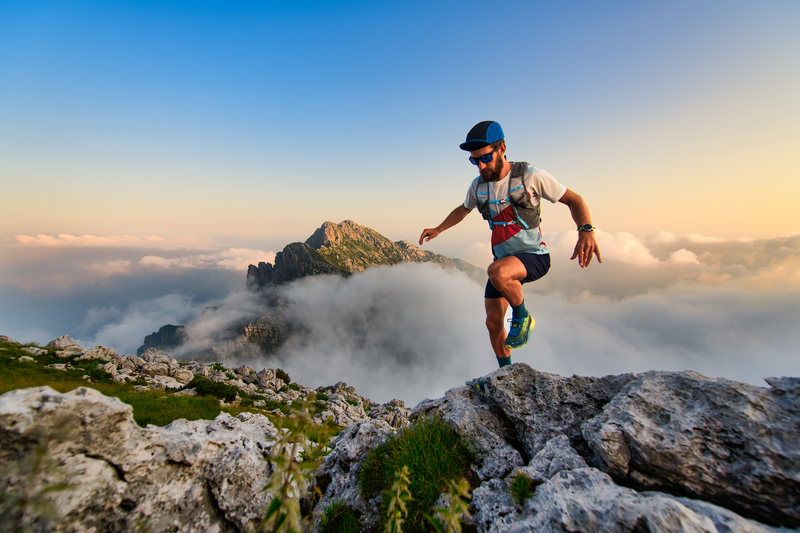
94% of researchers rate our articles as excellent or good
Learn more about the work of our research integrity team to safeguard the quality of each article we publish.
Find out more
ORIGINAL RESEARCH article
Front. Educ. , 08 January 2025
Sec. Digital Education
Volume 9 - 2024 | https://doi.org/10.3389/feduc.2024.1495724
This article is part of the Research Topic Promoting Innovative Learning-oriented Feedback Practice: Engagement, Challenges, and Solutions View all 7 articles
The online teaching and learning method can implement synchronous and asynchronous processes for theory courses using digital platforms through digital education systems. Implementing online teaching and learning for laboratory courses presents challenges, as synchronous is infeasible due to the requirement for specialized tools, equipment, and experimental materials. An asynchronous teaching and learning model can be used instead. In this study, we proposed an Asynchronous online teaching and learning model tailored for practice laboratory-based courses in engineering and technology education. This model is named “Development of a Laboratory-Based Learning Model via a Digital Platform Environment.” This model is proposed based on the student-feedback interaction model, and called the online feedback practice model. The proposed model is experimented with teaching and learning laboratory-based subjects. The experiment involved two groups of 200 students, including 100 students in the control group (face-to-face practice) and 100 students in the experimental group (online feedback practice). Both groups participated in a final evaluation through direct in-class assessments. The research results demonstrated that the model yields higher effectiveness than the traditional method, based on evaluating statistical analysis to compare average scores, outcomes, achievement rate, and assignment completion rate of two student groups. The model also gathered feedback from instructors teaching in the same field regarding the course's learning outcomes, which have been validated to meet AUN-QA standards. The survey results are also statistically evaluated and calculated through means, variances, standard deviations, and hypotheses using t-tests to demonstrate the effectiveness of the proposed model.
Teaching and learning methods in universities are commonly implemented through two prevalent approaches: traditional face-to-face instruction in a room or online teaching via, either synchronously or asynchronously, using online platforms through digital education systems.
Synchronous and asynchronous online teaching and learning methods are often used for theoretical subjects, especially within distance learning programs. Laboratory-based courses, particularly in engineering fields, are usually challenging to adapt to online teaching. This is because laboratory courses require learners to conduct experiments under direct guidance from instructors to acquire the necessary knowledge, skills, and attitudes. Therefore, implementing online learning for laboratory-based courses encounters numerous difficulties.
Asynchronous online education is also known as education on virtual learning platforms. The digital platform allows learners to study from anywhere using a computer or internet-connected device, rather than attending physical classes as in traditional methods. The learning content and materials can be accessed and updated through online learning software and other mobile applications. The outstanding feature of online education lies in the diverse interaction possibilities between instructors and learners. Learners have the autonomy to organize their learning schedule and study from anywhere, at any time, and this helps reduce travel time, costs, strain on transportation infrastructure, and various societal issues. Therefore, asynchronous interaction on virtual learning platforms is suitable for applying the feedback theory in designing the online teaching and learning models. The feedback in teaching and learning is principally about teachers informing students about strengths, weaknesses and how to improve, and highlights the centrality of the student role in sense-making and using comments to improve subsequent work (Carless and Boud, 2018). With the advancement of science and technology, humans are constantly seeking new methods and applying new technologies to serve their lives. Information technology has become a tool for improving various aspects of modern human society (Dias et al., 2020). With the development of technology today, we have become accustomed to the applications of new technologies in various fields, and the education system is no exception (ISCED, 2012). The development of digital technology has a significant impact on the education system through the teaching and learning process (Siemens, 2005). Digital technology, especially the internet, is becoming a profound influence on the teaching and learning process, for both learners and educators are effectively utilizing resources to disseminate and enhance their knowledge (Abuhassna and Yahaya, 2018).
Universities currently employ two primary methods of education: face-to-face teaching and online instruction, which can be either synchronous or asynchronous (Bignell and Parson, 2010). Face-to-face teaching is the traditional method in which learners and educators interact directly in the classroom to facilitate the teaching and learning process, while online involves delivering the educational process through digital platforms, utilizing the Internet communication network (Koneru, 2017; Dhakal, 2023). According to O'Donnell's (2012) research, online education delivered through digital platforms is highly effective in higher education. This is because students are adept at self-directed learning and are familiar with technology and internet-based interactions. The benefits of online education are apparent in both training programs and the academic achievements of university students. Anyaoku (2008) found that awareness of the increasing use of communication technology in classrooms is growing, particularly in developing countries. This suggests that relying solely on verbal communication or excessive verbal instruction to educate students is an ineffective approach. Instead, teachers should quickly transition to internet-related and web-based tasks within educational institutions. Two classifications of online teaching are synchronous online teaching and asynchronous online teaching (Hrastinski, 2008). For synchronous online teaching, the instructor and learners interact through the internet simultaneously to carry out the teaching and learning process. On the other hand, asynchronous online teaching does not require the instructor and learners to interact directly through the Internet simultaneously to conduct the teaching process (Setiadi et al., 2021; Alarifi and Song, 2024). For the asynchronous online teaching method, instructors can carry out the teaching process by providing instructional resources such as lecture videos, tutorial videos, images, and digital materials through an online teaching management platform. The learners can access the digital platform from anywhere and at any time to engage in their learning process (Smolyaninova and Bezyzvestnykh, 2019). The digital platform also provides learners with a space for interaction, both through synchronous and asynchronous methods, with the instructor and other class members.
Teaching on digital platforms is widely used in the era of technology 4.0 today (Chemsi et al., 2020; Restiglian et al., 2023). With the development of teaching management tools on digital platforms such as Moodle, Edmodo, MOOCs, and Google Classroom…, implementing the teaching process is very convenient (Ahmed and Mesonovich, 2019; Munoz and Towner, 2009). In technical education, Laboratory-based courses are subjects in which learners perform experiments to demonstrate theoretical knowledge acquired from theory subjects, aiming to gain profound understanding, solid skills, and familiarity with tools and equipment used in their field of study (Hmelo-Silver, 2004; Pumahapinyo and Suwannatthachote, 2014). The advent of high-speed internet today creates highly favorable conditions for educational institutions to develop remote learning methods (Sri Jothi et al., 2011; Tan et al., 2024). Distance education is well-suited for fields such as social sciences, economics, and languages, in which courses are predominantly theoretical. In the engineering and technology fields, certain subjects must be taught in workshops using specific tools and equipment to effectively convey the necessary knowledge, skills, and attitudes to learners, in addition to theoretical instruction.
In the traditional teaching method for practice subjects (Russell and Gabriela, 2011), the teacher demonstrates the example process only once during the demonstration phase. Students need to observe, take notes, and focus intently to remember the steps for following the instructions. After this demonstration, students replicate the demonstrated model while the teacher provides general observations and frequent guidance to help them develop the necessary skills. This model is called the face-to-face practice model (Figure 1).
For this research, we proposed a model for implementing laboratory-based courses in asynchronous online education. This model is proposed based on the student-feedback interaction model (Lipnevich and Smith, 2022), and called the online feedback practice model (Figure 2). In this online teaching method for practice subjects, the instructor supplies video, images, and text files, which are uploaded to the virtual classroom system for learners to prepare materials and demonstrate steps with step-by-step instructions. Learners access the system to view and read the materials as part of their learning process. Learners then submit to feedback their practical results to the instructor through video recordings of their actions, images, and report files, all of which are uploaded to the online virtual classroom system to assesse and get feedback message. The research results demonstrated that the model yields higher efficiency than the traditional method based on assessing the scores, achievement rate, assignment completion rate, and Course Learning Outcomes (CLOs), which have been validated to meet AUN-QA standards also evaluated.
Asynchronous online education has become a cornerstone of modern learning, especially in response to the challenges faced by traditional classroom settings. This method, which allows students to access learning materials and engage with instructors at their convenience, has proven effective for distance learning (Oxford University Press, 2015; Magalhães et al., 2020). The flexibility of online education not only reduces logistical barriers but also empowers learners to organize their schedules and engage in various digital interactions, enhancing their overall learning experience (Siemens, 2005). However, while the benefits of asynchronous online education are well-documented, significant challenges persist in delivering practical, laboratory-based learning experiences, particularly in STEM fields. This research aims to tackle challenges by proposing a new model for laboratory-based courses in a remote and asynchronous context.
Several studies have explored online learning models, particularly those focused on practice-based courses. For instance, Brinson (2015) examined the effectiveness of virtual laboratories and found no significant difference in academic achievement compared to traditional methods. This supports the notion that virtual laboratories can serve as a viable alternative in education. Additionally, Junaid and Sigala (2024) proposed models emphasizing teacher-student interaction through communication applications, aiming to foster meaningful learning experiences in tourism management. In another study, Hou et al. (2014) introduced a blended learning environment that integrates multiple devices for seamless course engagement. They noted the satisfaction of learners with the interface design but acknowledged limitations such as restricted schedule flexibility and a lack of practical tools. This highlights the need for more comprehensive solutions in remote learning environments. Flórez et al. (2023) conducted an evaluation of engineering students' preferences for various classroom settings during the COVID-19 pandemic. Their findings revealed that immersive virtual classrooms (IVC) received high acceptance among students, comparable to traditional face-to-face classrooms. Such insights provide a backdrop for exploring how innovative virtual environments can enhance learning experiences, particularly in hands-on disciplines.
Despite these advancements, the integration of effective online laboratory-based courses remains underemployed, especially in engineering and STEM fields. This gap presents an opportunity for innovative approaches that harness digital platforms to support practical education. The proposed “Development of a Laboratory-Based Learning Model via Digital Platform Environment” builds upon existing research while addressing the identified gaps. Unlike traditional models, which may primarily focus on theoretical instruction or limited practical engagement, this model aims to create a comprehensive digital framework that supports a full spectrum of laboratory-based learning experiences. For example, while Flórez et al. (2023) emphasized the effectiveness of IVC, our model seeks to enhance this concept by incorporating features specifically designed for practical skills development. This includes simulation tools, collaborative virtual environments, and adaptive learning technologies that cater to individual learning styles. Moreover, our model distinguishes itself from Junaid and Sigala (2024) by focusing not just on communication strategies but also on the integration of real-time data analytics and feedback mechanisms that can dynamically adjust to student needs. By leveraging these technologies, the proposed model aims to facilitate a deeper understanding of laboratory concepts, bridging the gap between theoretical knowledge and practical application.
Recent studies on feedback models are summarized in the research by Anastasiya A. Lipnevich and Ernesto Panadero. This research provides an overview of feedback models, including Kulhavy and Stock (1989): A Model From Information Processing; Sadler (1989): Seminal Work for Formative Assessment; and Bangert-Drowns, Kulik, Kulik, and Morgan (1991): The First Attempt to Meta-Analyze the Effects of Feedback. Studies on feedback in online learning are introduced in research by Jensen et al. (2021) which investigates how researchers in online learning understand feedback's role in teaching and learning and how these understandings shape research questions and recommendations for online feedback practices. According to Breuch (2004), online settings involve technology-mediated communication, defining peer feedback as technology-facilitated interactions among students. In this context, students submit their work online and engage in peer feedback exchanges (Jongsma et al., 2023; Latifi et al., 2021). This feedback model can be conducted through various online tools such as chat mediums, Facebook or Instagram community environments, blogs, discussion boards, collaborative writing software, as well as peer feedback-specific software and tools (Dawson et al., 2018). From this research, it shows that there are many feedback models and theories, some models are better suited for guiding empirical or theoretical. Choosing the right model and theory depends on the aims of the research (Lipnevich and Panadero, 2021).
The proposed model for laboratory-based learning via digital platforms is novel in several ways. First, it emphasizes a holistic approach that combines theoretical knowledge with practical application through simulations and collaborative projects. This dual focus aims to create a richer learning experience, particularly in fields where hands-on skills are essential. Additionally, the model incorporates real-time analytics to assess student performance and engagement continuously, and applies feedback theory in online teaching on digital platforms for teachers informing students about bout strengths, weaknesses and how to improve, and highlights the centrality of the student role in sense-making and using comments to improve subsequent work (Carless and Boud, 2018). By doing so, it allows for immediate adjustments to the learning experience, catering to diverse learner needs and enhancing overall effectiveness. This adaptability sets our approach apart from existing models, which may lack the infrastructure for such dynamic interactions.
Along with the development of information technology and digital platforms, online feedback practice brings numerous benefits, such as flexibility in terms of time and location, allowing learners to actively adjust their learning pace, saving time and costs, providing access to a global learning environment, enhancing learners' self-study abilities, offering vast learning resources from around the world, and enabling interaction between learners and instructors, as well as among learners worldwide.
For online feedback practice, instructors can conduct the teaching process by providing teaching resources such as lecture videos, instructional videos, images, and digital materials through an online teaching management platform to deliver lecture information to learners. Learners can access the digital platform at any time and from anywhere.
However, there is still limited research on teaching laboratory-based subjects using digital teaching platforms. Existing research only focuses on fields like medicine (Michela et al., 2019; Yun et al., 2022) and hotels, restaurants, and tourism management (Junaid and Sigala, 2024). The model of practical teaching for subjects in the fields of science, engineering, and technology on digital platforms for distance learning is not yet popular. In this research, the Laboratory-Based Learning Model via Digital Platform Environment can be applied to teach experimental subjects through online feedback practice and is suitable for fields in engineering and technology.
Feedback is described as a conversation between the teacher and a student, and cautioned that utterance that each party uses would be highly consequential for future student-teacher interactions and student learning progress (Lipnevich et al., 2016). Within the context, the feedback is delivered to the student. Students will inevitably vary on their personality, general cognitive ability, receptivity to feedback, prior knowledge, and motivation. The feedback itself may be detailed or sparse, aligned with the students' level of knowledge or not. It may be direct but delivered in a supportive fashion or may be unpleasantly critical. It may match what the student is expecting or be highly below or above those expectations. All these characteristics will contribute to students' differential processing of feedback (Lipnevich and Panadero, 2021). The revised student—feedback interaction model that described the context of feedback, its source characteristics of the message and learner, and focus on student processing, along with the outcomes of performance and learning. The revised model emphasized three main questions for student processing of feedback: Do I understand the feedback? How do I feel about the feedback? What am I going to do with the feedback? By answering these three questions, a learner generates self—or inner feedback, and this step is critical in any productive response to external feedback (Lipnevich and Smith, 2022).
The revised student—feedback interaction model shows that feedback message, student characteristics, and self—feedback (cognitive processing, affective processing, and behavioral processing) contribute to an action that may alter outcomes of student performance and learning (Lipnevich and Smith, 2022). So, in the online feedback practice process is proposed based on the student-feedback interaction model (Figure 2), instructors provide the course learning information and instructional materials (for example: the steps while recording the session as a video file, image and text files are prepared and uploaded to the virtual classroom system) and feedback message to learners, and receive learners feedback about outcomes of performance and learning. Learners participate in the learning process by accessing the system to view, read, and interact with feedback message, digital learning materials as needed, without limitations on how often they can review the resources or restrictions on time and location. Learners can actively undertake self—feedback and self—directed learning, following the instructions independently without being confined to classroom hours. To report their practical results, learners submit video recordings of their actions, image files, and report files through the online classroom system. That allows instructors to evaluate if learners' performance and learning have met the required skills and knowledge based on the CLOs, and to provide the feedback message. By being able to review the instructional actions, receive the feedback message, and self—feedback multiple times, along with the flexibility to arrange their learning time and space effectively, students are likely to achieve better CLOs compared to the traditional in-class method. The feedback message, and self-feedback process in the online feedback practice process is described as the Table 1.
The online teaching method also consists of five similar stages but employs a different instructional delivery format. Instruction is conveyed through a series of videos, images, and text files that are uploaded to the Moodle learning management system. In this process, students independently prepare their tools and materials and engage with the content according to the guidelines provided in the digital resources. This method emphasizes self-directed learning, where students take the initiative to manage their study materials and learning pace.
The experimental design employed a comparison method of the control and experimental groups. Learning conditions were the same between the two groups, such as teachers, students' level, curriculums, learning outcomes, assessment criteria, etc. Before the course commenced, students' foundational knowledge and skills were assessed to establish a baseline for both control group and experimental group. Following the completion of the course, the learning outcomes of the students were re-evaluated with the same assessment criteria. This dual assessment allows for comprehensive evaluation of pre-experimental teaching on student's level similarity of both groups, and post-experimental teaching on effectiveness of the online feedback practice (experimental group). Data collected included not only academic scores but also satisfaction levels, autonomy in learning, and the quality of interactions between students and instructors. The experimental models are described in detail in Figure 1 (control group) and 2 (experimental group) of the attached document.
For the face-to-face practice process (control group), the equipment is commonly used to be electronics laboratory, experimental equipment, and tools and devices. Instructional steps to achieve the CLOs are conducted in a fixed location, namely the classroom, and within the fixed time frame of that specific class session. During the demonstration steps, the teacher performs a single demonstration, requiring learners to observe, take notes, and carefully pay attention to remember the steps in order to follow the instructions accurately. Following the demonstration, learners replicate the modeled steps, with the teacher providing general observation and ongoing guidance to ensure students develop the necessary skills and knowledge required to meet the CLOs. In the traditional teaching method, instruction takes place directly in the laboratory, divided into five distinct periods over a total duration of 225 min, with each period lasting 45 min. This approach accommodates a class size of 25 students, facilitating direct engagement between the lecturer and the students. Online courses have also been widely implemented at the research institution, with data collected randomly and voluntarily. During the laboratory sessions, the instructor actively guides students through various practical activities, utilizing specific electronics laboratory equipment and tools. This hands-on approach aims to enhance experiential learning by allowing students to directly apply theoretical concepts in practical settings.
For the online feedback practice process (experimental group), the digital platform is used to be Moodle online learning management system (LMS). Because learning on a digital platform, so students have to actively equip themselves with practical learning devices to meet the course requirements, and are provided by teachers with detailed course information, and digital resources such as instructional videos, images, text files, group discussion tools, and teachers—students discussion tools. Learners access the system to view and read the materials as part of their learning process, then submitting to feedback their outcomes of performance and learning as a practical results to the online virtual classroom. Instructor receive the practical result feedback from students through video recordings of their actions, images, and report files. Instructors compare students' practical results with the CLOs to assess students' achievement of the CLOs, and provide feedback message to students for the next learning tasks. The process is repeated continuously until the end of the course. Learning rating scales are based on the level of achievement of the CLOs of their outcomes of performance and learning, including: Level 5 (Fair), Level 4 (Average), Level 3 (Good), Level 2 (Very Good), Level 1 (Excellent).
To evaluate the effectiveness of the control group and experimental group we conduct an independent samples t-test was employed to compare the end-of-term mean scores of both groups. The hypotheses were clearly defined as follows:
• H0: μX = μY (The mean score of the experimental group equals the mean score of the control group).
• H1: μX > μY (The mean score of the experimental group is higher than the mean score of control group).
In this context, μX represents the mean score of the experimental group, while μY signifies the mean score of the control group. The clarity of these hypotheses is crucial; both focus on comparing means, thus ensuring that we address the correct statistical parameters.
Statistical methods were applied to compare post-test the results of both control and experimental groups, facilitating an evaluation of the effectiveness of each teaching approach. These findings contribute to the growing body of research regarding the effectiveness of online feedback learning in higher education, particularly concerning practical, laboratory-based courses. Understanding the strengths and limitations of online feedback practice relative to traditional face-to-face practice is vital for optimizing student outcomes and enhancing educational quality.
We tested a new approach on the Basic Electronic Experiment course and Programmable Logic Controller, which is a part of the Automation major program and is being trained at Ho Chi Minh City University of Technology and Education, VietNam. This course consists of two credits and spans 15 weeks, with 25 students in each course. We experimented on two groups of 200 students. The control group, consisting of 100 students (81 males, 19 females, divided into four courses), followed the traditional in-person classroom practice model. The experimental group, consisting of 100 students (83 males, 17 females), engaged with the online feedback practice.
The research results demonstrated that the model yields higher efficiency than the traditional method based on assessing student scores using statistical analysis to compare the average scores of the two student groups of classes. Both groups have undergone evaluation tests on the same assessment and in the same format, which is a direct in-class assessment. The model also surveyed the opinions of 50 instructors teaching in the same field regarding the CLOs that meet AUN-QA standards. A statistical model is applied to evaluate the survey results and concludes the model's achievement rate. The measurements used in this model are mean, variance, and standard deviation. In our study, the LMS employed for remote training is Moodle, chosen for its proven effectiveness and popularity demonstrated by Basil and Oguguo (2020).
We performed an independent samples t-test to analyze the final exam scores for the Basic Electronic Experiment course: the control group and the experimental group, using a 10-point scale, as shown in Table 2. The results of the course's final grades are evaluated on a 5-level scale as follows: Level 5 (Fail): Points < 4.5; Level 4: Points 4.5–5.4; Level 3: Points 5.5–6.9; Level 2: Points 7.0–8.4; Level 1: Points 8.5–10.0. The results are summarized as shown in Figure 3 with “Series 1” for the control group and “Series 2” for the experimental group. The statistical analysis of the final course grades indicates that the average scores for both groups are comparable. The average score is 7.07 points for the control group, while the experimental group reaches 7.28 points. Overall, the grades for groups are similar. In the experimental group, higher levels of self-motivation and self-directed learning are necessary for the students. As a result, the dropout rate is slightly higher compared to the control group. However, for the online feedback practice (experimental group), students have the advantage of reviewing instructional materials and guidance from the teachers multiple times, which contributes to higher scores in the top level (Level 1) compared to the face-to-face practice (control group). On the contrary, for learners without autonomy, their learning results will not be good. By studying directly in class with direct supervision by teachers like Level 5, students do not pass subjects higher than studying directly in class.
The higher SD value of the experimental group (1.766) compared to the control group (1.418) shows that the scores in the online feedback learning had greater fluctuations than those in the face-to-face learning.
When assessing the content of average scores, we compare the average scores of both groups: control group and experimental group. Since these two groups are independent, we use the “T-test” method.
The calculated results indicate that, with a significance level of 0.05, the Rejection Region: Wα = (−1.64, +∞).
The calculated results indicate that, with a significance level of 0.05, the Rejection Region: Wα = (−1.64, +∞), the observed value of the test statistic is Zqs = −0.92. Since Zqs ∈ Wα, so Reject the H0 hypotheses. We can conclude that the average of final scores of the online feedback practice are higher than the face to face practice.
The evaluation result of the completion rate of the exercises for the entire course of both models, the face-to-face practice model and the online feedback l practice model, is presented in Figure 4. The results indicate that learners using the online feedback practice model have a higher completion rate for assignments, with the ratio of “More Than 90%” and “70%−90%” being higher than that of the face-to-face practice model. This is because learners can arrange their homework time flexibly and review lecture videos multiple times to better understand the lesson. However, for the indicators of 50%−70% and “ < 50%,” the face-to-face practice model has a higher completion rate than the online feedback practice model. This is because learners in the face-to-face practice model have to complete exercises within fixed time frames in class and listen to lecture content only once, which limits their ability to remember lectures and complete assignments. Regarding laboratory equipment for online learning, students are provided with a basic experimental kit, including essential electronic components and measuring instruments. This kit is designed to be consistent with the equipment used in face-to-face classes, ensuring consistency in the learning and practice process. However, some specialized equipment is still only available in the school laboratory, which may affect the learning experience of online students.
For the survey, we surveyed 50 instructors working in the same field. The survey content included the 9 CLOs that meet the AUN-QA standards (CLO1–CLO9). The assessment criteria for each CLOs were “Fair,” “Average,” “Good,” “Very Good,” and “Excellent” as shown in Figure 5. According to the results shown in Figure 5, a majority of experts have rated the online feedback practice model as “Excellent” and “Very Good” in terms of achieving CLOs with high rates. Only a few experts have given ratings at “Good,” “Average,” and “Fair” levels. This indicates that the online feedback practice model is highly effective in meeting the outcome standards of experiment-based subjects.
Next, we conducted statistics on the scores of 100 students who participated in the Basic Electronics Laboratory course on the experimental group and continued to participate in another course, Programmable Logic Controller, also on the experimental group. The statistical results are as shown in Table 3.
Table 3 shows the statistical outcomes of scores on a 10-point scale. The average score for students in both courses is 7.22, with a variance of 2.070 for Programmable Logic Controller, and 7.28, with a variance of 3.117, for Basic Electronics Laboratory. This indicates that the online feedback learning model is consistently effective across various subjects.
Our study provides compelling evidence for the effectiveness of the online feedback practice model in delivering experiment-based courses in higher education, specifically within the context of Ho Chi Minh City University of Technology and Education, Vietnam. This model is proposed based on the student-feedback interaction model (Lipnevich and Smith, 2022). While this online feedback practice model offers flexibility and self-directed learning. Learners regular receive feedback message after each learning task throughout the course. But, it also poses challenges related to learners' characteristics and self—feedback that can change the learners' learning outcomes (Lipnevich and Smith, 2022). These findings contribute to the growing body of literature on the online feedback practice in higher education, emphasizing the need for institutions to adapt to the ongoing shift toward online education accelerated by the COVID-19 pandemic.
In the traditional in-class teaching method, limitations regarding time, space, and accessible learning resources that can be revisited multiple times tend to restrict learning outcomes, as well as students' ability to achieve the skills and knowledge needed to meet CLOs. In contrast, the online digital teaching method allows students to review instructional actions multiple times using various resources such as video files, image files, and text-based guidance. Additionally, with the flexibility to arrange their learning time and space to fit their individual needs, this approach generally results in improved learning outcomes and better skill and knowledge acquisition, aligning more closely with course objectives.
In comparing our study to the work of Flórez et al. (2023), we note that while both studies examine different learning methods, they approach the topic from distinct angles. Flórez's research focuses on engaging virtual classrooms in synchronous distance learning, whereas our study contrasts online live instruction with asynchronous learning. Notably, our research presents detailed demographic information regarding study size and participants, which Flórez's study lacks. Both studies, however, converge on the conclusion that modern learning methods can achieve results equivalent to or superior to traditional classroom instruction. Additionally, our inclusion of instructor surveys provides insights into CLOs that Flórez's study does not address, allowing for a more comprehensive evaluation of the educational effectiveness.
The results of our study demonstrate that the online feedback practice model can serve as a viable alternative to the traditional face-to-face practice for experiment-based courses. This is particularly pertinent for institutions looking to expand their online offerings and maintain high educational standards. In the online feedback practice model, learners regular receive feedback message about learning requests, adaptation of learning outcomes to CLOs, how to improve the outcomes of performance and learning, and self—feedback by self—find out the feedback contents, self—analyze to properly understand the feedback contents, self—practice, adjust, and improve the learning outcomes. This feedback process contribute to improve the learners' outcomes of performance and learning such as the practical results and self—directed learning ability (Lipnevich and Smith, 2022). Institutions can provide accessible learning opportunities that rival those of conventional classroom settings. However, it is essential to recognize the limitations and challenges associated with the online feedback practice model. Our findings indicate that motivation and self—efficacy may contribute to learners' differential processing of self—directed learning and self—feedback in an online environment (Lipnevich and Panadero, 2021). This highlights the importance of robust support systems for online learners, including regular instructor communication, peer support networks, and access to academic advising and tutoring services.
The effectiveness of the online feedback practice model may also vary based on specific subject matter and course objectives. While our study centers on experiment-based courses in electronics and automation, further research is necessary to explore the model's effectiveness across diverse disciplines and educational contexts. Institutions should carefully assess the suitability of the online feedback practice model for different courses, considering factors such as the subject matter, learning objectives, and the unique characteristics of their student populations.
Moreover, the role of instructors within the online feedback practice model cannot be overstated. Successful online learning initiatives require instructor buy-in and active support. Therefore, institutions should invest in professional development for instructors, equipping them with the skills to design and deliver effective online courses while providing ongoing support to enhance the quality of the learning experience.
As we reflect on the long-term implications of this shift toward online learning, we recognize the benefits it offers in terms of flexibility, accessibility, and cost-effectiveness. Nonetheless, institutions face new challenges related to infrastructure, resources, and student support. Continued research and evaluation will be crucial for ensuring the quality and effectiveness of online learning programs and for identifying best practices that promote student success in the digital age.
Our study contributes valuable insights into the effectiveness of the online feedback practice model for experiment-based courses in higher education. We emphasize the potential benefits, such as flexibility and accessibility, while also acknowledging the challenges that may arise, particularly regarding student autonomy. As educational institutions adapt to this changing landscape, it is essential to carefully consider the implications of our findings and engage in ongoing research to maintain the quality and effectiveness of online learning programs. Future research should explore the model's scalability and applicability across different disciplines, ensuring that it meets the diverse needs of students in the evolving educational environment.
The primary objective of this study was to propose and evaluate an Asynchronous online teaching and learning model tailored for practice laboratory-based courses in engineering and technology education, specifically for distance education. This model is proposed based on the student-feedback interaction model (Lipnevich and Smith, 2022), and called the online feedback practice model. The findings of this research fill significant gaps in the literature by providing evidence that online feedback practice model can effectively deliver practical, experiment-based courses, a domain traditionally dominated by face-to-face practice.
The teaching experiment involving 200 students of both control and experimental groups revealed that the average scores of students in the online feedback practice model were higher than those in the traditional face-to-face practice model. This finding suggests that the online feedback practice methods can be as effective, if not more so, than traditional methods. Theoretical implications include the potential for the online feedback practice model to enhance accessibility and flexibility in education, challenging the notion that practical, hands-on subjects require in-person instruction. In the independent experiments with 100 students in the control group and 100 students in experimental group, the learning outcomes from the online feedback practice model were comparable to those from the traditional practice model. Expert assessments indicated that the online model meets most learning outcomes effectively. This demonstrates that the online feedback practice model can achieve similar educational goals as face-to-face practice, underscoring its viability as an alternative educational method. These findings support the theory that well-designed online learning environments can replicate the effectiveness of traditional classrooms.
This study concludes that the online feedback practice model is highly effective for laboratory-based courses and can be widely applied in educational institutions. The implications for theory include the validation of online feedback practice. Practically, institutions can expand their online course offerings while maintaining educational quality. Policy-wise, there is a call for greater investment in digital learning infrastructures and professional development for educators to optimize online teaching.
The study's primary limitation is its context-specific implementation, as the case study was conducted in Vietnam. The model's effectiveness may vary across different cultural and educational settings. Additionally, the reliance on average scores and expert surveys may not capture the full spectrum of student experiences and learning outcomes. Future research should address these limitations by conducting similar studies in diverse educational contexts and employing more comprehensive evaluation metrics. Future research should explore the adaptation of the online feedback practice model to various educational environments globally, examining its effectiveness across different cultures and institutional settings. To develop this model in the future, potential research directions include: (1) Expanding application to non-practical subjects, such as social sciences or arts; (2) Integrating AI to personalize learning experiences and automate result assessment; (3) Developing virtual reality and augmented reality tools to enhance interactivity; (4) Studying the long-term impact of the model on students' learning outcomes and professional skills. Additionally, research should focus on developing and assessing support systems that enhance student autonomy and motivation in online learning environments.
The original contributions presented in the study are included in the article/supplementary material, further inquiries can be directed to the corresponding author.
The studies involving humans were approved by HCMC Universities of Technology and Education. The studies were conducted in accordance with the local legislation and institutional requirements. The participants provided their written informed consent to participate in this study.
V-PD: Conceptualization, Data curation, Formal analysis, Visualization, Writing – review & editing, Investigation, Resources, Validation, Writing – original draft. T-LN: Conceptualization, Data curation, Methodology, Project administration, Resources, Writing – review & editing, Formal analysis, Funding acquisition, Validation, Visualization, Writing – original draft. V-HB: Conceptualization, Methodology, Writing – original draft, Formal analysis, Resources, Data curation, Investigation, Project administration, Software, Supervision, Validation, Visualization, Writing – review & editing. V-HT: Data curation, Formal analysis, Investigation, Resources, Software, Writing – review & editing, Methodology, Validation, Visualization, Writing – original draft. PP: Data curation, Formal analysis, Resources, Software, Writing – review & editing, Conceptualization, Investigation, Methodology, Writing – original draft. MD-N: Data curation, Formal analysis, Software, Writing – review & editing, Investigation, Methodology, Resources, Validation, Visualization, Writing – original draft.
The author(s) declare financial support was received for the research, authorship, and/or publication of this article. This research was supported by the Ho Chi Minh University of Technology and Education, project grant number CT 2022.06.SPK.06, funded by the Ministry of Education and Training, and hosted by the Ho Chi Minh University of Technology and Education, Vietnam.
The authors declare that the research was conducted in the absence of any commercial or financial relationships that could be construed as a potential conflict of interest.
All claims expressed in this article are solely those of the authors and do not necessarily represent those of their affiliated organizations, or those of the publisher, the editors and the reviewers. Any product that may be evaluated in this article, or claim that may be made by its manufacturer, is not guaranteed or endorsed by the publisher.
Abuhassna, H., and Yahaya, N. (2018). Students' utilization of distance learning through an interventional online module based on moore transactional distance theory. Eur. J. Math. Sci. Technol. Educ. 14, 3043–3052. doi: 10.29333/ejmste/91606
Ahmed, K., and Mesonovich, M. (2019). Learning management systems and student performance. Int. J. Sustain. Energy Dev. 7, 582–591s. doi: 10.20533/ijels.2046.4568.2019.0073
Alarifi, B. N., and Song, S. (2024). Online vs in-person learning in higher education: effects on student achievement and recommendations for leadership. Hum. Soc. Sci. Commun. 11:86. doi: 10.1057/s41599-023-02590-1
Anyaoku, A. (2008). Assessment of implementation of ICT – driven secondary education in Owerri municipal council of Imo State towards national development. J. Qual. Educ. 9:3.
Bangert-Drowns, R. L., Kulik, C.-L. C., Kulik, J. A., and Morgan, M. (1991). The instructional effect of feedback in test-like events. Rev. Educ. Res. 61, 213–238. doi: 10.3102/00346543061002213
Basil, C., and Oguguo, E. (2020). Effect of learning management system on Student's performance in educational measurement and evaluation. Educ. Inform. Technol. 26, 1471–1483. doi: 10.1007/s10639-020-10318-w
Bignell, S., and Parson, V. (2010). Best practice in virtual worlds teaching: a guide to using problem-based learning in second life. Available at: http://previewpsych.org/BPD2.0.pdf
Breuch, L.-A. K. (2004). Virtual Peer Review: Teaching and Learning About Writing in Online Environments. New York, NY: State University of New York Press. doi: 10.1353/book4797
Brinson, J. R. (2015). Learning outcome achievement in non-traditional (virtual and remote) versus traditional (hands-on) laboratories: a review of the empirical research. Comput. Educ. 87, 218–237. doi: 10.1016/j.compedu.2015.07.003
Carless, D., and Boud, D. (2018). The development of student feedback literacy: enabling uptake of feedback. Assess. Eval. Higher Educ. 43, 1315–1325. doi: 10.1080/02602938.2018.1463354
Chemsi, G., Sadiq, M., Radid, M., and Talbi, M. (2020). Study of the self-determined motivation among students in the context of online pedagogical activities. Int. J. Emerg. Technol. Learn. 15, 17–29. doi: 10.3991/ijet.v15i05.11392
Dawson, P., Henderson, M., Ryan, T., Mahoney, P., Boud, D., Phillips, M., et al. (2018). “Technology and feedback design,” in: Learning, Design and Technology: An International Compendium of Theory, Research, Practice and Policy, eds J. M. Spector, B. B. Lockee, and M. D. Childress (Cham: Springer). doi: 10.1007/978-3-319-17727-4_124-1
Dhakal, B. P. (2023). Digital pedagogy: an effective model for 21st century education. Acad. J. Mathe. Educ. 5, 1–9. doi: 10.3126/ajme.v5i1.54534
Dias, S. B., Hadjileontiadou, S. J., Diniz, J., and Hadjileontiadis, L. J. (2020). Deep LMS: a deep learning predictive model for supporting online learning in the Covid-19 era. Sci. Rep. 10, 19888–19888. doi: 10.1038/s41598-020-76740-9
Flórez, M., Collazos, C. A., and Hurtado, J. A. (2023). Evaluating an immersive virtual classroom as an augmented reality platform in synchronous remote learning. Information 14:543; doi: 10.3390/info14100543
Hmelo-Silver, C. (2004). Problem-based learning: what and how do students learn? Educ. Psychol. Rev. 16, e235–e266. doi: 10.1023/B:EDPR.0000034022.16470.f3
Hou, J., Zhang, K., Yang, X., and Gao, H. (2014). M-learning course development and practice based on tutor-directed online teaching. EITT 2014, 21–28. doi: 10.1109/EITT.2014.12
ISCED (2012). International Standard Classifcation of Education (ISCED) 2011. Succursale Centre-Ville Montreal, QC, Canada.
Jensen, L. X., Bearman, M., and Boud, D. (2021). Understanding feedback in online learning – a critical review and metaphor analysis. Comput. Educ. 173:104271. doi: 10.1016/j.compedu.2021.104271
Jongsma, M. V., Scholten, D. J., van Muijlwijk-Koezen, J. E., and Meeter, M. (2023). Online versus offline peer feedback in higher education: a meta-analysis. J. Educ. Comp. Res. 61, 329–354. doi: 10.1177/07356331221114181
Junaid, I., and Sigala, M. (2024). Is online learning effective for practice-based tourism courses? Tour. Hosp. Manage. 30, 225–238. doi: 10.20867/thm.30.2.6
Koneru, I. (2017). Exploring moodle functionality for managing open distance learning e-assessments. Turk. Online J. Dist. Educ. 18, 129–141. doi: 10.17718/tojde.340402
Kulhavy, R. W., and Stock, W. A. (1989). Feedback in written instruction: the place of response certitude. Educ. Psychol. Rev. 1, 279–308. doi: 10.1007/bf01320096
Latifi, S., Noroozi, O., Hatami, J., and Biemans, H. J. (2021). How does online peer feedback improve argumentative essay writing and learning? Innov. Educ. Teach. Int. 58, 195–206. doi: 10.1080/14703297.2019.1687005
Lipnevich, A. A., Berg, D., and Smith, J. K. (2016). “Toward a model of student response to feedback,” in Human Factors and Social Conditions in Assessment, eds G. T. L. Brown and L. Harris (New York, NY: Routledge), 169–185.
Lipnevich, A. A., and Panadero, E. (2021). A review of feedback models and theories: descriptions, definitions, and conclusions. Front. Educ. 6:720195. doi: 10.3389/feduc.2021.720195
Lipnevich, A. A., and Smith, J. K. (2022). Student – feedback interaction model: revised. Stud. Educ. Eval. 75:101208. doi: 10.1016/j.stueduc.2022.101208
Magalhães, P., Ferreira, D., Cunha, J., and Rosário, P. (2020). Online vs traditional homework: a systematic review on the benefits to students' performance. Comput. Educ. 152:103869. doi: 10.1016/j.compedu.2020.103869
Michela, B., Bagnasco, A., Aleo, G., Catania, G., Bona, M., Gabriele Scaglia, S., et al. (2019). The effectiveness of web-based learning in supporting the development of nursing students' practical skills during clinical placements: a qualitative study. Nurse. Educ. Pract. 37, 56–61. doi: 10.1016/j.nepr.2019.02.009
Munoz, C. L., and Towner, T. L. (2009). “Opening Facebook: how to use Facebook in the college classroom,” in: Proceedings of Society for Information Technology & Teacher Education International Conference, eds I. Gibson, R. Weber, K. McFerrin, R. Carlsen, and D. Willis (Chesapeake, VA: Association for the Advancement of Computing in Education (AACE)), 2623–2627.
O'Donnell, E. (2012). Virtual Learning Environments: Concepts, Methodologies, Tools and Applications. Hershey, PA: Information Science Reference. doi: 10.4018/978-1-4666-0011-9.ch503
Oxford University Press (2015). Learn about virtual learning environment/course management system content. Available at: http://tinyurl.com/o54enla
Pumahapinyo, S., and Suwannatthachote, S. (2014). Factors affecting the innovation-decision Process to adopt online graduate degree program in Thailand. Proceedings of International e-Learning Conference 2014, Bangkok, Thailand. Available at: http://tinyurl.com/qgnoct3
Restiglian, E., Raffaghelli, J. E., Gottardo, M., and Zoroaster, P. (2023). Pedagogical documentation in the era of digital platforms: early childhood educators' professionalism in a dilemma. Educ. Policy Anal. Arch. 31:7909. doi: 10.14507/epaa.31.7909
Russell, C. B., and Gabriela, W. (2011). A comparative study of traditional, inquiry-based, and research-based laboratory curricula: impacts on understanding of the nature of science. Chem. Educ. Res. Pract. 12, 57–67. doi: 10.1039/C1RP90008K
Sadler, D. R. (1989). Formative assessment and the design of instructional systems. Instr. Sci. 18, 119–144. doi: 10.1007/bf00117714
Setiadi, P. M., Alia, D., Sumardi, S., Respati, R., and Nur, L. (2021). “Synchronous or asynchronous? Various online learning platforms studied in Indonesia 2015–2020,” in Journal of Physics. Conference Series, 1987 (Bristol: IOP Publishing). doi: 10.1088/1742-6596/1987/1/012016
Siemens, G. (2005). Connectivism: a learning theory for the digital age. Int. J. Instruct. Technol. Distance Learn. 2:310.
Smolyaninova, O., and Bezyzvestnykh, E. (2019). Implementing teachers' training technologies at a federal university: E-portfolio, digital laboratory, PROLog module system. Int. J. Online Biomed. Eng. 15, 69–87. doi: 10.3991/ijoe.v15i04.9288
Sri Jothi, P., Neelamalar, M., and Prasad, R. S. (2011). Analysis of social networking sites: a study on effective communication strategy in developing brand communication. J. Media Commun. Stud. 3, e234–e242.
Tan, S. C., Voogt, J., and Tan, L. (2024). Introduction to digital pedagogy: a proposed framework for design and enactment. Pedag. Int. J. 19, 327–336. doi: 10.1080/1554480X.2024.2396944
Keywords: laboratory learning model, virtual learning environment, Moodle, digital pedagogy, digital platform, feedback theory
Citation: Dao V-P, Nguyen T-L, Bui V-H, Tran V-H, Phan PN-T and Nguyen M-D (2025) Development of a laboratory-based learning model via digital platform environment. Front. Educ. 9:1495724. doi: 10.3389/feduc.2024.1495724
Received: 13 September 2024; Accepted: 17 December 2024;
Published: 08 January 2025.
Edited by:
Wei Wei, Macao Polytechnic University, ChinaReviewed by:
Janika Leoste, Tallinn University, EstoniaCopyright © 2025 Dao, Nguyen, Bui, Tran, Phan and Nguyen. This is an open-access article distributed under the terms of the Creative Commons Attribution License (CC BY). The use, distribution or reproduction in other forums is permitted, provided the original author(s) and the copyright owner(s) are credited and that the original publication in this journal is cited, in accordance with accepted academic practice. No use, distribution or reproduction is permitted which does not comply with these terms.
*Correspondence: Van-Hong Bui, aG9uZ2J2QGhjbXV0ZS5lZHUudm4=
†ORCID: Van-Phuong Dao orcid.org/0009-0003-4803-9430
Thi-Luong Nguyen orcid.org/0009-0009-3426-3267
Van-Hong Bui orcid.org/0000-0002-0690-2027
Vu-Hoang Tran orcid.org/0000-0002-1352-4853
Phuong Nguyen-Truc Phan orcid.org/0009-0008-3863-0442
Disclaimer: All claims expressed in this article are solely those of the authors and do not necessarily represent those of their affiliated organizations, or those of the publisher, the editors and the reviewers. Any product that may be evaluated in this article or claim that may be made by its manufacturer is not guaranteed or endorsed by the publisher.
Research integrity at Frontiers
Learn more about the work of our research integrity team to safeguard the quality of each article we publish.