- Systems and Computing Engineering Department, Universidad de los Andes, Bogotá, Colombia
We are interested in how metaverse technologies can provide an environment for future virtual education beyond traditional videoconferencing technologies. This study investigates learning and the quality of the classroom experience by conducting classes in four metaverse environments: Meta Horizon Workrooms, Spatial, Mozilla Hubs, and Arthur. Through the analysis of questionnaires, we assess the influence of factors such as avatars, spatial arrangement, mobility, and extra functionalities on concentration, usability, presence, and learning. While we did not observe significant differences in learning outcomes, our analysis reveals substantial variations in the quality of the classroom experience. Specifically, higher immersion, concentration, and presence levels were observed in metaverses with limited movement and functionalities. Also, our findings emphasize the positive impact of avatars with realistic facial expressions on enhancing the overall experience. Finally, we present lessons learned and offer recommendations for class configurations in the metaverse based on our questionnaire analysis.
1 Introduction
Currently, we are witnessing a growing interest in virtual reality and the metaverse, technologies that are transforming the way we interact with the digital and physical world around us. In the wake of the COVID-19 pandemic, interest in virtual classes has grown rapidly, increasing the interest in new technologies and remote learning methods to facilitate distance education effectively. Tools that enable connections in both virtual reality and on mobile devices and computers have been explored for educational purposes (Eriksson, 2021). In this regard, virtual and augmented reality can significantly impact distance education, offering a multitude of possibilities to enhance the learning experience (Eriksson, 2021). These tools demonstrate outstanding potential for quickly connecting people in different parts of the world while minimizing the costs associated with physical presence. Additionally, they provide innovative environments and reduce external distractions by offering a fully immersive experience (Netland and Hines, 2021). Among the identified benefits are increased student motivation, improved spatial representation of knowledge, and the promotion of empirical learning (Hasenbein et al., 2022). These platforms have been effectively used to create virtual learning spaces replicating and improving the traditional educational setting (Yoshimura and Borst, 2020; Schier et al., 2022). Therefore, it is crucial to examine how the design of these environments can influence learning and the quality of the experience to determine the characteristics that can benefit learning experiences and educational use.
Our research focuses on analyzing the quality of the experience and learning in four metaverse environments: Meta Workrooms (WKRM), Spatial (SPAT), Mozilla Hubs (MHUB), and Arthur (ARTH). To achieve this, we conducted four different classes on the topic of “uses of extended reality” in these metaverses, targeting a university audience. This approach allows us to gather valuable information regarding favorable and unfavorable characteristics when designing a learning environment in the metaverse. Such insights are instrumental in decision-making when implementing these technologies in the field of education. Our primary motivation is to address the following question: How does the design of a metaverse environment influence learning and the quality of the experience when taking a virtual reality class?
Given the promising potential of virtual reality to enhance connectivity and presence among individuals, even when they are physically separated, our research gains significant relevance. We analyze the various design elements that constitute the virtual reality learning experience, aiming to identify those that most positively impact learning and the quality of the educational experience. As interest in virtual reality and metaverse technologies continues to grow, it becomes imperative to understand their capabilities and limitations to optimize their application in educational contexts. Our study contributes valuable insights into how the thoughtful design of metaverse environments can support effective teaching and learning, guiding future implementations of these innovative tools in the field of education.
The rest of this article is organized into several key areas. We begin by examining related works to frame our research within the current academic landscape. Following this, we detail the materials and methods employed in our study, leading to a presentation of our results. The discussion section then interprets these findings, and we conclude with an overview of the study's limitations and potential avenues for future research.
2 Related work
The use of Virtual Reality (VR) as an educational tool has been studied in academic literature, with several works exploring various factors that can influence learning in virtual environments. First, there are several literature reviews with different approaches. Won et al. (2023) studied 219 immersive VR tools for learning. They classify learning experiences in passive immersion, interactive observation of 3D objects, immersive role-playing, learning with haptics and extra modalities, and learning with personalized feedback. Most of these learning experiences are feasible in current metaverses, and our experimental design includes elements of all these categories except haptics. Mystakidis et al. (2021) Studied learning experiences in social virtual reality environments between 2004 and 2019, predecessor environments to current metaverses. They studied the learning outcomes, instructional design methods, learning effectiveness, and influencing factors. Childs et al. (2024) studied several cases of VR and AR technologies as enablers for distance education, and they found advantages in social interaction, student engagement, and focus. In contrast, they mentioned open issues related to the creation of content and widespread acceptance by teachers, among others. Of all the factors in this paper, our study concentrates on the technological aspects of current metaverses that could influence learning. In contrast to these literature reviews, our focus is on understanding, through experimentation, how current commercial metaverses influence learning experiences since these metaverses express the state of the art in immersive environments.
Factors that have been studied in particular VR learning experiences include avatar appearance, student positioning in the classroom, and peer behavior (Castilla et al., 2023). Overall, it has been found that a position close to the teacher, either in the front or center of the classroom, can be advantageous for learning and attention (Hasenbein et al., 2022; Bailenson et al., 2008; Blume et al., 2019). Additionally, studies have revealed that greater engagement and concentration are observed when peers show signs of focus (Hasenbein et al., 2022; Bailenson et al., 2008). These findings underscore the importance of carefully considering the design of virtual environments in education to optimize students' learning experiences.
Research in educational virtual reality environments has positively impacted student interest. The viability of VR classrooms as a replacement for asynchronous video-based learning environments has been positively evaluated (White et al., 2024). Similarly, the integration of virtual reality into three Coursera courses also showed positive results, resulting in high levels of student satisfaction and engagement with virtual reality activities (Urban et al., 2023). In contrast, the acceptance and willingness of teachers to use emerging technologies, such as augmented reality (AR) and VR, in teaching have also been investigated (Jang et al., 2021). Factors such as pedagogical and technological knowledge, social norms, and motivational support were found to influence teachers' perceptions of the usefulness and ease of use of these technologies. However, there are technical and user acceptance challenges, such as the low resolution of recorded videos and difficulties in note-taking in the virtual space (Date et al., 2020).
Similarly, spatial factors that play a role in the educational experience in traditional learning environments have been examined. Previous research has investigated how seating arrangements impact teaching-learning, corroborating the findings in virtual reality studies (Smith, 2017). These studies have shown that spatial proximity to the teacher is beneficial for student performance (LaCroix and LaCroix, 2017). Other classroom configurations, such as the Gallery Walk, which allows students to interact with different learning stations in space, have been explored. Although this setup motivates student interest, it has not necessarily proven to be more effective in terms of learning outcomes (Hooi et al., 2015). In summary, both in virtual reality environments and in traditional settings, the arrangement of spaces and classroom configurations play a crucial role in students' learning experiences. Proximity in location and a focus on effectiveness remain relevant research topics in the quest to optimize the educational process. In our current research, we do not have total control of the spatial layout in each metaverse. Still, we do study how the differences in predefined spatial layouts affect the required affordances and the overall experience.
Due to the COVID-19 pandemic, various forms of distance learning have been explored, including virtual reality classes and 3D virtual worlds such as Minecraft and MHUB. The purpose of this research has been to evaluate the effectiveness of virtual reality learning features in enhancing interaction and student engagement in online classes. It has been shown that 3D virtual classrooms can provide a more immersive and effective learning experience compared to traditional classrooms (Wardani et al., 2021). Additionally, platforms like MHUB have been found particularly useful for small groups with fewer than 25 people. Furthermore, the ability to express oneself through body language, such as hand gestures, has been found to be particularly useful from the teacher's perspective (Eriksson, 2021). Similarly, a study examined the effectiveness of VR classrooms as a replacement for video-based remote learning environments (White et al., 2024). Although the study's results show that there is no significant difference in performance between VR and Augmented Virtuality (AV) environments, students perceive VR classrooms to be less distracting and more interesting. In conclusion, the pandemic has driven the exploration of innovative approaches to distance learning, revealing the potential of virtual reality and 3D virtual environments to enrich the online educational experience.
The appearance of avatars plays a crucial role in virtual interaction, and several studies have investigated their influence both in specific tasks and in virtual reality learning environments. First, in non-immersive studies, it has been observed that avatars based on human faces create a stronger sense of presence (Suk and Laine, 2023). These findings align with research on team collaboration via chat, where better results were obtained when avatars resembled the people they represented. Additionally, teams whose members were more similar to each other were found to have greater trust in their peers and shared more information (Land et al., 2015). Second, research in virtual reality (VR) and augmented reality (AR) environments has explored the influence of avatars on students' perception of teaching effectiveness. One study (Baffour and Oyekoya, 2023) examined three avatar representations: one resembling the instructor, another stick-figure type, and a video recording of the instructor, while another study (Woodworth et al., 2019) evaluated four types of avatars: a depth-based mesh video model, a human-like video game style model, a robot model, and a hybrid model with the teacher's head replaced by a video of their face. Both studies highlight the importance of avatar appearance in students' perception and suggest that realism and familiarity with the instructor are critical factors for teaching effectiveness in virtual environments. Similarly, other research has revealed that students show more significant engagement with their peers when avatars take on a more cartoonish appearance (Hasenbein et al., 2022). Finally, Yee and Bailenson (2007) showed how avatars' appearance influences human behavior in general. Although our study leaves subjects free to choose the avatar they like, our hypothesis reflects to a certain degree this study, since the appearance and affordances of avatars in each platform affect the classroom experience. Avatar appearance in virtual environments plays a pivotal role in influencing user interaction and performance. This underscores the importance of studying avatar appearance choices in virtual learning and collaboration contexts, as it can significantly impact the learning experience.
A traditionally researched topic regarding concentration and learning is the impact of interruptions. Frequent interruptions have been observed to have a slightly negative effect on the learning process, as well as increasing stress levels and leading to errors (Conard and Marsh, 2014; Eyrolle and Cellier, 2000). These findings align with studies in the field of virtual reality, which have explored how distractions or distracted behavior from other students can hinder performance (Hasenbein et al., 2022; Bailenson et al., 2008). In summary, both in traditional and virtual environments, interruptions can significantly influence concentration and learning, underscoring the importance of considering this factor when designing educational settings.
An objective way to measure attention is through eye tracking. Eye tracking analysis in virtual classroom environments can be crucial to improving the quality of education, as recent studies suggest (Shengkai et al., 2023). This approach allows for collecting and analyzing learning data, identifying areas where students struggle, and providing real-time feedback to teachers. Furthermore, understanding students' visual attention in virtual environments through the exploitation of information on objects of interest and eye tracking is essential for designing adequate learning spaces (Bozki et al., 2021). By studying virtual classroom manipulations, such as seating positions and avatar display styles, student attention to content and virtual interactions can be enhanced. These research efforts highlight the potential of VR technology and eye tracking to transform education and improve student learning experiences.
Eye tracking analysis in virtual classroom environments can be crucial in understanding how different manipulations impact students' visual attention (Bozki et al., 2021). This approach investigates how seating positions, avatar display styles, and hand-raising behaviors influence attention toward peers, instructors, and lecture content. The results suggest that manipulations in the virtual classroom significantly impact student attention, underscoring the importance of carefully considering these aspects to maximize attention to educational content. Additionally, the design of virtual classrooms also plays a crucial role in student concentration and engagement (Maruko et al., 2023). Using Kansei engineering, elements such as colors and objects are explored to create an environment that facilitates concentration in class and reduces the feeling of isolation in online lessons. Designs that evoke a warm impression tend to improve concentration and reduce feelings of isolation, highlighting the importance of customizing the environment according to sensory preferences to enhance the learning experience. Both approaches emphasize the need to consider both technological and design aspects to create compelling virtual environments that enhance students' educational experiences.
Therefore, it is crucial to continue exploring factors that can enhance experiences in the virtual classroom and the use of current metaverses for this purpose. In this article, we present an approach aimed at understanding how the design of the current metaverse environment can influence learning and the overall quality of the experience. Our research focuses on evaluating key factors, from avatar design to spatial organization and interaction techniques, within four metaverse applications used in an educational context. This approach allows us to identify design features that are most advantageous for optimizing the use of these technologies in education. Thus, we contribute to the growing body of knowledge about virtual reality in teaching, providing valuable insights for decision-making in the implementation of these tools.
3 Materials and methods
3.1 Study description
In this study, we design a comparative analysis of four metaverse applications within an educational context to gather insights into participants' learning outcomes and the quality of their experiences. The examined factors encompass perceived realism, presence, usability, interest, and the learning outcomes achieved in each metaverse during instructional sessions. Our evaluation of design aspects extends from avatar design to spatial organization, movement control, and modes of interaction within the virtual environment and among users. Consequently, the primary objective of this research is to assess how metaverse environment design can impact the learning process and the overall quality of the virtual reality class experience. Our goal is to identify the design characteristics that prove advantageous for optimizing the utilization of these technologies in educational settings.
Concerning previous research, while the design of avatars has been extensively studied, this study compares the effects of avatars in different metaverses. It takes into consideration non-human avatars that are available in current metaverses. Furthermore, we investigate the impact of emerging facial tracking technologies, such as the Meta Quest Pro, within the WKRM application, a topic that has remained unexplored in prior investigations. While research abounds on the organization of learning spaces in physical and virtual environments, previous investigations predominantly concentrate on student placement within conventional classroom settings. In contrast, our study encompasses a broader examination, encompassing various learning space configurations, including the gallery walk, conventional classrooms with predefined seating, and open exploration classrooms. Finally, there is limited research on how interaction techniques influence virtual classroom experiences. Considering the unique interaction paradigms presented by each metaverse, this investigation holds particular significance for designing and optimizing these virtual learning environments.
3.2 Selected metaverses
The metaverse applications selected for this study—WKRM, MHUB, SPAT, and ARTH—were chosen for their alignment with beneficial characteristics identified in prior research on virtual learning environments. At the moment of this research, these applications were popular and distinct, each facilitating remote interaction via avatars in a customizable metaverse space, and all were supported in immersive technologies. This selection strategy is rooted in evidence suggesting that features such as advanced facial tracking in WKRM and flexible spatial arrangements in SPAT and ARTH enhance student engagement and information retention.
The selection was further guided by the desire to explore a range of environments—from traditional setups to exploratory spaces—to investigate how different design elements impact the learning experience. Each application's unique features and their influence on realism, presence, and interaction play a crucial role in evaluating their effectiveness in educational settings, as outlined in Table 1. This approach ensures a comprehensive assessment of how virtual environments can optimize learning outcomes.
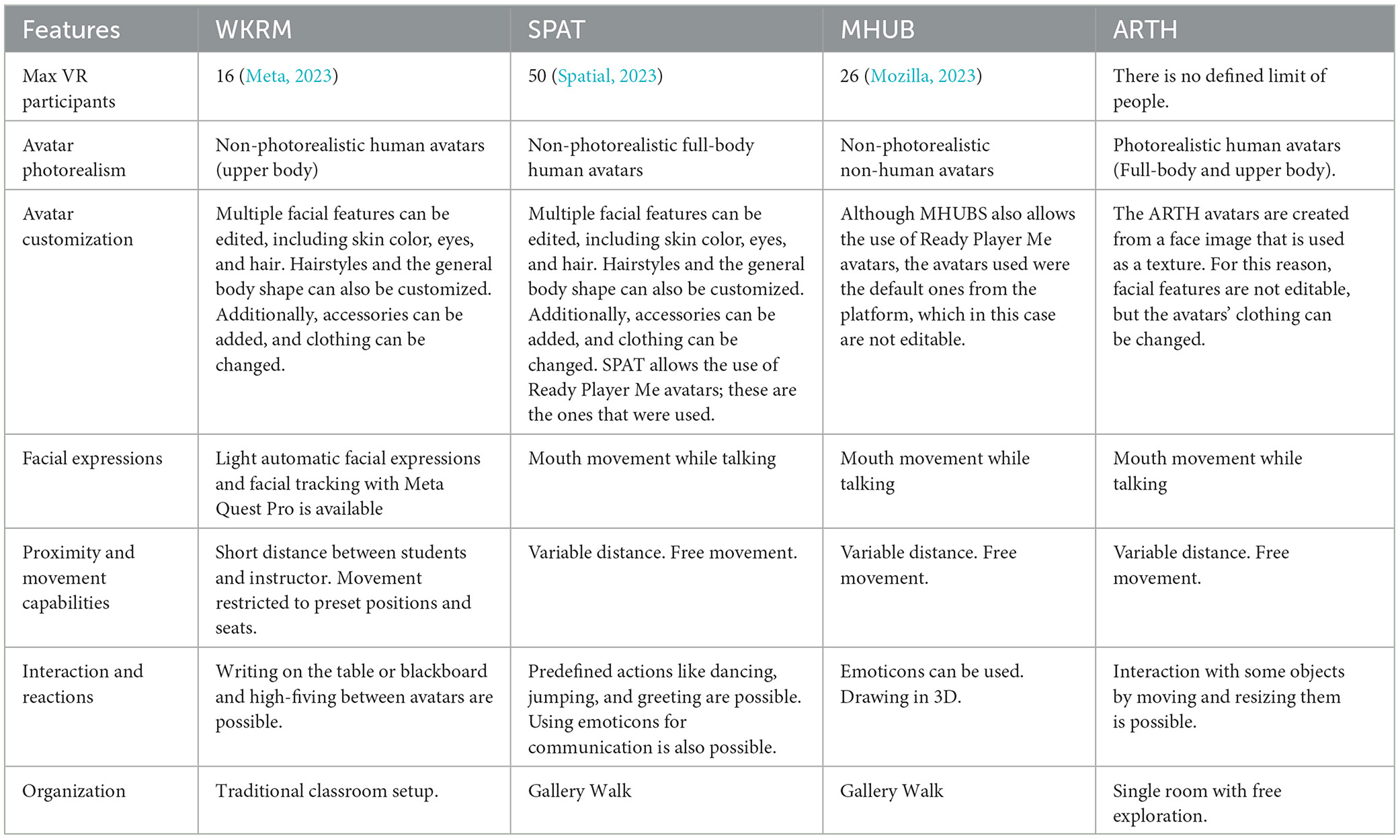
Table 1. Description of metaverse features: maximum number of participants, photorealism, facial expression, proximity, and spatial organization.
Finally, although we wanted to study as many distinct commercial metaverses as possible, we limited the number of experiences to a reasonable number for a within-subjects study. As we will mention in Section 3.4, each subject tried all experiences, and the overall experiment time was about two hours. Since our subjects were committed to us for four days and the first day and last day were committed to introductory and conclusion sessions, we designed two sessions on two consecutive days of one hour each in order to avoid fatigue.
3.3 Hypotheses
H1: Metaverses with avatars featuring more natural (or faithful) facial expressions will allow students to perceive less discomfort and more immersion. An example of how avatars look in each metaverse is shown in Figure 1. With discomfort, we mean negative results from the experience, such as less immersion, dizziness, or headache. With immersion, we suggest the feeling of being in the classroom presented in the experiment, listening to the lecturers, and being aware of the surroundings and other avatars around. We gave these definitions in similar words to subjects in questionnaires about their experience. In this regard, WKRM stands out as the application with the highest expressiveness, thanks to the possibility of face and eye tracking. Also, ARTH looks promising as it has the most photorealistic avatars from the selection since their avatars are created based on a facial photo. SPAT's avatars exhibit limited facial expression and lack photorealism, likely causing neither discomfort nor a sense of naturalness. Finally, MHUB, being the least realistic of the applications, features blinking and mouth movement synchronized with the avatars, potentially fostering greater affinity.
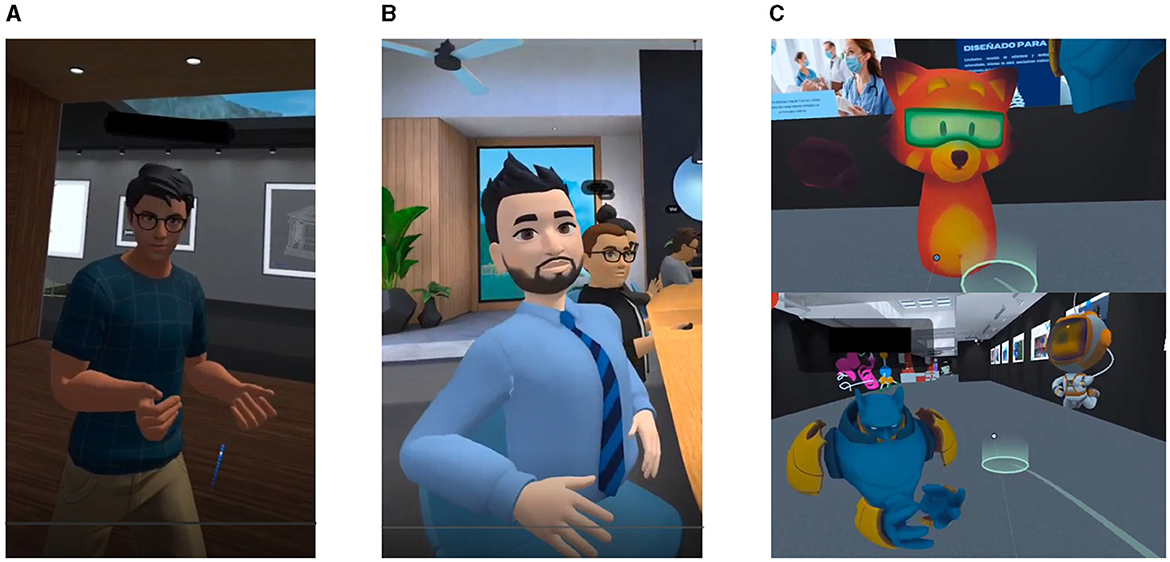
Figure 1. Examples of the avatars used in each metaverse. (A) ARTH Avatar. (B) SPAT Avatar. (C) WKRM Avatar. (D) MHUB Avatar.
H2: Applications that provide the option to sit or remain in a fixed location are less distracting and allow for greater concentration, resulting in improved learning outcomes. By distraction we mean stimuli different from the one proposed by instructors in each session. In the same way, concentration is achieved when students can pay all their attention to the stimuli proposed by instructors. Thus, it is assumed that applications like WKRM or ARTH, where the organizational structure consists of a single classroom or conference room, can enhance concentration and learning. In contrast, movement between rooms may diminish the quality of the learning experience.
H3: Applications with more interactions (walking, dancing, sending emojis, etc.) will be more distracting and negatively impact students' attention and learning. There are differences in the interaction capabilities present in the selected applications. Some of these applications enable users to perform a wide range of actions, such as walking, dancing, applauding, sending emojis and reactions, and interacting with virtual objects like writing on tables or boards. Consequently, it is expected that applications with a greater variety of functionalities may prove to be distracting and detrimental to attention and the learning process. Additionally, the need to learn how to use these functionalities could be distracting itself. WKRM is characterized by its limited interaction capacity, suggesting it may be the least distracting of the evaluated applications. In contrast, SPAT and MHUB offer predefined reactions, such as dancing or using emojis in SPAT, or drawing in MHUB. These additional interaction options could increase the risk of distraction and reduce attention during class. On the other hand, ARTH presents relatively limited interaction options, although it is possible to interact with some objects to resize and move them, placing it in an intermediate position in terms of potential distraction.
3.4 Experimental methodology
To effectively compare experiences across different metaverses, a within-subjects design was employed. This approach ensured that each participant experienced all metaverses, thus allowing a thorough evaluation of the variations between them. Different topics were addressed in each session to maintain interest and avoid monotony. Four different class configurations were conducted, each in a different metaverse and focused on a specific topic related to virtual reality applications. This design aimed to minimize the impact of topic repetition on the quality of the experience and provided a structured environment to evaluate the effectiveness of each metaverse configuration.
The topics covered in each metaverse were related to applications of virtual reality in different fields. The possibilities of virtual reality for these fields were demonstrated during each session or class, along with examples of commercial applications. The topics of each class are described in Table 2. Although the topics may have seemed very different, each session had the same format with differences in the field of application. In this way, we reduced the potential influence of repetitions on the quality of the experience. Additionally, the decision was made to vary the class topics to avoid adverse effects from the repetition of the same topic in multiple classes, as this could negatively influence the last sessions taken by each group.
The modality of all classes was lecture-based, where two instructors explained the topic, and the students listened. All classes had visual support with slides, and all except WKRM included 3D models. WKRM did not have this because the application did not support this functionality. Additionally, classes were recorded for later analysis.
The 26 students were divided into four groups to perform sessions with a small number of attendees, taking into account limitations in all metaverses. Therefore, four sessions were conducted in each metaverse, one for each group.
Each session for each group consisted of six or seven participants assuming the role of learners and two instructors. The classes had a duration of ~10 min, and surveys were employed to assess both learning outcomes and the quality of the experience. As a final step, students completed a comparative survey after attending the four sessions of the classes. The data from the first session was excluded as it was considered an introduction to this experiment. It can be argued that the duration of each session is too short for a significant comparison to current practices in online classes. However, since the hypotheses are mostly related to the experience of the sessions, 10 min per session is considered enough. Moreover, each subject takes about 25 min to have the entire experience in one metaverse, including preparation and final survey times. Making a session longer would mean taking more than 2 h per subject, which could become exhausting and negatively affect the study.
To assess students' learning, the Pre-Learning Questionnaire (PRELQ) and Post-Learning Questionnaire (POSTLQ) were administered. These assessments consisted of 5 questions related to the class topic. Although the PRELQ and POSTLQ had questions on the same topic, they differed from each other to avoid influencing the learning process during the session. The questions for the PRELQ and POSTLQ are listed in Table 3. We consider that although learning itself is not evaluated in any hypothesis, it could provide us with information about the differences in the quality of the experience and, therefore, contribute to our study of the factors that influence the dynamics and experience of the class.
Additionally, the Post-Experience Questionnaire (PEQ) was conducted to evaluate the quality of the experience when interacting with each virtual reality environment, considering aspects such as perceived realism, presence, usability, and interest. As a final step, the Comparative Final Questionnaire (FCQ) was administered, addressing the quality of the experience from a comparative perspective between applications.
The questionnaire items were selected and adapted from three sources: “SUS: A ‘Quick' and ‘Dirty' Usability Scale,” “Measuring Presence in Virtual Environments: A Presence Questionnaire,” and the questionnaire used in the study “Learning with simulated virtual classmates: Effects of social-related configurations on students' visual attention and learning experiences in an immersive virtual reality classroom” (Hasenbein et al., 2022; Witmer and Singer, 1998; Brooke, 1996). We did not use the full questionnaires to reduce the total number of questions, as they contained numerous items, and we wanted to avoid participants becoming fatigued and not answering the final questions attentively. The specific details of these questionnaires are detailed in Table 4, the individual questions are in Tables 5, 6, and the order of administration is illustrated in Figure 2.
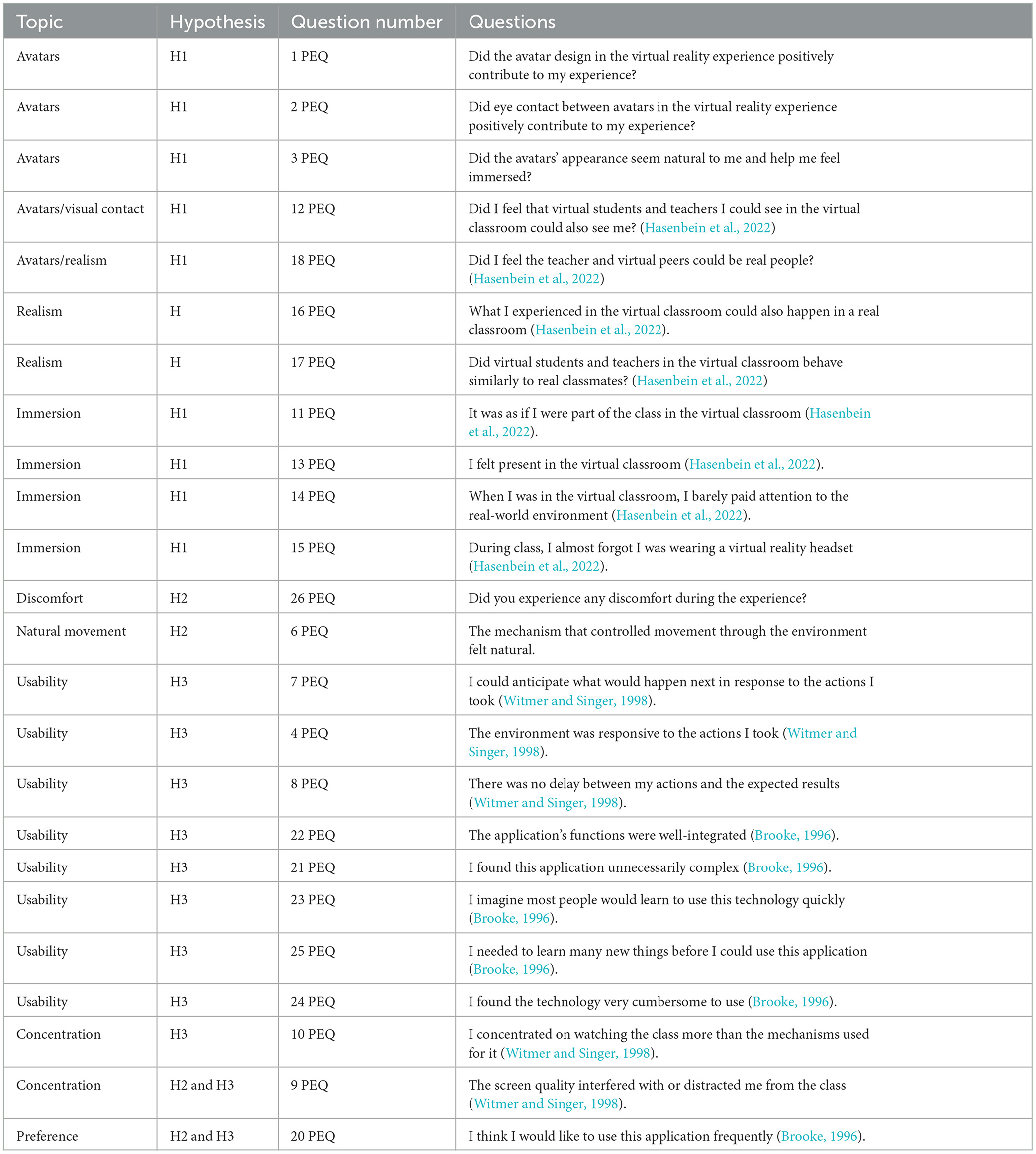
Table 5. Description of metaverse features related to hypotheses, question numbers, and questions for PEQ.
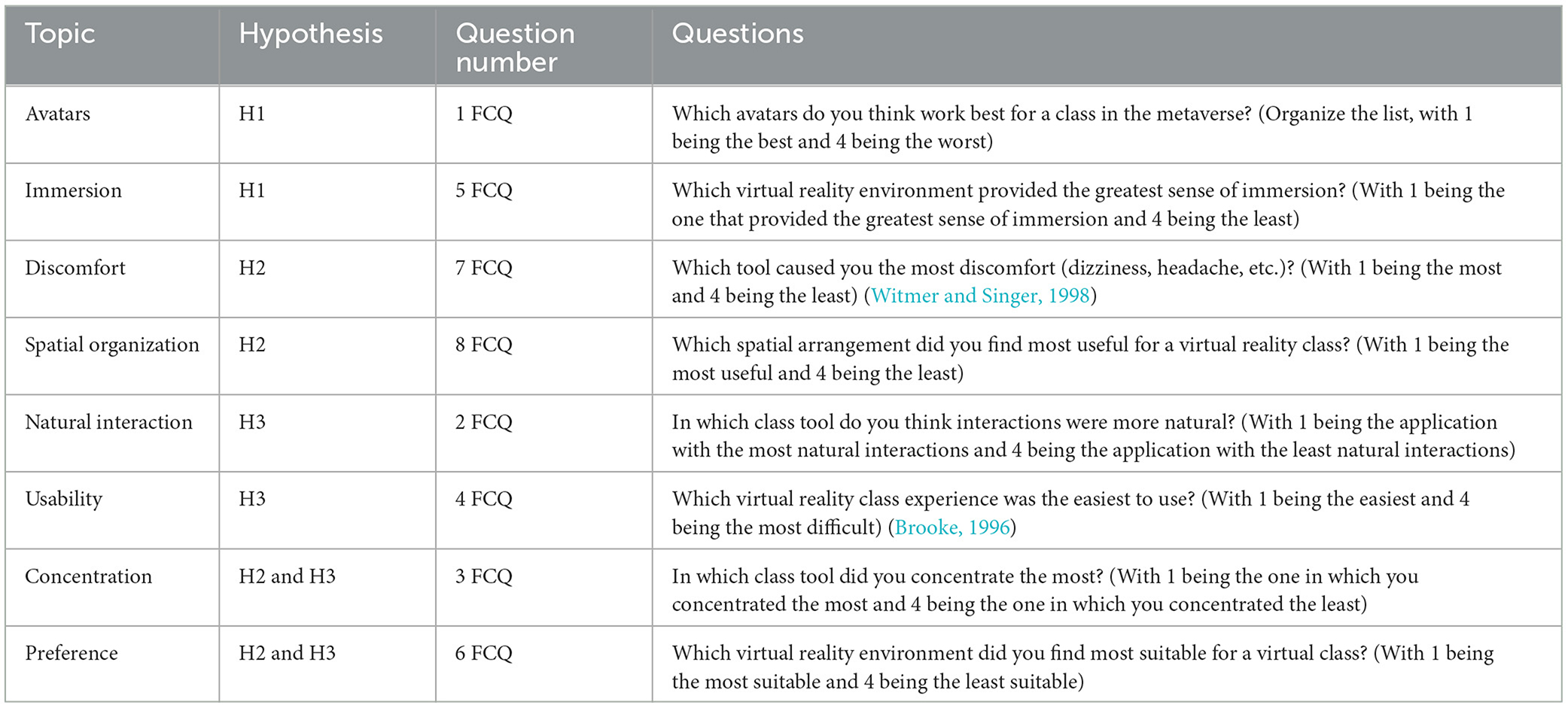
Table 6. Description of metaverse features related to hypotheses, question numbers, and questions for FCQ.
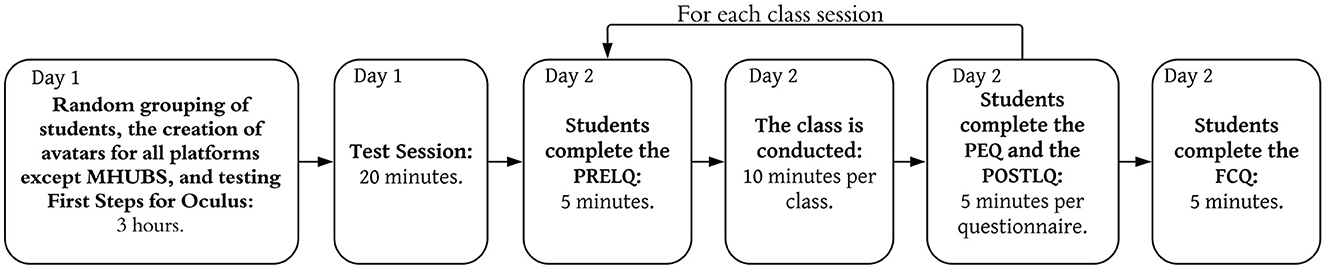
Figure 2. Flowchart of the experimental methodology. Distribution and sequence of tests, classes, and groups.
Allowing students to experience different classes in various metaverses facilitated the comparison of results obtained. Both instructors and participant groups were organized randomly. Two instructors were responsible for preparing the content and the tailored support material for each metaverse, as well as creating or adjusting the corresponding learning environments. The experiment took place over two days; the first day was a trial session, and the three remaining learning experiences were conducted on the second day over a period of two hours. Although the same class was taught in each metaverse, meaning that all four groups of students received the same class in the same metaverse, with the same instructors and identical lecture content, the topics covered in the classes for each metaverse were distinct from one another. While this approach may not be ideal for a complete comparison among metaverses and topics, it is at least a valid strategy to counterbalance potential learning effects that could occur when the same learner encounters the same topic in various learning environments while at the same time reducing the total number of sessions.
3.5 Participant characteristics
3.5.1 Participants with learner role
There were 26 participants in the learner role, including 10 undergraduate students, three graduate students, six professors, three teacher-researchers, and three individuals from other occupations. Of the group, 13% worked in the field of visual computing, while the remaining participants worked in areas related to computer science and programming. It is important to note that only 36% of the participants had tried a virtual reality headset before the XR event, and none had used virtual reality devices for more than two years. Although this audience is quite diverse, having a diverse audience gives us confidence that the factors influencing learning have been studied with a broad population. Although more studies could be conducted in the future with specific types of participants, we believe that our study demonstrates that metaverses could be helpful with a wide variety of learners.
The participants in the learner role were part of an introductory event focused on extended reality and the metaverse aimed at university students and professors. This event comprised a week of workshops, classes, and activities focused on extended reality technologies and the metaverse. During these activities, learners were introduced to virtual reality applications, games, educational and artistic applications, among others. By the time of the experiment, learners had already used these technologies for at least three hours over the preceding two days. Additionally, the day before the main sessions, students performed a setup session in each metaverse, where they created their avatars and learned the basic controls for each metaverse.
3.5.2 Participants with instructor role
Eight individuals, in addition to the students, assumed the role of instructors. Six were undergraduate students, and two were graduate students. Seven of these instructors were from the Computer Science department, and one was from the Design department. The instructors were responsible for the design and execution of the classes, including the setup of the corresponding metaverses. They received academic credit in a course on extended technologies for their contributions.
3.6 Ethical considerations
This study was approved by our university's ethics committee, ensuring that all procedures adhered to the highest ethical standards. Before taking part in the study, each participant provided written informed consent. The consent form detailed the study's procedures, including the use of head-mounted devices, and explained potential discomforts and side effects. Participants were made fully aware of their rights, including the option to withdraw from the study at any point without any consequences.
3.7 Data analysis methodology
3.7.1 Statistical tests
For the PRELQ and the POSTLQ, a statistical analysis is performed using SPSS. The Levene, Kolmogorov-Smirnov, and Shapiro-Wilk tests were conducted on the PRELQ and POSTLQ data to evaluate data normality and variance homogeneity. These test selections were based on their ability to provide dependable insights into data distribution characteristics, even in the presence of potential deviations from ideal conditions, ensuring the reliability and interpretability of our analyses. In both cases, there was insufficient evidence to assume homogeneity of variances. Additionally, the normality checks indicated that the data did not follow a normal distribution. Therefore, conducting an ANOVA directly on this data is not appropriate.
The difference between PRELQ and POSTLQ results is compared using a Friedman test. This test does not require the assumptions of homogeneity of variances or normality and is suitable for comparing medians in related groups.
Additionally, to assess the differences between the Learning Delta (LD), which is the POSTLQ result minus the PRELQ result, we conducted a repeated measures ANOVA using SPSS. To determine overall differences between the applications, we performed multivariate tests, including Pillai's Trace, Wilks' Lambda, Hotelling's Trace, and Roy's Largest Root. We also checked the assumption of sphericity and applied corrections using Greenhouse-Geisser and Huynh-Feldt to adjust the degrees of freedom if sphericity was violated. To identify specific pairwise differences between the applications. Finally, we conducted between-subjects effects tests to identify significant variations in the overall means across different subjects.
The results of the Final Comparative Questionnare (FCQ) undergo quantitative analysis through the application of an index. To calculate the FCQ index, the results of the FCQ questions are scored as follows: if the metaverse was ranked in the first place, it receives 4 points, in the second place 3 points, in the third place 2 points, and in the fourth place 1 point. These scores are then divided by the number of questions and the number of people who completed the test, and normalized with the highest possible score, which is 4.
3.7.2 Analysis per hypothesis
An analysis is conducted based on the responses gathered from the PEQ and FCQ. Responses addressing similar topics are grouped and analyzed in relation to the formulated hypotheses. This analysis is grounded in percentages and the number of participants who provided specific responses.
4 Results
4.1 Results from statistical tests
The results of the Friedman Test indicate that the asymptotic significance values for all metaverses are greater than the standard significance level (0.05). Therefore, no significant differences were found in the scores for learning before and after using WKRM. However, there was a slight (non-significant) increase in post-test scores for all metaverses, except MHUB.
4.1.1 Learning delta by metaverse
The deltas are calculated by subtracting the average value of PRELQ from POSTLQ. Normality and homogeneity of variance checks are conducted for the average deltas per metaverse. Here, we find that both homogeneity of variance and normality can be assumed.
4.1.2 Results of the learning by metaverse
Table 7 shows the descriptive statistics for the PRELQ and POSTLQ questionnaires. The general trend across all metaverses showed an improvement in average scores from before (PRELQ) to after (POSTLQ) the classes. The largest increase was observed in the SPAT metaverse, where the average score rose from 3.474 to 3.895. The smallest increase occurred in the MHUB metaverse, with the average score changing from 3.529 to 3.588.

Table 7. Descriptive statistics for PRELQ, POSTLQ and learning delta across the different metaverses.
4.1.3 Results of the learning deltas by metaverse
The repeated measures ANOVA results indicate that there are no significant differences between the metaverses based on the multivariate tests (Pillai's Trace, Wilks' Lambda, Hotelling's Trace, and Roy's Largest Root), all showing p-values >0.05. The Mauchly's Test of Sphericity was not significant (p = 0.183), suggesting that the assumption of sphericity is met. The within-subjects effects tests, including corrections for Greenhouse-Geisser and Huynh-Feldt, also show no significant differences (p > 0.05). The contrasts for linear, quadratic, and cubic trends were not significant either (p > 0.05). However, the between-subjects effects test revealed a significant difference (p = 0.038), indicating that there are significant variations in the overall means across different subjects. This suggests that individual differences between subjects may be influencing the measures, as shown in Table 8.
4.1.4 Final comparative questionnaire index
The results of the FCQ index can be seen in Table 9. It is evident that WKRM obtained the highest score, very close to the maximum value. Following WKRM is SPAT. In the last positions are ARTH and MHUB, with values very close to each other.
4.2 Results grouped by hypothesis
4.2.1 H1: Metaverses with avatars featuring more natural (or faithful) facial expressions will allow students to perceive less discomfort and more immersion
Questions 1, 2, 3, 12, and 18 of the PEQ (Figure 3) relate to avatars and eye contact. Avatars in WKRM had over 60% of participants to respond “strongly agree” in these questions. This was also found in the FCQ (Figure 4), where 96% of participants indicated that WKRM has the best avatars for a metaverse class. SPAT takes second place in questions 3, 12, and 18 of the PEQ and question 1 of the FCQ. In most cases, MHUB and ARTH rank lowest. However, ARTH's results are more evenly distributed across all response options. When analyzing the results of questions 1 in the PEQ and 1 in the FCQ, it can be seen that MHUB has the worst overall results in terms of whether avatars contribute to the classroom experience.
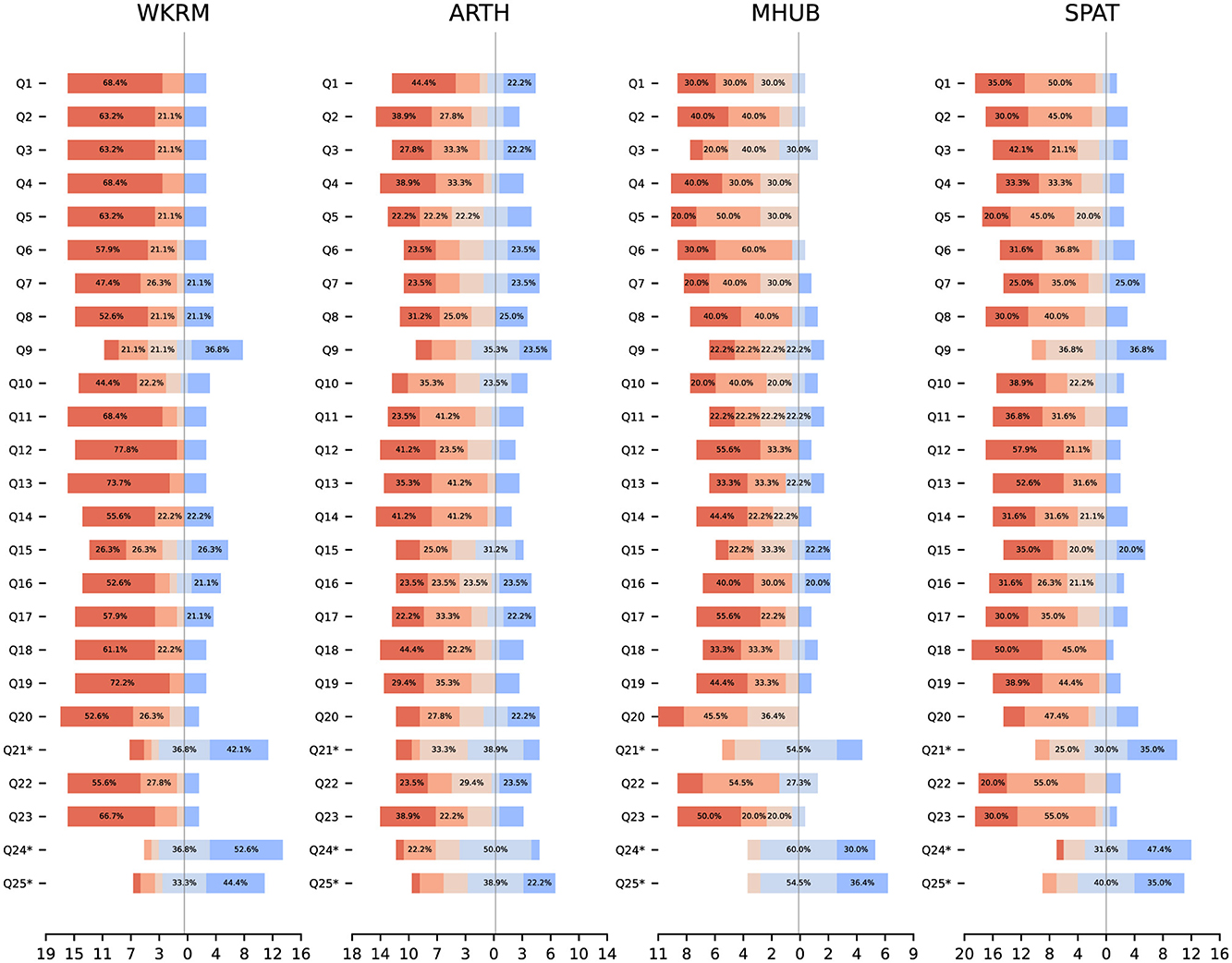
Figure 3. Post experience questionnaire (PEQ) results: these are divergent bar charts, with each chart representing the results of the 25 questions per application. The first chart is for WKRM, the second for ARTH, the third for MHUB, and the fourth for SPAT. The colors represent: “strongly agree,” “agree,” “neutral,” “disagree,” and “strongly disagree,” where “strongly agree” is red and “strongly disagree” is dark blue. On the x-axis, the count of individuals who selected a specific response option is shown. On the y-axis, the corresponding question number is displayed. Above the chart, the percentage of people who responded with each specific option is shown (only results above 20% are included for better visualization). The questions marked with an asterisk have a negative connotation.
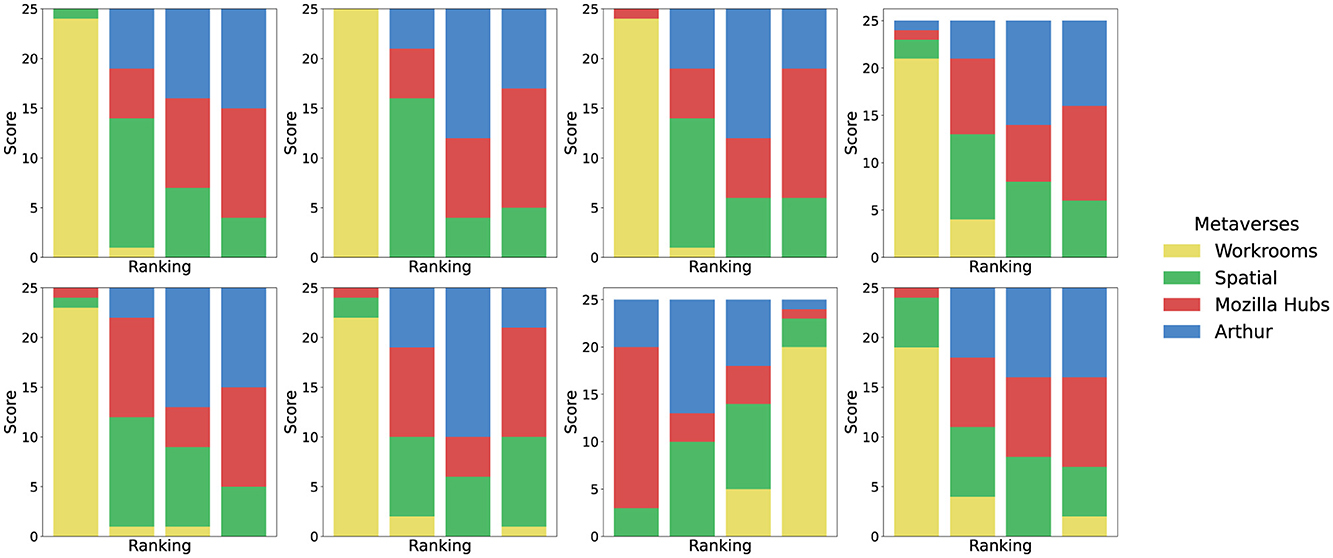
Figure 4. Divergent bar charts are used to display the results of the Final Comparative Questionnaire (FCQ). On the x-axis, the scale of application rankings is shown, and on the y-axis, the number of individuals who placed each application in each option is displayed. The legend indicates which color corresponds to each application.
The realism of the experience was measured in questions 16 and 17 of the PEQ (Figure 3). Here, it was found that the experience most closely resembling a real classroom was in WKRM, where over 50% of participants “strongly agree.” Second was MHUB, with 40 and 55.6% “strongly agree” responses regarding the overall experience and the behavior of teachers and students being possible in a real scenario. SPAT and ARTH were in third and fourth place, respectively. However, when analyzing questions 11 and 13 of the PEQ, which assessed the feeling of belonging to the class and presence, ARTH rose to third place, and MHUB dropped to fourth. Finally, questions 14 and 15 of the PEQ revealed that users disconnected from the real world to varying degrees, with more positive results for SPAT and WKRM and less for ARTH and MHUB, depending on the question.
4.2.2 H2: Applications that provide the option to sit or remain in a fixed location are less distracting and allow for greater concentration, resulting in improved learning outcomes
Question 6 of the PEQ, which assessed motion (see Table 10), revealed that the most natural mechanisms were those of WKRM, MHUB, and SPAT, with 79%, 90%, and 68.4% of users responding “Strongly agree” and “Agree,” respectively. Although WKRM was not the platform that received the most combined “strongly agree” and “agree” responses, it was the platform with the most “strongly agree” responses (57.9%). In contrast, ARTH was perceived as less natural, with only 23.5% of users answering “strongly agree” and having the highest percentage of “strongly disagree” (23.5%) answers among the four metaverses.
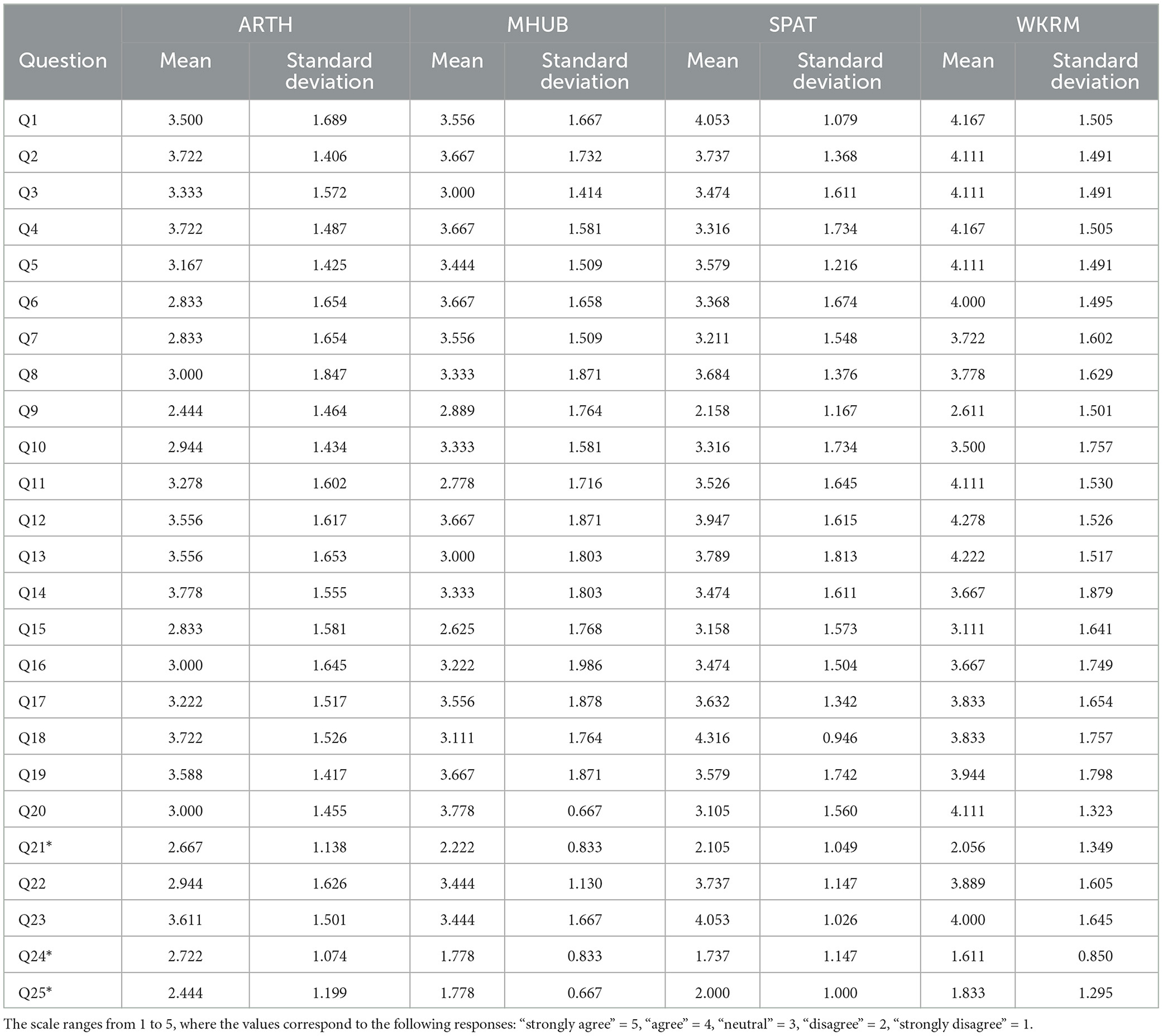
Table 10. Mean and standard deviation for each question of the PEQ in the metaverse (questions marked with * have negative connotations).
Similarly, in question 7 of the FCQ, which asked about the tool that caused the most discomfort, WKRM and SPAT had the best results (generated the least discomfort), while ARTH and MHUB had the worst (generated the most discomfort). At this point, there is a discrepancy with question 26 since when asked if any discomfort was experienced during the test, 75% of people reported at least one symptom of VR sickness in SPAT.
Regarding concentration during the experience, it was found that participants were able to concentrate much more in WKRM and SPAT and less in ARTH and MHUB (Figures 3, 4). In questions 10 PEQ and 3 FCQ, it was found that participants were most able to concentrate in WKRM, with 44.4% of participants responding “strongly agree” and 96% of participants ranking WKRM in the first place.
4.2.3 H3: Applications with more interactions (walking, dancing, sending emojis, etc.) will be more distracting and negatively impact students' attention and learning
When examining the results of “strongly agree” in questions 4, 5, 7, 8, and 22 PEQ and question 2 FCQ (Figures 3, 4), which inquire about the naturalness, intuitiveness, and integration of interactions with the environment, it can be seen that WKRM is in the first place. On the other hand, although the results for the other metaverses vary, looking at the combined percentages of “strongly agree” and “agree,” the results are generally better for SPAT, followed by MHUB, and ARTH. However, precisely according to question 7, the applications with the least intuitiveness were SPAT and ARTH, obtaining the highest percentages of “strongly disagree.”
Questions 21, 24, and 25 of the PEQ, and question 4 of the FCQ pertain to usability and difficulty of use of the metaverses. The results show that WKRM and SPAT were easier for participants, while MHUB and ARTH were more complicated, in that particular order. However, in question 23 of the PEQ, “I imagine most people would learn to use this technology quickly,” MHUB ranked second after WKRM, with 50% of people responding that they strongly agreed.
Finally, questions 6 FCQ and 20 PEQ (Figures 3, 4) ask, “Which virtual reality environment did you find most suitable for a virtual class?” and, “I think I would like to use this application frequently,” respectively. These questions show that WKRM has the best results, with 88% of people ranking it in first place in question 6 FCQ and more than 50% of “strongly agree” responses in question 20 PEQ. On the other hand, the results for the other metaverses do not seem conclusive, as the percentages and rankings are close, but in general, SPAT is in second place. In addition, although ARTH and MHUB rank lowest, the results suggest that ARTH may be more suitable for a class in the metaverse, while people would like to use MHUB more frequently.
4.3 Discussion
We begin the discussion by evaluating whether metaverses with avatars displaying more natural (or faithful) facial expressions allow students to perceive less discomfort and greater immersion, as stated in Hypothesis 1.
WKRM stood out in terms of avatar perception, especially regarding naturalness, eye contact, and immersion, as indicated by questions 1, 2, 3, 12, and 18 of the PEQ (Figure 3). This supports hypothesis 1, as WKRM features avatars with more facial expressiveness. In addition, instructors used the Meta Quest Pro, allowing students to observe the facial expressions and gaze tracking of WKRM's avatars. This factor may have played a crucial role in the greater acceptance of WKRM's avatars, possibly fostering a closer and more realistic connection. This is particularly relevant as eye contact between teachers and students is an essential form of non-verbal communication that helps teachers capture students' attention (Haataja et al., 2021).
The combination of eye and mouth movement positively influences social presence in virtual reality, as combining verbal explanations with eye movements induces a greater sense of co-presence (Kimmel et al., 2023). This could be one of the reasons why SPAT, despite having avatars with a similar appearance to WKRM, did not achieve the same results. SPAT's avatars have an anthropomorphic appearance that does not attempt to be overly realistic. Still, unlike WKRM, they have less facial expressiveness and limited eye movement, often looking straight ahead with minimal variation. Additionally, the avatars in SPAT had a predetermined walking style that did not appear natural. This type of unvaried expression and unnatural movement could have contributed to SPAT's results not being closer to WKRM. However, SPAT's second-place ranking supports hypothesis 1, as it features avatars with caricature-like appearances but not highly expressive faces.
The results for ARTH in several questions related to avatars are consistently low, which could be explained by the phenomenon known as the “uncanny valley.” This concept refers to the adverse emotional reaction people experience when encountering an avatar that closely resembles a human but exhibits subtle differences (Mori et al., 2012). ARTH stands out among the four evaluated metaverses for using users' photos as facial textures for avatars, attempting to achieve a realistic appearance, which possibly led to the perception of subtle strangeness mentioned by users. It is essential to note that these results are in line with hypothesis 1, which posits that avatars with less facial expressiveness and a non-caricature-like appearance will generate a less positive response from users.
Although non-human avatars show promise in entertainment areas in virtual reality (Krekhov et al., 2019b,a), our research suggests that they may not be equally promising for metaverse classes. This is evident with MHUB users, who, using object-based or animal-based avatars, considered them unsuitable for a metaverse class. These results indicate that although caricature-like avatars may cause less discomfort, they risk becoming distractions. Therefore, it may be time to consider an intermediate zone between realistic and caricature-like for an avatar to be most effective for metaverse classes.
The results for ARTH did not show a clear trend on the Likert scale on the PEQ, as each response option received a similar number of evaluations, especially in terms of immersion. These results may be due to issues with ARTH's avatars. During testing, discrepancies in avatars, such as representing male bodies for some females, were observed. Additionally, avatar heights seemed inconsistent, with some people having very short avatars while others had significantly taller ones. Variability in avatar form, with sudden changes in the presence or absence of legs, was also noted. These irregularities could have significantly contributed to an inconsistent immersion experience, explaining the obtained results.
We continue by evaluating whether metaverses that offer the option to sit or remain in a fixed location are less distracting and allow for greater concentration, resulting in improved learning outcomes, as proposed in hypothesis 2.
Based on observational information obtained from class recordings, we found that in applications that allowed students to move freely, they tended to explore the virtual environment more and pay less attention to the instructor and the class itself. Furthermore, the results showed that WKRM, with the most significant movement restrictions, is the platform that provides the most natural sense of movement for a classroom, which contributes to students focusing more easily on the lesson. On the other hand, metaverses like MHUB, SPAT, and ARTH, which offer more freedom of movement, were perceived as less natural and presented obstacles to concentration. These findings are consistent with hypothesis 2.
Among these three applications, MHUB is the least affected by the lack of natural movement, as its avatars lack legs. In contrast, in SPAT and ARTH, all avatars perform the same type of walking cycle because leg movement is automatically generated based on the user's head position. This type of virtual reality locomotion, with pre-recorded movements or static legs, can induce VR sickness (Lee et al., 2020). This is consistent with the results shown in the Figure 5.
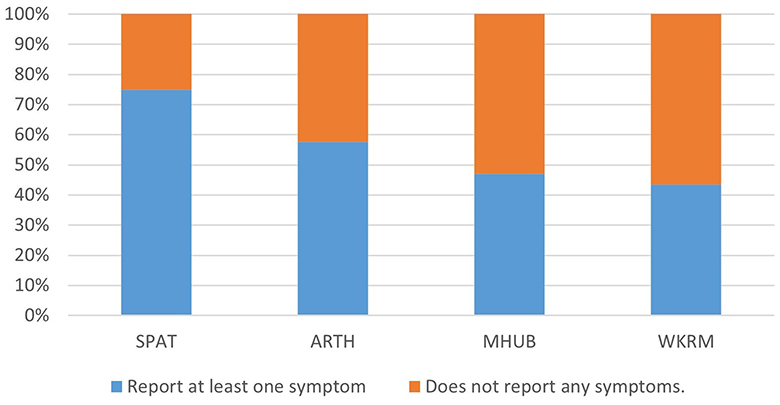
Figure 5. Percent of people who reported at least one virtual reality sickness symptom. Results of question 26 of the Post Experience Questionnaire (PEQ).
In summary, we can conclude that to promote a natural, immersive experience and facilitate concentration, it is essential to prioritize the naturalness of movement in the classroom. This naturalness can be achieved by restricting the freedom of movement and showing a more natural leg movement. If the former is not possible, it is better to opt for scenarios where avatars' legs are not visible. Therefore, although hypothesis 2 is partially fulfilled, findings suggest that ensuring the naturalness of movement is more important than limiting mobility.
We continue the analysis by evaluating whether metaverses with more interactions (walking, dancing, sending emojis, etc.) were more distracting and affected students' attention and learning, as proposed in hypothesis 3.
The classes delivered were traditional lectures, with two instructors providing information and expecting students to pay attention. In terms of program functionalities, only virtual presence in the classroom was required. With this premise, the results showed that WKRM was the tool that best suited the requirements and achieved the best interaction results. On the other hand, ARTH had interaction functionalities that needed to be considered when designing the lesson, resulting in several students becoming distracted by manipulating decorative elements (3D models) in the classroom instead of paying attention to the class. This could have been a possible cause of the negative concentration results.
Returning to hypothesis 3, contrasting WKRM and ARTH shows that if a program's functionalities align with the class's objectives, the exercise will be highly effective. However, if the class dynamics do not consider all the program's interaction possibilities, the functionalities left out of the pedagogical exercise may become a distraction factor. In other words, the hypothesis is partially correct because it is not about having many interaction functions but about using them to achieve the class's objectives.
This was true not only for ARTH but also for SPAT and MHUB, two programs that had more interaction functionalities than WKRM. In SPAT, the functionality that should have been considered was the degree of avatar movement, which resulted in students becoming distracted by exploring the space and its movement possibilities. In MHUB, the interaction functionalities among avatars through emojis were not considered, resulting in several people using emojis, which diverted students' attention.
Although not statistically significant, the learning Delta results show better results in WKRM than in the other applications. This supports the previous conclusion, as the permitted functionalities were few and more oriented toward the class dynamics. Additionally, we believe that the novelty factor may have been counterproductive for learning in this experiment.
Although metaverse technologies continuously evolve and improve, the conclusions drawn from the four metaverses studied in this research also apply to future metaverses. For instance, while MHUB was operational at the time of our experiment, it has since ceased to exist. The insights gained from WKRM, Spatial, MHUB, and ARTH provide a comprehensive understanding of the current state of the practice and remain relevant for analyzing and designing future metaverse environments.
5 Study limitations
Our within-subject design influences many variables in the study. Future work could use an experimental design based on a between-subject design, although extra considerations related to comparison between subjects, such as the number of subjects and effective ways to compare results of different subjects, will have to be taken into account. Four main limiting factors that could have influenced the results were identified while conducting the tests. The first and most significant factor was the novelty factor from participants. On the day before the tests, participants underwent a setup session for each metaverse and a trial session with one of the tools; however, these sessions did not mitigate the novelty factor, as they only tried one of the metaverses, and in the evaluated sessions, they did not experience the metaverse they had tested the day before. The novelty factor led to many cases where participants did not concentrate on the class due to the multitude of new functions they had access to. For future research, it is recommended to include more trial sessions for each metaverse to familiarize participants with the tools and reduce the impact of the learning curve.
The second factor was fatigue at the time of conducting the tests. On one hand, the time required to set up and prepare the applications varied among the metaverses, being faster in WKRM and SPAT and slower in ARTH and MHUB. Although this was not an evaluated factor, this time and ease of configuration should be considered as they can increase pre-class fatigue and affect users' attention. On the other hand, the sessions were conducted throughout a single day, so the accumulated fatigue during the day could have influenced the decrease in attention, affected the perceived quality of the platforms, and reduced overall performance in learning tests.
The third factor was the manner in which classes were conducted in different applications. For each metaverse, a class on a specific topic was held. Still, as not all topics were tested in each metaverse, it is impossible to determine whether the class's subject influenced the perception of the metaverses.
The last factor was the focus on the selected metaverses. Currently, numerous platforms allow people to meet and attend virtual classes. This study considered four specific metaverses, two of which are designed for collaboration in educational and professional aspects (WKRM and ARTH), and the other two are designed for socialization and entertainment purposes (MHUB and SPAT). Future work could consider other metaverses or newer versions with more functionality. We believe, however, that these four metaverses display a vast array of functionalities and a broad spectrum of potential within existing metaverse environments, thereby epitomizing the current state of practice.
6 Conclusions and future work
This study has conducted a comprehensive analysis of different metaverses and respective experiences for teaching in a virtual reality environment. Our main objective was to assess how these applications' avatars, organization, and functionalities influence the perception of metaverses, immersion, and concentration in a virtual reality class.
Our findings support Hypothesis 1, which posited that avatars with more natural facial expressions would generate less discomfort and greater immersion. WKRM, in particular, featuring highly expressive avatars, achieved outstanding results in terms of naturalness, eye contact, and immersion. The use of the Meta Quest Pro by instructors to track the facial expressions and gaze of avatars in WKRM likely played a crucial role in this increased acceptance.
Likewise, our research supports Hypothesis 2, which suggests that applications offering the option to sit or remain in a fixed location are less distracting and allow for greater concentration. WKRM, with movement restrictions, achieved a more natural sense of movement for classes, facilitating student concentration. On the other hand, metaverses like MHUB, SPAT, and ARTH, offering more freedom of movement, were perceived as less natural and presented obstacles to concentration. However, we also found evidence that the naturalness of movement is equally important. If this is not guaranteed, one possible option to avoid affecting the experience is to limit movement to some extent.
Hypothesis 3 proposed that applications with more interactions could be more distracting. However, our results show that the effectiveness of an application depends on how its functionalities align with the class's objectives. WKRM, which best matched the lesson requirements, achieved the best interaction results. On the other hand, ARTH, which had functionalities not considered in the class dynamics, resulted in student distraction.
This study has illuminated the importance of designing metaverse applications in educational contexts. Nevertheless, significant limitations have been identified. Participant inexperience with virtual reality technology and the absence of prior learning sessions may have influenced their concentration and perception. Additionally, key limitations include differences in class themes and potential participant fatigue due to configuration procedures and conducting sessions in a single day. Furthermore, focusing on four specific metaverses restricts the generalization of results to other virtual reality platforms.
In the future, it is suggested to include more learning sessions to familiarize participants with the technology and reduce the learning curve. Additionally, further research could address how to adapt application functionalities to avoid unnecessary distractions in educational environments, helping in the preselection of platforms for use.
Data availability statement
Publicly available datasets were analyzed in this study. This data can be found here: https://github.com/imagine-uniandes/MetaverseResearchData.
Ethics statement
The studies involving humans were approved by Comité de Ética Facultad de Ingeniería, Universidad de los Andes. The studies were conducted in accordance with the local legislation and institutional requirements. The participants provided their written informed consent to participate in this study.
Author contributions
VU: Conceptualization, Data curation, Formal analysis, Investigation, Methodology, Project administration, Software, Supervision, Validation, Visualization, Writing – original draft, Writing – review & editing. PF: Conceptualization, Formal analysis, Methodology, Project administration, Supervision, Writing – original draft, Writing – review & editing. VG: Conceptualization, Formal analysis, Methodology, Project administration, Supervision, Writing – original draft, Writing – review & editing.
Funding
The author(s) declare financial support was received for the research, authorship, and/or publication of this article. This study was conducted as part of the XR Camp 1 (CXR1) event within the Vivero Virtual project, funded by The Higher Ed XR Innovation Grant #TF2208-109389 by Unity Social Impact and Meta Immersive Learning.
Acknowledgments
We used the ChatGPT (GPT-3.5) generative AI model from OpenAI for English grammar correction and translation refinement.
Conflict of interest
The authors declare that the research was conducted in the absence of any commercial or financial relationships that could be construed as a potential conflict of interest.
Publisher's note
All claims expressed in this article are solely those of the authors and do not necessarily represent those of their affiliated organizations, or those of the publisher, the editors and the reviewers. Any product that may be evaluated in this article, or claim that may be made by its manufacturer, is not guaranteed or endorsed by the publisher.
References
Baffour, K. A., and Oyekoya, O. (2023). “Effect of look-alike avatars on students' perceptions of teaching effectiveness,” in Proceedings - 2023 IEEE Conference on Virtual Reality and 3D User Interfaces Abstracts and Workshops, VRW 2023 (Shanghai: Institute of Electrical and Electronics Engineers Inc.), 673–674.
Bailenson, J. N., Yee, N., Blascovich, J., Beall, A. C., Lundblad, N., and Jin, M. (2008). The use of immersive virtual reality in the learning sciences: digital transformations of teachers, students, and social context. J. Learn. Sci. 17, 102–141. doi: 10.1080/10508400701793141
Blume, F., Göllner, R., Moeller, K., Dresler, T., Ehlis, A.-C., and Gawrilow, C. (2019). Do students learn better when seated close to the teacher? A virtual classroom study considering individual levels of inattention and hyperactivity-impulsivity. Learn. Instruct. 61, 138–147. doi: 10.1016/j.learninstruc.2018.10.004
Bozki, E., Stark, P., Gao, H., Hasenbein, L., Hahn, J. U., Kasneci, E., et al. (2021). “Exploiting object-of-interest information to understand attention in VR classrooms,” in 2021 IEEE Virtual Reality and 3D User Interfaces (VR) (Lisboa), 597–605.
Castilla, N., Higuera-Trujillo, J. L., and Llinares, C. (2023). The effects of illuminance on students' memory. A neuroarchitecture study. Build. Environ. 228:109833. doi: 10.1016/j.buildenv.2022.109833
Childs, E., Mohammad, F., Stevens, L., Burbelo, H., Awoke, A., Rewkowski, N., et al. (2024). An overview of enhancing distance learning through emerging augmented and virtual reality technologies. IEEE Transact. Visual. Comp. Graph. 30, 4480–4496. doi: 10.1109/TVCG.2023.3264577
Conard, M. A., and Marsh, R. F. (2014). Interest level improves learning but does not moderate the effects of interruptions: an experiment using simultaneous multitasking. Learn. Ind. Differ. 30, 112–117. doi: 10.1016/j.lindif.2013.11.004
Date, T., Nagamune, T., and Sasakura, M. (2020). “Acceptability of high school class in VR and its evaluation,” in Proceedings of the International Conference on Information Visualisation, Vol. 2020 (Melbourne, VIC: Institute of Electrical and Electronics Engineers Inc.), 220–225.
Eriksson, T. (2021). “Failure and success in using Mozilla hubs for online teaching in a movie production course,” in Proceedings of 2021 7th International Conference of the Immersive Learning Research Network, iLRN 2021 (Eureka, CA: Institute of Electrical and Electronics Engineers Inc.).
Eyrolle, H., and Cellier, J.-M. (2000). The effects of interruptions in work activity: field and laboratory results. Appl. Ergon. 31, 537–543. doi: 10.1016/S0003-6870(00)00019-3
Haataja, E., Salonen, V., Laine, A., Toivanen, M., and Hannula, M. S. (2021). The relation between teacher-student eye contact and teachers' interpersonal behavior during group work: a multiple-person gaze-tracking case study in secondary mathematics education. Educ. Psychol. Rev. 33, 51–67. doi: 10.1007/s10648-020-09538-w
Hasenbein, L., Stark, P., Trautwein, U., Queiroz, A. C. M., Bailenson, J., Hahn, J. U., et al. (2022). Learning with simulated virtual classmates: Effects of social-related configurations on students' visual attention and learning experiences in an immersive virtual reality classroom. Comp. Hum. Behav. 133:107282. doi: 10.1016/j.chb.2022.107282
Hooi, K., Keong, T. T. K., and Khor, C. C. (2015). Is Gallery Walk an Effective Teaching and Learning Strategy for Biology? Singapore: Springer, 55–59.
Jang, J., Ko, Y., Shin, W. S., and Han, I. (2021). Augmented reality and virtual reality for learning: an examination using an extended technology acceptance model. IEEE Access 9, 6798–6809. doi: 10.1109/ACCESS.2020.3048708
Kimmel, S., Jung, F., Matviienko, A., Heuten, W., and Boll, S. (2023). “Let's face it: influence of facial expressions on social presence in collaborative virtual reality,” in Proceedings of the 2023 CHI Conference on Human Factors in Computing Systems (New York, NY: ACM), 1–16.
Krekhov, A., Cmentowski, S., Emmerich, K., and Krüger, J. (2019a). “Beyond human:animals as an escape from stereotype avatars in virtual reality games,” in Proceedings of the Annual Symposium on Computer-Human Interaction in Play (New York, NY: ACM), 439–451.
Krekhov, A., Cmentowski, S., and Krüger, J. (2019b). “The illusion of animal body ownership and its potential for virtual reality games,” in 2019 IEEE Conference on Games (CoG) (London), 1–8.
LaCroix, K., and LaCroix, S. (2017). Does seat location matter? A review of the proximity effect in large and small classrooms. Commun. Coll. Enter. 23, 58–69.
Land, S. F. V. D., Schouten, A. P., Feldberg, F., Huysman, M., and van den Hooff, B. (2015). Does avatar appearance matter? How team visual similarity and member-avatar similarity influence virtual team performance. Hum. Commun. Res. 41, 128–153. doi: 10.1111/hcre.12044
Lee, J., Lee, M., Kim, G. J., and Hwang, J.-I. (2020). “Effects of virtual gait visualization in walk-in-place on body ownership and presence,” in Extended Abstracts of the 2020 CHI Conference on Human Factors in Computing Systems (New York, NY: ACM), 1–7.
Maruko, K., Mouri, K., Okamoto, M., and Matsubara, Y. (2023). “Design of VR classroom toward Kansei engineering analysis,” in Proceedings - 2023 14th IIAI International Congress on Advanced Applied Informatics, IIAI-AAI 2023 (Koriyama: Institute of Electrical and Electronics Engineers Inc.), 740–741.
Meta (2023). Horizon Workrooms virTual Office & Meetings. Available at: https://forwork.meta.com/horizon-workrooms/ (accessed October 20, 2024).
Mori, M., MacDorman, K. F., and Kageki, N. (2012). The uncanny valley. IEEE Robot. Automat. Mag. 19, 98–100. doi: 10.1109/MRA.2012.2192811
Mozilla (2023). FAQ for Mozilla Hubs. Available at: https://hubs.mozilla.com/docs/hubs-faq.html#what-is-the-capacity-of-a-hubs-room (accessed October 20, 2024).
Mystakidis, S., Berki, E., and Valtanen, J.-P. (2021). Deep and meaningful e-learning with social virtual reality environments in higher education: a systematic literature review. Appl. Sci. 11:2412. doi: 10.3390/app11052412
Netland, T., and Hines, P. (2021). “Teaching in virtual reality: experiences from a lean masterclass,” in Learning in the Digital Era, eds. D. J. Powell, E. Alfnes, M. D. Q. Holmemo, and E. Reke (Cham. Springer International Publishing), 155–162.
Schier, F., Chandran, K., and McGinity, M. (2022). “Teachinvr: a virtual reality classroom for remote education,” in 2022 IEEE Conference on Virtual Reality and 3D User Interfaces Abstracts and Workshops (VRW) (Christchurch), 283–286.
Shengkai, L., Mouri, K., Okamoto, M., and Matsubara, Y. (2023). “Learning analytics in VR: what if we can collect learning logs in VR classroom,” in Proceedings - 2023 International Conference on Artificial Intelligence and Education, ICAIE 2023 (Kobe: Institute of Electrical and Electronics Engineers Inc.), 52–56.
Smith, C. (2017). The influence of hierarchy and layout geometry in the design of learning spaces. J. Learn. Spaces 6, 59–67.
Spatial (2023). How Many People Can You Have in One Space - Overflow Groups/Instancing. Available at: https://support.spatial.io/hc/en-us/articles/12818484755092-How-Many-People-Can-You-Have-in-One-Space-Overflow-Groups-Instancing#:~:text=Spatial%20supports%2050%20users%20per%20space (accessed October 20, 2024).
Suk, H., and Laine, T. H. (2023). Influence of avatar facial appearance on users' perceived embodiment and presence in immersive virtual reality. Electronics 12:583. doi: 10.3390/electronics12030583
Urban, A. D., Varela, P., Pingel, J., Liu, Y., Wandres, L., and Duvoisin, M. (2023). “How virtual reality (VR) impacts learner engagement in online courses: initial findings,” in 2023 IEEE Learning with MOOCS, LWMOOCS 2023 - Conference Proceedings (Cambridge, MA: Institute of Electrical Electronics Engineers Inc.).
Wardani, R., Jati, H., Nurkhamid Indrihapsari, Y., Setialana, P., Budiyanto, A. M., and Ardiansyah, M. N. (2021). Improving online learning interactivity with 3d virtual classroom models. J. Phys. Conf. Ser. 2111:012028. doi: 10.1088/1742-6596/2111/1/012028
White, M., Kupin, A., Inzerillo, S., Banerjee, N. K., and Banerjee, S. (2024). “Evaluating the effectiveness of VR classrooms as a replacement for traditional asynchronous video-based learning environments,” in Proceedings - 2024 IEEE International Conference on Artificial Intelligence and eXtended and Virtual Reality, AIxVR 2024 (Los Angeles, CA: Institute of Electrical and Electronics Engineers Inc.), 147–156.
Witmer, B. G., and Singer, M. J. (1998). Measuring presence in virtual environments: a presence questionnaire. Presence Teleoperat. Virt. Environ. 7, 225–240. doi: 10.1162/105474698565686
Won, M., Ungu, D. A. K., Matovu, H., Treagust, D. F., Tsai, C.-C., Park, J., et al. (2023). Diverse approaches to learning with immersive virtual reality identified from a systematic review. Comp. Educ. 195:104701. doi: 10.1016/j.compedu.2022.104701
Woodworth, J. W., Lipari, N. G., and Borst, C. W. (2019). “Evaluating teacher avatar appearances in educational VR,” in 2019 IEEE Conference on Virtual Reality and 3D User Interfaces (VR) (Osaka), 1235–1236.
Yee, N., and Bailenson, J. (2007). The proteus effect: the effect of transformed self-representation on behavior. Hum. Commun. Res. 33, 271–290. doi: 10.1111/j.1468-2958.2007.00299.x
Keywords: applied computing, education, E-learning, human-centered computing, human-computer interaction (HCI), interaction paradigms, virtual reality, metaverse
Citation: Uribe V, Figueroa P and Gomez V (2024) The influence of metaverse environment design on the quality of experience in virtual reality classes: a comparative study. Front. Educ. 9:1451859. doi: 10.3389/feduc.2024.1451859
Received: 19 June 2024; Accepted: 17 September 2024;
Published: 16 December 2024.
Edited by:
Yu-Chun Kuo, Rowan University, United StatesReviewed by:
Jorge C. S. Cardoso, University of Coimbra, PortugalMichael Vallance, Future University Hakodate, Japan
Copyright © 2024 Uribe, Figueroa and Gomez. This is an open-access article distributed under the terms of the Creative Commons Attribution License (CC BY). The use, distribution or reproduction in other forums is permitted, provided the original author(s) and the copyright owner(s) are credited and that the original publication in this journal is cited, in accordance with accepted academic practice. No use, distribution or reproduction is permitted which does not comply with these terms.
*Correspondence: Pablo Figueroa, cGZpZ3Vlcm9AdW5pYW5kZXMuZWR1LmNv