- 1University of Teacher Education in Special Needs (HfH), Zürich, Switzerland
- 2Centre for Linguistics and the Graduate School of Teaching, University of Leiden, Leiden, Netherlands
- 3Department of Computational Linguistics, University of Zurich, Zürich, Switzerland
Introduction: Sign language fluency is an area that has received very little attention within research on sign language education and assessment. Therefore, we wanted to develop and validate a rating scale of fluency for Swiss German Sign Language (Deutschschweizerische Gebärdensprache, DSGS).
Methods: Different kinds of data were collected to inform the rating scale development. The data were from (1) focus group interviews with sign language teachers (N = 3); (2) annotated DSGS data from users/learners with various levels of proficiency (i.e., deaf native signers of DSGS, hearing sign language interpreters, and beginning learners of DSGS, approximately CEFR level A1-A2) (N = 28) who completed different signing tasks that were manipulated by preparation time; (3) feedback from raters (N = 3); and (4) complimented with theory from spoken and sign language fluency.
Results: In the focus group interview, sign language teachers identified a number of fluency aspects. The annotated DSGS data were analyzed using different regression models to see how language background and preparation time for the tasks can predict aspects of fluency (e.g., number and duration of pauses). Whereas preparation time showed only a slight effect in the annotated data, language background predicted the occurrence of fluency features that also informed the scale development. The resulting rating scale consisted of six criteria, each on a six-point scale. DSGS performances (N = 162) (same as the annotated data) from the different groups of DSGS users/learners were rated by three raters. The rated data were analyzed using multi-facet Rasch measurement. Overall, the rating scale functioned well, with each score category being modal at some point on the continuum. Results from correlation and regression analysis of the annotated data and rated DSGS performances complemented validity evidence of the rating scale.
Discussion: We argue that the different sources of data serve as a sound empirical basis for the operationalized “DSGS fluency construct” in the rating scale. The results of the analyses relating performance data to ratings show strong validity evidence of the second version of the rating scale. Together, the objective fluency measures explained 88% of the variance in the rating scores.
Introduction
Sign language fluency, framed as one component of a general sign language proficiency, is an area that has received very little attention within research on sign language education and assessment. While the components of proficiency in spoken languages have been described within models of communicative language ability (e.g., CLA; Bachman and Palmer, 1996) there have been no attempts so far to define sign language proficiency within such a model. Components within a model of CLA are, for example, grammar, vocabulary, pragmatic competence, and fluency. While some research studies have addressed selected aspects of sign language fluency (e.g., Lupton, 1998; Notarrigo and Meurant, 2022), we wanted to extend our current knowledge about the construct of fluency in sign languages in general, but also with a particular focus on Swiss German Sign Language (Deutschschweizerische Gebärdensprache, DSGS). We also wanted to see how fluency manifests itself in deaf and hearing DSGS signers with different levels of sign language proficiency. Sign language fluency is central for sign language mediated communication—when we know the observable and measurable aspects of fluency, we can include these fluency aspects in sign language research, teaching, and assessment. The latter point is relevant for the purpose of the study at hand: the development and validation of a fluency rating scale.
For the theoretical basis of this study, we will address (1) research studies in spoken language fluency, followed by (2) a general description on the structure of sign languages, and complemented (3) with research studies on sign language fluency, and (4) approaches to rating scale development.
Fluency in spoken languages
Fluency in spoken language is investigated in foreign language learning research and assessment because it is one of the most salient features of spoken language proficiency (Derwing et al., 2004). Fluency in spoken language has two notions (Lennon, 1990). The first notion comes from the way “fluency” is used in lay terms. This broad notion of fluency refers to overall proficiency in (oral) language use. For instance, to qualify language proficiency for a CV, people may use the term “fluent” to denote a high (oral) proficiency. The current paper, however, is concerned with the narrow notion of fluency which involves the ease or fluidity with which a speaker can go through all the steps to get from a message to articulation. This means that, within this narrow definition, fluency can be measured by investigating the temporal aspects of speech: speed, pauses, repetitions, and repairs (Tavakoli et al., 2020). This framework of fluency will serve as the basis for our study, supplemented with studies on sign language fluency.
Segalowitz (2010) made a distinction between such measurable aspects of fluency (“utterance fluency”) and the underlying ability of the speaker to go through all the processes easily (“cognitive fluency”). The third aspect that plays a role is “perceived fluency,” at least in the field of language assessment, in which raters make a judgment about the level of proficiency based on what they perceive about the fluency of the speaker. Concerning spoken language, many researchers have investigated the relation between utterance fluency and perceived fluency (e.g., Bosker et al., 2013; Cucchiarini et al., 2000; Derwing et al., 2004; Kormos and Dénes, 2004) and it can be concluded that raters, when given a precise definition of (aspects of utterance) fluency, indeed base their judgements on speed of speech, pauses, repetitions, and repairs (Bosker et al., 2013; see Suzuki et al., 2021 for a meta-analysis). There has also been some research to investigate the relation between cognitive fluency and utterance fluency. For instance, researchers have investigated the effect of task difficulty (when a task becomes cognitively harder at some stage in the speech production process) on utterance fluency. From these studies, it can be concluded that increasing cognitive difficulty (Goldman-Eisler, 1973; Felker et al., 2019) or syntactic difficulty (Sadri Mirdamadi and De Jong, 2015) leads to a decrease in utterance fluency (more silent pauses, more filled pauses, slow-down in speech). Also, allowing more pre-task planning time leads to an increase in utterance fluency (Yuan and Ellis, 2003).
Whether investigating the relationship between utterance fluency and cognitive fluency or investigating the relationship between utterance fluency and perceived fluency, the notion of utterance fluency should be the most straightforward to measure as it is the only tangible aspect of fluency. Within utterance fluency, three main types of fluency have been proposed and are usually measured. First of all, speed fluency refers to the pace at which a person speaks and can be measured by number of syllables per second. Breakdown fluency is the number of times speech gets interrupted when speech “breaks down.” These breakdowns can be silent pauses or filled pauses (such as “uhm”). Fewer and shorter breakdowns mean more fluent communication compared to many and long breakdowns. Repair fluency deals with the number of repetitions and self-repairs where fewer repetitions and repairs mean more fluent communication. Like speed fluency, the measures for breakdown and repair fluency are usually normalized for (spoken) time, to allow comparison between speaking performances of different lengths.
Structure of sign languages
A widely held misconception of sign languages is that they are universal. Sign languages share some features arising from their common visual-spatial modality, and they also appear to share some features of gestural communication (e.g., Emmorey and Herzig, 2003). However, sign languages are distinct from each other, as cross-sign language studies have shown (Zeshan, 2004a,2004b; Zeshan and Perniss, 2008). There is even evidence of regional variation within some sign languages, for example, the sign language under investigation: Swiss German Sign Language (Deutschschweizerische Gebärdensprache, DSGS). DSGS has no standardized form but is composed of five regional dialects (Boyes Braem, 1984).
The structure of the lexicon of sign languages is different than for spoken languages (e.g., Johnston and Schembri, 2007; König et al., 2012). The lexicon is split into a native and a non-native sign language lexicon. Only the native lexicon is relevant for the purpose of this study. The native lexicon consists of two subcategories, the conventional and the productive lexicon. The conventional (or, established) lexicon is made up of signs that show a stable form-meaning relationship; for example, the German Sign Language sign for AUTO (“car”) can be used in different contexts without any change in meaning (König et al., 2012) [AUTO is an example of a sign language gloss, a label reflecting a principal aspect of the meaning of a sign (Ebling, 2016). Glosses are typically written in all caps. In this paper, the German glosses for DSGS signs are complemented with the English meaning in parentheses.]. Sign forms that are part of the productive lexicon are produced and understood in a specific context to convey a specific meaning. The signs themselves are not conventionalized, but their sub-lexical units are. The sub-lexical units of productive signs are combined in a specific context to convey, for example, the meaning of “a person is passing by.” To represent the concept of “person,” the signer needs to select a specific handshape (often a single upright index finger) and the location, movement, and orientation of the hand, then transmit the meaning of how and from where the person is passing by and with what kind of path (straight, wavy, etc.) (Johnston and Schembri, 2007).
The articulators in sign languages have been divided into two distinct categories: manual and non-manual components (Boyes Braem, 1995). The manual components are the hands and the arms. Non-manual components include, for example, mouth, cheeks, eyes, eyebrows, eye gaze and positions and movements of the head and the upper torso (Boyes Braem, 1995; Sutton-Spence and Woll, 1999). Manual and non-manual components are usually produced simultaneously. Non-manual components convey relevant linguistic information, for example, differentiating a statement from a question by means of different head positions and raised eyebrows (Boyes Braem, 1995). It is known from studies on adult learners of sign language as L2 that the coordination of manual and non-manual components poses a challenge in their acquisition process (e.g., Woll, 2013).
Prosody in sign languages
Sign language prosody is realized by modifying the manual and non-manual components of signs on the lexical and sentence level (Brentari et al., 2018). Such markers of prosody in sign languages include, for example, a longer movement of a sign, or the final hold of a sign (i.e., pausing; Wilbur and Malaia, 2018) together with non-manual activities, such as eye gaze, eyebrow movements, movement of the head or upper torso. The co-occurrence of manual and non-manual components in sign language prosody support the differentiation between meaning and structure, e.g., raised eyebrows differentiate between a statement and a question (Brentari, 1998). Non-manual markers are used to signals prosodic boundaries between sentences (Sandler, 2012), sentence types (Wilbur, 2000), and are used to structure larger units of discourses (Sandler, 2012).
Sign language fluency is related to intonation and prosody in sign language. The use of complex prosodic markers might requires a high level of proficiency in both prosody and fluency (Kanto and Haapanen, 2020). However, “[t]he connections between prosodic, foreign accent and fluency features in sign language demand further study.” (Kanto and Haapanen, 2020, p. 101).
Fluency in sign languages
The available research on fluency in sign languages is rather sparse. Even though some of the aspects of fluency in spoken languages (e.g., pauses, speed of speaking) can also be found in sign languages, others are modality-specific, such as the simultaneous coordination of manual and non-manual activities. In one of the first studies on fluency in a sign language, pauses and signing speed were some of the aspects that were also reported for American Sign Language (ASL; Lupton, 1998). Some studies investigated the difference between deaf L1 and hearing L2 users of a sign language, for example, utterance fluency in Finnish Sign Language (FinSL; Sipronen and Kanto, 2022). Even though the results show a lot of variation within and between groups (L1: n = 5; L2: n = 5), the authors conclude that L1 users of FinSL produce more signs (i.e., speed of signing) and have fewer and shorter breakdowns (i.e., number and length of pauses) than L2 users. A difference in signing speed between deaf L1 and hearing L2 learners of a sign language has also been reported in studies for ASL and FinSL (ASL: Cull, 2014; Hilger, 2013; FinSL: Sipronen, 2018). Differences in signing speed were also observed within deaf sign language users who acquired French Belgium Sign Language (Langue des signes de Belgique francophone, LSFB) at different stages of their life (Notarrigo, 2017).
A closer look at different types of pauses—as has been suggested for spoken languages (i.e., filled, unfilled)—shows a different pattern in sign language. Notarrigo and Meurant (2014) argue that the concept of “unfilled pauses” does not hold for LSFB since even when no manual activities can be observed, there always will be activities in the non-manual channel (e.g., eye gaze, movements of the head). As for manual components during unfilled pauses, Sipronen (2018) observed in her FinSL data that signers remove their hands from signing space (i.e., moved the hands down). The concept of “filled pauses” has been confirmed in a study on hesitation markers in Sign Language of the Netherlands (Nederlandse Gebarentaal, NGT; Spijker and Oomen, 2023). Filled pauses can be realized by using the PALM-UP non-lexical sign/gesture or finger wiggling. PALM-UP is a one- or two-handed non-lexical sign which is placed in neutral signing space, palms facing up. Pauses can occur at the beginning, the end, or between signs (Notarrigo, 2017). The existence of filled pauses like PALM-UP have also been reported for ASL (Emmorey, 2002).
There also seems to be a consistent use of non-manual components during the production of pauses and potential (dis)fluency markers like the PALM-UP in LSFB (Notarrigo and Meurant, 2014). This result for LSFB shows the importance of coordinating manual and non-manual activities during (dis)fluent signing. The co-occurrence of non-manual behavior (in this case: a change in eye-gaze) with manual hesitation markers has also been reported in a study for NGT (Spijker and Oomen, 2023). The authors of the NGT study also found a difference in the use of manual hesitation markers in monologs and dialogs: PALM-UP is used most often as a hesitation marker in dialogs, and holds (i.e., no motion of the hands) in monologs.
Notarrigo (2017) identified in her research further aspects of fluency, like repetitions, the use of an index directed toward an unspecific location as markers of fluency in sign language. In a different study on LSFB also reformulations were observed (Notarrigo and Meurant, 2022).
The reviewed studies on spoken and sign language fluency will partly inform the development of the fluency rating scale for DSGS. In relation to the study at hand, we would expect to see (also) performance differences across the three proficiency groups of sign language users (i.e., deaf native signers, hearing sign language interpreters, and beginning learners of DSGS), for example, regarding number or duration of produced pauses.
Assessment of fluency in sign languages
To the best of the authors’ knowledge, no specific fluency measure for sign languages exists. There are, however, instruments that assess fluency as part of a larger sign language proficiency construct, for example, the Sign Language Proficiency Interview (SLPI; Newell et al., 1983). The SLPI is an adaptation of the Oral Proficiency Interview for English and was originally developed to assess proficiency of ASL in hearing faculty and staff at the Rochester Institute of Technology, USA. Signed productions are analyzed according to their form and function at eleven different proficiency levels ranging from “No Functional Skills” to “Superior Plus.” “Fluency” is represented in the criterion “Production and Fluency” as a sub-construct to assess if the signing is at normal rate with appropriate pausing (Caccamise and Newell, 1999). An additional description of this criterion ranges from “native/near-native” to “very slow.” No further information is available.
Approaches to rating scale development
Different approaches to rating scale development have been proposed in the literature (for an overview, see Knoch et al., 2021). Fulcher (2003) compares two contrasting approaches to rating scale development: (1) the measurement-driven (e.g., expert opinions’) or (2) performance-driven approaches (e.g., performances of learners). One possible measurement-driven approach is, for example, the “a priori” method. Here, individuals or a committee who are experts in language teaching and assessment are consulted for scale development. The bases are often existing descriptors of previous rating scales. This approach is often used in proficiency testing and allows the testing of general communicative language ability (Fulcher et al., 2011). The disadvantage of this “a priori” method is that it does not describe language development (e.g., Montee and Malone, 2013). In contrast, performance-driven approaches rely on actual speaking performances, which need to be transcribed. Performance features are then identified that will inform the actual descriptors or criteria of the rating scale (Fulcher et al., 2011). Different levels based on the identified features can be established by using discriminant analysis, and each level has a different set of descriptors that go back to the primary analysis of the speaking performances. The disadvantage of this approach is that it cannot be generalized outside a specific language testing context (Fulcher et al., 2011).
Montee and Malone (2013) identified four main approaches to rating scale development: (1) “a priori” scales, (2) theoretically-based scales, (3) empirical-derived scales, (4) and learning-goal or syllabus-derived scale development. (1) The “a priori” scale approach reflects the same method as presented by Fulcher et al. (2011) as an example for measurement-driven approaches. (2) Scales that are derived or informed by theories of language acquisition to reflect language learning progression. This approach should reflect current knowledge of language acquisition. (3) Empirical-derived scales are comparable to the example from Fulcher et al. (2011) on performance-based rating scale development. (4) Scales that are motived or informed by learning-goals or a language syllabus. This approach is good for achievement testing, but not for large scale testing. Whereas Fulcher (2003) argues more for a dichotomous approach of rating scale development (i.e., measurement- vs. performance-driven), Montee and Malone (2013) go one step further and argue for “hybrid” approaches, that is, a combination of different approaches.
Knoch et al. (2021) investigated in a systematic review of the literature if the dichotomy of measurement-driven vs. performance-based approaches is held in real-world rating scale construction. For this purpose, the authors reviewed 36 peer-reviewed articles. One of their findings is that 32 of the 36 studies used 3 or more sources (up to 7) for rating scale development. Of these 36 studies, 35 drew from performance data, 34 on the involvement of raters, and 19 on a review of the literature, for example, on a theory of language. Knoch et al. (2021) conclude that “real-world rating scale development typically relies on a variation of sources” (p. 618). The authors propose a model of test-external and test-internal sources that influence the rating scale construct. Test-external sources include (1) theory/literature review, (2) expert intuition, (3) language proficiency frameworks, (4) target language use domain analysis, (5) existing scales, and (6) curriculum/syllabus. Test-internal sources include (1) performance samples, (2) rater background, (3) rater feedback and (4) rater performance data, and (5) assessment tasks.
For the purpose of the current study, the development of a fluency rating scale for DSGS, we also drew from different sources during the rating scale development (both test-external and test-internal): (1) the review of the literature on spoken and sign language fluency, (2) expert intuitions (i.e., a focus group interview with sign language teachers, feedback from annotators), (3) performance samples (i.e., analysis of signing tasks), (4) and raters’ feedback. With these different sources we build a rating scale which may be adapted and used in future DSGS assessments. The objective of the current study is to answer the question of how to characterize a valid fluency rating scale for DSGS.
There are a number of studies that report on different methodologies to establish validity evidence on sign language tests. Examples are the American Sign Language Sentence Reproduction Test (Hauser et al., 2008) and the Sign Repetition Test for Swedish Sign Language (Holmström et al., 2023). For the purpose of our study, we refer to the Standards for Educational and Psychological Testing [American Educational Research Association (AERA), American Psychological Association (APA), and National Council on Measurement in Education (NCME), 2014, henceforth the Standards].
According to the Standards, validity refers to the degree to which evidence and theory support the interpretations of test scores for proposed uses (p. 11). Therefore, to develop a valid test, test developers must begin by stating the proposed interpretation of the test scores. This includes specifying the construct the test is intended to measure. As the current study does not develop a test, we will not validate interpretations of test scores. However, to reach the objective of characterizing a valid rating scale, we do seek sources of evidence to operationalize the construct of fluency. These sources of evidence can be linked to the sources of evidence as stated in the Standards.
Research question
The review of the literature on spoken and sign language fluency and the different approaches to rating scale development have informed the following main research question:
What are the characteristics of a valid rating scale for Swiss German Sign Language fluency?
In order to address this research question, we will draw on input from theory and different kinds of empirical data.
Materials and methods
A mixed-method approach (e.g., Jang et al., 2014) was applied. The different approaches will be addressed in this section.
This section consists of six main parts: (1) Description of the different study participant groups (sign language teachers for the focus group, DSGS users/learners of signing tasks, raters of DSGS performances), (2) the development of instruments (metadata questionnaire) and materials (focus group protocol, signing tasks), (3) methods for data processing and analysis (i.e., analysis of the focus group interview, creation of annotation schema, transcription and annotation of signing tasks, statistical analysis of annotated data), and (4) development and use of the fluency rating scale (version 1 and 2), (5) statistical analysis of rated DSGS performances, and (6) statistical analysis of annotated and rated data together.
Participant groups
For the purpose of this study, we recruited (1) sign language teachers for the focus group interview, (2) deaf and hearing DSGS users with different proficiency levels to complete the signing tasks, and (3) raters to assess the DSGS performances of the signing tasks. Prior to the study, ethical approval was obtained from the first author’s university. All study participants received information about the goal of the project and signed an informed consent form, both of which were available in written German and DSGS.
Sign language teachers for focus group interview
Three deaf sign language teachers participated in the focus group interview. Two of them were female, one was male. They were 46, 47, and 78 years old. All three consider themselves culturally deaf and belong to the Deaf1 community. Two have at least one deaf parent, one has hearing parents. Two of them have previously worked as sign language teachers and the other still does. Two teachers indicated that they use DSGS as their preferred means of communication in everyday life (One did not provide any information, but it can be assumed that s/he also used DSGS in everyday life since working as a sign language teacher requires a high level of DSGS proficiency, cultural knowledge, and interaction within the Deaf community). The focus group was moderated by a deaf researcher.
Sign language users completing the signing tasks
The group of sign language users completing the tasks (N = 28) was made up of three different sub-groups who differed in regard to their DSGS proficiency/experience: (1) deaf native signers of DSGS (L1 DSGS; n = 8); (2) advanced hearing users of DSGS as L2 (in this case: sign language interpreters; L2 SLI; n = 9), and (3) beginning hearing learners of DSGS as L2 (at CEFR levels A1 and A2; L2 A1/A2; n = 11). Of these 28 study participants, 2 were male, 25 female, and 1 identified as diverse. Their age ranged between 20 and 61 years (M = 40.53, SD = 11.84) (see also Supplementary Table 1).
All L1 DSGS participants (n = 8) were between 20 and 48 years old (M = 33.25, SD = 8.01). They had deaf parents and the majority (7 out of 8) reported having used DSGS (or another sign language) with their family at home (one participant started to learn DSGS at age 10). All L1 DSGS participants used DSGS as their primary means of communication in everyday life. They defined themselves as culturally deaf and were members of the Deaf community. Asked about their own DSGS competence on an 11-point standardized self-assessment scale for language proficiency (LEAP-Q; Marian et al., 2007) study participants placed themselves between 9 and 10 for DSGS comprehension (where 0 = no proficiency and 10 = perfect proficiency) (M = 9.87, SD = 0.35) and between 7 and 10 for DSGS production (M = 8.87, SD = 1.12).
The L2 SLI participants (n = 9) were all trained sign language interpreters (SLI). They were between 28 and 61 years old (M = 40.89, SD = 11.34) and had been using DSGS for between 7 and 28 years (M = 17.67, SD = 7.40). They rated their DSGS comprehension skills between 4 and 8 (M = 7.22, SD = 1.3) and their DSGS productive skills between 3 and 8 (M = 6.78, SD = 1.56) on the LEAP-Q. All were currently working as sign language interpreters.
The L2 A1/A2 users (n = 11) were between 23 and 61 years old (M = 45.54, SD = 12.68). They had been learning DSGS for 2 to 9 years (M = 3.9, SD = 2.38). They rated their DSGS comprehension skills between 2 and 5 (M = 3.18, SD = 0.98) and their DSGS productive skills between 1 and 5 (M = 3.0, SD = 1.26) on the LEAP-Q. The information provided about study participants’ DSGS course work is incomplete. But since we only asked for study participants attending in-person A1 and A2 level courses, those who provided no—or only limited—information can be assumed to be at CEFR level A1 or A2.
All 28 participants were recruited through personal and professional networks of the research team. They were reimbursed for their travel expenses to the HfH in Zurich and received 50.- Swiss Francs as compensation.
Raters of signed performances
All three deaf raters were female and 36, 41, and 42 years old. They were trained sign language teachers who had obtained their qualification between 2009 and 2022 and were currently working in this profession. The same three raters were involved in rating DSGS performances with version 1 and version 2 of the fluency rating scale.
Instruments and materials development
Metadata questionnaire
For the purpose of the study, a metadata questionnaire was developed. It was filled out by all study participants. The questionnaire was provided online in LimeSurvey.2 The questionnaire items were available in written German and DSGS. The survey consisted of nine parts. Parts 1 and 2 included items on the background information of the participants, such as their year of birth, gender, where they grew up and have lived, their cultural and audiological hearing status, and possible use of hearing aids or cochlear implants. Part 3 asked about the hearing status of and language use with deaf and hearing family members. Parts 4 and 5 included questions about participants’ schooling and professional education, including questions about their current position/work experience. Parts 6, 7, and 9 focused on participants’ language skills with a particular focus on their DSGS and German skills. Part 8 focused on where (hearing) participants had learned DSGS.
Focus group protocol
Focus groups are a qualitative research method which is also used within applied linguistics (Dörnyei, 2007) with the goal to bring together a group of people sharing a similar background (as in our case: sign language teachers) to discuss a specific topic, exchange experiences, provide feedback, or share ideas on a topic specified by a researcher (Ho, 2013; Krueger and Casey, 2015).
The goal of the focus group in this study was to learn from the intuitions of experts what characteristics of signing can be indicators of sign language fluency to inform the rating scale development. For this purpose, a focus group protocol was developed. The focus group was moderated by a deaf researcher. The protocol of the focus group defined three parts. In Part 1, members of the focus group discussed, and named and categorized, what the possible aspects of fluency in DSGS could be. In Part 2, members received a short input on different aspects of fluency (based on theory for spoken languages, e.g., pausing, repetitions, speed of signing) by the moderator and had to match it with the results of Part 1. In Part 3, participants saw some performances of DSGS users from the signing tasks (see section “Signing tasks”) and applied the different aspects from Part 1 and 2 to the performances. The focus group was video-recorded for later analysis.
Signing tasks
In order to investigate the construct of fluency in DSGS, tasks parallel to those developed at Leiden University for Dutch L2 learners of spoken English were developed for the current study. The 12 tasks were manipulated by task complexity (simple, complex) and preparation time (with and without). First, the study participants had to complete six tasks with no preparation time: a simple story-telling task, a complex story-telling task, a simple arrangement task, a complex arrangement task, and a set of two tasks which started with a simple part (comparison) followed by a more complex part (reasoning). Following these, six similar tasks with preparation time were completed. The signing tasks were embedded in a PowerPoint presentation. All instructions were either available in written German (L2 SLI and L2 A1/A2 groups) or in DSGS (L1 DSGS group). Four different versions of the tasks with (1) storytelling, (2) tasks with seating plan/arrangement were created, and (3) two different sets of tasks for comparing and reasoning were created. Thus, we could create four versions of the PowerPoint to make sure that participants never repeated the same versions of the storytelling, arrangement, and compare and reasoning tasks (Supplementary Table 2).
Each version of the signing tasks was administered in the same way: First, study participants saw or read a general instruction about the signing tasks, followed by the instructions to introduce themselves in DSGS, before the actual tasks began. The participants saw instruction on a slide in German or DSGS and on the next slide(s) was the actual task (pictures, sometimes with short texts). For the first six tasks, they received only a short time to look at the pictures before they should start signing (without preparation). In the final six tasks, they had two minutes preparation time for each task. Figure 1 shows an example of a simple arrangement task without preparation time (Task 3, Sub-Type 1, Version A). The signing tasks were piloted with two deaf L1 signers and two hearing users of DSGS. The results of the pilot led to revisions in the task instructions and the layout of the pictures of the tasks. The pilot data was not included in the analysis.
Individual sessions were scheduled for each participant. Each session lasted approximately 20–30 min and took place at the HfH in Zurich. Each session was video-recorded for later transcription and annotation. The sessions with the deaf participants were moderated by a deaf researcher, the sessions with the hearing participants by a hearing researcher. These transcribed and annotated data informed the development of the first and second version of the fluency rating scale. Due to financial constraints, only 6 of the 12 tasks could be annotated across all study participants.
Data processing and analysis of focus group and signing tasks
Analysis of focus group interview
The focus group discussion was video-recorded from three different angles and afterward translated into spoken German by a sign language interpreter. The translation (audio file) was automatically transcribed by the commercial speech-to-text provider Amberscript3 and later post-edited (i.e., double-checking of the text files with the audio file) by a Bachelor student of the sign language interpreting program at the HfH. The clean transcripts were then analyzed using ATLAS.ti,4 a commercial software for the analysis of qualitative research data. Data was only processed and stored locally. We applied the so called “scissor-and-sort” technique (Stewart and Shamdasani, 2014). Applying this method did not require the entire transcript to be coded, but only selected sections that were relevant to the research purpose (Stewart and Shamdasani, 2014), i.e., aspects of fluency to inform the development of the rating scale. The step of selecting relevant sections in the transcript was done by the first author.
In the next step, coding categories were developed as the basis for the analysis of the transcripts. The first version of the coding categories was informed by themes that occurred during the selections of the sections in the transcript. Another Bachelor student of the sign language interpreter program at the HfH, who already was experienced in coding interviews, applied the coding categories to the transcript. To familiarize her-/himself with the software and the process of coding, the student received a different transcript (i.e., memory log of a rater with feedback to the first version of the rating scale). Then s/he started with the coding of the focus group transcript. During the process of coding the focus group transcript the student had regular exchanges with the first author (i.e., in person and by email) to solve issues in the application of the codes. In this process, the coding categories were revised (e.g., sub-codes were removed in favor of main codes only, new codes were added, old codes were removed; the wording of the codes was not changed). The process of coding “may require several passes through the transcript as categories of topics evolve” (Stewart and Shamdasani, 2014, p. 124). The student applied version 2 of the coding categories again to the focus group transcript. The final version of the document with the coding categories included 11 categories (version 1: 12), for example, pauses, repetitions, use of non-manual components, signing speed, self-corrections, rhythm of signing, and different categories related to fingerspelling. The coding categories can be found in Table 1.
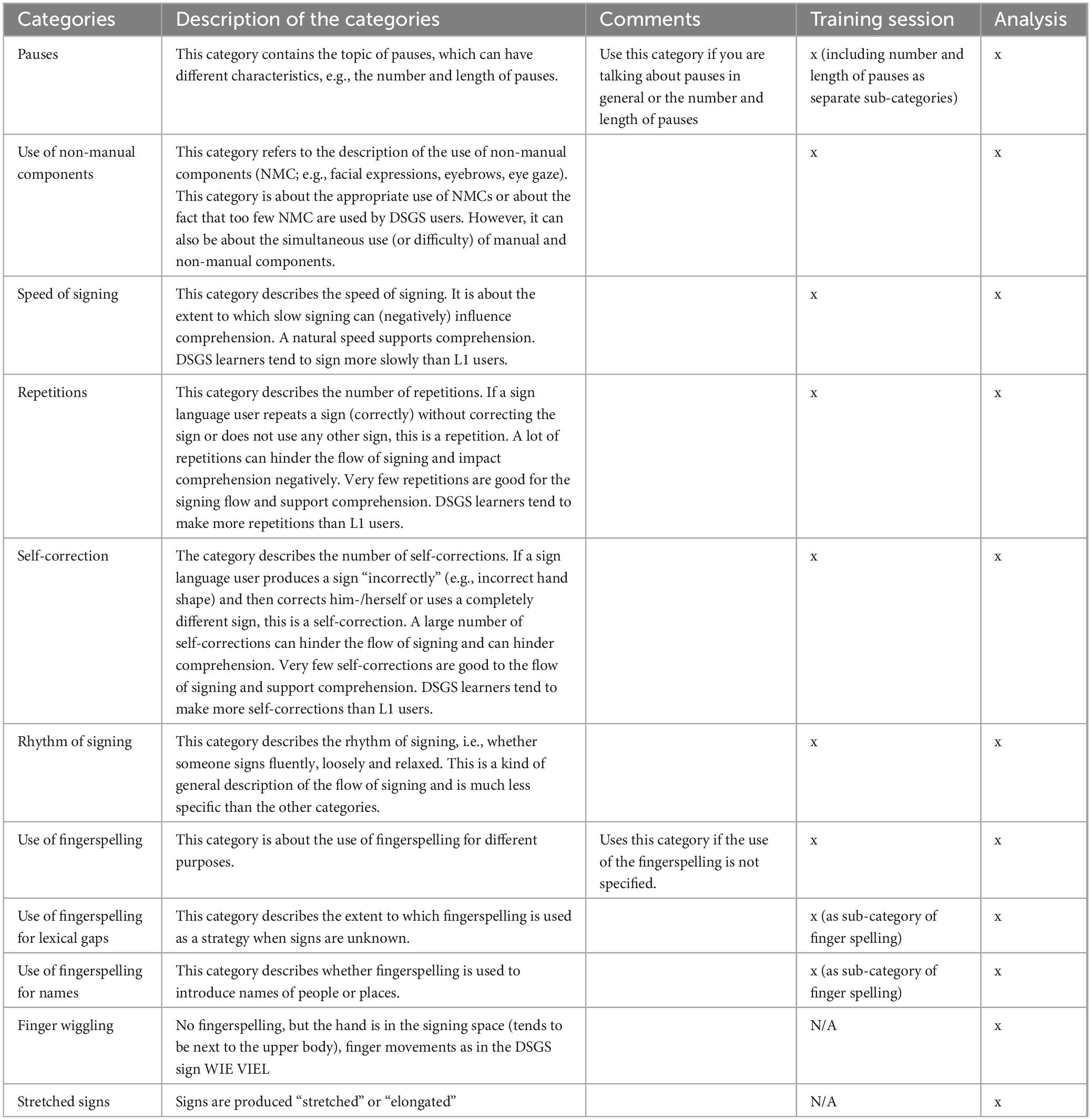
Table 1. Overview of coding categories applied to the focus group transcript (training session and analysis).
Transcription and annotations of the signing tasks
In the first stage of the DSGS fluency project, we adopted the schema and the process for transcribing and annotating continuous sign language data developed by Battisti et al. (2024). The schema encompasses the identification and labeling of the manual and non-manual components co-occurring at the sign and sentence levels (see Structure of sign languages). Each of these components corresponds to a tier within an annotation transcript in iLex, a software for sign language research developed by the University of Hamburg (Hanke and Storz, 2008). For the purpose of our study, we enhanced this schema by incorporating elements relevant to sign language fluency, such as pauses.
The process included the transcription and annotation of the data in iLex (Figure 2). In the transcription step, the video data were segmented and tokenized; while in the annotation step, the data were enriched with information (e.g., Konrad, 2011). For the remainder of this paper, the term “annotation” will be used to refer to both concepts.
Among the manual components, glosses were segmented and labeled as either productive or lexical signs (see Structure of sign languages). In the gloss tier, we identified specific manual markers—as informed by theory from sign language fluency—that we labeled as “manual markers of hesitations,” including non-lexical signs/gestures, for example, PALM-UP, finger wiggling, and holds (see Fluency in sign languages).
With regard to non-manual components, we were interested in the use of mouth actions (mouthing and mouth gestures), eyebrow movement, head movement, and gaze movement. In a specific tier, four categories of hesitation were annotated: (1) pauses, (2) stretched signs, (3) self-corrections, and (4) sign repetitions.
Due to financial constraints, it was not possible to annotate all 12 tasks from all 28 participants. In order to make an informed decision how to reduce the number of tasks that should be annotated, the spoken Dutch data were analyzed. 20 Dutch learners of English at A2 level performed the twelve tasks (Naber, 2021) which were analyzed automatically for fluency through a PRAAT (Boersma and Weenink, 2016) script (De Jong et al., 2021). The results revealed that if there were differences between simple and complex tasks, these were in the opposite direction from hypothesized. Likely, an order effect led to the result that the complex tasks were sometimes carried out with more fluency compared to the simple matched counterpart-tasks. As hypothesized, however, planning time did lead to more fluent spoken performances. Therefore, only the six simple tasks under the “with and without preparation time” conditions were annotated. An additional measure to reduce the amount of annotation time was to transcribe only the first 15 and the middle 15 s of the signed performances. In the middle of the task, signers are likely to perform at their level of proficiency. Additionally, by including the initial 15 s, we maximize the potential effect of preparation, as the start of the performance includes initial conceptualization of what to say. In total 82.86 min of DSGS videos were annotated.
Four deaf sign language teachers with experience in conducting research (n = 3) and annotating DSGS data in iLex (n = 2) annotated the DSGS video data. The annotators had an initial training and then annotated the data on their own. They established regular exchanges to clarify annotation issues in DSGS using Glide,5 a video chat app. Since it was not possible to introduce a four-eye principle for some of the annotations to investigate inter-annotator reliability, the four annotators established conventions within the group and solved disagreements through their regular exchange in the Glide group. The conventions were documented in written form and made accessible to all annotators.
Statistical analysis of annotated DSGS data (signing tasks)
For the statistical analyses of the annotated data and the rated DSGS performances, we will refer to the numbering of the ten versions of the signing tasks as introduced in the section “Signing tasks” (see Supplementary Table 3).
In our exploratory analysis, we investigated speed of signing, occurrences of hesitation categories, duration of pauses, and co-occurrence of manual and non-manual components to identify features in DSGS fluency that differ across three levels of language background (see Sign language users completing the signing tasks) and across two levels of preparation time (without and with).
The hesitation categories in this analysis included pauses, stretched signs, sign repetitions, and self-corrections, categorized into three language groups. Sign repetitions and self-corrections were grouped due to their low frequency in the dataset.
Here, we describe only the final models that contributed to the development of the sign language fluency rating scale. All models were fitted utilizing the lme4 package in R (Bates et al., 2015), with language background and preparation time included as fixed effects. To account for within-subject variability, the models incorporated random intercepts for participants and tasks. We did not include random slopes for the fixed effects by-subject or by-item, as these did not yield significant results.
Firstly, we fitted a linear mixed-effect model (LMM) with the speed of signing as the outcome, defined as the log of the total signing time without pauses (first hesitation category) divided by the total number of glosses in the annotated data.
Secondly, we assessed the influence of the fixed effects on each hesitation category by examining the count of hesitation categories divided by the annotated seconds (excluding the length of hesitations) to adjust for varying signing timespans using an LMM.
For the first hesitation category, pauses, we additionally investigated differences in pause duration among language groups and across preparation time conditions. Pause duration was defined as the total pausing time divided by the total number of pauses. We constructed an LMM, again considering language background and preparation time as fixed effects without an interaction term and including random intercepts for participants and tasks.
Lastly, we investigated the occurrence of non-manual markers, such as mouth gestures and gaze, eyebrow, and head movements during instances of hesitations. For each non-manual marker, we fitted a generalized linear mixed-effects model (GLMM) specifying a binomial family due to the binary nature of the response variable (presence or absence of the non-manual component). In each model, as fixed effects, in addition to language background and preparation time, we included the hesitation category to analyze its impact on the non-manual component. Only participants were included as random effects to accommodate individual differences.
Development of the DSGS fluency rating scales
Two versions of the DSGS fluency rating scale were developed. First, the development of version 1 will be presented, followed by the revision that resulted in version 2 of the fluency rating scale.
DSGS fluency rating scale: version 1
Version 1 was informed by (1) by theory from spoken and sign language fluency research, (2) a preliminary analysis of the focus group interview (the analysis of the focus group transcript as described in this paper was only used for version 2 of the rating scale), and (3) informal feedback from the annotators. Version 1 consisted of ten criteria. All criteria were rated on a 6-point scale, but only the extreme points were described (e.g., 1 = very rare; 6 = very often). The scale’s wordings were different across all criteria (the first seven criteria addressed frequency, while, for example, the criteria for “speed of signing” used 1 = too slow and 6 = too fast, see Supplementary Image 1). Important to mention is that the scales for the different criteria were not in the same order. That means, “1” did not always mean that it was positive or negative for sign language fluency, i.e., the order of the scales was mixed across all criteria. The rating scale was implemented in Excel.
Version 1 was used to rate the performances of the L2 A1/A2 learners (a total of 53 performances, each approximately 30 s long, i.e., the 15 annotated seconds in the beginning and the middle of the performances). The three raters were trained by one of the deaf researchers. The training was conducted onsite at the first author’s university. Raters were trained individually. They first received input on the scale and definitions of the criteria (also available in DSGS online), then they rated a few performances and discussed uncertainties with the deaf trainer, and provided some feedback on the rating scale which was documented by the deaf trainer. After the initial training, the raters received a document with links to a secure server with the DSGS performances (videos) and the individual online rating scales. All raters judged all 53 performances. They had six weeks to complete the rating. Part of the results of version 1 informed the revision of the scale. Version 1 of the rating scale will be presented in the “Results” section (feedback from the raters).
DSGS fluency rating scale: version 2
Based on (1) theory from spoken and sign language fluency (as in version 1), (2) feedback from the raters on the use of rating scale version 1, (3) the analyzed transcripts from the focus group, and (4) the statistical analysis of the annotated data, version 1 of the fluency rating scale was revised and resulted in version 2. Version 2 was used to rate the six performances of all the participants who participated in the signing tasks (N = 28), that is, the L1 DSGS, L2 SLI, and L2 A1/A2 groups. In six cases, only five DSGS performances were available. All DSGS performances were rated by all raters (162 performances). The same three raters were trained together in an online session by a deaf trainer. Similar to the rating of the performances with version 1, raters received a document with links to a secure server to the videos and the individual scoring sheets online (in Microsoft Excel). They had two months to complete the rating task and had the possibility to get in touch with the trainer of the rating scale. Version 2 of the rating scale will be presented in the “Results” section.
Analysis of rated performances with version 2
We analyzed the rater and DSGS performance data for version 2 of the rating scale, as well as the rating scale structure, with many-facets Rasch measurement (MFRM; Linacre, 1994) using the software package Facets (Linacre, 2023). A 6-facet model was specified:
Rater: The three raters.
Signer: The 28 signers (participants completing the signing tasks).
Language background: The three different language backgrounds of the signers (L1 DSGS, n = 8; L2 SLI, n = 9; and L2 A1/A2; n = 11). This facet was dummied (i.e., all elements were anchored at 0 logits) as the signer’s language was nested within the signers. The facet was included to study differences between the three signer groups.
Task: The 10 tasks (i.e., four versions of the simple story task, four versions of the simple arrangement task, and two versions of the compare/reasoning task, see Supplementary Table 2).
Preparation: The two different conditions for the administration of the tasks (with and without preparation time). This facet was dummied (i.e., both elements were anchored at 0 logits) as the preparation condition was nested within the tasks. The facet was included to study differences between the two preparation conditions.
Criterion: The six rating criteria of the rating scale.
We first ran the analysis with all the data to study model fit, rater reliability, language background interactions, effects of preparation time, and overall rating scale structure. In a second step, we ran the analysis separately for each rating criterion (including only the data for the respective criterion each time) to study the criteria’s individual scale structures.
Analysis of annotated data and rated performances with version 2
The goal of this statistical analysis is to see if the aspects of fluency in the annotated data can predict the ratings of the DSGS performances. For this reason, we will concatenate the measures from the annotations in all tasks and correlate aspects of fluency of the annotated concatenated data (objective scores) with their “equivalent” in the rated data (fair averages of the specific criteria, i.e., subjective scores). For example, we correlate the number of pauses per second with the fair average score for the criterion number of pauses. Similarly, we correlate objective and subjective scores for average length of pauses, average sign duration (the inverse of signing speed), repetitions, and self-corrections. We would expect negative correlations since, for example, the lower the number of annotated pauses is, the higher the ratings will be with the criterion “number of pauses” (i.e., max. 6 = fewer pauses). We use transformations of the measures when data are not normally distributed. Additionally, we apply a multiple regression analysis with all objectives scores as independent variables and the overall calculated fluency score of the rated data as the dependent variable. This allows us to gauge the total explained variance of overall rating scores by the objective measures.
Results
In this section, first the results from version 1 of the rating scale will be presented, i.e., (1) raters’ feedback. This is followed by the presentation of additional data analyses, i.e., (2) the focus group interview, (3) the statistical analysis of the annotated data, and (4) of the rated DSGS performances, and (5) how the annotated data can predict the ratings of the DSGS performances. All analyses (1) to (5) have informed version 2 of the rating scale.
Results from the use of version 1 of the DSGS fluency rating scale
Results from raters’ feedback
Raters raised the issue that the 6-point scale was not clear across all criteria, sometimes “1,” “6” or even the “middle” of the scale is an indicator for “appropriate” fluency. For example, it is not necessarily appropriate for fluency when someone signs too fast (i.e., “6” on the scale). This was changed in a revised version of the scale (i.e., “6” is always appropriate for sign language fluency across all criteria). Additionally, the different criteria for pauses were not always clear to the raters, it was hard to separate them conceptually. As a consequence, the number of criteria for pauses in version 2 was reduced from four to three criteria. The raters also mentioned that there was a lack of criteria addressing specific non-manual components (e.g., gaze, eyebrows, or mouth activities). The raters also suggested to make the rating scale visually more attractive. These results were implemented into version 2 of the DSGS fluency rating scale.
Results that informed version 2 of the DSGS fluency rating scale
Results from focus group interview
The goal of the focus group interview was to tap into the experiences of sign language teachers regarding different aspects of fluency. During the focus group interview, participants discussed different aspects of fluency, received input on fluency, and applied the discussed aspects of fluency to actual DSGS performances. The goal of the analysis of the focus group interview was to see which aspects of fluency the sign language teachers discussed. In total, 218 times one of the coding categories was applied throughout the transcript and informed the revision of the rating scale (version 2). Three of the eleven coding categories (all related to fingerspelling) were not applied at all. The frequency of the applied coding categories is presented in Table 2. The use of non-manual components was not included as a separate criterion since manual and non-manual components are (mostly) produced together in sign language production (e.g., Hilger et al., 2015). Consequently, non-manual components were taken together with the production of pauses. Rhythm was not included in version 2 of the rating scale since it is a holistic criterion, whereas the other criteria are analytic in nature. The category “stretched signs” was also not included in version 2 of the rating scale as a separate criterion because it has an overlap with “speed of signing,” that is, producing many stretched signs results in fewer signs/slow signing. Finger wiggling, applied as a separate code to the transcripts, was categorized under pauses. All remaining categories were included into version 2 of the fluency rating scale.
Statistical results from annotated data (signing tasks)
Speed of signing
We first analyzed the speed of signing. Being in the L2 A1/A2 language group significantly increased the average duration of a sign by approximately 1.50 times, or 50%, compared to the L1 DSGS reference group. This effect was significant (p < 0.001). The difference between L2 SLI and DSGS was not significant, nor was the effect of preparation time. The random effects indicated some variability in sign duration across individuals (SD = 0.136), but no variability across different tasks (SD = 0) (Table 3) (All descriptive statistics of the output Tables 3–5 can be found in Supplementary Table 4).
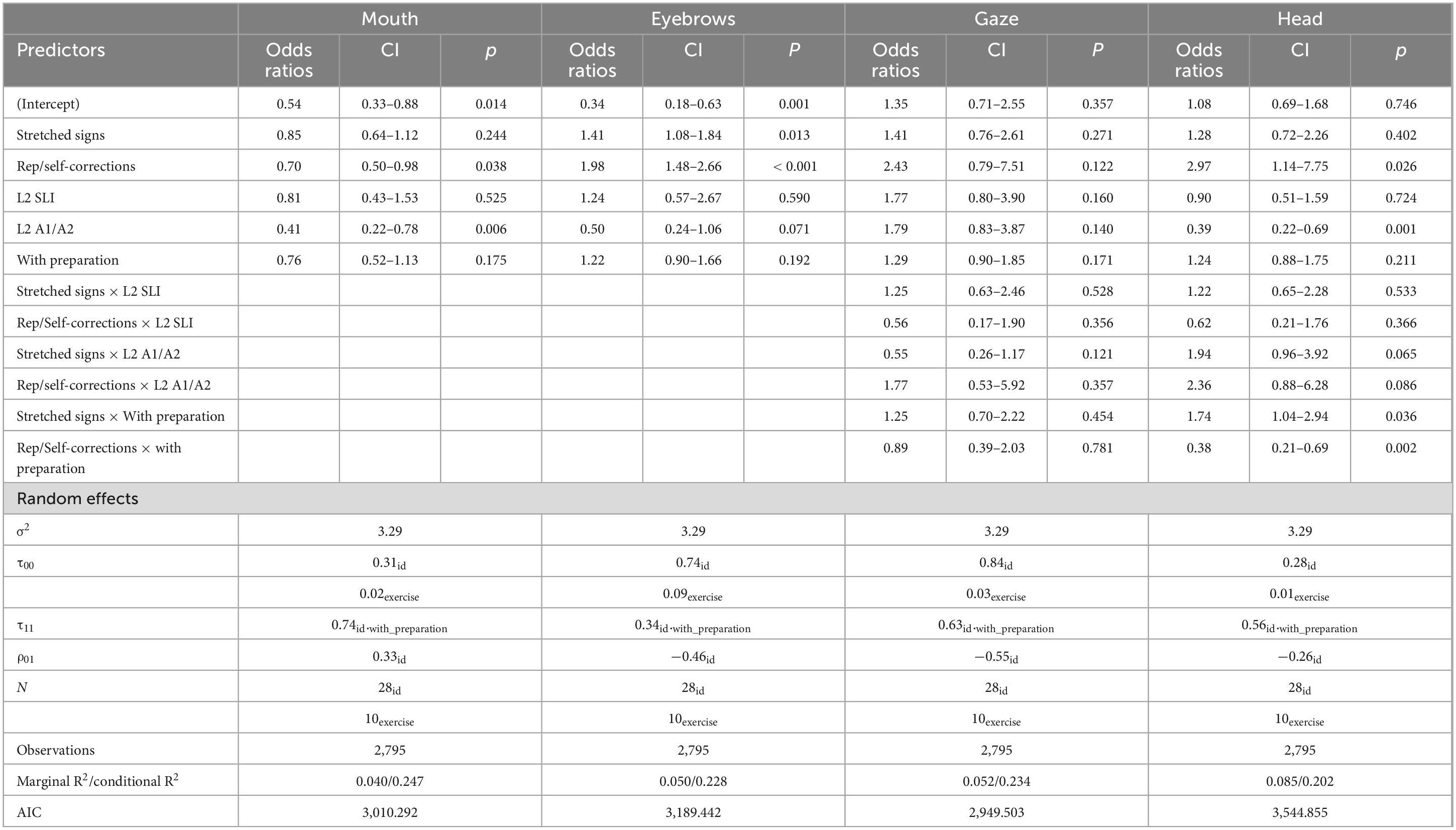
Table 5. Results of the glmer models examining the effect of hesitation types, language backgrounds, preparation time, and their interaction on non-manual components (binary outcomes).
Hesitation categories: pauses, stretched signs, and sign repetitions/self-corrections
To explore the relationship between hesitation categories and language background, we employed LMMs for each hesitation category (see Statistical analysis of annotated DSGS data). Table 4 summarizes the outcomes of these models.
In the first model, which focused on pauses, the dependent variable was the log of the count of pauses divided by the annotated seconds. L2 SLI participants exhibited approximately 1.589 times more pauses per second compared to those in the L1 DSGS group. Similarly, L2 A1/A2 participants showed approximately 2.064 times more pauses per second compared to L1 DSGS participants. Both groups demonstrated statistically significant effects (p = 0.048 and p = 0.002, respectively), indicating that language proficiency significantly influences the number of pauses per second. No significant effect of preparation time was found. Variability in the outcome is partially explained by differences between participants, while no variability was observed between different tasks.
In the second model, the dependent variable was the log of the count of stretched signs divided by the annotated seconds. Neither the L2 SLI nor the L2 A1/A2 language background showed statistically significant effects compared to the L1 DSGS group. Additionally, preparation time did not show a statistically significant effect. The variability in the outcome across participants and tasks underscores individual differences and contextual influences on hesitation behaviors.
In the third model, which focuses on sign repetitions/self-corrections, participants from the L2 A1/A2 group exhibited approximately 2.127 times more sign repetitions and self-corrections compared to L1 DSGS participants (p = 0.040). The comparison between L2 SLI and L1 DSGS was not significant, neither was the effect of preparation time. Random effects analysis revealed a variance of 0.246 between participants, corresponding to a standard deviation of 0.496. In contrast, no variability was observed between different tasks, indicated by zero variance and standard deviation (Supplementary Image 2 shows examples of self-corrections and stretched signs).
Supplementary Image 3 illustrates the effect of language background and preparation time on the three hesitation categories analyzed (i.e., pauses, stretched signs, sign repetitions/self-corrections).
Duration of the pauses
The model investigating pause duration revealed that language background significantly impacted the log duration of pauses, defined as the total pausing time divided by the number of pauses for each task. The L2 A1/A2 group showed a significant increase in pause duration by a factor of approximately 2.231 (p < 0.001), suggesting that pauses were more than twice as long for this group compared to the reference group. The comparison between L2 SLI and L1 DSGS was not significant. Preparation time tended to decrease pause duration by a factor of 0.897, with this effect approaching significance (p = 0.091). The random effects indicated substantial variability in pause duration across individuals and tasks (see Table 3).
Non-manual markers
The relationships between hesitation categories and non-manual components were analyzed using GLMMs for each component: mouth actions, and gaze, eyebrow, and head movement. Each model included fixed effects representing hesitation categories, language background, and preparation time, as well as random effects accounting for individual variability among participants and tasks.
Table 5 reports the results of the selected models. The model analyzing mouth actions indicates that certain factors significantly influenced their likelihood. Specifically, repetitions and self-corrections (hesitation category 3) and L2 A1/A2 were associated with significantly lower odds of mouth actions (OR = 0.70, p = 0.038, and OR = 0.41, p = 0.006, respectively), that is the likelihood or probability of observing mouth actions was lower compared to L1 DSGS group. There were no significant effects for preparation time.
For eyebrow movements, the results indicate that stretched signs and sign repetitions/self-corrections significantly increased the odds of eyebrow movements compared to the reference hesitation category, that is pauses (OR = 1.41, p = 0.013, and OR = 1.98, p < 0.001, respectively). Participants in the L2 A1/A2 group showed a non-significant trend toward reducing the odds of eyebrow movements and preparation time showed a non-significant increase in odds.
For gaze and head movement, the best models included interactions. For gaze movement, none of the predictors, including interactions, were statistically significant, suggesting that hesitation category, language background, and preparation time did not have a clear impact on the occurrence of gaze movement. In contrast, for head movement, L2 A1/A2 significantly decreased the odds (OR = 0.39, p = 0.001). In addition, sign repetitions/self-corrections significantly increased the odds of head movement (OR = 2.97, p = 0.026). Finally, the interactions between stretched signs and preparation time, as well as sign repetitions/self-corrections and preparation time, were significant, indicating complex relationships between these factors and head movement (OR = 1.74, p = 0.036; and OR = 0.38, p = 0.002, respectively).
In summary, in this dataset, participants in the L1 DSGS group (our reference group) generally signed faster and produced fewer pauses compared to both L2 SLI and L2 A1/A2 participants. L2 A1/A2 participants showed more sign repetitions and self-corrections compared to the L1 DSGS participants. Neither language background nor preparation time had statistically significant effects on the production of stretched signs.
L2 A1/A2 participants exhibited longer pauses compared to both L1 DSGS and L2 SLI groups. Preparation time tended to decrease pause duration.
Regarding non-manual components, L1 DSGS participants produced more of these components than L2 SLI and L2 A1/A2 participants. Specifically, they exhibited more mouth actions and head movements than L2 A1/A2 participants. Across all dependent variables, the amount of explained variance by the fixed effects ranged considerably, with marginal R2 as reported in Tables 3–5 between 0.040 and 0.406.
Version 2 of the fluency rating scale
Version 2 of the fluency rating scale was informed by (1) theory from spoken and sign language fluency (as in version 1), (2) feedback from the raters on the rating scale version 1, (3) the analyzed transcripts from the focus group, and (4) the statistical analysis of the annotated data. Supplementary Table 5 provides an overview of the sources (of data) used to develop the six criteria of the fluency rating scale.
Version 2 of the DSGS fluency rating scale consisted of four areas with six criteria: (1) Pauses (three criteria), (2) speed (one criterion), (3) repetition (one criterion), and (4) self-corrections (one criterion). The definition of the criteria can be found in Supplementary Table 6. Figure 3 displays version 2 of the rating scale.
Statistical analysis of rated DSGS performances
MFRM measures and model fit
Table 6 and Figure 4 display a summary of the MFRM model measures. The data fit the model well, with Rasch measures explaining 59.49% of the variance. Fit indices (Infit and Outfit MS) across all facets were close to 1 with small standard deviations. For the facets rater, language background, task, preparation, and criterion, individual fit indices did not exceed 0.5 and 1.5. For the signer facet, four elements (i.e., four signers) displayed Infit MS of 1.52 to 1.75, however we decided not to omit these from the analysis as Infit MS below 2.0 do not degrade the overall measurement (Linacre, 2002). All other signers’ Infit MS fell within 0.5 and 1.5.
Raters could be separated into 14 levels of severity. As shown in Figure 4, this difference was due to Rater 3 being markedly more severe than Rater 1 and Rater 2; however, all three raters showed good levels of intra-rater reliability with Infit MS between 0.80 and 1.13. Signers displayed a separation index of 7.10, which was expected as the three signer groups differed in their DSGS proficiency. The tasks could not be separated into more than 1 level (separation index = 1.96). This was also expected as we only included “simple” tasks in the rating design. Finally, the elements in the criterion facet could be separated into 14 levels, with criterion 1 (number of pauses) receiving the lowest ratings and criterion 6 (self-correction) the highest ratings overall. The reliability of all separation coefficients was high, ranging from 0.79 (for the task facet) to 1.00 (for the criterion facet).
Effects of signer language background and task preparation time
As shown in Figure 5, the L1 DSGS group achieved the highest average rating for most tasks, except for Task 12 and Task 33, where the L2 SLI group slightly outperformed the L1 DSGS group. The L2 A1/A2 group received the lowest average rating for all tasks by some margin. A bias analysis across all 30 pairs (10 tasks times 3 language groups) revealed only two significant results, again for Task 12 and Task 33, both of which showed slight bias against the L1 DSGS group compared to the L2 SLI group (for Task 12; t(154) = 2.05, p = 0.042; with a small effect size, d = 0.39) and the L2 A1/A2 group (for Task 33; t(120) = 2.16, p = 0.033; with a small effect size, d = 0.33). That is, the L1 DSGS group was at a slight disadvantage (statistically) for Task 12 and Task 33. These results indicate that the rating scale in general produced ratings in line with expected language differences and without displaying unfair bias toward any of the language groups (except for Task 12 and Task 33, which should perhaps be revised).
Figure 6 shows the average ratings of all 10 tasks for the two preparation conditions. The average ratings between the two conditions are very similar, with the biggest difference for Task 33 (0.52 scale points; however as described above Task 33 should be revised). A bias analysis for all possible pairs revealed no significant results, indicating that task preparation time did not have an effect on the ratings.
Rating scale structure
MFRM also produces rating scale statistics and probability distributions for the scale categories, which can be used to interpret the functioning of the scale and scale points. Table 7 presents the scale statistics and Figure 7 the probability distributions for the complete data (Overall) and for each criterion separately (Criterion 1–6) for the ratings with version 2 of the rating scale. The Data columns in Table 7 show how often each score category (1–6) was assigned by the raters. The Quality Control columns display the validity of the score categorization, including average and expected measure and Outfit Mean Square for each score category; average measures should increase with each score point. The Rasch-Andrich Thresholds columns report step calibrations of the rating scale structure and their associated standard errors; the step calibrations should be in ascending order for rating scales where higher scores are equivalent to higher abilities (see Linacre, 2023, pp. 222–223 for detailed descriptions of the reported measures). Finally, the probability curves in Figure 7 show the probability for each score in relation to the logit measure; each score should be the most probable (modal) at some point along the logit scale.
Overall, the scale functioned well, with each score category being modal at some point on the continuum (see Overall in Figure 7). However, the average measure of scale category 2 was lower than the average measure of scale category 1 (marked by an asterisk in the Overall column in Table 7), likely because scale category 1 was used very infrequently by the raters (in only 3% of the ratings overall and not at all for Criteria 5 and 6, see Table 7). When inspecting the scale statistics for the individual criteria, additional inconsistencies can be observed. While Criteria 1 and 2 functioned well across all scale categories, Criterion 3 displayed a low average measure for scale category 3, severely disordered Rasch-Andrich thresholds for scale category 4, and low probabilities for scale categories 4 and 5. For Criterion 4, scale category 5 was never the most probable and it also showed a disordered Rasch-Andrich threshold. Finally, criteria 5 and 6 were by far the easiest for the learners to achieve high scores on, with no ratings for scale category 1 and very few ratings for categories 2 and 3, resulting in a low probability for category 3 in Criterion 6.
Results of annotated data and rated performances with version 2
We concatenated per study participant, over all tasks: total duration, total number of pauses, total pause duration, total number of glosses, total number of repetitions, and total number of self-corrections. Additionally, the total number of times pauses were accompanied by the non-manual components brows, head, mouth, and gaze were also summed over all tasks. Next, from these summed timings and counts, we calculated the following measures, in alignment with the criteria in the rating scale: number of pauses per second, average pause duration, average sign duration, number of repetitions per second, and number of self-corrections per second. For use of the four non-manual components, we calculated the percentage of time pauses were accompanied by the non-manuals brows, head, mouth, or gaze. We checked normality using the Shapiro–Wilk test for normality and by visual inspection of the data. After a log transformation on average pause duration and average sign duration, and a square root transformation on pauses per second, these variables could be assumed to be reasonably normally distributed. For self-corrections per second, a rank transformation resulted in a reasonably normal distribution. The variables repetitions per second and non-manual gaze per pause could not be transformed to a reasonably normal distribution and will be omitted from the analyses (see Supplementary Table 7 for all descriptive statistics of this analysis). The strength of a correlation will be evaluated following Plonsky and Oswald’s (2014) proposal: (1) close to 0.25 as small, (2) 0.40 as medium, (3) and 0.60 as strong.
The results of the correlation show that most objectives scores were related to the specific ratings. For non-manual component use, only the measure of head-movements was related to the specific rating (see Table 8). Finally, we predicted the overall fair average score for overall fluency with the independent variables that were reasonably normally distributed. Using the step-function in R (backward selection), except the rank of the number of correlations per second and the non-manual components use of brows and mouth, the variables significantly contributed to the prediction of
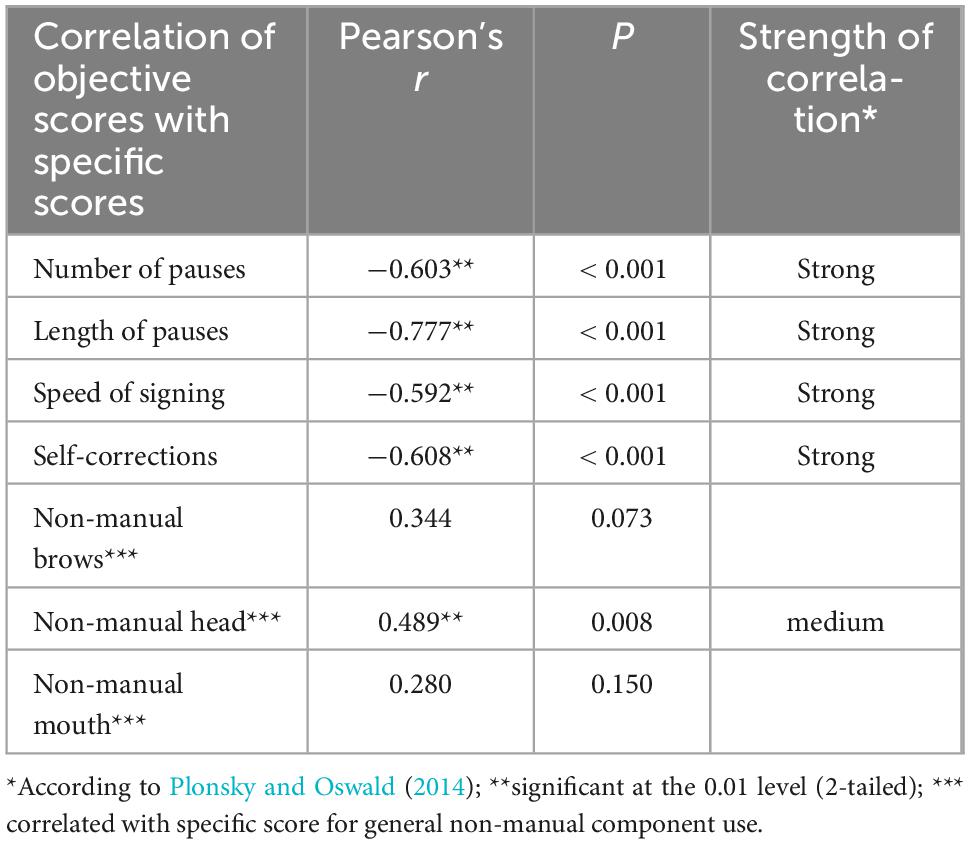
Table 8. Correlations of objective scores (annotated data) with specific scores (rated data) across all study participants (N = 28).
the overall score [F(6, 21) = 24.7, p < 0.001], leading to a total of explained variance of 88% (see Supplementary Table 8).
Discussion
We started out in this paper with the question about the characteristics of a valid fluency rating scale for DSGS. We collected different kinds of empirical data (i.e., raters’ feedback, focus group interviews with sign language teachers, annotated data from DSGS users/learners with different levels of proficiency, feedback from raters, rated DSGS performances), supplemented with existing research on spoken and sign language fluency to inform the criteria of the fluency rating scale. That is, we combined different approaches for rating scale development as has been suggested in the literature (e.g., Knoch et al., 2021). In a next step, the fluency rating scale was used by three trained deaf raters on 162 performances from the DSGS users/learners (N = 28) with varying degrees of proficiency (i.e., L1 DSGS, L2 sign language interpreters, and L2 A1/A2 learners).
Evidence of validity
We argue that the different sources of data serve as a sound empirical basis for the operationalized “DSGS fluency construct” in the rating scale version 2. As stated in the Introduction, the current paper does not seek to validate a fully-fledged test. Our focus is only on the construct of fluency in a general sense and on how to operationalize this construct into a rating scale. Acknowledging that validity evidence should be about interpretation of test scores (which we do not claim), we can still link our sources of evidence for validity to those as stated in the Standards. The analyses reported in this paper would fall under test content validity, validity based on internal structure, and validity based on relations to other variables. First of all, drawing on existing theories of spoken and sign language fluency as well as on intuitions by experts can be seen as evidence on the validity of test content. Similarly, the analyses investigating performance samples add to the content validity evidence of the final scale. The many-facet Rasch measurements, investigating to what extent the different criteria within the scale can indeed be distinguished, fall under validity evidence based on internal structure. The other analyses in this paper add evidence on the validity based on relations to other variables. For instance, we compare measures/scores under two conditions (with and without planning time) and compare measures/scores across three proficiency groups. Finally, the analysis gauging amount of explained variance between rater scores from performance measures likewise adds evidence on the validity based on relations to other variables. Together, the objective fluency measures explained 88% of the variance in the rating scores. This is in the same order of magnitude as the 84% of explained variance reported by Bosker et al. (2013) on spoken L2 Dutch performances. In their study, similar to the multiple regression analyses reported here, ratings on fluency were predicted by objective measures of fluency. Therefore, our regression analysis can be seen as strong evidence of validity, as the subjective ratings reflect the objectively measured aspects of fluency as indicated by theory to a great extent.
Revision to the fluency rating scale (version 2)
The results of the MFRM rating scale analysis suggest that Criterion 3 (i.e., the use of non-manual components during the production of pauses) should either be revised, dropped altogether, or perhaps be combined with Criterion 1 or 2, as the scale did not function very well for this criterion. The statistical analysis of the annotated data and the correlation of the annotated data with the rated DSGS performances suggest that Criterion 3 could be revised. First of all, the combination of non-manual components during the production of pauses should be avoided in a revised rating scale since it could lead to (1) a high cognitive demands on the raters since they have to evaluate two aspects at the same time (e.g., McNamara, 1996) and (2) non-manual components also occur simultaneously with other aspects of fluency apart from pauses (e.g., Spijker and Oomen, 2023). Based on the results mentioned above, Criterion 3 could be revised to assess the use of non-manual components alone, or more specifically mouth activities and head movements because these non-manual components produced the most differences between L1 DSGS and L2 A1/A2 groups. Future research should investigate in more detail the use of non-manual components in (dis)fluent signing and how this could be operationalized in a fluency rating scale.
In addition, the description of score category 1 should be changed throughout, e.g., by omitting “very” in the scale category descriptions for all criteria, as scale category 1 was hardly used at all, even for the L2 A1/A2 group. Another consideration could be to further change the description of category 1 for Criteria 5 and 6 to better align these criteria’s difficulty to the other criteria (e.g., by using “some” instead of “many” in the description). Also, considering low probabilities of at least one scale category in four of the six criteria, the number of scale points could be reduced from six to five; this in turn may also solve the disordered Rasch-Andrich threshold of category 5 for Criterion 4. We have created a version 3 of the DSGS fluency rating scale (Supplementary Image 4).
Future application and evaluation of the fluency rating scale (version 3) could improve the scale’s overall validity and adapted and used in a DSGS assessment. In a future scenario, the DSGS assessment as well as the rating scale could be linked to the CEFR. The DSGS rating scale could be linked with the general CEFR fluency scale and/or the sign language fluency scale of the CEFR Companion Volume (Council of Europe, 2020).
Limitations
We experienced some limitations in this study. In the annotation process, financial constraints meant that we were not able to establish annotator reliability (signing tasks), that is, a certain number of DSGS videos should have been transcribed by two annotators.
We were able to include only self-reports on the sign language proficiency of all study participants. The self-ratings of the L2 SLI and the L2 A1/A2 groups produced some surprising overlap—even though one would not expect any. Due to the absence of any objective DSGS measure at the time of this study, self-ratings could not be compared to DSGS measures. In future studies DSGS measures will be used to assess the DSGS proficiency of L2 study participants.
We could have had more validity evidence if the tasks that we used were more differentiating in fluency performance. With the current tasks, there were only a few effects of preparation time on aspects of fluency in the performance data. It is not clear why that was the case. Our manipulation of complexity of tasks did likewise not lead to expected differences in performance, which was already shown for the spoken data by Dutch L2 English learners (Naber, 2021). Therefore, we decided not to investigate complex versus simple tasks in our DSGS performance data.
Also because of budget constraints, we were only able to annotate six of the twelve signing task performances of all study participants. More annotations and more ratings would have given us more empirical support for our analyses.
Conclusion
Despite the limitations of this study, we were able to confirm findings in DSGS from previous research on fluency from other sign languages, for example, pausing, repetitions, and speed of signing (e.g., Lupton, 1998; Notarrigo, 2017; Sipronen, 2018; Sipronen and Kanto, 2022). We were also able to find clear similarities to features of fluency in spoken language, for example, speed of speech, number and duration of pauses, but also a weaker contribution of repetitions and self-corrections (e.g., Bosker et al., 2013; Suzuki et al., 2021). Even though non-manual components contributed to the overall construct of fluency in the DSGS rating scale, a separate criterion for non-manual components was added in the revised rating scale (version 3, Supplementary Image 4). A clear modality-specific difference between spoken and sign languages is the simultaneous occurrence of non-manual and manual activities in (dis)fluent signing (Spijker and Oomen, 2023).
Data availability statement
The raw data supporting the conclusions of this article will be made available by the authors upon request.
Ethics statement
The studies involving humans were approved by the Center for Research and Development, HfH. The studies were conducted in accordance with the local legislation and institutional requirements. The participants provided their written informed consent to participate in this study.
Author contributions
TH: Writing – original draft, Writing – review and editing, Formal analysis, Methodology, Validation, Conceptualization, Data curation, Funding acquisition. NJ: Methodology, Conceptualization, Writing – original draft, Writing – review and editing, Formal analysis, Validation. FH: Formal analysis, Methodology, Writing – original draft, Writing – review and editing, Validation. KT: Conceptualization, Data curation, Writing – review and editing, Supervision. SS-M: Conceptualization, Data curation, Writing – review and editing. AB: Formal analysis, Methodology, Writing – original draft, Writing – review and editing. RP: Conceptualization, Data curation, Writing – review and editing. SE: Methodology, Writing – review and editing. SR: Conceptualization, Data curation, Writing – review and editing. SC: Data curation, Investigation, Writing – review and editing.
Funding
The authors declare that financial support was received for the research, authorship, and/or publication of this article. This project was funded by the Swiss National Science Foundation (SNSF) Spark funding scheme (grant number: 196797), https://data.snf.ch/grants/grant/196797.
Acknowledgments
We would like to thank all of our deaf and hearing study participants—without their participation we would not have been able to conduct this study. We also would like to express our gratitude to the students of sign language interpreting program from the HfH for the checking and coding of the focus group transcripts.
Conflict of interest
The authors declare that the research was conducted in the absence of any commercial or financial relationships that could be construed as a potential conflict of interest.
Publisher’s note
All claims expressed in this article are solely those of the authors and do not necessarily represent those of their affiliated organizations, or those of the publisher, the editors and the reviewers. Any product that may be evaluated in this article, or claim that may be made by its manufacturer, is not guaranteed or endorsed by the publisher.
Supplementary material
The Supplementary Material for this article can be found online at: https://www.frontiersin.org/articles/10.3389/feduc.2024.1466936/full#supplementary-material
Footnotes
- ^ The term “Deaf” (capitalized) is used when referring to sociocultural entities such as “Deaf community,” whereas the more inclusive “deaf” (not capitalized) refers to individuals rather than groups to account for the increasing diversity of identities and language practices of people who are deaf or hard-of-hearing (Kusters et al., 2017).
- ^ https://www.limesurvey.org
- ^ Amberscript is compliant with the General Data Protection Regulation (GDPR) of the European Union; https://www.amberscript.com/en/data-security-and-privacy/.
- ^ https://atlasti.com
- ^ https://glide.me
References
American Educational Research Association (AERA), American Psychological Association (APA), and National Council on Measurement in Education (NCME) (2014). Standards for Educational and Psychological Testing. Washington, DC: American Educational Research Association.
Bachman, L. F., and Palmer, A. (1996). Language Testing in Practice. Oxford: Oxford University Press.
Bates, D., Mächler, M., Bolker, B., and Walker, S. (2015). Fitting linear mixed-effects models using lme4. J. Stat. Softw. 67, 1–48. doi: 10.18637/jss.v067.i01
Battisti, A., Tissi, K., Sidler-Miserez, S., and Ebling, S. (2024). “Advancing annotation for continuous data in swiss german sign language,” in Proceedings of the LREC-COLING 2024 11th Workshop on the Representation and Processing of Sign Languages: Evaluation of Sign Language Resources, eds E. Efthimiou, S.-E. Fotinea, T. Hanke, J. A. Hochgesang, J. Mesch, and M. Schulder (ELRA Language Resources Association), 163–174.
Bosker, H. R., Pinget, A.-F., Quené, H., Sanders, T., and De Jong, N. H. (2013). What makes speech sound fluent? The contributions of pauses, speed and repairs. Lang. Testing 30, 159–175. doi: 10.1177/0265532212455394
Boyes Braem, P. (1984). “Studying swiss german sign language dialects,” in Recent Research on European Sign Languages, eds F. Loncke, P. Boyes Braem, and Y. Lebrun (Netherlands: Swets & Zeitlinger), 93–103.
Boyes Braem, P. (1995). Einführung in die Gebärdensprache und ihre Erforschung, Vol. Vol. 11. Frederick, MD: Signum-Verlag.
Brentari, D., Falk, J., Giannakidou, A., Herrmann, A., Volk, E., and Steinbach, M. (2018). Production and comprehension of prosodic markers in sign language imperatives. Front. Psychol. 9:770. doi: 10.3389/fpsyg.2018.00770
Caccamise, F., and Newell, W. (1999). Section 13: An Overview of the Sign Communication Proficiency Interview (SCPI): History, Development, Methodology, & Use. Unpublished manuscript. Rochester Institute of Technology, National Technical Institute for the Deaf.
Council of Europe (2020). Common European Framework of Reference for Languages: Learning, Teaching, Assessment. Companion Volume. Strasbourg: Council of Europe Publishing.
Cucchiarini, C., Strik, H., and Boves, L. (2000). Quantitative assessment of second language learners’ fluency by means of automatic speech recognition technology. J. Acoustical Soc. Am. 107, 989–999. doi: 10.1121/1.428279
Cull, A. (2014). Production of Movement in Users of American Sign Language and its Influence on Being Identified as “Non-Native”. Dissertation. Washington, DC: Gallauder University.
De Jong, N. H., Pacilly, J., and Heeren, W. (2021). PRAAT scripts to measure speed fluency and breakdown fluency in speech automatically. Assess. Educ. Principles Policy Pract. 28, 456–476. doi: 10.1080/0969594X.2021.1951162
Derwing, T. M., Rossiter, M. J., Munro, M. J., and Thomson, R. I. (2004). Second language fluency: Judgments on different tasks. Lang. Learn. 54, 655–679. doi: 10.1111/j.1467-9922.2004.00282.x
Ebling, S. (2016). Automatic Translation from German to Synthesized Swiss German Sign Language. Dissertation. Zürich: Universität Zürich.
Emmorey, K. (2002). Language, Cognition, and the Brain: Insights from Sign Language Research. Mahwah, NJ: Lawrence Erlbaum Associates.
Emmorey, K., and Herzig, M. (2003). “Categorical versus gradient properties of classifier constructions in ASL,” in Perspectives on Classifier Constructions in Sign Languages, ed. K. Emmorey (Mahwah, NJ: Erlbaum), 221–246.
Felker, E. R., Klockmann, H. E., and De Jong, N. H. (2019). How conceptualizing influences fluency in first and second language speech production. Appl. Psycholinguistics 40, 111–136. doi: 10.1017/S0142716418000474
Fulcher, G., Davidson, F., and Kemp, J. (2011). Effective rating scale development for speaking tests: Performance decision trees. Lang. Testing 28, 5–29. doi: 10.1177/0265532209359514
Goldman-Eisler, F. (1973). Psycholinguistics: Experiments in Spontaneous Speech (2. print). Cambridge, MA: Academic Press.
Hanke, T., and Storz, J. (2008). “iLex – a database tool for integrating sign language corpus linguistics and sign language lexicography,” in Proceedings of the LREC 2008 Workshop Proceedings, 64–67, (European Language Resources Association).
Hauser, P., Supalla, T., and Bavelier, D. (2008). “American sign language sentence reproduction test: Development and implications,” in Sign Languages: Spinning and Unraveling the Past, Present and Future. TISLR9, Forty Five Papers and Three Posters From the 9th. Theoretical Issues in Sign Language Research Conference, ed. R. Müller de Quadros (Petrópolis: Editora Arara Azul), 160–172.
Hilger, A. (2013). Production Fluency in Spoken Language Users Acquiring a Sign Language as an L2 in Adulthood. Undergraduate thesis. Champaign, IL: University of Illinois at Urban-Champaign.
Hilger, A. I., Loucks, T. M., Quinto-Pozos, D., and Dye, M. W. (2015). Second language acquisition across modalities: Production variability in adult L2 learners of American sign language. Sec. Lang. Res. 31, 375–388. doi: 10.1177/0267658315570648
Ho, D. G. E. (2013). “Focus groups,” in The Encyclopedia of Applied Linguistics, ed. C. A. Chapelle (Hoboken, NJ: Blackwel Publisher), 1–7. doi: 10.1002/9781405198431.wbeal0418
Holmström, I., Schönström, K., and Ryttervik, M. (2023). Development of a sign repetition task for novice L2 signers. Lang. Assess. Quart. 21, 33–59. doi: 10.1080/15434303.2023.2256320
Jang, E. E., Wagner, M., and Park, G. (2014). Mixed methods research in language testing and assessment. Annu. Rev. Appl. Ling. 34, 123–153. doi: 10.1017/S0267190514000063
Johnston, T., and Schembri, A. (2007). Australian Sign Language: An Introduction to Sign Language Linguistics. Cambridge: Cambridge University Press.
Kanto, L., and Haapanen, U.-M. (2020). “Fluency in sign language,” in Fluency in L2 Learning and Use, eds M. Lintunen and M. Mutta (Bristol: Multilingual Matters), 96–110.
Knoch, U., Deygers, B., and Khamboonruang, A. (2021). Revisiting rating scale development for rater-mediated language performance assessments: Modelling construct and contextual choices made by scale developers. Lang. Testing 38, 602–626. doi: 10.1177/0265532221994052
König, S., Konrad, R., and Langer, G. (2012). “Lexikon: Der Wortschatz der DGS [Lexicon: The vocabulary of German Sign Language],” in Handbuch Deutsche Gebärdensprache: Sprachwissenschaftliche und anwendungsbezogene Perspektiven, Vol. 50, eds H. Eichmann, M. Hansen, and J. Heßmann (Vienna: Signum Verlag), 111–164.
Konrad, R. (2011). Die LEXIKALISCHE STRUKTUR der Deutschen Gebärdensprache im Spiegel Empirischer Fachgebärdenlexikographie. Zur Integration der Ikonizität in ein Korpusbasiertes Lexikonmodell. Tübingen: Gunter Narr Verlag.
Kormos, J., and Dénes, M. (2004). Exploring measures and perceptions of fluency in the speech of second language learners. System 32, 145–164. doi: 10.1016/j.system.2004.01.001
Krueger, R. A., and Casey, M. A. (2015). Focus Groups: A Practical Guide for Applied Research, 5th Edn. Thousand Oaks, CA: Sage Publications.
Kusters, A., De Meulder, M., and O’Brien, D. (2017). Innovations in Deaf Studies: The Role of Deaf Scholars. Oxford: Oxford University Press.
Lennon, P. (1990). Investigating fluency in EFL: A quantitative approach. Lang. Learn. 40, 387–417. doi: 10.1111/j.1467-1770.1990.tb00669.x
Linacre, J. M. (2023). A user’s guide to FACETS Rasch-Model Computer Programs. Program Manual 3.87.0. Available online at: https://www.winsteps.com/a/Facets-Manual.pdf (accessed June 2, 2024).
Marian, V., Blumenfeld, H. K., and Kaushanskaya, M. (2007). The language experience and proficiency questionnaire (LEAP-Q): Assessing languag profiles in bilinguals and multilinguals. J. Speech Lang. Hear. Res. 50, 940–967. doi: 10.1044/1092-4388(2007/067)
Montee, M., and Malone, M. E. (2013). “Writing scoring criteria and score reports,” in The Companion to Language Assessment, ed. A. J. Kunnan (Hoboken, NJ: John Wiley & Sons, Inc), 847–859.
Naber, T. (2021). Measuring Fluency in English as a Second Language: A Quantitative approach on the effects of Complexity, Planning and Task Design. Unpublished Master Thesis. Netherlands: Leiden University.
Newell, W., Caccamise, F., Boardman, K., and Ray Holcomb, B. (1983). Adaptation of the language proficiency interview (LPI) for assessing sign communicative competence. Sign Lang. Stud. 41, 311–347.
Notarrigo, I. (2017). Marqueurs de (dis)fl Uence en Langue des Signes de Belgique Francophone. PhD Dissertation. Belgium: University of Namur.
Notarrigo, I., and Meurant, L. (2014). “Nonmanuals and markers of (dis)fluency in French belgian sign language (LSFB),” in Proceedings of the 6th Workshop on the Representation and Processing of Sign Languages: Beyond the Manual Channel. 9th International Conference on Language Resources and Evaluation (LREC2014), (Reykjavik), 135–142. Available at: http://www.lrec-conf.org/proceedings/lrec2014/index.html
Notarrigo, I., and Meurant, L. (2022). (Dis)fluency Markers in French Belgian Sign Language – LSFB [Online presentations]. Online Dissemination Event of the SNSF Project “Approaching and Validating the Construct of Fluency in Swiss German Sign Language (DSGS), Online.
Plonsky, L., and Oswald, F. L. (2014). How big is “big”? Interpreting effect sizes in L2 research: Effect sizes in L2 research. Lang. Learn. 64, 878–912. doi: 10.1111/lang.12079
Sadri Mirdamadi, F., and De Jong, N. H. (2015). The effect of syntactic complexity on fluency: Comparing actives and passives in L1 and L2 speech. Sec. Lang. Res. 31, 105–116. doi: 10.1177/0267658314554498
Sandler, W. (2012). The phonological organization of sign languages: Sign language phonology. Lang. Ling. Compass 6, 162–182. doi: 10.1002/lnc3.326
Sipronen, S. (2018). Pace and Pause Flexibility in Finnish Sign Language. Master thesis. Finland: University of Jyväskylä.
Sipronen, S., and Kanto, L. (2022). Utterance fluency in finnish sign language L1 and L2 signing. Finnish J. Ling. 34, 149–177.
Spijker, L., and Oomen, M. (2023). Hesitation markers in sign language of the Netherlands A corpus-based study. Sign Lang. Stud. 23, 164–196.
Stewart, D., and Shamdasani, P. (2014). Focus Groups: Theory and Practice, 3rd Edn. Thousand Oaks, CA: SAGE.
Sutton-Spence, R., and Woll, B. (1999). “Chapter one: Linguistics and sign linguistics,” in The Linguistics of British Sign Language, (Cambridge: Cambridge University Press), 1–21.
Suzuki, S., Kormos, J., and Uchihara, T. (2021). The relationship between utterance and perceived fluency: A meta-analysis of correlational studies. Modern Lang. J. 105, 435–463. doi: 10.1111/modl.12706
Tavakoli, P., Nakatsuhara, F., and Hunter, A. (2020). Aspects of fluency across assessed levels of speaking proficiency. Modern Lang. J. 104, 169–191. doi: 10.1111/modl.12620
Wilbur, R. (2000). “Phonological and prosodic layering of non-manuals in American Sign Language,” in The Signs of Language Revisited: Ananthology to Honor Ursula Bellugi and Edward Klima, eds K. Emmorey and H. Lane (Mahwah, NJ: Lawrence Erlbaum Associates), 215–243.
Wilbur, R. B., and Malaia, E. (2018). “A new technique for analyzing narrative prosodic effects in sign languages using motion capture technology,” in Linguistik Aktuell/Linguistics Today, Vol. 247, eds A. Hübl and M. Steinbach (Amsterdam: John Benjamins Publishing Company), 15–40.
Woll, B. (2013). “Second language acquisition of sign language,” in The Encyclopedia of Applied Linguistics, ed. C. A. Chapelle (Hoboken, NJ: Blackwell Publishing Ltd).
Yuan, F., and Ellis, R. (2003). The effects of pre-task planning and on-line planning on fluency, complexity and accuracy in L2 monologic oral production. Appl. Ling. 24, 1–27. doi: 10.1093/applin/24.1.1
Zeshan, U. (2004a). Hand, head, and face: Negative constructions in sign languages. Ling. Typol. 8, 1–58.
Zeshan, U. (2004b). Interrogative constructions in sign languages: A cross-linguistic perspective. Language 80, 7–39.
Keywords: sign language fluency, sign language fluency rating scale, Swiss German Sign Language (Deutschschweizerische Gebärdensprache, DSGS), rating scale development, rating scale validation, multi-facet Rasch analysis, regression analysis
Citation: Haug T, de Jong NH, Holzknecht F, Tissi K, Sidler-Miserez S, Battisti A, Perrollaz R, Ebling S, Reinhard S and Caminada S (2024) Development and validation of a fluency rating scale for Swiss German Sign Language. Front. Educ. 9:1466936. doi: 10.3389/feduc.2024.1466936
Received: 18 July 2024; Accepted: 04 November 2024;
Published: 03 December 2024.
Edited by:
Diane Lillo-Martin, University of Connecticut, United StatesReviewed by:
Bencie Woll, University College London, United KingdomYan Liu, Carleton University, Canada
Christian Rathmann, Humboldt University of Berlin, Germany
Copyright © 2024 Haug, de Jong, Holzknecht, Tissi, Sidler-Miserez, Battisti, Perrollaz, Ebling, Reinhard and Caminada. This is an open-access article distributed under the terms of the Creative Commons Attribution License (CC BY). The use, distribution or reproduction in other forums is permitted, provided the original author(s) and the copyright owner(s) are credited and that the original publication in this journal is cited, in accordance with accepted academic practice. No use, distribution or reproduction is permitted which does not comply with these terms.
*Correspondence: Tobias Haug, dG9iaWFzLmhhdWdAaGZoLmNo