child Health Burlo Garofolo (IRCCS), ItalyReviewed by:
Giorgio Radetti, Ospedale di Bolzano, ItalyCopyright © 2024 Chen, Huang and Tian. This is an open-access article distributed under the terms of the Creative Commons Attribution License (CC BY). The use, distribution or reproduction in other forums is permitted, provided the original author(s) and the copyright owner(s) are credited and that the original publication in this journal is cited, in accordance with accepted academic practice. No use, distribution or reproduction is permitted which does not comply with these terms.
*Correspondence: Lu Tian, MTk1NzkyMTc5QHFxLmNvbQ==
†These authors have contributed equally to this work
Disclaimer: All claims expressed in this article are solely those of the authors and do not necessarily represent those of their affiliated organizations, or those of the publisher, the editors and the reviewers. Any product that may be evaluated in this article or claim that may be made by its manufacturer is not guaranteed or endorsed by the publisher.
Research integrity at Frontiers
Learn more about the work of our research integrity team to safeguard the quality of each article we publish.