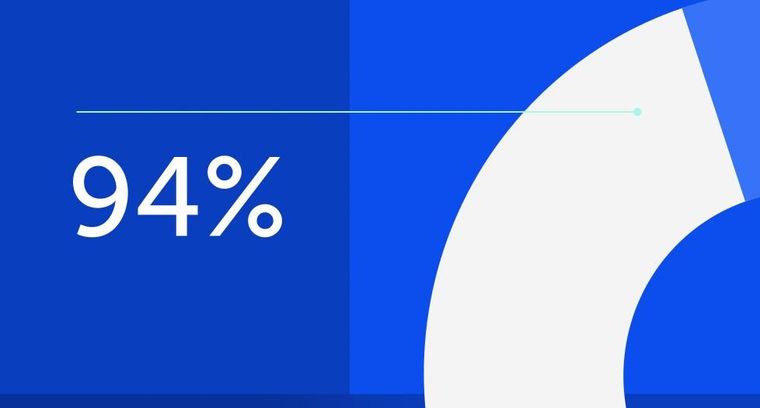
94% of researchers rate our articles as excellent or good
Learn more about the work of our research integrity team to safeguard the quality of each article we publish.
Find out more
EDITORIAL article
Front. Educ., 21 February 2025
Sec. Assessment, Testing and Applied Measurement
Volume 10 - 2025 | https://doi.org/10.3389/feduc.2025.1571177
This article is part of the Research TopicActionable Learning Analytics in Education: An opportunity to close the learning loopView all 7 articles
Editorial on the Research Topic
Actionable learning analytics in education: an opportunity to close the learning loop
Actionable learning analytics (LA) has the potential to revolutionize education by providing real-time, practical insights that can directly influence teaching strategies and learning processes. Unlike traditional LA approaches that focus on performance monitoring and external interventions, actionable LA aims to close the feedback loop by delivering data-informed insights that empower stakeholders (learners, educators, parents, etc.) themselves to make informed decisions. This Research Topic, drawing on data from over half a million learners across five different countries, explores how actionable LA can be leveraged to promote self-regulation, metacognition, and motivation in learners, transforming passive data collection into active educational interventions for real change.
LA has traditionally focused on descriptive, predictive, or prescriptive approaches to student performance (e.g., Brass et al., 2023; Chiera et al., 2021; Gabriel et al., 2022; Herodotou et al., 2020). Data is collected, analyzed, and presented to educators and policymakers to evaluate student outcomes and sometimes inform future educational delivery for other students. However, rarely does this feedback loop offer actionable insights that can be immediately used by current students to enhance their learning. For LA to truly impact learning, it must go beyond performance monitoring to facilitate self-reflection, goal setting, and adaptive learning strategies; that is, LA must be actionable.
To achieve these outcomes, this Research Topic brings together articles that address three key themes: theoretical frameworks for integrating learning analytics into learning processes, empirical studies on the impact of LA on student outcomes, and case studies demonstrating practical applications of LA in educational settings. Together, these contributions offer a comprehensive view of how LA can be transformed into an active agent of change in educational practice.
Several articles in this Research Topic highlight methodological innovations that advance the field of LA by developing new tools and approaches for analyzing educational data. For example, van Berk et al. build on the Train Track Task (Bryce and Whitebread, 2012) by digitizing it to capture log data and analyzing these to track students' monitoring and control behaviors during problem-solving tasks. This innovative approach employs finite state machines triangulated with think-aloud data to interpret complex behavioral patterns, offering educators actionable insights to support learning strategy development in real-time.
Another methodological innovation comes from Vasco et al., who compare two analytical methods—Discrete Time Markov Chains and Epistemic Network Analysis—for evaluating group-level discourse in computer-supported collaborative learning. By analyzing the affordances of each method, the authors demonstrate how these approaches can reveal patterns in collaborative interactions that might otherwise go unnoticed. These methods offer new ways for educators to interpret discourse data, improving their ability to foster metacognitive awareness and enhance collaborative learning outcomes.
Designing effective LA systems requires careful consideration of both technological and pedagogical elements. Ouhaichi et al. explore using multimodal learning analytics to identify key design considerations that enhance the practical impact of these tools in educational settings. By stressing the human factor in LA, their Multimodal Design Framework provides practical guidelines for integrating data sources to support students' real-time learning, social engagement, and adaptive strategies.
Tempelaar et al., explore how LA systems can be tailored to align with students' unique learning dispositions. By examining patterns in students' engagement with learning tasks and tools, their work highlights the importance of personalizing LA to meet diverse learner needs. These insights underscore the potential of LA to intertwine traditional learning metrics with affective measures to identify and address deeper understandings of learning behaviors.
The longitudinal case studies in this Research Topic provide valuable insights into the practical applications of LA in diverse educational settings. Johnson et al. explore how social network analysis can be used to monitor decision-making patterns in a large Australian school. The study demonstrates how these metrics can be translated into actionable insights to support an expansive perspective on education. This pragmatic approach to learning analytics emphasizes the importance of contextualizing data within human activity systems recognizing the uncertain and ongoing nature of learning.
Barragán and Marcelo examine the use of standardized government tests as an educational quality indicator in Colombia. The study highlights the challenges of relying solely on standardized assessments to evaluate educational outcomes and calls for a more comprehensive approach that incorporates LA to address discrepancies between policy goals and student performance. By expanding the scope of LA, this research demonstrates the potential for more context-sensitive approaches to educational evaluation.
The contributions to this Research Topic collectively emphasize the need for a more holistic approach to LA. As widely noted (e.g., Dawson et al., 2019; Rets et al., 2023; Viberg et al., 2018), LA often tends to focus on outcomes, such as academic performance and knowledge acquisition. However, as several articles in this Research Topic demonstrate, it is equally important to consider non-cognitive outcomes, such as motivation, self-efficacy, and metacognitive skills. These factors all play a critical role in students' ability to regulate their own learning and achieve long-term academic success.
Furthermore, as highlighted by these articles, learning cannot be separated from its cultural and historical context; individuals and communities shape and are shaped by their environments. Therefore, LA needs to shift from tracking isolated learning transactions to understanding and adapting to complex learning systems. These nuanced perspectives challenge the one-size-fits-all approach to learning strategies and underscore the need for personalized LA interventions.
Despite the promising findings presented in this Research Topic, several challenges remain in the field of actionable LA. Of these, the key challenge is the need for greater collaboration between researchers, educators, and policymakers to ensure that LA systems are designed and implemented in ways that meet the needs of all stakeholders. The case studies in this Research Topic provide valuable examples of how such collaborations can be achieved, but more work is needed to scale these efforts across diverse educational contexts.
This Research Topic has demonstrated that actionable LA has the potential to close the educational loop. That is, actionable LA has the power to bridge the gap between data collection and practical application. As the field of LA continues to evolve, we need to prioritize actionable insights that empower learners and other stakeholders to ensure that the innovation emerging from the field of LA addresses the real challenges of learning and teaching and not only the challenges that are (relatively) easy to understand and measure.
JK: Conceptualization, Project administration, Writing – original draft, Writing – review & editing. FG: Writing – original draft, Writing – review & editing, Conceptualization. MK: Writing – original draft, Writing – review & editing, Conceptualization. IR: Writing – original draft, Writing – review & editing, Conceptualization. BR: Writing – original draft, Writing – review & editing, Conceptualization.
The authors declare that the research was conducted in the absence of any commercial or financial relationships that could be construed as a potential conflict of interest.
All claims expressed in this article are solely those of the authors and do not necessarily represent those of their affiliated organizations, or those of the publisher, the editors and the reviewers. Any product that may be evaluated in this article, or claim that may be made by its manufacturer, is not guaranteed or endorsed by the publisher.
Brass, T., Kennedy, J. P., Gabriel, F., Neill, B., Devis, D., and Leonard, S. (2023). Learning analytics for lifelong career development: a framework to support sustainable formative assessment and self-reflection in programs developing career self-efficacy. Front. Artif. Intell. 6:1173099. doi: 10.3389/frai.2023.1173099
Bryce, D., and Whitebread, D. (2012). The development of metacognitive skills: evidence from observational analysis of young children's behavior during problem-solving. Metacogn. Learn. 7, 197–217. doi: 10.1007/s11409-012-9091-2
Chiera, B., Korolkiewicz, M., and Schultz, L. (2021). “Learning from learning analytics: how much do we know about patterns of student engagement?” in Big Data in Education: Pedagogy and Research. Policy Implications of Research in Education, ed. T. Prodromou (Cham: Springer). doi: 10.1007/978-3-030-76841-6_8
Dawson, S., Joksimovic, S., Poquet, O., and Siemens, G. (2019). “Increasing the impact of learning analytics,” in Proceedings of the 9th International Conference on Learning Analytics and Knowledge (Tempe, AZ, USA). doi: 10.1145/3303772.3303784
Gabriel, F., Cloude, E. B., and Azevedo, R. (2022). “Using learning analytics to measure motivational and affective processes during self-regulated learning with advanced learning technologies,” in Social and Emotional Learning and Complex Skills Assessment: An Inclusive Learning Analytics Perspective, eds. Y. E. Wang, S. Joksimović, M. O. Z. San Pedro, J. D. Way, and J. Whitmer (Cham: Springer International Publishing), 93–108. doi: 10.1007/978-3-031-06333-6_6
Herodotou, C., Naydenova, G., Boroowa, A., Gilmour, A., and Rienties, B. (2020). How can predictive learning analytics and motivational interventions increase student retention and enhance administrative support in distance education? J. Learn. Analy. 7, 72–83. doi: 10.18608/jla.2020.72.4
Rets, I., Herodotou, C., and Gillespie, A. (2023). Six practical recommendations enabling ethical use of predictive learning analytics in distance education. J. Lear. Anal. 10, 149–167. doi: 10.18608/jla.2023.7743
Keywords: actionable learning analytics, methodological innovations, educational feedback, real-time analysis, learning data analysis, performance improvement
Citation: Kennedy JP, Gabriel F, Korolkiewicz M, Rets I and Rienties B (2025) Editorial: Actionable learning analytics in education: an opportunity to close the learning loop. Front. Educ. 10:1571177. doi: 10.3389/feduc.2025.1571177
Received: 05 February 2025; Accepted: 11 February 2025;
Published: 21 February 2025.
Edited and reviewed by: Gavin T. L. Brown, The University of Auckland, New Zealand
Copyright © 2025 Kennedy, Gabriel, Korolkiewicz, Rets and Rienties. This is an open-access article distributed under the terms of the Creative Commons Attribution License (CC BY). The use, distribution or reproduction in other forums is permitted, provided the original author(s) and the copyright owner(s) are credited and that the original publication in this journal is cited, in accordance with accepted academic practice. No use, distribution or reproduction is permitted which does not comply with these terms.
*Correspondence: JohnPaul Kennedy, am9obi5rZW5uZWR5QHVuaXNhLmVkdS5hdQ==
Disclaimer: All claims expressed in this article are solely those of the authors and do not necessarily represent those of their affiliated organizations, or those of the publisher, the editors and the reviewers. Any product that may be evaluated in this article or claim that may be made by its manufacturer is not guaranteed or endorsed by the publisher.
Research integrity at Frontiers
Learn more about the work of our research integrity team to safeguard the quality of each article we publish.