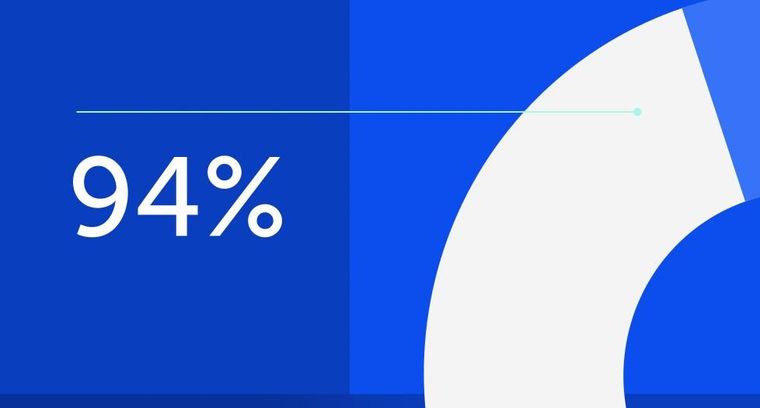
94% of researchers rate our articles as excellent or good
Learn more about the work of our research integrity team to safeguard the quality of each article we publish.
Find out more
ORIGINAL RESEARCH article
Front. Educ., 16 April 2025
Sec. Digital Learning Innovations
Volume 10 - 2025 | https://doi.org/10.3389/feduc.2025.1578451
This article is part of the Research TopicHarnessing Generative AI for Inclusive Education: Opportunities and ChallengesView all 3 articles
Artificial intelligence (AI) has become a key factor in higher education, revolutionizing both teaching methods and student learning. This study aimed to identify the perceptions of university students and professors on the use of AI in academic education. A descriptive study with a quantitative approach was conducted using the deductive method and a Likert scale questionnaire. The results revealed a predominantly low perception of AI in university teaching, with 71.5% of students and 73.1% of professors expressing skepticism about its application. Additionally, 59% of students and 65.9% of professors evaluated the impact of AI on the learning process unfavorably, indicating concerns about its effectiveness in enhancing education. Ethical and privacy considerations showed a contrast, with 84% of students displaying high confidence while 73.2% of professors maintaining moderate trust. These findings highlight a perception gap and a lack of confidence in assessing the impact of AI, emphasizing the urgent need for training programs, comprehensive policies, and evaluation methodologies. Addressing these concerns is crucial for harnessing AI’s potential in higher education and preparing students and professors for an increasingly digitized academic environment.
Artificial intelligence (AI) is a key element in the transformation of higher education, influencing both teaching methods and students’ learning experiences (Chen et al., 2020). In countries with advanced economies, AI applications have been progressively integrated into educational environments, demonstrating a potential to personalize teaching and improve learning effectiveness (Tahiru, 2021). These applications include learning platforms that adapt content according to the needs of individual learners, automated assessment systems, and AI-assisted writing tools that provide feedback on aspects such as grammar, punctuation, and style (Barrot, 2024; Holmes and Tuomi, 2022; Zawacki-Richter et al., 2019).
Research in educational AI has taken hold in academia, benefiting students, professors, and institutions (Chen et al., 2023; Hwang et al., 2020; Nemorin et al., 2023). AI has transformed traditional models toward personalized and adaptive data-driven approaches (Cota-Rivera et al., 2024). It has become a driver of innovation, transforming pedagogy, administration, student outcomes, and educational inclusion (Lee et al., 2024). Its benefits reach both students and educators (Al Dhaen, 2022).
The use of generative AI software, such as ChatGPT, Scribe, DALL-E2, and Wordtune, has increased exponentially in academia (Summers et al., 2024). However, their easy accessibility has led to debates about the impact they may have on the quality of learning in higher education (Kasneci et al., 2023; Mustofa et al., 2025). Among the most discussed aspects are the veracity of the content generated, the contribution to more effective learning, their influence on personal development, and the implications for future employability (Chan and Hu, 2023). ChatGPT was quickly adopted by the general public and reached 100 million users within the first 2 months after its public launch, making it the fastest-growing consumer app in history (Hu, 2023; Lee et al., 2024).
Society has enthusiastically embraced the advancement of AI for its ability to optimize operations and improve productivity (Bughin et al., 2018; Hashmi and Bal, 2024). Beyond the incorporation of technology, education must develop AI literacy for responsible and critical use (Tayan et al., 2024). This concept encompasses understanding AI from a technical point of view and its ethical use (Abdelghani et al., 2023; Crabtree, 2023; Dong, 2024; Hwang et al., 2023), linking to digital literacy that combines creativity, curiosity, and problem-solving (Law et al., 2018), and enables the safe use of technology in education (Antonietti et al., 2022; Paetsch et al., 2023).
Studies support the development of AI educational solutions tailored to local contexts and learners’ needs (Stöhr et al., 2024). Academia, as a center of knowledge dissemination, is analyzing the implications of AI (Hashmi and Bal, 2024), with various reactions to generative AI reflecting societal debates (Chiu, 2024). Institutional responses have been divided, with some universities choosing to ban its use due to concerns about academic ethics and potential misuse in assignments and assessments (Kelion, 2018). In contrast, other institutions have adopted a more inclusive stance, recognizing AI as an educational opportunity. A notable example is the Massachusetts Institute of Technology (MIT), which has incorporated AI into its curriculum, allowing students to experiment with its capabilities and limitations (Ackerman, 2021; Hashmi and Bal, 2024).
There is currently no comprehensive framework for integrating AI into academia (Bowles and Kruger, 2023), which necessitates a cultural evolution that recognizes and adapts AI to pedagogical approaches (Castelli and Manzoni, 2022; Cooper, 2023). This entails training in the responsible use of AI (Chiu, 2023), and the development of standards for its appropriate application (Chiu et al., 2023). In this scenario, the article analyses the perceptions of students and professors on the incorporation of AI in academic training to foster a methodological and curricular transformation. This includes the development of a regulatory framework that encourages the professor to adopt AI tools, discourages their prohibition, and promotes their ethical use.
The incorporation of AI in education has been taking place for approximately 60 years and has been transforming the way we relate to our environment (Tzirides et al., 2023, 2024). The development of AI in education has increased its importance worldwide (Yao and Wang, 2024). Applications of AI technologies are present in our daily lives, especially in the context of education (Zhang and Aslan, 2021). The application of AI technology in educational settings has been considered as one of the most significant developments (Zawacki-Richter et al., 2019).
Universities must provide up-to-date learning tools that respond to the changing technological environment (Bearman et al., 2023; Lee et al., 2024). AI training will be fundamental to professional preparation (Thurzo et al., 2023), while its application can foster more equitable education (McGrath et al., 2023). Curriculum and pedagogical practices require updating in the face of this changing landscape (Thurzo et al., 2023), with particular attention to the ethical use of generative AI tools (Michel-Villarreal et al., 2023). This ethical integration enhances learning, critical thinking, and information valuation (Summers et al., 2024), contributing to capacity building (Lim et al., 2023), and facilitating personalized experiences (Summers et al., 2024) that enhance knowledge and informed decision-making (Sharma and Sharma, 2023).
University faculty tend to resist the adoption of new technologies, including AI, because of the need to transform their teaching practices (Crawford et al., 2023; McGrath et al., 2023). While some perceive it as an opportunity, others see it as a threat, especially because of the risk of it supplanting their work (Al Dhaen, 2022; Bearman et al., 2023; Lee et al., 2024; McGrath et al., 2023; Schiff, 2021; Zhai et al., 2021). In higher education, there are concerns that AI facilitates plagiarism, harms scientific output, and disseminates biased or erroneous information (Alhaidry et al., 2023; Bozkurt, 2023; Choi et al., 2023; Peres et al., 2023; Shoja et al., 2023). In addition, ethical and privacy issues arise (Crawford et al., 2023; Lodge et al., 2023). Despite these challenges, many educators are incorporating AI into their pedagogical application, and others are hesitant to integrate it (Al Dhaen et al., 2022; Choi et al., 2023; Murugesan and Cherukuri, 2023).
Many professors believe that AI still lacks precision for application in teaching and learning, as it is still in a developmental phase (McGrath et al., 2023). Adoption varies by user; for example, females report lower AI proficiency than males (Lee et al., 2024; McGrath et al., 2023), and non-native learners or learners with marked accents may have difficulty using speech recognition technologies, which affects communication (Wang et al., 2023). Given these challenges, educators must adjust teaching and assessment to optimize learning and avoid misuse of generative AI (Alhaidry et al., 2023; Pearce and Chiavaroli, 2023). To this end, it is key for universities to implement policies and promote research on the ethical implications of AI (Bearman et al., 2023). Given the constant advancement of technology, it is critical to prepare both students and faculty to meet the challenges posed by the incorporation of AI in higher education (Crawford et al., 2023).
Higher education has reacted in various ways to generative artificial intelligence (GenAI), such as ChatGPT and DALL-E (Douglas, 2023). Initially, many institutions banned it as a malpractice that should be sanctioned (Douglas, 2023). Over time, several incorporated it to enhance learning rather than limit it (Hashmi and Bal, 2024). This divide forces educators to balance the ethical use of AI with its value in professional training. As a foundation for educating ethical students and professionals, higher education faces challenges and opportunities with GenAI (Chiu, 2024). However, the current debate prioritizes policy formulation over researching the future of education. Analyze its impact on pedagogy, assessment, and learning outcomes (Chiu, 2024).
The urgency of reforming educational institutions is evident, given their fundamental role in preparing future professionals to face and thrive in a more competitive work environment. However, there is still limited analysis on how academia should be transformed to focus on the most relevant competencies in this new era of AI. This study aims to bridge that gap by providing insight into student and faculty perceptions of the use of AI in university education. Although the potential of AI to revolutionize teaching is widely recognized, it is worth questioning whether it has truly ushered in a new pedagogical paradigm. Despite advances, its integration into classrooms remains partial due to concerns about privacy and information security, lack of training, inequalities in technological access, scarcity of resources, and fear of reduced human interaction. To maximize the benefits of AI in education, it is critical to overcome these challenges through a holistic approach that combines technological innovation with ethical and pedagogical considerations.
This study adopted a descriptive, non-experimental design with a quantitative approach. This methodology responds to the purpose of identifying and analyzing the perceptions of students and professors on the use of AI in university education. The method adopted was deductive, using the survey technique, applying the questionnaire on a Likert scale (1-Strongly Disagree; 2-Disagree; 3-Neutral; 4-Agree; 5-Strongly Agree). The questionnaire design was organized around six key competencies related to AI in higher education: (1) Applications of AI in teaching (Holmes et al., 2019), (2) Impact on the learning process (Zawacki-Richter et al., 2019), (3) Perceptions and attitudes of the actors involved (Huang et al., 2021; Lee et al., 2024), (4) Ethical and privacy aspects (Regan and Jesse, 2019), (5) Challenges and limitations (Akinwalere and Ivanov, 2022; Crompton and Burke, 2023); and, (6) Evaluation and measurement of impact (Hwang et al., 2020; Ouyang and Jiao, 2021). This structure, based on the existing literature and the objectives of the study, has allowed an approach to the different dimensions of the use of AI in university education, both in its practical and ethical aspects.
The survey was validated using the judgment of three experts according to the methodology, who provided their comments for a correct formulation of the questionnaire; Cronbach’s alpha was also used, where a value of 0.873 was obtained, which demonstrates a high level of reliability and internal consistency in the measurement of the study variables.
The research was conducted at the Universidad Nacional Toribio Rodríguez de Mendoza de Amazonas during the 2024-II academic semester. The survey was sent through the institutional e-mails of professors and students between August and November, with the participation of the university’s Information Technology Office. A total of 41 professors and 144 students responded to the survey. This sample reflected the distribution of students and professors in terms of gender, academic level, and area, providing a solid basis for the analysis of perceptions about the use of AI in university education (Table 1).
Data analysis was carried out with descriptive statistical methods using the Statistical Package for the Social Sciences (SPSS), version 26. This technique allowed for an in-depth analysis of the responses, which facilitated the understanding of perceptions about the use of AI in university teaching. Frequency analysis was used to obtain a complete picture of professors’ and student’s opinions.
The results obtained from the survey on a Likert scale were transformed into three levels (high, medium, and low) to have a better interpretation of the results. For this purpose, the number of items for each competence was determined to obtain the interval between levels.
This competency refers to the knowledge and understanding of the various ways in which AI is used in the educational context, including intelligent tutoring systems, automated assessment, adaptive content, and virtual assistants for professors and students (Holmes et al., 2019). Figure 1 reflects the main applications of AI in education.
The results reveal a predominantly low perception of 71.5% among students and 73.1% among professors regarding the application of AI in university teaching, suggesting a general distrust of its benefits in the educational context. This low acceptance could indicate a significant gap between the benefits of AI and the practical experiences of the participants, as well as a possible resistance to change in traditional teaching methods. The consistency in percentages between the two groups points to systemic challenges in the adoption of AI in higher education, possibly stemming from a lack of exposure to effective applications, doubts about the real ability of AI to personalize learning, or a lack of confidence in the technology to improve academic performance. These findings underline the urgent need for training and familiarization programs on AI applications in education for both students and professors and suggest that low awareness could represent a significant barrier to the successful implementation of AI technologies in the academic environment.
This competence encompasses an understanding of how AI influences the way students acquire knowledge and skills, including personalizing learning, improving information retention, and developing critical thinking skills (Zawacki-Richter et al., 2019). Figure 2 shows how they perceive the influence of AI on the learning process.
Figure 2. Perception of the impact of AI on the learning process. Data was obtained from the survey application.
The data show a worrying trend, 59% of students and 65.9% of professors rate the impact of AI on the learning process unfavorably. This negative perspective suggests a widespread questioning of the effectiveness of AI tools in enhancing the educational experience. The disparity between students and professors, although slight, may indicate that the former are more cautious, perhaps due to less forceful direct experiences or a greater reluctance to modify their established study methods. Participants are unaware of AI’s ability to personalize content, improve analytical reasoning, and foster autonomy in learning. This opinion could be influenced by the lack of successful experiences or by poor implementation of these technologies at the university.
This underlines the urgency of demonstrating practically and convincingly the benefits of AI in real educational contexts. In addition, they point to the possible existence of a discrepancy between the theories of these technologies and their actual implementation in university classrooms. This situation poses significant challenges for those responsible for educational innovation and technology development, who will need to address these perceptions to achieve successful integration of AI in higher education.
This competency focuses on the ability to understand and evaluate options, expectations, and concerns of students, faculty, and administrators with the implementation and use of AI in higher education (Huang et al., 2021; Lee et al., 2024). Figure 3 shows the perceptions and attitudes of the actors involved with the implementation of AI in higher education.
Figure 3. Perception of the attitudes of the actors involved in the use of AI. Data was obtained from the survey application.
The results show an interesting distribution in perceptions of AI in higher education, with 66.6% of students showing a medium level perception of the actors’ attitudes, while, among professors, 46.3% are also at a medium level, with 34.2% at a low level. This partial convergence at the mean level suggests a cautious but not completely negative attitude toward AI in both groups. However, the significant proportion of professors with low perception indicates greater polarization in this group. These data could reflect a combination of expectations and reservations among students, possibly due to limited but promising exposure to AI applications. On the other hand, the sharper division among professor could stem from varied experiences or concerns about how AI will affect their roles and teaching methods. This distribution underscores the need for training and practical demonstration programs that address the different perspectives and concerns of both groups, with a particular focus on allaying fears and skepticism among more reticent professors.
This competency involves understanding the ethical implications of using AI in education, including data privacy issues, equity in access and use of technology, and potential biases in AI systems (Regan and Jesse, 2019). Figure 4 presents perceptions regarding the main ethical and privacy issues related to the use of artificial intelligence in education.
Figure 4. Perception of ethical and privacy aspects in the use of AI. Data was obtained from the survey application.
The data reveal a marked consensus in perceptions of ethical trust and privacy in the use of AI at the university level. A striking 84% of students show a high level of trust, suggesting a positive and open attitude toward the ethical application of AI in their education. On the contrary, the professor presents a more cautious stance, 73.2% show a medium level of perception, indicating a more reserved or ambivalent attitude. Surprisingly, only 19.5% of faculty perceive a high level of ethical trust and privacy in the use of AI for university professional development. This disparity between students and faculty reflects different levels of knowledge or understanding of the ethical implications of AI, or different doubts about its impact on privacy and academic integrity. The high confidence of students could be due to a greater familiarity with the technology, while faculty caution could be influenced by a greater awareness of potential ethical issues. These results indicate the need for further dialog about the ethical implications of AI in education and specific training programs for the professor to address their concerns and increase their confidence in the ethical use of these technologies.
It refers to the ability to identify and address potential barriers to the implementation and effective use of AI in higher education, such as the digital divide, resistance to change, and constraints (Akinwalere and Ivanov, 2022; Crompton and Burke, 2023). Figure 5 provides a representation of student and faculty perceptions of the main challenges and limitations of AI implementation in higher education.
Figure 5. Perception of the challenges and limitations in the use of AI. Data was obtained from the survey application.
The results show a significant disparity in the perception of the challenges and limitations of using AI in the university context. While the majority of students (54.2%) perceive a low level of limitations and challenges, followed by 38.9% with a medium level, professors perceive a more cautious perspective, with 43.9% perceiving a medium level of challenges and 34.1% a high level. This difference indicates that students have a more optimistic view of AI integration in their education, underestimating potential obstacles, while professors are more aware or concerned about the potential challenges of AI application in university professional development. This difference in perceptions could be attributed to different levels of exposure to the technology, different responsibilities in the educational process, or a deeper understanding by professors of the pedagogical challenges associated with integrating new technologies into the curriculum. This demonstrates the need to align expectations for the effective implementation of AI in higher education.
This competency consists of the ability to design and apply assessment methods that effectively measure the impact of AI tools on learning outcomes, student satisfaction, and university educational efficiency (Hwang et al., 2020; Ouyang and Jiao, 2021). Figure 6 shows the perceptions of the main approaches to assessing and measuring the impact of AI tools in higher education.
Figure 6. Perception of the evaluation and measurement of the impact of the use of AI. Data was obtained from the survey application.
The results of the evaluation and measurement of the impact of the use of AI in university education reveal a predominantly low perception among the academic community. The majority of students (61.1%) perceive a low level of assessment and measurement of impact, suggesting a lack of understanding of how the effectiveness of AI in their education is being evaluated. On the other hand, faculty show a slightly more optimistic but still cautious outlook, with 53.7% perceiving a medium level and 43.9% a low level. This distribution would reflect a greater awareness on the part of professors of evaluation efforts, although still insufficient. The absence of a structured plan for the use of AI in the university and the lack of clear mechanisms to measure its impact are factors in these low perceptions. Furthermore, the worrying overuse of AI in the university community without clear guidance suggests an urgent need to develop comprehensive policies and strategies for the implementation and evaluation of AI use in the academic environment, thus ensuring a more systematic and beneficial approach for the entire educational community.
The results of the study reflect a predominantly low perception of the application of Artificial Intelligence (AI) in university education, with 71.5% of students and 73.1% of professors expressing a low perception of its advantages. These results are consistent with the statements of Lee et al. (2024) and Arowosegbe et al. (2024), who also observed a very generalized distrust toward the educational value of AI at the university level. This similarity in percentages between students and faculty suggests that resistance to AI in higher education is a phenomenon that transcends roles within the institution. This degree of perception could be rooted in a combination of factors, such as lack of exposure to successful examples of AI applications, concern about the loss of the human dimension in education, and fear of the loss of traditional teaching and learning techniques.
This cautious attitude may be related to the concerns noted by Chen et al. (2023) and Holmes and Tuomi (2022) about academic integrity and ethical issues associated with the use of AI in education. The potential for AI to facilitate plagiarism or impair the authenticity of academic work is a prominent concern. Furthermore, as García-Chitiva et al. (2024) and Chung (2024) point out, the lack of appropriate support and training for effective AI applications may contribute to these negative perceptions. This training gap not only affects the technical capacity to use AI tools but may also aggravate fears and misunderstandings about their potential impact on the quality of education. The absence of clear institutional guidelines and policies on the ethical use of AI in academic contexts may also be fueling this mistrust.
The study revealed a negative evaluation of the impact of AI on the learning process by both students and professors. These results are consistent with the statements of Damasevicius and Sidekerskiene (2024) and Sathish et al. (2023), who highlight uncertainty and lack of consensus among faculty on best practices related to AI technological advances in higher education. This lack of consensus may be due to the rapid evolution of AI technologies, which exceeds the ability of educational institutions to develop and implement consistent policies and practices. In addition, the difference in higher education disciplines and methods complicates the adoption of a homogeneous approach to AI integration.
Furthermore, concerns expressed by study participants about the potential for AI to generate inaccurate information and evade plagiarism detection systems meet the warnings of Vasudevan et al. (2024) about the challenges posed by the use of tools such as ChatGPT in educational settings. These concerns are not unfounded, as generative AI, while advanced, can still produce erroneous or biased information. The speed with which these tools can generate convincing text poses significant challenges to methods of assessing and verifying the authenticity of student work. This highlights the need to develop new assessment strategies and teaching methods that foster critical thinking and information synthesis skills.
The distribution of perceptions of AI in higher education, showing a trend toward a medium level among both students and faculty, reflects a cautious but not completely negative attitude. This finding is in line with the findings of Alfarsi et al. (2020) and Alzahrani (2023), who stress the need for more research to address the challenges and improve the levels of acceptance of AI among educators and students. This middle ground suggests that there is an open path for education and demonstration of the potential benefits of AI in education. It also indicates that, while there are reservations, there is also an openness to the possibility that AI can improve educational processes if applied appropriately and ethically.
Lee and You (2024) and Quinde et al. (2024) also highlight the importance of understanding these perceptions in developing effective applications of AI in educational settings. In this regard, the work of Kim et al. (2024) on student-AI interaction provides an example of how student attitudes toward AI can influence the effectiveness of AI-based interventions. This study highlights the importance of taking into account individual differences and students’ attitudes when designing and implementing AI solutions in education, suggesting that a personalized approach, taking into account students’ prior attitudes and skills, could be more effective than a uniform implementation of AI in all educational contexts.
Importantly, in line with Hashmi and Bal (2024) and Chiu (2024), the emergence of generative AI, such as ChatGPT, has created both opportunities and challenges for the traditional model of education. The study highlights the need to address concerns, provide adequate support, and foster awareness among educators and students about the capabilities and limitations of AI to ensure its effective integration into the learning environment. This involves not only technical training in the use of AI tools but also education about their ethical implications, limitations, and potential to transform teaching and learning processes. In addition, it is essential to develop clear regulatory frameworks and ethical guidelines for the use of AI in higher education that address concerns about privacy, equity, and academic integrity.
Regarding the use of AI in university education, students and decent students express their distrust about the positive impact, which reflects a general distrust in its educational value (Arowosegbe et al., 2024; Lee et al., 2024; Quinde et al., 2024). Although some participants use AI tools in teaching roles and recognize their potential for improvement, there are concerns about academic integrity, ethical issues, and adequate training for effective implementation (Chung, 2024; García-Chitiva et al., 2024; Lee et al., 2024; Lee and Shim, 2024). While the potential of AI to improve learning experiences is recognized, the results show that a significant part of the university community remains hesitant to adopt AI as a pedagogical strategy, highlighting the importance of addressing concerns and providing comprehensive support to foster a more positive perception and effective integration of AI tools in higher education.
The data reveals a worrying trend in which students and professors express a negative evaluation of the impact of AI on the learning process. This sentiment is supported by findings that highlight the ambiguity and lack of consensus among teaching staff regarding best practices related to recent technological advances in AI within Higher Education (Damasevicius and Sidekerskiene, 2024; Sathish et al., 2023; Supelette, 2023). Additionally, the ChatGPT study highlights potential challenges, such as the risk of generating inaccurate information and evading plagiarism detection systems, which could contribute to unfavorable perceptions of AI in education (Vasudevan et al., 2024). These results underscore the importance of addressing concerns, providing appropriate support, and increasing awareness among educators and students about the capabilities and limitations of AI to ensure its effective integration into the learning environment.
The distribution of perceptions of AI in higher education stands out as a medium level of perception among both students and professors, indicating a cautious, though not entirely negative, attitude toward AI in both groups. This finding is consistent with the existing literature on AI adoption in education, which highlights the need for further research to address challenges and improve acceptance levels among educators and students (Alfarsi et al., 2020; Alzahrani, 2023; Lee and You, 2024; Quinde et al., 2024). Understanding these perceptions is crucial to developing effective AI applications in educational settings, taking into account factors such as students’ drawing skills and attitudes toward AI to tailor AI interventions that effectively support learning processes (Kim et al., 2024).
The results of the research, together with the existing literature, suggest that a holistic approach is needed to ensure the success of AI in higher education by addressing ethical concerns, providing appropriate training, and developing strategies for integrating AI in a way that does not replace human interaction in the educational process. This will require a coordinated effort to co-create solutions that harness the potential of AI while preserving the core values of higher education, involving academic institutions, technology developers, educators, and students. In addition to technological progress, there is also a need for cultural and educational change, preparing students for a future in which interaction with artificial intelligence is integrated into many aspects of professional life and the workforce.
The results show that, with notable differences between students and professors, there is a generally low or cautious perception of the use of artificial intelligence (AI) in higher education. While undergraduates are more optimistic, professors are more apprehensive regarding their application and effects on learning. The need to develop more robust training programs and assessment methods is highlighted by this gap in perceptions.
By balancing the optimism of students with the practical concerns of professors, the study highlights the importance of a balanced approach to integrating AI into higher education. This approach aims to facilitate the exchange of ideas and experiences on the use of AI in learning and to promote a deeper dialog among all stakeholders in the university environment. The alignment of expectations and the development of a shared vision could lead to an ethical and effective implementation of AI while respecting core educational values. In addition to maximizing AI’s benefits in the academic setting, such a holistic strategy will better prepare the university community for the challenges and opportunities that the digital age brings to higher education.
No guidelines or rules exist for using AI in higher education, creating a regulatory and operational vacuum. To ensure responsible and effective implementation, higher education institutions need to develop comprehensive regulatory frameworks that address ethical, pedagogical, and technical aspects.
To identify best practices and standards in different academic contexts, the study suggests conducting a comparison between universities on the use of AI. They also recommend developing specific ethical policies for each educational program that address issues of privacy, equitable access, algorithmic transparency, and academic integrity.
Similarly, an integration of AI that respects the particular principles and goals of each discipline would be ensured by the development of specific ethical policies for each educational program. These policies should address issues like privacy, equity in AI access and application, algorithmic transparency, and maintaining academic integrity. Implementing these recommendations would help create a robust and adaptive framework for using AI responsibly and efficiently in academia.
The original contributions presented in the study are included in the article/supplementary material, further inquiries can be directed to the corresponding author.
The patients/participants provided written informed consent to participate in this study. Written informed consent was obtained from the individual(s) for the publication of any potentially identifiable images or data included in this article.
YR: Funding acquisition, Resources, Writing – review & editing. OC: Conceptualization, Formal analysis, Writing – original draft, Writing – review & editing. YM: Data curation, Investigation, Writing – review & editing. JA: Formal analysis, Investigation, Writing – review & editing. ES: Conceptualization, Investigation, Visualization, Writing – original draft, Writing – review & editing. AC: Conceptualization, Data curation, Software, Writing – original draft. RC: Resources, Visualization, Writing – original draft.
The author(s) declare that no financial support was received for the research and/or publication of this article.
The authors declare that the research was conducted in the absence of any commercial or financial relationships that could be construed as a potential conflict of interest.
The authors declare that Gen AI was used in the creation of this manuscript. The AI Chatgtp Plus, version 4.0 was used to make grammatical corrections in the English language.
All claims expressed in this article are solely those of the authors and do not necessarily represent those of their affiliated organizations, or those of the publisher, the editors and the reviewers. Any product that may be evaluated in this article, or claim that may be made by its manufacturer, is not guaranteed or endorsed by the publisher.
Abdelghani, R., Sauzéon, H., and Pierre-Yves, O. (2023). Generative AI in the classroom: can students remain active learners? arXiv. doi: 10.48550/arXiv.2310.03192
Ackerman, D. (2021). Helping students of all ages flourish in the era of artificial intelligence. Available online at: https://news.mit.edu/2021/raise-artificial-intelligence-0518.
Akinwalere, S. N., and Ivanov, V. (2022). Artificial intelligence in higher education: challenges and opportunities. Border Crossing 12, 1–15. doi: 10.33182/bc.v12i1.2015
Al Dhaen, F. (2022). “The use of artificial intelligence in higher education – systematic review” in COVID-19 challenges to university information technology governance. Cham: Springer, 269–285.
Al Dhaen, E., Stone, M. D., and Mahmood, M. (2022). “Higher education institutional strategies to sustain quality education” in Assessing University Governance and Policies in Relation to the COVID-19 Pandemic, 37–48.
Alfarsi, G., Tawafak, R. M., ElDow, A., Malik, S. I., Jabbar, J., Al Sideiri, A., et al. (2020). General view about an artificial intelligence Technology in Education Domain. Proceedings of the International Conference on Culture Heritage, Education, Sustainable Tourism, and Innovation Technologies, 120–127.
Alhaidry, H. M., Fatani, B., Alrayes, J. O., Almana, A. M., and Alfhaed, N. K. (2023). ChatGPT in dentistry: a comprehensive review. Cureus 15:e38317. doi: 10.7759/cureus.38317
Alzahrani, L. (2023). Analyzing students’ attitudes and behavior toward artificial intelligence Technologies in Higher Education. Int. J. Recent Technol. Eng. 11, 65–73. doi: 10.35940/ijrte.F7475.0311623
Antonietti, C., Cattaneo, A., and Amenduni, F. (2022). Can teachers’ digital competence influence technology acceptance in vocational education? Comput. Hum. Behav. 132:107266. doi: 10.1016/j.chb.2022.107266
Arowosegbe, A., Alqahtani, J. S., and Oyelade, T. (2024). Students’ perception of generative AI use for academic purpose in UK higher education. Preprints 2024051158. doi: 10.20944/preprints202405.1158.v1
Barrot, J. S. (2024). “Globalisation and higher education: integrating generative artificial intelligence into global higher educational systems: implications for practice, policy, and research” in Fourth international handbook of globalisation, education and policy research. Cham: Springer, 231–250.
Bearman, M., Ryan, J., and Ajjawi, R. (2023). Discourses of artificial intelligence in higher education: a critical literature review. High. Educ. 86, 369–385. doi: 10.1007/s10734-022-00937-2
Bowles, D. C., and Kruger, J. S. (2023). Generating employable, intelligent graduates in a world with generative AI: thoughts for educators. Pedagogy Health Promot. 9, 75–77. doi: 10.1177/23733799231175171
Bozkurt, A. (2023). Generative artificial intelligence (AI) powered conversational educational agents: the inevitable paradigm shift. Asian J. Distance Educ. 18, 198–204. doi: 10.5281/zenodo.7716416
Bughin, J., Hazan, E., Lund, S., Dahlstrom, P., Wiesinger, A., and Subramaniam, A. (2018). Skill shift: automation and the future of the workforce. Available online at: https://www.voced.edu.au/content/ngv:79805
Castelli, M., and Manzoni, L. (2022). Special issue: generative models in artificial intelligence and their applications. Appl. Sci. 12:4127. doi: 10.3390/app12094127
Chan, C. K. Y., and Hu, W. (2023). Students’ voices on generative AI: perceptions, benefits, and challenges in higher education. Int. J. Educ. Technol. High. Educ. 20:43. doi: 10.1186/s41239-023-00411-8
Chen, L., Chen, P., and Lin, Z. (2020). Artificial intelligence in education: a review. IEEE Access 8, 75264–75278. doi: 10.1109/ACCESS.2020.2988510
Chen, Y., Jensen, S., Albert, L. J., Gupta, S., and Lee, T. (2023). Artificial intelligence (AI) student assistants in the classroom: designing Chatbots to support student success. Inf. Syst. Front. 25, 161–182. doi: 10.1007/s10796-022-10291-4
Chiu, T. K. F. (2023). The impact of generative AI (GenAI) on practices, policies and research direction in education: a case of ChatGPT and Midjourney. Interact. Learn. Environ. 32, 6187–6203. doi: 10.1080/10494820.2023.2253861
Chiu, T. K. F. (2024). Future research recommendations for transforming higher education with generative AI. Comput. Educ. Artif. Intell. 6:100197. doi: 10.1016/j.caeai.2023.100197
Chiu, T. K. F., Xia, Q., Zhou, X., Chai, C. S., and Cheng, M. (2023). Systematic literature review on opportunities, challenges, and future research recommendations of artificial intelligence in education. Comput. Educ. Artif. Intell. 4:100118. doi: 10.1016/j.caeai.2022.100118
Choi, J. H., Hickman, K. E., Monahan, A., and Schwarcz, D. B. (2023). ChatGPT goes to law school. Journal of Legal Education 71, 387–400. doi: 10.2139/ssrn.4335905
Chung, C.-J. (2024). Preservice teachers’ perceptions of AI in education. AI-EDU Arxiv 1–5, 1–5. doi: 10.36851/ai-edu.vi0.4155
Cooper, G. (2023). Examining science education in ChatGPT: an exploratory study of generative artificial intelligence. J. Sci. Educ. Technol. 32, 444–452. doi: 10.1007/s10956-023-10039-y
Cota-Rivera, E. I., González, M. E., Bernal, L. A., Marquez, M. Y., Herrera, A. M., and Martinez, M. A. (2024). “Transforming education with the power of artificial intelligence: case studies” in Enhancing Higher Education and Research With OpenAI Models, 113–140.
Crabtree, M. (2023). What is AI Literacy? A Comprehensive Guide for Beginners. Available online at: https://www.datacamp.com/blog/what-is-ai-literacy-a-comprehensive-guide-for-beginners
Crawford, J., Cowling, M., and Allen, K.-A. (2023). Leadership is needed for ethical ChatGPT: character, assessment, and learning using artificial intelligence (AI). J. Univ. Teach. Learn. Pract. 20, 1–19. doi: 10.53761/1.20.3.02
Crompton, H., and Burke, D. (2023). Artificial intelligence in higher education: the state of the field. Int. J. Educ. Technol. High. Educ. 20, 1–19. doi: 10.1186/s41239-023-00392-8
Damasevicius, R., and Sidekerskiene, T. (2024). “AI as a teacher: a new educational dynamic for modern classrooms for personalized learning support” in AI-Enhanced Teaching Methods, 1–24.
Dong, C. (2024). How to build an AI tutor that can adapt to any course and provide accurate answers using large language model and retrieval-augmented generation. arXiv. doi: 10.48550/arXiv.2311.17696
Douglas, W. (2023). ChatGPT is going to change education, not destroy it. Available online at: https://www.technologyreview.com/2023/04/06/1071059/chatgpt-change-not-destroy-education-openai/
García-Chitiva, M. d. P., Valdes-Ramirez, D., Zavala, G., and Vázquez-Villegas, P. (2024). “Readiness of the student Community for Using Artificial Intelligence in higher education” in 10th international conference on higher education advances (HEAd’24), 1–8.
Hashmi, N., and Bal, A. S. (2024). Generative AI in higher education and beyond. Bus. Horiz. 67, 607–614. doi: 10.1016/j.bushor.2024.05.005
Holmes, W., Bialik, M., and Fadel, C. (2019). Artificial Intelligence in Education. Promise and Implications for Teaching and Learning. Available online at: https://www.researchgate.net/publication/332180327.
Holmes, W., and Tuomi, I. (2022). State of the art and practice in AI in education. Eur. J. Educ. 57, 542–570. doi: 10.1111/ejed.12533
Hu, K. (2023). ChatGPT sets record for fastest-growing user base - analyst note. Available online at: https://www.reuters.com/technology/chatgpt-sets-record-fastest-growing-user-base-analyst-note-2023-02-01/
Huang, J., Saleh, S., and Liu, Y. (2021). A review on artificial intelligence in education. AJIS 10:206. doi: 10.36941/ajis-2021-0077
Hwang, Y., Lee, J. H., and Shin, D. (2023). What is prompt literacy? An exploratory study of language learners' development of new literacy skill using generative AI. arXiv. doi: 10.48550/arXiv.2311.05373
Hwang, G.-J., Xie, H., Wah, B. W., and Gašević, D. (2020). Vision, challenges, roles and research issues of artificial intelligence in education. Comput. Educ. Artif. Intell. 1:100001. doi: 10.1016/j.caeai.2020.100001
Kasneci, E., Sessler, K., Küchemann, S., Bannert, M., Dementieva, D., Fischer, F., et al. (2023). ChatGPT for good? On opportunities and challenges of large language models for education. Learn. Individ. Differ. 103:102274. doi: 10.1016/j.lindif.2023.102274
Kelion, L. (2018). AI “poses less risk to jobs than feared” says OECD. Available online at: https://www.bbc.com/news/technology-43618620.
Kim, J., Ham, Y., and Lee, S.-S. (2024). Differences in student-AI interaction process on a drawing task: focusing on students’ attitude towards AI and the level of drawing skills. Australas. J. Educ. Technol. 40, 19–41. doi: 10.14742/ajet.8859
Law, N., Woo, D., Torre, J.de la, and Wong, G. (2018). A global framework of reference on digital literacy skills for indicator 4.4.2. Available online at: https://www.voced.edu.au/content/ngv%3A87544
Lee, D., Arnold, M., Srivastava, A., Plastow, K., Strelan, P., Ploeckl, F., et al. (2024). The impact of generative AI on higher education learning and teaching: a study of educators’ perspectives. Comput. Educ. Artif. Intell. 6:100221. doi: 10.1016/j.caeai.2024.100221
Lee, Y.-S., and Shim, T. (2024). A study on the application of university students’ perception of generative AI. Korean Assoc. Study Relig. Educ. 76, 139–152. doi: 10.58601/kjre.2024.03.30.08
Lee, H.-S., and You, J.-W. (2024). Exploring college students’ educational experiences and perceptions of generative AI: the case of a university. J. Korea Contents Assoc. 24, 428–437. doi: 10.5392/JKCA.2024.24.01.428
Lim, W. M., Gunasekara, A., Pallant, J. L., Pallant, J. I., and Pechenkina, E. (2023). Generative AI and the future of education: Ragnarök or reformation? A paradoxical perspective from management educators. Int. J. Manag. Educ. 21:100790. doi: 10.1016/j.ijme.2023.100790
Lodge, J. M., Thompson, K., and Corrin, L. (2023). Mapping out a research agenda for generative artificial intelligence in tertiary education. Australas. J. Educ. Technol. 39, 1–8. doi: 10.14742/ajet.8695
McGrath, C., Cerratto, T., Juth, N., and Palmgren, P. J. (2023). University teachers’ perceptions of responsibility and artificial intelligence in higher education - an experimental philosophical study. Comput. Educ. Artif. Intell. 4:100139. doi: 10.1016/j.caeai.2023.100139
Michel-Villarreal, R., Vilalta-Perdomo, E., Salinas-Navarro, D. E., Thierry-Aguilera, R., and Gerardou, F. S. (2023). Challenges and opportunities of generative AI for higher education as explained by ChatGPT. Educ. Sci. 13:856. doi: 10.3390/educsci13090856
Murugesan, S., and Cherukuri, A. K. (2023). The rise of generative artificial intelligence and its Impact on Education: the promises and perils. Computer 56, 116–121. doi: 10.1109/MC.2023.3253292
Mustofa, R. H., Kuncoro, T. G., Atmono, D., Hermawan, H. D., and Sukirman,. (2025). Extending the technology acceptance model: the role of subjective norms, ethics, and trust in AI tool adoption among students. Comput. Educ. Artif. Intell. 8:100379. doi: 10.1016/j.caeai.2025.100379
Nemorin, S., Vlachidis, A., Ayerakwa, H. M., and Andriotis, P. (2023). AI hyped? A horizon scan of discourse on artificial intelligence in education (AIED) and development. Learn. Media Technol. 48, 38–51. doi: 10.1080/17439884.2022.2095568
Ouyang, F., and Jiao, P. (2021). Artificial intelligence in education: the three paradigms. Comput. Educ. Artif. Intell. 2:100020. doi: 10.1016/j.caeai.2021.100020
Paetsch, J., Franz, S., and Wolter, I. (2023). Changes in early career teachers’ technology use for teaching: the roles of teacher self-efficacy, ICT literacy, and experience during COVID-19 school closure. Teach. Teach. Educ. 135:104318. doi: 10.1016/j.tate.2023.104318
Pearce, J., and Chiavaroli, N. (2023). Rethinking assessment in response to generative artificial intelligence. Med. Educ. 57, 889–891. doi: 10.1111/medu.15092
Peres, R., Schreier, M., Schweidel, D., and Sorescu, A. (2023). On ChatGPT and beyond: how generative artificial intelligence may affect research, teaching, and practice. Int. J. Res. Mark. 40, 269–275. doi: 10.1016/j.ijresmar.2023.03.001
Quinde, G. A. L., Muñoz, M. Y. T., Suárez, J. M. R., Villarreal, R. E. P., Vélez, W. A. Z., and Laínez, A. A. D. P. (2024). Perception of university students on the use of artificial intelligence (AI) tools for the development of autonomous learning. Revista de Gestão Social e Ambiental 18:e06170. doi: 10.24857/rgsa.v18n2-136
Regan, P. M., and Jesse, J. (2019). Ethical challenges of edtech, big data and personalized learning: twenty-first century student sorting and tracking. Ethics Inf. Technol. 21, 167–179. doi: 10.1007/s10676-018-9492-2
Sathish, S., Kesavan, D., Marianand, N., and Naveen, X. (2023). “Artificial intelligence and students learning: a study on outcome of technological usage (AI) in higher education platform” in 2023 9th international conference on advanced computing and communication systems (ICACCS), 2077–2083.
Schiff, D. (2021). Out of the laboratory and into the classroom: the future of artificial intelligence in education. AI & Soc. 36, 331–348. doi: 10.1007/s00146-020-01033-8
Sharma, M., and Sharma, S. (2023). A holistic approach to remote patient monitoring, fueled by ChatGPT and Metaverse technology: the future of nursing education. Nurse Educ. Today 131:105972. doi: 10.1016/j.nedt.2023.105972
Shoja, M. M., Van de Ridder, J. M. M., and Rajput, V. (2023). The emerging role of generative artificial intelligence in medical education, research, and practice. Cureus 15:e40883. doi: 10.7759/cureus.40883
Stöhr, C., Ou, A. W., and Malmström, H. (2024). Perceptions and usage of AI chatbots among students in higher education across genders, academic levels and fields of study. Comput. Educ. Artif. Intell. 7:100259. doi: 10.1016/j.caeai.2024.100259
Summers, A., Haddad, M.El, Prichard, R., Clarke, K.-A., Lee, J., and Oprescu, F. (2024). Navigating challenges and opportunities: nursing student’s views on generative AI in higher education. Nurse Educ. Pract., 79,:104062. doi: 10.1016/j.nepr.2024.104062
Supelette, M. (2023). O Impacto da Inteligência Artificial na EaD. Apresentações Trabalhos Científicos. doi: 10.17143/ciaed.XXVIIICIAED.2023.233244
Tahiru, F. (2021). AI in education. J. Cases Informat. Technol. 23, 1–20. doi: 10.4018/JCIT.2021010101
Tayan, O., Hassan, A., Khankan, K., and Askool, S. (2024). Considerations for adapting higher education technology courses for AI large language models: a critical review of the impact of ChatGPT. Mach. Learn. Appl. 15:100513. doi: 10.1016/j.mlwa.2023.100513
Thurzo, A., Strunga, M., Urban, R., Surovková, J., and Afrashtehfar, K. I. (2023). Impact of artificial intelligence on dental education: a review and guide for curriculum update. Educ. Sci. 13:150. doi: 10.3390/educsci13020150
Tzirides, A. O., Saini, A. K., Cope, B., Kalantzis, M., and Searsmith, D. (2023). Cyber-social research: emerging paradigms for interventionist education research in the Postdigital era, 85–102.
Tzirides, A. O., Zapata, G., Kastania, N. P., Saini, A. K., Castro, V., Ismael, S. A., et al. (2024). Combining human and artificial intelligence for enhanced AI literacy in higher education. Comput. Educ. Open 6:100184. doi: 10.1016/j.caeo.2024.100184
Vasudevan, A., Vorfi Lama, A., and Sain, Z. H. (2024). The game-changing impact of AI Chatbots on education ChatGPT and beyond. J. Informat. Syst. Technol. Res. 3, 38–44. doi: 10.55537/jistr.v3i1.770
Wang, T., Lund, B. D., Marengo, A., Pagano, A., Mannuru, N. R., Teel, Z. A., et al. (2023). Exploring the potential impact of artificial intelligence (AI) on international students in higher education: generative AI, Chatbots, analytics, and international student success. Appl. Sci. 13:6716. doi: 10.3390/app13116716
Yao, N., and Wang, Q. (2024). Factors influencing pre-service special education teachers’ intention toward AI in education: digital literacy, teacher self-efficacy, perceived ease of use, and perceived usefulness. Heliyon 10:e34894. doi: 10.1016/j.heliyon.2024.e34894
Zawacki-Richter, O., Marín, V. I., Bond, M., and Gouverneur, F. (2019). Systematic review of research on artificial intelligence applications in higher education – where are the educators? Int. J. Educ. Technol. High. Educ. 16:39. doi: 10.1186/s41239-019-0171-0
Zhai, X., Chu, X., Chai, C. S., Jong, M. S. Y., Istenic, A., Spector, M., et al. (2021). A review of artificial intelligence (AI) in education from 2010 to 2020. Complexity 2021, 1–18. doi: 10.1155/2021/8812542
Keywords: artificial intelligence, GenAI, university education, learning tools, educational innovation
Citation: Reina Marín Y, Cruz Caro O, Maicelo Rubio YdC, Alva Tuesta JN, Sánchez Bardales E, Carrasco Rituay AM and Chávez Santos R (2025) Artificial intelligence as a teaching tool in university education. Front. Educ. 10:1578451. doi: 10.3389/feduc.2025.1578451
Received: 17 February 2025; Accepted: 25 March 2025;
Published: 16 April 2025.
Edited by:
Ayse Saliha Sunar, University of Warwick, United KingdomReviewed by:
Isolde Malmberg, University of Music and Performing Arts Vienna, AustriaCopyright © 2025 Reina Marín, Cruz Caro, Maicelo Rubio, Alva Tuesta, Sánchez Bardales, Carrasco Rituay and Chávez Santos. This is an open-access article distributed under the terms of the Creative Commons Attribution License (CC BY). The use, distribution or reproduction in other forums is permitted, provided the original author(s) and the copyright owner(s) are credited and that the original publication in this journal is cited, in accordance with accepted academic practice. No use, distribution or reproduction is permitted which does not comply with these terms.
*Correspondence: Omer Cruz Caro, b21lci5jcnV6QHVudHJtLmVkdS5wZQ==
†ORCID: Yuri Reina Marín, orcid.org/0000-0002-9402-4104
Omer Cruz Caro, orcid.org/0000-0001-5664-3222
Yecenia del Carmen Maicelo Rubio, orcid.org/0009-0009-6033-5113
Judith Nathaly Alva Tuesta, orcid.org/0000-0003-1850-1535
Einstein Sánchez Bardales, orcid.org/0009-0002-8577-4330
Angelica María Carrasco Rituay, orcid.org/0000-0002-2724-4684
River Chávez Santos, orcid.org/0000-0002-3705-8682
Disclaimer: All claims expressed in this article are solely those of the authors and do not necessarily represent those of their affiliated organizations, or those of the publisher, the editors and the reviewers. Any product that may be evaluated in this article or claim that may be made by its manufacturer is not guaranteed or endorsed by the publisher.
Research integrity at Frontiers
Learn more about the work of our research integrity team to safeguard the quality of each article we publish.