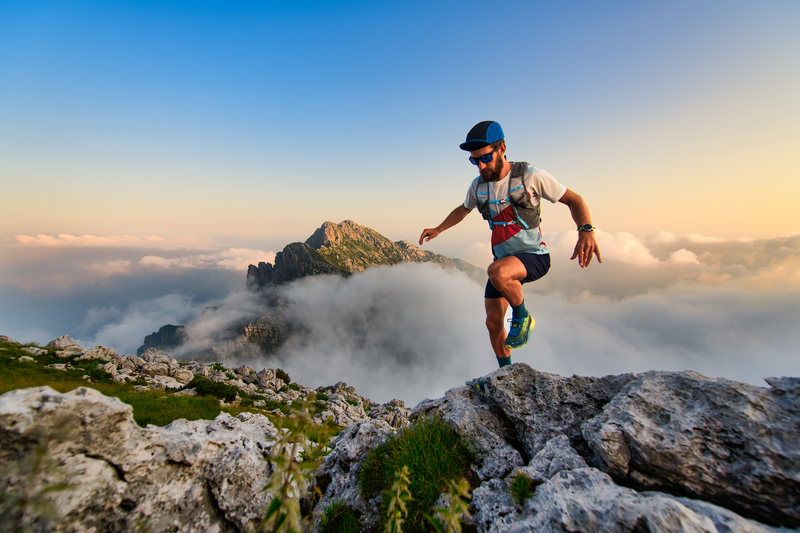
95% of researchers rate our articles as excellent or good
Learn more about the work of our research integrity team to safeguard the quality of each article we publish.
Find out more
OPINION article
Front. Educ. , 13 November 2024
Sec. Digital Learning Innovations
Volume 9 - 2024 | https://doi.org/10.3389/feduc.2024.1445654
In the modern educational landscape, the pace of technological change has ushered in a transformation where artificial intelligence (AI) is integrated into learning and development in nearly all areas. A lot of that transformation has been through the rise of micro-credentialing, whereby the need for flexible, skill-specific education becomes very close to the needs of the changing global workforce (Kiiskilä et al., 2022). Often referred to as mini-qualifications for demonstrating a certain level of competency in a certain area, micro-credentials are increasingly being recognized for the potential role they play in filling in the gaps left by the traditional configurations of education (Keoy et al., 2024). They provide a channel through which the learner is able to both obtain and demonstrate the skill in a way that is both effective and relevant to their career. McGreal and Olcott (2022) critically examine the role of micro-credentials in higher education, highlighting their potential as strategic tools for institutional adaptation while cautioning that they are not a universal solution to institutional challenges. They emphasize the importance of a strategic assessment by university leaders to determine whether micro-credentials align with their institutional goals and resources, rather than automatically adopting them as a revenue-generating initiative (McGreal and Olcott, 2022).
Artificial Intelligence (AI) refers to the simulation of human intelligence in machines programmed to think and learn like humans. About micro-credentialing, AI covers most technologies ranging from machine learning to natural language processing and down to expert systems. Figure 1 visually summarizes the relationship between AI technologies—Neural Networks, Natural Language Processing, and Expert Systems—and their specific applications in micro-credentialing. Recent advances in AI are largely driven by neural networks, particularly deep learning models. These networks, inspired by the brain's architecture, excel at pattern recognition and prediction from large datasets. In micro-credentialing, neural networks enhance personalized learning by analyzing extensive learner data, enabling more effective educational technologies. Machine learning algorithms use overflowing data sets to identify patterns in data and infer incomplete information about learners to propose a personalized learning pathway. NLP helps develop intelligent tutoring systems so the students themselves can turn in for the facility to obtain real-time feedback and facilitate interactive learning experiences. Expert systems, mimicking human expert decisions, help devise curricula and assessments and make them adaptive by a learner's needs. For example, IBM uses AI to develop tailored reskilling programs, and platforms like Coursera use AI to suggest courses to learners based on their learner profiles. Of course, all of these AI technologies will help enhance micro-credentialing by increasing the personalization, efficiency, and responsiveness of education to learners' and industry demands. Artificial Intelligence in micro-credentialing will prove to be a game-changer, adding a crucial aspect to any learning experience: personalization (McGreal, 2024). Artificial Intelligence technologies analyze extensive datasets of behavior, preferences, and performance of the learners to develop an educational trajectory that is not only in sync with the learning style of the individual but is also adaptive to the changing requirements of the industry (Blaj-Ward et al., 2023). Personalization like this, scaling learner engagement and success through AI, will make learning a dynamic and frictionless process that will be responsive to the demands of the learner and the larger economy (McGreal, 2024).
In recent years, several studies have explored the transformative potential of AI in education, particularly in micro-credentialing. Pirkkalainen et al. describe the key features of higher education micro-credential platforms, pointing out ethical implications regarding data privacy and algorithmic bias. This points to the fact that ethical concerns have to be dealt with carefully to ensure a responsible AI implementation within educational institutions (Pirkkalainen et al., 2023). Ahmat et al. (2021) review the challenges and opportunities for higher education to move toward micro-credential certification in a manner curator-oriented, focusing on how such innovations could resolve skill gaps and increase workforce readiness. Their work highlighted economic benefits and has the potential of micro-credentials in providing better employability (Ahmat et al., 2021). Orman et al. (2023) intend to scrutinize the role of AI in democratizing education and mitigating the digital divide. It requires specific efforts to enhance infrastructure and literacy in the digital aspect of underserved communities. It highlights the possible contributions AI can make to bridge educational gaps (Orman et al., 2023). Tian et al. (2022) discuss the integration of AI into blockchain technology to increase credibility and enhance micro-credential security. Their study outlines how such integration in technology would lead to heightened security and trustworthiness of educational credentials (Tian et al., 2022). Brown et al. give an overview of the complete global landscape of micro-credentialing in their paper, reviewing how micro-credentials fit within the interrelationship of lifelong learning and employability. Their work presents an opportunity for micro-credentials to become a more established component of education in the 21st century (Brown et al., 2021). Zawacki-Richter et al. (2019) conducted a systematic review of AI applications in higher education settings that define the most relevant key areas: profiling, assessment, adaptive systems, and intelligent tutoring systems. The study underlines the challenges and opportunities that AI brings to educational contexts (Zawacki-Richter et al., 2019). Rajabalee (2023) proposes a systematic analysis of the literature about implementing micro-credentials within academic contexts and underlines their potential: namely, becoming recognized credits that would be transferable in formal higher education.
In light of the above, and although the application of AI in micro-credentialing seems promising, this raises some pertinent questions and issues, such as data privacy, access to equity, and long-run effects on employment. This paper interacts with the AI revolution in micro-credentialing to posit that, though not void of challenges, it is an imperative and, indeed, developmental phase education needs to go through and is likely to birth overwhelmingly positive benefits for learners worldwide if thoughtfully managed.to birth overwhelmingly positive benefits for learners worldwide if thoughtfully managed.
This use of artificial intelligence in the integration of micro-credentialing systems heralds a new era of personalized education: a transformation in how we learn and how we are assessed in skills and knowledge. This will bring the strength of AI to be harnessed and tapped into personalized learning in response to individual learners' needs, optimizing their educational paths and probably accelerating their career advancement.
The benefits of AI in micro-credentialing lie at the foundation. Primary among them is the fact that AI allows for dynamic learning environments that evolve in real time with a learner's progress, preferences, and performance. Such learning analytics will allow AI to understand how learners interact with material, tune task difficulty, or suggest more resources to better assist learners with the struggles they face. This kind of customization was never before possible within traditional educational settings, in which one-size-fits-all approaches too frequently predominate.
Most importantly, AI will be used for the identification of the gaps in the skills pool and the trends in industry which are emerging, enabling the programs to be updated regularly and issue micro-credentials that are most relevant to the market need today. This will keep learners competitive in the workforce and address skill shortages that have left industries in a tough spot, aligning education more with economic requirements. For example, job listings, industry releases, professional development trends, and the like can all go into an algorithm that would then present to or automatically enroll learners into micro-courses that enhance their employability. More generally, automation of the processes of assessment and feedback through AI will reduce the administrative overhead and will enable scalable solutions in credentialing. Automated grading systems and AI-enhanced evaluations will provide instantaneous, detailed feedback to learners—a capability missing from today's skills-based educational tools, primarily due to the resource-intense nature of providing human-generated feedback on many assignments.
However, AI in micro-credentialing is not without its challenges. It must address issues regarding data privacy, algorithmic bias, and the digital divide if it is going to live up to its potential in this space. Nevertheless, with the benefits of increased access, more personalized learning, and closer alignment to labor market demand, AI-driven micro-credentialing is bound to be a transforming force in the education system—an improvement in the way qualifications are to be achieved and recognized in the digital age.
Most of the criticism in this regard focuses on data privacy and the risk for increased digital divide in AI for education. The proponents of skepticism argue that AI and digital platforms might skew reliance toward the better-off in terms of the access of technology, leaving the low-income population behind. Moreover, because data collection is pervasive, huge privacy concerns are attached to AI applications. However, strong data protection regulations such as those contained in the EU GDPR, which offers solid privacy protections, might mitigate these concerns. Moreover, with enhanced efforts to expand digital infrastructure and literacy into low-income and rural areas, the disparities of AI-enhanced learning will be low, hence making the distribution of educational benefits more equal. On the one hand, the integration of AI into micro-credentialing has various advantages for education; on the other, it has created a whole set of concerns and opposition that has to be taken into account to make sure that the innovations go in the best interests of all the stakeholders. Chief among such concerns are problems related to data privacy, the digital divide, and potential biases in the AI algorithms.
Data privacy seems to be one of the major concerns, as AI needs access to huge volumes of personal information to be able to tailor the learning experience effectively. As others argue, data collection and analysis might lead to possible privacy breaches in case it is not handled properly. The good thing is that this kind of situation necessitates the existence of good data protection measures. This would mean that there are strict policies in handling data and security protocols that ensure the information regarding the learners is protected. Further, clearly stating how the data would be used and getting consent from the users can help reduce issues of privacy and ensure trust in AI systems.
Another critical issue is that AI-driven micro-credentialing will exclude some people who are maybe not in a position to fully exploit the programs on account of lack of access to the technological infrastructure for the same. People of low socioeconomic status or in remote locations may not have the technological infrastructure that would enable them to take full advantage of those programs. There is a need for targeted initiatives in this regard, as this will increase both digital literacy and access to technology. Partnerships among governments, educational institutions, and the private sector will ensure the deployment of affordable technology and internet access, thus guaranteeing that AI's benefits are more evenly distributed among the people.
Algorithmic bias is one of the main issues that need to be addressed. AI is as neutral as the data it learns from; hence, bad data will generate bad decisions and therefore perpetuate discrimination. This serves to underscore the need to make investments in AI systems developed using diverse and inclusive data sets, and continuously monitor and adapt the systems to steer clear of practices considered unfair. Furthermore, the embedding of regulatory frameworks in the process—to be fair in its algorithms—can even be more protective against bias in ensuring that AI-driven micro-credentialing becomes an enabler for educational equity. All these issues, addressed proactively, will pave the way for the full realization of AI potential for micro-credentialing, enabling very personalized, efficient, and fair educational opportunities fit for the modern workforce.
A wealth of literature and practical applications testify to the practical effect and potential of AI in micro-credentialing. For instance, according to one research by MIT scholars, AI-based individualized learning systems can reduce the time taken to achieve fluency in a subject by up to 50%. Table 1 shows a comparison of AI-driven micro-credentialing platforms, highlighting the AI technologies used, the level of personalization features, their relevance to industry needs, and the success rates achieved. AI-driven micro-credentialing programs are now being rolled out across corporations like IBM, which was able to reskill its workforce in essential areas like cybersecurity. This has not only brought about improved performance for these job variants but also saw employee satisfaction and retention rates rising equally as well (Alam, 2022; Robert et al., 2024). According to the studies, not only has there been improved performance for these job variants, but employee satisfaction and retention rates have also risen equally well. Besides, Coursera's AI-powered learning path personalization enables higher course completion rates and superior learner outcomes. For instance, the University of Queensland leveraged AI to analyze data on student performance and enable personalized interventions that drastically reduced dropout rates (Crawford et al., 2024). This collection of case studies illustrates very clearly the opportunities brought about by AI in increasing learning efficiency, improving educational outcomes, and streamlining education trajectories to meet labor-market needs better. Using concrete examples of successful implementations allows us to shift this appreciation toward revealing AI's potential as applied to micro-credentialing.
The use of AI in education has to be balanced in a manner such that it becomes an instrument of empowerment but not exclusion. Policies and practices have to be aimed at putting AI tools within the reach of all demographic and socioeconomic groups without exception so as not to worsen already existing inequalities. Those must include, but will not be limited to, the deployment of necessary hardware and software, as well as training and support to educators and learners. Only such inclusive strategies can make full use of the potential of AI to democratize education so that advanced, personalized learning opportunities are within everyone's reach, regardless of background or resources.
Identifying and mitigating this research gap is necessary to maximize the benefits that will accrue to the educational frameworks. In fact, it can even be called for longitudinal research into these dynamics, pinpointing the necessity of assessing the long-term implications that micro-credentials have on the equity in career growth, income increments, and job security. Finally, equity and access around these programs warrant further research. With AI-driven micro-credentialing, it needs to be understood how people from underrepresented groups are affected, which includes minorities, those in rural areas, and those with low economic statuses. This calls for understanding the possible barriers such groups may face and aiming to reduce them in fostering inclusive educational opportunities.
Further research also needs to be carried out on the equity and potential biases of AI algorithms used within micro-credentialing to ensure that the recommendations and assessments are bias-free. In addition, AI-enhanced learning outcomes need to be compared to their traditional counterparts to establish the contexts in which micro-credentials offer unique advantages or otherwise fall short. There is also a need to investigate the psychological impact of the personalized AI learning systems in terms of motivation and anxiety in order to ensure that such innovations promote learner wellbeing effectively. Other areas of critical research will include acceptance of micro-credentials in different sectors, development of appropriate regulatory and ethical frameworks, economic analysis of implementation costs and benefits of introducing AI, changing roles for educators, and technical challenges of integrating AI within existing infrastructure. Filling these gaps would significantly help in enhancing understanding and applications of AI in education toward accelerating the power of micro-credentials to revolutionize learning and career development in a holistic manner.
Challenges of data privacy, equity, and algorithmic bias associated with AI-driven micro-credentialing demand a multi-dimensional approach. Robust encryption techniques, transparency about disposing of data, and explicit consent of the user at each point are some measures to allay concerns over data privacy. Following the principles of privacy by design, it is incumbent upon educational institutions and platforms to rigorously work along the lines of regulations like GDPR. Particularly in this sphere, such equity issues relate to the digital divide and raise focused initiatives for increasing access to technology and digital literacy within acting communities. The collaboration of the government, educational institutions, and tech companies can facilitate the spreading of more affordable devices and reliable internet access. To address algorithm bias, diversified representative datasets have to be developed for AI systems' training. Continuous monitoring and auditing of AI algorithms would help detect and rectify biases, thereby arriving at impartial judgments. Moreover, such policy regulatory frameworks for this field and ethical guidelines will also enhance transparency and accountability for AI uses. If all these aspects are kept in mind with appropriate care, the use of AI-based micro-credentialing can be broadly inclusive, fair, privacy-preserving, and hence more effective and trustworthy.
The integration of AI into micro-credentialing will be one of the breakthrough elements in the push toward more personalized, efficient, and inclusive education systems. It provides a more dynamic and responsive learning environment through the closer alignment of educational pathways with the needs and realities of the individual within the market. The bottom line: Issues of privacy, equity, and access need to be part of the new paradigm but are surmountable with well-planned policy and careful, ethical technology use. All these players—policy makers, educators, technologists, and learners themselves—need to converge their efforts in order to realize the promise of AI in making educational experiences increasingly personal, accessible, and effective for all. What the AI revolution in micro-credentialing portends, therefore, is not just the business of new technologies but a full reinvention of how education can be the bridge to opportunity and success in a world of increasing complexity.
PS: Conceptualization, Writing – original draft, Writing – review & editing. JJosep: Conceptualization, Writing – original draft, Writing – review & editing. BS: Conceptualization, Writing – original draft. AS: Conceptualization, Writing – original draft. AC: Conceptualization, Writing – original draft. JJose: Conceptualization, Writing – original draft.
The author(s) declare that no financial support was received for the research, authorship, and/or publication of this article.
The authors declare that the research was conducted in the absence of any commercial or financial relationships that could be construed as a potential conflict of interest.
All claims expressed in this article are solely those of the authors and do not necessarily represent those of their affiliated organizations, or those of the publisher, the editors and the reviewers. Any product that may be evaluated in this article, or claim that may be made by its manufacturer, is not guaranteed or endorsed by the publisher.
Ahmat, N. H. C., Bashir, M., Razali, A. R., and Kasolang, S. (2021). Micro-credentials in higher education institutions: challenges and opportunities. Asian J. Univ. Educ. 17, 281–290. doi: 10.24191/ajue.v17i3.14505
Alam, A. (2022). “Employing adaptive learning and intelligent tutoring robots for virtual classrooms and smart campuses: reforming education in the age of artificial intelligence,” in Advanced Computing and Intelligent Technologies: Proceedings of ICACIT 2022 (Springer), 395–406. doi: 10.1007/978-981-19-2980-9_32
Blaj-Ward, L., Narang, A., and Garrett, J. (2023). “Cultivating sustainable mentoring relationships: Micro-credentials and the SDGs,” in Mentoring Within and Beyond Academia: Achieving the SDGs, 39–56. Available at: https://www.scopus.com/inward/record.uri?eid=2-s2.0-85181264171&partnerID=40&md5=8dbddb36c8255f75519f51a4f2e07930 (accessed June 4, 2024).
Brown, M., Mhichíl, M. N. G., Beirne, E., and Lochlainn, C. M. (2021). The global micro-credential landscape: charting a new credential ecology for lifelong learning. J. Learn. Dev. 8:525. doi: 10.56059/jl4d.v8i2.525
Crawford, J., Allen, K.-A., Pani, B., and Cowling, M. (2024). When artificial intelligence substitutes humans in higher education: the cost of loneliness, student success, and retention. Stud. High. Educ. 49, 883–897. doi: 10.1080/03075079.2024.2326956
Keoy, K. H., Koh, Y. J., Iqbal, J., Anjum, S. S., Yeo, S. F., Cherukuri, A. K., et al. (2024). Streamlining micro-credentials implementation in higher education institutions: considerations for effective implementation and policy development. J. Inf. Knowl. Manag. 23:2350069. doi: 10.1142/S0219649223500697
Kiiskilä, P., Hanafy, A., and Pirkkalainen, H. (2022). “Features of micro-credential platforms in higher education,” in Proceedings of the 14th International Conference on Computer Supported Education (CSEDU 2022), 81–91. doi: 10.5220/0011030600003182
McGreal, R. (2024). “Empowering micro-credentials using blockchain and artificial intelligence,” in Global Perspectives on Micro-Learning and Micro-Credentials in Higher Education, 75–90. doi: 10.4018/979-8-3693-0343-6.ch006
McGreal, R., and Olcott, D. (2022). A strategic reset: micro-credentials for higher education leaders. Smart Learn. Environ. 9:9. doi: 10.1186/s40561-022-00190-1
Orman, R., Simsek, E., and Çakir, M. A. K. (2023). Micro-credentials and reflections on higher education. High. Educ. Eval. Dev. 17, 96–112. doi: 10.1108/HEED-08-2022-0028
Pirkkalainen, H., Sood, I., Padron Napoles, C., Kukkonen, A., and Camilleri, A. (2023). How might micro-credentials influence institutions and empower learners in higher education? Educ. Res. 65, 40–63. doi: 10.1080/00131881.2022.2157302
Rajabalee, Y. (2023). The implementation of micro-credentials in formal and informal learning: a systematic literature review. Int. J. Learn. Teach. 9:328. doi: 10.18178/ijlt.9.4.328-336
Robert, A., Potter, K., and Frank, L. (2024). “The impact of artificial intelligence on students' learning experience,” in Wiley Interdisciplinary Review Computer Statistic.
Tian, R., Kong, L., Min, X., and Qu, Y. (2022). “Blockchain for AI: a disruptive integration,” in 2022 IEEE 25th International Conference on Computer Supported Cooperative Work in Design (CSCWD), 938–943. doi: 10.1109/CSCWD54268.2022.9776023
Keywords: artificial intelligence (AI), micro-credentialing, personalized learning, data privacy, learning experience
Citation: Shanmughan P, Joseph J, S BN, M AS, S AC and Jose J (2024) The AI revolution in micro-credentialing: personalized learning paths. Front. Educ. 9:1445654. doi: 10.3389/feduc.2024.1445654
Received: 07 June 2024; Accepted: 12 September 2024;
Published: 13 November 2024.
Edited by:
Alba García Barrera, Universidad a Distancia de Madrid, SpainReviewed by:
Thomas F. Heston, University of Washington, United StatesCopyright © 2024 Shanmughan, Joseph, S, M, S and Jose. This is an open-access article distributed under the terms of the Creative Commons Attribution License (CC BY). The use, distribution or reproduction in other forums is permitted, provided the original author(s) and the copyright owner(s) are credited and that the original publication in this journal is cited, in accordance with accepted academic practice. No use, distribution or reproduction is permitted which does not comply with these terms.
*Correspondence: Jobin Jose, am9iaW4uam9zZUBtYXJpYW5jb2xsZWdlLm9yZw==
Disclaimer: All claims expressed in this article are solely those of the authors and do not necessarily represent those of their affiliated organizations, or those of the publisher, the editors and the reviewers. Any product that may be evaluated in this article or claim that may be made by its manufacturer is not guaranteed or endorsed by the publisher.
Research integrity at Frontiers
Learn more about the work of our research integrity team to safeguard the quality of each article we publish.