- 1Graduate School of Education, Stanford University, Stanford, CA, United States
- 2Department of Psychology, Stanford University, Stanford, CA, United States
- 3Division of Developmental Behavioral Pediatrics, School of Medicine, Stanford University, Stanford, CA, United States
- 4Machine Learning Team, Cambium Assessment Inc., Washington, DC, United States
- 5Department of Speech and Hearing Sciences, University of Washington, Seattle, WA, United States
Introduction: The speed at which students can accurately read and understand connected text is at the foundation of reading development. Timed reading measures go under a variety of names (e.g., reading fluency, reading efficiency, etc) and involve different levels of demands on comprehension, making it hard to interpret the extent to which scores reflect differences in reading efficiency versus comprehension.
Methods: Here we define a new measure of silent sentence reading efficiency (SRE) and explore key aspects of item development for an unproctored, online SRE assessment (ROAR-SRE). In doing so, we set forth an argument for developing sentences that are simple assertions, with an unambiguous answer, requiring minimal background knowledge and vocabulary. We then run a large-scale validation study to document convergent validity between ROAR-SRE and other measures of reading. Finally we validate the reliability and accuracy of using artificial intelligence (AI) to generate matched test forms.
Results: We find that a short, one-minute SRE assessment is highly correlated with other reading measures and has exceptional reliability. Moreover, AI can automatically generate test forms that are matched to manually-authored test forms.
Discussion: Together these results highlight the potential for regular screening and progress monitoring at scale with ROAR-SRE.
Introduction
The use of assessments to identify students struggling with foundational reading skills is a priority across the country (Catts and Hogan, 2020; Odegard et al., 2020; Fletcher et al., 2021; Jones, 2022; Rice and Gilson, 2023). Assessments of phonological awareness, letter-sound knowledge, and decoding skills are widely used for screening and benchmarking early in elementary school (Fletcher et al., 2021), and are written into many states’ dyslexia screening legislation (Ward-Lonergan and Duthie, 2018; Zirkel, 2020). But as reading skills develop, the fluency with which children can read connected text becomes particularly important (Silverman et al., 2013). “Efficient word recognition” was highlighted in the original conceptualization of the “simple view of reading” (Hoover and Gough, 1990), and fluent reading has been implicated as a bridge between decoding skills and reading comprehension (Pikulski and Chard, 2005; Silverman et al., 2013). Children with dyslexia and other word reading difficulties often struggle to achieve fluency, and struggles with word reading speed and fluency have always been core to the definition of dyslexia (Lyon et al., 2003; Catts et al., 2024). In this paper we describe the development of a silent sentence reading efficiency (SRE) measure that was designed to be fast, reliable, efficient at scale, and targeted to the issues with speed/fluency that present a bottleneck for so many struggling readers.
Even though oral reading tasks have long been the focus of screeners, silent reading tasks have some advantages in pursuit: They can assess reading ability without requiring students to read aloud; they are not influenced by issues with articulation or pronunciation; they are amenable to administration in large, group settings (e.g., a classroom); and, if digitized, they can be scaled to an entire district or state, dramatically lowering the resources required for universal screening. The SRE measure developed here is built as part of the Rapid Online Assessment of Reading (ROAR), an online platform consisting of a suite of reading assessments. To date, validation studies have been conducted to explore the relationship between, first, a single word recognition measure (ROAR-SWR) and other standardized assessments of basic reading skills (Yeatman et al., 2021; Ma et al., 2023), and second, a phonological awareness (PA) measure (ROAR-PA) and individually administered measures of PA (Gijbels et al., 2023). One way that these two ROAR tasks differ from traditional reading assessments is that they are administered online, rather than face-to-face, and elicit silent responses from students, rather than verbal responses. The initial validation studies of these silent measures showed excellent correspondence to conventional measures that require individually scoring verbal responses (Yeatman et al., 2021; Gijbels et al., 2023; Ma et al., 2023). The focus of the present paper is the development of the third task, Silent Sentence Reading Efficiency (ROAR-SRE), which is designed to assess the speed or efficiency with which a student can read simple sentences for understanding. The goal of the ROAR-SRE task is to isolate reading efficiency by minimizing comprehension demands while maintaining checks for understanding. This stands in contrast to other silent reading measures that confound comprehension and efficiency leading to a less interpretable score (Wagner et al., 2010; Johnson et al., 2011; Wagner, 2011).
Traditional measures that are most similar to ROAR-SRE are sometimes referred to as sentence reading fluency tasks, and while they are not administered online, they do elicit silent responses from students. For example, the Woodcock Johnson (WJ) Tests of Achievement “Sentence Reading Fluency” subtest (Schrank et al., 2014), and Test Of Silent Reading Efficiency and Comprehension (TOSREC; Wagner et al., 2010), rely on an established design: A student reads a set of sentences and endorses whether each sentence is true or false. For example, the sentence, Fire is hot, would be endorsed as True. A student endorses as many sentences as they can within a fixed time limit (usually 3 min). The final score is the total number of correctly endorsed sentences minus the total number of incorrectly endorsed sentences.
Both the WJ and TOSREC are standardized to be administered in a one-on-one setting (though TOSREC can also be group administered) and the stimuli consist of printed lists of sentences which students read silently and mark True/False with a pencil. Even though the criteria for item development on these assessments is not specified in detail, there is a growing literature showing the utility of this general approach. First of all, this quick, 3 min assessment is straightforward to administer and score and has exceptional reliability, generally between 0.85 and 0.90 for alternate form reliability (Wagner et al., 2010; Johnson et al., 2011; Wagner, 2011). Moreover, this measure has been shown to be useful for predicting performance on state reading assessments: For example, Johnson and colleagues demonstrated that TOSREC scores could accurately predict students who did not achieve grade-level performance benchmarks on end-of-the-year state testing of reading proficiency (Johnson et al., 2011).
Further evidence for validity comes from the strong correspondence between silent sentence reading measures such as the TOSREC and Oral Reading Fluency (ORF) measures (Denton et al., 2011; Johnson et al., 2011; Wagner, 2011; Kim et al., 2012; Price et al., 2016; Kang and Shin, 2019). ORF is one of the most widely used measures of reading development in research and practice, and some have even argued for ORF as an indicator of overall reading competence (Fuchs et al., 2001). ORF is widely used to chart reading progress in the classroom, providing scores with units of words per minute that can be examined longitudinally [e.g., for progress monitoring (Good et al., 2002; Hoffman et al., 2009; Cummings et al., 2013)], compared across classrooms and districts, and can inform policy decisions such as how to confront learning loss from the Covid-19 pandemic (Domingue et al., 2021, 2022). Even though silent reading and ORF are highly correlated, the measures also have unique variance (Hudson et al., 2008; Wagner, 2011; Kim et al., 2012) and, theoretically, have different strengths and weaknesses. For example, even though there are strong empirical connections between ORF and reading comprehension (Kim et al., 2014), ORF does not require any understanding of the text and has been labeled by some as “barking at print” (Samuels, 2007). Silent reading, on the other hand, is the most common form of reading, particularly as children advance in reading instruction. In line with this theoretical perspective, Kim and colleagues found that silent sentence reading fluency was a better predictor of reading comprehension than ORF starting in second grade (Kim et al., 2012). Thus, given the practical benefits of silent reading measures (easy to administer and score at scale), along with the strong empirical evidence of reliability, concurrent, and predictive validity, and face validity of the measure, an online measure of silent sentence reading efficiency would be useful for both research and practice.
The strength of silent reading fluency/efficiency tasks is also their weakness: On the one hand, these tasks include comprehension, which bolsters the argument for the face validity of silent reading measures. On the other hand, what is meant by comprehension in these sentence reading tasks is often ill-defined and, thus, a low score lacks clarity on whether the student is struggling due to difficulties with “comprehension” or “efficiency/fluency.” As a concrete example, sentences in the TOSREC incorporate low frequency vocabulary words (e.g., porpoise, bagpipes, locomotive, greyhounds, buzzards) meaning that vocabulary knowledge as well as specific content knowledge (e.g., knowledge about porpoises, bagpipes and locomotives) will affect scores. While this design decision might be a strength in some scenarios (e.g., generalizability to more complex reading measures such as state testing), it presents a challenge for interpretability. An interpretable construct is critical if scores are used to individualize instruction. For example, does a fourth grade student with a low TOSREC score need targeted instruction and practice focused on (a) building greater automaticity and efficiency in reading or (b) vocabulary, syntax and background knowledge. Our goal in designing a new silent sentence reading efficiency measure was to more directly target reading efficiency by designing simple sentences that are unambiguously true or false and have minimal requirements in terms of vocabulary, syntax and background knowledge. Ideally, this measure could be used to track reading rate in units of words per minute, akin to a silent reading version of the ORF task, but with a check to ensure reading for understanding. If combined with measures of vocabulary, syntax, morphology, and inferencing skills, it could break down reading comprehension into its component processes.
To consider the ideal characteristics of these sentences, it may be helpful to begin by considering the ORF task which is used to compute an oral reading rate (words per minute) for connected text. In an ORF task, the test administrator can simply count the number of words read correctly to assess each student’s reading rate. Translating this task to a silent task that can be administered at scale online poses an issue because an administrator is unable to monitor the number of sentences read by the student. A student could be instructed to press a button on the keyboard after the completion of a sentence in order to proceed to the next one. However, the validity of this method depends on the student’s ability to exhibit restraint and wait until the completion of each sentence before proceeding to the next sentence.
In the interest of preserving the validity of the interpretations of the scores, we retain the True/False endorsement of the TOSREC and WJ, but reframe its use. That is, for the ROAR-SRE task, the endorsement of True/False should be interpreted as an indication that the student has read the sentence, rather than as an evaluation of comprehension per se. In this context, if the student has difficulty comprehending a sentence, or if the student takes a long time to consider the correct answer because the sentence is confusing, syntactically complex, or depends on background knowledge and high-level reasoning, we lose confidence in the inferences that we can make about a student’s reading efficiency. As such, it is important that sentences designed for this task are simple assertions that are unambiguously true or false.
However, creating sentences to adhere to these basic standards may not always be straightforward. For example, the statement “the sky is blue” may be true for a student in the high-plain desert in Colorado but may be a controversial statement for a student in Seattle. Thus, careful consideration must be given to crafting sentences that do not depend on specific background knowledge and are aligned with the goal of measuring reading efficiency. To support this goal, we propose the following item statistics to guide the process of evaluating field-tested items for their suitability in a sentence reading efficiency task: proportion-correct (also referred to in this paper as “agreement rate”), average response time, and sentence length.
Departing from the traditional use of the proportion-correct statistic for assessing item difficulty, a value near 1 in this context indicates that the truth of a sentence is unambiguous. Consequently, a lower value suggests that a statement is controversial or confusing, which in turn does not meet the criteria for a simple, unambiguous assertion. The response time statistic can signal that a statement is confusing or otherwise difficult to parse. Simple assertions associated with shorter response times are ideal, while longer response times may indicate confusion, particularly in the case of short sentences. Ideally, response time should incrementally increase with sentence length. In the first section of this paper (Study 1), we define criteria based on these three statistics and then systematically review sentences classified into two groups: sentences suitable for a sentence reading efficiency task, and sentences that are not ideal for this task. After arriving on a list of suitable stimuli, we next run two validation studies (Study 2 and Study 3) to compare performance on ROAR-SRE to the TOSREC (Wagner et al., 2010), Woodcock Johnson (WJ; Schrank et al., 2014) and Test of Word Reading Efficiency (TOWRE; Torgesen et al., 2011). Finally, Study 4 considers how the use of these statistics can scale for developing a large item bank for progress monitoring, relying on automated, AI-based approaches to generating and evaluating new sentences.
Study 1: creation and analysis of sentence reading efficiency items
Study 1: methods
Study 1 was an exploratory analysis to understand which sentences make good stimuli for the construct of “Sentence Reading Efficiency.” In this study, we authored 200 sentences (hereafter SRE-Pilot) with the intention of being (a) unambiguously true or false, (b) requiring minimal background knowledge, and (c) using simple vocabulary words and syntactic structure. We built a simple web application with PsychoPy and hosted it on Pavlovia for data collection (Peirce and MacAskill, 2018; Peirce et al., 2019). Participants (ages 5 to adulthood) were instructed to endorse as many sentences as possible within two separate three-minute blocks. The first block consisted of the 200 SRE-Pilot sentences presented in a random order. The second block consisted of stimuli from the TOSREC (used with permission), presented in the predetermined order of the published assessment, with a separate form for each grade level. To properly assign the appropriate grade level form, the web application asked participants to select their grade and the appropriate TOSREC stimulus list was selected for the grade (the 8th grade form was used for everyone in eighth grade or higher); SRE-Pilot used the same item bank in a random order irrespective of grade.
Study 1 comprised two distinct samples of participants: (1) recruited at Stanford University and University of Washington, aged 5 to 39 years-old, and (2) recruited from local school partnerships, many of whom were identified as experiencing difficulties with reading, within the grade range of third to seventh grade (see Table 1). A total of 173 participants completed the online task. Because each trial is a two-alternative forced choice (2AFC), participants who are guessing would be expected to answer approximately 50% of the items correctly. There were 16 participants who performed below 60% correct and were excluded due to a high likelihood of random guessing, leaving us with a final sample of 151 participants (participants with low accuracy also tend to respond very quickly relative to their peers, indicative of random guessing).
The primary objective of this first study was to investigate three item-level statistics for classifying sentences into those that are appropriate for the task and those that are problematic due to their controversial nature or potential to cause confusion. In this section, we conduct a qualitative inspection of the flagged items to assess if they are, indeed, in violation of the basic requirement of simple assertions that are obviously true or false.
Code to reproduce analyses and figures is available at: https://github.com/yeatmanlab/ROAR-SRE-Public.
Study 1: results
Flagging criteria
Further analyses consider 199 SRE-Pilot sentences that had responses from at least 25 participants. To evaluate these sentences, we calculated three item-level statistics for each sentence: the proportion of participants who agreed with the answer key’s truth of the assertion (referred to as the agreement rate), the average response time, and the length of the sentence. Figure 1 plots the sentences along two dimensions based on the average response time and the agreement rate. In the context of a sentence reading efficiency task, it’s crucial for items to be relatively easy and clearly interpretable as either true or false. To ensure this, sentences with a low agreement rate (<85%; 38 sentences) were flagged and filtered out of the final item bank. These flagged sentences underwent a closer inspection to discern the qualitative characteristics that make them unsuitable for the assessment.
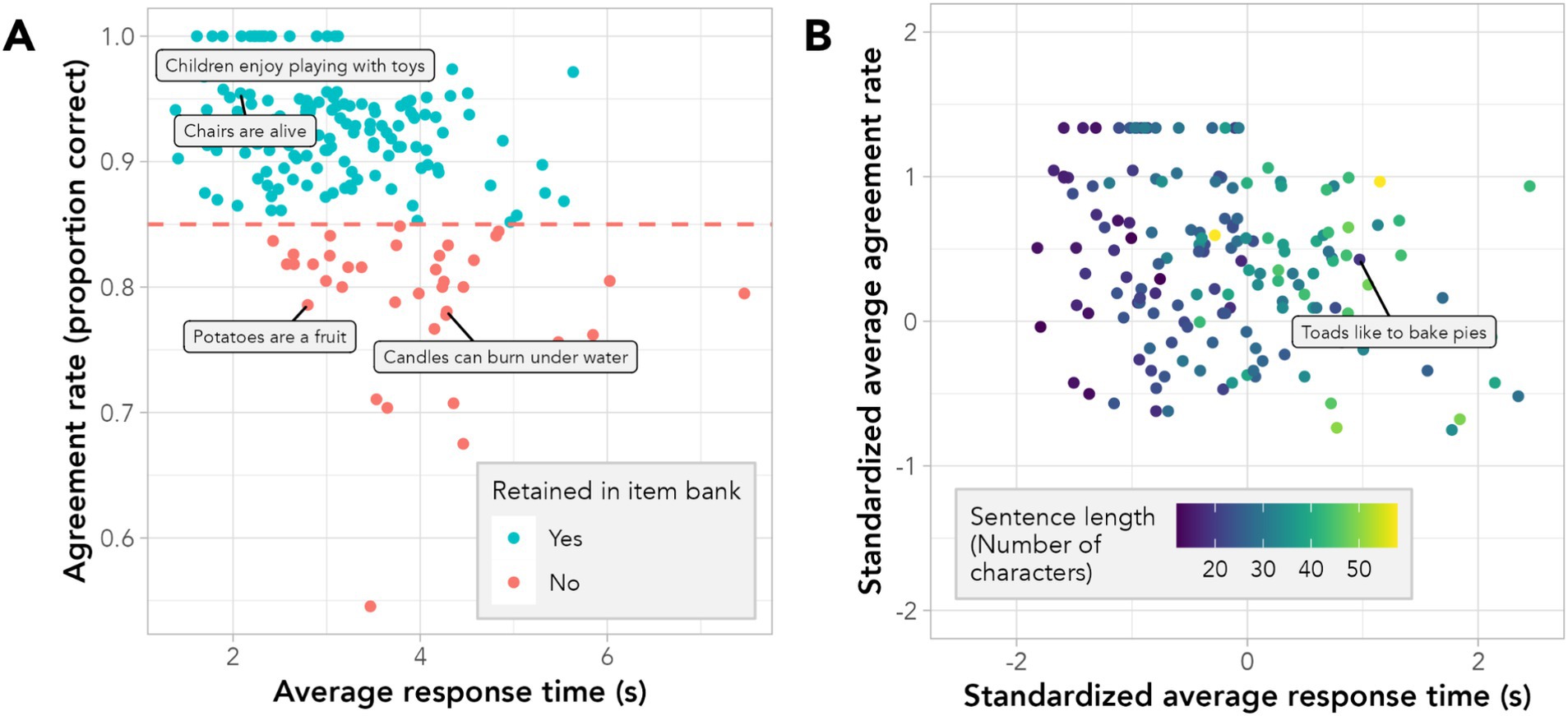
Figure 1. (A) Scatterplot of the agreement rate (y-axis) and average response time (x-axis) for 199 SRE-Pilot items. Sentences with an agreement rate less than 85% were filtered out from the final item bank. (B) Scatterplot of the standardized average agreement rate (y-axis) and standardized response time (x-axis) for 161 SRE-Pilot items. The gradient color of the dots on the plot corresponds to the number of words in each sentence, with darker colored dots indicating shorter sentences and lighter colored dots indicating longer sentences.
Reviewing flagged versus suitable sentences
Suitable sentences were ideal for their unambiguity — either they were clear statements that seemed to resonate with the lived experiences of students’ lives, or they were fantastical in nature and clearly not true. For instance, simple true statements such as “Children enjoy playing with toys,” “A pillow can be very soft,” and “Sandwiches are food” were quickly endorsed by students, perhaps because they are aligned with their everyday experiences. In contrast, false statements that are outlandish, such as “Chairs are alive,” and “Lizards like to cook pasta” are easily recognized as false by students, perhaps because they do not interfere with students’ expectations of what constitutes a true, lived experience.
Sentences with low agreement rates seemed confusing or ambiguous in nature. For instance, “Potatoes are fruit,” may have been confused with the notion that tomatoes are fruit, and it also depends on background knowledge that will vary among participants. Similarly, the assertion “Candles burn underwater” also received low agreement, as some may be familiar with a science experiment that demonstrates that a candle can burn with a flame beneath the water level for a brief period. While the correct answer might seem obvious to some, the intention of the question might be ambiguous to others leading to confusion as to the correct answer.
It is worth noting that not all sentences with low agreement rates seemed to pose confusing or controversial scenarios for some students. In fact, some sentences that are closer to the criterion’s threshold appear to be simple assertions. For example, “Water is always cold” and “All fish live in the water” had agreement rates of 82 and 83%, respectively. One feature of these sentences is the inclusion of modifiers like “always” and “all” which seemed to have confused participants to try to consider fringe cases where the statement might not always be true. There were also sentences consisting of only a few words that exhibited an above-average agreement rate, but also a longer-than average response time such as “Toads like to bake pies” (see Figure 1). There was no clear consensus on why participants took longer to respond to this sentence; it could be due to students having to spend too much time thinking through the scenario. However, the sentence was ultimately removed because the extended reaction time and short sentence length indicated a certain level of confusion. After removing 38 sentences with low agreement rates and 1 short sentence that had a longer-than average response time, 160 sentences remained for construction of a final test form.
Creating the final test form
To obtain an equal number for true and false sentences in the final sample, the remaining 160 sentences were categorized into three item difficulty (“easy,” “medium,” “hard”) bins based on the agreement rate. Sentences with an agreement rate above 95% were classified as “easy,” those between 95 and 90% as “medium,” and those below 90% as “hard.” The R package MatchIt was utilized to generate pairs of true and false sentences for each difficulty by using agreement rate as a covariant to estimate propensity scores. Every true sentence was matched with an available false sentence that had the closest propensity score, and any unmatched sentences were removed from the final item bank. Once the matched pairs were established, a total of 130 sentences were selected, comprising 40 easy, 64 medium, and 26 hard sentences. These sentences were arranged in ascending order of difficulty, from easy to hard, and then randomized within their respective difficulty bins. This process resulted in the fixed order form, now referred to as SRE-Fixed, that will be used for future iterations of the sentence reading efficiency task. The mean Flesch–Kincaid readability statistic of SRE-Fixed items was 3.03 (SD = 3.12).
How stable is silent sentence reading efficiency across different sentence constructions?
In the process of determining which sentences are appropriate for a sentence reading efficiency task, a fundamental question remained: how similar are responses to SRE stimuli versus standardized reading assessment such as the TOSREC? Figure 2 illustrates a high correlation between total scores based on (a) a random sample of SRE items (SRE-Pilot), (b) SRE-Fixed and (c) grade-specific TOSREC test forms. Despite the meticulous curation of the SRE-Fixed form, Sentence Reading Efficiency seems to be a stable construct that (a) is reliably measured with a short, online assessment and (b) varies substantially across participants. The variability in reading efficiency is so substantial that the intricacies of the sentences play a relatively minor role in comparison. Factors like sentence length will, of course, impact scores but do not seem to have a large impact on the rank ordering of participants. Thus, authoring sentences with a specific framework can aid in the interpretability but sentence characteristics are not the primary factor driving individual differences.
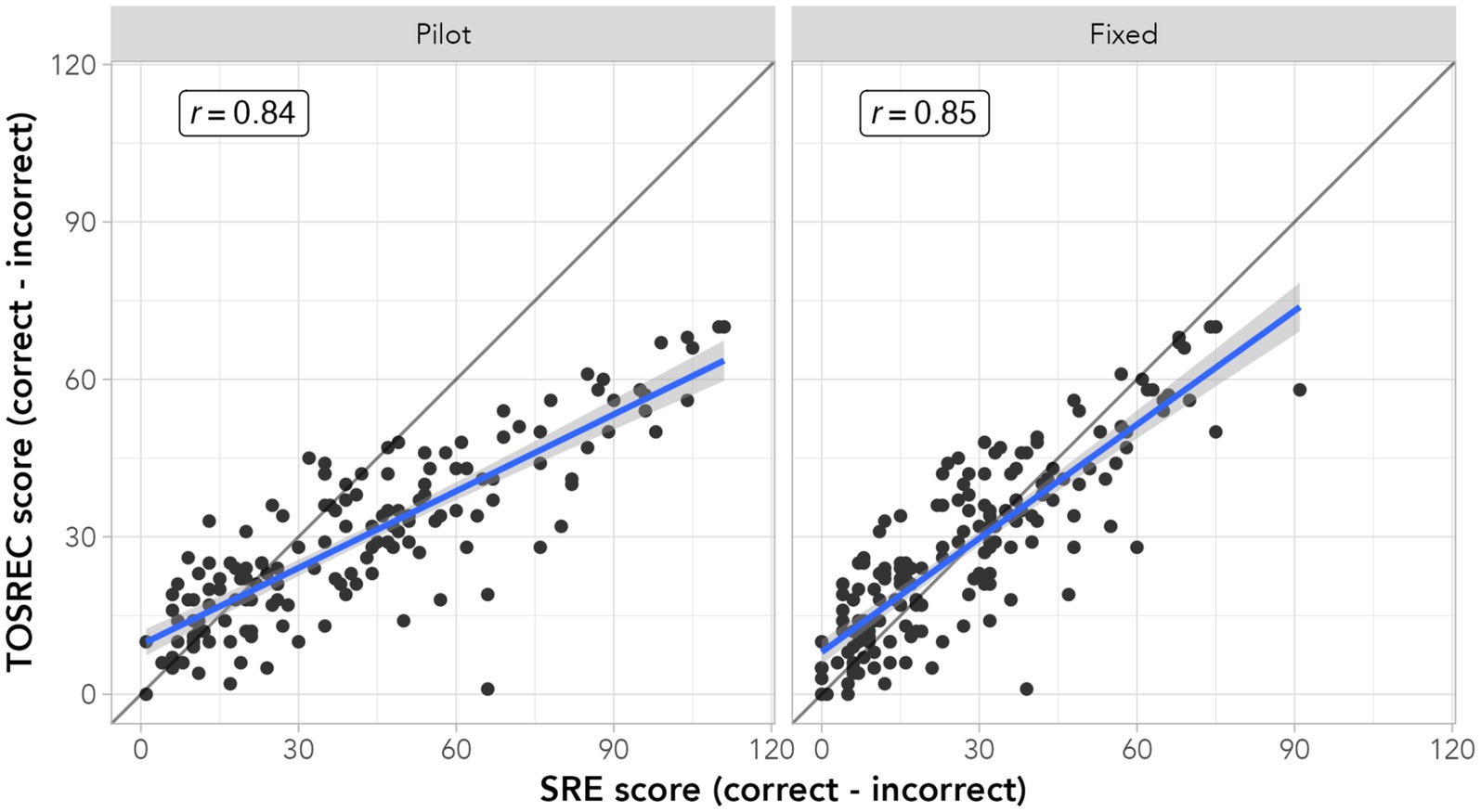
Figure 2. Scatterplot of the TOSREC final score (y-axis) and SRE score (x-axis) for both the pilot and final fixed form, highlighting the strong relationship between the two measures regardless of decisions concerning the sentence stimuli.
Study 1: discussion and limitations
We proposed a revised construct to the conventional sentence reading fluency task, which we refer to as sentence reading efficiency. This new construct entails a revised interpretation of the purpose of true/false statements used commonly in silent sentence reading fluency tasks (Wagner et al., 2010; Johnson et al., 2011; Wagner, 2011), whereby the focus is less on testing comprehension and more on assessing speed or efficiency. Consequently, sentences must be simple and unambiguous without low-frequency vocabulary words and with minimal requirements in terms of background knowledge.
Through our case study, we demonstrate that agreement rate, response time, and sentence length seem to effectively distinguish problematic sentences from suitable sentences. Sentences that were highly agreeable contained unambiguous assertions that did not require specific content knowledge to validate their truth (unambiguously True), or were fantastical and unrelated to real-world experiences (unambiguously False). Conversely, flagged sentences varied in their reasons for being challenging. These included there being reasonable arguments for either a true or false endorsement, depicting scenarios that required imaginative thinking to resolve, or otherwise being generally confusing. A major limitation of this study was that the qualitative review of flagged sentences is susceptible to confirmation bias, and there are likely other factors at play beyond the ones we considered.
This first study provides guidance on how to craft and flag sentences that could potentially result in inaccurate inferences of reading efficiency. Our analysis suggests that sentences should be written to either resonate with lived experience for students (true sentences) or should be fantastical in nature (false sentences). Study 1 resulted in a clearer definition of the SRE construct and a SRE test form (“SRE-Fixed”) that could be studied in a larger, quantitative validation study (Study 2).
Study 2: validation of ROAR-SRE in a school setting
The goal of Study 2 was to validate ROAR-SRE as a rapid screening tool in a school setting. To this end we first examine the correspondence between ROAR-SRE and TOSREC in a large and diverse sample spanning first through eighth grade. This analysis serves to determine (a) the reliability of an online sentence reading efficiency measure in a natural, large-scale school setting, and (b) examine the suitability of using a single form of simple sentences in a fixed order (SRE-Fixed) to measure reading efficiency across a broad age range.
Study 2: methods
ROAR assessments were administered to 3,660 participants, across 23 schools through a research-practice-partnership (RPP) model. Many of these schools specialized in supporting students with language-based learning difficulties such as dyslexia, dyscalculia, or dysgraphia (see Table S1 for school demographics). Four ROAR assessments were included in this research: ROAR Single Word Recognition (ROAR-SWR; Yeatman et al., 2021; Ma et al., 2023), ROAR Sentence Reading Efficiency (ROAR-SRE; White et al., 2022; Burkhardt et al., 2023), ROAR Phonological Awareness (ROAR-PA; Gijbels et al., 2023), and ROAR Vocabulary (ROAR-Vocab). At each ROAR administration, students completed a varying mix of the assessments, depending on the interests of their district. The following analyses focus on ROAR-SRE. For Study 2, a new version of ROAR-SRE was built to (a) precisely log timing and (b) provide the option for light gamification to be more engaging for young children (Figure 3). All participants in Study 2 completed the gamified version of the assessment.
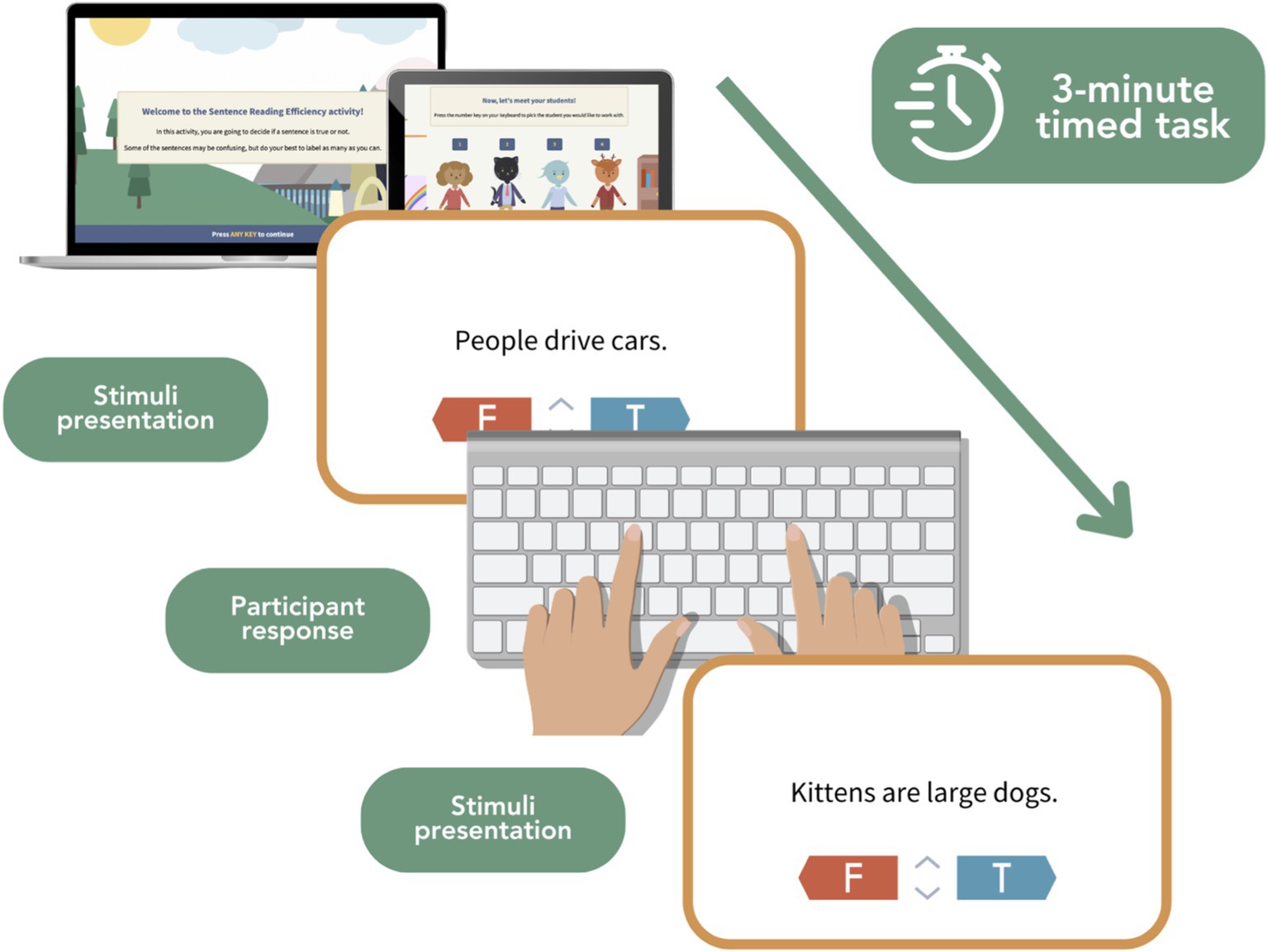
Figure 3. Gamified ROAR-SRE task. The new version of ROAR-SRE implemented for Study 2 allowed students to choose an animated character to narrate their task instructions. The task was embedded in a short story to be engaging for young participants. Optionally, the ROAR-SRE task could be run without characters or gamification for older students.
Data were analyzed with generalized additive models (GAMs) to link ROAR-SRE raw scores to TOSREC standard scores. Rather than fitting a separate model for each age/grade, we instead fit a single GAM with a 2d smoother on ROAR-SRE raw scores and age. We used a tensor smoother since the two covariates (raw score and age) have different units. We set k = 3 (three basis functions or knots) to ensure that we did not overfit the data. The model syntax was as follows:
Study 2: results
Comparison of ROAR-SRE and TOSREC
We first ask whether the SRE-Fixed form created in Study 1 can serve as a measure of silent sentence reading efficiency across a broad age range spanning 1st through 8th grade. To answer this question, we analyzed the data of 1,727 1st - 8th graders (Figures 4, 5) who completed the SRE-Fixed and TOSREC forms (TOSREC has separate test forms for each grade). We first analyzed the distribution of the participants’ accuracy and response time for SRE-Fixed and TOSREC items (Figure 4) and noted a bimodal distribution. Most students were very accurate on both SRE-Fixed (median = 94.9%) and TOSREC (median = 85.7%) with an interquartile range of median RTs spanning 1,941–3,423 ms for SRE-Fixed and 2,879–5,143 ms for TOSREC. However, there was also a cluster of students with extremely fast (<1,000 ms) or slow (>20,000 ms) response times, and accuracy near chance (<65% correct) likely indicating that they were not taking the assessment seriously and engaging in rapid guessing or idle behavior. Both these behaviors result in scores not representative of true ability. Hartigan’s dip test (Hartigan and Hartigan, 1985) confirmed a bimodal distribution of response accuracy on both SRE-Fixed (D = 0.039, p < 0.000001) and TOSREC (D = 0.014, p = 0.026). Based on these criteria, we excluded 133 participants who met the criteria of less than 65% correct, and median response time less than 1,000 ms or greater than 20,000 ms.
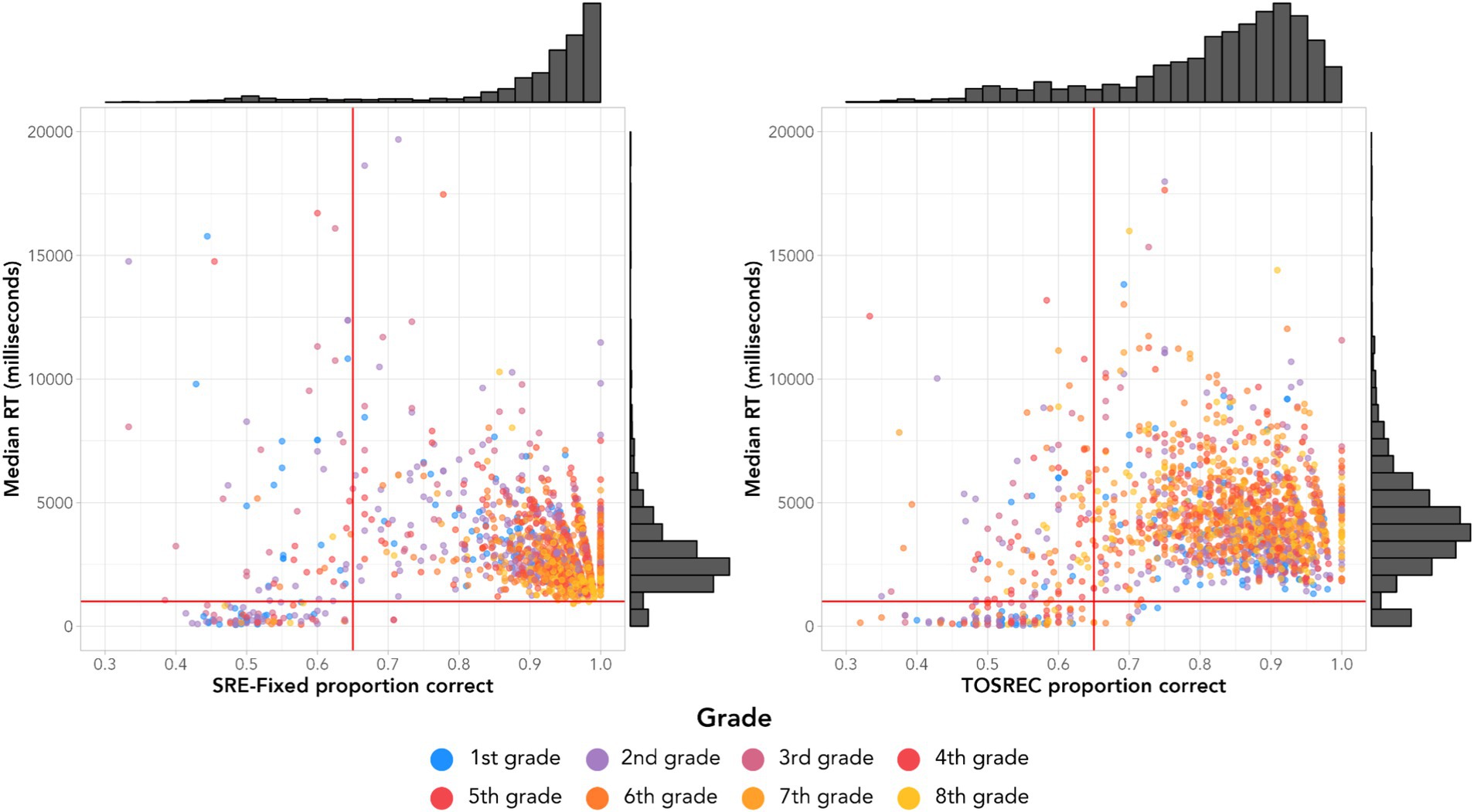
Figure 4. Distribution of median response time (RT) and proportion correct for SRE and TOSREC. Participants displaying extremely rapid responses performed near chance on both assessments indicative of disengagement and/or rapid guessing behavior. The same cut points, as indicated by the red lines, were established to flag subjects exhibiting this behavior. Note that SRE exhibits less variation in response time and proportion correct than TOSREC indicating that SRE items adhere to the criteria for determining suitable sentences that was established in Study 1.
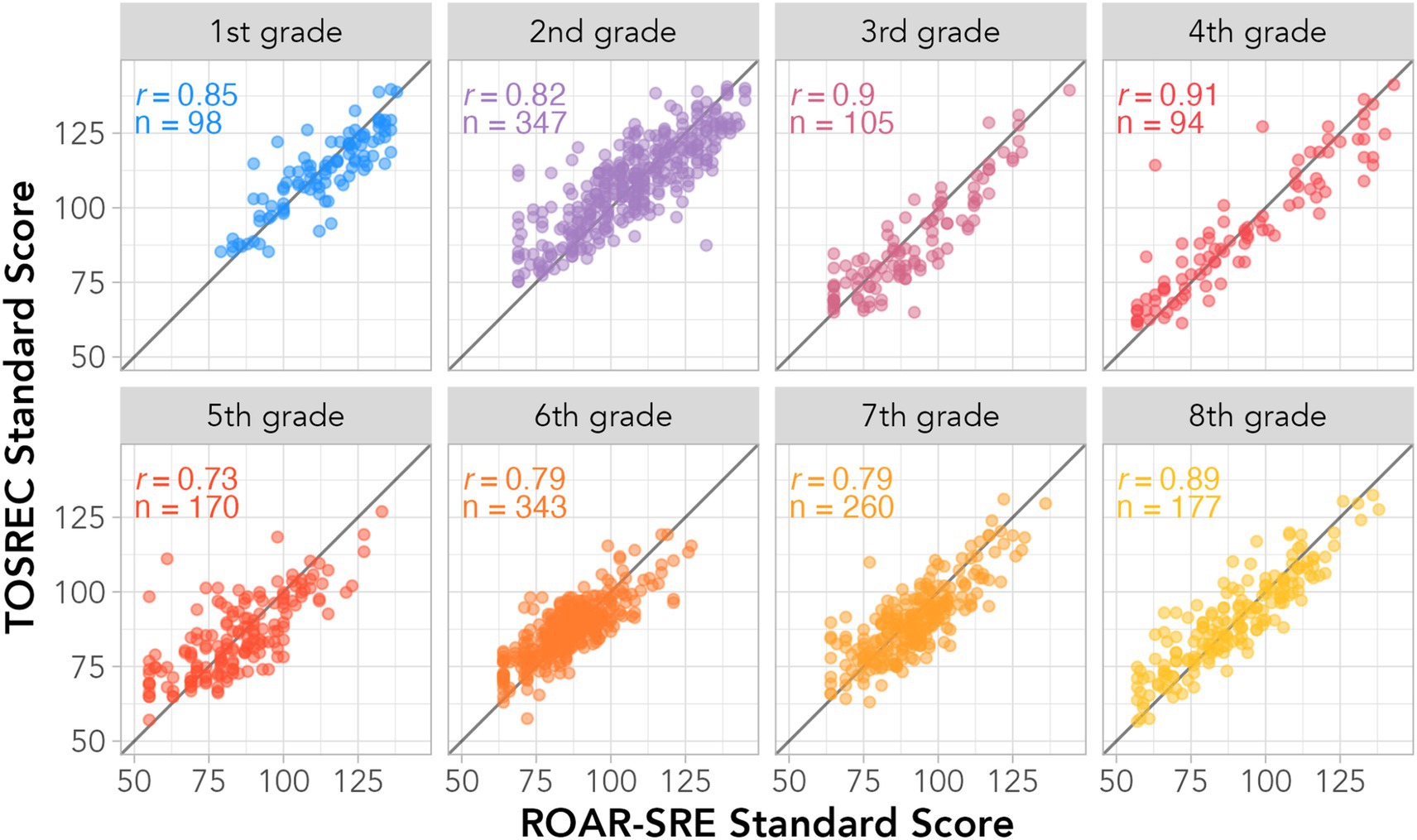
Figure 5. ROAR-SRE provides a reliable and valid measure of reading efficiency and comprehension between grades 1 and 8. Age-standardized scores on ROAR-SRE accurately predict age standardized TOSREC scores for every age (r = 0.87 based on a generalized additive model; r = 0.87 based on a local regression model). This means that (a) ROAR-SRE has high test-reliability (greater than r = 0.87) and (b) the consistent measurement scale adopted by ROAR-SRE is a valid measure of reading efficiency and comprehension between grades 1 and 8.
We then fit a generalized additive model (GAM) using tensor smoothing and the default parameters in the mgcv package (Wood and Wood, 2015; Wood, 2017) to link ROAR-SRE scores and age to TOSREC Standard scores. We found a strong and systematic relationship between ROAR-SRE and TOSREC for students across this broad age range (Figure 5). Moreover, the correlation between ROAR-SRE and TOSREC was similar across every grade level (e.g., r = 0.85 in 1st grade and r = 0.89 in 8th grade). The stability of the SRE - TOSREC relationship across an 8 year developmental window is surprising given that the simple sentences might seem to be a more suitable measure for younger versus older students. This finding supports the notion that sentence reading efficiency is a reliable construct across the grades and that items need not vary in syntax, vocabulary or content knowledge to accurately measure reading efficiency.
Study 2: discussion and limitations
We found that a single test form of simple sentences predicted a substantial portion of the variance in TOSREC scores across grades 1–8. This finding indicates that reading efficiency—or the speed with which students can silently read sentences for understanding—is the primary source of variability in performance on an assessment that was designed to measure a variety of reading skills with test forms that progress in difficulty across the grades. We argue that reading efficiency has the benefit of increased interpretability since items were designed to have minimal comprehension demands. This interpretation is supported by the fact that there is much less variability in the accuracy with which students answer questions on SRE versus TOSREC (Figure 4). However, the relationship between SRE and other measures of comprehension will be an important question for future research. It is well established that reading efficiency construct is important in reading development and contributes to comprehension (Pikulski and Chard, 2005; Kim et al., 2012, 2014; Silverman et al., 2013). Moreover, since TOSREC has been shown to be highly predictive of high-stakes, summative reading assessments [e.g., state tests (Johnson et al., 2011)], we surmise that ROAR-SRE is likely to show similar results. However, evaluating the predictive validity of ROAR-SRE as a screener and the precision of the tool for progress monitoring is an important future direction.
A limitation of Study 2 was that both measures (SRE and TOSREC) were presented in the same online platform meaning that some of the shared variance could be due to extraneous factors such as student engagement in an unproctored online assessment. Thus further validation is warranted to compare ROAR-SRE to a wider battery of measures of word and sentence reading (which we undertake in Study 3).
Study 3: construct validity of ROAR-SRE: validation against individually administered reading assessments
To confirm that the validation results in Study 2 did not reflect something esoteric about either the way that (a) measures were implemented in the ROAR platform or (b) participants interact with unproctored online assessments, we ran an additional study of construct validity to compare ROAR-SRE to individually administered, standardized assessments of reading fluency, decoding, and reading speed.
Study 3: methods
Participants for Study 3 were recruited through two methods. The initial set of validation data was obtained from a longitudinal study of children with dyslexia (ages 8–14; grades 2–8), where the trained researcher coordinators individually administered standardized assessments and participants then completed ROAR-SRE. The rest of the sample comprised 3rd grade students from a local school district that agreed to participate in the validation study (see Table S1 for school demographics). 3rd grade was selected for validation because it is the most common age for a dyslexia diagnosis. To conduct in-person validations in schools, a team of 7 researcher coordinators administered assessments to the students. All research coordinators completed human subjects research training, practiced extensively, and shadowed senior administrators before conducting assessments on students. Moreover, each research coordinator completed training with feedback until they were able to reliably administer each assessment.
The selection of students was based on the interest of parents and teachers. Prior to the research, parents and guardians were given the opportunity to opt their students out of the research. Teachers were also informed, and their interest in the research was conveyed to the district superintendent, who then notified the research team. Students were pulled out of their classrooms to complete the following standardized, individually-administered reading assessments: (1) Woodcock Johnson IV Tests of Achievement Sentence Reading Fluency (WJ-SRF) which is similar to ROAR-SRE - participants silently read sentences as quickly as possible and endorse as true of false - but it is administered on paper in a one-on-one setting; (2) Letter Word Identification (WJ-LWID) in which participants read words out loud and are scored for accuracy; (3) Word Attack (WJ-WA) in which participants read pseudowords out loud and are scored for accuracy (Schrank et al., 2014); (4) Test of Word Reading Efficiency Sight Word Efficiency (TOWRE-SWE) in which participants read lists of real words as quickly and accurately as possible; (5) Phonemic Decoding Efficiency (TOWRE-PDE) in which participants read lists of pseudowords as quickly and accurately as possible (Torgesen et al., 2011). Each student had also completed ROAR-SRE within 2 months prior as part of their regular school day without the presence of researchers.
Study 3: results
Construct validity of ROAR-SRE
We found a strong correlation between ROAR-SRE and WJ-SRF in both samples (r = 0.82, r = 0.91) (Figures 6AB). In addition, ROAR-SRE was moderately correlated with untimed, single word reading accuracy (WJ-LWID, r = 0.69), untimed pseudoword reading accuracy (WJ-WA, r = 0.59), real word list reading speed (TOWRE-SWE, r = 0.66), and pseudoword list reading speed (TOWRE-PDE, r = 0.57). This pattern of correlations supports the notion that sentence reading efficiency is a separable, yet highly related construct, to single word reading speed and accuracy.
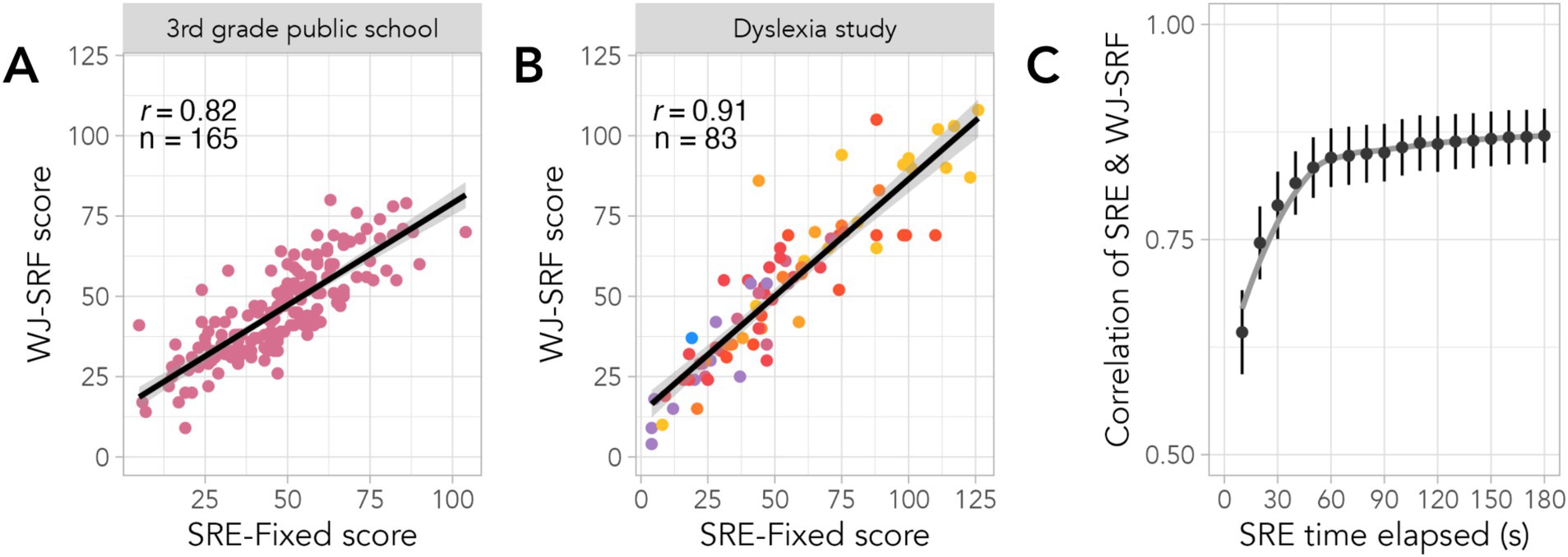
Figure 6. ROAR-SRE is highly correlated to standardized, individually-administered, in-person assessments of reading fluency. (A) 3rd grade public school sample demonstrating a strong correlation between ROAR-SRE completed unproctored in the classroom and WJ SRF which was individually administered to each student. (B) N = 83 participants from ongoing dyslexia studies within the lab (grades 2–8 or higher; colors indicate grades as Figure 5) were individually administered WJ and completed ROAR-SRE at home. (C) Correlation between ROAR-SRE and WJ-SRF as a function of assessment time.
What is the ideal length of a silent sentence reading efficiency assessment?
Many assessments of sentence reading fluency/efficiency are 3 min by convention but previous work has not systematically analyzed the relationship between assessment length and reliability. Study 3 employed a newer version of the ROAR-SRE web application that precisely logged timing information. This timing information was used to calculate each participants’ ROAR-SRE score at 10 s time intervals which was then correlated against the full 3 min WJ-SRF scores. The correlation between ROAR-SRE and TOSREC increased as a function of assessment length. However, the correspondence between the two measures hit a peak between 60 and 90 s (Figure 6C) indicating that the remaining assessment time did not further contribute to the reliability of the measure.
Study 3: discussion and limitations
Study 3 demonstrated that the unproctored, online ROAR-SRE assessment was highly correlated with a similar, standardized measure delivered one-on-one in person (WJ SRF). This provides strong evidence for the concurrent validity of an online measure. Moreover, the stronger correspondence between sentence reading (WJ-SRF) versus single word decoding (WJ-LWID and WJ-WA) and single word reading efficiency (TOWRE) measures demonstrated that sentence and word reading are related but dissociable constructs as highlighted in other work (Silverman et al., 2013). Finally, the analysis of assessment length demonstrated that even a quick 1 min SRE measure achieves high reliability. This finding opens the possibility of more regular progress monitoring with a quick and automated 1 min assessment. Moreover, given the correspondence between ROAR-SRE, TOSREC, WJ and TOWRE scores, there is reason to be optimistic that ROAR-SRE could also serve to predict end-of-year outcomes on measures of comprehension. However the predictive validity of ROAR-SRE remains an open question for future research. Moreover, Study 3 employed a limited set of outcome measures that leave open the questions of (a) how well ROAR-SRE predicts more comprehensive summative assessments and (b) how strong the relationship is between silent sentence reading efficiency and oral read fluency (ORF). ORF is widely used by schools for progress monitoring and benchmarking and, based on other studies, we expect a lot of similarity between oral and silent reading (Denton et al., 2011; Wagner, 2011; Price et al., 2016). However, a direct comparison between ROAR-SRE and various ORF measures is an important future direction in order to determine if (a) one measure is superior for a given application versus (b) both measures provide complementary information. Finally, Study 3 only examined one SRE-Fixed test form; for this measure to be useful in a school context parallel form reliability is critical. We tackle this question in Study 4.
Study 4: comparison of human-authored versus AI-authored items
Previous work (1) explored the potential of prompting a large language model (LLM) to generate new true and false sentences to enhance the item bank (White et al., 2022), and (2) created a “item response simulator” (based on fine-tuning a large language model) to calibrate these LLM-generated items and created parallel test forms for ROAR-SRE (Zelikman et al., 2023). This approach separates the item generation process (which can use a variety of models that need not be as large as the current state-of-the-art), and the item calibration process which uses a simulation to arrange items into matched test forms. The goals of Study 4 were to (a) assess the validity of these AI-generated test forms in a large and diverse sample and (b) determine the alternate form reliability for ROAR-SRE. The two AI-generated test forms used in Study 4 were the exact forms generated by the item response simulator in Zelikman et al. (2023). In brief, these test forms were created through a process of prompt engineering as well as training a neural network model to predict student response patterns to new, GPT-generated items.
Study 4: methods
ROAR assessments were administered to 1,110 students (grades 1–12), across 11 schools across three states through an RPP model (see Table S1 for school demographics). Each participant completed two separate three-minute long ROAR-SRE test forms: (1) SRE-Fixed from Study 2/3 and (2) one of two AI-generated parallel test forms from the student response simulator. The order of the test forms was randomized across participants. Data points were excluded from analysis based on the criteria for random guessing and disengagement established in Study 2.
Study 4: results
Scores on the three test forms were highly correlated (r = 0.88 for SRE-Fixed and AI-Form-A; r = 0.89 for SRE-Fixed and AI-Form-B; Figure 7 top panel) indicating that (a) AI generated parallel test forms from the student response simulator are well matched to human authored test forms, and (b) ROAR-SRE has exceptional parallel form reliability. We next computed parallel form reliability as a function of assessment time and found that a 60 s ROAR-SRE assessment was highly reliable (r = 0.79 for AI-Form-A; r = 0.80 for AI-Form-B; Figure 7 bottom panel) and that reliability only marginally increased after 60 s. The median difference between AI-Form A and SRE-Fixed was 3.5 and the median difference between AI-Form B and SRE-Fixed was 3.
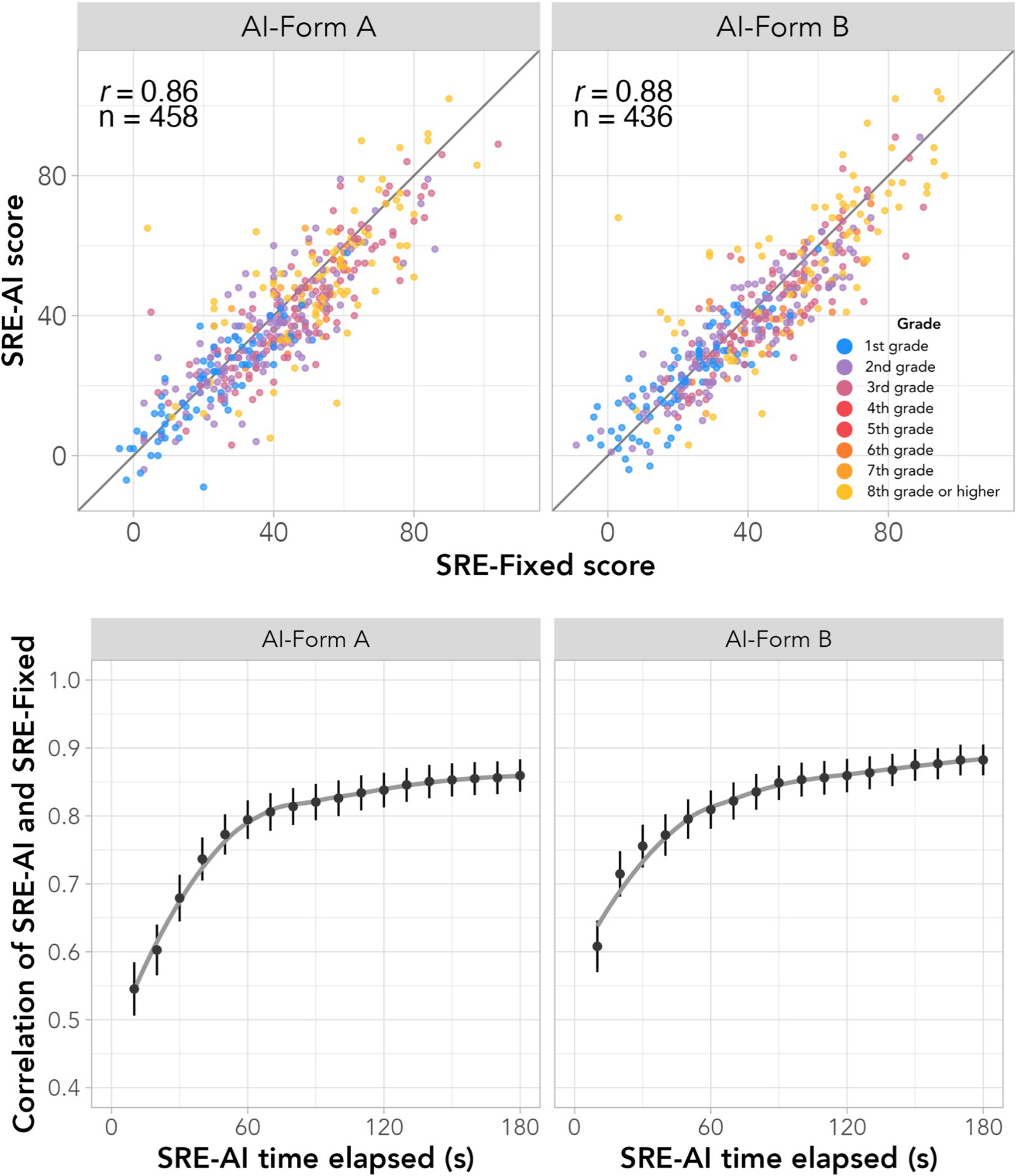
Figure 7. Parallel form reliability for ROAR-SRE. (Top panel) Correlation between scores on the human-authored SRE-Fixed form versus two AI-authored forms. (Bottom panel) parallel form reliability as a function of assessment length.
Study 4: discussion and limitations
Study 4 validated the technical advancements of previous work (Zelikman et al., 2023) in a real world setting. Specifically, we validated that an LLM could be trained to generate parallel test forms and that, in practice, these AI-generated forms are consistent with human-authored forms. However the correspondence between scores on AI-generated and human-authored test forms were not perfect suggesting that the Item Response Simulator from Zelikman et al. (2023) might need additional modifications for zero-shot parallel test form generation. Alternatively, post-hoc equating methods could be used to equate scores across forms (van der Linden, 2013; Kolen and Brennan, 2014).
The technical advance in automated form generation, coupled with the reliability and scalability of ROAR-SRE, open the possibility of more regular progress monitoring that is potentially integrated with other technology and products used in the classroom. However, even though it is theoretically possible to scale this approach to generate an infinite number of matched test forms, it is also important to proceed with caution and continue to document the edge cases where generative AI makes mistakes. For example, items still need to be examined by a human for suitability in a given context (Zelikman et al., 2023). Moreover, the variability in stimuli generated by the LLM has not been carefully examined and it is likely that additional work will need to be done to ensure that the distribution of AI-authored forms truly incorporates the wealth of human knowledge on assessment design. Thus, at each phase of development it is important to incorporate the voices of many stakeholders - from teachers to school administrators to students, researchers and technology developers.
General discussion
Literacy unlocks a new form of communication through written language. Skilled readers are largely able to use written language and spoken language interchangeably; from a neuroscience standpoint, the literate brain processes speech and text using much of the same circuitry (Preston et al., 2016; Deniz et al., 2019; Yeatman and White, 2021). However, achieving this level of literacy requires systematic instruction coupled with years of practice. The challenge for the young reader is to master foundational reading skills such that word recognition becomes effortless and automatic and text can be decoded with a level of fluency such that comprehension of written language and spoken language are equivalent (Yeatman, 2022). Even though the end goal of literacy is comprehension, the barrier for many children is mastering foundational skills: individual words must be decoded accurately and efficiently to achieve fluency at the word, sentence, and paragraph level. Particularly for children with dyslexia, mastering decoding and fluency is a considerable challenge (Wolf and Katzir-Cohen, 2001; Katzir et al., 2006; Peterson and Pennington, 2012; Reis et al., 2020). Even though comprehension is the end goal of reading instruction, reading fluency is the bottleneck for many children (particularly but not limited to those with dyslexia). Here we developed a new measure of silent sentence reading efficiency that was designed with minimal comprehension demands in order to provide more specific, diagnostic information on the development of reading fluency. We use the term “efficiency” to emphasize specific design decisions that were intended to make the measure tap more directly into the rate at which children are able to read as opposed to other measures of “reading fluency” which incorporate a variety of other constructs including prosody of oral reading, syntactic knowledge, vocabulary, background knowledge and inferencing skills. Through a series of validation studies, we showed a quick 1 min measure that is scored in real time is (a) highly reliable, (b) explains most of the variance in other measures of reading fluency, (c) can efficiently be deployed at scale, and (d) is amenable to automated item generation with AI.
A straightforward extension of this work would be to study ROAR-SRE as a progress monitoring tool within a multi-tiered system of support (Deno et al., 2001; Al Otaiba and Fuchs, 2002; Fletcher et al., 2006; Miciak and Fletcher, 2020). Since reliable scores can be obtained in a minute, and parallel forms can be generated with AI, weekly or even daily ROAR-SRE probes should be possible to assess growth curves under different intervention approaches. Equating test forms remains a challenge for many other assessments [e.g., ORF (Francis et al., 2008)] and another strength of the SRE construct is that the items are short and simple sentences which are straightforward to design and provide ample flexibility for equating. The SRE construct is mainly designed to assess speed or efficiency of word reading (with a check on understanding); establishing sufficient speed can be a major barrier for children with dyslexia (Catts et al., 2024). Thus, we see ROAR-SRE as being particularly useful within the context of monitoring a student’s response to intervention (RTI) in a multi-component, evidence-based dyslexia intervention program. In combination with measures of decoding (Yeatman et al., 2021; Ma et al., 2023), and phonological awareness (Gijbels et al., 2023), SRE will be useful in more accurately pinpointing the root of a student’s reading difficulties and adjusting instruction accordingly.
Another strength of ROAR-SRE is that it spans a broad age range and is a reliable measure from elementary through high-school. Thus, it might also hold utility as a quick screener for dyslexia. In most districts it is not currently standard of practice to screen for dyslexia or decoding issues more broadly after 2nd or 3rd grade and dyslexia screening legislation usually focuses on kindergarten through second grade. However many students continue to struggle and their struggles either go unnoticed or are misattributed to poor comprehension since reading comprehension is the most common assessment target above 3rd grade. Though comprehension is, of course, a specific struggle for many students (Nation et al., 2010; Foorman et al., 2018; Spencer and Wagner, 2018), there is a growing spotlight on decoding problems being the bottle-neck for others (Wang et al., 2019). For example, the most recent National Assessment of Educational Progress (NAEP) found that measures of ORF and pseudoword reading were correlated with performance on the NAEP (White et al., 2021). This opens the possibility that some children who perform poorly on the NAEP (and state reading assessments) have yet to establish foundational decoding skills and, for this subset of students, poor decoding might be conflated with poor comprehension unless additional assessments are used to dissociate these skills. Fortunately, for students with decoding challenges there is a robust science of reading laying out how to teach decoding across the grades (Castles et al., 2018; Lovett et al., 2021).
An open question is how SRE should fit into the broader landscape of reading assessments. For example, many schools rely on ORF to benchmark reading development (Fuchs et al., 2001; Domingue et al., 2022). A more nuanced comparison of (a) the constructs measured by ORF versus SRE and (b) the psychometric properties of each measure will be important for determining the most efficacious use of this new measure. Since achieving fluent reading is the main barrier for children with dyslexia as they progress through schooling, SRE might be useful for screening and assessing intervention efficacy. However, the design decisions that went into SRE could also be a limitation for certain applications. For example, if the goal is a quick screener to predict end-of-the-year, high stakes assessments, then minimizing demands on vocabulary, syntax and background knowledge might be a weakness. With the goal of prediction, the best performing screener is usually the one that is most similar to the outcome. Thus, in this use case, SRE should be combined with other measures that specifically target vocabulary, morphology, syntax and inferencing skills.
Reading development is often conceptualized as a sequence or hierarchy of interrelated skills with phonological awareness and letter sound knowledge forming the foundation upon which single word decoding and then sentence reading are built (Hudson et al., 2008; Castles et al., 2018). Under the simple view of reading, comprehension is the interaction between skills in decoding and oral language (Hoover and Gough, 1990). Under this framework, SRE can be viewed as a high-level decoding skill that bridges between basic decoding knowledge and the automaticity that is required to fluidly map written language to spoken language (Pikulski and Chard, 2005; Kim et al., 2014). This framework predicts a causal relationship whereby the development of more basic skills like phonological awareness and single word reading predict the development of higher level skills like SRE which, in turn, directly influences comprehension. However this hypothesis will need to be tested with longitudinal data.
In summary, we developed a new measure indexing the speed at which students can read sentences for comprehension. We presented an argument for the face validity of this measure along with a sequence of validation studies establishing reliability and construct validity in a laboratory and school setting, for students spanning grades 1–12. We believe that the construct of sentence reading efficiency is more interpretable than other, related measures, and will provide a useful bridge between basic reading skills and higher-level indices of reading comprehension.
Data availability statement
The datasets presented in this article are not readily available due to data sharing agreements with partnering school districts that have strict stipulations on data re-use and sharing. Requests to access the datasets should be directed to anllYXRtYW5Ac3RhbmZvcmQuZWR1.
Ethics statement
The studies involving humans were approved by Stanford University Institutional Review Board. The studies were conducted in accordance with the local legislation and institutional requirements. The ethics committee/institutional review board waived the requirement of written informed consent for participation from the participants or the participants’ legal guardians/next of kin.
Author contributions
JY: Conceptualization, Data curation, Formal analysis, Funding acquisition, Investigation, Methodology, Project administration, Resources, Software, Supervision, Validation, Visualization, Writing – original draft, Writing – review & editing. JT: Data curation, Formal analysis, Investigation, Methodology, Visualization, Writing – original draft, Writing – review & editing. AB: Investigation, Methodology, Writing – original draft, Writing – review & editing. WM: Formal analysis, Investigation, Methodology, Resources, Writing – original draft, Writing – review & editing. JM: Methodology, Software, Writing – original draft, Writing – review & editing. MY: Methodology, Writing – original draft, Writing – review & editing. LG: Data curation, Investigation, Methodology, Writing – original draft, Writing – review & editing. CT-F: Investigation, Methodology, Project administration, Writing – original draft, Writing – review & editing. AR-H: Investigation, Methodology, Project administration, Resources, Software, Writing – original draft, Writing – review & editing.
Funding
The author(s) declare that financial support was received for the research, authorship, and/or publication of this article. This work was funded by NICHD R01HD095861 Advanced Educational Research and Development Fund, Stanford-Sequoia K-12 Research Collaborative, Stanford Impact Labs, and Neuroscience: Translate grants to J.D.Y.
Acknowledgments
We would like to thank the school districts, families and students that made this research possible through a research practice partnership model. We would also like to thank Nick Haber and Eric Zelikman for technical support in applying previous work to create AI-generated parallel test forms, Tonya Murray, Albu Ungashe and other research coordinators for support of research practice partnerships, and Joshua Lawrence and Rebecca Silverman for helpful feedback and discussion of the manuscript.
Conflict of interest
BA was employed by Cambium Assessment Inc.
The remaining authors declare that the research was conducted in the absence of any commercial or financial relationships that could be construed as a potential conflict of interest.
The reviewer RH declared a shared affiliation with the author LG to the handling editor at the time of review.
Publisher’s note
All claims expressed in this article are solely those of the authors and do not necessarily represent those of their affiliated organizations, or those of the publisher, the editors and the reviewers. Any product that may be evaluated in this article, or claim that may be made by its manufacturer, is not guaranteed or endorsed by the publisher.
References
Al Otaiba, S., and Fuchs, D. (2002). Characteristics of children who are unresponsive to early literacy intervention: a review of the literature. Remedial Spec. Educ. 23, 300–316. doi: 10.1177/07419325020230050501
Burkhardt, A., Yablonski, M., Mitchell, J., Gijbels, L., and Yeatman, J.D. (2023). “Developing items for a silent reading efficiency task.” in National Council on Measurement In Education (NCME) Conference, Chicago, IL, United States.
Castles, A., Rastle, K., and Nation, K. (2018). Ending the reading wars: reading acquisition from novice to expert. Psychol. Sci. Public Interest 19, 5–51. doi: 10.1177/1529100618772271
Catts, H. W., and Hogan, T. P. (2020). Dyslexia: an ounce of prevention is better than a pound of diagnosis and treatment. PsyArXiv. doi: 10.31234/osf.io/nvgje
Catts, H. W., Terry, N. P., Lonigan, C. J., Compton, D. L., Wagner, R. K., Steacy, L. M., et al. (2024). Revisiting the definition of dyslexia. Ann. Dyslexia. 74, 282–302. doi: 10.1007/s11881-023-00295-3
Cummings, K. D., Park, Y., and Bauer Schaper, H. A. (2013). Form effects on DIBELS next oral reading fluency progress- monitoring passages. Assess. Eff. Interv. 38, 91–104. doi: 10.1177/1534508412447010
Deniz, F., Nunez-Elizalde, A. O., Huth, A. G., and Gallant, J. L. (2019). The representation of semantic information across human cerebral cortex during listening versus reading is invariant to stimulus modality. J. Neurosci. 39, 7722–7736. doi: 10.1523/JNEUROSCI.0675-19.2019
Deno, S. L., Fuchs, L. S., Marston, D., and Shin, J. (2001). Using curriculum-based measurement to establish growth standards for students with learning disabilities. Sch. Psych. Rev. 30, 507–524. doi: 10.1080/02796015.2001.12086131
Denton, C. A., Barth, A. E., Fletcher, J. M., Wexler, J., Vaughn, S., Cirino, P. T., et al. (2011). The relations among oral and silent reading fluency and comprehension in middle school: implications for identification and instruction of students with reading difficulties. Sci. Stud. Read. 15, 109–135. doi: 10.1080/10888431003623546
Domingue, B. W., Dell, M., Lang, D., Silverman, R., Yeatman, J., and Hough, H. (2022). The effect of COVID on oral reading fluency during the 2020–2021 academic year. AERA Open 8:23328584221120254. doi: 10.1177/23328584221120254
Domingue, B. W., Hough, H. J., Lang, D., and Yeatman, J. (2021). Changing patterns of growth in oral reading fluency during the COVID-19 pandemic. Working Paper. Policy Analysis for California Education, PACE. Available at: https://eric.ed.gov/?id=ED612595 (Accessed September 9, 2024).
Fletcher, J. M., Francis, D. J., Foorman, B. R., and Schatschneider, C. (2021). Early detection of dyslexia risk: development of brief, teacher-administered screens. Learn. Disabil. Q. 44, 145–157. doi: 10.1177/0731948720931870
Fletcher, J. M., Lyon, G. R., Fuchs, L. S., and Barnes, M. A. (2006). Learning disabilities: From identification to intervention. New York: Guilford PRess.
Foorman, B. R., Petscher, Y., and Herrera, S. (2018). Unique and common effects of decoding and language factors in predicting reading comprehension in grades 1–10. Learn. Individ. Differ. 63, 12–23. doi: 10.1016/j.lindif.2018.02.011
Francis, D. J., Santi, K. L., Barr, C., Fletcher, J. M., Varisco, A., and Foorman, B. R. (2008). Form effects on the estimation of students’ oral reading fluency using DIBELS. J. Sch. Psychol. 46, 315–342. doi: 10.1016/j.jsp.2007.06.003
Fuchs, L. S., Fuchs, D., Hosp, M. K., and Jenkins, J. R. (2001). Oral reading fluency as an Indicator of reading competence: a theoretical, empirical, and historical analysis. Sci. Stud. Read. 5, 239–256. doi: 10.1207/S1532799XSSR0503_3
Gijbels, L., Burkhardt, A., Ma, W. A., and Yeatman, J. D. (2023). Rapid online assessment of reading and phonological awareness (ROAR-PA).
Good, R. H., Gruba, J., and Kaminski, R. A. (2002). “Best practices in using dynamic indicators of basic early literacy skills (DIBELS) in an outcomes-driven model,” in Best practices in school psychology IV, Vols, ed. A. Thomas (Washington, DC, US: National Association of School Psychologists, xv), 1–2.
Hartigan, J. A., and Hartigan, P. M. (1985). The dip test of Unimodality. Ann. Stat. 13, 70–84. doi: 10.1214/aos/1176346577
Hoffman, A. R., Jenkins, J. E., and Dunlap, S. K. (2009). Using DIBELS: a survey of purposes and practices. Read. Psychol. 30, 1–16. doi: 10.1080/02702710802274820
Hoover, W. A., and Gough, P. B. (1990). The simple view of reading. Read. Writ. 2, 127–160. doi: 10.1007/BF00401799
Hudson, R. F., Pullen, P. C., Lane, H. B., and Torgesen, J. K. (2008). The complex nature of reading fluency: a multidimensional view. Read. Writ. Q. 25, 4–32. doi: 10.1080/10573560802491208
Johnson, E. S., Pool, J. L., and Carter, D. R. (2011). Validity evidence for the test of silent reading efficiency and comprehension (TOSREC). Assess. Eff. Interv. 37, 50–57. doi: 10.1177/1534508411395556
Jones, C. (2022). Why California is among last states not screening children for dyslexia. EdSource. Available at: https://edsource.org/2022/why-is-california-one-of-the-last-states-to-not-screen-children-for-dyslexia/682543 (Accessed August 8, 2023).
Kang, E. Y., and Shin, M. (2019). The contributions of reading fluency and decoding to reading comprehension for struggling readers in fourth grade. Read. Writ. Q. 35, 179–192. doi: 10.1080/10573569.2018.1521758
Katzir, T., Kim, Y., Wolf, M., O’Brien, B., Kennedy, B., Lovett, M., et al. (2006). Reading fluency: the whole is more than the parts. Ann. Dyslexia 56, 51–82. doi: 10.1007/s11881-006-0003-5
Kim, Y.-S. G., Park, C. H., and Wagner, R. K. (2014). Is oral/text reading fluency a “bridge” to reading comprehension? Read. Writ. 27, 79–99. doi: 10.1007/s11145-013-9434-7
Kim, Y.-S., Wagner, R. K., and Lopez, D. (2012). Developmental relations between reading fluency and reading comprehension: a longitudinal study from grade 1 to grade 2. J. Exp. Child Psychol. 113, 93–111. doi: 10.1016/j.jecp.2012.03.002
Kolen, M. J., and Brennan, R. L. (2014). Test equating, scaling, and linking: Methods and practices. New York, NY: Springer.
Lovett, M. W., Frijters, J. C., Steinbach, K. A., Sevcik, R. A., and Morris, R. D. (2021). Effective intervention for adolescents with reading disabilities: combining reading and motivational remediation to improve outcomes. J. Educ. Psychol. 113, 656–689. doi: 10.1037/edu0000639
Lyon, G. R., Shaywitz, S. E., and Shaywitz, B. A. (2003). A definition of dyslexia. Ann. Dyslexia 53, 1–14. doi: 10.1007/s11881-003-0001-9
Ma, W. A., Richie-Halford, A., Burkhardt, A., Kanopka, K., Chou, C., Domingue, B., et al. (2023). ROAR-CAT: rapid online assessment of reading ability with computerized adaptive testing.
Miciak, J., and Fletcher, J. M. (2020). The critical role of instructional response for identifying dyslexia and other learning disabilities. J. Learn. Disabil. 53, 343–353. doi: 10.1177/0022219420906801
Nation, K., Cocksey, J., Taylor, J. S. H., and Bishop, D. V. M. (2010). A longitudinal investigation of early reading and language skills in children with poor reading comprehension. J. Child Psychol. Psychiatry 51, 1031–1039. doi: 10.1111/j.1469-7610.2010.02254.x
Odegard, T. N., Farris, E. A., Middleton, A. E., Oslund, E., and Rimrodt-Frierson, S. (2020). Characteristics of students identified with dyslexia within the context of state legislation. J. Learn. Disabil. 53, 366–379. doi: 10.1177/0022219420914551
Peirce, J., Gray, J. R., Simpson, S., MacAskill, M., Höchenberger, R., Sogo, H., et al. (2019). PsychoPy2: experiments in behavior made easy. Behav. Res. Methods 51, 195–203. doi: 10.3758/s13428-018-01193-y
Peterson, R. L., and Pennington, B. F. (2012). Developmental dyslexia. Lancet 379, 1997–2007. doi: 10.1016/S0140-6736(12)60198-6
Pikulski, J. J., and Chard, D. J. (2005). Fluency: bridge between decoding and reading comprehension. Read. Teach. 58, 510–519. doi: 10.1598/RT.58.6.2
Preston, J. L., Molfese, P. J., Frost, S. J., Mencl, W. E., Fulbright, R. K., Hoeft, F., et al. (2016). Print-speech convergence predicts future reading outcomes in early readers. Psychol. Sci. 27, 75–84. doi: 10.1177/0956797615611921
Price, K. W., Meisinger, E. B., Louwerse, M. M., and D’Mello, S. (2016). The contributions of Oral and silent reading fluency to reading comprehension. Read. Psychol. 37, 167–201. doi: 10.1080/02702711.2015.1025118
Reis, A., Araújo, S., Morais, I. S., and Faísca, L. (2020). Reading and reading-related skills in adults with dyslexia from different orthographic systems: a review and meta-analysis. Ann. Dyslexia 70, 339–368. doi: 10.1007/s11881-020-00205-x
Rice, M., and Gilson, C. B. (2023). Dyslexia identification: tackling current issues in schools. Interv. Sch. Clin. 58, 205–209. doi: 10.1177/10534512221081278
Samuels, S. J. (2007). The DIBELS tests: is speed of barking at print what we mean by reading fluency? Read. Res. Q. 42, 563–566.
Schrank, F. A., McGrew, K. S., Mather, N., Wendling, B. J., and LaForte, E. M. (2014). Woodcock-Johnson IV tests of achievement. Rolling Meadows, IL: Riverside Publishing.
Silverman, R. D., Speece, D. L., Harring, J. R., and Ritchey, K. D. (2013). Fluency has a role in the simple view of reading. Sci. Stud. Read. 17, 108–133. doi: 10.1080/10888438.2011.618153
Spencer, M., and Wagner, R. K. (2018). The comprehension problems of children with poor reading comprehension despite adequate decoding: a Meta-analysis. Rev. Educ. Res. 88, 366–400. doi: 10.3102/0034654317749187
Torgesen, J. K., Wagner, R., and Rashotte, C. (2011). TOWRE 2: Test of word reading efficiency : Pearson Clinical Assessment.
van der Linden, W. J. (2013). Some conceptual issues in observed-score equating. J. Educ. Meas. 50, 249–285. doi: 10.1111/jedm.12014
Wagner, R. K. (2011). Relations among Oral reading fluency, silent reading fluency, and reading comprehension: a latent variable study of first-grade readers. Sci. Stud. Read. 15, 338–362. doi: 10.1080/10888438.2010.493964
Wagner, R. K., Torgesen, J. K., Rashotte, C. A., and Pearson, N. A. (2010). Test of silent reading efficiency and comprehension. Pro Ed.
Wang, Z., Sabatini, J., O’Reilly, T., and Weeks, J. (2019). Decoding and reading comprehension: a test of the decoding threshold hypothesis. J. Educ. Psychol. 111, 387–401. doi: 10.1037/edu0000302
Ward-Lonergan, J. M., and Duthie, J. K. (2018). The state of dyslexia: recent legislation and guidelines for serving school-age children and adolescents with dyslexia. Lang. Speech Hear. Serv. Sch. 49, 810–816. doi: 10.1044/2018_LSHSS-DYSLC-18-0002
White, J., Burkhardt, A., Yeatman, J., and Goodman, N. (2022). Automated generation of sentence reading fluency test items. Proceedings of the Annual Meeting of the Cognitive Science Society 44. Available at: https://escholarship.org/uc/item/3804p0ff (Accessed June 20, 2022).
White, S., Sabatini, J., Park, B. J., Chen, J., Bernstein, J., and Li, M. (2021). The 2018 NAEP Oral reading fluency study. NCES 2021–025. National Center for Education Statistics. Available at: https://files.eric.ed.gov/fulltext/ED612204.pdf (Accessed September 9, 2024).
Wolf, M., and Katzir-Cohen, T. (2001). Reading fluency and its intervention. Sci. Stud. Read. 5, 211–239. doi: 10.1207/S1532799XSSR0503_2
Yeatman, J. D. (2022). The neurobiology of literacy. In M. J. Snowling and C. Hulme, K. Nation (Eds.), The science of reading: A handbook (2nd ed., pp. 533–555). Wiley Blackwell. doi: 10.1002/9781119705116.ch24
Yeatman, J. D., Tang, K. A., Donnelly, P. M., Yablonski, M., Ramamurthy, M., Karipidis, I. I., et al. (2021). Rapid online assessment of reading ability. Sci. Rep. 11:6396. doi: 10.1038/s41598-021-85907-x
Yeatman, J. D., and White, A. L. (2021). Reading: the confluence of vision and language. Annu. Rev. Vis. Sci. 7, 487–517. doi: 10.1146/annurev-vision-093019-113509
Zelikman, E., Ma, W. A., Tran, J. E., Yang, D., Yeatman, J. D., and Haber, N. (2023). “Generating and evaluating tests for K-12 students with language model simulations: a case study on sentence reading efficiency.” in Proceedings of the 2023 Conference on Empirical Methods in Natural Language Processing, eds. H. Bouamor, J. Pino, and K. Bali (Association for Computational Linguistics). pp. 2190–2205.
Keywords: dyslexia, reading fluency assessment, screening tools, reading fluency and comprehension, progress monitoring, psychometrics
Citation: Yeatman JD, Tran JE, Burkhardt AK, Ma WA, Mitchell JL, Yablonski M, Gijbels L, Townley-Flores C and Richie-Halford A (2024) Development and validation of a rapid and precise online sentence reading efficiency assessment. Front. Educ. 9:1494431. doi: 10.3389/feduc.2024.1494431
Edited by:
Maria Cutumisu, McGill University, CanadaReviewed by:
Morgan Les DeBusk-Lane, Gallup, United StatesRoxanne Hudson, University of Washington, United States
Copyright © 2024 Yeatman, Tran, Burkhardt, Ma, Mitchell, Yablonski, Gijbels, Townley-Flores and Richie-Halford. This is an open-access article distributed under the terms of the Creative Commons Attribution License (CC BY). The use, distribution or reproduction in other forums is permitted, provided the original author(s) and the copyright owner(s) are credited and that the original publication in this journal is cited, in accordance with accepted academic practice. No use, distribution or reproduction is permitted which does not comply with these terms.
*Correspondence: Jason D. Yeatman, anllYXRtYW5Ac3RhbmZvcmQuZWR1
†These authors have contributed equally to this work