- 1School of Stomatology, Changsha Medical University, Changsha, China
- 2School of Life Sciences, Beijing University of Chinese Medicine, Beijing, China
- 3The Hunan Provincial Key Laboratory of the TCM Agricultural Biogenomics, Changsha Medical University, Changsha, China
- 4Hunan key laboratory of the research and development of novel pharmaceutical preparations, School of Pharmaceutical Science, Changsha Medical University, Changsha, China
Background: Previous research on the association between risk factors and gestational diabetes mellitus (GDM) primarily comprises observational studies with inconclusive results. The objective of this study is to investigate the causal relationship between 108 traits and GDM by employing a two-sample Mendelian randomization (MR) analysis to identify potential risk factors of GDM.
Methods: We conducted MR analyses to explore the relationships between traits and GDM. The genome-wide association studies (GWAS) for traits were primarily based on data from the UK Biobank (UKBB), while the GWAS for GDM utilized data from FinnGen. We employed a false discovery rate (FDR) of 5% to account for multiple comparisons.
Results: The inverse-variance weighted (IVW) method indicated that the genetically predicted 24 risk factors were significantly associated with GDM, such as “Forced expiratory volume in 1-second (FEV1)” (OR=0.76; 95% CI: 0.63, 0.92), “Forced vital capacity (FVC)” (OR=0.74; 95% CI: 0.64, 0.87), “Usual walking pace” (OR=0.19; 95% CI: 0.09, 0.39), “Sex hormone-binding globulin (SHBG)” (OR=0.86; 95% CI: 0.78, 0.94). The sensitivity analyses with MR-Egger and weighted median methods indicated consistent results for most of the trats.
Conclusion: Our study has uncovered a significant causal relationship between 24 risk factors and GDM. These results offer a new theoretical foundation for preventing or mitigating the risks associated with GDM.
Introduction
Gestational diabetes mellitus (GDM) is the occurrence of hyperglycemia of varying severity due to impaired glucose tolerance, which is first diagnosed during pregnancy (1, 2). According to the International Diabetes Federation, it is estimated that GDM will affect one out of every six live newborns worldwide in 2019 (3). GDM significantly impacts both maternal and fetal health, as indicated by previous studies (4). Furthermore, GDM not only worsens short-term adverse outcomes during pregnancy (5–7) but also increases the long-term likelihood of developing type 2 diabetes mellitus (T2DM) among women (8, 9), which has been linked to various complications (10–16).
Observational research has identified multiple associations between various risk factors and GDM (17–19). However, these investigations are susceptible to confounding variables that may influence their findings. Additionally, the casual association proposed by these observational studies may lack statistical validity due to inconsistent study designs, conflicting findings, and substantial variability across different settings. Consequently, there is inadequate evidence available within these associations to establish a direct causal link between risk factors and GDM.
In order to address the aforementioned challenges, we employed Mendelian randomization (MR) as a method to mitigate biased estimation and reverse causation in the relationship between traits and GDM. In MR analysis, single nucleotide polymorphisms (SNPs), a type of genetic variation, are utilized as instrumental variables (IVs). Statistical techniques are utilized in this approach to evaluate the presence of a causal association between exposures and outcomes. Genetic variants serve as suitable IVs due to their random distribution during meiosis. Consequently, they exhibit reduced susceptibility to confounding influences. Hence, if these genetic variants are randomly distributed within a population, the observed causal relationships between exposures and outcomes are not likely due to potential confounders such as environmental risks, lifestyle choices, or socioeconomic status (20). Thus, MR design is employed in this study to systematically investigate the causal associations between 108 traits and GDM to identify the potential risk factors of GDM.
Methods
Study design
Utilizing datasets obtained from genome-wide association studies (GWAS), we identified significant SNPs associated with 108 traits as exposure variables. These SNPs were employed as IV, and a MR analysis was conducted to evaluate the causal relationship between the 108 traits and GDM.
Data sources
To adhere to the principles of a two-sample MR design, we sourced exposure and outcome data from distinct European populations as previously described (21–23). We extracted minimally adjusted GWAS summary statistics for our variables of interest from the largest available sample. This dataset included individuals of both sexes and European or mixed ancestry. Our selection process for summary statistics of 108 exposure variables followed a previously described procedure outlined in Supplementary Figure 1 (24). While most exposure GWAS studies utilized data from the UKBB (detailed information can be found in Supplementary Table 1), the dataset for GDM, as outcome, relied on information sourced from FinnGen, a significant biomedical research initiative based in Finland.
Selection of IVs
IVs were selected for the MR analysis based on specific criteria. The criteria included a significant genetic relationship between IVs and exposure, with a P-value < 5× 10-8. Independent IVs were identified by performing clumping within a 10 Mb window and considering linkage disequilibrium (LD) with an R2 value below 0.001. Furthermore, following previous studies, only IVs with a minor allele frequency (MAF) greater than 0.01 were considered in our analysis. Palindromic SNPs were excluded from the analysis due to their intermediate allele frequencies (25). F-statistics were computed to assess the strength of the IVs; values exceeding 10 indicated reduced likelihood of weak instrument bias (refer to Supplementary Table 2) (26).
MR analysis and sensitivity analysis
The main technique employed in the MR analysis was the IVW method. Furthermore, both the weighted median technique and MR-Egger approaches were utilized. The MR-Egger intercept test was employed to evaluate the existence of horizontal pleiotropy. To address potential outliers, pleiotropy-corrected data from MR-PRESSO were incorporated. The degree of heterogeneity was examined using the Cochrane Q value. We conducted a leave-one-out sensitivity analysis to evaluate how each IV impacted causal relationships and ensure robustness of findings. The calculation of causal effects involved the use of odds ratios (ORs) along with their corresponding 95% confidence intervals (CIs). Multiple comparisons were conducted using a false discovery rate (FDR) of 5%. All MR analyses in R were conducted using the TwoSampleMR package.
Results
Out of a pool of 108 variables, SNPs were selected as IVs for potential risk factors according to predetermined inclusion and exclusion criteria. The findings were interpreted based on FDR-adjusted threshold. Using the IVW technique in MR analysis, we found significant relationships of 24 genetically predicted risk factors, such as “Apoliprotein A” (OR= 0.83; 95% CI: 0.76, 0.91), “Forced expiratory volume in 1-second (FEV1)” (OR=0.76; 95% CI: 0.63, 0.92), “Insulin-like growth factor 1 (IGF-1)” (OR=1.16; 95% CI: 1.08, 1.26) and “Usual walking pace” (OR=0.19; 95% CI: 0.09, 0.39), with GDM (Figures 1, 2, Supplementary Table 3). The F-statistics for the IVs of the 24 risk factors ranged from 28.62 to 9445.10, indicating good instrument strength (Supplementary Table 2). Except for triglycerides, we found that 23 risk factors consistently showed a significant association with GDM in the same direction when analyzed using both MR-Egger and weighted median techniques (Supplementary Table 3). The scatter plot in Figure 3 illustrated the causal relationships between all the 24 traits and GDM. The possible heterogeneity was also examined (Figure 4, Supplementary Table 4). Horizontal pleiotropy was estimated in our causality assessment based on analysis using MR-Egger technique as shown in Supplementary Table 5, and MR-PRESSO analyses indicated consistent findings after removing outlier IVs (Supplementary Table 6). The leave-one-out analysis demonstrated that no single SNP was solely responsible for the observed outcomes, as shown in Supplementary Figure 2.
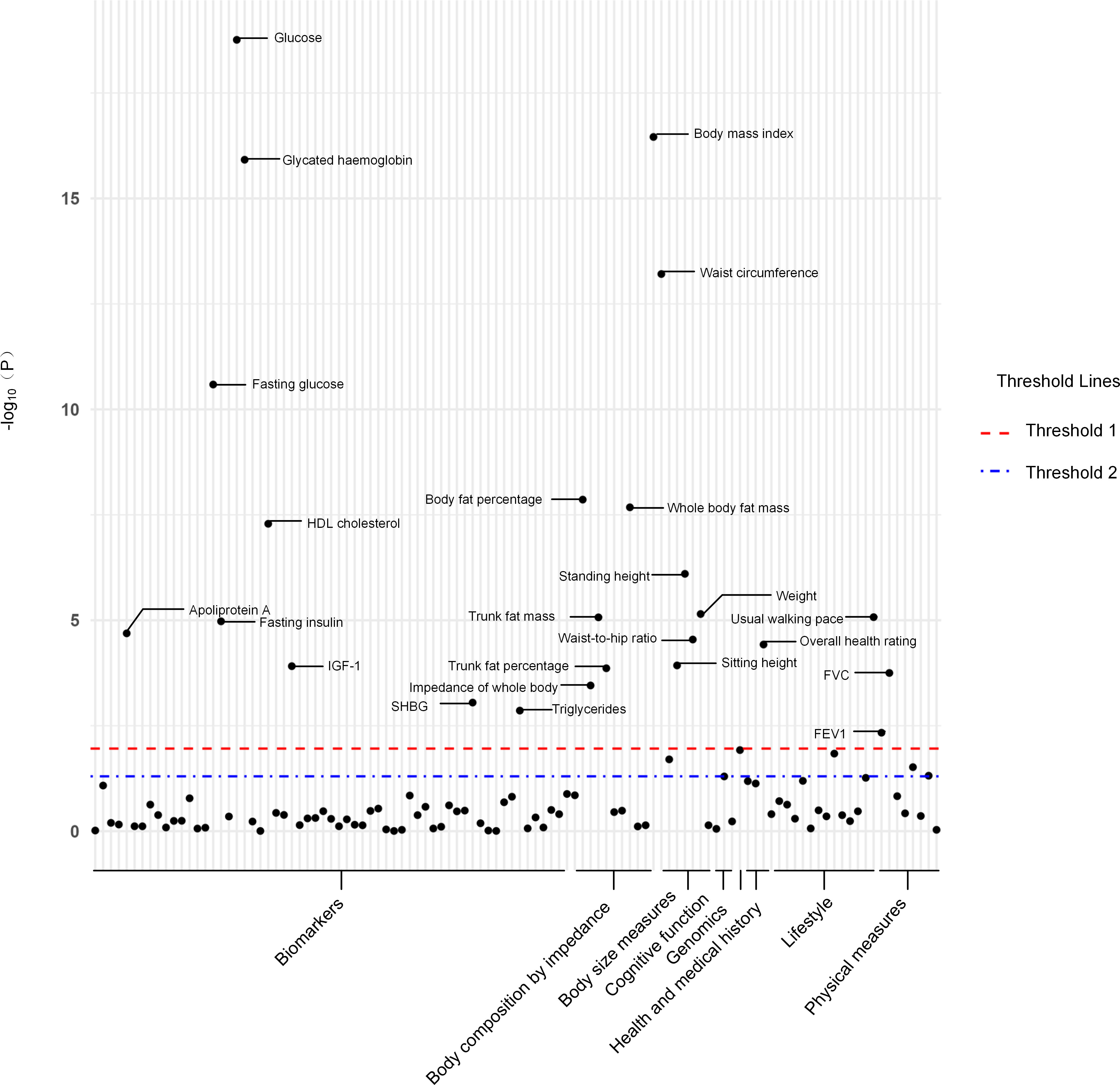
Figure 1 The P-value distribution of associations between 24 risk factors and GDM in the Mendelian randomization analysis. The red dashed line indicates the significance threshold adjusted by false discovery rate. The blue dash-dotted line indicates the suggestive significance threshold, set at P = 0.05. FVC, Forced vital capacity; FEV1, Forced expiratory volume in 1-second; HDL, High-density lipoprotein; IGF-1, Insulin-like growth factor 1; SHBG, Sex hormone-binding globulin.
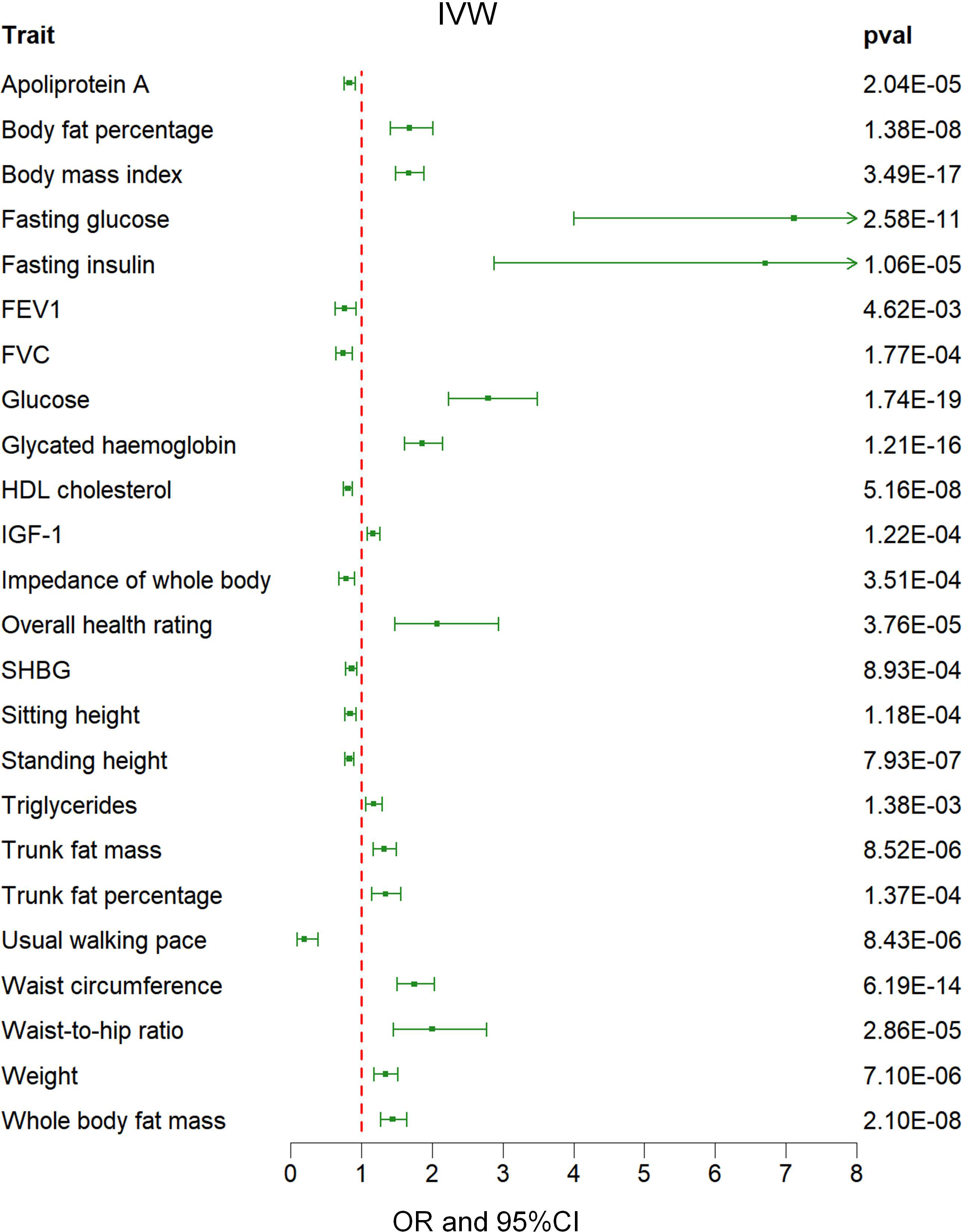
Figure 2 Associations between genetically predicted 24 risk factors and GDM examined by IVW methods. GDM, Gestational Diabetes Mellitus; IVW, inverse-variance weighted; FVC, Forced vital capacity; FEV1, Forced expiratory volume in 1-second; HDL, High-density lipoprotein; IGF-1, Insulin-like growth factor 1; SHBG, Sex hormone-binding globulin; OR, odds ratio; CI, confidence interval.
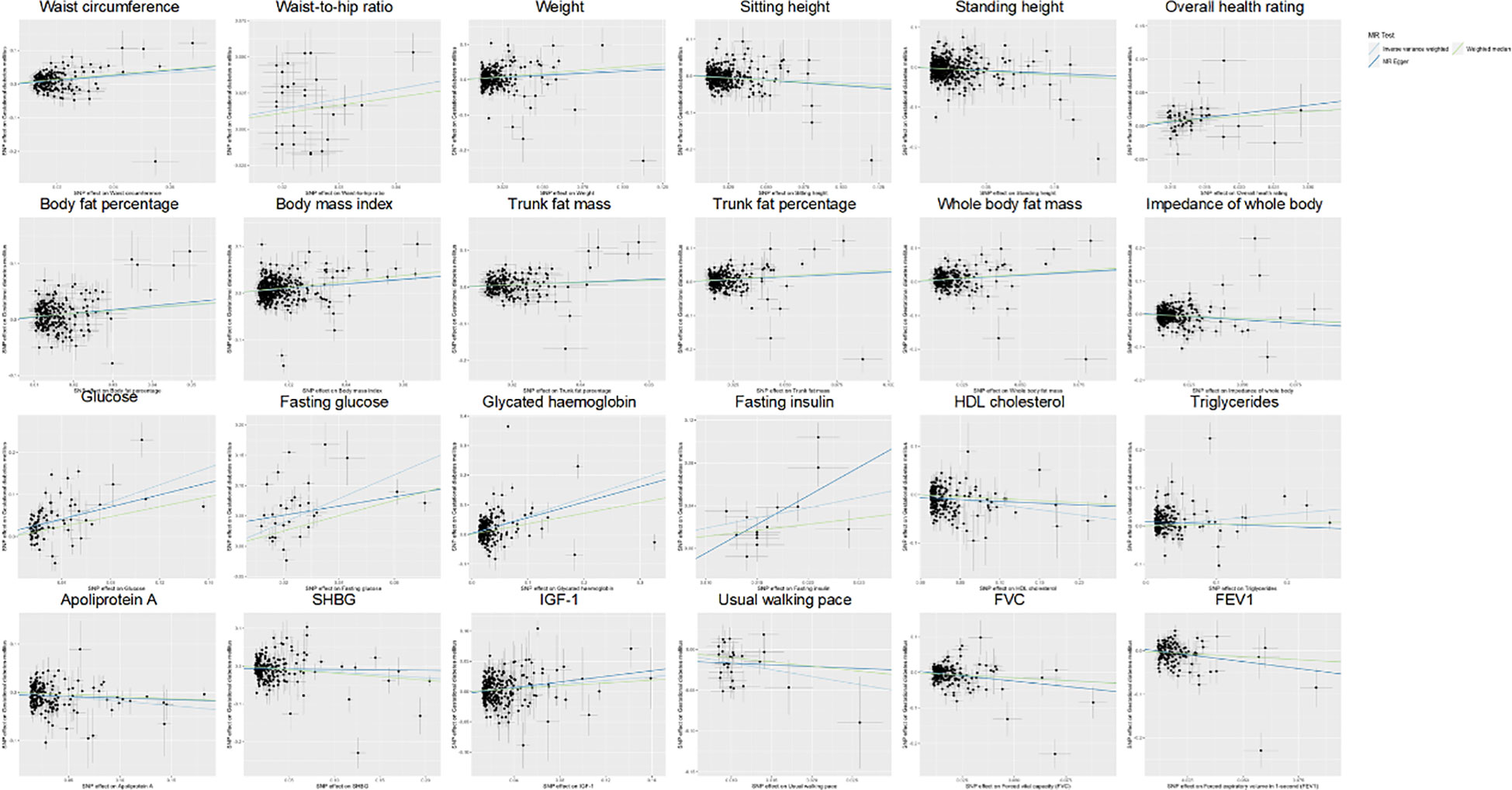
Figure 3 Scatter plot showing the causal effects of 24 risk factors on GDM. SNP, single nucleotide polymorphism; GDM, Gestational Diabetes Mellitus; HDL, High-density lipoprotein; SHBG, Sex hormone-binding globulin; IGF-1, Insulin-like growth factor 1; FVC, Forced vital capacity; FEV1, Forced expiratory volume in 1-second.
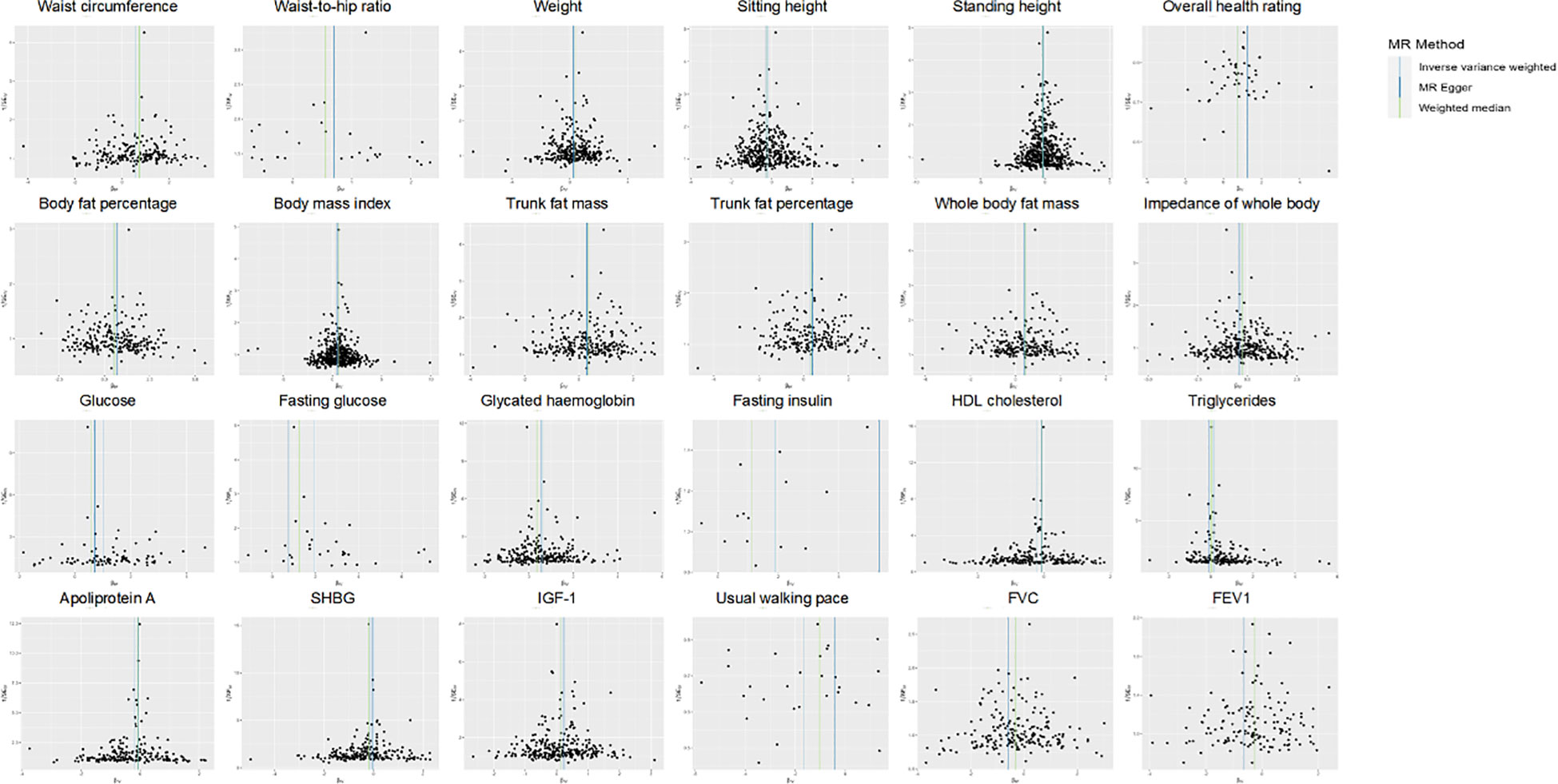
Figure 4 Funnel plot indicating the causal associations of 24 risk factors on GDM. SNP, single nucleotide polymorphism; GDM, Gestational Diabetes Mellitus; HDL, High-density lipoprotein; SHBG, Sex hormone-binding globulin; IGF-1, Insulin-like growth factor 1; FVC, Forced vital capacity; FEV1, Forced expiratory volume in 1-second; IV, instrumental variable; SE, standard error.
Discussion
This study employed a two-sample MR analysis to investigate the causal relationship between traits and GDM. The analysis incorporated GWAS summary statistics from public databases. The findings indicated significant causal associations between 24 risk factors and the risk of GDM. These risk factors will be discussed in detail across four subsequent paragraphs based on their respective categories.
Body size measures and body composition by impedance analysis
Body size measurements and body composition evaluated using impedance analysis are important indicators for assessing obesity. Overweight or obese women have up to a four-fold increased risk of developing GDM (27). We found a significant causal relationship between GDM and various body size measurements such as body mass index (BMI), weight, waist-to-hip ratio (WHR), and waist circumference (WC) in our study. Additionally, we observed correlations among several measures of body composition determined through impedance analysis including trunk fat mass, trunk fat percentage, whole-body fat mass, and body-fat percentage. Previous studies have demonstrated a strong association between gestational weight gain and both gestational impaired glucose tolerance and GDM (28, 29). Obesity and being overweight are significant risk factors for acquiring GDM (4). A recent study revealed that obesity and visceral adiposity are correlated with an elevated risk of developing GDM. Furthermore, it highlighted that among these factors, visceral adiposity specifically poses a higher risk for GDM (17). BMI, as a measure of general obesity, has been reported to show an association with the prevalence of GDM. Specifically, there is evidence suggesting that every 1 kg/m² increase in pre-pregnancy BMI leads to a rise in GDM prevalence by 0.92% (30). Central obesity refers to an excessive accumulation of abdominal fat which can be assessed using markers such as WHR and WC measurements (31). Previous literature has demonstrated an association between maternal central obesity in the first trimester of pregnancy and a higher occurrence of GDM (31, 32). The presence of visceral adipose tissue can be easily explained as it directly contributes to the pathogenesis of hyperglycemia. It does so by secreting various substances such as thrombogenic agents, inflammatory compounds, and inhibitors of adiponectin. These substances negatively impact glucose metabolism, increase insulin resistance and facilitate the development of metabolic syndrome along with subsequent cardiovascular diseases (33, 34).
Biomarkers
A meta-analysis revealed a significant association between elevated fasting glucose levels and a nearly two-fold increase in the risk of developing GDM (27). Moreover, a recent study has established an association between elevated fasting glucose during the initial stages of pregnancy and the subsequent onset of GDM (35). Enquobahrie et al. reported that for every increase in triglyceride content by 20 mg/dL, there is a 10% higher likelihood of developing GDM (36). Hypertriglyceridemia increases the risk for macrosomia due to factors such as insulin resistance caused by elevated triglycerides along with reduced lipoprotein lipase function. Macrosomia results in excessive fetal growth, obesity, as well as accumulation and release of fatty acids in cord blood and fetal adipose tissue (37). Previous research demonstrates an inverse correlation between serum HDL-c concentration and the risk of GDM and macrosomia. Additionally, even a slight increase in HDL-c levels serves as a protective factor against these conditions (38). Apolipoprotein A-1 is the primary lipoprotein associated with HDL-c. In contradiction to our results, a previous cohort study reported no association between serum Apolipoprotein A-1 levels, insulin resistance, or the risk of GDM in human subjects (39). However, since this study was observational, it cannot fully eliminate potential confounding variables as contributors to this discrepancy. Sex hormone binding globulin (SHBG), derived from the liver, is expressed in the placenta and acts as a regulator of sex steroid hormones. SHBG levels in the first trimester of pregnancy have been identified as reliable biomarkers for GDM (40, 41). There exists a negative correlation between SHBG and T2DM (42). Several previous studies have not only identified HbA1c as a diagnostic tool for GDM (43–45) but have also established a relationship between an HbA1c level above 7% in early pregnancy and adverse maternal outcomes (45). Fetal IGF-1 plays a crucial role in fetal growth due to its mitogenic and metabolic properties (46). According to Schwartz et al, an increase in IGF-1 concentration within umbilical cord blood contributes to accelerated intrauterine fetal growth (47).
Physical measures
Forced vital capacity (FVC) and FEV1 are commonly used indicators of lung function (48, 49). Consistent with previous research, our study identified a significant inverse causal association between FVC, FEV1, and GDM. A prior study has reported a significant association between restrictive ventilatory dysfunction assessed through FVC and FEV1 measurements with an elevated risk of T2DM, whereas no such relationship was observed for obstructive ventilatory dysfunction evaluated using the FEV1/FVC ratio (50). Emerging evidence indicates that inflammatory markers such as C-reactive protein and interleukin-6 might contribute to the association between T2DM and decreased FEV1 and FVC (51).
Lifestyle
Our study identified a negative causal relationship between usual walking pace and GDM, consistent with prior research in this field. Previous studies have suggested a robust correlation between the usual walking pace and a decreased likelihood of developing GDM (52). Furthermore, these studies revealed that women reporting faster speeds or longer durations during regular walks exhibited reduced risks of developing GDM when compared to those with slower speeds and shorter durations (52). Exercise leads to a significant increase in muscle glucose uptake. Exercise can increase muscle glucose uptake by up to 100 times when compared to resting conditions in humans (53). Increased glucose supply to the contracting skeletal muscles is made possible by the increase in blood flow and capillary recruitment during exercise (54).
Strengths and limitations
The current study possesses three significant strengths. Firstly, previous observational studies have suggested an increased risk of GDM onset in association with long-term maternal residence in an environment characterized by a mixture of PM2.5, PM10, NO2, and PM2.5 chemical constituents (55). However, the MR method can help mitigate the influence of confounding factors on the results. This method involves selecting a SNP that is strongly associated with the exposure of interest as the IV. By utilizing this SNP, individuals can be randomly assigned to the exposure, ensuring comparability of the population in terms of both known and unknown confounders. Secondly, we conducted a comprehensive investigation of the causal relationship between 108 traits and GDM. Thirdly, we employed a variety of sensitivity analyses to verify the results of our main analyses using IVW method. Lastly, during our assessment of pleiotropy within the MR analysis framework, we utilized MR-PRESSO method to provide outlier-corrected results.
However, our study has several limitations. Firstly, the scope of our investigation was limited to people of European descent. Consequently, the generalizability is impacted by this restriction. Further studies on diverse population groups are still needed in future research. Secondly, there might be potential selection bias affecting our results as individuals who died due to competition risk related outcomes might be missed in the GWAS analysis. Thirdly, due to the utilization of GWAS summary data, we were unable to stratify the data by variables such as age and gender, potentially introducing population bias. Finally, we could not assess any potential non-linear association between risk factors and GDM. Future research utilizing extensive biobanks may offer insights into the complex relationship between traits and GDM.
Conclusion
The present study has established a substantial and causative association between multiple risk factors and GDM. The MR analysis revealed statistically significant inverse associations of usual walking pace, FEV1 and FVC with GDM risk. This finding introduces novel insights that can guide future strategies aimed at preventing or mitigating the risks associated with GDM.
Data availability statement
The original contributions presented in the study are included in the article/Supplementary Material. Further inquiries can be directed to the corresponding authors.
Author contributions
QF: Writing – original draft. RC: Writing – original draft. SX: Writing – original draft. YD: Writing – original draft. CH: Writing – original draft. BH: Writing – original draft. TJ: Writing – original draft. BZ: Writing – original draft. MB: Writing – review & editing. SL: Writing – review & editing.
Funding
The author(s) declare financial support was received for the research, authorship, and/or publication of this article. This study was supported by the BUCM Precision Cultivation Program (Grant No. JZPY-202205) and the BUCM Research Development Fund (Grant No. 2021-ZXFZJJ-052).
Conflict of interest
The authors declare that the research was conducted in the absence of any commercial or financial relationships that could be construed as a potential conflict of interest.
Publisher’s note
All claims expressed in this article are solely those of the authors and do not necessarily represent those of their affiliated organizations, or those of the publisher, the editors and the reviewers. Any product that may be evaluated in this article, or claim that may be made by its manufacturer, is not guaranteed or endorsed by the publisher.
Supplementary material
The Supplementary Material for this article can be found online at: https://www.frontiersin.org/articles/10.3389/fendo.2023.1276836/full#supplementary-material
Abbreviations
GDM, Gestational Diabetes Mellitus; MR, Mendelian randomization; IVs, instrumental variables; GWAS, genome-wide association study; UKBB, UK Biobank; IVW, inverse-variance weighted; FDR, false discovery rate; FEV1, Forced expiratory volume in 1-second; FVC, Forced vital capacity; SHBG, Sex hormone-binding globulin; HDL, High-density lipoprotein; OR, odds ratio; CI, confidence interval; T2DM, type 2 diabetes; SNPs, single nucleotide polymorphisms; LD, linkage disequilibrium; MAF, minor allele frequency; IGF-1, Insulin-like growth factor 1.
References
1. Greco E, Calanducci M, Nicolaides KH, Barry EVH, Huda MSB, Iliodromiti S. Gestational diabetes mellitus and adverse maternal and perinatal outcomes in twin and singleton pregnancies: a systematic review and meta-analysis. Am J Obstetrics Gynecology (2023) S0002-9378(23):00545–8. doi: 10.1016/j.ajog.2023.08.011
2. Liang X, Zhang J, Wang Y, Wu Y, Liu H, Feng W, et al. Comparative study of microvascular structural changes in the gestational diabetic placenta. Diabetes Vasc Dis Res (2023) 20(3):14791641231173627. doi: 10.1177/14791641231173627
3. Magliano DJ, Boyko EJ, I.D.F.D.A.t.e.s. committee. IDF Diabetes Atlas. In: Idf diabetes atlas. Brussels: International Diabetes Federation© International Diabetes Federation (2021).
4. Zhou T, Du S, Sun D, Li X, Heianza Y, Hu G, et al. Prevalence and trends in gestational diabetes mellitus among women in the United States, 2006-2017: A population-based study. Front In Endocrinol (2022) 13:868094. doi: 10.3389/fendo.2022.868094
5. Billionnet C, Mitanchez D, Weill A, Nizard J, Alla F, Hartemann A, et al. Gestational diabetes and adverse perinatal outcomes from 716,152 births in France in 2012. Diabetologia (2017) 60(4):636–44. doi: 10.1007/s00125-017-4206-6
6. Farrar D, Simmonds M, Bryant M, Sheldon TA, Tuffnell D, Golder S, et al. Hyperglycaemia and risk of adverse perinatal outcomes: systematic review and meta-analysis. BMJ (Clinical Res ed.) (2016) 354:i4694. doi: 10.1136/bmj.i4694
7. Reece EA, Leguizamón G, Wiznitzer A. Gestational diabetes: the need for a common ground. Lancet (London England) (2009) 373(9677):1789–97. doi: 10.1016/S0140-6736(09)60515-8
8. Vounzoulaki E, Khunti K, Abner SC, Tan BK, Davies MJ, Gillies CL. Progression to type 2 diabetes in women with a known history of gestational diabetes: systematic review and meta-analysis. BMJ (Clinical Res ed.) (2020) 369:m1361. doi: 0.1136/bmj.m1361
9. Yu Y, Arah OA, Liew Z, Cnattingius S, Olsen J, Sørensen HT, et al. Maternal diabetes during pregnancy and early onset of cardiovascular disease in offspring: population based cohort study with 40 years of follow-up. BMJ (Clinical Res ed.) (2019) 367:l6398. doi: 10.1136/bmj.l6398
10. Su M, Hu R, Tang T, Tang W, Huang C. Review of the correlation between Chinese medicine and intestinal microbiota on the efficacy of diabetes mellitus. Front Endocrinol (Lausanne) (2022) 13:1085092. doi: 10.3389/fendo.2022.1085092
11. Xu Z, Zhang P, Chen Y, Jiang J, Zhou Z, Zhu H. Comparing SARC-CalF with SARC-F for screening sarcopenia in adults with type 2 diabetes mellitus. Front Nutr (2022) 9:803924. doi: 10.3389/fnut.2022.803924
12. Chen J, Li X, Liu H, Zhong D, Yin K, Li Y, et al. Bone marrow stromal cell-derived exosomal circular RNA improves diabetic foot ulcer wound healing by activating the nuclear factor erythroid 2-related factor 2 pathway and inhibiting ferroptosis. Diabetes Med (2023) 40(7):e15031. doi: 10.1111/dme.15031
13. Luo M, Cao Q, Wang D, Tan R, Shi Y, Chen J, et al. The impact of diabetes on postoperative outcomes following spine surgery: A meta-analysis of 40 cohort studies with 2.9 million participants. Int J Surg (2022) 104:106789. doi: 10.1016/j.ijsu.2022.106789
14. Mi W, Xia Y, Bian Y. Meta-analysis of the association between aldose reductase gene (CA)n microsatellite variants and risk of diabetic retinopathy. Exp Ther Med (2019) 18(6):4499–509. doi: 10.3892/etm.2019.8086
15. Yu T, Xu B, Bao M, Gao Y, Zhang Q, Zhang X, et al. Identification of potential biomarkers and pathways associated with carotid atherosclerotic plaques in type 2 diabetes mellitus: A transcriptomics study. Front Endocrinol (Lausanne) (2022) 13:981100. doi: 10.3389/fendo.2022.981100
16. Yang YY, Shi LX, Li JH, Yao LY, Xiang DX. Piperazine ferulate ameliorates the development of diabetic nephropathy by regulating endothelial nitric oxide synthase. Mol Med Rep (2019) 19(3):2245–53. doi: 10.3892/mmr.2019.9875
17. Alwash SM, McIntyre HD, Mamun A. The association of general obesity, central obesity and visceral body fat with the risk of gestational diabetes mellitus: Evidence from a systematic review and meta-analysis. Obes Res Clin Pract (2021) 15(5):425–30. doi: 10.1016/j.orcp.2021.07.005
18. Benhalima K, Van Crombrugge P, Moyson C, Verhaeghe J, Vandeginste S, Verlaenen H, et al. Estimating the risk of gestational diabetes mellitus based on the 2013 WHO criteria: a prediction model based on clinical and biochemical variables in early pregnancy. Acta Diabetologica (2020) 57(6):661–71. doi: 10.1007/s00592-019-01469-5
19. Luo J, Wang X, Yuan L, Guo L. Association of thyroid disorders with gestational diabetes mellitus: a meta-analysis. Endocrine (2021) 73(3):550–60. doi: 10.1007/s12020-021-02712-2
20. Bowden J, Davey Smith G, Burgess S. Mendelian randomization with invalid instruments: effect estimation and bias detection through Egger regression. Int J Epidemiol (2015) 44(2):512–25. doi: 10.1093/ije/dyv080
21. Jiang Y, Chen R, Xu S, Ding Y, Zhang M, Bao M, et al. Assessing causal associations of hyperparathyroidism with blood counts and biochemical indicators: a Mendelian randomization study. Front Endocrinol (Lausanne) (2023) 14. doi: 10.3389/fendo.2023.1295040
22. Chen R, Xu S, Ding Y, Li L, Huang C, Bao M, et al. Dissecting causal associations of type 2 diabetes with 111 types of ocular conditions: a Mendelian randomization study. Front Endocrinol (2023) 14. doi: 10.3389/fendo.2023.1307468
23. Fu Q, Chen R, Ding Y, Xu S, Huang C, He B, et al. Sodium intake and the risk of various types of cardiovascular diseases: A Mendelian randomization study. Front Endocrinol (Lausanne) (2023). doi: 10.3389/fnut.2023.1250509
24. Walker VM, Vujkovic M, Carter AR, Davies NM, Udler MS, Levin MG, et al. Separating the direct effects of traits on atherosclerotic cardiovascular disease from those mediated by type 2 diabetes. Diabetologia (2022) 65(5):790–9. doi: 10.1007/s00125-022-05653-1
25. Hemani G, Zheng J, Elsworth B, Wade KH, Haberland V, Baird D, et al. The MR-Base platform supports systematic causal inference across the human phenome. ELife (2018) 7:e34408. doi: 10.7554/eLife.34408
26. Burgess S, Thompson SG. Avoiding bias from weak instruments in Mendelian randomization studies. Int J Epidemiol (2011) 40(3):755–64. doi: 10.1093/ije/dyr036
27. Habibi N, Mousa A, Tay CT, Khomami MB, Patten RK, Andraweera PH, et al. Maternal metabolic factors and the association with gestational diabetes: A systematic review and meta-analysis. Diabetes Metab Res Rev (2022) 38(5):e3532. doi: 10.1002/dmrr.3532
28. Hedderson MM, Gunderson EP, Ferrara A. Gestational weight gain and risk of gestational diabetes mellitus. Obstetrics Gynecology (2010) 115(3):597–604. doi: 10.1097/AOG.0b013e3181cfce4f
29. Saldana TM, Siega-Riz AM, Adair LS, Suchindran C. The relationship between pregnancy weight gain and glucose tolerance status among black and white women in central North Carolina. Am J Obstetrics Gynecology (2006) 195(6):1629–35. doi: 10.1016/j.ajog.2006.05.017
30. Torloni MR, Betrán AP, Horta BL, Nakamura MU, Atallah AN, Moron AF, et al. Prepregnancy BMI and the risk of gestational diabetes: a systematic review of the literature with meta-analysis. Obes Rev (2009) 10(2):194–203. doi: 10.1111/j.1467-789X.2008.00541.x
31. Yao D, Chang Q, Wu Q-J, Gao S-Y, Zhao H, Liu Y-S, et al. Relationship between maternal central obesity and the risk of gestational diabetes mellitus: A systematic review and meta-analysis of cohort studies. J Diabetes Res (2020) 2020:6303820. doi: 10.1155/2020/6303820
32. Madhavan A, Beena Kumari R, Sanal MG. A pilot study on the usefulness of body mass index and waist hip ratio as a predictive tool for gestational diabetes in Asian Indians. Gynecological Endocrinol (2008) 24(12):701–7. doi: 10.1080/09513590802444134
33. Martin AM, Berger H, Nisenbaum R, Lausman AY, MacGarvie S, Crerar C, et al. Abdominal visceral adiposity in the first trimester predicts glucose intolerance in later pregnancy. Diabetes Care (2009) 32(7):1308–10. doi: 10.2337/dc09-0290
34. Basraon SK, Mele L, Myatt L, Roberts JM, Hauth JC, Leveno KJ, et al. Relationship of early pregnancy waist-to-hip ratio versus body mass index with gestational diabetes mellitus and insulin resistance. Am J Perinatology (2016) 33(1):114–21. doi: 10.1055/s-0035-1562928
35. Hao M, Lin L. Fasting plasma glucose and body mass index during the first trimester of pregnancy as predictors of gestational diabetes mellitus in a Chinese population. Endocr J (2017) 64(5):561–9. doi: 10.1507/endocrj.EJ16-0359
36. Enquobahrie DA, Williams MA, Qiu C, Luthy DA. Early pregnancy lipid concentrations and the risk of gestational diabetes mellitus. Diabetes Res Clin Pract (2005) 70(2):134–42. doi: 10.1016/j.diabres.2005.03.022
37. Herrera E, Ortega-Senovilla H. Disturbances in lipid metabolism in diabetic pregnancy - Are these the cause of the problem? Best Practice & Research. Clin Endocrinol Metab (2010) 24(4):515–25. doi: 10.1016/j.beem.2010.05.006
38. Jin W-Y, Lin S-L, Hou R-L, Chen X-Y, Han T, Jin Y, et al. Associations between maternal lipid profile and pregnancy complications and perinatal outcomes: a population-based study from China. BMC Pregnancy Childbirth (2016) 16:60. doi: 10.1186/s12884-016-0852-9
39. Retnakaran R, Ye C, Connelly PW, Hanley AJ, Sermer M, Zinman B. Serum apoA1 (Apolipoprotein A-1), insulin resistance, and the risk of gestational diabetes mellitus in human pregnancy-brief report. Arteriosclerosis Thrombosis Vasc Biol (2019) 39(10):2192–7. doi: 10.1161/ATVBAHA.119.313195
40. Maged AM, Moety GAF, Mostafa WA, Hamed DA. Comparative study between different biomarkers for early prediction of gestational diabetes mellitus. J Maternal-fetal Neonatal Med (2014) 27(11):1108–12. doi: 10.3109/14767058.2013.850489
41. Smirnakis KV, Plati A, Wolf M, Thadhani R, Ecker JL. Predicting gestational diabetes: choosing the optimal early serum marker. Am J Obstetrics Gynecology (2007) 196(4):410.E1–410.E7. doi: 10.1016/j.ajog.2006.12.011
42. Ding EL, Song Y, Malik VS, Liu S. Sex differences of endogenous sex hormones and risk of type 2 diabetes: a systematic review and meta-analysis. JAMA (2006) 295(11):1288–99. doi: .1001/jama.295.11.1288
43. Hughes RCE, Moore MP, Gullam JE, Mohamed K, Rowan J. An early pregnancy HbA1c ≥5.9% (41 mmol/mol) is optimal for detecting diabetes and identifies women at increased risk of adverse pregnancy outcomes. Diabetes Care (2014) 37(11):2953–9. doi: 10.2337/dc14-1312
44. Renz PB, Cavagnolli G, Weinert LS, Silveiro SP, Camargo JL. HbA1c test as a tool in the diagnosis of gestational diabetes mellitus. PloS One (2015) 10(8):e0135989. doi: 10.1371/journal.pone.0135989
45. Xodo S, Londero AP, D'Agostin M, Novak A, Galasso S, Pittini C, et al. Is glycated hemoglobin A1c level associated with adverse pregnancy outcomes of women affected by pre-gestational diabetes? Medicina (Kaunas) (2021) 57(5):461. doi: 10.3390/medicina57050461
46. Bloomfield FH, Spiroski A-M, Harding JE. Fetal growth factors and fetal nutrition. Semin In Fetal Neonatal Med (2013) 18(3):118–23. doi: 10.1016/j.siny.2013.03.003
47. Schwartz R, Teramo KA. Effects of diabetic pregnancy on the fetus and newborn. Semin In Perinatology (2000) 24(2):120–35. doi: 10.1053/sp.2000.6363
48. Hankinson JL, Eschenbacher B, Townsend M, Stocks J, Quanjer PH. Use of forced vital capacity and forced expiratory volume in 1 second quality criteria for determining a valid test. Eur Respir J (2015) 45(5):1283–92. doi: 10.1183/09031936.00116814
49. Zhu LM, Zeng D, Lei XC, Huang J, Deng YF, Ji YB, et al. KLF2 regulates neutrophil migration by modulating CXCR1 and CXCR2 in asthma. Biochim Biophys Acta Mol Basis Dis (2020) 1866(12):165920. doi: 10.1016/j.bbadis.2020.165920
50. Peng Y, Zhong G-C, Wang L, Guan L, Wang A, Hu K, et al. Chronic obstructive pulmonary disease, lung function and risk of type 2 diabetes: a systematic review and meta-analysis of cohort studies. BMC Pulmonary Med (2020) 20(1):137. doi: 10.1186/s12890-020-1178-y
51. Wannamethee SG, Shaper AG, Rumley A, Sattar N, Whincup PH, Thomas MC, et al. Lung function and risk of type 2 diabetes and fatal and nonfatal major coronary heart disease events: possible associations with inflammation. Diabetes Care (2010) 33(9):1990–6. doi: 10.2337/dc10-0324
52. Zhang C, Solomon CG, Manson JE, Hu FB. A prospective study of pregravid physical activity and sedentary behaviors in relation to the risk for gestational diabetes mellitus. Arch Internal Med (2006) 166(5):543–8. doi: 10.1001/archinte.166.5.543
53. Richter EA. Is GLUT4 translocation the answer to exercise-stimulated muscle glucose uptake? Am J Physiol Endocrinol Metab (2021) 320(2):E240–e243. doi: 10.1152/ajpendo.00503.2020
54. Sjøberg KA, Frøsig C, Kjøbsted R, Sylow L, Kleinert M, Betik AC, et al. Exercise increases human skeletal muscle insulin sensitivity via coordinated increases in microvascular perfusion and molecular signaling. Diabetes (2017) 66(6):1501–10. doi: 10.2337/db16-1327
Keywords: risk factor, gestational diabetes mellitus, Mendelian randomization, UK biobank, FinnGen
Citation: Fu Q, Chen R, Xu S, Ding Y, Huang C, He B, Jiang T, Zeng B, Bao M and Li S (2024) Assessment of potential risk factors associated with gestational diabetes mellitus: evidence from a Mendelian randomization study. Front. Endocrinol. 14:1276836. doi: 10.3389/fendo.2023.1276836
Received: 13 August 2023; Accepted: 14 December 2023;
Published: 08 January 2024.
Edited by:
Guoju Li, Qingdao Women and Children’s Hospital, ChinaReviewed by:
Haiping Duan, Qingdao Municipal Center for Disease Control and Prevention, ChinaAnamaria Savu, University of Alberta, Canada
Xiao Ye, Zhejiang Provincial People’s Hospital, China
Copyright © 2024 Fu, Chen, Xu, Ding, Huang, He, Jiang, Zeng, Bao and Li. This is an open-access article distributed under the terms of the Creative Commons Attribution License (CC BY). The use, distribution or reproduction in other forums is permitted, provided the original author(s) and the copyright owner(s) are credited and that the original publication in this journal is cited, in accordance with accepted academic practice. No use, distribution or reproduction is permitted which does not comply with these terms.
*Correspondence: Meihua Bao, bWhiYW83OEAxNjMuY29t; Sen Li, c2VubGlAY29ubmVjdC5oa3UuaGs=
†These authors have contributed equally to this work and share first authorship