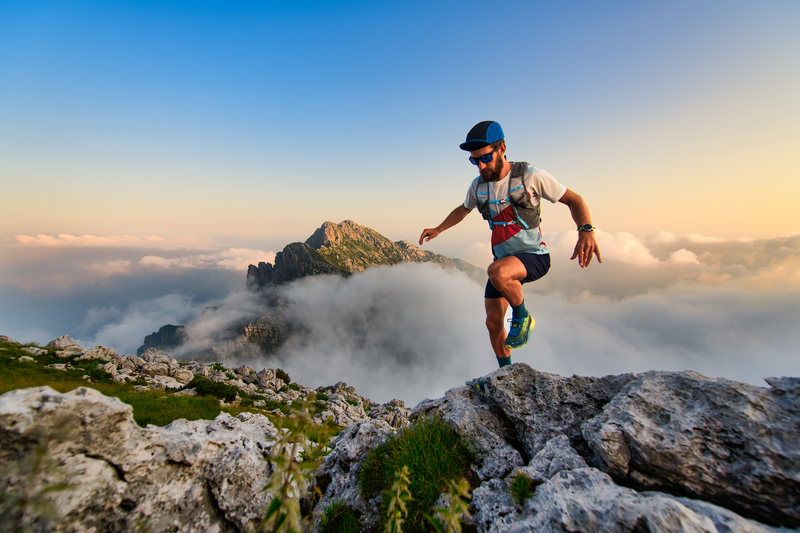
95% of researchers rate our articles as excellent or good
Learn more about the work of our research integrity team to safeguard the quality of each article we publish.
Find out more
ORIGINAL RESEARCH article
Front. Educ. , 22 November 2024
Sec. STEM Education
Volume 9 - 2024 | https://doi.org/10.3389/feduc.2024.1474572
This article is part of the Research Topic Building Tomorrow’s Biomedical Workforce: Evaluation of How Evidence-Based Training Programs Align Skill Development and Career Awareness with a Broad Array of Professions View all 17 articles
The career paths of PhD scientists often deviate from their doctoral theses. As a result, the need to integrate student-centered career and professional development training is important to meet the needs of doctoral students. Qualifying exams (QEs) represent a significant milestone in progression toward graduation within most PhD Programs in the United States. These exams are commonly administered 2–3 years into a PhD program following the completion of coursework, with the primary objective of evaluating whether the candidate possesses the necessary knowledge and skills to progress with their dissertation research. To enhance the value of QEs and intentionally align them with the diverse career trajectories of our students, we explored the inclusion of student-centered assessments in a track with a Pharmaceutical Sciences PhD program. In this PhD program, one component of QEs is a series of monthly, written cumulative exams focused on recent scientific literature in the faculty and students’ discipline. To create a student-centered QE, the student and a faculty member collaborated to develop personalized assessments focused on career exploration and in alignment with individual student’s career goals. All students enrolled in the PhD track (n = 8) were invited to participate in a survey about their experience with the redesigned QE. A combination of Likert scale and short answer questions were collected; quantitative items were analyzed with descriptive statistics and qualitative items with thematic coding. A subset of survey participants (n = 5) participated in a focus group regarding their experience with both the Traditional Model QE and the redesigned Pilot Model QE. Two faculty interviews were conducted regarding the design, content, procedures, and evaluation of student QEs. The study design and analysis were grounded in the cognitive apprenticeship framework, with a focus on how the QEs were situated within the four domains of this framework: content, methods, sequencing, and sociology. Results revealed that this student-centered QE approach was perceived to be more aligned with student career aspirations and to have a high interest level and value for students without placing a substantial additional burden on participants. This suggests that it is a feasible mechanism for integrating student-centered assessment into QEs.
The structure of most doctoral programs in the United States consists of one to two years of foundational and elective coursework, qualifying exams (QE)s, and additional years of independent research culminating in a dissertation (Goldman and Massy, 2000; Hartnett and Katz, 1977; National Academies of Sciences, Engineering, and Medicine, 2018; Walker et al., 2008). While the design of these elements can vary across discipline, program, and institution, they are generally present. There are a variety of terms used to refer to QEs across disciplines, programs and institutions including cumulative, qualifying, comprehensive, candidacy, preliminary, and general exam (McLaughlin et al., 2023); throughout this paper, these exams will be referred to as QEs. While the formats of QEs can vary, they typically occur midway through a PhD program and function as a gateway to attain candidacy status (McLaughlin et al., 2023), underscoring their role as pivotal assessments in doctoral studies.
In contrast to many professional degree programs, individual PhD programs are not accredited by a formal organization resulting in a lack of established standardized competencies, outcomes, and expectations across programs. As such, extant literature suggests that PhD programs vary widely in how they organize, assess, schedule, and support students during QEs. Methods for developing and administering QEs seem to stem from historic precedence, previous norms, and occasional modification resulting from internal/external review processes (McLaughlin et al., 2023). In many cases, each PhD program develops their own competency and outcome goals, though standards have been suggested by the National Academies (National Academies of Sciences, Engineering, and Medicine, 2018). There is little empirical evidence supporting specific QE practices, beyond ensuring students understand the purpose and format of QEs and receive support during their administration (McLaughlin et al., 2023).
Given the high stakes nature of QEs, it is crucial that they are well-designed and closely aligned with the core competencies defined by PhD programs. Factors such as economic pressures and social and cultural trends can impact evolving expectations of student skill development and what is expected of them upon completing an educational degree program (Gerritsen-van Leeuwenkamp et al., 2017) Such objectives require an integration of knowledge, skills, and attitudes, as well as the application of these so-called competencies in different authentic situations (Baartman et al., 2006; Baartman et al., 2007; Van Merriënboer and Kirschner, 2007). Since the nature of assessment can influence how students learn and how teachers teach (Watkins et al., 2005), researchers have argued for the importance of alignment between learning and assessment (Biggs, 1996; Cohen, 1987).
In their report on graduate education, the overarching competencies for STEM PhD training identified by the National Academies of Sciences, Engineering, and Medicine (2018) included: (1) “Develop Scientific and Technological Literacy and Conduct Original Research” and (2) “Develop Leadership, Communication, and Professional Competencies.” Although this work has attempted to define PhD training competencies, it remains unclear whether PhD programs have adopted these standards and, critically, there is scant evidence of doctoral students being assessed based on competencies relevant to their career objectives particularly with QEs.
Professional competencies are specific to career goals and therefore should be tailored to meet the individual needs of students based on their career aspirations (McLaughlin et al., 2019; Ramadoss et al., 2022; Olsen et al., 2020), however the primary assessments in PhD training tend to be discipline- and thesis-project specific. High stakes exams often serve a solely evaluative purpose, which poses a challenge when they are heavily emphasized in the curriculum, limiting opportunities for formative assessment and feedback. Curriculum design should prioritize the inclusion of formative assessments and feedback (Morris et al., 2021) enabling students to enhance their learning through active engagement with and application of feedback (French et al. (2024).
Despite ongoing initiatives at universities across the United States, career and professional development programming persists as a “hidden curriculum” resulting in disparities between the core training mechanisms and the career aspirations and outcomes of many PhD students (Elliot et al., 2020). Hidden curriculum is defined as “unwritten, unspoken, and often unintended lessons, values, and norms that students learn in educational settings through the structure, culture, and interactions of the institution rather than through formal instruction” (Griffith and Smith, 2020). This discrepancy arises because PhD training typically adheres to an apprentice model where most training occurs in the laboratory of investigators conducting research driven largely by grant funding. Moreover, foundational coursework and QEs are influenced by faculty preferences and focus on specialized knowledge, theories, and methodologies within the discipline. Also, dissertation committees are primarily composed of subject matter experts who evaluate the student’s scientific progression. While discipline-specific coursework, faculty-designed assessments, and technical skills are crucial aspects of PhD education, this concentrated approach frequently neglects vital career and professional competencies. Furthermore, it often fails to make implicit knowledge explicit, creating barriers for students who are unaware of or lack access to the hidden curriculum. These omissions can lead to discrepancies in professional development and affect career readiness.
PhD training has historically been considered an apprenticeship in which students serve as an learner and the research advisor the mentor. Expanding on this approach, we consider doctoral training through the cognitive apprenticeship framework. The cognitive apprenticeship framework aims to make the implicit cognitive processes of experts, like STEM faculty, more transparent to students (Minshew et al., 2021). This increased visibility provides students with the opportunity to observe and practice these processes. This framework offers guidance to faculty regarding how to effectively and explicitly share their expertise through the development of learning opportunities that promote and encourage student proficiency in a specific discipline (Minshew et al., 2021; Collins et al., 1989). It highlights the apprenticeship aspects of socialization while also focusing on the cognitive skills essential for advanced problem-solving tasks prevalent in STEM fields. This approach balances the importance of both socialization and cognitive development in addressing complex challenges.
There are four domains within the cognitive apprenticeship framework: content, methods, sequencing, and sociology. The content domain includes the knowledge and information relevant to a specific domain or discipline that apprentices must learn and become proficient in. This domain includes the following categories: domain knowledge, heuristic strategies, learning strategies, and control strategies. The method domain encompasses the methods, tactics, and approaches employed by professionals to resolve issues, make decisions, and achieve objectives within the domain. This domain includes the following categories: exploration, scaffolding, coaching, reflection, articulation, and modeling. The sequencing domain refers to the arrangement and sequence through which learning tasks and experiences are structured to support the progressive acquisition and proficiency of knowledge and skill. This domain includes the following categories: increasing complexity, increasing diversity, and global to local skills. The sociology domain centers on the interpersonal dimensions of learning in apprenticeships, encompassing how learners engage with peers, collaborate, and integrate into the community of practice to cultivate expertise. This domain includes the following categories: situated learning, communities of practice, cooperation, and collaboration (Collins et al., 1989; Minshew et al., 2021). Deliberately situating PhD training and assessment within the cognitive apprenticeship framework could serve as a mechanism for increased transparency, clarity of expectations, explicit sharing of expertise, and purposefully embedding relevant and meaningful opportunities for authentic practice to promote student proficiency.
Uncertainty regarding expectations, exam structure, and value of QEs can increase stress and anxiety, leading to a potential negative impact on student performance and inequity in the process (Harding-DeKam et al., 2012; Nerad and Cerny, 1999). This warrants careful consideration, particularly within the context of diversity and inclusivity (McLaughlin et al., 2023), as QEs are a critical hurdle for advancing to the dissertation stage and have been noted as a possible point of attrition in the STEM pipeline (Wilson et al., 2018). There is clear evidence that incorporating methods to reduce stress, clarifying the purpose and setting clear expectations for QEs, offering more structured support for students, and enhancing flexibility of QE formats can improve student experiences and reduce attrition related to QEs (McLaughlin et al., 2023; Harding-DeKam et al., 2012; Nerad and Cerny, 1999). This evidence underscores the potential value of utilizing cognitive apprenticeship (i.e., explicating the implicit) and addressing the hidden curriculum.
There is a demand for research that can inform best practices regarding QE formatting and assessment and evaluate the impact of QE formatting on student outcomes and alignment with student needs (McLaughlin et al., 2023). In this study, we explore a Pilot Model QE format that builds upon the extant Traditional Model QE to integrate tailored student-centered career and professional competencies through a collaborative process between faculty and students. In addition to aligning Pilot Model QEs with students’ career aspirations, this process required faculty to explicate their expectations and evaluation criteria for students thereby increasing transparency.
This study was implemented within a health professions School at a large, publicly funded, high research activity university in the southeastern United States. The school is comprised of ~120 full-time faculty and ~ 105 doctoral students housed within five divisions encompassing various disciplines within the biomedical and social sciences. This study focused specifically on part of the written QEs in a doctoral program within a division concentrated on basic science related to pharmaceutical sciences at a large public research university in the southeastern United States. The division consists of approximately 50 faculty members, including primary, adjunct, and emeritus professors. About one-third of these faculty members are actively involved in administering QEs, and approximately 8 students in this division complete the QE for this program each year.
This study utilized a multi-phased approach to (1) explicate the Traditional and Pilot Model QEs utilized in the first and second year; (2) explore student perspectives of the Traditional and Pilot Model QEs; and (3) dig deeper into student experiences with the Pilot Model QE. During all phases, relevant findings were mapped to the cognitive apprenticeship framework to elucidate how various aspects of the Pilot Model QE aligned with the cognitive apprenticeship domains. One faculty member (n = 1) utilized the Pilot Model QE while the others (n = 9) utilized the Traditional Model QE, for a total of 10 rated QEs (k = 10). All participants were students and faculty from a division concentrated on the basic science of pharmaceutical sciences as described in more detail below. In Phase 1, faculty members (n = 2) participated in an interview, one faculty member used the Traditional Model QE approach, and one utilized the Pilot Model QE approach. All students were invited to take part in surveys (n = 8 participated in Phase 2) and focus groups (n = 5 participated in Phase 3), with the invitation and data collection purposefully managed by a study team member who had been newly hired and hence unfamiliar to both students and faculty in order to reduce potential for response bias from either survey or focus group participants. Furthermore, participants in both survey and focus groups were explicitly informed that all data would be de-identified and/or shared in aggregate trends in order to protect participant privacy and encourage veracity of reporting. Subsequent analysis, visualization, and interpretation of the data was first generated and reviewed by study team members who were not involved in the QE process (e.g., not faculty QE administrators). Only after the data had been analyzed and interpreted by other study team members were the findings shared with the study team member who also served as a QE administrator. In all cases, participation was voluntary and without compensation, and participants were informed of the purpose and duration of their participation and protection of their data and planned uses thereof. The information about participating was included either at the start of the survey or in email invitations to focus group or faculty participants respectively, in accordance with approved ethical research practices (Institutional Review Board # IRB # 18–3140).
The purpose of Phase 1 was to describe the Traditional and Pilot Model QE format, clarity of expectations, assessment processes, and relationship to program defined competencies, professional development, and student career aspirations. Faculty interviews and document analyses were utilized until sufficient data for describing the models were collected. Two faculty members participated in a one-hour in-person semi-structured interview to provide insight into structure, process, expectations, and communication. Faculty Member 1 and Faculty Member 2 were both tenured faculty members who trained PhD students at the institution. Faculty member 1 was also a tenured faculty member who trained students in the School and served as the Director of Graduate Studies Program, and Principal Investigator (PI) of the pilot study (see Methods and Limitations sections for additional discussion of protocol decisions made to reduce potential bias).
Document review and analyses were conducted of the following: the graduate school handbook, emails between faculty and staff delineating QE topics, QE rubrics, QE topics, and required and suggested QE reference lists from faculty. Data analysis involved thematic coding by a single coder with education research training, a study team member who was not known to any participants interviewed prior to participating in the study. The analysis of faculty interviews was focused on contextualizing and obtaining descriptive insights to build upon information gathered from the document review. Aspects of model descriptions that corresponded with the domains of cognitive apprenticeship were specifically noted. Member-checking was utilized to ensure accuracy of model descriptions. The results of these analyses were used to inform a comprehensive framework for the Traditional and Pilot Model QEs.
The purpose of Phase 2 was to assess student perceptions regarding their experiences with the Traditional and Pilot Model QE, specifically with regard to transparency, fairness, clarity of expectations, interest level, value, program and career alignment. A mixed-methods study design was employed and included administering and analyzing quantitative and qualitative data from surveys. This approach was selected to support a comprehensive analysis, provide preliminary insight, and guide the development of Phase 3.
Phase 2 participants were doctoral students in a division focused on the basic science of pharmaceutical sciences completing their 2nd-year of the PhD program (n = 8). Participants were believed to represent a broad array of career and scientific interests (e.g., medicinal chemistry, informatics, biochemistry; industry R&D, academia, entrepreneurism), with example career interests drawn from previous discussions with the Director of Graduate Studies Program. Participants were recruited via email using contact information of the study team member without any prior affiliation with the department to increase participant comfort with responding. The initial invitation was followed by reminders, and respondents were informed of the components of participation (e.g., duration, data protections, purpose/data usage, voluntary nature) as part of the initial survey or focus group invitation.
All students that participated in the Pilot Model QE completed Qualtrics survey after their final QE (n = 8, 100% response rate). The number of QEs completed by students varied depending on the points earned for each QE, with a maximum of four points possible per QE. To complete the QE process successfully, students needed to receive a passing score of 24 points across their QEs. The purpose of the survey was to measure student perception regarding transparency, fairness, clarity of expectations, interest level, value, program and career alignment surrounding program defined processes and competencies for each QE they completed. Students were surveyed about their perspectives of each QE topic for each construct (e.g., clarity of expectations). The survey included 25 Likert-scale type items, generally measured on a scale from 1 to 5 including matrix ratings for each QE corresponding the 6 main constructs of interest, as well as 6 short answer questions corresponding to the ratings provided for construct (10 items per matrix, for a total of 99 ratings across all 25 questions). The anchors for each item were aligned with the construct (e.g., extremely satisfied to extremely dissatisfied) (Full survey included in Supplemental File 1)
Due to the small sample size, analysis of quantitative items utilized descriptive statistics. Specifically, measures of central tendency (mean) and dispersion (standard deviation) were calculated for each construct. Participant ratings were averaged across QEs using the Traditional Model QE (k = 9), and within the single QE using the Pilot Model QE (k = 1). Inferential statistics were not used to make comparisons since only one faculty member utilized the Pilot Model QE in this study. Qualitative data were obtained from short-answer survey responses, which were analyzed thematically to uncover deeper insights and contextual nuances, with the results from both methods compared and integrated to provide well-rounded interpretation of the findings. Aspects of the survey corresponding to the domains of the cognitive apprenticeship model were noted.
The purpose of Phase 3 was to assess student perceptions of the Traditional and Pilot Model QE; specifically with regard to time, transparency, fairness, clarity of expectations, interest level, value, program and career alignment. Qualitative methods were employed to obtain rich descriptive qualitative data to cross-reference with document review/faculty interviews and garner deeper insights regarding hidden curriculum, transparency, accessibility, clarity of expectations surrounding program defined competencies and specifically QEs within the PhD Program.
Phase 3 participants were recruited from Phase 2 participants via email, including reminder emails. The recruitment email provided details about participation in the study and their consent was confirmed verbally during participation in the focus groups. Five students participated in the focus group (62.5% response rate).
A semi-structured focus group was conducted consisting of 14 questions. Considerations to mitigate bias were carefully taken into account while designing the focus group questions. The focus group sessions were designed and conducted by an independent researcher, with no involvement from the Principal Investigator. To reduce bias and ensure confidentiality, the facilitator, an external administrator with no prior relationship with the participants, led the discussions and carried out the analysis. Additionally, participants were informed prior to participating in the focus groups that the data would be de-identified to ensure anonymity and prevent any potential impact on their ongoing relationships within their departments. Scripts were developed and used to uncover critical aspects of participant experiences surrounding their QEs and explicate findings from the Phase 2 survey. Transcripts were edited to remove vocal clutter such as filler words and nonverbal sounds, ensuring clarity and focus on the content of the participants’ responses. A single coder utilized thematic coding to analyze the data.
To identify themes in focus group data, a thorough review of the transcripts was conducted. This included reading them multiple times to gain a comprehensive understanding of the content. Initial codes were generated by labeling significant and relevant segments of text that aligned with research questions. These codes were then grouped into broader categories to uncover recurring patterns and ideas. Each category was reviewed and refined to ensure it accurately reflected the data, and themes were defined and named accordingly. Select illustrative quotes from the data were incorporated to support and substantiate each theme, ensuring that the findings were rooted in the participants’ responses.
Focus group results corresponding to the domains of the cognitive apprenticeship model were noted. Thematic analysis was employed to categorize findings into broader themes that reflect the operationalization of cognitive apprenticeship domains. This process involved assessing the alignment of these themes with the framework’s principles and validating the findings through cross-referencing. Exemplars were selected to illustrate direct quotes that corresponded to each of the cognitive apprenticeship domains and reflect how the focus group results were situated within the theoretical framework.
The studies involving human participants were reviewed by the Office of Human Research Ethics, which has determined that this submission does not constitute human subjects research as defined under federal regulations [45 CFR 46.102 (e or l) and 21 CFR 56.102(c)(e)(l)] (NHSR per Institutional Review Board, IRB # 18–3140). Participants were informed about study participation via email, and consent to participate was obtained either by digital participation acknowledgement (survey) or verbal participation acknowledgement (focus groups and interviews).
This section presents descriptions of the 2023–2024 Traditional and Pilot Model QEs, formulated through content analysis of the graduate school handbook, select emails between faculty and staff delineating QE topics, QE rubrics, QE topics, required and suggested QE reference lists from faculty and insights gathered from two faculty interviews (Figure 1). Both models adhered to the university requirements for QEs which allow flexibility in how Schools and Departments structure PhD examinations. The graduate school handbook outlines the following characteristics of Written and Oral Qualifying exams:
1. Assess the extent and currency of the candidate’s knowledge in a manner that is as comprehensive and searching as the best practices of that field require,
2. Test the candidate’s knowledge of all transferred courses,
3. Discover any weakness in the candidate’s knowledge that need to be remedied by additional course or other instruction; and
4. Determine the candidate’s fitness to continue to work toward a doctorate. (The graduate school handbook, 2023)
Figure 1. Schematic representation of the Traditional Model QE in the program that was studied compared to the Pilot Model QE.
The PhD program in which the QE innovation was piloted has two components to the written QE. The first is a series of cumulative exams (colloquially referred to as “cumes” within this division), focused on recent scientific literature that start after the second semester of the program and continue for up to 12 months, with students accumulating a maximum of four points per exam until they reach a total of 24 points. The second is a written proposal on the student’s thesis, which also serves as the prospectus for the dissertation research.
During their graduate program orientation, students were provided a digital copy of the graduate school handbook which outlines the above general requirements regarding QE exams. According to faculty interviewed, the Traditional Model QE consisted of a faculty member identifying a topic, generally describing the topic to the students, and offering a set of related publications for students to read. In this doctoral program, students were given the topics and recommended readings about a month in advance to ensure ample preparation time. The format of the QEs varied and could include written exams, in-class or take-home, open or closed book, and were designed to take around 2–3 h to complete. Both faculty members noted that QE topics were usually selected based on the research or interest area of the faculty who administered them. Aligning QE topics with faculty interests typically inherently corresponds with broader scientific and technical objectives and competencies of the training programs. Yet as one faculty member noted, there is an inherently broad scientific content included, “we do not have any of our competencies defined in such a granular way that any exam that does not have a chemical biology as the basis in terms of publications that were used, would not fit within that umbrella.” (Faculty interview, 4:14–4:35). Reinforcing the focus exclusively on scientific thought and content in the Traditional Model, “the only aspect of professional development that was ever captured in any of the cumes would be around scientific discourse.” (Faculty Member 1).
In the Traditional Model QE, all students received the same QE question from an individual faculty member.
“All of our training is focused on one of the outcomes that we have articulated…And that outcome is…language, the scientific, technical literacy, technical development, developing a project. So that’s the first outcome. The second outcome is professional development for exploration. Professional conferences, etc. and we do not really assess those. We just write a little bit. And we assess oral communication. That’s probably about it” (Faculty Member 1).
Prior to their exam, students received an email reminder that their QE was approaching, and they were provided with the date and time of their exam along with instructions from individual faculty members which included a list of assigned readings and their QE topic. In contrast to the Pilot Model QE, students did not receive rubrics that detailed how their response to the QE would be scored. Furthermore, students were not explicitly consulted or involved in identifying or developing QE topics.
The Pilot Model for QEs was differentiated from the Traditional Model QE by the inclusion of a collaborative process between faculty and students to create personalized, competency-based assessments. Similar to the Traditional Model QE, these assessments focused on discipline-specific competencies and addressed the requirements outlined in the graduate school handbook. However, unlike the Traditional Model QE, the Pilot Model QEs were designed to be student-centered meaning that they integrated competencies related to career exploration and aligned with individual students’ career goals. The Pilot Model QEs intentionally sought student input in order to co-create a tailored QE experience aligning with students’ career goals and interests (see Table 1).
In this model, Faculty Member 1 met with students about 6 weeks before their exam to discuss their career aspirations. Building on the guidelines included in the graduate school handbook, faculty and students collaboratively developed the Pilot Model QE topic around students’ self-identified career aspirations. Faculty Member 1 provided students with an opportunity to suggest the topic or help formulate it. Faculty Member 1 utilized five different QE topics based on students’ career aspirations (see Table 1).
The Pilot Model QE was a take-home exam, and students had a month to complete it. In contrast to the Traditional Model QE, in the Pilot Model QE students received an assessment rubric electronically along with their QE topic. Additionally, Faculty Member 1 followed up with students to ensure they comprehended the topic and the evaluation criteria (Table 2).
Student survey results indicated higher ratings in the areas of clarity of expectations, level of interest, alignment with dissertation research, training goals, career goals, and value and on QEs that used Pilot Model QE vs. the Traditional Model QE (see Table 3; see Supplemental Data File for survey data). Survey results indicated that students perceived the Pilot Model QE required more time to prepare for (though equivalent times to complete the two) and was less aligned with their expectations. For instance, students indicated that they typically spent less than 6 h (ordinal mode) preparing for Traditional QEs, versus between 6 and10 hours’ time spent preparing for Pilot QEs. In contrast, students’ completion times were roughly equivalent, likely hitting a ceiling effect as this was the highest choice presented to participant raters, with both Traditional QE and Pilot QE completion time for each type of QE estimated at greater than 21 h, respectively, (ordinal mode). Perhaps not surprisingly, given the high time needs for QE completion overall satisfaction with time preparing for exam (M = 3.5, SD = 0.93) and satisfaction with time completing exam (M = 3.63, SD = 0.92) were both slightly positive, but fell within the neutral range (3 = neither satisfied nor dissatisfied), suggesting room for improvement across QE preparation time and duration overall (note that this was measured in sum but not separately for Pilot vs. Traditional QEs).
Not all students provided responses to the short answer questions on the survey. However, the responses provided by students were consistent with the findings resulting from the Likert Scale items regarding the Traditional and Pilot Model QEs. For example, students expressed higher levels of interest in the Pilot Model QE. One student responded on the short answer section of the survey: “I think the Pilot Model QE was the most interesting because it dealt the most with our career goals. So I definitely spent more time than just reading a couple of papers.” Similarly, students’ short-answer responses indicated they perceived the Pilot Model QE to align with their career aspirations and to require more time to complete.
Through an analysis of the focus group data, the following themes emerged concerning both the Traditional and Pilot Model QEs: clarity of expectations, content, and format, student time commitment, methods of evaluation, distribution of feedback, interest level, value, elements of hidden curriculum, and alignment with career aspirations. Analysis findings will be discussed further in the following sections.
Students felt that expectations regarding general QE pass/fail procedures in both the Traditional and Pilot Model QEs were well communicated. They reported receiving a copy of the graduate school handbook during their graduate program orientation, which outlined these procedures. Additionally, students indicated a thorough understanding of how the QE pass/fail system operates. Students reported that their expectations regarding the format of their Traditional Model QEs differed depending on the exam and the faculty member administering it. They noted variability among faculty in terms of the clarity and specificity of their Traditional Model QE instructions and the structure of the QE itself. Due to what was perceived by students as unclear guidance from faculty, some students were not certain whether the Traditional Model QE would be open-book or closed-book. Consequently, many assumed it would be closed book, prompting them to prepare more extensively.
In fact, extending this theme, students perceived a general lack of clarity regarding their QE topics within the Traditional Model QEs. One student described their Traditional Model QEs in the following way: “…they would usually have focus points. They usually were like this is the topic and this is what I might ask about…It’s never broken down more than that.”
Expectations for Traditional Model QEs were delivered “verbally and in writing” to students but the extent to which expectations were delivered varied across faculty members. In all cases prompts and required readings were delivered in advance. Students reported that they were not provided with information regarding the timing or format for receiving feedback and/or grades on their exams. The QE topics’ content typically focused on faculty interests in the Traditional Model QE. According to one focus group participant: “all of the topics are niche because it’s very much focused on interests/what the professor wants.” Students described the core components of their Traditional Model QEs as follows: they reported being prompted to recall information from the readings, analyze and interpret data, and critically assess the material covered in the required QE readings.
Students expected that faculty would choose Traditional Model QE topics aligned with the faculty member’s own areas of expertise or research interest. Their expectations were met, as faculty selected QE topics aligned with their respective expertise or fields of interest. Students reported that the format and administration of QEs varied based on the faculty responsible for their development. They had mixed feelings regarding the varying format of their QEs and expressed that the format of QEs was often unclear, inconsistent, and varied across faculty:
I guess I wasn’t sure if it was open or closed note – that was kind of up in the air. It depended for each professor what kind they chose. They told us ahead of time, and it was vague, if they remembered to.
While students reported understanding the actual number of points they were required to earn to pass, students universally expressed frustration with a lack of transparency regarding the evaluation methods of their QEs. They lacked clarity on a number of details about how points were assigned, whether there was a grading curve, or what criteria were used to assess their work. They reported that evaluation methods were unpredictable and varied across professors. They were unclear what the criteria were to earn points.
It is very hard to tell the metric of what you need to do to pass a three to get to a four…and if everyone does that to get to a four it is probably going to get readjusted.
Students explicitly noted that no rubrics were provided with their Traditional Model QEs. There was also considerable ambiguity and frustration expressed by students regarding what type of feedback they would receive and when they would receive it. The duration for receiving feedback from faculty varied significantly, with many students receiving only scores and minimal to no additional feedback on their QEs. Students reported that in their program, it was not standard procedure to get feedback, beyond a numeric score, on QEs:
Usually they just send an email with your score…and sometimes but not always they’ll say if you would like your exam, you come pick it up from the professor.
Students reported finding value in reading content and being exposed to topics outside of their interest area and field. They expressed that this helped equip them with skills and knowledge necessary for connecting with others academically in a scientific community. They also valued getting a sense of what was current and interesting based on the Traditional Model QE topic selections of expert faculty. Students expressed differing perspectives about the importance of reading required materials for their traditional QE.
I appreciated getting in the mindset of reading papers but sometimes it felt…like a waste of time to be reading these papers…this will never apply…but reading papers and…training myself how to read a scientific paper was very useful.
According to students, study preparation time involved a variety of activities for the Traditional Model QE which included: a thorough review of required and suggested materials, clarification of unfamiliar definitions, and identification and summarization of the key concepts presented in the resources, thoroughly reading required and suggested materials, clarifying unfamiliar definitions, and identifying and summarizing the general ideas presented in the resources provided by faculty. Students initially perceived the Traditional Model QE as requiring considerably less time overall. Upon further inquiry, it became clear that they were primarily referring to the time spent completing the exam itself, without factoring in the preparation time as they viewed “study time as separate.”
Students reported that their level of interest in the Traditional Model QEs varied based on relevance to their research interests or career aspirations. They also mentioned that professors’ enthusiasm about a QE topic likewise influenced their interest level.
I think a big part of that is just subjective interest in that topic…have I heard of this person before that’s on the paper…this topic…and I’ve been meaning to read about it anyway…and to some extent…the level of enthusiasm of the professor…which hard to communicate because we mostly communicate over email. Some professors.
Would just be like here’s three papers and then some professors could be like – here’s three papers, and here’s why they are important. And I found that very motivating…like okay, I actually need to pay attention to this because all this other stuff is built on top of that.
When asked how their QEs aligned with their training goals and research focus, students described the difference between their graduate training goals and their research focus:
Graduate training goals are a lot broader than your research focus…I’m not just here to finish this project and publish a paper. I’m here to become an independent scientist.
Several students were able to identify what their post-graduation career plans were more broadly (e.g., industry scientist, academic faculty member, postdoctoral scientific training), however they did not express explicit career goals (e.g., specific sectors with associated job titles) when queried. While most students felt that Traditional Model QE exam topics did not explicitly align with their research focus, they did feel that Traditional Model QE exam topics connected broadly to their career goals.
Students indicated that faculty expectations were well-defined for the Pilot Model QE, presumably in part due to the high level of student engagement in developing the Pilot Model QEs – which included meeting with the Pilot Model QE faculty member to discuss and develop their QE (six weeks prior to the due date) and reception of a rubric with their QE topic (1 month before its due date). The faculty member was guiding, flexible, and worked collaboratively with students as they developed the QE topics, for instance one student described the interaction as such:
He sent out a schedule to meet…I met him in person and then went through it, and he gave out some ideas of varying options…they were very vague…like if you are thinking about industry…here are some things you could do…he threw out some ideas based on what you thought your career trajectory might be…he said you could diverge from what he listed.
In the Pilot Model QE, the format of the QEs varied according to students’ career aspirations. Faculty member 1 collaborated with individual students to tailor the QE based on guidelines outlined in the graduate school handbook, along with student interest and career aspirations. One student described being permitted to “customize it however I wanted” highlighting a contrast with their experience of the Traditional Model QE. Another student in the focus group expressed that they found it challenging to design a QE tailored to their own interests and proposed that having a set of examples would have been beneficial for guidance: “it would have been helpful to see some examples of what other students had done…I’m not creative.” While the formats of the tailored Pilot Model QE prompts varied, students noted that faculty expectations regarding each prompt within the Pilot QE format were clearly communicated.
According to students, Pilot Model QE evaluation methods were “outlined very well.” Students were provided with a rubric “from the beginning…we had it before we started.” They noted and expressed appreciation for the level of feedback they received on their Pilot Model QE: “I was impressed with how thoroughly he read it, because I know he had a lot to read.” Students reported that they found faculty feedback more valuable when it was relevant to their career goals.
I guess based on the topics it does not seem like it would really be that necessary if it’s on a topic chosen by the faculty…but like if Pilot QE instructor had you do something relevant to your future, career…
Students found the Pilot Model QE to be valuable both in terms of participation and feedback, noting its relevance to their interests and career aspirations. They collectively agreed that working with Faculty Member 1 to select a Pilot Model QE topic aligned with their career goals was a meaningful experience. They indicated that working on a project aligned with their career goals felt purposeful and advantageous for their professional development as it involved critical thinking about topics pertinent to their professional interests. Students reported placing higher value on receiving faculty feedback on their QEs when their QEs aligned with their research and career goals. They found the feedback on their Pilot Model QE particularly valuable because it was directly relevant to their future career objectives. One student shared the following about their experience with the Pilot Model QE:
I got value out of my [QE] because the topic I chose was related to my project…so it is definitely a direct benefit to what I was doing in life.
Students described the type of preparation they did for the Pilot Model QE as fundamentally distinct from the Traditional Model QEs, in that:
Preparation was extremely different and probably the most time for me personally…but that was only different in that I had to write more like a literature review than take a two-hour written exam.
Initially, students mentioned that the Pilot Model QE required slightly more time than the Traditional Model QE. They explained that the open-ended nature of the Pilot Model QE made it challenging to determine when to stop working on it. Students also relayed concerns about the potential for an overwhelming workload if all exams were structured in this format.
Students expressed a high level of interest in the Pilot Model QE because it directly aligned with their career interest. One student described it as “right up my alley.” Students conveyed that their Pilot Model QEs were deliberately designed to align with their career goals. Throughout the focus group discussions, multiple students demonstrated awareness of the so-called hidden curriculum that can be used to better navigate their QEs. Some students reported that they actively sought clarification from faculty about prompts and QE formats, engaged in discussions with faculty regarding QE question development, followed up with faculty with questions about their QEs, gathered insights from senior students about QEs, and asked peers for explanations about unfamiliar aspects of the QE process. Additionally, one student displayed visible surprise when others mentioned consulting older students about QEs and seeking further clarification from faculty regarding QEs. When students were asked about additional factors influencing their expectations regarding their QEs, one student provided the following response:
Senior students…I got more information from the students because what is in the handbook is vague. It’s like a paragraph that makes sense…from the students you kind of get the gist…this professor might never return a grade…or this one might curve it in such a way, or this one asks a question about the data or figures…and this one asks…
The Pilot Model QE incorporated elements from all four cognitive apprenticeship domains and built on the Traditional Model QE by intentionally and explicitly integrating elements from the sociology domain. Exemplary quotes reflecting the cognitive apprenticeship domains are provided in Table 4.
Table 4. Mapping of Pilot Model QE to cognitive apprenticeship using model descriptions and exemplary quotes.
Societal expectations regarding student outcomes after graduation evolve alongside economic and socio-cultural developments. Meeting these objectives requires integrating knowledge, skills, and attitudes, and situating these competencies within diverse authentic contexts (Baartman et al., 2006; Baartman et al., 2007; van Merriënboer and Kirschner, 2007). Given that assessments impact student outcomes (Boud and Falchicov, 2006; Boud and Falchicov, 2007; Watkins et al., 2005), learning activities should be constructively aligned with assessment criteria (Biggs, 1996; Cohen, 1987 as cited in Gerritsen-van Leeuwenkamp et al., 2017). Creating a QE process that includes some components that are flexible, collaborative, agile, and aligned with student interests could ensure that these assessments effectively prepare students for contemporary – and evolving – needs of the workforce.
Educational institutions are responsible for ensuring the quality of assessments, which poses a challenge given the lack of overarching conceptualization of assessment quality (Gerritsen-van Leeuwenkamp et al., 2017). This study revealed considerable variability across QEs in the clarity of expectations, evaluation methods, and feedback mechanisms. Utilizing a student-centered approach that situated student career goals and interests within the QE was generally perceived by students as valuable, interesting, and well aligned with their goals. This supports other research that highlights the impact of student-centered approaches to training and assessment, such as situated learning and context personalization (Bernacki and Walkington, 2018; Darwin et al., 2022). Establishing QE design criteria that promote the consistent use of student-centered strategies - and explicitly communicating these design criteria to students - may help minimize ambiguity, improve transparency, increase motivation, and enhance assessment quality.
Expectations for how students will be assessed in the doctoral curriculum are often perceived by students as unclear, a finding described in the literature (e.g., McLaughlin et al., 2023) and supported by this study. Per the cognitive apprenticeship framework, the expertise that faculty utilize to evaluate learners should be made visible (Collins et al., 1989). As such, students should be provided with clear guidelines regarding evaluation methods and mechanisms for receiving explicit feedback regarding their QEs. Students in this study felt that receiving a rubric along with their Pilot Model QE topic increased clarity of expectations, decreased student stress, and increased transparency surrounding the QE process. Rubrics have been demonstrated to be an effective tool for enhancing consistency in assessment (Cockett and Jackson, 2018). In the Pilot Model QE, Faculty Member 1 designed the rubric based on the QE. We suggest that faculty and students should collaborate to develop and implement QE rubrics irrespective of the type or discipline specific focus of QE. Evidence suggests that student involvement in the design and implementation of the rubric is essential for its effectiveness (Cockett and Jackson, 2018). Moreover, we recommend that PhD programs should consider providing faculty with a rubric template for developing student-centered QEs. This may help address time constraints and enhance clarity regarding expectations for content, format, and evaluation method for both students and faculty. In this pilot, rubrics were crafted for each of the 5 QE topics (e.g., 5R analysis, build a website). These instances can be documented as examples, and one can envision this collection growing over time, contributing to a comprehensive catalog. Over time, we suggest building and compiling a repository of past exams and exam categories that align with diverse science-related career paths. Establishing such a catalog could help alleviate concerns related to selecting topics and the time needed to create student-centered QEs and corresponding evaluation methods. Researchers should also explore the impact of collaborating with community mentors to develop student-centered QEs for graduate students in STEM that focus on students’ career aspirations and career outcomes. Furthermore, this collaboration would enable field experts to assess student-centered QEs, ensuring their content validity. Finally, we suggest that independent of QE type, faculty can identify ways to incorporate some aspects that are student career focused without diminishing the value of QEs in established discipline specific competencies. For example, many written QEs are proposal based, and NSF proposals require a broader impact section. Using this as a model, it would be straightforward to have students to address career specific competencies in a “career” section. For take home QEs based on critical evaluation of the literature or solving specific problem, the examples in the pilot we describe could easily be modified. Future research will need to replicate and extend these initial findings to other QE formats to evaluate whether similar modifications could be equally impactful.
The findings of this study underscore the necessity for greater transparency and clarity within the Traditional Model QEs. The results suggest that collaborating with students to select QE topics aligned with their career goals, having faculty clearly outline expectations through rubrics detailing evaluation criteria, and offering explicit feedback on QE exams can significantly improve transparency and clarity for students. These findings are in agreement with prior work in the literature, which emphasizes the need for transparency and intentional design of aligning learning objectives, assessments, and instructional strategies (e.g., Liera et al., 2023). The system should provide clear objectives that explicitly define the skills students are expected to acquire, as well as explicate the methods for teaching and assessing these skills (Liera et al., 2023). Additionally, given the changing landscape of assessment quality, scholars should consider evaluating the relevance of these models longitudinally as career goals evolve and assessing their influence on individuals’ long-term career outcomes.
In general, this study demonstrates the utility of the cognitive apprenticeship framework for conceptualizing and understanding QEs. Educators can articulate and evaluate implicit knowledge explicitly, not only in teaching but also through assessment. Results from this pilot study indicate that the Pilot Model QEs integrated elements from all four domains of the cognitive apprenticeship framework and explicitly integrated critical subdomains from the sociology domain. This intentional integration is especially important for aligning with professional development, career aspirations, and student outcomes. Researchers should continue to explore the use of cognitive apprenticeship to guide development of assessments for graduate students. These exams are programmatic gateways to advanced training stages and implementing evidence-based strategies can enhance evaluation tools, ensure alignment of outcomes with training objectives, and elevate the student learning experience (McLaughlin et al., 2023). A systematic approach to qualifying exams holds promise for advancing these critical objectives in preparing the biomedical workforce for future challenges (McLaughlin et al., 2023).
Several limitations were noted in the study, including those that impact generalizability, applicability over time and across career goals, feasibility, generation of custom topics. Although the small sample size may limit generalizability, this reflects the preliminary nature of the investigation within a single department, and was mitigated by a multi-phased, mixed methods approach. In addition, it is unclear if our findings would generalize beyond a written QE format, as only one form of QE, specifically a written QE, was administered to students in this pilot study. Future directions should evaluate additional formats such as oral, projects, portfolios, and other creative solutions to doctoral assessments. Another potential limitation was the risk of bias stemming from the proximity, as lead author, of the investigator and administering faculty of the Pilot Model QE to the study. To address this concern, measures were taken to mitigate bias: an administrator from outside the department conducted faculty interviews and focus groups, administered the student surveys, and performed the subsequent data analysis. Furthermore, this was administered in only one department initially. Future work should replicate findings across departments, disciplines, and institutions.
We acknowledge that students’ career aspirations often evolve over time. Research on career choices among STEM doctoral students documents a 20% change in career preferences from early to later stages of training (Sauermann and Roach, 2012); (Gibbs et al., 2014). This observation is consistent with our observations on student exit surveys among our Pharmeceutical Sciences doctoral trainees over the past decade, which reveal that 67% of students start their studies with well-defined career goals that persist throughout their education, while the remaining 33% modify and refine their career paths at various stages of their academic journey. Future research should focus on examining the long-term impact of tailored student-centered QEs focused on students’ career aspirations on their career outcomes as well as determine the extent that QEs should include student-centered versus discipline-specific components. The current pilot included 10% student-centered assessment, and this seems like a reasonable magnitude for initial assessment.
In addition, a challenge lies in the perceived substantial time investment demanded from faculty members for the development, administration, evaluation, and provision of explicit feedback to students on the QE. Similarly, students initially expressed` apprehension regarding the preparation and completion time associated with the Pilot Model QE when compared to the Traditional Model QE. However, upon closer scrutiny, students found that the time commitment required by the Traditional Model QE was comparable to that of the Pilot Model QE.
Working collaboratively with faculty to design QEs that match student interests and career goals might come naturally to some students, but not all. Creating a QE topic centered on students’ career goals can be challenging, often necessitating clear guidance and examples from previous exams.
QE exams currently serve as a pivotal gateway in doctoral programs, assessing readiness for dissertation research. Due to the high stake’s nature of these exams, it is critical to establish best practices for developing QEs content, format, and evaluation methods. By incorporating student-centered and adaptable strategies, such as those that align with the cognitive apprenticeship model, and providing clear guidelines and rubrics, the transparency and effectiveness of QEs can be enhanced. This approach not only supports students’ career aspirations but also ensures the development of competent and independent scientists who are prepared to overcome challenges in their future fields.
The original contributions presented in the study are included in the article/Supplementary material, further inquiries can be directed to the corresponding author/s.
The studies involving humans were approved by the Office of Human Research Ethics. The studies were conducted in accordance with the local legislation and institutional requirements. The participants provided their written informed consent to participate in this study.
AD: Conceptualization, Data curation, Formal analysis, Investigation, Methodology, Writing – original draft, Writing – review & editing. JM: Conceptualization, Formal analysis, Funding acquisition, Methodology, Supervision, Writing – original draft, Writing – review & editing. RLL: Conceptualization, Formal analysis, Funding acquisition, Methodology, Supervision, Writing – original draft, Writing – review & editing. PB: Conceptualization, Formal analysis, Funding acquisition, Methodology, Writing – original draft, Writing – review & editing. MJ: Conceptualization, Formal analysis, Funding acquisition, Methodology, Supervision, Writing – original draft, Writing – review & editing.
The author(s) declare that financial support was received for the research, authorship, and/or publication of this article. This work was supported by the National Science Foundation grant 2325518 to Jarstfer, McLaughlin, Layton, and Brandt and National Institute of General Medical Sciences grant 1R01GM140282 to Layton and Brandt.
The authors declare that the research was conducted in the absence of any commercial or financial relationships that could be construed as a potential conflict of interest.
All claims expressed in this article are solely those of the authors and do not necessarily represent those of their affiliated organizations, or those of the publisher, the editors and the reviewers. Any product that may be evaluated in this article, or claim that may be made by its manufacturer, is not guaranteed or endorsed by the publisher.
The Supplementary material for this article can be found online at: https://www.frontiersin.org/articles/10.3389/feduc.2024.1474572/full#supplementary-material
Baartman, L. K. J., Bastiaens, T. J., Kirschner, P. A., and Van Der Vleuten, C. P. M. (2006). The wheel of competency assessment: presenting quality criteria for competency assessment programs. Stud. Educ. Eval. 32, 153–170. doi: 10.1016/j.stueduc.2006.04.006
Baartman, L. K. J., Bastiaens, T. J., Kirschner, P. A., and van der Vleuten, C. P. M. (2007). Evaluating assessment quality in competence-based education: a qualitative comparison of two frameworks. Educ. Res. Rev. 2, 114–129. doi: 10.1016/j.edurev.2007.06.001
Bernacki, M., and Walkington, C. (2018). The role of situational interest in personalized learning. J. Educ. Psychol. [Preprint] 110, 864–881. doi: 10.1037/edu0000250
Biggs, J. (1996). Enhancing teaching through constructive alignment. High. Educ. 32, 347–364. doi: 10.1007/BF00138871
Boud, D., and Falchicov, N. (2006). Aligning assessment with long-term learning. Assess. Eval. High. Educ. 31, 399–413. doi: 10.1080/02602930600679050
Boud, D., and Falchicov, N. (2007). Rethinking assessment for higher education: Learning for the longer term. London: Routledge.
Cockett, A., and Jackson, C. (2018). The use of assessment rubrics to enhance feedback in higher education: an integrative literature review. Nurse Educ. Today 69, 8–13. doi: 10.1016/j.nedt.2018.06.022
Cohen, D. K. (1987). Educational technology, policy, and practice. Educ. Eval. Policy Anal. 9, 153–170. doi: 10.3102/01623737009002153
Collins, A., Brown, J. S., and Newman, S. E. (1989). “Cognitive apprenticeship: teaching the crafts of reading, writing, and mathematics” in Knowing, learning, and instruction: Essays in honor of Robert Glaser, ed. L. B. Resnick (Hillsdale, NJ: Lawrence Erlbaum Associates, Inc.), 453–494.
Darwin, T., Walkington, C., and Pruitt-Britton, T. (2022). “Connecting learning in higher education to students’ career and personal interests” in Handbook of research on opening pathways for marginalized individuals in higher education. eds. S. P. Huffman, D. D. Cunningham, M. Shavers, and R. Adamson (Hersey, PA: IGI Global), 147–170. doi: 10.4018/978-1-6684-3819-0.ch009
Elliot, D. L., Bengtsen, S. E., Guccione, K., and Kobayashi, S. (2020). The hidden curriculum in doctoral education. Cham: Palgrave Pivot.
French, S., Dickerson, A., and Mulder, R.A (2024) A review of the benefits and drawbacks of high-stakes final examinations in higher education. High. Educ. 88, 893–918. doi: 10.1007/s10734-023-01148-z
Gerritsen-van Leeuwenkamp, K. J., Joosten-ten Brinke, D., and Kester, L. (2017). Assessment quality in tertiary education: an integrative literature review. Stud. Educ. Eval. 55, 94–116. doi: 10.1016/j.stueduc.2017.08.001
Gibbs, K. D., McGready, J., Bennett, J. C., and Griffin, K. (2014). Biomedical science ph.D. career interest patterns by race/ethnicity and gender. PLoS One 9:e114736. doi: 10.1371/journal.pone.0114736
Goldman, C. A., and Massy, W. F. (2000). The Phd factory: Training and employment of science and engineering doctorates in the United States. Bolton, MA: Anker Pub Co.
Griffith, J., and Smith, M. S. (2020). The hidden curriculum in higher education. New York: Routledge.
Harding-DeKam, J. L., Hamilton, B., and Loyd, S. (2012). The hidden curriculum of doctoral advising. NACADA J 32, 5–16. doi: 10.12930/0271-9517-32.2.5
Hartnett, R. T., and Katz, J. (1977). The education of graduate students. J. High. Educ. 48, 646–664. doi: 10.1080/00221546.1977.11776583
Liera, R., Liera, R., Rodgers, A. J., Irwin, L. N., and Posselt, J. R. (2023). Rethinking doctoral qualifying exams and candidacy in the physical sciences: learning toward scientific legitimacy. Phys. Rev. Phys. Educ. Res. 19:020110. doi: 10.1103/PhysRevPhysEducRes.19.020110
McLaughlin, J. E., Minshew, L. M., Gonzalez, D., Lamb, K., Klus, N. J., Aubé, J., et al. (2019). Can they imagine the future? A qualitative study exploring the skills employers seek in pharmaceutical sciences doctoral graduates. PLoS One 14:e0222422. doi: 10.1371/journal.pone.0222422
McLaughlin, J. E., Morbitzer, K., Meilhac, M., Poupart, N., Layton, R. L., and Jarstfer, M. B. (2023). Standards needed? An exploration of qualifying exams from a literature review and website analysis of university-wide policies. Stud. Grad. Postdoct. Educ. 15, 19–33. doi: 10.1108/SGPE-11-2022-0073
Minshew, L. M., Olsen, A. A., and McLaughlin, J. E. (2021). Cognitive apprenticeship in STEM graduate education: a qualitative review of the literature. AERA Open 7. doi: 10.1177/23328584211052044
Morris, R., Perry, T., and Wardle, L. (2021). Formative assessment and feedback for learning in higher education: a systematic review. Rev. Educ. 9:e3292. doi: 10.1002/rev3.3292
National Academies of Sciences, Engineering, and Medicine (2018) in Graduate STEM education for the 21st century. eds. A. Leshner and A. Scherer (Washington, DC: National Academies Press).
Nerad, M., and Cerny, J. (1999). Postdoctoral patterns, career advancement, and problems. Science 285, 1533–1535. doi: 10.1126/science.285.5433.1533
Olsen, A. A., Minshew, L. M., Jarstfer, M. B., and McLaughlin, J. E. (2020). Exploring the future of graduate education in pharmaceutical fields. Med Sci. Educ. 30, 75–79. doi: 10.1007/s40670-019-00882-3
Ramadoss, D., Bolgioni, A. F., Layton, R. L., Alder, J., Lundsteen, N., Stayart, C. A., et al. (2022). Using stakeholder insights to enhance engagement in PhD professional development. PLoS One 17:e0262191. doi: 10.1371/journal.pone.0262191
Sauermann, H., and Roach, M. (2012). Science PhD career preferences: levels, changes, and advisor encouragement. PLoS One 7:e36307. doi: 10.1371/journal.pone.0036307
van Merriënboer, J. J. G., and Kirschner, P. A. (2007). Ten steps to complex learning: A systematic approach to four-component instructional design. Mahwah, NJ: Lawrence Erlbaum Associates Publishers.
Walker, G. E., Golde, C. M., Jones, L., Conklin Bueschel, A., and Hutchings, P. (2008). The formation of scholars: Rethinking doctoral education for the twenty-first century. 1st Edn. San Francisco: Jossey-Bass.
Watkins, D., Dahlin, B., and Ekholm, M. (2005). Awareness of the backwash effect of assessment: a phenomenographic study of the views of Hong Kong and Swedish lecturers. Instr. Sci. 33, 283–309. doi: 10.1007/s11251-005-3002-8
Keywords: qualifying exams, PhD training, professional development, career exploration, PhD competencies
Citation: Davidson AO, McLaughlin JE, Layton RL, Brandt PD and Jarstfer MB (2024) Innovations in qualifying exams: toward student-centered doctoral training. Front. Educ. 9:1474572. doi: 10.3389/feduc.2024.1474572
Received: 01 August 2024; Accepted: 06 November 2024;
Published: 22 November 2024.
Edited by:
Veronica A. Segarra, Goucher College, United StatesReviewed by:
Veronica Womack, Northwestern University, United StatesCopyright © 2024 Davidson, McLaughlin, Layton, Brandt and Jarstfer. This is an open-access article distributed under the terms of the Creative Commons Attribution License (CC BY). The use, distribution or reproduction in other forums is permitted, provided the original author(s) and the copyright owner(s) are credited and that the original publication in this journal is cited, in accordance with accepted academic practice. No use, distribution or reproduction is permitted which does not comply with these terms.
*Correspondence: Michael B. Jarstfer, amFyc3RmZXJAdW5jLmVkdQ==
Disclaimer: All claims expressed in this article are solely those of the authors and do not necessarily represent those of their affiliated organizations, or those of the publisher, the editors and the reviewers. Any product that may be evaluated in this article or claim that may be made by its manufacturer is not guaranteed or endorsed by the publisher.
Research integrity at Frontiers
Learn more about the work of our research integrity team to safeguard the quality of each article we publish.