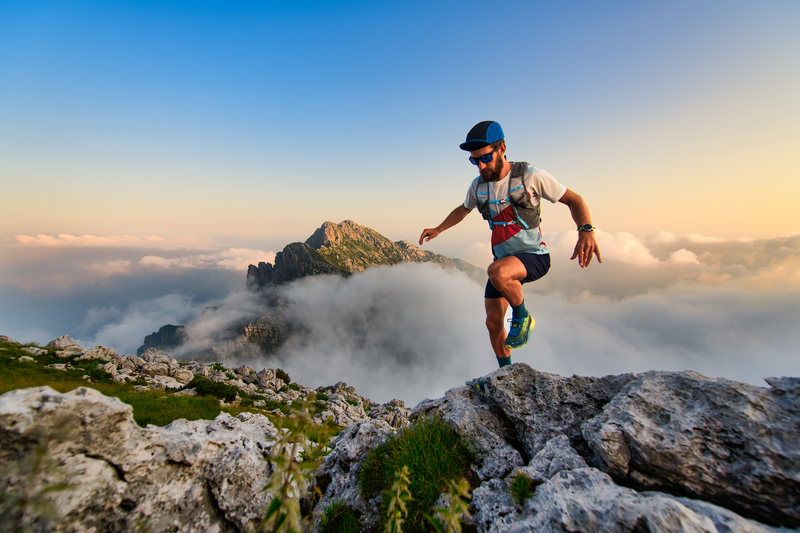
94% of researchers rate our articles as excellent or good
Learn more about the work of our research integrity team to safeguard the quality of each article we publish.
Find out more
BRIEF RESEARCH REPORT article
Front. Educ. , 18 September 2024
Sec. STEM Education
Volume 9 - 2024 | https://doi.org/10.3389/feduc.2024.1452570
This study assesses the capabilities of OpenAI’s ChatGPT-4 and ChatGPT-4o in solving mathematics problems from the National Assessment of Educational Progress (NAEP) across grades 4, 8, and 12. Results indicate that ChatGPT-4o slightly outperform ChatGPT-4 and both models generally surpass U.S. students’ performance across all grades, content areas, item type, and difficulty level. However, both models perform worse on geometry and measurement than on algebra and face more difficulties with high-difficulty mathematics items. This investigation highlights the strengths and limitations of AI as a supplementary educational tool, pinpointing areas for improvement in spatial intelligence and complex mathematical problem-solving. These findings suggest that while AI has the potential to support instruction in specific mathematical areas like algebra, there remains a need for careful integration and teacher-mediated strategies in areas where AI is less effective.
Artificial Intelligence (AI) has become increasingly essential in educational applications, notably enhancing personalized learning, adaptive assessments, and interactive environments. Tools such as adaptive learning systems, intelligent tutoring, robotics, virtual tutors, and game-based learning are transforming educational practices by providing dynamic interactions and tailored experiences that adapt to individual learning preferences (Chen et al., 2020; Nurwahid and Ashar, 2024). These models are potentially effective in alleviating math anxiety and enhancing confidence (Inoferio et al., 2024; Kumar et al., 2023), thereby improving math learning outcomes (Fang et al., 2019; Hwang, 2022).
The integration of large language models (LLMs) like GPT into educational settings introduces new potential that extends beyond the capabilities of previous AI tools (Kasneci et al., 2023; Yan et al., 2024). While LLMs are adept at generating coherent and contextually appropriate responses, their ability to process complex language queries and provide explanations offers a promising avenue for enhancing educational content delivery (Huber et al., 2024; Kasneci et al., 2023). These models are designed to synthesize information across diverse domains, potentially offering more personalized learning experiences and support (Huber et al., 2024; Kasneci et al., 2023).
However, it’s important to approach their capabilities with an understanding of the current technological limitations. While promising, LLMs are not infallible and their performance can vary significantly depending on the task and the specificity of the data they have been trained on (Kasneci et al., 2023; Rane, 2023). Challenges such as ensuring the accuracy of AI-generated content, addressing biases in the training data, and maintaining an appropriate balance between automated and human instruction are critical to their effective deployment (Huber et al., 2024; Yan et al., 2024). As LLMs become more integrated into educational systems, a clear understanding of their functionalities and limitations is essential to leverage their benefits effectively and responsibly.
Recent studies highlight the expanding use of AI in U.S. schools: 18% of teachers currently utilize AI, with another 15% having tried it, and about 60% of districts plan to increase AI training by late 2024 (Diliberti et al., 2024). AI is heavily used in STEM and English language arts, notably in platforms like Google Classroom (80%), adaptive systems such as Khan Academy and i-Ready (61%), and AI-driven chatbots (51%) (Diliberti et al., 2024). Further, 47% of education leaders use AI daily, and 68% of educators have tried it at least once (Microsoft Education Team, 2024). A U.S. Census Bureau survey also shows AI’s broad adoption across various sectors, including its integration into educational administrative processes (McElheran et al., 2024). These findings affirm the significant role of AI in shaping educational practices and policies.
The application of AI in education, especially mathematics, has been well-documented, with meta-analyses showing the impacts of various AI systems. These systems, including intelligent tutoring and robotics, typically demonstrate an average effect size of 0.35 on learning outcomes among elementary students (Hwang, 2022). Intelligent tutoring systems, for example, are more effective than no instruction, showing an effect size of 0.33, but offer negligible benefits compared to traditional human instruction among secondary or postsecondary students (Fang et al., 2019). Interestingly, while chatbots exhibit an average effect size of 0.48 (Alemdag, 2023) and 0.96 (Wu and Yu, 2024) across various performance outcomes, their impact on mathematics performance has not been specifically analyzed by meta-analysis studies, indicating a potential area for further research.
While traditional AI applications facilitate basic tutoring and feedback mechanisms, they often struggle with the complex, open-ended problem-solving required in advanced mathematics (Hashim et al., 2022). These traditional systems typically rely on predefined algorithms and datasets that may not effectively adapt to the unpredictable variables present in advanced mathematics (Hashim et al., 2022). This limitation becomes apparent in scenarios that require high levels of reasoning, logical deduction, and real-world problem integration−areas where AI has traditionally struggled (Davis, 2024; Dahal et al., 2024).
LLMs like GPT-4 and its optimized variant, GPT-4o, introduce new dimensions to AI applications in education, particularly in mathematics. Unlike their predecessors, these models are built on more expansive and diverse training datasets and advanced algorithms that allow for a deeper understanding and integration of complex mathematical concepts (Henry, 2024; Hagendorff et al., 2023). They are designed to provide human-like responses that are not only contextually relevant and highly personalized, enhancing their utility in educational settings (Huber et al., 2024; Yan et al., 2024).
For instance, GPT-4 has been effective in tackling complex mathematical challenges by synthesizing various principles to provide contextually relevant and logically sound solutions. This represents a notable progression from previous models, which often struggled with integrating and contextualizing different mathematical concepts (McClure, 2024). Studies have shown that GPT-4 successfully solves up to 84.3% of challenging competition-level mathematics problems, demonstrating a significant improvement from the capabilities of its predecessors (McClure, 2024).
GPT-4o is optimized for faster response times and includes improvements in its pattern recognition algorithms, which enhance its effectiveness in processing complex text-based mathematical equations and word problems (Ofgang, 2024). While there are discussions in popular articles about potential multimedia capabilities (Noone, 2024; Ofgang, 2024), it’s important to clarify that GPT-4o, fundamentally, remains a text-based model designed to process and generate text. Any multimedia processing would require additional systems to convert inputs into text before GPT-4o can interpret them. The reported capabilities for handling multimedia inputs and outputs need to be extensively validated in research settings.
Preliminary findings from classroom settings suggest that interactions with GPT-4’s explanations can lead to improved understanding and confidence in handling complex mathematics, showing potential advantages over traditional teaching methods (Kumar et al., 2023). While these observations are promising, it is important to approach them with caution, as the real-world applicability and scalability of these models in diverse educational environments remain to be fully proven.
Advancements in LLMs like ChatGPT-4 and ChatGPT-4o have shown significant promise, yet it’s essential for educators and students to recognize these models’ limitations to effectively leverage their capabilities. Notable issues include the generation of incorrect information, referred to as “hallucinations” (Kumar et al., 2023), and observed variability in problem-solving effectiveness over time (Chen et al., 2024). Furthermore, these models encounter difficulties with complex mathematical problems, crucial for advanced mathematics education (Hagendorff et al., 2023; Huber et al., 2024; Remoto, 2024; University of Copenhagen-Faculty of Science, 2024).
The real-world effectiveness of these models, particularly under rigorous assessment conditions such as those provided by the National Assessment of Educational Progress (NAEP) mathematics exams—often referred to as the “National Report Card”—remains to be thoroughly assessed. The study utilizes NAEP exams to evaluate these LLMs due to their comprehensive and rigorous nature, serving as robust benchmarks for testing AI capabilities within educational settings. The standardized nature of NAEP exams ensures comparability across various states and over time, spanning a wide range of topics and complexities. This diversity and standardization are vital for assessing AI’s adaptability and problem-solving proficiency in realistic educational contexts. NAEP’s objective measurement of educational achievement, free from curriculum biases, provides a solid foundation for assessing AI performance and allows for detailed comparative analysis between AI and student performances. Collectively, these aspects validate the study’s findings and underscore the potential and limitations of AI applications in education.
The NAEP administers tests to students in 4th and 8th grades every two years, while assessments for 12th grade occur less frequently (NAEP, 2017). These grades represent critical stages in the U.S. educational system, capturing early learning, middle school transitions, and pre-college competencies. This range allows for testing AI across developmental phases in mathematical understanding. Each grade level reflects distinct curricular milestones, enabling an examination of how well AI can adapt to different educational standards and expectations.
The NAEP mathematics items encompass a wide range of mathematical topics such as algebra, data analysis and statistics, geometry, measurement, and number properties and operations, reflecting the broad scope of mathematical knowledge. For 4th Grade, NAEP mathematics items focus on basic arithmetic, simple geometry, and initial problem-solving skills. For 8th Grade, it introduces algebraic concepts, more complex geometry, and data interpretation. For 12th Grade, it covers advanced algebra, calculus, statistics, and more sophisticated mathematical reasoning.
Each item was categorized by NAEP as ‘easy’, ‘medium’, or ‘hard’. NAEP categorizes items based on the complexity of skills tested1, the cognitive processes required, and historical performance data indicating how students typically perform on these items. This classification helps in assessing AI’s capability to handle a range of simple to complex problems, paralleling human performance across these categories.
NAEP mathematics items were further classified into one of four response types: ECR (Extended Constructed Response): Requires detailed, extended answers, testing the respondent’s ability to generate comprehensive, long-form responses; MC (Multiple Choice): Tests the respondent’s quick decision-making and recognition of correct answers from set options. SCR (Short Constructed Response): Needs concise, direct answers, assessing the respondent’s precision in brief responses. SR (Selected Response): Similar to multiple choice but may include true/false or yes/no answers, assessing basic recognition skills. These items were selected to ensure a representation of various mathematical skills and cognitive processes evaluated in standardized testing environments.
The study employed items from the latest available NAEP mathematics exams, specifically the 2022 assessments for 4th (30 items) and 8th grades (30 items) and the 2013 (10 items) and 2009 assessment for 12th grade (27 items), retrieved from NAEP’s question tool (National Center for Education Statistics [NCES], 2022). All items from the selected NAEP mathematics exams are included.
This selection of ChatGPT-4 and ChatGPT-4o for our study reflects their widespread adoption in educational environments, as documented by Diliberti et al. (2024). The preference for these models is based on their accessibility via the user-friendly ChatGPT interface, which is more commonly utilized by teachers and students than the more complex GPT API. This focus ensures our research assesses the practical utility and impact of AI tools as they are integrated and used within educational settings.
ChatGPT-4 (OpenAI, 2023) and ChatGPT-4o (OpenAI, 2024) were tasked with responding to these items using a standardized prompt designed to simulate a high-performing student’s test-taking scenario: “Hi ChatGPT, imagine you’re one of the top math students currently taking a mathematics test. Please do your best to answer the following questions and explain your reasoning for each one to help me understand better.” All the test questions were provided one after another to ChatGPT-4 and ChatGPT-4o. The responses generated by ChatGPT-4 and ChatGPT-4o were evaluated against the official answer keys provided by NAEP to determine correctness.
We conducted a descriptive analysis to determine the distribution of correct and incorrect responses across grades, content areas, and item types. This preliminary analysis revealed variations in response patterns, which informed the configuration of our multiple logistic regression model.
To analyze the performance differences between ChatGPT-4 and ChatGPT-4o, a multiple logistic regression was employed. This model was used to analyze the binary outcomes of mathematics item responses (correct = 1, incorrect = 0), allowing for the assessment of the probability of a correct answer based on several predictors: AI model indicator (ChatGPT-4o vs. ChatGPT-4), grade level, content area, question type, and item difficulty. We employed the Variance Inflation Factor (VIF) to assess multicollinearity among the predictors in our logistic regression model, ensuring that correlations between variables do not unduly influence our findings.
The multiple logistic regression model can be expressed as:
The intercept, β0, represents the baseline log-odds of a correct answer by ChatGPT-4 on easy algebra items for the 12th grade in the ECR format. Each coefficient, βi, in this model quantifies the log-odds effect of the corresponding predictor on the probability of a correct answer, controlling for the influence of other factors in the model.
Significant coefficients were evaluated for their effect size and direction to determine how each factor influences the likelihood of a correct response. Positive coefficients indicate a higher likelihood of a correct answer, whereas negative coefficients suggest a decreased likelihood. The decision to exclude interaction terms was based on preliminary analyses that showed minimal interaction effects between predictors. This simplification was made to maintain model clarity and focus on primary effects.
Before delving into the logistic regression analysis, it is essential to present a detailed breakdown of the performance data, which serves as the basis for employing such a model. Here, we analyze the performance of ChatGPT-4 and ChatGPT-4o across different grades, content areas, question types, and difficulty levels, and compare these results with the performance of U.S. students on the same NAEP tests (Table 1).
Descriptive statistics reveal that ChatGPT-4o sightly outperformed ChatGPT-4 and both surpassed student performance by significant margins across different grades, content areas, question types, and difficulty levels. In algebra, data analysis, statistics, and probability, and number properties and operations, the models markedly outperformed students with AI accuracy rates almost doubled student accuracy rates. However, in geometry and measurement, their performance is higher than student outcomes but close to student performance. This pattern was also evident in the performance differences by question type, where AI models outperformed student to a much lesser degree on MC questions than on other types. The AI models demonstrated more superior performance compared to students on median or hard items than on easy items.
The VIF results indicate that there is no significant multicollinearity among the predictors in the model, with all VIF values well below the commonly used threshold of concern (5 or 10), suggesting that the predictors in the model can be considered sufficiently independent for robust analysis.
The Likelihood Ratio Test results indicate that incorporating the main effects of AI model, content areas, grades, question type, and difficulty levels into the GLM significantly enhances the model’s ability to predict accuracy compared to a null model (p = 0.0002904). Furthermore, McFadden’s Pseudo- R2 value of 0.16 suggests that the model with these predictors explains approximately 16% more variance in accuracy than the null model alone, demonstrating a moderate improvement in model fit and confirming the relevance of these factors in the analysis.
Although ChatGPT-4o exhibited a slightly higher overall accuracy rate compared to ChatGPT-4 (76% vs. 70%), the results indicate that this difference is not statistically significant (Table 2). The analysis did not reveal statistically significant differences in accuracy rates by grade level or question type. However, the AI models demonstrated notably poorer performance in geometry and measurement compared to algebra. Specifically, the odds ratios of 0.18 for geometry and 0.21 for measurement indicate that the odds of these AI models answering correctly in these areas is only 18% and 21%, respectively, of the odds of answering algebra questions correctly, when all other factors are held constant.
The results of this study reveal capabilities and limitations of AI models, particularly ChatGPT-4 and ChatGPT-4o, in mastering various mathematical concepts across grade levels. The models excel in computational tasks and procedural logic, as seen in their adept handling of algebra and number properties. However, their performance in subjects requiring spatial reasoning, such as geometry and measurement, as well as in complex problem-solving scenarios, highlight limitations, revealing critical gaps in AI’s educational utility.
The study highlights how geometry and measurement present unique challenges for text-based AI models even for GPT-4o which has claimed to have better visual capability than GPT-4. These content areas require spatial reasoning and the ability to process visual information, which are capabilities not naturally suited to AI models that primarily handle text. The necessity to interpret diagrams and visualize spatial relationships, which are poorly represented in textual formats, leads to significant performance decrements. Furthermore, the predominance of text in AI training datasets limits these models’ exposure to and proficiency with spatially oriented content, which is crucial for subjects like geometry. This misalignment is compounded by architectural limitations where current AI models excel in recognizing patterns within text data but struggle with abstract and visual-spatial reasoning.
Hard questions expose the limitations in AI’s problem-solving capabilities, as these often require integrating multiple concepts and processing a higher cognitive load. Such tasks demand a deep understanding and synthesis of information, which can be challenging if the AI’s training data do not adequately cover the requisite depth or scope of topics.
The findings from this analysis provide crucial insights for educators and policymakers integrating AI into educational frameworks. Recognizing AI’s strengths enables the design of instructional strategies that utilize AI for routine tasks such as algebra and number operations, thereby freeing human instructors to concentrate on more complex pedagogical duties. Conversely, identifying AI’s limitations allows for targeted human-led instruction in areas like geometry and measurement, ensuring students receive a comprehensive and high-quality education.
Incorporating education on AI’s limitations within curricula is crucial. This strategy not only equips students to utilize these technologies effectively but also emphasizes the importance of human judgment in complex scenarios, including ethical dilemmas and advanced problem-solving tasks. Enhancing awareness of AI’s boundaries helps foster an educational environment that values human intellectual capacities and critical thinking—skills that remain indispensable in domains AI cannot yet master. Graham (2006) underscores the pedagogical importance of understanding technological limits, bolstering the case for such educational content.
It is imperative that educational policies emphasize the development of spatial reasoning and complex problem-solving skills—areas where AI tools typically underperform yet are essential for addressing real-world challenges. Innovative teaching methods that prioritize these skills are vital. For instance, Sorby et al. (2022) and Wei et al. (2024) have demonstrated a strong correlation between spatial abilities and success in STEM fields, suggesting that spatial skills interventions can substantially enhance mathematical problem-solving capabilities and future STEM achievements.
AI should complement, not replace, traditional educational methods, serving as a supplemental tool that enhances teaching efficacy. This “human in the loop” approach supports the integration of AI in handling well-defined tasks while ensuring that complex, abstract, and creative content delivery remains the province of human educators. Such a balanced model optimally leverages technological and human resources, permitting timely educator interventions to refine AI outputs.
Given the variability in AI’s performance, there is a pressing need to reevaluate the assessment strategies currently used in educational settings. Traditional assessments, especially those easily managed by AI, may fail to capture the full breadth of a student’s understanding. Educators should consider adopting more varied and inventive assessment methods, such as project-based learning, portfolios, and open-ended tasks, which more accurately reflect student comprehension and capabilities (Arter and Spandel, 1992; Balleisen et al., 2024; Bartholomew and Strimel, 2018).
Educators must engage in continuous professional development to keep pace with rapidly evolving technological tools and pedagogical methods. Supportive policies should underpin training programs that not only instruct on AI utilization but also on its effective integration with traditional teaching practices to maximize educational outcomes. These initiatives should promote a pedagogical evolution that adjusts to technological advancements without undue reliance on them.
As AI technologies continue to evolve, educational strategies must adapt to prepare students for future landscapes where AI is more prevalent. Policies should focus on cultivating skills in students that AI is unlikely to master soon, such as ethical decision-making, creativity, and interpersonal skills. Strategic investments in curriculum development that anticipates shifts in the workforce and technological trends are essential. This proactive approach will ensure that students are not only prepared to use AI effectively but also to excel in areas where human expertise remains irreplaceable.
Future research should focus on enhancing AI’s ability to manage complex, multimodal problem-solving tasks, including the integration of advanced neural network architectures that enhance visual processing, abstract reasoning, and decision-making. Studies could aim to develop AI models that more closely emulate human cognitive processes, especially in interpreting complex visual inputs and creatively synthesizing this information to solve problems.
Investigations should also consider how AI can be seamlessly integrated with traditional teaching methods to function as a dynamic teaching assistant, providing real-time adjustments to learning paths based on student performance and engagement. Research could further assess the impact of AI-driven personalized learning environments on student learning outcomes in various educational settings.
Longitudinal studies are essential to assess the enduring impacts of AI within educational systems. Such research could compare the performance, engagement, and learning outcomes of students over several years, contrasting groups that use traditional methods with those employing AI-enhanced approaches, to provide insights into the sustainability and long-term effectiveness of AI in education.
Further research is needed to understand how AI technologies are transforming the roles and responsibilities of educators, including their perceptions of AI, changes in instructional strategies, and the professional development required to integrate AI tools effectively.
It is crucial to explore the ethical dimensions of AI use in education, focusing on issues such as privacy, data security, and potential biases in AI algorithms. Research should also scrutinize the equity of AI educational tools to ensure they do not exacerbate existing educational disparities but rather contribute to a more inclusive educational environment.
Engaging in cross-disciplinary research that incorporates cognitive science, education theory, and computer science could lead to more holistic AI solutions tailored for educational purposes. These collaborations could foster innovations that are both technologically advanced and pedagogically sound, ensuring that AI tools are effectively adapted to the complex realities of classroom environments.
Given the global impact of AI in education, comparative studies across different nations and educational systems are vital. These studies could identify best practices and cultural considerations in AI integration, informing international policy-making and promoting global cooperation in the development of AI educational technologies that are culturally sensitive and globally accessible.
While this study underscores the potential of AI in enhancing areas like algebra and numerical operations, it also reveals significant challenges in spatial reasoning and complex mathematical problem-solving. A balanced approach to integrating AI within educational frameworks, supported by continuous research and development, is essential. By proactively addressing these challenges, the educational community can ensure that AI acts as a beneficial adjunct to human creativity, enhancing the educational experience for all students.
The raw data and programming code supporting the conclusions of this article are available in the Supplementary material.
XW: Conceptualization, Data curation, Formal analysis, Funding acquisition, Investigation, Methodology, Project administration, Resources, Software, Supervision, Validation, Visualization, Writing – original draft, Writing – review and editing.
The author(s) declare financial support was received for the research, authorship, and/or publication of this article. The research reported here was supported by the Institute of Education Sciences, U.S. Department of Education, through Grant R324P230002 to Digital Promise. The opinions expressed are those of the authors and do not represent the views of the Institute or the U.S. Department of Education.
The author declares that the research was conducted in the absence of any commercial or financial relationships that could be construed as a potential conflict of interest.
All claims expressed in this article are solely those of the authors and do not necessarily represent those of their affiliated organizations, or those of the publisher, the editors and the reviewers. Any product that may be evaluated in this article, or claim that may be made by its manufacturer, is not guaranteed or endorsed by the publisher.
The Supplementary Material for this article can be found online at: https://www.frontiersin.org/articles/10.3389/feduc.2024.1452570/full#supplementary-material
Alemdag, E. (2023). The effect of chatbots on learning: A meta-analysis of empirical research. J. Res. Technol. Educ. 58, 1–23. doi: 10.1080/15391523.2023.225569
Arter, J. A., and Spandel, V. (1992). Using portfolios of student work in instruction and assessment. Educ. Meas. 11, 36–44. doi: 10.1111/j.1745-3992.1992.tb00230
Balleisen, E. J., Howes, L., and Wibbels, E. (2024). The impact of applied project-based learning on undergraduate student development. High Educ. 87, 1141–1156.
Bartholomew, S. R., and Strimel, G. J. (2018). Factors influencing student success on open-ended design problems. Int. J. Technol. Design Educ. 28, 753–770. doi: 10.1007/s10798-017-9415-2
Chen, L., Zaharia, M., and Zou, J. (2024). How is ChatGPT’s behavior changing over time? Harvard data science review. arXiv [Preprint]. doi: 10.1162/99608f92.5317da4 arXiv:2307.09009.
Chen, X., Xie, H., and Hwang, G.-J. (2020). A multi-perspective study on artificial intelligence in education: Grants, conferences, journals, software tools, institutions, and researcher. Comput. Educ. Artif. Intell. 1:100005. doi: 10.1016/j.caeai.2020.10000
Dahal, N., Luitel, B., and Pant, B. (2024). “Exploring capabilities and limitations of generative AI chatbots in solving math algorithm problems,” in Proceedings of the 15th international congress on mathematical education, (Sydney, NSW).
Davis, E. (2024). Mathematics, word problems, common sense, and artificial intelligence. Bull. Am. Math. Soc. 61, 287–303. doi: 10.1090/bull/182
Diliberti, M. K., Schwartz, H. L., Doan, S., Shapiro, A., Rainey, L. R., and Lake, R. J. (2024). Using artificial intelligence tools in K–12 classrooms. Santa Monica, CA: RAND Corporation.
Fang, Y., Ren, Z., Hu, X., and Graesser, A. C. (2019). A meta-analysis of the effectiveness of ALEKS on learning. Educ. Psychol. 39, 1278–1292. doi: 10.1080/01443410.2018.149582
Graham, C. R. (2006). Theoretical considerations for understanding technological pedagogical content knowledge (TPACK). Stud. Cult. Politics Educ. 27, 43–51. doi: 10.1080/0159630050051026
Hagendorff, T., Fabi, S., and Kosinski, M. (2023). Human-like intuitive behavior and reasoning biases emerged in large language models but disappeared in ChatGPT. Nat. Comput. Sci. 3, 833–838. doi: 10.1038/s43588-023-00527-x
Hashim, S., Omar, M. K., Jalil, H. A., and Sharef, N. M. (2022). Trends on technologies and artificial intelligence in education for personalized learning: Systematic literature review. Int. J. Acad. Res. Prog. Educ. Dev. 12, 884–903. doi: 10.6007/IJARPED/v11-i1/1223
Henry, J. (2024). ChatGPT upgrade: GPT-4 Turbo model now better at math, coding, and more is OpenAI’s chatbot becoming more human-cognitive? New York, NY: TechTimes.
Huber, S. E., Kiili, K., Nebel, S., Ryan, R. M., Sailer, M., and Ninaus, M. (2024). Leveraging the potential of large language models in education through playful and game-based learning. Educ. Psychol. Rev. 36:25. doi: 10.1007/s10648-024-09868-z
Hwang, S. (2022). Examining the effects of artificial intelligence on elementary students’ mathematics achievement: A meta-analysis. Sustainability 14:13185. doi: 10.3390/su14201318
Inoferio, H., Espartero, M., Asiri, M., Damin, M., and Chavez, J. (2024). Coping with math anxiety and lack of confidence through AI-assisted Learning. Environ. Soc. Psychol. 9:28. doi: 10.54517/esp.v9i5.222
Kasneci, E., Sessler, K., Küchemann, S., Bannert, M., Dementieva, D., Fischer, F., et al. (2023). ChatGPT for good? On opportunities and challenges of large language models for education. Learn. Individ. Differ. 103:23. doi: 10.1016/j.lindif.2023.10227
Kumar, H., Rothschild, D. M., Goldstein, D. G., and Hofman, J. (2023). Math education with large language models: Peril or promise? SSRN. doi: 10.2139/ssrn.464165
McClure, P. (2024). AI now surpasses humans in almost all performance benchmarks. Available online at: https://newatlas.com/technology/ai-index-report-global-impact/ (accessed August 12, 2024).
McElheran, K., Li, J. F., Brynjolfsson, E., Kroff, Z., Dinlersoz, E., Foster, L., et al. (2024). AI adoption in America: Who, what, and where. J. Econ. Manag. Strategy 33, 375–415. doi: 10.1111/jems.1257
Microsoft Education Team (2024). Explore insights from the AI in education report. Available online at: https://www.microsoft.com/en-us/education/blog/2024/04/explore-insights-from-the-ai-in-education-report/ (accessed August 12, 2024).
NAEP (2017). National Assessment of Education Progress (NAEP). https://nces.ed.gov/statprog/handbook/pdf/naep.pdf (accessed August 12, 2024).
National Center for Education Statistics [NCES] (2022). NAEP questions tool. Washington, DC: National Center for Education Statistics [NCES].
Noone, G. (2024). OpenAI launches GPT-4o, flaunting ability of model to detect user emotions. Available online at: https://techmonitor.ai/technology/ai-and-automation/openai-launches-gpt-4o (accessed August 12, 2024).
Nurwahid, M., and Ashar, S. (2024). A literature review: The use of Artificial Intelligence (AI) in mathematics learning. Proc. Int. Conf. Religion Sci. Educ. 3, 337–344.
Ofgang, E. (2024). GPT-4o: What educators need to know. Available online at: https://www.techlearning.com/how-to/gpt-4o-how-to-use-it-to-teach (accessed August 12, 2024)
OpenAI (2023). ChatGPT-4. Available online at: https://www.openai.com/ (accessed August 12, 2024).
OpenAI (2024). ChatGPT-4o. Available online at: https://www.openai.com/ (accessed August 12, 2024).
Rane, N. (2023). Enhancing mathematical capabilities through ChatGPT and similar generative artificial intelligence: Roles and challenges in solving mathematical problems. SSRN. doi: 10.2139/ssrn.460323
Remoto, J. (2024). ChatGPT and other AIs: Personal relief and limitations among mathematics-oriented learners. Environ. Soc. Psychol. 9:11. doi: 10.54517/esp.v9i1.191
Sorby, S. A., Duffy, G., and Yoon, S. Y. (2022). Math instrument development for examining the relationship between spatial and mathematical problem-solving skills. Educ. Sci. 12:828. doi: 10.3390/educsci1211082
University of Copenhagen-Faculty of Science (2024). New study pinpoints the weaknesses in AI. Rockville, MD: ScienceDaily.
Wei, X., Zhang, S., and Zhang, J. (2024). Identifying student profiles in a digital mental rotation task: Insights from the 2017 NAEP math assessment. Front. Educ. 9:1423602. doi: 10.3389/feduc.2024.142360
Wu, R., and Yu, Z. (2024). Do AI chatbots improve students learning outcomes? Evidence from a meta-analysis. Br. J. Educ. Technol. 55, 10–33. doi: 10.1111/bjet.1333
Keywords: artificial intelligence, ChatGPT-4, ChatGPT-4o, NAEP, mathematics education
Citation: Wei X (2024) Evaluating chatGPT-4 and chatGPT-4o: performance insights from NAEP mathematics problem solving. Front. Educ. 9:1452570. doi: 10.3389/feduc.2024.1452570
Received: 21 June 2024; Accepted: 26 August 2024;
Published: 18 September 2024.
Edited by:
Vagelis Plevris, Qatar University, QatarReviewed by:
Enkhbold Nyamsuren, University College Cork, IrelandCopyright © 2024 Wei. This is an open-access article distributed under the terms of the Creative Commons Attribution License (CC BY). The use, distribution or reproduction in other forums is permitted, provided the original author(s) and the copyright owner(s) are credited and that the original publication in this journal is cited, in accordance with accepted academic practice. No use, distribution or reproduction is permitted which does not comply with these terms.
*Correspondence: Xin Wei, eHdlaUBkaWdpdGFscHJvbWlzZS5vcmc=
Disclaimer: All claims expressed in this article are solely those of the authors and do not necessarily represent those of their affiliated organizations, or those of the publisher, the editors and the reviewers. Any product that may be evaluated in this article or claim that may be made by its manufacturer is not guaranteed or endorsed by the publisher.
Research integrity at Frontiers
Learn more about the work of our research integrity team to safeguard the quality of each article we publish.