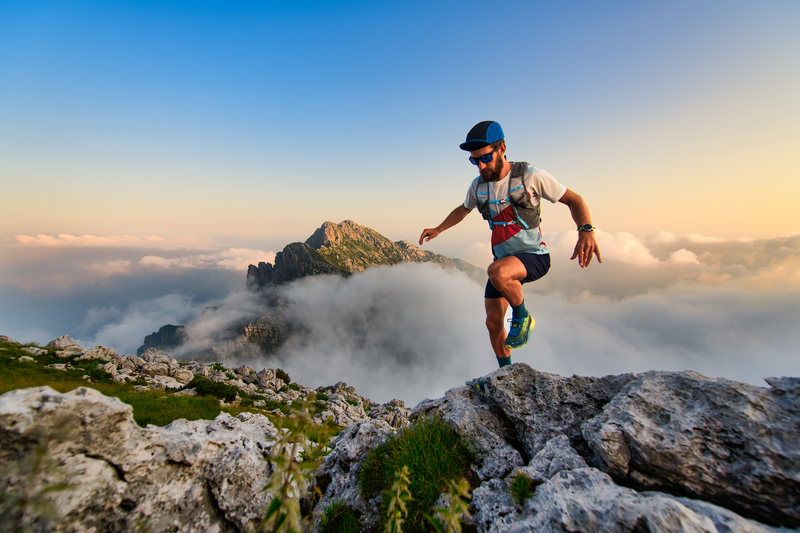
95% of researchers rate our articles as excellent or good
Learn more about the work of our research integrity team to safeguard the quality of each article we publish.
Find out more
ORIGINAL RESEARCH article
Front. Educ. , 03 October 2024
Sec. Digital Education
Volume 9 - 2024 | https://doi.org/10.3389/feduc.2024.1483853
This article is part of the Research Topic Cognitive Benefits of Technologies Applied to Learning in Education View all 19 articles
Introduction: Generative Artificial Intelligence (Gen AI) is rapidly transforming education holds immense potential for enhancing learning experiences and fostering innovation skills crucial for success in today’s rapidly changing job market. However, successful integration depends on student adoption. This study investigates factors influencing business students’ intention to use Gen AI in Innovation courses, focusing on the role of Perceived Enjoyment.
Method: A cross-sectional predictive analysis was conducted using data from 92 business undergraduate students in a Peruvian higher education institution. A survey questionnaire, adapted from Teo and Noyes, was used to measure perceived enjoyment, usefulness, ease of use, attitude toward, and intention to use Gen AI tools.
Results: The study found a strong positive relationship between Perceived Enjoyment and the intention to use Gen AI in Innovation courses. Furthermore, Perceived Enjoyment was positively associated with perceived ease of use. Interestingly, perceived usefulness did not show a significant effect on the intention to use Gen AI.
Conclusion: Our finding challenges the traditional emphasis on perceived usefulness as the primary driver of technology acceptance. Instead, our results suggest that prioritizing user enjoyment and ease of use in the design and implementation of Gen AI tools may be a more effective strategy for promoting their adoption in educational settings. This shift in focus from utility to experience could be crucial in unlocking the full potential of Gen AI to transform education.
Rapid technological advancements are reshaping the educational landscape, challenging educators to prepare students for an increasingly innovative and adaptable job market (Ortíz and Fumás, 2020). Traditional teaching methods often struggle to cultivate the critical and soft skills demanded by modern employers (Prince and Felder, 2006; Succi and Canovi, 2019). In response, educators are turning to emerging technologies, particularly Generative Artificial Intelligence (Gen AI), to enhance learning experiences and foster innovation skills (Børte et al., 2023).
Gen AI has demonstrated significant potential in education, improving learning outcomes, enhancing productivity, providing personalized instruction, and increasing student engagement (Gilson et al., 2023). It also supports collaborative learning and constructivist pedagogies (Prentzas, 2013). However, the successful integration of Gen AI in education depends on students’ willingness to adopt and use these tools effectively.
The Technology Acceptance Model (TAM), proposed by Davis (1987), focuses on perceptions of usefulness and ease of use, as essential variables for understanding the adoption of new technologies in educational settings, particularly in the case of innovative tools such as Gen AI. Unlike Rogers’ Diffusion of Innovations Theory (Rogers, 1962), which examines adoption at the macro level, TAM allows to get more detail about how individual factors, such as perceived enjoyment, influence students’ intention to use these technologies. Although technology acceptance models like UTAUT and UTAUT2 incorporate additional constructs such as price value and habits (Venkatesh et al., 2012), they do not delve into perceived enjoyment as a key driver of innovative technology use. Given that Gen AI is characterized by its accessibility, where its price is not a determining factor, TAM emerges as a robust theoretical framework and as an effective methodology for understanding the technology adoption dynamics in academic settings, enabling to get more accurate assessments of the impact of perceived enjoyment on the use of these innovations, allowing to foster creativity skills and critical thinking among students.
ChatGPT represents a significant advance in artificial intelligence, where one of its main features is the ability to convincingly emulate human conversations. By learning from its past mistakes, ChatGPT can rapidly adapt to new interaction contexts and deliver more realistic and effective exchanges (Deng and Lin, 2023; Farrokhnia et al., 2023). In the field of education, AI has demonstrated its potential to improve educational quality, teaching-learning processes and career opportunities (Hamal et al., 2022). Generative AI tools such as ChatGPT-4, Gemini, and Copilot have become essential resources for educators in tasks like writing and learning, as well as academic assessment, lesson planning, personalized content creation or differentiation and adaptive instruction (Popenici and Kerr, 2017; Kasneci et al., 2023). Hence, these tools significantly reduce educators’ workload, allowing them to focus more on interactive and adaptive pedagogy (Kiryakova and Angelova, 2023).
Similarly, students can benefit from Gen AI tools in several activities, including creative writing, essay writing, prompt generation, scheduling, and question answering (Taecharungroj, 2023). This interactions may significantly improve student engagement, by fostering peer-to-peer collaboration, and broadening access to educational resources, contributing to a more inclusive and dynamic educational experience (Strzelecki, 2023; Cotton et al., 2023).
Therefore, our study investigates the impact of Perceived Enjoyment on business undergraduate students’ intention to use Gen AI in Innovation courses. By exploring this relationship, we aim to bridge the gap between technological advancements and effective pedagogical practices, contributing to the growing body of knowledge on technology adoption in higher education (Lai, 2017).
Over the past decade, higher education has undergone significant transformations driven by technological advancements and digitalization (Fülöp et al., 2022). Artificial Intelligence (AI), augmented reality, and gamification have enhanced student-technology interactions (Fülöp et al., 2022; Prentzas, 2013), with educators playing a crucial role in facilitating this integration (Teo, 2011). Universities now offer a range of digital teaching tools to meet the demands of digitally proficient students (Fülöp et al., 2022; Lewis et al., 2024).
Generative AI (Gen AI), a recent advancement in this field, has shown promise in enhancing reading, writing, learning, and academic evaluation processes through its ability to emulate human conversations (Bozkurt, 2023). It has also improved student engagement, peer collaboration, and access to educational resources (Strzelecki, 2023; Cotton et al., 2023).
In recent years, Gen AI has gained significant popularity due to its remarkable advances in various fields. However, some readers may not be fully familiar with its underlying components. Gen AI encompasses several branches, including Large Language Models (LLMs), such as ChatGPT and Gemini, which are known for their unique capabilities to simplify many daily tasks for people of diverse backgrounds and social statuses (Agathokleous et al., 2023).
Another key branch is Generative Antagonistic Networks (GANs), mainly used in image generation, such as DALL-E. In addition, the transformer architecture, fundamental to many GenAI models, enables a wide range of applications beyond text generation.
However, Gen AI faces challenges such as limitations in deep understanding, quality evaluation of responses, and risks of biases and discrimination (Farrokhnia et al., 2023). These issues have prompted educators to adapt their teaching methods, fostering critical and creative approaches to integrate Gen AI in education in an effective and ethical way (Barros et al., 2023).
Innovation encompasses three core aspects: creativity, knowledge, and operationalization capacity (Tura et al., 2008; Saunila and Ukko, 2012). These dimensions rely heavily on human capacity, which Lawson and Samson (2001) define as the ability to transform ideas into beneficial products, processes, and systems. Assink (2006) further emphasizes that disruptive innovation involves exploring radical ideas and leveraging resources to create marketable innovations.
In educational settings, (Gen AI) has emerged as a powerful tool to develop innovation skills. By supporting teaching activities, Gen AI stimulates student interest and enhances critical thinking and creativity (Kiryakova and Angelova, 2023), expands problem-solution spaces, facilitating greater knowledge generation and connections, ultimately leading to superior innovation performance (Bouschery et al., 2023).
The Technology Acceptance Model (TAM) (Davis et al., 1989) provides a framework for understanding the adoption of such innovative tools. TAM posits that perceived usefulness (PU) and perceived ease of use (PEU) are key determinants of technology acceptance. Teo and Noyes (2011) extended TAM by incorporating perceived enjoyment (PE) as an external variable, finding that it significantly influences PU, PEU, and intention to use technology.
Recent studies suggest that PE may have an even more significant effect on intention to use than PU (Lee et al., 2019). This highlights the importance of considering enjoyment alongside traditional TAM variables when examining the adoption of innovative technologies in educational settings.
By fostering the adoption of tools like Gen AI through consideration of factors such as perceived enjoyment, educators can enhance students’ innovation skills, aligning with the core dimensions of innovation and preparing students for the demands of an increasingly technology-driven workplace (Barros et al., 2023; Rogers et al., 2014).
Perceived enjoyment, defined as the extent to which using a technology is inherently pleasurable (Davis et al., 1992), has emerged as a crucial factor in educational technology adoption. This construct extends beyond traditional Technology Acceptance Model (TAM) variables, often rivaling or exceeding the impact of perceived usefulness and ease of use (Teo and Noyes, 2011; Venkatesh et al., 2012).
Across various educational technologies, including augmented reality (AR), virtual reality (VR), MOOCs, and mobile learning, perceived enjoyment consistently influences adoption intentions and usage behavior (Tao et al., 2019; Ghobadi et al., 2022). For instance, in AR and VR contexts, enjoyment not only predicts usage intentions but also positively affects academic performance (Lee et al., 2013; Ghobadi et al., 2022). Similarly, in mobile learning and Web 2.0 technologies, perceived enjoyment significantly impacts adoption intentions and future teaching practices (Mubuke et al., 2017; Teo et al., 2018).
Recent studies on AI-powered educational tools like ChatGPT further emphasize the role of perceived enjoyment. Xu and Thien (2024) found that enjoyment mediates various factors influencing ChatGPT adoption for language learning. However, Rahman et al. (2023) noted that trust moderates the relationship between perceived enjoyment and attitudes towards ChatGPT use, highlighting the complex interplay of factors in AI technology adoption.
The influence of perceived enjoyment extends to emerging educational technologies such as the metaverse (Al-Adwan et al., 2023) and broader applications like fintech and branded sports apps (Winarno et al., 2021; Won et al., 2023). This widespread impact underscores the need for developers and educators to prioritize enjoyable user experiences in their technological designs.
Importantly, the role of perceived enjoyment in technology adoption may vary across cultures and contexts (Sánchez-Prieto et al., 2017). For instance, in some collectivist cultures, social influence might moderate the effect of perceived enjoyment on adoption intentions (Tarhini et al., 2017). Additionally, the relationship between perceived enjoyment and other factors like self-efficacy and facilitating conditions can significantly impact the overall acceptance of educational technologies (Abdullah and Ward, 2016).
As educational technologies continue to evolve, understanding the nuanced role of perceived enjoyment becomes increasingly crucial. Future research should explore how perceived enjoyment interacts with other factors in emerging technologies, potentially leading to more comprehensive models of technology acceptance in educational settings (Scherer et al., 2019).
Generative Artificial Intelligence (GenAI) offers significant opportunities in education, although its adoption has several challenges, as its limited ability to assess the quality of responses, introducing biases that threaten the integrity of educational content (Farrokhnia et al., 2023). Also, the production of incorrect or fabricated responses affects their reliability (Baidoo-Anu and Ansah, 2023). Therefore, these issues require educators to adapt their methodologies to tackle these limitations (Barros et al., 2023).
Risks associated with privacy and ethical implications are another concern among students and teachers. The handling of sensitive data and automated profiling present ethical challenges that could affect trust and acceptance of these technologies in the educational setting (Chan and Hu, 2023).
Regarding academic integrity, the use of GenAI poses the risk of enabling plagiarism and diminishing the development of critical thinking (Michel-Villarreal et al., 2023). Although teachers acknowledge the benefits, their non-supervised and indiscriminate use will cultivate technological dependence, emphasizing the need of clear educational policies for their responsible use (Chan and Lee, 2023).
Rather than banning their use, various institutions suggest strengthening critical and ethical digital literacy to ensure appropriate and equitable use of technology (Chan and Lee, 2023; Saúde et al., 2024; Singh et al., 2024). This is relevant to avoid disparities in academic achievement, especially among students with less access to advanced technologies (Singh et al., 2024). The adoption of GenAI also requires that educational programs get aligned with technological trends like Industry 4.0 to develop critical digital skills necessary for an increasingly digitized labor market (Abulibdeh et al., 2024).
Perceived enjoyment acts as a catalyst in this process, enabling faster adoption of GenAI even among students with limited digital skills. A rapid adoption not only stimulates the development of professional skills, but also contributes to reduce the gap in learning outcomes. By integrating enjoyment as a key variable in pedagogical design, an effective redesign of teaching-learning strategies can be promoted, preparing students to face the challenges of the modern work environment.
Therefore, the integration of enjoyment as a central variable in pedagogical design is critical to preparing students for the challenges of the modern work environment. While addressing the risks and limitations of GenAI, a focus on perceived enjoyment may be key to successful and beneficial adoption in the educational setting.
The evidence presented suggests that there are operational relationships between perceived enjoyment, perceived usefulness, perceived ease of use, attitude toward use and intention to use. The hypotheses under consideration are illustrated in Figure 1.
H1: Perceived Enjoyment (PE) has a direct and significant effect on Perceived Usefulness (PU) among the business students.
H2: Perceived Enjoyment (PE) has a direct and significant effect on Perceived Ease of Use (PEU) among the business students.
H3: Perceived Enjoyment (PE) has a direct and significant effect on Intention to Use (ITU) among the business students.
H4: Perceived Ease of Use (PEU) has a direct and significant effect on Attitude Toward Use (ATU) among the business students.
H5: Perceived Usefulness (PU) has a direct and significant effect on Attitude Toward Use (ATU) among the business students.
H6: Attitude Toward Use (ATU) acts as a mediator between Perceived Ease of Use (PEU) and Perceived Usefulness (PU) and Intention to use (ITU) among the business students.
Our study aims to explore the relationships between perceived enjoyment, perceived usefulness, perceived ease of use, attitude toward use and intention to use ChatGPT as a learning tool.
This study utilizes a cross-sectional predictive analysis, where data were gathered at a specific point in time to measure key variables and explore potential correlations and trends. Participants were selected using convenience sampling (Etikan et al., 2016), a non-probabilistic sampling method. Given that this study was conducted as part of an educational intervention within an Innovation course, convenience sampling was deemed the most suitable methodological approach. The dependent variable used was intention to use, while the independent variables were perceived enjoyment, perceived ease of use, and perceived usefulness, following the model proposed by Teo and Noyes (2011). Accordingly, a structural equation modeling approach (PLS-SEM) was employed, where these variables represent constructs related to technology adoption.
The study was developed in 2023 within the framework of a course called “Business Innovation”, where the use of Design Thinking was taught for 4 weeks, and ChatGPT was used as a support tool for the training of students. The work developed with Gen AI was carried out in groups, totaling 20 teams composed of 4 to 5 students per group.
The students were informed about the objectives of Challenge-Based Learning (CBL), focused on the Sustainable Development Goals (SDGs). The pedagogical process followed the five stages of Design Thinking: problem definition, insight discovery, ideation and solution generation, solution decision matrix, prototyping and testing, applying AI in each of these stages. After the end of the project, each student answered a survey, where the questions were based on the items raised by Teo and Noyes (2011).
92 students from a Peruvian higher education college participated in the study: 39 were male (42%) and 53 were female (58%); their ages ranged from 18 to 52 years (mean = 29.18, SD = 6.54). The students were selected through non-probabilistic sampling. Ethical procedures were followed by the institution, then the participants were briefed on the study and informed of their rights of participation.
Data were obtained through an anonymous online survey using the Google Forms platform between July and September 2023. Participants completed the questionnaire during their free time, outside class hours. The institution followed the appropriate ethical procedures, and participants were informed about the study and their participation rights.
The survey was administered to students from a higher education institution located in Lima, Peru. The students participated in the Business Innovation course, where an educational intervention was carried out using ChatGPT for the formulation of innovative business projects, framed in the 17 Sustainable Development Goals (SDGs), in groups of 4 to 5 students.
A survey questionnaire was devised by comprising items that were adapted from a scale developed by Teo and Noyes (2011). This scale comprises 11 Likert-type items with five possible responses: 1 = extremely dissatisfied, 2 = generally dissatisfied, 3 = undecided, 4 = generally satisfied, and 5 = extremely satisfied. The instrument was translated into Spanish according to established guidelines for the translation and cross-cultural validation of instruments (Tsang et al., 2017). The scale showed good internal consistency in this study, ω = 0.92.
The psychometric properties of the latent constructs were evaluated using exploratory factor analysis (EFA) (Table 1). The results demonstrate satisfactory levels of reliability and validity for all latent variables, as evidenced by the Cronbach’s α values exceeding 0.767 and composite reliability (CR) surpassing 0.732 (Hair et al., 2014). Furthermore, the convergent validity of the reflective models was assessed, revealing factor loadings greater than 0.700 and average variance extracted (AVE) values above 0.625, indicating an adequate fit (Hair et al., 2014). These findings support the suitability of the variables for subsequent analyses.
To test the proposed hypotheses, we used partial least squares structural equation modeling (PLS-SEM) as shown in Hair et al. (2014), Richter et al. (2016) and Shiau et al. (2019). PLS-SEM is a powerful approach that allows for the simultaneous modeling of the relationships between both measured variables (the measurement model) and latent variables (the structural model). This method was particularly appropriate for this study because it enables a comprehensive analysis of the intricate relationships between the variables of interest.
To assess the structural model’s relevance and predictive power, the multiple correlation coefficient (R2 = 0.517) and Stone-Geisser’s predictive relevance test (Q2 = 0.127) were used and show good fit measures (Chin and Todd, 1995; Chin, 2009). Also, the standardized root mean square residual coefficient (SRMR = 0.052) has an appropriate adjustment level (Hu and Bentler, 1998). It is possible to confirm the significance of the model, since the measures of the model’s fit show appropriate levels and predictive capacity (R2 = 0.517; Q2 = 0.127; SRMR = 0.052; Hair et al., 2017). Table 2 summarizes PLS-SEM results.
Our findings indicate that perceived enjoyment influences the perceived usefulness of Gen AI among the students, therefore H1 is supported. H2 is accepted as students’ perceived enjoyment by using Gen AI on innovation has a direct effect on perceived ease of use. High levels of perceived enjoyment increase the intention to use Gen AI, supporting H3. Also, a higher level of perceived ease of use increases students’ attitude to use, supporting H4. Regarding H5, students’ perceived usefulness of Gen AI exhibits non-significant effects on attitude to use, hence H5 is rejected in the model. Students’ attitude to use has positive effects on job satisfaction even though the direct effect is small. Therefore, results support H6. Table 3 summarizes the hypotheses testing results.
This study was designed to explore the relationships between perceived enjoyment, perceived usefulness, perceived ease of use, attitude toward use, and intention to use ChatGPT as a learning tool among business students. Our findings elucidate the factors that influence the adoption of Gen AI in higher education.
Our results confirm that perceived enjoyment plays a crucial role in the adoption of technology (Yu et al., 2017; Song and Han, 2009), significantly affecting perceived usefulness, perceived ease of use, and the intention to use Gen AI among business students. This aligns with Teo and Noyes (2011), who highlighted the importance of perceived enjoyment in technology adoption. When students find the use of Gen AI tools enjoyable, they are more likely to perceive these tools as useful and easy to use, thereby enhancing their intention to adopt such technologies in their learning processes.
Interestingly, contrary to the traditional assumptions of the Technology Acceptance Model (TAM) as proposed by Davis et al. (1989), our study indicates that perceived usefulness does not significantly affect attitude toward use. This suggests that within the context of Gen AI adoption among business students, perceived usefulness may not be the primary driver of their attitudes toward its use (Lee et al., 2019). Instead, enjoyment and ease of use experienced while interacting with Gen AI tools seem to play a more influential role. This finding prompts a reevaluation of the components of TAM, particularly in the educational technology context, and suggests that user enjoyment could be a more significant determinant of technology adoption than previously understood.
However, the use of Gen AI can have negative implications in terms of educational justice (Singh et al., 2024), particularly in environments where access to information technologies is limited. For example, in emerging countries like Peru, Internet access does not have the same speed in different regions, and there are cities where Internet coverage is limited. Therefore, it is possible to identify factors other than technology that can deepen knowledge gaps. Additionally, although most of the Gen IA tools offer free access, they also have premium (paid) versions with more advanced functionalities (number of queries, quality of responses, type of content generated, etc.), limiting this premium access to individuals or organizations that do not have the resources to pay for the access. It is therefore essential to study in subsequent studies whether the introduction of Gen IA could end up extending educational gaps.
The practical implications of our findings are two-fold. Educators should prioritize enhancing the perceived enjoyment of Gen AI tools to boost adoption rates. This can be achieved by integrating engaging and interactive elements into these technologies, such as gamification and interactive simulations, which can create a more enjoyable learning experience. Additionally, software and technology developers should focus on creating user-friendly interfaces and personalized learning experiences that meet individual preferences to further improve ease of use and enjoyment.
Our study has some limitations, where the cross-sectional design limits our ability to establish causality, and the sample from a single institution may affect the generalizability of the findings. Future research should employ longitudinal designs to understand the evolution of these relationships over time and include a more diverse demographic to enhance the external validity of the results. Moreover, exploring the moderating effects of individual differences and specific educational outcomes on the relationship between perceived usefulness and attitude toward use could provide deeper insights.
Despite these limitations, our study adds valuable insights to the TAM literature by challenging the conventional role of perceived usefulness and highlighting the importance of perceived enjoyment in the adoption of new technologies. Future studies should investigate the dimensionality of perceived enjoyment and its effects across different technologies, user populations, and cultural contexts to better understand its role in the broader framework of technology acceptance.
By highlighting the importance of perceived enjoyment, our study offers a novel perspective on Gen AI adoption among business students. As educators and developers work together to integrate Gen AI into higher education, prioritizing enjoyment and ease of use will be crucial for the future of teaching. This will not only foster widespread acceptance but also maximize the educational benefits of these innovative technologies.
In summary, our findings highlight that perceived enjoyment is a key factor in the adoption of Gen AI tools, while perceived usefulness does not directly influence the attitude towards their use. Ease of use, mediated by enjoyment, plays a fundamental role in adoption. This suggests that, for the successful integration of Gen AI in the classroom, educational experiences centered on enjoyment should be prioritized. The main findings are summarized below:
1. Perceived Enjoyment (PE) significantly influences students’ intention to use Gen AI tools in Innovation.
2. Perceived Usefulness (PU) did not show a significant effect on the attitude towards using Gen AI, challenging traditional technology acceptance models.
3. Perceived Ease of Use (PEU), mediated by perceived enjoyment, positively influences students’ attitude towards using these tools.
4. A pedagogical approach that prioritizes user experience and enjoyment may be key to promoting the effective adoption of Gen AI in educational settings and reducing gaps in academic performance.
5. The practical implications for educators and developers are to create intuitive, enjoyment-centered technological tools to maximize their adoption in teaching, ensuring an equitable and sustainable educational impact.
The datasets presented in this article are not readily available because they are proprietary data. Requests to access the datasets should be directed to bm51bmV6bUBwdWNwLmVkdS5wZQ==.
The studies involving humans were approved by the Direccion de Investigacion ISIL. The studies were conducted in accordance with the local legislation and institutional requirements. The participants provided their written informed consent to participate in this study.
JRC: Conceptualization, Investigation, Project administration, Resources, Visualization, Writing – original draft, Writing – review and editing. NN: Data curation, Formal analysis, Funding acquisition, Methodology, Resources, Software, Supervision, Validation, Writing – original draft, Writing – review and editing.
The author(s) declare that no financial support was received for the research, authorship, and/or publication of this article.
The authors declare that the research was conducted in the absence of any commercial or financial relationships that could be construed as a potential conflict of interest.
All claims expressed in this article are solely those of the authors and do not necessarily represent those of their affiliated organizations, or those of the publisher, the editors and the reviewers. Any product that may be evaluated in this article, or claim that may be made by its manufacturer, is not guaranteed or endorsed by the publisher.
Abdullah, F., and Ward, R. (2016). Developing a general extended technology acceptance model for E-learning (GETAMEL) by analysing commonly used external factors. Comput. Hum. Behav. 56, 238–256.
Abulibdeh, A., Zaidan, E., and Abulibdeh, R. (2024). Navigating the confluence of artificial intelligence and education for sustainable development in the era of industry 4.0: Challenges, opportunities, and ethical dimensions. J. Clean. Prod. 437, 140527–140527.
Agathokleous, E., Saitanis, C. J., Fang, C., and Yu, Z. (2023). Use of ChatGPT: What does it mean for biology and environmental science? Sci. Total Environ. 888, 164154–164154. doi: 10.1016/j.scitotenv.2023.164154
Al-Adwan, A., Li, N., Al-Adwan, A., Abbasi, G., Albelbisi, N., and Habibi, A. (2023). “Extending the technology acceptance model (TAM) to predict university students’ intentions to use metaverse-based learning platforms. Educ. Inform. Technol. 28, 15381–15413. doi: 10.1007/s10639-023-11816-3
Assink, M. (2006). Inhibitors of disruptive innovation capability: A conceptual model. Eur. J. Innov. Manag. 9, 215–233.
Baidoo-Anu, D., and Ansah, O. (2023). Education in the era of generative artificial intelligence (AI): Understanding the potential benefits of ChatGPT in promoting teaching and learning. J. AI, 7, 52–62.
Barros, A., Prasad, A., and Martyna, Ś. (2023). Generative artificial intelligence and academia: Implication for research, teaching and service. Manag. Learn. 54:1445. doi: 10.1080/15548627.2020.1797280
Børte, K., Nesje, K., and Lillejord, S. (2023). Barriers to student active learning in higher education. Teach. High. Educ. 28, 597–615.
Bouschery, S. G., Blazevic, V., and Piller, F. T. (2023). Augmenting human innovation teams with artificial intelligence: Exploring transformer-based language models. J. Prod. Innov. Manag. 40, 139–153.
Bozkurt, A. (2023). Generative artificial intelligence (AI) powered conversational educational agents: The inevitable paradigm shift. Asian J. Distance Educ. 18, 198–204.
Chan, C. K. Y., and Hu, W. (2023). Students’ voices on generative AI: Perceptions, benefits, and challenges in higher education. Int. J. Educ. Technol. High. Educ. 20:43.
Chan, C. K. Y., and Lee, K. K. W. (2023). The AI generation gap: Are Gen Z students more interested in adopting generative AI such as ChatGPT in teaching and learning than their Gen X and millennial generation teachers? Smart Learn. Environ. 10:60.
Chin, W. W. (2009). “Bootstrap cross-validation indices for PLS path model assessment,” in Handbook of partial least squares: Concepts, methods and applications. Berlin: Springer Berlin Heidelberg, 83–97.
Chin, W. W., and Todd, P. A. (1995). On the use, usefulness, and ease of use of structural equation modeling in MIS research: A note of caution. MIS Q. 19, 237–246.
Cotton, D. R. E., Cotton, P. A., and Shipway, J. R. (2023). Chatting and cheating: Ensuring academic integrity in the era of ChatGPT. Innov. Educ. Teach. Int. 61, 228–239.
Davis, F. D. (1987). User acceptance of information systems: The technology acceptance model (TAM). Available online at: https://umich.edu/
Davis, F. D., Bagozzi, R. P., and Warshaw, P. R. (1989). Technology acceptance model. J. Manag. Sci. 35, 982–1003.
Davis, F. D., Bagozzi, R. P., and Warshaw, P. R. (1992). Extrinsic and intrinsic motivation to use computers in the workplace1. J. Appl. Soc. Psychol. 22, 1111–1132.
Deng, J., and Lin, Y. (2023). The benefits and challenges of ChatGPT: An overview. Front. Comput. Intell. Syst. 2:81–83. doi: 10.54097/fcis.v2i2.4465
Etikan, I., Musa, S. A., and Alkassim, R. S. (2016). Comparison of convenience sampling and purposive sampling. Am. J. Theoretical Appl. Stat. 5, 1–4.
Farrokhnia, M., Banihashem, S. K., Noroozi, O., and Wals, A. (2023). A SWOT analysis of ChatGPT: Implications for educational practice and research. Innov. Educ. Teach. Int. 61, 460–474.
Fülöp, M., Breaz, T., He, X., Ionescu, C., Cordoş, G., and Stanescu, S. (2022). The role of universities’ sustainability, teachers’ wellbeing, and attitudes toward e-learning during COVID-19. Front. Public Health 10:981593. doi: 10.3389/fpubh.2022.981593
Ghobadi, M., Shirowzhan, S., Ghiai, M. M., Mohammad Ebrahimzadeh, F., and Tahmasebinia, F. (2022). Augmented reality applications in education and examining key factors affecting the users’ behaviors. Educ. Sci. 13:10.
Gilson, A., Safranek, C. W., Huang, T., Socrates, V., Chi, L., Taylor, A., et al. (2023). How does ChatGPT perform on the United States medical licensing examination? The implications of large language models for medical education and knowledge assessment. JMIR Med. Educ. 9:e45312.
Hair, J. F., Babin, B. J., and Krey, N. (2017). Covariance-based structural equation modeling in the journal of advertising: Review and recommendations. J. Adv. 46:454.
Hair, J. F., Sarstedt, M., Ringle, C. M., Smith, D., and Reams, R. (2014). Partial least squares structural equation modeling (PLS-SEM): A useful tool for family business researchers. J. Fam. Bus. Strategy 5, 105–115. doi: 10.1016/j.jfbs.2014.01.002
Hamal, O., El Faddouli, N.-E., Harouni, M. H. A., and Lu, J. (2022). Artificial Intelligent in Education. Sustainability 14:2862.
Hu, L. T., and Bentler, P. M. (1998). Fit indices in covariance structure modeling: Sensitivity to underparameterized model misspecification. Psychol. Methods 3:424.
Kasneci, E., Seßler, K., Küchemann, S., Bannert, M., Dementieva, D., Fischer, F., et al. (2023). ChatGPT for good? On opportunities and challenges of large language models for education. Learn. Individ. Differ. 103:102274. doi: 10.1097/ACM.0000000000005636
Kiryakova, G., and Angelova, N. (2023). ChatGPT–A challenging tool for the university professors in their teaching practice. Educ. Sci. 13, 1056–1056.
Lai, P. C. (2017). The literature review of technology adoption models and theories for the novelty technology. J. Inform. Syst. Technol. Manag. 14, 21–38.
Lawson, B., and Samson, D. (2001). DEVELOPING INNOVATION CAPABILITY IN ORGANISATIONS: A DYNAMIC CAPABILITIES APPROACH. Int. J. Innov. Manag. 05, 377–400.
Lee, H.-G., Chung, S., and Lee, W.-H. (2013). Presence in virtual golf simulators: The effects of presence on perceived enjoyment, perceived value, and behavioral intention. New Media Soc. 15, 930–946.
Lee, J., Kim, J., and Choi, J. Y. (2019). The adoption of virtual reality devices: The technology acceptance model integrating enjoyment, social interaction, and strength of the social ties. Telemat. Inform. 39, 37–48.
Lewis, C. C., Fretwell, C. E., Ryan, J., and Parham, J. B. (2024). Faculty use of established and emerging technologies in higher education: A unified theory of acceptance and use of technology perspective. Int. J. High. Educ. 2, 22–34.
Michel-Villarreal, R., Vilalta-Perdomo, E., Salinas-Navarro, D., Thierry-Aguilera, R., and Gerardou, F. (2023). Challenges and opportunities of generative ai for higher education as explained by ChatGPT. Educ. Sci. 13:856.
Mubuke, F., Ogenmungu, C., Masaba, A. K., and Andrew, W. (2017). The predictability of perceived enjoyment and its impact on the intention to use mobile learning systems. Asian J. Comput. Sci. Inform. Technol. 7, 1–5.
Ortíz, J., and Fumás, V. (2020). Technological innovation and the demand for labor by firms in expansion and recession. Econ. Innov. New Technol. 29, 417–440.
Popenici, S. A. D., and Kerr, S. (2017). Exploring the impact of artificial intelligence on teaching and learning in higher education. RPTEL 12:22.
Prentzas, J. (2013). “Artificial intelligence methods in early childhood education,” in Artificial intelligence, evolutionary computing and metaheuristics. studies in computational intelligence, Vol. 427, ed. X. S. Yang (Berlin: Springer).
Prince, M. J., and Felder, R. M. (2006). Inductive teaching and learning methods: Definitions, comparisons, and research bases. J. Eng. Educ. 95, 123–138.
Rahman, M. S., Sabbir, M. M., Zhang, D. J., Moral, I. H., and Hossain, G. M. S. (2023). Examining students’ intention to use ChatGPT: Does trust matter? Australas. J. Educ. Technol. 39, 51–71. doi: 10.14742/ajet.8956
Richter, N. F., Cepeda-Carrión, G., Roldán Salgueiro, J. L., and Ringle, C. M. (2016). European management research using partial least squares structural equation modeling (PLS-SEM). Eur. Manag. J. 34, 589–597.
Rogers, E. M., Singhal, A., and Quinlan, M. M. (2014). Diffusion of innovations: An integrated approach to communication theory and research. Milton Park: Routledge, 432–448.
Sánchez-Prieto, J. C., Olmos-Migueláñez, S., and García-Peñalvo, F. J. (2017). MLearning and pre-service teachers: An assessment of the behavioral intention using an expanded TAM model. Comput. Hum. Behav. 72, 644–654.
Saúde, S., Barros, J., and Almeida, I. (2024). Impacts of generative artificial intelligence in higher education: Research trends and students’ perceptions. Soc. Sci. 13:410.
Saunila, M., and Ukko, J. (2012). A conceptual framework for the measurement of innovation capability and its effects. Baltic J. Manag. 7, 355–375.
Scherer, R., Siddiq, F., and Tondeur, J. (2019). The technology acceptance model (TAM): A meta-analytic structural equation modeling approach to explaining teachers’ adoption of digital technology in education. Comput. Educ. 128, 13–35.
Shiau, W. L., Sarstedt, M., and Hair, J. F. (2019). Internet research using partial least squares structural equation modeling (PLS-SEM). Internet Res. 29, 398–406.
Singh, E., Vasishta, P., and Singla, A. (2024). AI-enhanced education: Exploring the impact of AI literacy on generation Z’s academic performance in Northern India. Q. Assur. Educ. [Epub ahead of print].
Song, Y., and Han, J. (2009). “Is enjoyment important? An empirical research on the impact of perceive enjoyment on adoption of new technology,” in Proceedings of the 2009 international conference on information management, innovation management and industrial engineering, Vol. 4, (Xi’an: IEEE), 511–514.
Strzelecki, A. (2023). Students’ acceptance of ChatGPT in higher education: An extended unified theory of acceptance and use of technology. Innov. High. Educ. 49, 223–245.
Succi, C., and Canovi, M. (2019). Soft skills to enhance graduate employability: Comparing students and employers’ perceptions. Stud. High. Educ. 45, 1834–1847.
Taecharungroj, V. (2023). What can ChatGPT Do?” Analyzing early reactions to the innovative AI chatbot on twitter. Big Data Cogn. Comput. 7:35.
Tao, D., Fu, P., Wang, Y., Zhang, T., and Qu, X. (2019). Key characteristics in designing massive open online courses (MOOCs) for user acceptance: An application of the extended technology acceptance model. Interact. Learn. Environ. 30, 882–895.
Tarhini, A., Hone, K., Liu, X., and Tarhini, T. (2017). Examining the moderating effect of individual-level cultural values on users’ acceptance of E-learning in developing countries: A structural equation modeling of an extended technology acceptance model. Interact. Learn. Environ. 25, 306–328.
Teo, T. (2011). Factors influencing teachers’ intention to use technology: Model development and test. Comput. Educ. Comput. Educ. 57, 2432–2440.
Teo, T., and Noyes, J. (2011). An assessment of the influence of perceived enjoyment and attitude on the intention to use technology among pre-service teachers: A structural equation modeling approach. Comput. Educ. 57, 1645–1653.
Teo, T., Sang, G., Mei, B., and Hoi, C. K. W. (2018). Investigating pre-service teachers’ acceptance of Web 2.0 technologies in their future teaching: A Chinese perspective. Interact. Learn. Environ. 27, 530–546.
Tsang, S., Royse, C. F., and Terkawi, A. S. (2017). Guidelines for developing, translating, and validating a questionnaire in perioperative and pain medicine. Saudi J. Anaesthes. 11(Suppl. 1), S80–9.
Tura, T., Vesa Harmaakorpi, and Sanna Pekkola. (2008). Breaking inside the black box: Towards a dynamic evaluation framework for regional innovative capability. Sci. Public Policy Sci. Public Policy 35, 733–744.
Venkatesh, V., Thong, J. Y., and Xu, X. (2012). Consumer acceptance and use of information technology: Extending the unified theory of acceptance and use of technology. MIS Q. 36, 157–178.
Winarno, W. A., Mas’ud, I., and Palupi, T. W. (2021). Perceived enjoyment, application self-efficacy, and subjective norms as determinants of behavior intention in Using OVO applications. J. Asian Finance Econ. Bus. 8, 1189–1200.
Won, D., Chiu, W., and Byun, H. (2023). Factors influencing consumer use of a sport-branded app: The technology acceptance model integrating app quality and perceived enjoyment. Asia Pac. J. Market. Logist. 35, 1112–1133.
Xu, X., and Thien, L. M. (2024). Unleashing the power of perceived enjoyment: Exploring Chinese undergraduate EFL learners’ intention to use ChatGPT for English learning. J. Appl. Res. High. Educ. [Epub ahead of print].
Keywords: generative artificial intelligence, higher education, technology adoption, perceived enjoyment, innovation education
Citation: Cano JR and Nunez NA (2024) Unlocking innovation: how enjoyment drives GenAI use in higher education. Front. Educ. 9:1483853. doi: 10.3389/feduc.2024.1483853
Received: 20 August 2024; Accepted: 11 September 2024;
Published: 03 October 2024.
Edited by:
Pedro Román-Graván, University of Seville, SpainReviewed by:
Divya Baranwal, Southern Methodist University, United StatesCopyright © 2024 Cano and Nunez. This is an open-access article distributed under the terms of the Creative Commons Attribution License (CC BY). The use, distribution or reproduction in other forums is permitted, provided the original author(s) and the copyright owner(s) are credited and that the original publication in this journal is cited, in accordance with accepted academic practice. No use, distribution or reproduction is permitted which does not comply with these terms.
*Correspondence: Nicolas A. Nunez, bm51bmV6bUBwdWNwLmVkdS5wZQ==
Disclaimer: All claims expressed in this article are solely those of the authors and do not necessarily represent those of their affiliated organizations, or those of the publisher, the editors and the reviewers. Any product that may be evaluated in this article or claim that may be made by its manufacturer is not guaranteed or endorsed by the publisher.
Research integrity at Frontiers
Learn more about the work of our research integrity team to safeguard the quality of each article we publish.