- 1Department of Biology, Weingarten University of Education, Weingarten, Germany
- 2Technical Education, Weingarten University of Education, Weingarten, Germany
There is a broad consensus in politics, economy and science about the importance of promoting STEM competences. To promote STEM skills in the classroom, we propose a cross-domain approach combining the STEM sub-domains of Biology and Technology/Engineering. Therefore, we have developed a model that illustrates how the STEM sub-domains of Biology and Technology/Engineering can complement each other in a cognitive process. Both sub-domains show similarities in working methods and consider structural and functional relationships. These synergies can be brought together in a design process. This approach leads to the question of the extent to which a cross-domain approach utilising a design process can be a learning opportunity for biological subject knowledge. Based on this model, we have created a learning arrangement on the topic of the ‘locomotor system’. Learners should use a design process to build a feeding machine inspired by structural-functional relationships in different locomotor systems. In a quasi-experimental study, we compared this Design approach with two alternative teaching approaches (a Reconstruction approach and a Biology approach). In the Reconstruction approach, students receive the same input on structural-functional relationships in locomotor systems but build their feeding machine according to a predetermined plan. In the Biology approach, no feeding machine is built; instead, structural-function relationships in locomotor systems are modelled using further examples from the animal world. 413 pupils (age: M = 12.53, SD = 0.818) took part in the study. Longitudinal and cross-sectional analyses emphasise the potential of the Design approach compared to the other methods and show significant differences in long-term learning outcomes. Path models illustrate that cognitive abilities (IQ) and participation in the ‘Design’-intervention group are decisive prerequisites for learning success. The results also show that students following the Design-approach develop a significantly improved understanding of concepts related to the musculoskeletal system. Using a design process combining biology and engineering presents a learning opportunity for students to develop long-term robust biological knowledge and understanding of biological concepts. Thus, using a design approach can be a cross-domain bridge for integrated STEM instruction in order to foster pupils’ knowledge acquisition and competences.
1 Introduction
Subject canon of Science, Technology, Engineering and Mathematics (STEM) disciplines (computer science, natural sciences, technology, engineering and mathematics) are crucial for technological progress and innovation in our society. They enable scientific discoveries, address global challenges, and are central to economic prosperity (Xie and Killewald, 2012; Ray, 2015; Bacovic et al., 2022). Promoting STEM skills in schools is considered essential to prepare young people for the future in a technologised world. Scientific and technological challenges of the future require an increasingly interdisciplinary approach in which scientific and technical issues are meaningfully related to each other (Lead States, 2013; National Research Council of the National Academies, 2011). The discussion about STEM education should be seen in the light of this background. STEM education can be understood as the sum of the individual disciplines (science, technology, engineering, and mathematics) or as an approach emphasising the connections between the disciplines or domains and referred to as interdisciplinary STEM education (Bryan et al., 2015; Gao et al., 2020).
One challenge for integrating an interdisciplinary STEM approach in schools is the more disciplinary perspective of teachers on teaching, who have usually studied one or two science subjects and are therefore hardly familiar with the other STEM disciplines (Brand, 2020). One way of convincing discipline-oriented teachers of the benefits of an interdisciplinary STEM approach is to determine the extent to which the interdisciplinary approach can lead to the acquisition of disciplinary and interdisciplinary subject knowledge alike. To achieve this, we have developed a learning arrangement in which learners not only acquire purely subject knowledge but are able to use this to solve an overarching problem by designing a product. Transferring domain-related subject knowledge into an interdisciplinary context, such as solving complex problems, is an important STEM skill (Honey et al., 2014, p. 52). Consequently, this learning arrangement aims to promote interdisciplinary STEM skills by supporting the acquisition of conceptual subject knowledge in its integration into a design process.
2 Theoretical background
For the development of interdisciplinary STEM approaches, promoting the acquisition of knowledge and its application and transfer to other areas, the challenge is to connect the STEM domains from the perspective of the respective subject didactics and from the interdisciplinary perspective in a theoretically justified way. Different approaches are being pursued at the policy level. In their ‘three dimensions of science learning’ model, the Next Generation Science Standards in the USA emphasise an interrelationship between science and technology in all three dimensions (core ideas, cross-cutting concepts, and practises) (Lead States, 2013). This interrelationship forms a fundamental pillar of science and technology education. Another approach is to relate activities in the different STEM domains to each other and to highlight similarities and differences to identify similarities in working methods (cf. National Research Council of the National Academies, 2011) and similarities in content, such as the consideration of structural and functional relationships (Reiser et al., 2021, 2023). In the context of our study, we concentrate on a cross-domain connection between the subject-specific didactic STEM sub-domains ‘Technology/Engineering’ and ‘Sciences’ (with a focus on Biology). Such an approach makes it possible, for example, to compare the cognitive processes of the different disciplines (Figure 1). It can be seen that both engineering and science undergo analytical and synthesising processes in their approach to problem-solving.
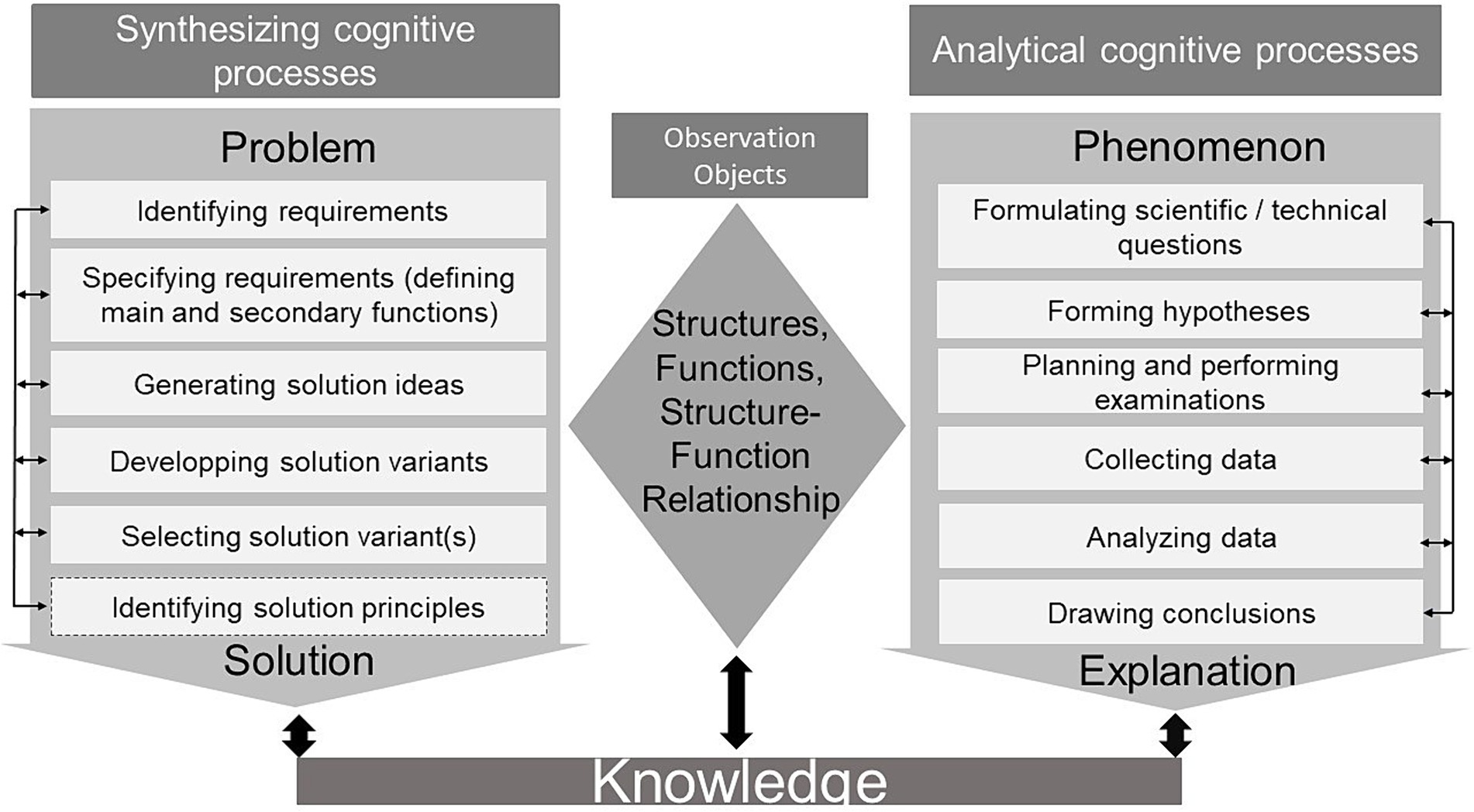
Figure 1. Gaining insights by integrating scientific and engineering perspectives into a problem-solving process.
In the engineering sciences, for example, insights are gained through a synthesising approach during design processes and in the natural sciences during modelling or the development of experimental setups. Analytical cognitive processes are used to investigate and explain phenomena and are inherent to both engineering and science. Both processes deal with structures and functions as well as their interrelationships. Insights into structure–function relationships acquired in an analytical cognitive process can be useful in generating solutions in a synthesising cognitive process. Furthermore, findings about structure–function relationships from a synthesising cognitive process can help to explain phenomena as analogies. The formation of analogies could represent a possible learning opportunity to acquire conceptual knowledge (Anderson and Krathwohl, 2001, p. 46).
About subject knowledge learning in interdisciplinary STEM lessons, the proposed framework model shows the potential that conceptual subject knowledge about structural and functional relationships can be learned in both cognitive processes (synthetic and analytical) and can then be, respectively, and reciprocally integrated into the other process. For example, knowledge about structural and functional relationships learned in an analytical process can be integrated into a synthesising process and given an application reference in product design. Similarly, designed products can be analysed for their inherent structural and functional relationships. In our study, learners are confronted with a problem for which they must build a product. However, in order to ensure the functionality of the product, the learners likely lack procedural knowledge about manufacturing. In order to bridge this knowledge deficit, the learners deal with a biological phenomenon. Examining this phenomenon follows the analytical path of cognition and focuses on conceptual biological knowledge (Anderson and Krathwohl, 2001, p. 46). This conceptual biological knowledge is based on formal and functional relationships that can be used to explain the biological phenomenon. The knowledge of these structural and functional relationships can now be used to replicate these structures and the functions on which they are based within the framework of the synthesising path of knowledge. Consequently, in our study, we assume that pupils acquire conceptual biological knowledge (Anderson and Krathwohl, 2001, p. 46) of structural and functional relationships within the framework of an analytical path of cognition and transfer this knowledge to a procedural level as a basis for problem-solving (Braithwaite and Sprague, 2021) in the construction of a product and apply it there. This raises the question of the extent to which the inclusion of conceptual subject knowledge in synthesising processes of cognition represents a learning opportunity that contributes to promoting the acquisition of conceptual subject knowledge. As a methodical approach to the interdisciplinary combination of synthesising and analytical knowledge, we chose a design approach.
2.1 Design approaches in the classroom? Design-based learning as an approach that promotes learning
Design as a working method shows potential for relating technology/engineering and biology as sub-domains to each other. This was referred to as a design approach. In the context of science learning, where the design process is utilised by learners in the school environment, the ‘design approach’ in educational research is referred to as ‘design-based learning’ (Rosa, 2016, with reference to Nelson, 1984). This conceptual shift enables a change of focus from a pure description of the product manufacturing process to a stronger focus on the learning process. Design-based learning (DBL) is a constructivist learning approach involving learners’ active design of products or artefacts due to a problem-solving process (Kamal and Junaini, 2019; Fortus and Vedder-Weiss, 2014). DBL combines the concept of problem-based learning with project-based learning (Gómez Puente et al., 2013; So et al., 2018; Zhang et al., 2022). Consequently, this approach offers touchpoints with methodological approaches that positively influence learning. In DBL, problem-based learning (PBL), openness to solutions, indirect instruction, and hands-on activities come together in an exploratory and reflective problem-solving process. The development of products goes hand in hand with hands-on activities in which learners actively engage with the problem. This can lead to deeper learning (Doppelt et al., 2008). In a systematic literature review, Oliveira and Bonito (2023, p. 15) emphasise that practical work (= hands-on activities) engages students in scientific topics and promotes their conceptual understanding. This contrasts with the findings of Schwichow et al. (2016). Their comparative study found that hands-on activities in science lessons have no general advantage over ‘paper-and-pencil training tasks’. The authors point out that their findings are consistent with the results of other studies (e.g., Klahr et al., 2007; Triona and Klahr, 2003) and are also supported by the meta-analysis by Smetana and Bell (2012), who compared practical with virtual tasks. Here, hands-on activities showed no general advantages in science lessons (Schwichow et al., 2016, p. 998). Oliveira and Bonito (2023) conclude that learning success is strongly influenced by how the activity is carried out. Consequently, it is not enough to carry out hands-on activities in order to have a positive influence on learning. Instead, an accompanying reflection in the form of mental activities (minds-on activities) is required. Problem-based learning offers a possible starting point for linking hands-on activities with a reflective activity. In DBL, problem-based learning results from the task set to learners to develop a product. This problem-based learning (Mandl, 2004) encourages learners to find a solution. Problem-based approaches promote active learning and knowledge building and provide a link to real-life and authentic problem-solving (Akcay, 2009, p. 28). Akınoğlu and Tandoğan (2007, p. 78), for example, show in an intervention study that students taught in a problem-based way achieve higher learning outcomes in terms of academic performance and concept learning in science classes. The learning outcomes of subject knowledge in problem-based learning arrangements are controversially discussed in the literature against the background of the degree of instruction. Mayer (2004, p. 18), as the conclusion of his study on the effectiveness of purely discovery-based PBL approaches, fundamentally doubts the effectiveness of these approaches on learning outcomes. This discussion reflects less criticism of a problem-based approach; instead, the solution approach is examined in terms of its effectiveness for the learners and their learning success. Considering this discussion is essential for using a design process insofar as it is open to solutions and characterised by a low level of direct instruction. Approaches with these characteristics are playing an increasingly important role in science learning. They promote a deeper understanding of scientific concepts by encouraging students to ask questions, identify problems and solve them systematically (Hmelo-Silver et al., 2007). These skills are critical for understanding and applying scientific methods to real-world problems (Marshall et al., 2009; Minner et al., 2010). As stated by Mayer (2004), the learning effectiveness of a learning programme with too much openness or a low level of instruction is criticised. Kirschner et al. (2006, p. 75) support this argument and state that there is hardly any evidence that a low level of instruction produces better cognitive processes. Similarly, an approach that is too open would place too much strain on the capacities of working memory, which would hinder long-term knowledge growth (Kirschner, 2002; Kirschner et al., 2006). Zhang et al. (2022, p. 1168) list international studies that show that inquiry-based learning opportunities (as learning opportunities with an indirect teaching character) correlate with a decline in science learning outcomes. In response to Kirschner’s criticism of learning settings with little instructional character (= indirect instruction), Hmelo-Silver et al. (2007) argue that problem-based learning programmes are by no means instruction-free but offer learners a considerable amount of assistance. They refer to the data from several studies (Lynch et al., 2005; Guthrie et al., 2004; Langer, 2001; Wu and Tsai, 2005), which show that learners particularly benefit from problem-and inquiry-based learning opportunities with appropriate support in the form of instruction. Lazonder and Harmsen (2016) came to the same conclusion in a meta-analysis of the effectiveness of instruction in problem-based learning programmes. In contrast, comparative studies on the learning effectiveness of direct and indirect instruction show that although direct instruction facilitates the rapid acquisition of information, this knowledge does not remain stable in the long term. Students who underwent learning arrangements with indirect instruction also showed stable long-term learning results (Dean and Kuhn, 2007; Wittwer and Renkl, 2008, 2010). Manishimwe et al. (2023) also show the effects of inquiry-based learning about biology lessons. Consequently, a design approach in an integrated stem lesson could provide a basis for an efficient learning environment due to possible synergy effects (PBL, hands-on, openness to solution, low level of direct instruction).
2.2 Designing as a working method
Analytical and synthesising cognitive processes are brought together in the development of designs. Design is an iterative, multi-step process that aims to create a manifest product that can serve as a technical solution to a problem (National Research Council, 2009, p. 82). The processes involved begin with clarifying the problem area and defining the properties that the desired technical solution must fulfil. To this end, ideas are collected—also from other domains—on how the required elements can be technically realised. Different materials that can be used to construct the partial solutions are analysed. Finally, the partial solutions are combined into a product. This product is then checked for its function and optimised if necessary. The entire process can have several iterations (Hafiz and Ayop, 2019; Reiser et al., 2021, 2023).
2.2.1 Motivational implications of a design approach
Learner’s motivation plays a vital role in promoting learning outcomes. Cerasoli et al. (2014) and Hulleman et al. (2010) both state that motivation can be a medium to strong predictor of learning-specific behaviour at school and of academic success. In this context, studies show that methodological approaches that promote learning and are used in the design approach (PBL, DBL, hands-on activities and a reflective approach) also have a positive effect on the motivation and interest of learners (Gnambs and Hanfstingl, 2016; Steinmayr et al., 2019; Cerasoli et al., 2014; Hulleman et al., 2010). Against this background, the results of interest research in STEM instructions should also be considered. Studies on PBL (Hasni and Potvin, 2015; Hmelo-Silver et al., 2007; Wijnia et al., 2011) as well as on DBL (Guedel, 2014; Weng et al., 2023) and the associated hands-on activities (Potvin and Hasni, 2014, p. 103; Holstermann et al., 2010, p. 751; Swarat et al., 2012; Oje et al., 2021) attribute motivational effects to these in the context of a reflexive (minds-on) approach.
Studies from interest research show apparent thematic differences in interest in the STEM disciplines concerning gender (Koul et al., 2012). Holstermann and Bögeholz (2007, p. 77 ff.), for example, found in a study of year 10 pupils that girls are more interested in topics relating to body awareness, health, and natural phenomena. Su and Rounds (2015) describe similar findings. Their study showed that gender-specific interest differences vary greatly depending on the STEM subject area. The most remarkable gender-specific differences in interests can be observed in engineering (here in favour of men). In contrast, no significant gender-specific interest differences were found in the biological and medical sciences or the technical aspects of scientific activities. Thus, referring to thematically orientated interests, gender may influence learning gains in cross-domain learning opportunities that are methodologically oriented towards an engineering approach.
2.3 Design and gender-specific academic performance in science lessons
In addition to the interest-related differences, the data on the gender-related performance of boys and girls in science classes are inconclusive. Martin et al. (2000) describe that boys perform better than girls. This contrasts with studies by Pant et al. (2013) or Duckworth and Seligman (2006), which show that girls perform slightly better than boys in science subjects. Vooren et al. (2022) point out that there are no differences in higher STEM education.
2.4 Design approaches in integrated STEM-education
The potential of using a design approach to implement integrated STEM learning opportunities is evident in instructional research. Benenson (2001) describes possible synergies of engineering practises with maths or science education. Hmelo et al. (2000, p. 251) see designing as a method of teaching complex biological/anatomical systems by emphasising functional specifications. In biology lessons, basic concepts are essential in explaining the diversity of individual biological phenomena (Schmiemann et al., 2012). One of these basic concepts is form and function (Jeffery et al., 2021). This concept can be used to describe and explain the functions of systems and organisms. These structures and functions can also be reconstructed and modelled as analogies (Howell et al., 2019) or integrated into a design process. There are indeed some studies indicating a positive impact of interdisciplinary, design-orientated STEM teaching on learners’ subject knowledge in the sub-domains of STEM (Mehalik et al., 2008; Apedoe et al., 2008) and problem-solving skills (Li et al., 2016). However, the results vary depending on the disciplines or domains selected for integration (Becker and Park, 2011; Guzey et al., 2016; Sanders, 2009, 2012). It is striking that comparatively few studies focussed on Biology amongst the studies on the impact of design approaches on learners’ scientific subject knowledge. Only Guzey et al. (2016) analyse the outcome of biological knowledge (on ecosystems). Their results show that special education pupils show improved knowledge, but the biological knowledge of pupils not belonging to the special education classes did not improve. Tipmontiane and Williams (2022) also see the positive potential of design approaches in Biology lessons. However, they also point out that design processes are used less frequently in biology disciplines than in other disciplines. They refer to the arguments of other authors (Guzey et al., 2016; Moore and Smith, 2014; Lazarowitz and Penso, 1992) who point out that the complexity of biological concepts makes it difficult to find a design reference to them. The study presented here is located in the context of this research gap. It aims to contribute to understanding what influence a design approach has on pupils’ biological conceptual knowledge.
3 Research questions
In the context of the proposed model and the learning opportunities arising from the procedure, we are investigating the question: To what extent does the transfer of biological subject knowledge into an engineering design process represent an opportunity to promote the acquisition of biological subject knowledge? To this end, we pose the following research questions:
Q1: How do interdisciplinary problem-solving processes using a design approach affect pupils’ knowledge acquisition of biological subject knowledge?
Q2: Which variables influence knowledge acquisition of biological subject knowledge in interdisciplinary design approaches?
Q3: What influence do interdisciplinary problem-solving processes using a design approach have on learning biological concepts?
4 Methods
4.1 Experimental design
These questions were investigated in a quasi-experimental research design. Three interventions on the topic of the locomotor system were developed and compared with each other:
A ‘Design group’ in which pupils independently construct a feeding machine whose function is inspired by form-function relationships of locomotor systems in living organisms.
A ‘Reconstruction group’ in which the pupils work on the same biological content and build the feeding machine according to a construction plan.
A ‘Biology group’ in which the pupils investigate the form and functional relationships for movement in different creatures by building models and analysing similarities in principles on blueprints.
All three interventions have the same duration (5 units of 90 min each.). The pupils work on the same content related to the movement of endoskeletons, exoskeletons and hydrostatic skeletons, as well as their structural and functional relationships. Here, the pupils deal with the concepts of ‘muscles contract and exert force’, ‘muscles have counter-play’, and ‘muscles have to tension joints’. The reconstruction group and the design group use these concepts to realise them structurally when making the required product. However, the interventions differ in terms of the approaches used, the problem provided and the level of instruction (see Table 1).
The Design group uses the structural and functional relationships of locomotor systems previously covered in class to translate these into partial technical solutions used to build a technical solution (feeding machine). The design process here offers reflected hands-on experiences. It is explorative, iterative, and not limited to a fixed solution (= the level of instruction is low). To solve this task, learners need to find a way to induce a movement in the feeding machine, make it reversible and reproducible, and ensure its stability. They need to know how joints or axes are constructed and function, how force can be exerted across the joint and how the movement can be reversed.
After working on the identical biological phenomena of skeletal movement in different locomotor systems, the Reconstruction group receives a construction plan for the feeding machine and produces its product according to these instructions. In this approach, the learners also use hands-on activities to solve a problem. However, the solution is predetermined by the plan provided and is only manufactured. In this respect, the Reconstruction approach differs from the design approach in the degree of direct instruction. The built solution (feeding machine) is then analysed for similarities with form and function relationships from locomotor systems. The pupils merely have to follow the instructions and later think about which concepts of movement generation were used at which point in the finished product.
The Biology intervention group does not construct a product (feeding machine). Instead, further examples of the different locomotor systems (functional model of a hand, model of a spider leg, construction of a snake body model) are modelled. The problem here is not one of developing a product that encompasses the entire project. Instead, the individual models are organised into types of locomotor systems for the problem: How does the movement work for each type of skeleton? Analogous structural and functional relationships are also considered for different construction plans. Therefore, the level of instruction in creating the models is relatively high. Apart from a model of the forearm function, enabling exploration and a free approach, the other functional models are produced according to instructions and then analysed.
In the study, the three approaches are analysed concerning the increase in conceptual knowledge about movement in musculoskeletal systems. To ensure the groups’ comparability, the participants’ cognitive prerequisites are measured. The learners’ intrinsic motivation is also analysed against the background of theoretical considerations on the possible effects of design learning approaches on learning. A comprehensive analysis of the influence of this design approach on intrinsic motivation was published in another article (Reiser et al., 2024b). The IQ of the participants, their age, their gender, and their participation in the respective intervention group were considered possible influencing variables. The data collection followed the experimental design shown in Figure 2.
Intrinsic motivation was assessed once in relation to the general Science/Biology lessons and once following the intervention. This article presents the motivation data in the context of their possible influence as a predictor of learning outcomes. Knowledge was tested three times in a pre-, post-, and follow-up design. The cognition test, which is used to assess cognitive performance and enables a more precise comparison of the pupils, was tested once.
4.2 Instruments
Three instruments are used to collect data in the study:
The CFT 20-R cognition test (Weiß and Weiß, 2006): the CFT 20-R is a language-independent intelligence test that measures basic intelligence ‘General Fluid Ability’ according to Cattell (1943). This comprises the ability to identify and solve figural relationships and formal logical reasoning problems of varying degrees of difficulty within a fixed time frame. Based on the test results, an intelligence quotient can be determined. The CFT 20-R is an established diagnostic test for the screening of fluid intelligence. It was validated for the age group of this study. The short form of the test (part 1 with 56 items) is used in the study.
The short scale of intrinsic motivation (German Abbreviation: KIM) by Wilde et al. (2009): the instrument consists of 12 items divided into four subscales: Interest/enjoyment, perceived competence, perceived freedom of choice, and pressure/tension, each with three items. Wilde et al. (2009) carried out their validation study on 176 pupils aged M = 10.4 SD = 0.48. Confirmatory factor analyses and associated structural equation models were used to demonstrate the structural validity of the scale. For further validation, correlations were found between the willingness to repeat the museum visit and, on the other hand, the evaluation according to school grades. These showed satisfactory values. Consequently, the KIM can be regarded as a valid, reliable, and time-efficient test instrument for assessing intrinsic motivation that is suitable for the target group. Its subscales use a placeholder for specific activities, which was replaced by the ‘Science/Biology lessons’ and by the name of the Intervention in the present surveys. The items of the scales were mixed to prevent the pupils from categorising them. The evaluation uses a five-point Likert scale (0 to 4, where 0 means ‘not at all true’ and 4 means ‘completely true’). For data analysis, the values of the 12 items were determined as an arithmetic mean.
The knowledge test MUSCLS (Reiser et al., 2024a): the MUSCLS measures knowledge of movement based on three concepts: the ‘actionist principle’, the ‘antagonist principle’, and the ‘muscles span across joints principle’ in 10 items distributed over two scales (AM and GP). Scale 1 ‘AM’ (German abbreviation for muscle attachment) deals with the attachment of muscles to endoskeletons and how the muscles induce movement. The items focus on the concept of muscles contract and exert traction on skeletal elements. In addition, the items capture the concept that muscles must stretch across joints in skeletons with rigid elements to induce movement. Scale 2 ‘GP’ (German abbreviation for antagonistic principle) deals with the antagonistic principle in muscularly induced movement sequences. The items on the scale cover the movement induction by muscle contraction and on the antagonist principle. The points achieved were summed up and given as a score. The test also provides ‘qualitative feedback’ on which concepts are mastered and to what extent. For this purpose, an evaluation is carried out based on the point level achieved for the respective items. If the pupils achieve one point for an item on the AM scale, they show they have understood the concept of the ‘actionist principle’. Achieving the second point shows that the concept of ‘muscles span over joints’ has also been understood. The GP scale allows similar conclusions to be drawn from its scoring. Achieving one point for an item on this scale relates to the ‘actionist principle’ concept. The evaluation with a second point shows that in addition to the concept of the ‘actionist principle’, the participants also know the concept of the ‘antagonist principle’. This evaluation can use 10 items to measure the actionist concept of the musculature. Six items measure the antagonist concept, and four items measure the concept ‘muscles span over joints’. In this way, an ‘IF X’ counting function in Excel can determine which scores were given for which items. These values can then be displayed as a percentage ratio (number of affected cases divided by the possible total number). The MUSCLS was evaluated and validated for the target age group of this study.
4.3 Sample
Only fully completed questionnaires (pre and post) were used in this study. The resulting sample comprised 413 pupils divided into the three intervention groups. The Biology group comprised 134 pupils (67 boys and 67 girls) aged M = 12.6 SD = 0.75. 148 pupils (78 boys and 70 girls) aged M = 12.35, SD = 0.91, participated in the Design group. 131 pupils (62 boys and 69 girls, age M = 12.60 SD = 0.74) underwent the Reconstruction group.
4.4 Analyses
Data analyses carried out were performed using the software IBM SPSS Statistics Version 29.000 (IBM Corporation, 2023) and the R packages lavaan, rstatix, tidyvers and ggubber (version 2023.09.01 + 494). Only the data from the fully completed questionnaires were used for the analysis. This corresponds to the number of participants from the sample description. First, the reliability of the measurements was determined based on internal consistency, and descriptive analyses were carried out. Then, a simple ANOVA was used to determine the comparability of the groups in terms of their cognitive performance. The BF10 was also calculated to provide a more valid interpretation of the results. Subsequently, longitudinal and cross-sectional analyses were carried out as a mixed ANOVA (3 × 3). The analysis was cross-checked using non-parametric methods. Possible influencing factors (IQ, gender, age, and the type of intervention attended) were analysed using path models, determined using a robust estimator (MLR). The learnt concepts of the MUSCLS were analysed using non-parametric tests (Friedmann ANOVA and Kruskal-Wallis tests). The significance level of the inferential statistics was set at a p-value of less than 0.05.
5 Results
5.1 Reliability of the measurement
The internal consistency of the respective measurement instruments was measured using Cronbach’s α (Table 2).
Also, based on the concepts of movement, the MUSCLS shows a Cronbach’s α between 0.815 and 0.924 in all groups and at all measurement times.
All instruments showed satisfactory to very good internal consistencies concerning the respective groups and measurement times (Blanz, 2015).
5.2 Descriptive statistics
The results of the descriptive statistics concerning the respective tests are shown in Table 3.
Normal distribution was checked using the Shapiro–Wilk-test. The results indicated that only the CFT data had a normal distribution in all groups. The data on the intrinsic motivation of the interventions was not normally distributed in two of the three intervention groups. The results of the knowledge test were not normally distributed in any group at any of the time points. The Henze-Zirkler-Test also showed no congruent multivariate normal distribution of the data. Levene tests of the knowledge scores achieved and the IQ test in the groups showed a given homoscedasticity. Based on the box-m test for the knowledge scores, it is assumed here that the covariance matrices are equal.
5.3 Cognitive ability of the participants in the intervention groups
The results of a simple analysis of variance showed that the three intervention groups do not differ significantly in terms of their cognitive ability F (2, 410) = 1.060, p =0.348, BF10 = 0.072. The additionally determined BF10 supports the assumption of a valid 0-hypothesis, according to which the three groups do not differ regarding cognitive ability.
5.4 Comparison of knowledge gains of the three intervention groups
Comparative analyses of the performance of the different intervention groups in the knowledge test were carried out at three different measurement times in a mixed design (3 × 3) (Figure 3).
The effects on knowledge acquisition were analysed using a mixed ANOVA (Table 4) with Huynh-Feldt adjustment (0.943) for the variables ‘time of measurement’ and the interaction effect of the variables ‘time of measurement’ and ‘group’, excluding one pupil with extreme values (>3-fold interquartile range).
The mixed-ANOVA reveals significant statistical differences both between the groups and the different measuring time points, including the interaction effects between the groups and the time points. The η2 values (0.022–0.049) indicate minor effects. Bonferroni post-hoc tests were performed to precisely identify and present these differences, both for within-group comparisons (Table 5) and between-group comparisons (Table 6).
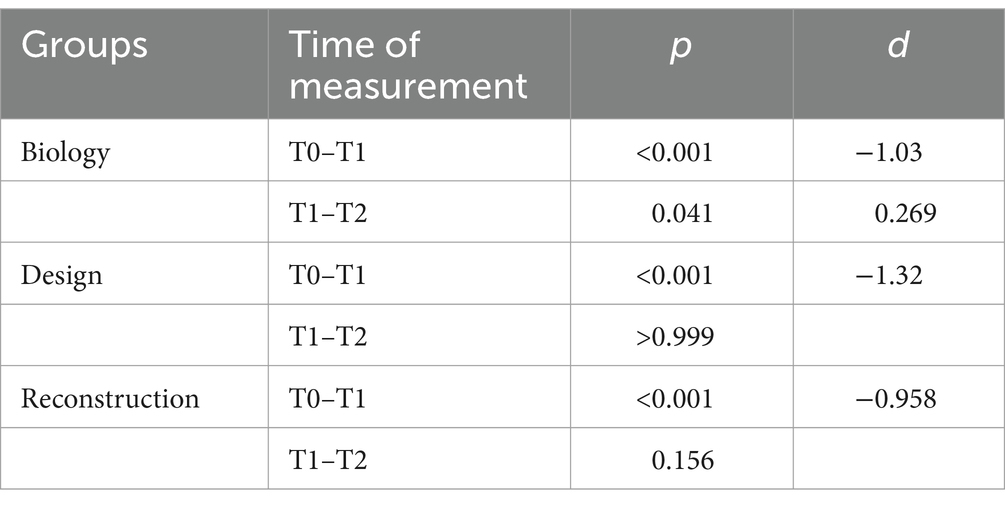
Table 5. Bonferroni post hoc test: pairwise comparisons within values of the group at the respective measurement times.
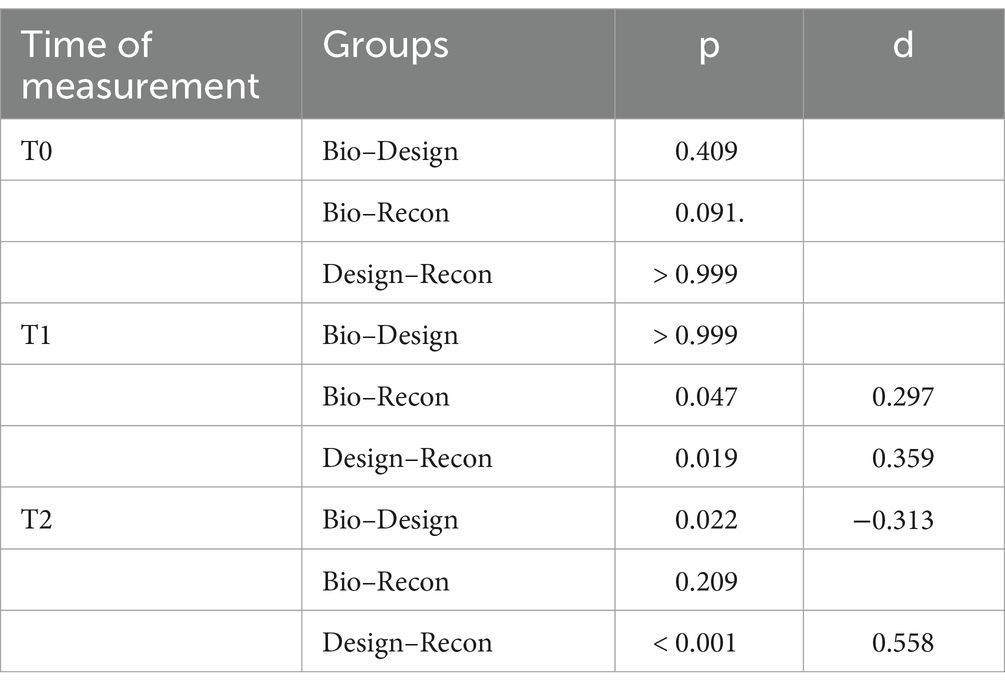
Table 6. Bonferroni post hoc test: pairwise comparisons between the groups at the different measurement times.
All groups show a significant increase in expertise as a result of their interventions (measurement time T1) (d > 0.950). However, this knowledge does not remain at the same level for all groups until the time of measurement (T2). In particular, there was a significant drop in knowledge in the Biology group (p = 0.041, d = 0.269). In contrast, the other intervention groups showed no statistically significant change in their knowledge.
The cross-sectional comparisons are shown in Table 6.
At T0, there was no significant difference between the groups. After the intervention was completed (T1), there were no significant differences in the knowledge scores between the Biology group and the Design group. In contrast, the Reconstruction group achieved significantly lower scores. At T2, the Design group showed significantly higher results compared to the other two groups. There are no significant differences between the Reconstruction group and the Biology group. Due to the violation of the normal distribution of the data, the mixed ANOVA was tested using non-parametric longitudinal and cross-sectional methods. Both the Friedmann ANOVA and the Kruskal Wallis tests carried out, as well as their post-hoc tests, confirm the results of the mixed ANOVA. The non-parametric tests were consistent with the results of the ANOVA.
5.5 Factors influencing knowledge acquisition
The acquisition of specialised knowledge represents a multifaceted variable shaped by various influencing factors. Several variables were analysed in this study, including gender, IQ, age, the chosen teaching approach, and intrinsic motivation. Path models were calculated for T1 and T2 to analyse these influences (Figure 4). Both models show a sufficient number of observed data points to clearly estimate all parameters in the model. The models, as shown in Figure 4, are therefore ‘just identified’.
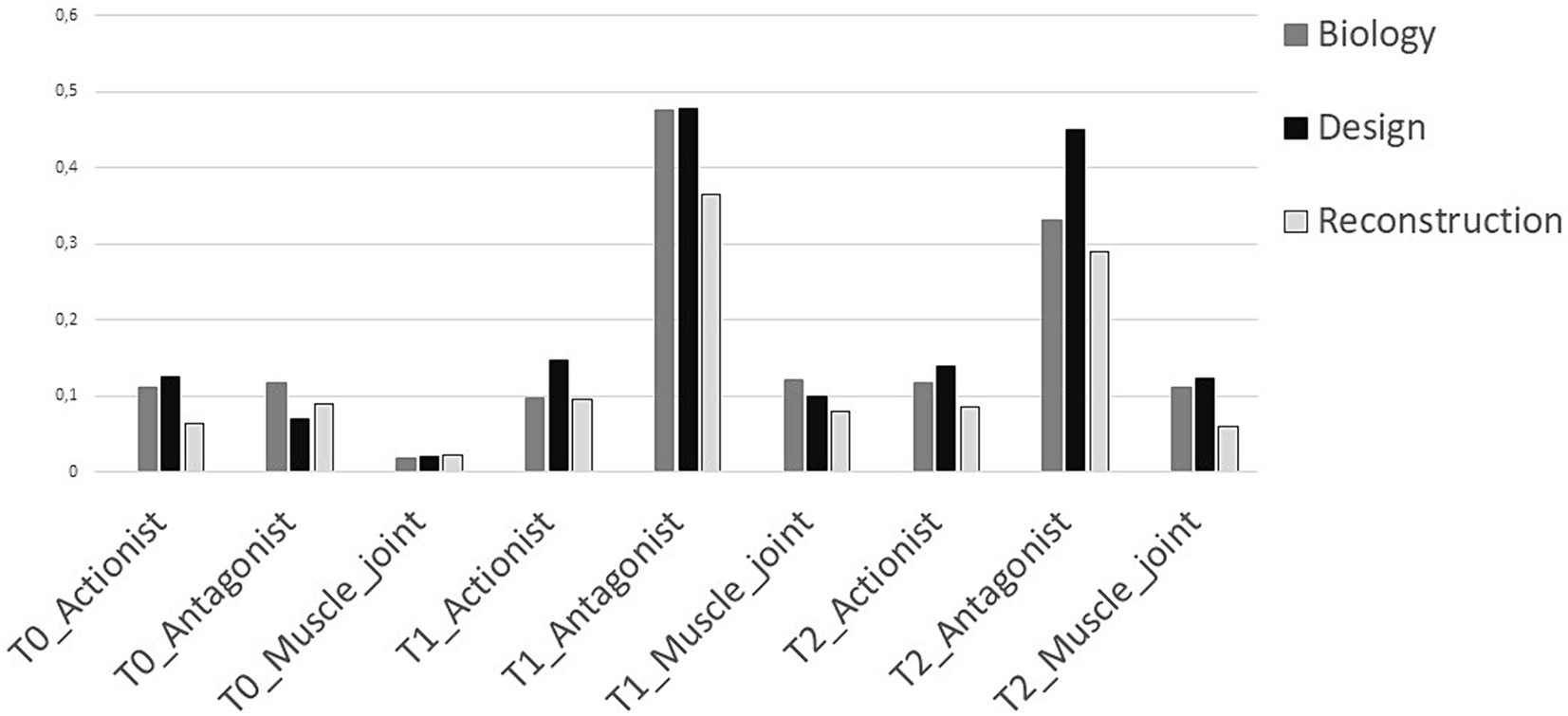
Figure 5. Intergroup comparisons of learning movement concepts over three measurement points. The numerical values on the y-axis refer to percentage values specified in decimal notation (50% = 0.5).
Path model 1 shows that motivation is statistically significantly influenced by the variables IQ (β = 0.139, p = 0.006) and the type of intervention attended. In particular, the Biology (β = −0.167, p = 0.002) and Reconstruction (β = −0.201, p < 0.001) approaches lead to significantly lower motivation values. In contrast, gender (p = 0.052) and age (p = 0.677) showed no significant influence.
At time T1, knowledge acquisition was significantly influenced by IQ (β = 0.294, p < 0.001), age (β = 0.103, p = 0.038) and participation in the Reconstruction approach (β = −0.142, p = 0.008). Gender, on the other hand, showed no significant influence (p = 0.471), and attending the Biology approach also showed no significant difference in terms of knowledge acquisition. The influence of motivation on knowledge is also insignificant (p = 0.053).
In path model T2, motivation refers to the same point in time as in model T1, so the influences of the exogenous variables remain identical. At measurement time T2, however, the influences of the variables on knowledge acquisition change. IQ remains a significant influencing variable (β = 0.228, p < 0.001). Participation in the Biology approach (β = −0.129, p = 0.016) and the Reconstruction approach (β = −0.224, p < 0.001) led to significantly poorer results. The variables age (p = 0.093), gender (p = 0.648) and the motivation values achieved (p = 0.055) show no significant effects.
5.6 Biological concepts
The comparison of the interventions about the concepts understood (‘actionist principle’, ‘antagonist principle’, and ‘muscles span over joints’) (Figure 5 and Table 7) was carried out using non-parametric group comparisons due to a large number of outliers.
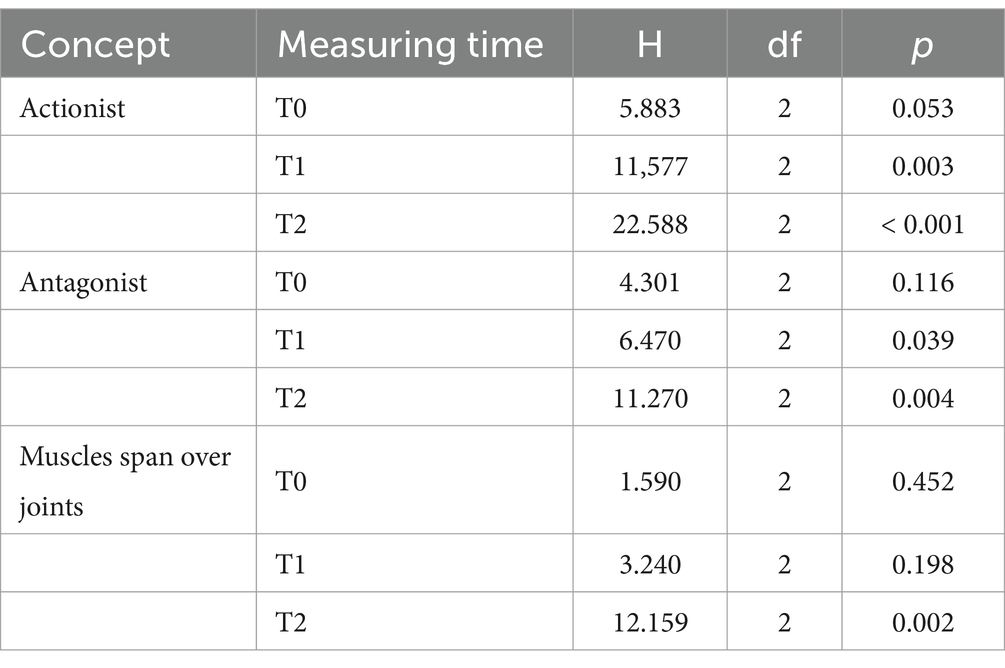
Table 7. Testing for differences between the intervention groups at the respective measuring times in relation to the understanding of the respective concepts.
Regarding the ‘actionist’ concept, the Kruskal-Wallis tests show significant differences between the intervention groups at the measurement times T1 and T2. For the ‘antagonist’ concept, significant differences between the intervention groups were only found at T1 and T2. About the concept of ‘muscles span over joints’, there are only significant differences between the groups at time point T2. The differences between groups at the various time points were analysed using Bonferroni post-hoc pairwise comparisons (Table 8).
At time T0, there were no significant differences in the pair comparisons. At time T1, a significant difference was found in the pairwise comparison between the Design group and the Reconstruction group (p = 0.002, r = 0.205). Despite this finding, the post-hoc tests showed no further significant differences at this point, which contrasts with the results of the Kruskal-Wallis tests. Several significant differences were found at the T2 measurement point. Particularly in the context of the ‘actionist’ concept, the Design group showed significantly higher values than the other two groups. However, the effect of these differences could be categorised as small in each case.
Regarding the concept of ‘antagonist’, the Design group showed significantly higher values than the Reconstruction group; however, the other group comparisons did not yield any significant results. With regard to the concept ‘muscles span over joints’, the post-hoc tests showed no significant differences between the Biology group and the Design group. Nevertheless, both groups showed significantly higher values than the Reconstruction group (Biology–Reconstruction p = 0.201, r = 0.161; Reconstruction–Design: p = 0.003, r = 0.202). Friedmann ANOVAs were performed for the longitudinal comparisons, as shown in Table 9. These analyses highlight the changes in the values for the respective concepts over the measurement times T0, T1, and T2, separately for the intervention groups.
The available results of the different Friedman tests about the values of the respective movement concepts suggest that the intervention groups differ statistically significantly across the measuring time points. These differences were tested for significance using Bonferroni post-hoc tests, as shown in Table 10.
In the context of the ‘actionist’ concept, all intervention groups show significant increases from T0 to T1, accompanied by large effect sizes. From T1 to T2, the values slightly decrease, with the Design group showing the least pronounced effect size.
With regard to the ‘antagonist principle’, the comparison from T0 to T1 shows a significant increase in learning with effect sizes (r > 0.500) in all groups. These increases remain significant in the Design group and the Reconstruction group from T1 to T2. In contrast, there was a significant decrease in the Biology group (r = 0.669).
The ‘muscles span over joints’ concept only led to a significant increase with a small effect size (r = 0.282) in the Biology group over the course of the measurements from T0 to T1. There were no other changes in the values in the pair comparisons.
6 Discussion
Promoting STEM skills in schools is key in preparing young people for the future in a technologised world. The scientific and technological challenges of the future are increasingly demanding interdisciplinary approaches in which scientific and technical issues are interrelated and ways of thinking and working are intertwined. In the context of the rather domain-specific training of STEM teachers, interdisciplinary approaches to problems pose a particular challenge in schools. Seeing acquired subject knowledge in an overarching context and applying it to solve interdisciplinary problems presents an important key competence in STEM education. Therefore, this study aimed to present an approach in which scientific and technical problems are combined within the framework of a design process and to investigate the influence of the transfer of biological structural knowledge into a design process on the acquisition of biological subject knowledge. For this purpose, three different hands-on learning arrangements were created, which differ in terms of their degree of freedom, openness to solutions, and their working methods. The three intervention groups (Biology, Design and Reconstruction) proved to be comparable in terms of their cognitive performance (IQ) (see result of the ANOVA, 5.4) and in their initial knowledge before the intervention (measuring pot T0).
The research question Q1, which asked for the effects of interdisciplinary, design-based problem-solving processes on pupils’ knowledge acquisition of biological subject knowledge, leads to interesting observations. A longitudinal analysis of the period between T0 and T1 showed that knowledge scores increased significantly in all three interventions. At T1, the Design group did not differ significantly from the Biology group, whilst the knowledge scores of the Reconstruction group were significantly lower. In the long-term comparison after 3 weeks (T1 to T2), it was found that the knowledge scores in the Design group had not changed significantly, which indicates long-term knowledge stability. In contrast, the Biology group showed a significant drop in knowledge scores. On the other hand, the Reconstruction group showed no significant changes and thus exhibited a certain degree of knowledge stability. The parallel increase in subject knowledge at time T1 in the Design and Biology group and the later drifting apart as a result of the drop in knowledge amongst the participants in the Biology group at T2 can be seen in the light of the results of comparative studies by Dean and Kuhn (2007) and Wittwer and Renkl (2008, 2010). These show that interventions with a greater degree of instruction enable a rapid increase in learning but that this knowledge does not remain stable in the long term. Compared to the Biology and the Design group, the Reconstruction group achieved the lowest increase in knowledge. This could be because this intervention group had less opportunity to reflect on their actions as part of their practical activities. By providing a production plan for the feeding machine, the cognitive load on the learners was reduced, whereby the learners did not have to think about their actions during construction but only had to carry them out. However, it is precisely this connection between ‘minds-on’ and ‘hands-on’ that supports a deeper understanding (Helmke, 2012; Reusser, 2006; Oliveira and Bonito, 2023).
The research question Q2 asked, ‘Which variables influence the acquisition of biological subject knowledge in interdisciplinary, design-based approaches?’ It is explained by the path models and the observations at different points in time (T1 and T2). In path model T1, it was found that motivation was statistically significantly influenced by certain variables. In particular, the variables IQ and the type of intervention attended (Biology and Reconstruction) significantly affected motivation. The Biology and Reconstruction approaches lead to significantly lower motivation values than the Design approach. Gender and age, on the other hand, showed no significant influence on the intrinsic motivation. At time T1, knowledge acquisition was significantly influenced by IQ, age, and participation in the Reconstruction approach. In contrast, gender and participation in the Biology approach did not show significant differences in knowledge acquisition. Motivation showed no significant influence on knowledge at this stage. In the path model for T2, the influences of the exogenous variables remain identical, but there were changes in the influences on specialised knowledge. The IQ remained a significant influencing variable. Participation in the Biology approach and the Reconstruction approach led to significantly poorer results than the Design approach. The variables age, gender, and the motivation values achieved show no significant effects on knowledge acquisition at T2. The influence of cognitive ability (IQ) on learning success is hardly surprising here; this correlation is often described in cognitive psychology (e.g., Sternberg and Grigorenko, 2003) and is considered well-documented. In line with the results of the mixed ANOVA, it is evident that the type of intervention attended (Design approach) significantly affects learning success. This can be explained by the fact that the design approach combines a variety of aspects from problem-based learning (PBL) (Akcay, 2009, 28; Akınoğlu and Tandoğan, 2007, 78), and design-based learning (DBL) (Doppelt et al., 2008) that are conducive to learning and combines this with ‘minds-on’ activities (Helmke, 2012; Reusser, 2006; Oliveira and Bonito, 2023). The lack of significant influence of gender on learning gains is in line with studies on gender studies in STEM and science, which show that female learners do not differ from the performance of male learners (Vooren et al., 2022).
Concerning the motivation, the data also show that IQ and the type of approach attended (with the best values for the design approach) are significant influencing factors. This picture is consistent with motivational research studies on hands-on activities (Holstermann et al., 2010; Swarat et al., 2012; Oje et al., 2021; Potvin and Hasni, 2014; Guedel, 2014) and problem-based learning (Reusser, 2005; Hasni and Potvin, 2015; Hmelo-Silver et al., 2007). Furthermore, the fulfilment of basic needs (autonomy, competence, and social relatedness) according to the self-determination theory (Deci and Ryan, 1993) can be used to explain the increase in learning gains based on using design approaches. Here, the three interventions differ in that the experience of autonomy is less pronounced in the Biology approach or the Reconstruction approach compared to the Design approach. In both approaches (Biology and Reconstruction), the solution to the problem is provided by detailed modelling/construction plans. However, the experience of autonomy is a very decisive aspect of instructional motivation (Patall et al., 2008; Reeve et al., 2003; Hofferber et al., 2015; Guay et al., 2008; Su and Reeve, 2011).
In the context of the results, the data indicate that the Design approach positively influences learning success more than the other two approaches. This is evident not only in terms of the scores achieved but also in the degree of understanding of the concepts. Concerning Q3, it can be stated that the students who followed the Design approach understood the concepts of the “actionist principle,” “antagonist principle,” and “muscles stretching over joints” better than the participants in the Reconstruction approach. And that the “actionist” concept was better understood compared to the Biology approach. This is particularly evident in the long-term comparison at measurement time T2. This could be explained by the following. For the design process, the concepts necessary for generating movement in locomotor systems also represent solution principles for the technical design. Forces must act via an axis/joint. There must be an actionist generating a movement and an antagonist causing the counter-movement. If these are not understood, they cannot be used in the technical solution. The product loses its functionality. In the design process, learners transfer the structural and functional relationships into the product-developing process. This cognitive transfer is already provided by blueprints in the Reconstruction approach. For learners of this intervention group, it is not necessary to apply the concepts; the construction plan already contains them. In the Biology group, the relationships between structure and function must be identified using several models of different skeleton types. This revision of the same concepts seen in different locomotor systems possibly leads to an initially similar learning success, but this appears to be less lasting (T2). One reason for this could be that product development by design leads to a more intensive engagement with the subject-specific content (Mehalik et al., 2008, p. 80, Apedoe et al., 2008).
The results of the study suggest that a design approach taking into account the transfer of scientific (biological) knowledge into a design process and confronting pupils with a problem with transfer performance (minds-on) can represent a learning opportunity to promote scientific competence. It supports learners in acquiring biological concept knowledge and helps them better understand biological principles. Learners are given the opportunity to use this conceptual subject knowledge from one subject area to solve problems in another subject area, which trains an overarching view of STEM subjects and promotes STEM skills. The approach encourages and promotes a positive environment for boys and girls alike. As an implication for science teaching, our results suggest that in order to maximise the learning-promoting effect, it is important that the design task is as open as possible. In this way, better learning growth and the acquisition of long-term stable knowledge can be achieved. Tasks that contain ready-made solutions should be viewed critically in this context and do not promote learning to the same extent. The comparable results of the Biology approach and the Design approach demonstrate that the Design approach does not impair subject-specific knowledge acquisition. On the contrary, the Design approach promotes long-term, stable knowledge and enables the application of subject-specific knowledge in an interdisciplinary context. This can be a valuable addition to traditional biology lessons.
In addition to the effects of a design-based approach on subject knowledge, the study also indicates which concepts of movement are easier or more difficult for learners to understand. Knowledge of the ‘actionist principle’ in the locomotor system is more easily understood by many pupils than knowledge of the antagonist principle. The concept of ‘muscles span over joints’ is more difficult for learners to understand. This shows that particular attention should be paid to this concept in teaching locomotor systems.
7 Conclusion
In our study, design-based working methods in science lessons show positive effects such as higher learning success and long-term knowledge stability. The design approach can help to promote a deeper understanding of concepts such as the actionist and antagonist principles. The results emphasise the importance of practise-oriented activities that enable the transfer of knowledge into design processes for the acquisition of scientific competence. They also show that interdisciplinary STEM learning arrangements combining Biology and Technology can positively affect the acquisition of biological subject knowledge and learners’ intrinsic motivation.
Data availability statement
The raw data supporting the conclusions of this article will be made available by the authors, without undue reservation.
Ethics statement
The requirement of ethical approval was waived by Ethics Committee of the University of Education Weingarten for the studies involving humans because this research is exempt from formal approval by the Ethics Committee according to the regulations of the Ethics Committee of the University of Education Weingarten and is based on a self-assessment checklist. All data were collected on an explicitly voluntary basis after obtaining parental consent and were completely anonymized. Inferences about participants are not possible. Neither participation nor non-participation in the study led to disadvantages for the individuals concerned. Authorisation for data collection has been granted by the Ministry of Education and Cultural Affairs (protocol code KM31-6499-3/4/3). The studies were conducted in accordance with the local legislation and institutional requirements. Written informed consent for participation in this study was provided by the participants’ legal guardians/next of kin. Written informed consent was obtained from the individual(s) for the publication of any potentially identifiable images or data included in this article.
Author contributions
MR: Conceptualization, Data curation, Formal analysis, Investigation, Methodology, Resources, Software, Supervision, Validation, Visualization, Writing – original draft, Writing – review & editing. MB: Conceptualization, Funding acquisition, Resources, Supervision, Writing – original draft. HW: Conceptualization, Funding acquisition, Methodology, Project administration, Supervision, Writing – original draft.
Funding
The author(s) declare that financial support was received for the research, authorship, and/or publication of this article. This work was funded by the VECTOR Foundation under Grant number (P2021-0083). This work was also supported by Open-Access-Funds of University of Education Weingarten.
Conflict of interest
The authors declare that the research was conducted in the absence of any commercial or financial relationships that could be construed as a potential conflict of interest.
Publisher’s note
All claims expressed in this article are solely those of the authors and do not necessarily represent those of their affiliated organizations, or those of the publisher, the editors and the reviewers. Any product that may be evaluated in this article, or claim that may be made by its manufacturer, is not guaranteed or endorsed by the publisher.
Abbreviations
STEM, Subject canon of Science, Technology, Engineering and Mathematics; PBL, Problem-based-learning; DBL, Design-based learning; KIM, Short scale on intrinsic motivation (German abbreviation); MUSCLS, Measuring instrument for understanding of the structural-functional correlation in locomotor systems. It is a Knowledge Test; AM, Scale in MUSCLS named according to a German abbreviation of the attachment of muscles; GP, Scale in MSUCLS named according to a German abbreviation of the antagonistic principle.
References
Akınoğlu, O., and Tandoğan, R. Ö. (2007). The effects of problem-based active learning in science education on students’ academic achievement, attitude and concept learning. Euras. J. Math. Sci. Technol. Educ. 3, 71–81. doi: 10.12973/ejmste/75375
Anderson, L. W., and Krathwohl, D. R. (2001). A Taxonomy for Learning, Teaching, and Assessing: A Revision of Bloom's Taxonomy of Educational Objectives. New York, NY: Longman.
Apedoe, X. S., Reynolds, B., Ellefson, M. R., and Schunn, C. D. (2008). Bringing engineering design into high school science classrooms: the heating/cooling unit. J. Sci. Educ. Technol. 17, 454–465. doi: 10.1007/s10956-008-9114-6
Bacovic, M., Andrijasevic, Z., and Pejovic, B. (2022). STEM education and growth in Europe. J. Knowl. Econ. 13, 2348–2371. doi: 10.1007/s13132-021-00817-7
Becker, K., and Park, K. (2011). Effects of integrative approaches among science, technology, engineering, and mathematics (STEM) subjects on students’ learning: a preliminary meta-analysis. J. STEM Educ. Innov. Res. 12, 23–37.
Benenson, G. (2001). The unrealized potential of everyday technology as a context for learning. J. Res. Sci. Teach. 38, 730–745. doi: 10.1002/tea.1029
Blanz, M. (2015). Gütekriterien von Testverfahren (Kap. 9.3) . Forschungsmethoden und Statistik für die Soziale Arbeit. Grundlagen und Anwendungen. Stuttgart: Kohlhammer. 255–9.
Braithwaite, D. W., and Sprague, L. (2021). Conceptual knowledge, procedural knowledge, and metacognition in routine and nonroutine problem solving. Cogn. Sci. 45:e13048. doi: 10.1111/cogs.13048
Brand, B. R. (2020). Integrating science and engineering practices: outcomes from a collaborative professional development. Int. J. STEM Educ. 7, 1–13. doi: 10.1186/s40594-020-00210-x
Bryan, L. A., Moore, T. J., Johnson, C. C., and Roehrig, G. H. (2015). “Integrated STEM education” in STEM Road Map: A Framework for Integrated STEM Education. eds. C. C. Johnson, E. E. Peters-Burton, and T. J. Moore (New York, NY: Routledge), 23–37.
Cattell, R. B. (1943). The measurement of adult intelligence. Psychol. Bull. 40, 153–193. doi: 10.1037/h0059973
Cerasoli, C. P., Nicklin, J. M., and Ford, M. T. (2014). Intrinsic motivation and extrinsic incentives jointly predict performance: a 40-year meta-analysis. Psychol. Bull. 140, 980–1008. doi: 10.1037/a0035661
Dean, D. Jr., and Kuhn, D. (2007). Direct instruction vs. discovery: the long view. Sci. Educ. 91, 384–397. doi: 10.1002/sce.20194
Deci, E. L., and Ryan, R. M. (1993). Die Selbstbestimmungstheorie der Motivation und ihre Bedeutung für die Pädagogik: The theory of self-determination of motivation and its relevance to pedagogics . Weinheim, Basel: Beltz.
Doppelt, Y., Mehalik, M. M., Schunn, C. D., Silk, E., and Krysinski, D. (2008). Engagement and achievements: a case study of design-based learning in a science context. J. Technol. Educ. 19, 22–39.
Duckworth, A. L., and Seligman, M. E. (2006). Self-discipline gives girls the edge: gender in self-discipline, grades, and achievement test scores. J. Educ. Psychol. 98, 198–208. doi: 10.1037/0022-0663.98.1.198
Fortus, D., and Vedder-Weiss, D. (2014). Measuring students' continuing motivation for science learning. J. Res. Sci. Teach. 51, 497–522. doi: 10.1002/tea.21136
Gao, X., Li, P., Shen, J., and Sun, H. (2020). Reviewing assessment of student learning in interdisciplinary STEM education. Int. J. STEM Educ. 7:11. doi: 10.1186/s40594-020-00225-4
Gnambs, T., and Hanfstingl, B. (2016). The decline of academic motivation during adolescence: an accelerated longitudinal cohort analysis on the effect of psychological need satisfaction. Educ. Psychol. 36, 1691–1705. doi: 10.1080/01443410.2015.1113236
Gómez Puente, S. M., Van Eijck, M., and Jochems, W. (2013). A sampled literature review of design-based learning approaches: a search for key characteristics. Int. J. Technol. Des. Educ. 23, 717–732. doi: 10.1007/s10798-012-9212-x
Guay, F., Ratelle, C. F., and Chanal, J. (2008). Optimal learning in optimal contexts: the role of selfdetermination in education. Can. Psychol. 49, 233–240. doi: 10.1037/a0012758
Guedel, K. (2014). Technikaffinität von Mädchen und Jungen der Sekundarstufe I [Affinity for technology among girls and boys in lower secondary school]. Doctoral dissertation. University of Geneva.
Guthrie, J. T., Wigfield, A., Barbosa, P., Perencevich, K. C., Taboada, A., Davis, M. H., et al. (2004). Increasing reading comprehension and engagement through concept-oriented reading instruction. J. Educ. Psychol. 96, 403–423. doi: 10.1037/0022-0663.96.3.403
Guzey, S. S., Moore, T. J., Harwell, M., and Moreno, M. (2016). Stem integration in middle school life science: student learning and attitudes. J. Sci. Educ. Technol. 25, 550–560. doi: 10.1007/s10956-016-9612-x
Hafiz, N. R. M., and Ayop, S. K. (2019). Engineering design process in stem education: a systematic. Int. J. Acad. Res. Bus. Soc. Sci. 9, 676–697. doi: 10.6007/IJARBSS/v9-i5/5998
Hasni, A., and Potvin, P. (2015). Student's interest in science and technology and its relationships with teaching methods, family context and self-efficacy. Int. J. Environ. Sci. Educ. 10, 337–366. doi: 10.12973/ijese.2015.249a
Helmke, A. (2012). Unterrichtsqualität und Lehrerprofessionalität [Instructional Quality and Teachers’ Professional Competence]. Seelze: Klett Kallmeyer.
Hmelo, C. E., Holton, D. L., and Kolodner, J. L. (2000). Designing to learn about complex systems. J. Learn. Sci. 9, 247–298. doi: 10.1207/S15327809JLS0903_2
Hmelo-Silver, C. E., Duncan, R. G., and Chinn, C. A. (2007). Scaffolding and achievement in problem-based and inquiry learning: a response to Kirschner, Sweller, and Clark (2006). Educ. Psychol. 42, 99–107. doi: 10.1080/00461520701263368
Hofferber, N., Eckes, A., Kovaleva, A., and Wilde, M. (2015). Die Auswirkung von autonomieförderndem Lehrerverhalten im Biologieunterricht mit lebenden Tieren [The effect of autonomy-promoting teacher behaviour in biology lessons with living animals]. Zeitschrift Didaktik Naturwissensch 21, 17–27. doi: 10.1007/s40573-014-0022-4
Holstermann, N., and Bögeholz, S. (2007). Interesse von Jungen und Mädchen an naturwissenschaftlichen Themen am Ende der Sekundarstufe I [gender-specific interests of adolescent learners in science topics]. Zeitschrift Didaktik Naturwissensch 13, 71–86.
Holstermann, N., Grube, D., and Bögeholz, S. (2010). Hands-on activities and their influence on students’ interest. Res. Sci. Educ. 40, 743–757. doi: 10.1007/s11165-009-9142-0
Honey, M., Pearson, G., and Schweingruber, H. (Eds.) (2014). STEM Integration in K-12 Education: Status, Prospects, and an Agenda for Research. Washington, DC: National Academies Press.
Howell, M. E., Booth, C. S., Sikich, S. M., Helikar, T., Roston, R. L., and Couch, B. A. (2019). Student understanding of DNA structure-function relationships improves from using 3D learning modules with dynamic 3D printed models. Biochem. Mol. Biol. Educ. 47, 303–317. doi: 10.1002/bmb.21234
Hulleman, C. S., Schrager, S. M., Bodmann, S. M., and Harackiewicz, J. M. (2010). A meta-analytic review of achievement goal measures: different labels for the same constructs or different constructs with similar labels? Psychol. Bull. 136, 422–449. doi: 10.1037/a0018947
Jeffery, C. J., Dorgan, K. M., and Pysh, L. (2021). Promoting a more integrated approach to structure and function. Integr. Comp. Biol. 61, 2020–2030. doi: 10.1093/icb/icab144
Kamal, A. A., and Junaini, S. N. (2019). The effects of design-based learning in teaching augmented reality for pre-university students in the ict competency course. Int. J. Sci. Technol. Res. 8, 2726–2730.
Kirschner, P. A. (2002). Cognitive load theory: implications of cognitive load theory on the design of learning. Learn. Instr. 12, 1–10. doi: 10.1016/S0959-4752(01)00014-7
Kirschner, P. A., Sweller, J., and Clark, R. E. (2006). Why minimal guidance during instruction does not work: an analysis of the failure of constructivist, discovery, problem-based, experiential, and inquiry-based teaching. Educ. Psychol. 41, 75–86. doi: 10.1207/s15326985ep4102_1
Klahr, D., Triona, L. M., and Williams, C. (2007). Hands on what? The relative effectiveness of physical versus virtual materials in an engineering design project by middle school children. J. Res. Sci. Teach. 44, 183–203. doi: 10.1002/tea.20152
Koul, R., Roy, L., and Lerdpornkulrat, T. (2012). Motivational goal orientation, perceptions of biology and physics classroom learning environments, and gender. Learn. Environ. Res. 15, 217–229. doi: 10.1007/s10984-012-9111-9
Langer, J. A. (2001). Beating the odds: teaching middle and high school students to read and write well. Am. Educ. Res. J. 38, 837–880. doi: 10.3102/00028312038004837
Lazarowitz, R., and Penso, S. (1992). High school students' difficulties in learning biology concepts. J. Biol. Educ. 26, 215–223. doi: 10.1080/00219266.1992.9655276
Lazonder, A. W., and Harmsen, R. (2016). Meta-analysis of inquiry-based learning: effects of guidance. Rev. Educ. Res. 86, 681–718. doi: 10.3102/0034654315627366
Lead States, N. G. S. S. (2013). Next Generation Science Standards: for States, by States. Washington, DC: National Academies Press.
Li, Y., Wang, K., Xiao, Y., and Froyd, J. E. (2016). Research and trends in STEM education: a systematic review of journal publications. Int. J. STEM educ.w 3:11. doi: 10.1186/s40594-016-0046-z
Lynch, S., Kuipers, J., Pyke, C., and Szesze, M. (2005). Examining the effects of a highly rated science curriculum unit on diverse students: results from a planning grant. J. Res. Sci. Teach. 42, 912–946. doi: 10.1002/tea.20080
Mandl, H. (2004). Gestaltung problemorientierter Lernumgebungen [Designing problem-orientated learning environments]. J. Lehrerinnen Lehrerbildung 4, 47–51.
Manishimwe, H., Shivoga, W. A., and Nsengimana, V. (2023). Enhancing students’ achievement in biology using inquiry-based learning in Rwanda. Int. J. Eval. Res. Educ. 12, 233–243. doi: 10.11591/ijere.v12i2.2337
Marshall, J. C., Horton, R., Igo, B. L., and Switzer, D. M. (2009). K-12 science and mathematics teachers’ beliefs about and use of inquiry in the classroom. Int. J. Sci. Math. Educ. 7, 575–596. doi: 10.1007/s10763-007-9122-7
Martin, M., Mullis, I. V., Gonzalez, E. J., Gregory, K. D., Smith, T. A., Chrostowski, S. J., et al. (2000). TIMSS 1999. International science report.
Mayer, R. E. (2004). Should there be a three-strikes rule against pure discovery learning? The case for guided methods of instruction. Am. Psychol. 59, 14–19. doi: 10.1037/0003-066X.59.1.14
Mehalik, M. M., Doppelt, Y., and Schuun, C. D. (2008). Middle-school science through design-based learning versus scripted inquiry: better overall science concept learning and equity gap reduction. J. Eng. Educ. 97, 71–85. doi: 10.1002/j.2168-9830.2008.tb00955.x
Minner, D. D., Levy, A. J., and Century, J. (2010). Inquiry-based science instruction—what is it and does it matter? J. Res. Sci. Teach. 47, 474–496. doi: 10.1002/tea.20347
Moore, T. J., and Smith, K. A. (2014). Advancing the state of the art of STEM integration. J. STEM Educ. Innov. Res. 15:5.
National Research Council (2009) in Engineering in K-12 Education: Understanding the Status and Improving the Prospects. eds. L. Katehi, G. Pearson, and M. Feder (Washington, DC: The National Academies Press).
National Research Council of the National Academies (2011). Framework for K-12 Science Education. Washington, DC: The National Academies Press.
Nelson, D. (1984). Transformations: Process and theory . Santa Monica, CA: Center for City Building Educational Programs.
Oje, O., Adesope, O., and Oje, A. V. (2021). “Work-in-Progress: The effects of hands-on learning on STEM Students' motivation and self-efficacy: a meta-analysis” in American Society for Engineering Education Conference.
Oliveira, H., and Bonito, J. (2023). Practical work in science education: a systematic literature review. Front. Educ. 8:1151641. doi: 10.3389/feduc.2023.1151641
Pant, H. A., Stanat, P., Schroeders, U., Roppelt, A., Siegle, T., and Pöhlmann, C. (2013). IQB-Ländervergleich 2012: Mathematische und naturwissenschaftliche Kompetenzen am Ende der Sekundarstufe I [IQB State Comparison 2012: Maths and Science Skills at the End of Lower Secondary Level]. Waxmann, Münster.
Patall, E. A., Cooper, H., and Robinson, J. C. (2008). The effects of choice on intrinsic motivation and related outcomes: a meta-analysis of research findings. Psychol. Bull. 134, 270–300. doi: 10.1037/0033-2909.134.2.270
Potvin, P., and Hasni, A. (2014). Interest, motivation and attitude towards science and technology at K-12 levels: a systematic review of 12 years of educational research. Stud. Sci. Educ. 50, 85–129. doi: 10.1080/03057267.2014.881626
Ray, R. (2015). STEM education and economic performance in the American States. Available online at: https://mpra.ub.uni-muenchen.de/65517 (Accessed June 02, 2024)
Reeve, J., Nix, G., and Hamm, D. (2003) Testing models of the experience of self-determination in intrinsic motivation and the conundrum of choice. J. Educ. Psychol . 95, 375–392. doi: 10.1037/0022-0663.95.2.375
Reiser, M., Binder, M., and Weitzel, H. (2021). “StartlearnING-an example for cross-domain learning arrangements combining engineering and biology” in 2021 IEEE integrated STEM education conference (ISEC) (pp. 272–275). IEEE.
Reiser, M., Binder, M., and Weitzel, H. (2023) “Startlearn ING“und die Ambivalenz eines domänenübergreifenden Lernarrangements [startlearn ING" and the ambivalence of a cross-domain learning arrangement]. Peer Tec der DGTB: Available online at: https://dgtb.de/arbeitsgruppen/publikationen/technikbildung-pdf/ (Accessed June 03, 2014)
Reiser, M., Binder, M., and Weitzel, H. (2024a). Development and validation of a measure instrument for the understanding of structural-functional correlations of the locomotor system (MUSCLS). J. Biol. Educ. doi: 10.1080/00219266.2024.2351380
Reiser, M., Binder, M., and Weitzel, H. (2024b). Effects of design-based learning arrangements in cross-domain, integrated STEM lessons on the intrinsic motivation of lower secondary pupils. Educ. Sci. 14:607. doi: 10.3390/educsci14060607
Reusser, K. (2005). Problemorientiertes Lernen.–Tiefenstruktur, Gestaltungsformen, Wirkung [Problem-Orientated Learning—In-Depth Structure, Design Forms, Effect]. Beiträge Lehrerbild. 23, 159–182.
Reusser, K. (2006). “Constructivism—from epistemological term to a renewal of didactical culture” in Didactics Based on Psychological Theories. From Hans Aeblis’ Cognitive Psychological Didactic to Modern Teaching and Learning Research. eds. M. Baer, M. Fuchs, P. Füglister, K. Reusser, and H. Wyss (Bern), 151–168.
Sanders, M. (2012). “Integrative STEM education as “best practice”” in Explorations of Best Practice in Technology, Design, and Engineering Education. ed. H. Middleton , vol. 2 (Queensland, Australia: Griffith Institute for Educational Research), 103–117.
Schmiemann, P., Linsner, M., Wenning, S., and Sandmann, A. (2012). Lernen mit biologischen Basiskonzepten. Der Math. Naturwissensch Unterricht 65, 105–109.
Schwichow, M., Zimmerman, C., Croker, S., and Härtig, H. (2016). What students learn from hands-on activities. J. Res. Sci. Teach. 53, 980–1002. doi: 10.1002/tea.21320
Smetana, L. K., and Bell, R. L. (2012). Computer simulations to support science instruction and learning: a critical review of the literature. Int. J. Sci. Educ. 34, 1337–1370. doi: 10.1080/09500693.2011.605182
So, W. W. M., Zhan, Y., Chow, S. C. F., and Leung, C. F. (2018). Analysis of STEM activities in primary students’ science projects in an informal learning environment. Int. J. Sci. Math. Educ. 16, 1003–1023. doi: 10.1007/s10763-017-9828-0
Steinmayr, R., Weidinger, A. F., Schwinger, M., and Spinath, B. (2019). The importance of students’ motivation for their academic achievement–replicating and extending previous findings. Front. Psychol. 10:1730. doi: 10.3389/fpsyg.2019.01730
Sternberg, R. J., and Grigorenko, E. L. (2003). Teaching for successful intelligence: principles, procedures, and practices. J. Educ. Gift. 27, 207–228. doi: 10.1177/016235320302700206
Su, Y., and Reeve, J. (2011). A meta-analysis of the effectiveness of intervention programs designed to support autonomy. Educ. Psychol. Rev. 23, 159–188. doi: 10.1007/s10648-010-9142-7
Su, R., and Rounds, J. (2015). All STEM fields are not created equal: people and things interests explain gender disparities across STEM fields. Front. Psychol. 6:189. doi: 10.3389/fpsyg.2015.00189
Swarat, S., Ortony, A., and Revelle, W. (2012). Activity matters: understanding student interest in school science. J. Res. Sci. Teach. 49, 515–537. doi: 10.1002/tea.21010
Tipmontiane, K., and Williams, P. J. (2022). The integration of the engineering design process in biology-related STEM activity: a review of Thai secondary education. ASEAN J. Sci. Eng. Educ. 2, 1–10. doi: 10.17509/ajsee.v2i1.35097
Triona, L. M., and Klahr, D. (2003). Point and click or grab and heft: comparing the influence of physical and virtual instructional materials on elementary school students’ ability to design experiments. Cogn. Instr. 21, 149–173. doi: 10.1207/S1532690XCI2102_02
Vooren, M., Haelermans, C., Groot, W., and van den Brink, H. M. (2022). Comparing success of female students to their male counterparts in the STEM fields: an empirical analysis from enrollment until graduation using longitudinal register data. Int. J. STEM Educ. 9, 1–17. doi: 10.1186/s40594-021-00318-8
Weng, C., Chen, C., and Ai, X. (2023). A pedagogical study on promoting students' deep learning through design-based learning. Int. J. Technol. Des. Educ. 33, 1653–1674. doi: 10.1007/s10798-022-09789-4
Wijnia, L., Loyens, S. M., and Derous, E. (2011). Investigating effects of problem-based versus lecture-based learning environments on student motivation. Contemp. Educ. Psychol. 36, 101–113. doi: 10.1016/j.cedpsych.2010.11.003
Wilde, M., Bätz, K., Kovaleva, A., and Urhahne, D. (2009). Überprüfung einer Kurzskala intrinsischer motivation (KIM): testing a short scale of intrinsic motivation. ZfDN 15, 31–45.
Wittwer, J., and Renkl, A. (2008). Why instructional explanations often do not work: a framework for understanding the effectiveness of instructional explanations. Educ. Psychol. 43, 49–64. doi: 10.1080/00461520701756420
Wittwer, J., and Renkl, A. (2010). How effective are instructional explanations in example-based learning? A meta-analytic review. A meta-analytic review. Educ. Psychol. Rev. 22, 393–409. doi: 10.1007/s10648-010-9136-5
Wu, Y.-T., and Tsai, C.-C. (2005). Effects of constructivist-oriented instruction on elementary school students’ cognitive structures. J. Biol. Educ. 39, 113–119. doi: 10.1080/00219266.2005.9655977
Xie, Y., and Killewald, A. A. (2012). Is American Science in Decline? Cambridge: Harvard Univ. Press, 2012.
Keywords: integrated STEM-education, learning biology, design-based learning, crossdomain, conceptual knowledge
Citation: Reiser M, Binder M and Weitzel H (2024) Design as a learning opportunity in biology lessons—a cross-domain STEM approach and its impact on biological subject knowledge. Front. Educ. 9:1462822. doi: 10.3389/feduc.2024.1462822
Edited by:
Gladys Sunzuma, Bindura University of Science Education, ZimbabweCopyright © 2024 Reiser, Binder and Weitzel. This is an open-access article distributed under the terms of the Creative Commons Attribution License (CC BY). The use, distribution or reproduction in other forums is permitted, provided the original author(s) and the copyright owner(s) are credited and that the original publication in this journal is cited, in accordance with accepted academic practice. No use, distribution or reproduction is permitted which does not comply with these terms.
*Correspondence: Markus Reiser, bWFya3VzLnJlaXNlckBwaC13ZWluZ2FydGVuLmRl