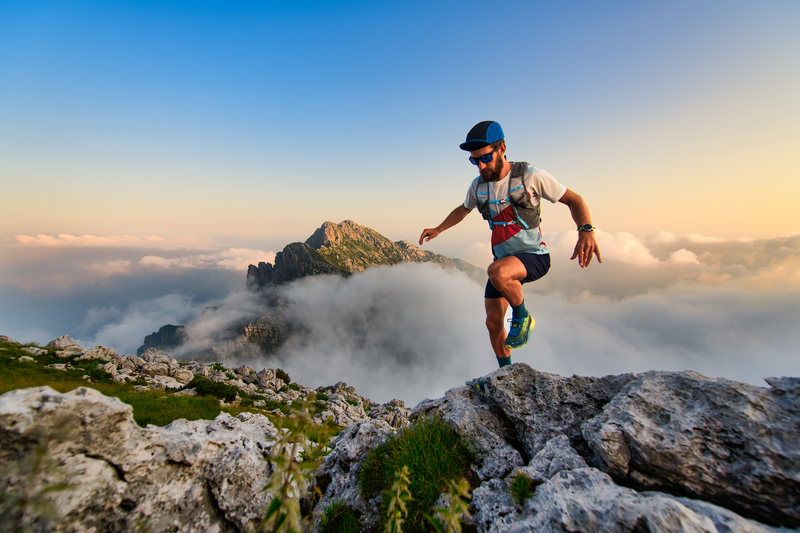
94% of researchers rate our articles as excellent or good
Learn more about the work of our research integrity team to safeguard the quality of each article we publish.
Find out more
ORIGINAL RESEARCH article
Front. Endocrinol. , 25 November 2024
Sec. Clinical Diabetes
Volume 15 - 2024 | https://doi.org/10.3389/fendo.2024.1408053
This article is part of the Research Topic Insights in Sleep and Metabolism View all 3 articles
Objectives: To investigate the causal relationship between type 2 diabetes mellitus (T2DM, exposure) and isolated REM sleep behavior disorder (iRBD, outcome).
Methods: Genome-wide association study (GWAS) data for iRBD comprised 9,447 samples, including 1,061 iRBD cases from the International RBD Study Group. Initially, we performed linkage disequilibrium score regression (LDSC) to explore the genetic correlation between T2DM and iRBD. Then the two-sample univariate MR (UVMR) analysis was conducted to examine the effects of T2DM and blood sugar metabolism-related factors on iRBD. Subsequently, we applied multivariable MR (MVMR) methods to further adjust for confounders. Lastly, we executed a network MR analysis, with cytokines and immune cell characteristics as potential mediators, aiming to investigate indirect effect of T2DM on iRBD.
Results: Results from LDSC suggest a genetic correlation between T2DM and iRBD (rg=0.306, P=0.029). UVMR analysis indicates that both T2DM (Odds Ratio [95% Confidence Interval] = 1.19 [1.03, 1.37], P = 0.017) and high blood glucose levels (1.55 [1.04, 2.30], P = 0.032) are risk factors for iRBD. Even after adjusting for confounders in MVMR, the association between T2DM and iRBD remains robust. Finally, results from network MR analysis suggest that T2DM may indirectly promote the development of iRBD by reducing levels of Stromal Cell-Derived Factor 2 in circulation and by increasing BAFF-receptor expression in IgD- CD38- B cells.
Conclusions: T2DM may promote the onset of iRBD by influencing immune-inflammatory responses. Our findings provide valuable insights and directions for understanding the pathogenesis of iRBD, identifying high-risk groups, and discovering new therapeutic targets.
Isolated rapid eye movement (REM) sleep behavior disorder (iRBD), a parasomnia characterized by the lack of muscle atonia and abnormal behaviors during REM sleep, significantly impacts sleep quality and poses safety and health risks to both patients and their bed partners (1). Critically, iRBD serves as a known precursor for severe neurodegenerative α-synucleinopathies such as Parkinson’s disease (PD), multiple system atrophy (MSA), and dementia with Lewy bodies (DLB). Studies have shown that upwards of 80% of those with iRBD are likely to develop PD, DLB, or MSA within a span of about 16 years (2–4). These afflictions are characterized by their severely debilitating impact, poor prognoses, and substantial reduction in the quality of life and longevity of sufferers. Consequently, a deep understanding of the risk factors and underlying mechanisms of iRBD is crucial for effective management and potentially for the prevention of its progression to more grave neurodegenerative diseases.
Numerous studies have established a strong link between Type 2 Diabetes Mellitus (T2DM) and alpha-synucleinopathies. Observational studies identify T2DM as an independent risk factor for PD (5–9) and DLB (10, 11), exacerbating symptoms and accelerating disease progression. Furthermore, pancreatic tissue samples from both T2DM and α-synucleinopathy patients show similar pathological changes, notably the deposition of phosphorylated α-synuclein in pancreatic β cells, suggesting parallel pathological mechanisms in these diseases (12). In vitro experiments have demonstrated that the characteristic deposit protein in diabetes, islet amyloid polypeptide (IAPP), interacts with alpha-synuclein, promoting its aggregation (13). Animal studies further indicate that the hyperglycemic state and insulin resistance in diabetes may trigger immune-inflammatory responses, facilitating the accumulation of α-synuclein and the onset of neurodegenerative changes (14, 15). These findings collectively support the role of T2DM in promoting α-synucleinopathies.
However, research on the relationship between T2DM and the precursor stage of alpha-synucleinopathies, iRBD, remains scarce and controversial. Using linkage disequilibrium score regression (LDSC), Krohn’s team identified a genetic correlation between T2DM and iRBD (rg = 0.66, se = 0.23, p = 0.0047) (16). In a community-based observational study, Wong and colleagues pinpointed diabetes as an independent risk factor for people with probable RBD (OR[95%] =1.37[1.04-1.82]) (17), while research from José Haba’s team refuted this association (18). It is pivotal to recognize that observational studies are vulnerable to confounding bias and reverse causality.
Mendelian Randomization (MR) is a statistical tool employing genetic variants as instruments to deduce the causal impact of exposures on outcomes (19). This method leverages the fact that the allocation of genetic variants at conception is impervious to environmental or lifestyle influences, making MR estimates more resistant to confounding factors and reverse causation biases (20). Moreover, genetic variant data are typically derived from genome-wide association studies (GWAS), known for their large sample sizes, consequently enhancing MR’s capability to robustly detect causal relationships (21).
To reduce biases from confounders and reverse causality, and to provide robust genetic evidence on the relationship between T2DM and iRBD, we conducted a two-sample MR analysis, considering T2DM as the exposure and iRBD as the outcome. Given that T2DM can lead to a range of complications through immune-inflammatory responses (22, 23), and abnormalities in cytokine levels and immune cell characteristics are observed in iRBD patients (24–27), we conducted a network MR analysis. This analysis focused on immune-related circulating protein levels, inflammatory factors, and immune cell characteristics as potential mediators to investigate the underlying immune-inflammatory mechanisms by which T2DM may lead to iRBD.
Figure 1 delineates the methodology of our two-sample MR analysis, exploring the link between T2DM and iRBD. Initially, we employed LDSC to assess the genetic correlation between these two conditions. Following this, with T2DM as the exposure factor and iRBD as the outcome factor, we conducted a univariate Mendelian Randomization analysis (UVMR) to investigate their gene-driven causal relationship. To further corroborate this result, UVMR was again utilized, to examine the connection between three glucose metabolic traits (blood glucose, glycated hemoglobin, and fasting insulin levels) and iRBD. The next phase of our study involved a multivariable MR (MVMR) method, integrating confounders like Body Mass Index (BMI), smoking habits, and educational level, to validate the robustness and consistency of the T2DM-iRBD association. Lastly, our analysis extended to a network MR approach with cytokines, immune-related circulating protein levels, and immune cell characteristics as potential mediators, delving into the underlying mechanisms of T2DM leading to iRBD. Our methodology adheres rigorously to the STROBE-MR guidelines (28).
Figure 1. The flowchart of study design. BMI, body mass index; T2DM, type 2 diabetes mellitus; iRBD, isolated REM sleep behavior disorder; MR, Mendelian randomization; IV, instrumental variables; IVW, Inverse variance weighted; MLA, Maximum likelihood analysis.
To strengthen causality inference and reduce bias from small sample sizes, we carefully selected the most comprehensive GWAS datasets for T2DM and iRBD available from the GWAS Catalogue (https://www.ebi.ac.uk/gwas/), focusing on individuals of European descent. The T2DM GWAS dataset, specifically, was derived from a meta-analysis conducted by Angli Xue’s team, combining data from MAGIC, DIAGRAM, and GERA, encompassing a total of 659,316 participants (29). For the outcome data, we chose a GWAS dataset of an iRBD cohort (1,061 cases and 8,386 controls) compiled by the International RBD Study Group (16). The iRBD cases were diagnosed using the International Classification of Sleep Disorders (2nd or 3rd Edition), inclusive of video polysomnography assessments. To ensure better comparability between the case and control groups, principal components analysis was used to adjust for population substructure, accounting for variables like gender and age. Data concerning metabolic traits related to blood sugar, confounders (BMI, education years, smoking status) and potential mediators (91 plasma cytokines, 106 immune-related circulating proteins, 731 immune cell characteristics) were sourced from the IEU online database (https://gwas.mrcieu.ac.uk/) and the GWAS Catalogue database (Table 1). All the aforementioned GWAS data are publicly accessible. Importantly, we retrieved exposure and outcome data from independent samples to avoid bias stemming from sample overlap.
In our study, we employed single-variate LDSC to estimate the heritability of both T2DM and iRBD. Additionally, to clarify the genetic correlations between these two conditions, we utilized the bivariate LDSC method. (30–34).
Genetic variants were selected as instrumental variables based on strong associations with the exposure (P < 5 × 10-8). To mitigate reverse causation bias, we excluded SNPs highly associated with the outcome () (35). To quantify the proportion of variance in exposure, the clue of single nucleotide polymorphisms (SNPs) was estimated by effect estimates (beta) and allele frequencies (EAF): (36). The instrument strength was estimated using the F-statistic: , where N represents the sample size and k is the number of instrumental variables (37). Only the SNP of F-statistic > 10 could be included in this study. A minor allele frequency (MAF) ≥0.01. We clumped independent SNPs based on European ancestry reference data (1000 Genomes Project, r²>0.001, genomic region=10,000 kb). Summary statistics were harmonized on alleles positively associated with exposures. Ambiguous palindromic SNPs (A/T, C/G) with a MAF>0.3 were excluded (38). To fulfill the independence assumption for MR analysis, SNPs associated with various factors, including BMI, smoking, alcohol consumption, years of schooling, occupation, head injury history, olfactory dysfunction, antidepressant drug usage, and antipsychotics usage (16–18, 39–41), were excluded based on the PhenoScanner query results. SNPs associated with confounding factors were displayed in Supplementary Table S4. The statistical power of the Mendelian randomization analysis was computed using the tool available at https://shiny.cnsgenomics.com/mRnd/ (42).
The primary analysis utilized the inverse-variance weighted (IVW) method (43), benchmarked against other MR methods robust to pleiotropy, including the maximum likelihood method (44), weighted median (45), weighted mode (46), MR-Egger regression (47) simple mode (48) and the MR-PRESSO (49). We initially investigated the genetically driven causal relationship between T2DM as the exposure and iRBD as the outcome. Subsequently, to validate our findings, we delved into the causal connections between three key blood glucose metabolic traits—blood glucose levels, glycated hemoglobin levels, and fasting insulin levels—and iRBD. Although MR effectively reduces confounding bias, it does not completely eliminate its influence. If the instrumental variables used in the analysis are associated with known risk or protective factors for the outcome, they violate the second assumption of MR, leading to biased results. Therefore, we employed MVMR to adjust for instrumental variables closely related to BMI, years of education, and smoking habits (16–18, 39–41), in order to assess the robustness of the relationship between T2DM and iRBD.
To investigate the potential immune-inflammatory mechanisms by which T2DM affects iRBD, we selected 91 plasma cytokines, 106 immune-related circulating proteins, and 731 immune cell characteristics as candidate mediators and conducted a network MR analysis—an MR-based mediation analysis. We sequentially calculated the total effect of T2DM on iRBD, the direct effect of T2DM on the mediators, and the direct effect of the mediators on iRBD. The magnitude of the mediation effect was estimated using the product-of-coefficients method, and the confidence interval was determined using the Delta method (50).
Cochran’s Q statistic assessed heterogeneity, serving as a potential indicator of pleiotropy in IVW estimators. Horizontal pleiotropy was identified through the p-value for the intercept in MR-Egger (47) and the global test in MR-PRESSO (49). MR-PRESSO also pinpointed outliers, prompting a reanalysis of all MR methods after outlier SNP exclusion. Leave-one-out analyses determined if a single SNP unduly influenced estimates.
All statistical tests were two-sided, a P value of less than 0.05 was considered statistically significant. All above statistical analyses were conducted by using the R-statistical software (version 4.3.1) with related R packages.
Supplementary Table S2 details the selected genetic instruments for our MR analyses. These genetic variants were strongly associated with their respective exposures, explaining a variance that ranged from 0.26% to 21.81%. Importantly, all instrumental variables had F-statistics exceeding 10, indicating that our results are unlikely to be affected by weak instrument bias. In terms of statistical power within our MR analysis, except for the exposure factor of fasting insulin with a power value of 0.86, all other exposures had power values of 1, signifying robust statistical power to detect potential causal relationships.
Bivariable LDSC analysis indicated a genetic correlation between T2DM and iRBD (rg = 0.306, P = 0.029;, Table 2; Supplementary Table S1). The UVMR analysis corroborated a gene-driven causal relationship, with an odds ratio (OR) of 1.19 (95% CI: 1.03-1.37; P = 0.017, Figures 2A, B). Subsequent secondary analyses using various methods supported these findings: maximum likelihood yielded an OR of 1.19 (95% CI: 1.03, 1.38, P = 0.016), weighted median produced an OR of 1.27 (95% CI: 1.02, 1.57, P = 0.031), simple mode estimated an OR of 1.76 (95% CI: 1.05, 2.96, P = 0.035), and MR-PRESSO calculated an OR of 1.19 (95% CI: 1.04, 1.27, P = 0.016). Notably, all analyses excluded the instrumental variable ‘rs13239186’ due to its strong association with smoking as identified in the PhenoScanner database, to avoid confounding bias (Supplementary Tables S2, 4). Sensitivity analyses, using Cochran’s Q statistic, showed no heterogeneity in the MR results. Furthermore, MR-Egger’s intercept and MR-PRESSO’s global test indicated no evidence of horizontal pleiotropy. MR-PRESSO also detected no outlier effects (Supplementary Table S5). Leave-one-out analysis suggested that the results were not driven by any single SNP (Figure 2C; Supplementary Table S6). Reverse Mendelian randomization did not support the existence of reverse causation (Supplementary Table S7).
Figure 2. The estimated causal effects of T2DM on iRBD using MR analysis. (A) presents the results using a scatter plot. (B) presents the results using a forest plot. (C) presents the results of leave-one-out analysis. Effect sizes are represented as odds ratios (OR), and the horizontal bars represent 95% confidence intervals (CIs). Statistically significant P-values are indicated with superscript letters and asterisks (*).
In our UVMR analysis examining the relationship between three blood sugar metabolism factors and iRBD, we discovered that elevated blood glucose levels increase the risk of iRBD, with an OR of 1.55 (95% CI: 1.04, 2.30, P = 0.03). Secondary analyses using Maximum likelihood and MR-PRESSO corroborated these findings, with both methods indicating an OR of 1.55 (95% CI: 1.04, 2.31, P = 0.03 for Maximum likelihood; 1.55, 95% CI: 1.04, 2.30, P = 0.03 for MR-PRESSO). Significantly, our analyses systematically excluded the instrumental variables ‘rs8047587’ and ‘rs34402524’, due to their strong associations with alcohol consumption and years of education, respectively (Supplementary Table S4). There was no evidence to suggest a causal link between HbA1c or fasting insulin levels and iRBD. Sensitivity analysis indicated heterogeneity in the relationship between HbA1c levels and iRBD (Q-value=0.01), and potential horizontal pleiotropy (Global P-value =0.01). The remaining analyses showed no disturbances from heterogeneity, horizontal pleiotropy, or outliers (Table 3; Supplementary Tables S8, 9).
Study conclusions were supported by weighted-median estimation, weighted-mode, and MR-Egger methods (Supplementary Table S2). Cochran’s Q statistic indicated no significant heterogeneity in SNP effects (p > 0.05). No evidence of potential horizontal pleiotropy was detected for the eight factors identified (p > 0. 05). To further assess the robustness of the results, we conducted MR-PRESSO tests on the included SNP loci. In addition, a leave-one-out sensitivity analysis was conducted to assess the influence of each SNP on the overall causal relationship. The results demonstrated that systematically removing individual SNPs and repeating the MR analysis did not reveal significant differences in the observed causal relationships (Supplementary Tables S3–5).
The results of the MVMR suggest that T2DM remains an independent risk factor for iRBD, even after adjusting for potential confounders such as years of education, smoking status, and BMI. This was demonstrated through various analytical methods: IVW-OR at 1.230 (95% CI: 1.031, 1.467, P = 0.022), Lasso-OR at 1.176 (95% CI: 1.003, 1.379, P = 0.046), MR-Egger-OR at 1.208 (95% CI: 1.011, 1.442, P = 0.037), and Weighted Median-OR at 1.278 (95% CI: 1.008, 1.619, P = 0.043) as shown in Figure 3 and Supplementary Table S10. Sensitivity analysis revealed no horizontal pleiotropy in the multivariable MR analyses, as indicated by MR-Egger regression (MR Egger intercept P value = 0.092).
Figure 3. The impact of T2DM on iRBD in the MVMR analysis after adjusting for confounders. Effect sizes are represented as odds ratios (OR), and the horizontal bars represent 95% confidence intervals (CIs). Statistically significant P-values are indicated with superscript letters and asterisks (*).
In our network MR analysis (Table 4), we identified significant associations: T2DM was observed to decrease plasma levels of Stromal Cell-Derived Factor 2 (SDF-2) (P=8.03E-03), with lower SDF-2 levels elevating the risk of iRBD (P=1.75E-03). The mediation effect of SDF-2 in the T2DM-iRBD relationship accounted for 7.03% (95% CI 0.11%–7.70%). Furthermore, T2DM was associated with an increase in BAFF-receptor (BAFF-R) on IgD- CD38- B cells (P = 3.08E-03), a characteristic that also heightened the risk of iRBD (P = 7.43E-04). The mediation effect of this immune characteristic in the T2DM-iRBD causal pathway was 11.17% (95% CI 6.22%–11.65%). Notably, none of the network MR analyses indicated the presence of heterogeneity and horizontal pleiotropy (Supplementary Tables S11, 12).
Table 4. Mediation effect of immune and inflammatory factors in the association between T2DM and iRBD.
In this pioneering MR study, we identified a significant association between elevated blood glucose levels, T2DM, and increased risk of iRBD. This link remained strong even after adjusting for BMI, smoking, and education. Mediation analysis highlighted an indirect pathway where T2DM contributes to iRBD by increasing BAFF-R on IgD- CD38- B cells and reducing plasma SDF-2 levels. These findings offer valuable insights into screening high-risk populations for iRBD and understanding its pathogenesis, representing a notable advance in the field.
Numerous observational studies have consistently demonstrated that T2DM is an independent risk factor for alpha-synucleinopathies such as PD (5–9) and DLB (10, 11), contributing to increased incidence, exacerbated symptoms, and accelerated disease progression. Valbuena’s team, through examination of pancreatic tissue slides, identified similar pathological changes in pancreatic β cells of both T2DM and alpha-synucleinopathy patients, notably the presence of phosphorylated alpha-synuclein (12). More significantly, Horvath’s group discovered in vitro that islet amyloid polypeptide (IAPP), a primary pathogenic deposit in T2DM patients, promotes the accumulation of alpha-synuclein amyloid (13). Furthermore, basic experimental findings indicate that insulin resistance and hyperglycemia can trigger immune-inflammatory responses, thereby fostering the deposition of alpha-synuclein and the onset of neurodegenerative changes (14, 15). These studies collectively highlight a profound connection between T2DM and alpha-synucleinopathies.
Research on the link between T2DM and iRBD, a precursor to alpha-synucleinopathies, is limited and presents conflicting findings. Wong et al. found diabetes to be an independent risk factor for probable RBD in a community-based observational study (17). In contrast, a Swiss study reported no such association (18). It’s crucial to acknowledge that the conclusions of observational studies may be skewed by confounding factors and reverse causality. Our research identified a genome-wide genetic correlation between T2DM and iRBD. Furthermore, we found that T2DM increases the incidence of iRBD, a conclusion supported by multiple models and robust against heterogeneity, pleiotropy, and outliers. The absence of a similar conclusion in the Swiss observational study may be attributed to its limited statistical power due to a small sample size, encompassing only 24 iRBD cases.
The exact mechanisms by which T2DM fosters the development of iRBD remain elusive. Previous research has demonstrated that high blood glucose can induce PD by modulating oxidative stress, and triggering inflammation reaction (51–57). Yi-Qing Lv and his team found that hyperglycemia leads to severe neuroinflammation and accelerates α-synuclein deposition in the central nervous system of mice (15). Similarly, Yan Sun and colleagues found a close link between fasting plasma glucose levels and the increased accumulation and phosphorylation of α-synuclein in the cortex, pre-commissural putamen, and dopaminergic neurons in the substantia nigra of T2DM monkeys’ brains (58). Based on these findings, we speculate that in T2DM patients, hyperglycemia-induced neuroinflammation and α-synuclein deposition may affect brain regions that inhibit spinal motor neurons during REM sleep (59), potentially triggering iRBD (59).
Our network MR analysis reveals that T2DM may promote the onset of iRBD by increasing BAFF-R on IgD- CD38- B cells and reducing circulating SDF-2 levels. Previous research has shown that T2DM, through hyperglycemia and insulin resistance, elevates pro-inflammatory cytokines and chemokines, triggering abnormal immune cell activation and tissue infiltration, leading to various complications (22, 23). Similar disturbances in cytokine levels and immune cell characteristics have been observed in iRBD patients (24–27). SDF-2, a small protein located in the endoplasmic reticulum, is closely linked with endoplasmic reticulum stress regulation (60). While its precise role remains unclear, low tissue expression of SDF-2 is associated with poor prognosis in various tumors. Additionally, SDF-2 can enhance nitric oxide (NO) release by interacting with endothelial nitric oxide synthase (eNOS), reducing the incidence of several cardiovascular diseases (61). Consequently, SDF-2 may have a protective role in the onset and progression of various diseases, including iRBD. The specific mechanisms by which T2DM regulates SDF-2 and its impact on iRBD, however, require further investigation. BAFFR, encoded by the TNFRSF13C gene and serving as a critical pro-survival receptor in cells (62, 63), is notably upregulated in T2DM within IgD- CD38- B cells. This upregulation boosts both the survival and proliferation of these cells, implying a potential role in iRBD development through an unidentified toxic immune response. Elucidating the specific mechanisms of this process demands more comprehensive research.
In summary, our study reveals the causal relationship between T2DM and iRBD, as well as the underlying immune-inflammatory mechanisms. This finding offers new insights for screening high-risk populations for iRBD and has significant public health implications. It is essential to regularly screen T2DM patients for sleep disorders, particularly when they frequently experience abnormal dreams or unusual behaviors during sleep, as this may indicate the comorbidity of T2DM and iRBD. More importantly, future research should explore whether T2DM-targeted treatments, such as hypoglycemic agents, insulin preparations, and GLP-1 receptor agonists, could serve as potential treatments for iRBD. Additionally, it is worth investigating whether interventions targeting specific inflammatory proteins and immune cells in diabetic patients can reduce the risk of iRBD.
Our study has several limitations. First, although we employed various analytical methods to validate our findings, larger iRBD GWAS sample sizes are still needed for MR analysis to further confirm the reliability of the results. A larger sample size would not only improve the precision of genotype-phenotype association estimates in GWAS data but also help reduce selection bias caused by specific population characteristics. Second, all GWAS data used in this study were derived from European populations, which limits the generalizability of our conclusions to other ethnic groups. Future research should include cross-ethnic MR analyses to further assess the robustness of our findings. Third, as our conclusions are primarily based on MR analysis, and previous cross-sectional studies on the relationship between T2DM and iRBD have shown inconsistent results, multi-center cohort studies are needed to provide real-world evidence supporting these conclusions. Fourth, it is important to acknowledge that MR analysis reflects the relationship between genetically driven exposures and outcomes, but genetic factors only account for part of the exposure, highlighting the inherent limitations of MR analysis. Finally, while this study has preliminarily identified the potential immune-inflammatory mechanisms by which T2DM may lead to iRBD, we have not explored the cell-cell interactions and related signaling pathways in depth. With the development of advanced analytical platforms (64–66), future research should focus on further mechanistic insights.
Our study indicates that T2DM and hyperglycemic conditions are risk factors for iRBD. Additionally, T2DM may indirectly contribute to the onset of iRBD by upregulating BAFFR expression in IgD- CD38- B cells and reducing circulating SDF-2 levels. Our findings offer new directions for screening high-risk populations for iRBD. The comorbidity of T2DM and iRBD, as well as their potential immune-inflammatory mechanisms, warrant further investigation in future studies.
The original contributions presented in the study are included in the article/Supplementary Material. Further inquiries can be directed to the corresponding authors.
F-JL: Conceptualization, Writing – review & editing, Formal analysis, Investigation, Methodology, Software, Writing – original draft. R-YZ: Conceptualization, Formal analysis, Investigation, Methodology, Software, Writing – original draft, Writing – review & editing, Data curation, Project administration, Supervision, Visualization. J-YL: Visualization, Writing – review & editing, Validation. Y-NL: Validation, Visualization, Writing – review & editing. Z-XZ: Validation, Visualization, Writing – review & editing. JZ: Writing – review & editing, Conceptualization, Supervision.
The author(s) declare that no financial support was received for the research, authorship, and/or publication of this article.
We extend our gratitude to everyone who participated in and collaborated on this research.
The authors declare that the research was conducted in the absence of any commercial or financial relationships that could be construed as a potential conflict of interest.
All claims expressed in this article are solely those of the authors and do not necessarily represent those of their affiliated organizations, or those of the publisher, the editors and the reviewers. Any product that may be evaluated in this article, or claim that may be made by its manufacturer, is not guaranteed or endorsed by the publisher.
The Supplementary Material for this article can be found online at: https://www.frontiersin.org/articles/10.3389/fendo.2024.1408053/full#supplementary-material
1. Dauvilliers Y, Schenck CH, Postuma RB, Iranzo A, Luppi PH, Plazzi G, et al. REM sleep behaviour disorder. Nat Rev Dis Primers. (2018) 4:19. doi: 10.1038/s41572-018-0016-5
2. Schenck CH, Boeve BF, Mahowald MW. Delayed emergence of a parkinsonian disorder or dementia in 81% of older men initially diagnosed with idiopathic rapid eye movement sleep behavior disorder: a 16-year update on a previously reported series. Sleep Med. (2013) 14:744–8. doi: 10.1016/j.sleep.2012.10.009
3. Iranzo A, Fernández-Arcos A, Tolosa E, Serradell M, Molinuevo JL, Valldeoriola F, et al. Neurodegenerative disorder risk in idiopathic REM sleep behavior disorder: study in 174 patients. PloS One. (2014) 9:e89741. doi: 10.1371/journal.pone.0089741
4. Postuma RB, Gagnon JF, Bertrand JA, Génier Marchand D, Montplaisir JY. Parkinson risk in idiopathic REM sleep behavior disorder: preparing for neuroprotective trials. Neurology. (2015) 84:1104–13. doi: 10.1212/WNL.0000000000001364
5. De Pablo-Fernandez E, Goldacre R, Pakpoor J, Noyce AJ, Warner TT. Association between diabetes and subsequent Parkinson disease: A record-linkage cohort study. Neurology. (2018) 91:e139–42. doi: 10.1212/WNL.0000000000005771
6. Chung SJ, Jeon S, Yoo HS, Kim G, Oh JS, Kim JS, et al. Detrimental effect of type 2 diabetes mellitus in a large case series of Parkinson's disease. Parkinsonism Relat Disord. (2019) 64:54–9. doi: 10.1016/j.parkreldis.2018.08.023
7. Yue X, Li H, Yan H, Zhang P, Chang L, Li T. Risk of parkinson disease in diabetes mellitus: an updated meta-analysis of population-based cohort studies. Med (Baltimore). (2016) 95:e3549. doi: 10.1097/MD.0000000000003549
8. Ray Dorsey E, Elbaz A, Nichols E, Abbasi N, Abd-Allah F, Abdelalim A, et al. Global, regional, and national burden of Parkinson's disease 1990-2016: a systematic analysis for the Global Burden of Disease Study 2016. Lancet Neurol. (2018) 17:939–53. doi: 10.1016/S1474-4422(18)30295-3
9. Marras C, Beck JC, Bower JH, Roberts E, Ritz B, Ross GW, et al. Prevalence of Parkinson's disease across North America. NPJ Parkinsons Dis. (2018) 4:21. doi: 10.1038/s41531-018-0058-0
10. Cheng CK, Tsao YC, Su YC, Sung FC, Tai HC, Kung WM. Metabolic risk factors of alzheimer's disease, dementia with lewy bodies, and normal elderly: A population-based study. Behav Neurol. (2018) 2018:8312346. doi: 10.1155/2018/8312346
11. Javanshiri K, Haglund M, Englund E. Cardiovascular disease, diabetes mellitus, and hypertension in lewy body disease: A comparison with other dementia disorders. J Alzheimers Dis. (2019) 71:851–9. doi: 10.3233/JAD-190485
12. Martinez-Valbuena I, Amat-Villegas I, Valenti-Azcarate R, Carmona-Abellan MDM, Marcilla I, Tuñon MT, et al. Interaction of amyloidogenic proteins in pancreatic β cells from subjects with synucleinopathies. Acta Neuropathol. (2018) 135:877–86. doi: 10.1007/s00401-018-1832-0
13. Horvath I, Wittung-Stafshede P. Cross-talk between amyloidogenic proteins in type-2 diabetes and Parkinson's disease. Proc Natl Acad Sci U.S.A. (2016) 113:12473–7. doi: 10.1073/pnas.1610371113
14. Kim DS, Choi HI, Wang Y, Luo Y, Hoffer BJ, Greig NH. A new treatment strategy for parkinson's disease through the gut-brain axis: the glucagon-like peptide-1 receptor pathway. Cell Transplant. (2017) 26:1560–71. doi: 10.1177/0963689717721234
15. Lv YQ, Yuan L, Sun Y, Dou HW, Su JH, Hou ZP, et al. Long-term hyperglycemia aggravates α-synuclein aggregation and dopaminergic neuronal loss in a Parkinson's disease mouse model. Transl Neurodegener. (2022) 11:14. doi: 10.1186/s40035-022-00288-z
16. Krohn L, Heilbron K, Blauwendraat C, Reynolds RH, Yu E, Senkevich K, et al. Genome-wide association study of REM sleep behavior disorder identifies polygenic risk and brain expression effects. Nat Commun. (2022) 13:7496. doi: 10.1038/s41467-022-34732-5
17. Wong JC, Li J, Pavlova M, Chen S, Wu A, Wu S, et al. Risk factors for probable REM sleep behavior disorder: A community-based study. Neurology. (2016) 86:1306–12. doi: 10.1212/WNL.0000000000002414
18. Haba-Rubio J, Frauscher B, Marques-Vidal P, Toriel J, Tobback N, Andries D, et al. Prevalence and determinants of rapid eye movement sleep behavior disorder in the general population. Sleep. (2018) 41(2):zsx197. doi: 10.1093/sleep/zsx197
19. Smith GD, Ebrahim S. 'Mendelian randomization': can genetic epidemiology contribute to understanding environmental determinants of disease? Int J Epidemiol. (2003) 32:1–22.doi: 10.1093/ije/dyg070
20. Lawlor DA, Harbord RM, Sterne JA, Timpson N, Davey Smith G. Mendelian randomization: using genes as instruments for making causal inferences in epidemiology. Stat Med. (2008) 27:1133–63. doi: 10.1002/sim.v27:8
21. Burgess S, Scott RA, Timpson NJ, Davey Smith G, Thompson SG. Using published data in Mendelian randomization: a blueprint for efficient identification of causal risk factors. Eur J Epidemiol. (2015) 30:543–52. doi: 10.1007/s10654-015-0011-z
22. Akbari M, Hassan-Zadeh V. The inflammatory effect of epigenetic factors and modifications in type 2 diabetes. Inflammopharmacology. (2020) 28:345–62. doi: 10.1007/s10787-019-00663-9
23. Daryabor G, Atashzar MR, Kabelitz D, Meri S, Kalantar K. The effects of type 2 diabetes mellitus on organ metabolism and the immune system. Front Immunol. (2020) 11:1582. doi: 10.3389/fimmu.2020.01582
24. Li Y, Yang Y, Zhao A, Luo N, Niu M, Kang W, et al. Parkinson's disease peripheral immune biomarker profile: a multicentre, cross-sectional and longitudinal study. J Neuroinflamm. (2022) 19:116. doi: 10.1186/s12974-022-02481-3
25. Stokholm MG, Iranzo A, Østergaard K, Serradell M, Otto M, Svendsen KB, et al. Assessment of neuroinflammation in patients with idiopathic rapid-eye-movement sleep behaviour disorder: a case-control study. Lancet Neurol. (2017) 16:789–96. doi: 10.1016/S1474-4422(17)30173-4
26. Farmen K, Nissen SK, Stokholm MG, Iranzo A, Østergaard K, Serradell M, et al. Monocyte markers correlate with immune and neuronal brain changes in REM sleep behavior disorder. Proc Natl Acad Sci U.S.A. (2021) 118. doi: 10.1073/pnas.2020858118
27. Zheng Y, Li Y, Cai H, Kou W, Yang C, Li S, et al. Alterations of peripheral lymphocyte subsets in isolated rapid eye movement sleep behavior disorder. Mov Disord. (2024) 39:1179–89. doi: 10.1002/mds.29798
28. Skrivankova VW, Richmond RC, Woolf BAR, Davies NM, Swanson SA, Vanderweele TJ, et al. Strengthening the reporting of observational studies in epidemiology using mendelian randomisation (STROBE-MR): explanation and elaboration. Bmj. (2021) 375:n2233. doi: 10.1136/bmj.n2233
29. Xue A, Wu Y, Zhu Z, Zhang F, Kemper KE, Zheng Z, et al. Genome-wide association analyses identify 143 risk variants and putative regulatory mechanisms for type 2 diabetes. Nat Commun. (2018) 9:2941. doi: 10.1038/s41467-018-04951-w
30. Bulik-Sullivan BK, Loh PR, Finucane HK, Ripke S, Yang J, Patterson N, et al. LD Score regression distinguishes confounding from polygenicity in genome-wide association studies. Nat Genet. (2015) 47:291–5. doi: 10.1038/ng.3211
31. Bulik-Sullivan B, Finucane HK, Anttila V, Gusev A, Day FR, Loh PR, et al. An atlas of genetic correlations across human diseases and traits. Nat Genet. (2015) 47:1236–41. doi: 10.1038/ng.3406
32. Finucane HK, Bulik-Sullivan B, Gusev A, Trynka G, Reshef Y, Loh PR, et al. Partitioning heritability by functional annotation using genome-wide association summary statistics. Nat Genet. (2015) 47:1228–35. doi: 10.1038/ng.3404
33. Gazal S, Finucane HK, Furlotte NA, Loh PR, Palamara PF, Liu X, et al. Linkage disequilibrium-dependent architecture of human complex traits shows action of negative selection. Nat Genet. (2017) 49:1421–7. doi: 10.1038/ng.3954
34. Zheng J, Erzurumluoglu AM, Elsworth BL, Kemp JP, Howe L, Haycock PC, et al. LD Hub: a centralized database and web interface to perform LD score regression that maximizes the potential of summary level GWAS data for SNP heritability and genetic correlation analysis. Bioinformatics. (2017) 33:272–9. doi: 10.1093/bioinformatics/btw613
35. Park S. A causal and inverse relationship between plant-based diet intake and in a two-sample mendelian randomization study. Foods. (2023) 12. doi: 10.3390/foods12030545
36. Codd V, Nelson CP, Albrecht E, Mangino M, Deelen J, Buxton JL, et al. Identification of seven loci affecting mean telomere length and their association with disease. Nat Genet. (2013) 45:427e1–2. doi: 10.1038/ng.2528
37. Palmer TM, Lawlor DA, Harbord RM, Sheehan NA, Tobias JH, Timpson NJ, et al. Using multiple genetic variants as instrumental variables for modifiable risk factors. Stat Methods Med Res. (2012) 21:223–42. doi: 10.1177/0962280210394459
38. Jiang M, Yan W, Zhang Y, Lu Z, Lu T, Zhang D, et al. Phosphodiesterase and psychiatric disorders: a two-sample Mendelian randomization study. J Transl Med. (2023) 21:560. doi: 10.1186/s12967-023-04368-0
39. Kang SH, Yoon IY, Lee SD, Han JW, Kim TH, Kim KW. REM sleep behavior disorder in the Korean elderly population: prevalence and clinical characteristics. Sleep. (2013) 36:1147–52. doi: 10.5665/sleep.2874
40. Postuma RB, Gagnon JF, Tuineaig M, Bertrand JA, Latreille V, Desjardins C, et al. Antidepressants and REM sleep behavior disorder: isolated side effect or neurodegenerative signal? Sleep. (2013) 36:1579–85. doi: 10.5665/sleep.3102
41. Frauscher B, Jennum P, Ju YE, Postuma RB, Arnulf I, Cochen De Cock V, et al. Comorbidity and medication in REM sleep behavior disorder: a multicenter case-control study. Neurology. (2014) 82:1076–9. doi: 10.1212/WNL.0000000000000247
42. Brion MJ, Shakhbazov K, Visscher PM. Calculating statistical power in Mendelian randomization studies. Int J Epidemiol. (2013) 42:1497–501. doi: 10.1093/ije/dyt179
43. Burgess S, Bowden J, Fall T, Ingelsson E, Thompson SG. Sensitivity analyses for robust causal inference from mendelian randomization analyses with multiple genetic variants. Epidemiology. (2017) 28:30–42. doi: 10.1097/EDE.0000000000000559
44. Xue H, Shen X, Pan W. Constrained maximum likelihood-based Mendelian randomization robust to both correlated and uncorrelated pleiotropic effects. Am J Hum Genet. (2021) 108:1251–69. doi: 10.1016/j.ajhg.2021.05.014
45. Bowden J, Davey Smith G, Haycock PC, Burgess S. Consistent estimation in mendelian randomization with some invalid instruments using a weighted median estimator. Genet Epidemiol. (2016) 40:304–14. doi: 10.1002/gepi.2016.40.issue-4
46. Hartwig FP, Davey Smith G, Bowden J. Robust inference in summary data Mendelian randomization via the zero modal pleiotropy assumption. Int J Epidemiol. (2017) 46:1985–98. doi: 10.1093/ije/dyx102
47. Bowden J, Davey Smith G, Burgess S. Mendelian randomization with invalid instruments: effect estimation and bias detection through Egger regression. Int J Epidemiol. (2015) 44:512–25. doi: 10.1093/ije/dyv080
48. Xu J, Zhang S, Tian Y, Si H, Zeng Y, Wu Y, et al. Genetic causal association between iron status and osteoarthritis: A two-sample mendelian randomization. Nutrients. (2022) 14. doi: 10.3390/nu14183683
49. Verbanck M, Chen CY, Neale B, Do R. Detection of widespread horizontal pleiotropy in causal relationships inferred from Mendelian randomization between complex traits and diseases. Nat Genet. (2018) 50:693–8. doi: 10.1038/s41588-018-0099-7
50. Carter AR, Gill D, Davies NM, Taylor AE, Tillmann T, Vaucher J, et al. Understanding the consequences of education inequality on cardiovascular disease: mendelian randomisation study. Bmj. (2019) 365:l1855. doi: 10.1136/bmj.l1855
51. Bassil F, Canron MH, Vital A, Bezard E, Li Y, Greig NH, et al. Insulin resistance and exendin-4 treatment for multiple system atrophy. Brain. (2017) 140:1420–36. doi: 10.1093/brain/awx044
52. Wen L, Duffy A. Factors influencing the gut microbiota, inflammation, and type 2 diabetes. J Nutr. (2017) 147:1468s–75s. doi: 10.3945/jn.116.240754
53. Elmarakby AA, Sullivan JC. Relationship between oxidative stress and inflammatory cytokines in diabetic nephropathy. Cardiovasc Ther. (2012) 30:49–59. doi: 10.1111/j.1755-5922.2010.00218.x
54. Van Dyken P, Lacoste B. Impact of metabolic syndrome on neuroinflammation and the blood-brain barrier. Front Neurosci. (2018) 12:930. doi: 10.3389/fnins.2018.00930
55. Nayak S, Soon SQ, Kunjal R, Ramadoo R, Baptiste O, Persad J, et al. Relationship between adiponectin, inflammatory markers and obesity in type 2 diabetic and non-diabetic Trinidadians. Arch Physiol Biochem. (2009) 115:28–33. doi: 10.1080/13813450902758785
56. Thundyil J, Lim KL. DAMPs and neurodegeneration. Ageing Res Rev. (2015) 24:17–28. doi: 10.1016/j.arr.2014.11.003
57. Hickman S, Izzy S, Sen P, Morsett L, El Khoury J. Microglia in neurodegeneration. Nat Neurosci. (2018) 21:1359–69. doi: 10.1038/s41593-018-0242-x
58. Sun Y, Guo C, Yuan L, Li W, Wang ZY, Yue F, et al. Cynomolgus monkeys with spontaneous type-2-diabetes-mellitus-like pathology develop alpha-synuclein alterations reminiscent of prodromal parkinson's disease and related diseases. Front Neurosci. (2020) 14:63. doi: 10.3389/fnins.2020.00063
59. Boeve BF. REM sleep behavior disorder: Updated review of the core features, the REM sleep behavior disorder-neurodegenerative disease association, evolving concepts, controversies, and future directions. Ann N Y Acad Sci. (2010) 1184:15–54. doi: 10.1111/j.1749-6632.2009.05115.x
60. Gong W, Martin TA, Sanders AJ, Jiang A, Sun P, Jiang WG. Location, function and role of stromal cell−derived factors and possible implications in cancer (Review). Int J Mol Med. (2021) 47:435–43. doi: 10.3892/ijmm.2020.4811
61. Siragusa M, Fröhlich F, Park EJ, Schleicher M, Walther TC, Sessa WC. Stromal cell-derived factor 2 is critical for Hsp90-dependent eNOS activation. Sci Signal. (2015) 8:ra81. doi: 10.1126/scisignal.aaa2819
62. Pieper K, Grimbacher B, Eibel H. B-cell biology and development. J Allergy Clin Immunol. (2013) 131:959–71. doi: 10.1016/j.jaci.2013.01.046
63. Smulski CR, Eibel H. BAFF and BAFF-receptor in B cell selection and survival. Front Immunol. (2018) 9:2285. doi: 10.3389/fimmu.2018.02285
64. Lai H, Li Y, Zhang H, Hu J, Liao J, Su Y, et al. exoRBase 2.0: an atlas of mRNA, lncRNA and circRNA in extracellular vesicles from human biofluids. Nucleic Acids Res. (2022) 50:D118–d128. doi: 10.1093/nar/gkab1085
65. Li Y, He X, Li Q, Lai H, Zhang H, Hu Z, et al. EV-origin: Enumerating the tissue-cellular origin of circulating extracellular vesicles using exLR profile. Comput Struct Biotechnol J. (2020) 18:2851–9. doi: 10.1016/j.csbj.2020.10.002
Keywords: type 2 diabetes mellitus, isolated REM sleep behavior disorder, Mendelian randomization, genetic correlation, causal association
Citation: Zhang R-Y, Li J-Y, Liu Y-N, Zhang Z-X, Zhao J and Li F-J (2024) The causal relationship between type 2 diabetes mellitus and isolated REM sleep behavior disorder: results from multivariable and network Mendelian randomization analysis. Front. Endocrinol. 15:1408053. doi: 10.3389/fendo.2024.1408053
Received: 27 March 2024; Accepted: 25 October 2024;
Published: 25 November 2024.
Edited by:
Daniele Corbo, University of Brescia, ItalyReviewed by:
Yuchen Li, Stanford University, United StatesCopyright © 2024 Zhang, Li, Liu, Zhang, Zhao and Li. This is an open-access article distributed under the terms of the Creative Commons Attribution License (CC BY). The use, distribution or reproduction in other forums is permitted, provided the original author(s) and the copyright owner(s) are credited and that the original publication in this journal is cited, in accordance with accepted academic practice. No use, distribution or reproduction is permitted which does not comply with these terms.
*Correspondence: Ru-Yu Zhang, emhhbmdydXl1MDEyOUAxNjMuY29t; Fu-Jia Li, bGlmajIzQG1haWwyLnN5c3UuZWR1LmNu
Disclaimer: All claims expressed in this article are solely those of the authors and do not necessarily represent those of their affiliated organizations, or those of the publisher, the editors and the reviewers. Any product that may be evaluated in this article or claim that may be made by its manufacturer is not guaranteed or endorsed by the publisher.
Research integrity at Frontiers
Learn more about the work of our research integrity team to safeguard the quality of each article we publish.