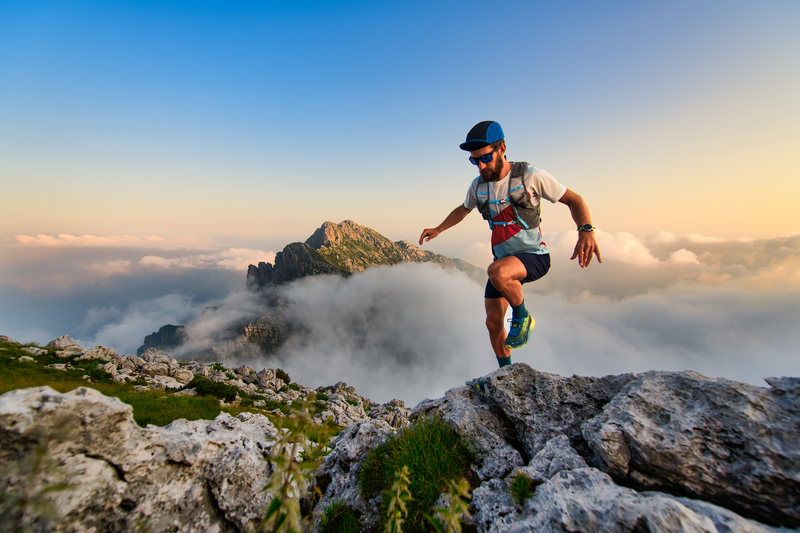
94% of researchers rate our articles as excellent or good
Learn more about the work of our research integrity team to safeguard the quality of each article we publish.
Find out more
ORIGINAL RESEARCH article
Front. Endocrinol. , 20 May 2021
Sec. Obesity
Volume 12 - 2021 | https://doi.org/10.3389/fendo.2021.654747
Aims: To explore associations between polymorphisms of IGF2-related genes including H19, IGF2, IGF2BP2 and IGF2R and Metabolic syndrome (MetS) susceptibility in the Chinese Han population.
Methods: 66 subjects with MetS and 257 control subjects were collected for inclusion in a case-control study. PCR-RFLP was used to investigate polymorphisms in the H19, IGF2, IGF2BP2 and IGF2R genes. Elisa was used to detect the serum IGF2 concentrations.
Results: Females carrying the GG and AG genotypes of rs680 (IGF2) exhibited a lower risk of MetS, compared with those harboring AA (adjusted OR = 0.388, p = 0.027), while GG and AG genotypes were associated with lower fasting glucose and HbA1c. In males, the Waist-to-Hip Ratio (WHR) and the level of TG were significantly higher in GG and AG genotypes than in the AA genotype of rs680 in IGF2. Levels of HDL-c were lower in men with GG and AG genotypes compared with those carrying the AA genotype. Serum IGF2 concentrations did not change among different genotypes. Finally, multifactor dimensionality reduction (MDR) analysis identified interactions between four polymorphisms: rs3741279 (H19), rs680 (IGF2), rs1470579 (IGF2BP2) and rs629849 (IGF2R).
Conclusions: Our study suggests that IGF2-related genes including H19, IGF2, IGF2BP2 and IGF2R genes may play pivotal roles in the development of MetS.
Metabolic syndrome (MetS) is a disorder that encompasses a group of symptoms including central obesity, hypertension, hyperglycemia and dyslipidemia (1). The incidence of MetS has increased widely in recent years and always parallels the incidence of obesity and type 2 diabetes (T2DM). According to the International Diabetic Federation (IDF) diabetes atlas, the prevalence of global diabetes was 8.8% in 2015 and is predicted to increase to 10.4% by 2040. Though it’s difficult to measure the global incidence of MetS, it is estimated that around one quarter of the world population has MetS, since MetS is about three times more common than diabetes (2). The 2010–2012 China National Nutrition and Health Survey (CNNHS) showed that the overall standardized prevalence of MetS was 24.2% in China during that time-period (3).
There is growing correlational evidence that polygenic inheritance may be an important underlying mechanism in the development of MetS. Multiple genetic studies have identified numerous mutations that are related to MetS; in particular, genome-wide association studies (GWASs) have maximally revealed MetS-related genetic variants (4–6).
IGF2 is a part of the insulin-like growth factor (IGF) system which is a complex composed of two peptide hormones (IGF-1 and IGF-2), two receptors (IGF-1R and IGF-2R) and six IGF binding proteins (IGFBP1-6) (7). The concentration of IGF2 is three times higher than that of IGF1, but our understanding of IGF2’s functions lags behind our understanding of IGF1 (8). Recent studies have identified IGF2’s role in diabetes and fatty liver (9, 10). With regard to glucose homeostasis, IGF2BP2 is reported to be correlated with serum insulin levels and fasting blood glucose levels (11, 12). Furthermore, GWAS identified a relationship between the variants of IGF2BP2 and risk of T2DM and its complications (13, 14). Similarly, IGF2R has been shown to play key roles in glucose metabolism (15, 16). Our previous work confirmed the associations between IGF2R and MetS (17). Long non-coding RNA H19 (H19) is an imprinted gene located downstream of IGF2 that regulates the transcription and translation of IGF2 (18, 19). H19 is a vital regulator of glucose and lipid metabolism in T2DM and obese individuals (20, 21). In view of the pivotal role of IGF systems and H19 in metabolic processes, it is probable that genetic variants of these genes may at least partially explain the occurrence of MetS.
In this study, we selected one or two common single nucleotide polymorphisms (SNP) of each of these genes (H19, IGF2, IGF2BP2 and IGF2R) and analyzed associations between these variants and the risk of MetS in the Chinese Han population. Additionally, we estimated the role of interactions between these genes in the risk of MetS.
This case-control study was approved by the ethics committee of Sir Run Run Shaw Hospital and was conducted from March to May 2010. The case participants and control participants in our study were recruited from community-based epidemiological studies of diabetes and related metabolic disorders. The study was carried out in the Caihe and Gongshu communities of Hangzhou, Zhejiang province, China. The inclusion criteria for MetS cases were in accordance with standards established by the Joint Committee for Developing Chinese Guidelines on Prevention and Treatment of Dyslipidemia in Adults (JCDCG) (22). This standard was established based on Chinese Han population’s demographic characteristics and was most suitable for our study samples. In brief, individuals with ≥3 of the following abnormalities were considered to have MetS: Central obesity [waist circumference (WC), >90 cm for men and >85 cm for women]; hypertriglyceridemia (≥1.70 mmol/l); high density lipoprotein cholesterol (HDL c; <1.04 mmol/l); elevated blood pressure (BP; ≥130/85 mmHg or current treatment for hypertension); and hyperglycemia [fasting plasma glucose (FPG) ≥6.1 mmol/l or 2 h postprandial glucose (2 h PG) ≥7.8 mmol/l]. All subjects had no previous history of cancer, cardiovascular disease, chronic inflammatory disease or renal disease. Among all the participants, 66 were diagnosed with Metabolic Syndrome (MetS) and 257 were non-MetS controls.
All participants underwent informed consent and were interviewed face-to-face by trained medical staff to collect participant demographic data, baseline lifestyle and health status data using a standardized questionnaire.
Participants who were not diagnosed as diabetic received a 75 g oral glucose tolerance test (OGTT), while participants who had previously been diagnosed as diabetic were administered a 100 g carbohydrate (steamed bread meal) test. Venous blood samples were obtained at 0 and 2 h after either OGTT or steamed bread meal test for subsequent biochemical tests including FPG, 2hPG, total cholesterol (TC), high density lipoprotein-cholesterol (HDL-c), low density lipoprotein-cholesterol (LDL-c), triglyceride (TG), uric acid (UA), urine albumin-to-creatinine ratio (UACR), serum creatinine (CREA), and serum urea nitrogen (BUN) using an autoanalyzer (Aeroset, Chicago, IL, USA). Blood samples for subsequent laboratory analyses were centrifuged immediately and the collected serum was stored at −80 °C. Glycosylated hemoglobin A1c (HbA1c) was measured by ion-exchange high-performance liquid chromatography (Hemoglobin Testing System; Bio-Rad, Hercules, CA, USA). Plasma insulin evels were measured using a radioimmunoassay kit (Beijing North Institute of Biological Technology, China). The homeostatic model assessment of insulin resistance (HOMA-IR) value was used to evaluate the level of insulin sensitivity and calculated as follows: fasting blood glucose (mmol/L) × fasting serum insulin (mU/L)/22.5 (23).
Physical examinations were carried out on all participants by physicians according to standard methods, including measurements of height, weight, waist, and blood pressure. Blood pressure was measured in triplicate and then averaged. Body mass index (BMI) was calculated by dividing body weight by height squared. MRI scans were performed at the level of the umbilicus between L4 and L5 with the subject in a supine position. Abdominal visceral fat area (VFA) and abdominal subcutaneous fat area (SFA) were calculated using SliceOmatic software (version 4.2).
Peripheral blood (5 ml) was collected from all MetS patients and healthy controls. Genomic DNA was extracted using a DNA extraction kit (Tiangen Biotech; Beijing, China) by following the manufacturer’s instructions and stored at −80°C until use.
The serum IGF2 concentrations were detected using an enzyme-linked immunosorbent (Elisa) assay kit (E-EL-H6037, Elabscience, China) according to the manufacturer’s protocol.
Several metabolism-related SNPs were chosen based on previous reports or bioinformatic analyses. The rs3741219 and rs217727 SNPs in the H19 gene were previously reported to be closely related to type 2 diabetes mellitus (T2DM) (24), while the rs1470579 SNP in the IGF2BP2 gene and the rs680 SNP in the IGF2 gene have been demonstrated to play pivotal roles in the development of T2DM (25), and the rs629849 SNP in the IGF2R gene was reported to be associated with obesity (26). Therefore, we selected these SNPs for analysis in our study. Genotyping of the selected SNPs was performed using PCR-RFLP. The primers designed for each SNP are listed in Supplementary Table 1. For each amplification reaction, we used 25 µl PCR reaction mixtures consisting of 10 µg genomic DNA, 5 pmol of each primer and 1× PCR mix, and we utilized reaction setting and annealing temperatures for each SNP as previously reported (27–29). All PCR products were digested with specific restriction enzymes and the fragments were resolved by electrophoresis on a 3% agarose gel (Figure 1). To confirm the PCR-RFLP genotyping results, 20% of the samples were randomly selected for repeated assays, and no discrepancies were detected between the repeat analyses.
Figure 1 PCR-RFLP analysis of five SNPs. (A) rs3741219 T > C in the H19 gene, (B) rs217727 C > T in H19 gene, (C) rs680 A > G in IGF2 gene, (D) rs1470579 A > C in IGF2BP2 gene, (E) rs629849 G > A in IGF2R gene.
A Hardy–Weinberg equilibrium (HWE) was evaluated to compare the observed and expected frequencies in MetS and non-MetS control subjects by means of a chi-squared (χ2) test. Only SNPs that passed this test (p >0.05) were included in the following analyses. All continuous variables are reported as the mean ± standard deviation (SD), and variables that are not normally distributed are expressed as median value (interquartile range). Standard’s-test was used to estimate differences in the distribution of demographic characteristics between case and control subjects for continuous variables, and Fisher’s exact test was performed for categorical variables. Logistic regression was used to evaluate the association between each SNP and the risk of MetS after adjusting for potential covariates including age, smoking and drinking status. A two-sided test with type error level (α) set at 5% was used in all statistical analyses.
Furthermore, multifactor dimensionality reduction (MDR) was used to analyze SNP-SNP interactions, cross-validation consistency (CVC), the testing balanced accuracy and the sign test. Considering the influence of linkage disequilibrium (LD) among SNPs of the same gene, we selected one SNP from each high LD pair (r2 >0.5). Results were regarded as significant when p <0.05. All statistical analyses were performed in SPSS (version 26.0 for Mac; SPSS Inc, Chicago, IL, USA).
The detailed clinical and biochemical parameters of all participants are described in Table 1. The mean age for MetS patients and non-MetS controls were 66.69 ± 8.71 and 62.84 ± 6.30 years, respectively. As expected, participants with MetS had more risk factors than non-MetS controls, including higher BMI, fasting glucose, 2 h plasma glucose, fasting insulin, 2 h serum insulin, HOMA-IR, HbA1c, blood pressure, triglycerides, HDL-c and levels of fat contents (p <0.05 for all participants). However, there were no significant differences in sex, smoking status, drinking status, LDL-c, CREA, or BUN between the two groups.
Considering that H19 and IGF2 are imprinted genes which are regulated and expressed in a sex-specific manner, we divided our data by sex in our follow-up analyses. Logistic regression analysis was performed to evaluate the association of the five SNPs with susceptibility to MetS using both dominant and additive models after adjusting for age, smoking and drinking status. Genotype frequencies of the five SNPs of both sexes are presented for MetS patients and healthy controls in Supplementary Table 2. In females, frequencies of the AA, AG and GG genotypes in the rs680 SNP were 35.03, 54.78 and 10.19% in the MetS group, and 59.38, 37.50 and 3.12% in the non-MetS control group, respectively, and this difference was statistically significant (p = 0.036). However, there were no obvious significant differences in genotype frequencies of other SNPs including rs3741219, rs217727, rs1470579 and rs629849 between MetS and non-MetS control samples. In males, there were no statistically significant differences between the two groups in genotype frequencies of any of the five SNPs. All genotype distributions for each group were in Hardy–Weinberg equilibrium.
Results of associations between SNPs and the risk of MetS in the females are shown in Table 2. There were no significant associations for rs3741219, rs217727 in the H19 gene, or for rs629849 in the IGF2R gene and rs1470579 in the IGF2BP2 gene for either sex. However, there was a significant association between the rs680 SNP of the IGF2 gene and the risk of MetS in females, though not in males (Supplementary Table 3). Compared to the AA genotype, females with the AG genotype exhibited a decreased risk of MetS in the additive model (crude OR = 0.404 (0.182–0.897), p = 0.026, adjusted OR = 0.388 (0.168–0.897), p = 0.027). When analyzed in a dominant model including GG and AG genotypes of rs680, a lower risk of MetS was observed compared with the homozygous variant AA genotype (crude OR = 0.369 (0.169–0.803), p = 0.012, adjusted OR = 0.340 (0.150–0.767), p = 0.01).
Table 2 Associations of all the SNPs of H19, IGF2, IGF2BP2 and IGF2R with risk of MetS in female samples.
We further analyzed the possible influences of polymorphism in the IGF2 gene on anthropometric and clinical features and serum IGF-II concentrations. In females, rs680 was significantly correlated with plasma glucose level under the dominant model. Compared to the AA genotype, the GG and AG genotypes were negatively correlated with fasting glucose levels (mmol/L) (4.94 (4.61, 5.51) vs 4.78 (4.50, 5.17), p = 0.022), and HbA1c (%) (5.70 (5.40, 6.03) vs 5.50 (5.30, 5.80), p = 0.043). Furthermore, the rs680 SNP was also significantly correlated with LDL-c levels: individuals carrying either the GG or AG genotype exerted lower LDL-c level than those carrying the AA genotype (2.42 ± 0.59 vs 2.62 ± 0.69, p = 0.040). However, no other significant correlations were observed between rs680 and clinical traits in females (Table 3). In males, individuals with the GG or AG genotype had statistically higher WHR and TG levels and lower HDL-c level than those with the AA genotype (Supplementary Table 4). Serum IGF2 quantitation was detected using IGF2 Elisa kit. The results showed that individuals with AA genotype had higher serum IGF2 concentrations than those with AG and GG genotypes (206.78±68.65 ng/mL vs 179.79±56.05 ng/mL vs 174.36±39.99 ng/mL). However, the difference did not reach the statistical significance (Supplementary Table 5).
To further evaluate the impact of SNP-SNP interaction among all the SNPs of H19, IGF2BP2, IGF2R and IGF2 on MetS risk, we performed an MDR analysis. The results obtained from the MDR analysis for two- to four-locus models are presented in Table 4. We found a significant three-locus model involving H19 (rs3741219), IGF2BP2 (rs1470579), and IGF2 (rs680) which had the highest testing accuracy (0.6075) and exhibited good CVC (10/10), indicating a potential SNP-SNP interaction. Additionally, a two-locus model containing IGF2BP2 (rs1470579) and IGF2 (rs680), and a four-locus model containing H19 (rs3741219), IGF2BP2 (rs1470579), IGF2 (rs680) and IGF2R (rs629849) also had high testing accuracy and good CVC.
Table 4 Best gene–gene interaction models of H19/IGF2/IGF2BP2/IGF2R pathway genes by the multifactor dimensionality reduction (MDR) in female samples.
Gene–environment interactions between SNPs and clinical characteristics of MetS were analyzed by MDR. The results obtained from the MDR analysis for one- to four-locus models are presented in Supplementary Tables 6–9. We found that blood glucose, blood pressure, WC and triglycerides were independent risk factors for MetS. Furthermore, we found a significant three-locus model involving H19 (rs3741219), IGF2 (rs680) and blood glucose which had the highest testing accuracy (0.7853) and exhibited good CVC (10/10). The three-locus model involving IGF2BP2 (rs1470579), IGF2 (rs680) and blood pressure had the highest testing accuracy (0.7574) and good CVC (10/10). As for WC, two-locus model involving H19 (rs3741219) and WC had high testing accuracy and good CVC. Finally, four-locus model involving H19 (rs3741219), IGF2BP2 (rs1470579), IGF2 (rs680) and triglycerides exhibited good CVC which indicated the SNPs-triglycerides interactions.
Metabolic syndrome (MetS) is regarded as a polygenic metabolic disorder accompanied by abnormal lipid and glucose metabolism (30). Few diseases are determined purely by a single genetic or environmental factor, especially chronic diseases. For this reason, if we want to understand disease susceptibility, it is necessary to study gene–gene or gene–environment interactions. In the case of MetS, however, most of the previous literature has focused on association studies exploring the contribution of single polymorphisms to the development of MetS. It’s more plausible to study the association between multiple genes in the same signaling pathway or the same system and the risk of MetS. In this study, we thus explored systematically the association between several IGF2-related genes and MetS susceptibility in the Chinese Han population for the first time. Our findings will help to detect the roles of IGF2-related genes including IGF2, IGF2BP2, IGF2R and H19 in the etiology of MetS. To increase the detection rate, we selected representative SNPs which have previously been reported to be related to the development of metabolic disease, such T2DM and obesity. In the present study, we identified the AG and GG genotypes of rs680 in the IGF2 gene as candidate resistant factors to the development of MetS in females. Although no effects were detected for SNPs in the H19, IGF2BP2 and IGF2R genes on the occurrence of MetS, interactions between H19, IGF2, IGF2BP2 and IGF2R genes on MetS were confirmed by MDR analyses.
There is growing evidence indicating that the IGF system plays an important role in glucose and lipid metabolism (31, 32). Furthermore, because concentrations of IGF vary with age, sex and pregnancy status (33), we analyzed our data according to gender. Interestingly, we found that, for women, but not for men, the GG and AG genotypes of rs680 in IGF2 were protective factors for MetS, and were negatively related with fasting glucose and HbA1c. However, in males, the GG and AG genotypes of rs680 in IGF2 were positively correlated with WHR and TG while negatively related with levels of HDL-c. Though several studies have previously revealed an association between IGF2 (rs680) and obesity, BMI or plasma glucose levels (34–36), these results were previously controversial, and the association between rs680 and the risk of MetS was still unclear. The controversy between the gender in our study might be due to the fact that IGF2 is an imprinted gene whose transcription is achieved by methylation of the differentially methylated region (DMR) on the maternal allele (8). Heijmans et al. (37) reported that the rs680 SNP in IGF2 is strongly related to methylation of multiple CpG sites within the IGF2 DMR, while another study reported that the variant allele is associated with higher serum IGF2 concentrations than the wide type allele in middle-aged men (34). IGF2 is regarded as an important regulator of adipocyte physiology and its high level is closely associated with being overweight (35, 38), which might partially explain our results. As for females, Gatford et al. (39) reported that pregnant women with variant allele of rs680 in IGF2 exhibited lower circulating IGF1 concentration than those with the wide type allele. However, this effect was not observed in non-pregnant women (39). IGF1 has been reported to play an important role in maintaining normal glucose homeostasis (40). Thus, we postulate that IGF2 polymorphism probably influences the IGF1 or IGF2 levels which ultimately regulate glucose metabolism. To confirm our speculation, we detected serum IGF2 levels using Elisa kit and we found a decreasing tendency in serum IGF2 concentrations of individuals with GG genotype, though the data did not have statistical significance. SD O'Dell et al. (34) reported that serum IGF2 level in males with GG was lower than those with AA genotype. But Rashad et al. (27) found no differences in serum IGF2 levels between GG and AA genotypes. The contradiction among these studies may be attribute to the different ethnicity. However, more experimental evidence is needed to confirm this finding.
Previous studies have reported relationships between H19 (rs217727), IGF2BP2 (rs1470579) and T2DM susceptibility (24, 25, 41). Yang et al. revealed that the minor allele “A” in rs629849 is associated with obesity in the Korean population (26). One puzzling aspect of our findings in this study is that we failed to find any statistically significant associations between these three polymorphisms and MetS susceptibility. This contradiction might be due to heterogeneity in samples and environmental factors between the studies, and our limited sample size.
IGF2BP2 was reported to regulate IGF2 mRNA translation via binding to IGF2 L3 5’ UTR as an essential IRES trans-acting factor (ITAF) (42, 43). H19 harbored four binding sites with IGF2BP2 mRNA (19) and shared the same loci with IGF2 which indicated the interactions among these three genes. MDR is a newly established approach mainly used to detect the combined effects of multiple factors in disease susceptibility. Traditional statistical methods like logistic regression analysis encounter dimension problems in studying gene–gene interactions which could be solved by the use of MDR. In our study, we showed through MDR analysis that there is an interplay among H19 (rs3741219), IGF2BP2 (rs1470579), and IGF2 (rs680), indicative of the interactive role of these polymorphisms in MetS occurrence, which is consistent with the abovementioned reports. In view of the significant associations between rs680 in IGF2 and risk of MetS in single locus analysis, we speculate that the IGF2 gene plays a major part in the interaction. Furthermore, we found the interactions between each SNPs and clinical characteristics of MetS.
There are some limitations in the present study. Firstly, our sample size was relatively small. Despite this, significant differences between groups were still detected and we believe that a larger sample size would further confirm our results. Secondly, the study was based on observational and as such was particularly vulnerable to potential biases such as information or selection bias. Thirdly, we did not measure the gene expression activities of each polymorphism. Finally, though we used MDR as a bioinformatics method for identifying gene–gene interactions, it wasn’t possible to quantitatively assess the disease risk of the genotype combinations. A new weighted risk score-based multifactor dimensionality reduction (WRSMDR) analysis seems like a good and feasible option for the assessment of disease risk in future studies (44).
In conclusion, our work systematically studied the association between polymorphisms in four genes (H19, IGF2, IGF2BP2 and IGF2R) with risk of MetS in the Chinese Han population. The IGF2 variant rs680 was negatively correlated with fasting glucose and HbA1c, and might be a promising protective variant for the risk of MetS in females. In males, we failed to find the association between the rs680 SNP in IGF2 and the risk of MetS. While GG genotype of IGF2 rs680 was positively correlated with WHR and TG, and negatively correlated with HDL-c. Moreover, this study confirmed that interactions occur among the H19, IGF2, IGF2BP2 and IGF2R genes, and supported a previously-reported correlation between this system and the risk of MetS. Our conclusions should be further evaluated with a larger size sample.
The original contributions presented in the study are included in the article/Supplementary Material. Further inquiries can be directed to the corresponding author.
WG: Conceptualization, methodology, investigation, and writing—original draft writing. JL: Methodology and investigation. XL: Conceptualization, methodology, and supervision. NS: Investigation and validation. YZ: Investigation and validation. BT: Conceptualization and methodology. HL: Conceptualization, supervision, writing—review and editing, and funding acquisition. All authors contributed to the article and approved the submitted version.
This work was supported by grants from the National Natural Science Foundation of China (81873653).
The authors declare that the research was conducted in the absence of any commercial or financial relationships that could be construed as a potential conflict of interest.
The Supplementary Material for this article can be found online at: https://www.frontiersin.org/articles/10.3389/fendo.2021.654747/full#supplementary-material
Supplementary Table 1 | PCR Primer sequences in gene polymorphisms.
Supplementary Table 2 | The Genotypic Frequency of Polymorphisms Between MetS and non-MetS Groups.
Supplementary Table 3 | Associations of all the SNPs of H19, IGF2, IGF2BP2 and IGF2R with risk of MetS in male samples.
Supplementary Table 4 | Associations of the IGF2 rs680 clinical traits in male samples.
Supplementary Table 5 | Associations of the IGF2 rs680 with serum IGF2 concentrations in the whole samples.
Supplementary Table 6 | Best gene–gene interaction models of H19/IGF2/IGF2BP2/IGF2R pathway genes and blood glucose by the multifactor dimensionality reduction (MDR) in female samples.
Supplementary Table 7 | Best gene–gene interaction models of H19/IGF2/IGF2BP2/IGF2R pathway genes and blood pressure by the multifactor dimensionality reduction (MDR) in female samples.
Supplementary Table 8 | Best gene–gene interaction models of H19/IGF2/IGF2BP2/IGF2R pathway genes and WC by the multifactor dimensionality reduction (MDR) in female samples.
Supplementary Table 9 | Best gene–gene interaction models of H19/IGF2/IGF2BP2/IGF2R pathway genes and triglycerides by the multifactor dimensionality reduction (MDR) in female samples.
1. Samson SL, Garber AJ. Metabolic Syndrome. Endocrinol Metab Clin North Am (2014) 43:1–23. doi: 10.1016/j.ecl.2013.09.009
2. Saklayen MG. The Global Epidemic of the Metabolic Syndrome. Curr Hypertens Rep (2018) 20:12. doi: 10.1007/s11906-018-0812-z
3. Li Y, Zhao L, Yu D, Wang Z, Ding G. Metabolic Syndrome Prevalence and its Risk Factors Among Adults in China: A Nationally Representative Cross-Sectional Study. PloS One (2018) 13:e0199293. doi: 10.1371/journal.pone.0199293
4. Locke AE, Kahali B, Berndt SI, Justice AE, Pers TH, Day FR, et al. Genetic Studies of Body Mass Index Yield New Insights for Obesity Biology. Nature (2015) 518:197–206. doi: 10.1038/nature14177
5. Shungin D, Winkler TW, Croteau-Chonka DC, Ferreira T, Locke AE, Magi R, et al. New Genetic Loci Link Adipose and Insulin Biology to Body Fat Distribution. Nature (2015) 518:187–96. doi: 10.1038/nature14132
6. Wagschal A, Najafi-Shoushtari SH, Wang L, Goedeke L, Sinha S, deLemos AS, et al. Genome-Wide Identification of microRNAs Regulating Cholesterol and Triglyceride Homeostasis. Nat Med (2015) 21:1290–7. doi: 10.1038/nm.3980
7. Denley A, Cosgrove LJ, Booker GW, Wallace JC, Forbes BE. Molecular Interactions of the IGF System. Cytokine Growth Factor Rev (2005) 16:421–39. doi: 10.1016/j.cytogfr.2005.04.004
9. Shapiro MR, Wasserfall CH, McGrail SM, Posgai AL, Bacher R, Muir A, et al. Insulin-Like Growth Factor Dysregulation Both Preceding and Following Type 1 Diabetes Diagnosis. Diabetes (2020) 69:413–23. doi: 10.2337/db19-0942
10. Kessler SM, Laggai S, Van Wonterg E, Gemperlein K, Muller R, Haybaeck J, et al. Transient Hepatic Overexpression of Insulin-Like Growth Factor 2 Induces Free Cholesterol and Lipid Droplet Formation. Front Physiol (2016) 7:147. doi: 10.3389/fphys.2016.00147
11. Wood AR, Jonsson A, Jackson AU, Wang N, van Leewen N, Palmer ND, et al. A Genome-Wide Association Study of IVGTT-Based Measures of First-Phase Insulin Secretion Refines the Underlying Physiology of Type 2 Diabetes Variants. Diabetes (2017) 66:2296–309. doi: 10.2337/db16-1452
12. Chen BH, Hivert MF, Peters MJ, Pilling LC, Hogan JD, Pham LM, et al. Peripheral Blood Transcriptomic Signatures of Fasting Glucose and Insulin Concentrations. Diabetes (2016) 65:3794–804. doi: 10.2337/db16-0470
13. Dai N, Zhao L, Wrighting D, Kramer D, Majithia A, Wang Y, et al. IGF2BP2/IMP2-Deficient Mice Resist Obesity Through Enhanced Translation of Ucp1 mRNA and Other mRNAs Encoding Mitochondrial Proteins. Cell Metab (2015) 21:609–21. doi: 10.1016/j.cmet.2015.03.006
14. Montesanto A, Bonfigli AR, Crocco P, Garagnani P, De Luca M, Boemi M, et al. Genes Associated With Type 2 Diabetes and Vascular Complications. Aging (Albany NY) (2018) 10:178–96. doi: 10.18632/aging.101375
15. Minchenko DO, Tsymbal DO, Davydov VV, Minchenko OH. Expression of Genes Encoding IGF1, IGF2, and IGFBPs in Blood of Obese Adolescents With Insulin Resistance. Endocr Regul (2019) 53:34–45. doi: 10.2478/enr-2019-0005
16. Villuendas G, Botella-Carretero JI, Lopez-Bermejo A, Gubern C, Ricart W, Fernandez-Real JM, et al. The ACAA-insertion/deletion Polymorphism At the 3’ UTR of the IGF-II Receptor Gene is Associated With Type 2 Diabetes and Surrogate Markers of Insulin Resistance. Eur J Endocrinol (2006) 155:331–6. doi: 10.1530/eje.1.02217
17. Xihua L, Shengjie T, Weiwei G, Matro E, Tingting T, Lin L, et al. Circulating miR-143-3p Inhibition Protects Against Insulin Resistance in Metabolic Syndrome Via Targeting of the Insulin-Like Growth Factor 2 Receptor. Transl Res (2019) 205:33–43. doi: 10.1016/j.trsl.2018.09.006
18. Nordin M, Bergman D, Halje M, Engstrom W, Ward A. Epigenetic Regulation of the Igf2/H19 Gene Cluster. Cell Prolif (2014) 47:189–99. doi: 10.1111/cpr.12106
19. Runge S, Nielsen FC, Nielsen J, Lykke-Andersen J, Wewer UM, Christiansen J. H19 RNA Binds Four Molecules of Insulin-Like Growth Factor II mRNA-binding Protein. J Biol Chem (2000) 275:29562–9. doi: 10.1074/jbc.M001156200
20. Zhang N, Geng T, Wang Z, Zhang R, Cao T, Camporez JP, et al. Elevated Hepatic Expression of H19 Long Noncoding RNA Contributes to Diabetic Hyperglycemia. JCI Insight (2018) 3(10):e120304. doi: 10.1172/jci.insight.120304
21. Geng T, Liu Y, Xu Y, Jiang Y, Zhang N, Wang Z, et al. H19 lncRNA Promotes Skeletal Muscle Insulin Sensitivity in Part by Targeting Ampk. Diabetes (2018) 67:2183–98. doi: 10.2337/db18-0370
22. Joint Committee for Developing Chinese guidelines on Prevention and Treatment of Dyslipidemia in Adults. [Chinese Guidelines on Prevention and Treatment of Dyslipidemia in Adults]. Zhonghua Xin Xue Guan Bing Za Zhi (2007) 35:390–419.
23. Matthews DR, Hosker JP, Rudenski AS, Naylor BA, Treacher DF, Turner RC. Homeostasis Model Assessment: Insulin Resistance and Beta-Cell Function From Fasting Plasma Glucose and Insulin Concentrations in Man. Diabetologia (1985) 28:412–9. doi: 10.1007/BF00280883
24. Ghaedi H, Zare A, Omrani MD, Doustimotlagh AH, Meshkani R, Alipoor S, et al. Genetic Variants in Long Noncoding RNA H19 and MEG3 Confer Risk of Type 2 Diabetes in an Iranian Population. Gene (2018) 675:265–71. doi: 10.1016/j.gene.2018.07.002
25. Rao P, Wang H, Fang H, Gao Q, Zhang J, Song M, et al. Association Between IGF2BP2 Polymorphisms and Type 2 Diabetes Mellitus: A Case-Control Study and Meta-Analysis. Int J Environ Res Public Health (2016) 13(5):574. doi: 10.3390/ijerph13060574
26. Yang SA. Association Between Exonic Polymorphism (Rs629849, Gly1619Arg) of IGF2R Gene and Obesity in Korean Population. J Exerc Rehabil (2015) 11:282–6. doi: 10.12965/jer.150239
27. Rashad NM, El-Shal AS, Abd Elbary EH, Abo Warda MH, Hegazy O. Impact of Insulin-Like Growth Factor 2, Insulin-Like Growth Factor Receptor 2, Insulin Receptor Substrate 2 Genes Polymorphisms on Susceptibility and Clinicopathological Features of Hepatocellular Carcinoma. Cytokine (2014) 68:50–8. doi: 10.1016/j.cyto.2014.02.006
28. Xia Z, Yan R, Duan F, Song C, Wang P, Wang K. Genetic Polymorphisms in Long Noncoding RNA H19 Are Associated With Susceptibility to Breast Cancer in Chinese Population. Medicine (Baltimore) (2016) 95:e2771. doi: 10.1097/MD.0000000000002771
29. Huang Q, Yin JY, Dai XP, Pei Q, Dong M, Zhou ZG, et al. IGF2BP2 Variations Influence Repaglinide Response and Risk of Type 2 Diabetes in Chinese Population. Acta Pharmacol Sin (2010) 31:709–17. doi: 10.1038/aps.2010.47
30. Eckel RH, Grundy SM, Zimmet PZ. The Metabolic Syndrome. Lancet (2005) 365:1415–28. doi: 10.1016/S0140-6736(05)66378-7
31. Shapiro MR, Atkinson MA, Brusko TM. Pleiotropic Roles of the Insulin-Like Growth Factor Axis in Type 1 Diabetes. Curr Opin Endocrinol Diabetes Obes (2019) 26:188–94. doi: 10.1097/MED.0000000000000484
32. Adamek A, Kasprzak A. Insulin-Like Growth Factor (IGF) System in Liver Diseases. Int J Mol Sci (2018) 19(5):1308. doi: 10.3390/ijms19051308
33. Yu H, Mistry J, Nicar MJ, Khosravi MJ, Diamandis A, van Doorn J, et al. Insulin-Like Growth Factors (IGF-I, Free IGF-I and IGF-II) and Insulin-Like Growth Factor Binding Proteins (IGFBP-2, IGFBP-3, IGFBP-6, and ALS) in Blood Circulation. J Clin Lab Anal (1999) 13:166–72. doi: 10.1002/(SICI)1098-2825(1999)13:4<166::AID-JCLA5>3.0.CO;2-X
34. O’Dell SD, Miller GJ, Cooper JA, Hindmarsh PC, Pringle PJ, Ford H, et al. Apal Polymorphism in Insulin-Like Growth Factor II (IGF2) Gene and Weight in Middle-Aged Males. Int J Obes Relat Metab Disord (1997) 21:822–5. doi: 10.1038/sj.ijo.0800483
35. Roth SM, Schrager MA, Metter EJ, Riechman SE, Fleg JL, Hurley BF, et al. IGF2 Genotype and Obesity in Men and Women Across the Adult Age Span. Int J Obes Relat Metab Disord (2002) 26:585–7. doi: 10.1038/sj.ijo.0801927
36. Queiroz EM, Candido AP, Castro IM, Bastos AQ, Machado-Coelho GL, Freitas RN. IGF2, LEPR, POMC, PPARG, and PPARGC1 Gene Variants are Associated With Obesity-Related Risk Phenotypes in Brazilian Children and Adolescents. Braz J Med Biol Res (2015) 48:595–602.
37. Heijmans BT, Kremer D, Tobi EW, Boomsma DI, Slagboom PE. Heritable Rather Than Age-Related Environmental and Stochastic Factors Dominate Variation in DNA Methylation of the Human IGF2/H19 Locus. Hum Mol Genet (2007) 16:547–54. doi: 10.1093/hmg/ddm010
38. Alfares MN, Perks CM, Hamilton-Shield JP, Holly JMP. Insulin-Like Growth factor-II in Adipocyte Regulation: Depot-Specific Actions Suggest a Potential Role Limiting Excess Visceral Adiposity. Am J Physiol Endocrinol Metab (2018) 315:E1098–E107. doi: 10.1152/ajpendo.00409.2017
39. Gatford KL, Heinemann GK, Thompson SD, Zhang JV, Buckberry S, Owens JA, et al. Circulating IGF1 and IGF2 and SNP Genotypes in Men and Pregnant and Non-Pregnant Women. Endocr Connect (2014) 3:138–49. doi: 10.1530/EC-14-0068
40. Clemmons DR. Role of Insulin-Like Growth Factor Iin Maintaining Normal Glucose Homeostasis. Horm Res (2004) 62 Suppl 1:77–82. doi: 10.1159/000080763
41. Vatankhah Yazdi K, Kalantar SM, Houshmand M, Rahmanian M, Manaviat MR, Jahani MR, et al. SLC30A8, CDKAL1, TCF7L2, KCNQ1 and IGF2BP2 are Associated With Type 2 Diabetes Mellitus in Iranian Patients. Diabetes Metab Syndr Obes (2020) 13:897–906. doi: 10.2147/DMSO.S225968
42. Dai N, Rapley J, Angel M, Yanik MF, Blower MD, Avruch J. mTOR Phosphorylates IMP2 to Promote IGF2 mRNA Translation by Internal Ribosomal Entry. Genes Dev (2011) 25:1159–72. doi: 10.1101/gad.2042311
43. Mu Q, Wang L, Yu F, Gao H, Lei T, Li P, et al. Imp2 Regulates GBM Progression by Activating IGF2/PI3K/Akt Pathway. Cancer Biol Ther (2015) 16:623–33. doi: 10.1080/15384047.2015.1019185
Keywords: metabolic syndrome, single nucleotide polymorphisms, H19, IGF2, IGF2BP2
Citation: Gui W, Liang J, Lin X, Shi N, Zhu Y, Tan B and Li H (2021) Association of Genetic Variants in IGF2-Related Genes With Risk of Metabolic Syndrome in the Chinese Han Population. Front. Endocrinol. 12:654747. doi: 10.3389/fendo.2021.654747
Received: 17 January 2021; Accepted: 15 April 2021;
Published: 20 May 2021.
Edited by:
Luca Busetto, Università degli Studi di Padova, ItalyReviewed by:
Monica D’Adamo, University of Rome Tor Vergata, ItalyCopyright © 2021 Gui, Liang, Lin, Shi, Zhu, Tan and Li. This is an open-access article distributed under the terms of the Creative Commons Attribution License (CC BY). The use, distribution or reproduction in other forums is permitted, provided the original author(s) and the copyright owner(s) are credited and that the original publication in this journal is cited, in accordance with accepted academic practice. No use, distribution or reproduction is permitted which does not comply with these terms.
*Correspondence: Hong Li, c3Jyc2huZm1Aemp1LmVkdS5jbg==
†These authors have contributed equally to this work
Disclaimer: All claims expressed in this article are solely those of the authors and do not necessarily represent those of their affiliated organizations, or those of the publisher, the editors and the reviewers. Any product that may be evaluated in this article or claim that may be made by its manufacturer is not guaranteed or endorsed by the publisher.
Research integrity at Frontiers
Learn more about the work of our research integrity team to safeguard the quality of each article we publish.