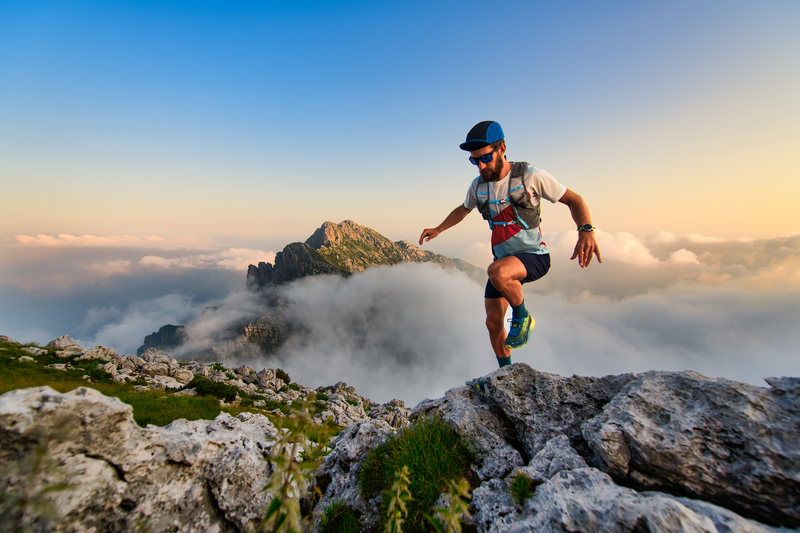
94% of researchers rate our articles as excellent or good
Learn more about the work of our research integrity team to safeguard the quality of each article we publish.
Find out more
ORIGINAL RESEARCH article
Front. Endocrinol. , 31 October 2022
Sec. Clinical Diabetes
Volume 13 - 2022 | https://doi.org/10.3389/fendo.2022.1036426
This article is part of the Research Topic Emerging Talents in Clinical Diabetes View all 7 articles
Background: Artificial intelligence (AI), which has been used to diagnose diabetic retinopathy (DR), may impact future medical and ophthalmic practices. Therefore, this study explored AI’s general applications and research frontiers in the detection and gradation of DR.
Methods: Citation data were obtained from the Web of Science Core Collection database (WoSCC) to assess the application of AI in diagnosing DR in the literature published from January 1, 2012, to June 30, 2022. These data were processed by CiteSpace 6.1.R3 software.
Results: Overall, 858 publications from 77 countries and regions were examined, with the United States considered the leading country in this domain. The largest cluster labeled “automated detection” was employed in the generating stage from 2007 to 2014. The burst keywords from 2020 to 2022 were artificial intelligence and transfer learning.
Conclusion: Initial research focused on the study of intelligent algorithms used to localize or recognize lesions on fundus images to assist in diagnosing DR. Presently, the focus of research has changed from upgrading the accuracy and efficiency of DR lesion detection and classification to research on DR diagnostic systems. However, further studies on DR and computer engineering are required.
Diabetic retinopathy (DR) is a complication of diabetes that affects the fundus blood vessels. It poses a major threat to vision loss and blindness in patients with diabetes (1). In 2015, approximately 415 million people worldwide were diagnosed with diabetes. This number is expected to increase to 642 million by 2040 (2). It is estimated that more than one-third of people with diabetes worldwide have some form of DR, and approximately one-tenth of people with diabetes have vision-threatening DR, including proliferative DR and diabetic macular edema (3). Regular screening is crucial for patients with DR; however, traditional detection methods are inadequate for many patients. Traditional screening or diagnostic methods require professional doctors and are time-consuming, laborious, and expensive. As a result, large-scale early screening remains a significant challenge.
With the emergence and continuous development of artificial intelligence, the integration of AI in healthcare, including screening of major ophthalmic diseases, becomes a research hotspot. AI refers to the use of computers to simulate intelligent behavior with little or no human intervention, which is a broad term (4). This technology involves many aspects, including traditional machine learning (ML) and deep learning (DL) (5). In the phase of traditional ML, through the feature extraction, AI can localize lesions on retinal images to diagnose and grade DR based on imaging biomarkers, including microaneurysms, hard exudation, cotton-wool spots, and macular edema. With the emergence of DL, which uses multilevel and multineuron learning algorithms, several system using DL for DR screening has been constructed and achieved higher specificity and sensitivity than the ones using traditional ML (6, 7). As AI technology continuously developing, more and more AI research is combining knowledge-driven and data-driven approaches, which is also reflected in DR research (8). The integration of AI in DR screening will significantly improve diagnostic efficiency, save workforce and financial resources, and make remote diagnosis possible in remote and poor areas, which is a very promising field.
Bibliometric studies have already been conducted on the AI application in ophthalmic diseases (9–11). Considering the current research situation, an updated bibliometric study on the application of AI in DR was decided to be conducted based on the present research results. This study aimed to use bibliometric methods to analyze the literature retrieved from the Web of Science Core Collection (WoSCC) to assess the global status of the application of AI in DR research and analyze the hotspots and trends. For instance, the scientific citation index literature on AI’s application in DR screening and diagnosis was analyzed using bibliometric methods. The analysis highlighted data relevant to countries and regions, institutions, journals, research categories, keywords, and references. A fundamental objective of our research is to develop a repeatable and unbiased strategy to explore the dynamic frontiers of knowledge in research field. Therefore, active areas, future development areas, and potential barriers to the application of AI in the screening and diagnosis of DR were particularly examined in this study. This report aimed to provide resources for AI professionals, ophthalmologists, diagnostic physicians, and medical imaging researchers.
On October 3, 2022, all citation data published between January 1, 2012, and June 30, 2022 were downloaded from WoSCC. Two authors (Guangxi Zuo and Ruoyu Wang) verified the data independently. The retrieval formula was TS = (AI or “Artificial Intelligence” or “neural network” or “transfer learning” or “Machine Learning” or “Deep Learning” or automat* or algorithm) AND TS = (“diabetic eye disease” or “diabetic retinopathy” or “diabetic macular edema”). The search selected English literature and articles and excluded early access, proceedings papers, book chapters, data papers, and retracted publications.
To ensure accuracy of the study, the data were manually expurgated after reading the titles and abstracts of study without exception to obtain the most accurate analysis results. The criteria for manual exclusion were as follows:1) the research subject was not DR, and 2) the research method excluded AI. Details of the filtering process are shown in Figure 1. The data included in this study were all about the application of AI in detecting and grading DR. We analyzed general data, countries or regions, institutions, citing journals, subject categories, references, and keywords using CiteSpace 6.1.R3. All citation features have been included in this article.
Figure 1 Frame flow diagram showing the detailed selection criteria and bibliometric analysis steps for the study of the application of artificial intelligence in diabetic retinopathy.
We analyzed 858 studies published between January 1, 2012, and June 30, 2022, focusing on the application of AI in detecting and grading DR. The data collected and counted from WoSCC were de-duplicated by means of the duplication removal function of the CiteSpace software to confirm the number of verified data.
The annual number of articles on the application of AI in detecting and grading DR increased steadily between 2012 and 2019. It began to rise in 2020 and exceeded 200 for the first time in 2021. The number of publications per year is shown in Figure 2.
Figure 2 Annual number of publications on the application of artificial intelligence in diabetic retinopathy from 2012 to June 2022.
The CiteSpace software’s default settings were used to count the number of papers in each country and to analyze the cooperation relationships between countries and regions. Overall, the citations involved 77 countries and regions. The size of each label and the yellow node area in Figure 3 represent the number of citations. The top three countries with large yellow nodes were China, India, and the United States, with 232, 216, and 139 articles, respectively. The cooperative relationships between countries were represented by the links between nodes. Countries with more links are more influential. The influence of a country is illustrated in Figure 3 by the dimension of the purple circle. Notably, the purple circle corresponding to the “United States” label has the largest area. (0.35), implying that articles published in the United States have the greatest overall influence in the field of AI application in detecting and grading DR. The data in Table 1 confirm this conclusion. The H-index can precisely reflect a country’s academic achievement (12). The more links a country has, the higher its centrality and the wider the purple circle. Generally, China has the highest publication count, while the United States is regarded as the most influential country.
Figure 3 Cooperation of countries or regions that contributed to publications on the use of artificial intelligence for diagnosing diabetic retinopathy from 2012 to 2022.
Table 1 Top 10 countries or regions with publications on the application of AI in DR from 2012 to June 2022.
The top 10 institutions of the selected articles focusing on the application of AI in detecting and grading DR are presented in Table 2. The data displayed are the results of CiteSpace’s default setting. However, 5 out of 10 are Chinese institutions because China has the maximum sum of publications. Institutions in the United States, Singapore, India, Egypt, and Pakistan were included in addition to Chinese institutions. The size of the nodes in Figure 4 is positively correlated with the number of articles published by each institution. Furthermore, the links between labels reflect cooperative relationships among institutions. Regarding academic achievements, the United States and a Pakistanian institution had the highest H-index. Two of the top five institutions with the highest H-indices were Chinese institutions.
Table 2 Top 10 institutions with publications on the application or the use of AI in DR from 2012 to June 2022.
Figure 4 Cooperation of institutions that contributed to publications on the use of artificial intelligence in diabetic retinopathy from 2012 to June 2022.
The literature in cited journals constitutes the knowledge base of the references, and the research area of highly cited journals is an active interest or emerging area. We used CiteSpace to map and overview literature co-citation relationships in the field of journal research, with the citing journal map on the left and the cited journal map on the right. Notably, the two colored paths shown in Figure 5 represent the reference relationships for the highly active research areas. Given the correlation between color and quantity, the classification of journals with the highest number of papers was represented by the red path. The knowledge-based research fields of AI application in detecting and grading DR in the past decade consist of engineering technology, computer, medicine, medical imaging, ophthalmology, and biology, which constitute the hot subjects involved in the research frontier, including systems, mathematics, neurology, information science, and ophthalmology.
Figure 5 Dual map overlay of journals that contributed to publications on the use of artificial intelligence in diabetic retinopathy from 2012 to June 2022.
The top 10 subject categories of the citing journals and the cited journals/proceedings regarding citations are presented in Tables 3, 4. The most common areas of the study cited in journals included engineering technology/biology. The discipline with the most involvement in the cited journals was classified as engineering technology/ophthalmology/biology.
Table 4 Top 10 cited journals of publications on the use of AI in DR from January 2012 to June 2022.
The emerging keywords developed over time were analyzed based on the keyword co-occurrence cooperative network analysis chart, in order to better understand the application of AI in the field of DR diagnosis and gradation in the past decade, reflecting the shift in research hotspots. CiteSpace’s default setting was replaced by the following modes: “Year Per Slice” = 1, “Top N%” = 10.0%, and “Minimum Duration” = 1. The results are shown in Figure 6. The burst detection analysis can detect great changes in the number of references in a certain period to determine the fading or rising of a certain subject word or keyword. The emerging keywords for the investigated timeline are depicted as red squares in Figure 6. The burst keywords from January 2012 to June 2021 included retinal image (2012–2017), localization (2012–2014), segmentation (2012–2017), diagnosis (2012–2016), quantification (2013–2015), identification (2013–2015), automated detection (2013–2017), red leision (2014–2018), matched filter (2015–2018), automatic detection (2016–2018), artificial intelligence (2020–2022), and transfer learning (2021–2022).
Figure 6 Keywords with the strongest citation bursts for publications on the use of artificial intelligence in diabetic retinopathy from January 2012 to June 2022.
The cited articles were closely related to the research topics. Therefore, a knowledge base for using AI in DR research can be efficiently established through co-citation analysis of cited references. We created a co-citation reference timeline map to identify research topics. Figure 7 summarizes and presents research topics in the form of clusters, with the size of the clusters listed on the right. The nodes on the timeline in the figure represent the time at which a document was first cited. Furthermore, the node’s size represents the accumulated times the study was cited. The larger the size, the more frequently the document was mentioned. The top label “#0 automated detection” was obtained in the topic generation phase from 2007 to 2014. Table 5 presents the top 10 citing literature from “times cited in all databases” among the relevant literature based on the application of AI in DR. It was determined that AI techniques could be promising for the application of AI in the diagnosis and grading of DR. However, there were still some limitations when it comes to clinical applications.
Figure 7 Co-cited reference timeline map of publications on the use of artificial intelligence in diabetic retinopathy from January 2012 to June 2022.
Based on the above outcomes, the amount of published literature on DR AI detection and intelligent diagnosis has significantly increased over the past 4 years. This indicates that, as the demand for screening, diagnosis, classification, and follow-up of DR increases, so has the interest in the application of AI in DR.
The development of artificial intelligence takes the clinical practice of ophthalmology as a research frontier (21), with DR being the most studied eye disease (22). DL algorithms were verified to be highly sensitive and specific (6, 7) in detecting and classifying DR and related eye diseases, which is a promising research field. Regarding the amount of national literature, China has the largest number of articles; however, a few articles have been highly cited. The United States has the highest centrality and H-index, indicating that it is in the lead in this research field. Moreover, the United Kingdom, India, and Pakistan have considerable centrality and influence. The top three research institutions by the number of publications were the United States, China, and Singapore. Regarding the H-index, Oregon Health and Science University in the United States and the National University of Sciences and Technology in Pakistan strongly influence this area. The focus of AI research has changed from upgrading the accuracy and efficiency of DR detection and classification to research on DR diagnostic systems. AI is applied to the diagnosis and classification of DR, which is usually performed based on fundus photography and optical coherence tomography (OCT) analysis, using retinal vessel segmentation and directional local contrast to detect lesions, including microaneurysms and hard exudation. Furthermore, clustering timelines based on emerging keywords can identify active areas of interest, research frontiers, and commonly cited references of AI in DR diagnosis. In 2018, the United States Food and Drug Administration approved an AI system that could detect referential DR from retinal photographs. This is the first independent diagnostic system approved in the medical field (13). In addition, this indicates that AI DR intelligent diagnosis systems have broad prospects in clinical applications and are expected to promote the reform of clinical diagnosis systems.
Through clustering analysis of the results of the co-cited articles, it can be easily determined that “automated detection” and “intergrated DR index”, were the most important knowledge bases in the early stages of studies. This illustrates that the focal point of this study was to improve the efficiency and accuracy of DR lesion detection and classification.
Automated monitoring and screening for DR could significantly reduce manpower and time. Meanwhile, early treatment can reduce visual impairment caused by disease progression. Therefore, automatic detection of DR has been a hot topic in this field. The automatic detection technology for DR has been constantly improving. In the early stage, the intelligent recognition and diagnosis of DR-related lesions in fundus images were mainly detected by machine learning(ML). During this period, a variety of ML tools for DR screening were developed, such as decision tree, support vector machine (SVM), artificial neural network (ANN), Bayesian classifier, and so on. However, the recognition efficiency of traditional ML is limited, and sometimes manual confirmation is needed. Therefore, some scholars have tried to integrate various algorithms by adding ensemble learning algorithms on the basis of ML, so that the computer can identify the lesions related to DR through different strategies. Antal et al. (23) established a new ensemble-based system to detect microaneurysms and grade DR, and achieved an area under the curve (AUC) of 0.875. Since 2016, due to the gradual application of DL in automatic DR recognition, the efficiency and accuracy of automatic detection have been greatly improved.
Previous studies have shown that it is difficult to distinguish fundus images from normal images in patients with DR by using traditional measurement methods based on texture features. In contrast, the advantage of the integrated index is that it only examines the value of one composite index, which represents the degree of deviation from the normal, to make a correct diagnosis. The latter can improve the accuracy of lesion detection and disease classification and significantly facilitate the work of clinicians. Acharya et al. (24) developed an integrated DR index (IDRI) comprising textural features that can be used to diagnose normal and different stages of DR.
The emerging keywords between 2012 and 2017 were “retinal image,” “localization,’’ “segmentation,”and “diagnosis.” Therefore, this indicates that the initial research focused on the study of intelligent algorithms used to segment and localize lesions on retinal images to assist in the diagnosis of DR. Retinal images provide valuable information related to the human eye; therefore, assessment of the characteristics of retinal blood vessels is important for disease diagnosis based on vascular pathology (25). A fundus image database is an important training and testing object for AI recognition and diagnostic systems. Franklin et al. (26) developed a novel automatic blood vessel segmentation method for fundus images that separated each image pixel into blood and non-blood vessels for automatic recognition of microaneurysms in retinal images. The detection of retinal microaneurysms using a multilayer perceptron neural network is helpful for ophthalmologists in diagnosis, and follow-up DR. Diagnostic localization on fundus images and OCT were as follows: exudates (17), optic disk (27), and dark and bright lesions (28). The efficiency of machine recognition during this period was limited and sometimes required manual confirmation, which differed from the current intelligent diagnosis that depended on DL (29). The traditional ML recognition system is commonly used as an auxiliary tool for clinicians to screen and diagnose (30).
The emerging keywords between 2013 and 2017 were “quantification,” “identification,’’ and “automated detection.” Therefore, this indicates that quantization processing of image features is gradually applied in DR automatic recognition. Finding and counting lesions, including microaneurysms, or exudation, on retinal images is crucial for the clinical diagnosis of DR. However, it is time-consuming and can be affected by human error. Accordingly, automatic layer detection technology for the human retina has been developed to improve its efficiency. Traditional diagnosis relies on experience and tends to supervisor error; therefore, an objective standard is required to measure the result of the diagnosis; that is, the automatic quantification of blood vessels in human retina is the guarantee of reducing the subjective error of intelligent diagnosis and enhancing accuracy (31). Wu et al. (32) developed a computer-aided quantification framework for the automatic detection of exudates and microaneurysms and compared the morphological characteristics of moderate and severe non-proliferative DR. The results showed that computer-aided quantification of DR could be a practical method for clinicians to better study DR.
The emerging keywords between 2015 and 2018 were “red lesion,” and “matched filter.” Therefore, this indicates that as an essential biomarker for the early diagnosis of DR, the detection of red lesions, including microaneurysms and hemorrhages, has become an important research direction in the intelligent diagnosis of DR. A matched filter is a segmentation algorithm that can be used to perform vessel extraction and detect microaneurysms. It is vital to detect red lesions, especially microaneurysms, from color fundus images for the early diagnosis of DR. However, accurate automatic detection of microaneurysms on color retinal images is still a challenging problem. One of the important solutions is to screen microaneurysms in images by vessel extraction using a multiscale matched filter. Filter-based microaneurysm detection algorithms are examples of vessel extraction (33–36). In addition to microaneurysms, researchers are also actively exploring the association of other biomarkers with DR to provide new directions for the intelligent diagnosis and staging of DR. For example, Niu et al. (37) highlighted that the area and number of hard exudate and hyperreflective lesions were related to DR staging. Cheung et al. (38) investigated the relationship between the geometric variables of retinal blood vessels measured from retinal photographs and the 6-year incidence and progression of DR.
The emerging keywords between 2016 and 2018 is “automatic detection”. In this period, DL algorithms, including the application of convolutional neural networks (CNN) in DR, drive these advances and transformations. Deep learning(DL) is a set of computational methods that allow algorithms to program themselves by learning from a large number of examples. These examples show only the desired behavior, not explicit calculation rules.(6) Compared with the traditional supervised learning, DL greatly improves the accuracy of automatic detection while reducing the workload, and has obvious advantages. In 2016, Abràmoff et al.(14) demonstrated that integrating convolutional neural networks on top of existing pathology-based diabetic retinopathy significantly improved the recognition performance of referable diabetic retinopathy compared to the same algorithm without DL techniques. CNN is one of the renowned DL algorithms in image-related applications (39). It has made great advances in the field of computer vision research by constantly revising and self-learning to complete the task (40, 41). In 2016, Gulshan et al. (6) created an algorithm to automatically detect DR and DME based on retinal fundus photos using deep CNN. This study demonstrated that deep ML based algorithms have high sensitivity and specificity for detecting referable diabetic retinopathy(RDR) in the evaluation of retinal fundus photos of adult diabetic patients. Many studies have shown that CNN has become a major tool for DR screening, staging and prediction, and possesses extensive application prospects (42, 43).
The emerging keywords between 2020 and 2022 were “Artificial intelligence” and “transfer learning.” This indicates that artificial intelligence including transfer learning is a hot topic in recent research. Although DL models provide automatic feature extraction and classification, it still needs a large number of annotated data sets to start training such intelligent models. The model based on transfer learning is widely used by researchers to overcome the problem of insufficient annotation data and computational overhead. Le et al. (44) constructed a retrained CNN for DR classification based on OCTA images with 83.76% sensitivity and 90.82% specificity via transfer learning.
AI is an intelligent system mainly based on DL, which has developed rapidly in recent years. This research field involves the deep integration of multi-disciplinary knowledge and technology, and requires the cooperation and sharing of multi-disciplinary technical resources. At present, there are still problems such as data standardization, insufficient clinical verification and products waiting to come into service. Although opportunities and challenges coexist in the research of AI-assisted DR screening, with the gradual deepening of the study and the joint efforts of relevant interdisciplinary researchers, the clinical application of AI-assisted diagnosis of DR in ophthalmology is expected to make greater progress.
The constraints of AI in diagnosing ophthalmic diseases were classified into the following five categories after summarizing the limitations of the top 10 cited articles in Table 5: 1) the design of intelligent diagnostic systems is complicated by complex clinical conditions and subjective evaluation criteria; 2) the sample size of the AI training model is relatively limited, and the actual validity requires confirmation; 3) the existing auxiliary diagnostic system is incapable of independent diagnosis, and the specific clinical application requires the advice of ophthalmologists or professionals; 4) inconsistent clinical reference standards may lead to differences in the performance of intelligent algorithms; and 5) the interpretability of the existing intelligent diagnostic system is unsatisfactory.
The following are the recommendations made to promote the application of AI technology in the diagnosis and intelligent classification of DR. First, in order to develop more robust and available diagnostic systems, more types and larger data sets are required. A unified high-quality DR image database and continuous optimization and expansion of the database are required to meet the diagnostic needs of DR to solve the problem of the number of samples. For example, establishing a database that can integrate data from different sources and address fragmented data could be useful for further diabetic research (45). Second, the research scope is required to be comprised of more common disease types, such as glaucoma and age-related macular degeneration (46, 47). Third, a unified output standard for DR disease diagnosis is required to guarantee the versatility of various systems. Fourth, more ophthalmologists in various professional levels should be involved in the dataset’s screening phase and the algorithm’s examination stage to obtain a clinical diagnosis. Fifth, the legal status of AI diagnosis should be guaranteed, and when the technology is advanced, AI can be approved for independent diagnosis. Finally, relevant professionals should strengthen technical research and enhance technology visualization to further improve the usage rate of AI technology.
There are a few latent limitations in this study. Firstly, given that the research method of this study is to analyze the previous literature, the prospective of the study may not be accurate enough. Meanwhile, there is a time period from research to publication. The published articles are often out of sync with the actual research time. Secondly, only English literature in the WoSCC database, a prominent academic database, was analyzed. Literature in other databases or other languages was not included considering the impracticability of fusing and analyzing data from different databases or data in different languages at the same time. Thirdly, the study mainly analyzed the application of AI technology in onefold DR cases, and did not analyze the application of AI in multiple retinal diseases.
Recently, intelligent algorithm training based on image analysis has attracted increasing attention. AI’s application in the DR screening and diagnosis is being studied worldwide. Particularly, the United States currently has the greatest clout in this research field. The application of AI in the screening and diagnosis of DR has significantly altered the clinical setting of ophthalmologists and patients. These technologies provide more rigorous, faster, and remote diagnostic services. However, these methods have certain limitations. For example, the sample size of the AI training model was limited, and the actual effectiveness of its clinical use requires confirmation. In addition, existing models can only be used as auxiliary diagnostic tools, and ophthalmologists or professionals still need to provide suggestions for clinical application. Furthermore, most contemporary studies are still at the system development and testing stage, and a sophisticated diagnostic system is yet to be developed. Initial research focused on the analysis of intelligent algorithms used to localize or recognize lesions on fundus images to assist in the diagnosis of DR. Currently, the focus of research has shifted from upgrading the accuracy and efficiency of DR lesion detection and DR classification to research on DR diagnostic systems. Therefore, acquiring more national and ethnic origins and merging more complex ophthalmic data is a necessity in training and testing the algorithm in order to address the existing limitations. However, it is a challenging task. In addition to computer engineering specialists who mainly develop algorithms, ophthalmologists in various professional levels are also needed to be involved in this research.
The original contributions presented in the study are included in the article/supplementary material. Further inquiries can be directed to the corresponding authors.
RW and GZ conceived and designed the analysis, performed the analysis, and wrote the manuscript; KL and WL revised the manuscript; ZX, YH, and WY designed the research, acquired the article information, and revised the manuscript. All authors contributed to the article and approved the submitted version.
Supported by Shenzhen Fund for Guangdong Provincial High-level Clinical Key Specialties (SZGSP014) and Sanming Project of Medicine in Shenzhen (SZSM202011015).
The authors declare that the research was conducted in the absence of any commercial or financial relationships that could be construed as a potential conflict of interest.
All claims expressed in this article are solely those of the authors and do not necessarily represent those of their affiliated organizations, or those of the publisher, the editors and the reviewers. Any product that may be evaluated in this article, or claim that may be made by its manufacturer, is not guaranteed or endorsed by the publisher.
1. Whiting DR, Guariguata L, Weil C, Shaw J. IDF diabetes atlas: Global estimates of the prevalence of diabetes for 2011 and 2030. Diabetes Res Clin Pract (2011) 94(3):311–21. doi: 10.1016/j.diabres.2011.10.029
2. Wang W, Lo A. Diabetic retinopathy: Pathophysiology and treatments. Int J Mol Sci (2018) 19(6):1816. doi: 10.3390/ijms19061816
3. Yau JWY, Rogers SL, Kawasaki R, Lamoureux EL, Kowalski JW, Bek T, et al. Global prevalence and major risk factors of diabetic retinopathy. Diabetes Care (2012) 35(3):556–64. doi: 10.2337/dc11-1909
4. Hamet P, Tremblay J. Artifificial intelligence in medicine. Metabolism (2017) 69S:S36–40. doi: 10.1016/j.metabol.2017.01.011
5. Balyen L, Peto T. Promising artificial intelligence-machine learning-deep learning algorithms in ophthalmology. Asia Pac J Ophthalmol (Phila) (2019) 8(3):264–72. doi: 10.22608/APO.2018479
6. Gulshan V, Peng L, Coram M, Stumpe MC, Wu D, Narayanaswamy A, et al. Development and validation of a deep learning algorithm for detection of diabetic retinopathy in retinal fundus photographs. JAMA (2016) 316(22):2402. doi: 10.1001/jama.2016.17216
7. Ting DSW, Cheung CY, Lim G, Tan GSW, Quang ND, Gan A, et al. Development and validation of a deep learning system for diabetic retinopathy and related eye diseases using retinal images from multiethnic populations with diabetes. JAMA (2017) 318(22):2211–23. doi: 10.1001/jama.2017.18152
8. Kermany DS, Goldbaum M, Cai W, Valentim CCS, Liang H, Baxter SL, et al. Identifying medical diagnoses and treatable diseases by image-based deep learning. Cell (2018) 172(5):1122–1131.e9. doi: 10.1016/j.cell.2018.02.010
9. Dong Y, Liu Y, Yu J, Qi S, Liu H. Mapping research trends in diabetic retinopathy from 2010 to 2019: A bibliometric analysis. Med (Baltimore) (2021) 100(3):e23981. doi: 10.1097/MD.0000000000023981
10. Saeed AQ, Sheikh Abdullah SNH, Che-Hamzah J. Abdul Ghani AT accuracy of using generative adversarial networks for glaucoma detection: Systematic review and bibliometric analysis. J Med Internet Res (2021) 23(9):e27414. doi: 10.2196/27414
11. Zhao J, Lu Y, Qian Y, Luo Y, Yang W. Emerging trends and research foci in artificial intelligence for retinal diseases: Bibliometric and visualization study. J Med Internet Res (2022) 24(6):e37532. doi: 10.2196/37532
12. Hirsch JE. An index to quantify an individual’s scientifific research output. Proc Natl Acad Sci U. S. A. (2005) 102:16569–72. doi: 10.1073/pnas.0507655102
13. Gargeya R, Leng T. Automated identification of diabetic retinopathy using deep learning. Ophthalmology (2017) 124(7):962–9. doi: 10.1016/j.ophtha.2017.02.008
14. Abràmoff MD, Lou Y, Erginay A, Clarida W, Amelon R, Folk JC, et al. Improved automated detection of diabetic retinopathy on a publicly available dataset through integration of deep learning. Invest Ophthalmol Vis Sci (2016) 57(13):5200–6. doi: 10.1167/iovs.16-19964
15. Abràmoff MD, Lavin PT, Birch M, Shah N, Folk JC. Pivotal trial of an autonomous AI-based diagnostic system for detection of diabetic retinopathy in primary care offices. NPJ Digit Med (2018) 1:39. doi: 10.1038/s41746-018-0040-6
16. Roy AG, Conjeti S, Karri SPK, Sheet D, Katouzian A, Wachinger C, et al. ReLayNet: retinal layer and fluid segmentation of macular optical coherence tomography using fully convolutional networks. BioMed Opt Express (2017) 8(8):3627–42. doi: 10.1364/BOE.8.003627
17. Giancardo L, Meriaudeau F, Karnowski TP, Li Y, Garg S, Tobin KW, et al. Exudate-based diabetic macular edema detection in fundus images using publicly available datasets. Med Image Anal (2012) 16(1):216–26. doi: 10.1016/j.media.2011.07.004
18. Decencière E, Cazuguel G, Zhang X, Thibault G, Klein J-C, Meyer F, et al. TeleOphta: Machine learning and image processing methods for teleophthalmology. IRBM (2013) 34(2):196–203. doi: 10.1016/j.irbm.2013.01.010
19. Abràmoff MD, Folk JC, Han DP, Walker JD, Williams DF, Russell SR, et al. Automated analysis of retinal images for detection of referable diabetic retinopathy. JAMA Ophthalmol (2013) 131(3):351. doi: 10.1001/jamaophthalmol.2013.1743
20. Quellec G, Charrière K, Boudi Y, Cochener B, Lamard M. Deep image mining for diabetic retinopathy screening. Med Image Anal (2017) 39:178–93. doi: 10.1016/j.media.2017.04.012
21. Scheetz J, He M, Wijngaarden P. Ophthalmology and the emergence of artificial intelligence. Med J Aust (2021) 214(4):155. doi: 10.5694/mja2.50932
22. Zhao J, Lu Y, Zhu S, Li K, Jiang Q, Yang W. Systematic bibliometric and visualized analysis of research hotspots and trends on the application of artificial intelligence in ophthalmic disease diagnosis. Front Pharmacol (2022) 13:930520. doi: 10.3389/fphar.2022.930520
23. Antal B, Hajdu A. An ensemble-based system for microaneurysm detection and diabetic retinopathy grading. IEEE Trans Biomed Eng (2012) 59(6):1720–6. doi: 10.1109/tbme.2012.2193126
24. Acharya UR, Ng EYK, Tan J-H, Sree SV, Ng K-H. An integrated index for the identification of diabetic retinopathy stages using texture parameters. J Med Syst (2011) 36(3):2011–20. doi: 10.1007/s10916-011-9663-8
25. Mendonça AM, Campilho A. Segmentation of retinal blood vessels by combining the detection of centerlines and morphological reconstruction. IEEE Trans Med Imag (2006) 25(9):1200–13. doi: 10.1109/tmi.2006.879955
26. Franklin SW, Rajan SE. An automated retinal imaging method for the early diagnosis of diabetic retinopathy. Technol Health Care (2013) 21(6):557–69. doi: 10.3233/thc-130759
27. Esmaeili M, Rabbani H, Dehnavi AM, Dehghani A. Automatic detection of exudates and optic disk in retinal images using curvelet transform. IET Image Process (2012) 6(7):1005. doi: 10.1049/iet-ipr.2011.0333
28. Akram UM, Khan SA. Automated detection of dark and bright lesions in retinal images for early detection of diabetic retinopathy. J Med Syst (2011) 36(5):3151–62. doi: 10.1007/s10916-011-9802-2
29. Wang Y, Yang J, Yang J, Zhao X, Chen Y, Yu W. Progress of artificial intelligence in diabetic retinopathy screening. Diabetes/Metab Res Rev (2020) 37(5):e3414. doi: 10.1002/dmrr.3414
30. Noronha K, Nayak KP. Automated diagnosis of maculopathy stages using entropies and hu’s invariant moments. J Med Imaging Health Inf (2013) 3(4):494–501. doi: 10.1166/jmihi.2013.1189
31. Waheed A, Waheed Z, Akram MU, Shaukat A. Removal of false blood vessels using shape based features and image inpainting. J Sens (2015) 2015:1–13. doi: 10.1155/2015/839894
32. Wu H, Zhang X, Geng X, Dong J, Zhou G. Computer aided quantification for retinal lesions in patients with moderate and severe non-proliferative diabetic retinopathy: A retrospective cohort study. BMC Ophthalmol (2014) 14(1):126. doi: 10.1186/1471-2415-14-126
33. Al-Rawi M, Qutaishat M, Arrar M. An improved matched filter for blood vessel detection of digital retinal images. Comput Biol Med (2007) 37(2):262–7. doi: 10.1016/j.compbiomed.2006.03.003
34. Chaudhuri S, Chatterjee S, Katz N, Nelson M, Goldbaum M. Detection of blood vessels in retinal images using two-dimensional matched filters. IEEE Trans Med Imaging (1989) 8(3):263–9. doi: 10.1109/42.34715
35. Cinsdikici MG, Aydın D. Detection of blood vessels in ophthalmoscope images using MF/ant (matched filter/ant colony) algorithm. Comput Methods Programs Biomed (2009) 96(2):85–95. doi: 10.1016/j.cmpb.2009.04.005
36. Hoover AD, Kouznetsova V, Goldbaum M. Locating blood vessels in retinal images by piecewise threshold probing of a matched filter response. IEEE Trans Med Imaging (2000) 19(3):203–10. doi: 10.1109/42.845178
37. Niu S, Yu C, Chen Q, Yuan S, Lin J, Fan W, et al. Multimodality analysis of hyper-reflective foci and hard exudates in patients with diabetic retinopathy. Sci Rep (2017) 7(1):1568. doi: 10.1038/s41598-017-01733-0
38. Cheung CY-L, Sabanayagam C, Law AK-P, Kumari N, Ting DS, Tan G, et al. Retinal vascular geometry and 6 year incidence and progression of diabetic retinopathy. Diabetologia (2017) 60(9):1770–81. doi: 10.1007/s00125-017-4333-0
39. Thanki R, Borra S. Application of machine learning algorithms for classification and security of diagnostic images. Mach Learn Bio-Signal Anal Diagn Imaging, Academic Press (2019) 273–92. doi: 10.1016/b978-0-12-816086-2.00011-4
40. Zago GT, Andreão RV, Dorizzi B, Teatini Salles EO. Retinal image quality assessment using deep learning. Comput Biol Med (2018) 103:64–70. doi: 10.1016/j.compbiomed.2018.10.004
41. Lee J-G, Jun S, Cho Y-W, Lee H, Kim GB, Seo JB, et al. Deep learning in medical imaging: General overview. Korean J Radiol (2017) 18(4):570. doi: 10.3348/kjr.2017.18.4.570
42. Shaban M, Ogur Z, Mahmoud A, Switala A, Shalaby A, Abu Khalifeh H, et al. A convolutional neural network for the screening and staging of diabetic retinopathy. PloS One (2020) 15(6):e0233514. doi: 10.1371/journal.pone.0233514
43. Galdran A, Chelbi J, Kobi R, Dolz J, Lombaert H, Ben Ayed I, et al. Non-uniform label smoothing for diabetic retinopathy grading from retinal fundus images with deep neural networks. Trans Vision Sci Technol (2020) 9(2):34. doi: 10.1167/tvst.9.2.34
44. Le D, Alam M, Yao CK, Lim JI, Hsieh Y-T, Chan RVP, et al. Transfer learning for automated OCTA detection of diabetic retinopathy. Trans Vision Sci Technol (2020) 9(2):35. doi: 10.1167/tvst.9.2.35
45. Wu H, Wei Y, Shang Y, Shi W, Wang L, Li J, et al. iT2DMS: A standard-based diabetic disease data repository and its pilot experiment on diabetic retinopathy phenotyping and examination results integration. J Med Syst (2018) 42(7):131. doi: 10.1007/s10916-018-0939-0
46. Lee EB, Wang SY, Chang RT. Interpreting deep learning studies in glaucoma: Unresolved challenges. Asia-Pac J Ophthalmol (2021) 10(3):261–7. doi: 10.1097/APO.0000000000000395
Keywords: artificial intelligence, diabetic retinopathy, bibliometric, CiteSpace, systematic analysis
Citation: Wang R, Zuo G, Li K, Li W, Xuan Z, Han Y and Yang W (2022) Systematic bibliometric and visualized analysis of research hotspots and trends on the application of artificial intelligence in diabetic retinopathy. Front. Endocrinol. 13:1036426. doi: 10.3389/fendo.2022.1036426
Received: 04 September 2022; Accepted: 17 October 2022;
Published: 31 October 2022.
Edited by:
Paulo Matafome, University of Coimbra, PortugalReviewed by:
Francisco Pereira, Instituto Politécnico de Coimbra, PortugalCopyright © 2022 Wang, Zuo, Li, Li, Xuan, Han and Yang. This is an open-access article distributed under the terms of the Creative Commons Attribution License (CC BY). The use, distribution or reproduction in other forums is permitted, provided the original author(s) and the copyright owner(s) are credited and that the original publication in this journal is cited, in accordance with accepted academic practice. No use, distribution or reproduction is permitted which does not comply with these terms.
*Correspondence: Zhiqiang Xuan, emhxeHVhbkBjZGMuemouY24=; Yongzhao Han, aGFueW9uZ3poYW8uMTk5QDE2My5jb20=; Weihua Yang, YmVuYmVuMDYwNkAxMzkuY29t
†These authors have contributed equally to this work and share first authorship
Disclaimer: All claims expressed in this article are solely those of the authors and do not necessarily represent those of their affiliated organizations, or those of the publisher, the editors and the reviewers. Any product that may be evaluated in this article or claim that may be made by its manufacturer is not guaranteed or endorsed by the publisher.
Research integrity at Frontiers
Learn more about the work of our research integrity team to safeguard the quality of each article we publish.