- 1Centro de Investigación en Ciencias Humanas y de la Educación - CICHE, Facultad de Ciencias de la Educación, Carrera de Educación Básica, Universidad Tecnológica Indoamérica, Ambato, Ecuador
- 2Carrera de Educación Básica, Universidad Tecnológica Indoamérica, Ambato, Ecuador
University dropout is a social issue that directly impacts both families and the state, characterized by disparities in enrollment and graduation based on various factors. The primary objective of this study was to analyze the determinants of university dropout supported by multifactorial analysis at a private university in Ecuador. This research was conducted within a post-positivist paradigm with a quantitative approach, utilizing digitized statistical records of students and results from a personalized survey sent to dropouts via email. Out of a total of 1,078 students admitted and/or enrolled over 5 years, a sample was analyzed from the 484 who dropped out. Student dropout is associated with personal, familial, economic, academic, and institutional factors, contributing to school abandonment with an estimated probability of 44.90%. In conclusion, a higher dropout rate was observed among male students from public institutions who did not complete the first cycle, despite having a higher number of enrollments at the university. Finally, the Multilevel Logistic Regression model can predict with 76.44% accuracy the behavior of the independent variables affecting school dropout.
1 Introduction
University dropout represents a global challenge with significant social, economic, and political repercussions, emphasizing educational inequality that restricts access and graduation for a limited number of students (Enguídanos et al., 2023; Lorenzo-Quiles et al., 2023; Mascia et al., 2023). Despite efforts made to reduce this issue from the 1970s to the present, the dropout rate has shown alarming persistence, especially in developing countries. Globally, the European Union, for instance, worked in 2020 on plans to increase the graduation rate of the population aged 30 to 34, reducing the percentage of people without tertiary education by 40% (De La Cruz-Campos et al., 2023). In Spain, 20% of the population that begins their studies drops out at the initial levels, decreasing as they advance in their careers. Italy has high university dropout rates (Álvarez Ferrandiz, 2021; Delogu et al., 2024).
In Latin America, university dropout rates have been notably high, reaching 47% in 2007 and 50% in 2012, with the Dominican Republic at 76%, Bolivia at 73.3%, and Uruguay at 72% (Al-hawamdeh and Alam, 2022; Martelo et al., 2017). Investment in higher education, both in scientific and technological aspects, plays a crucial role in this problematic situation. Policies in Colombia and Chile have contributed to a 50% increase in dropout rates due to political conflicts and social movements that affect academic stability, while in the Dominican Republic and Bolivia, the rate exceeds 70%. In contrast, Cuba has a dropout rate of 25% (Mundial, 2017). The lack of equity in higher education is reflected in economic inequality, as students from lower-income quintiles are less likely to access higher education (Alvarado-Uribe et al., 2022; Vadivel et al., 2023; Wang et al., 2022).
Ecuador also faces high university dropout rates, ranging between 12 and 30% in the early years of study, despite investments and government policies implemented in recent years with a focus on improving the quality of higher education (Buenaño et al., 2024; Núñez-Naranjo et al., 2021). Studies indicate more favorable terminal efficiency rates in private university institutions (Delogu et al., 2024). Studies such as Buenaño et al. (2024) indicate that the factors leading to school dropout in Ecuador are instructional and academic in nature, with terminal efficiency rates being more favourable in private universities. Therefore, in Ecuador, student retention is closely linked to graduation, as if the retention rate in the early levels does not reach 50%, the percentage will decrease by the time the program is completed.
University dropout not only affects families but also the government, as the resources invested are wasted, leading to increased inequality, poverty, and limited progress in scientific and academic development (Humphrey, 2008). In this context, the purpose of this study is based on the opportunity to provide updated information on university dropout rates. Accordingly, a real case study is considered within the social context of Ecuador.
University dropout is analyzed by considering temporal and spatial factors. Regarding temporality, it can be classified into initial or early dropout, early dropout, late dropout, and academic lag. Initial or early dropout occurs when students are admitted but do not enroll. Early dropout happens when students leave their studies after completing the initial levels of their program. Late dropout occurs when students leave after completing more than half of the program (Behr et al., 2020; Gutierrez-Pachas et al., 2023; Segura et al., 2022). Dropout due to academic lag refers to situations where students complete their program but do not obtain a degree due to curricular requirements or graduation processes. From a spatial perspective, university dropout addresses internal, institutional, and higher education system factors. Internal dropout involves a student’s decision to switch to another program within the same institution. Institutional dropout occurs when a student leaves their current university and resumes studies at another institution. Dropout from the higher education system occurs when a student permanently discontinues their academic pursuits (Horstschräer and Sprietsma, 2015; Salgado-Orellana et al., 2019; Wild et al., 2023).
Given the above, this study analyzes the determinants of university dropout in a private institution in the central region of Ecuador as a particular case study, using both descriptive and predictive methods. Historical data from students who began their first year in 2014 and were followed through 2019 were used for this purpose. The categories considered include academic, institutional, personal, economic, and familial factors, with specific variables. The data were collected and processed through data collection sheets and surveys. Therefore, the study begins with the following questions: What are the determining factors of dropout in higher education institutions? What are the most impactful variables in this issue? How can school dropout in a higher education institution be predicted according to relevant determining factors?
2 Approaches and related works
University dropout is a polysemic term, and much depends on the model used to study it, as well as the measures taken to reduce or eliminate it. In this study, dropout is defined as the interruption of continuous studies due to a permanent withdrawal, influenced either positively or negatively. This withdrawal involves values and behaviors associated with internal, external, and circumstantial factors, including personal, socio-familial, academic, institutional, and economic aspects. Ultimately, this interruption prevents the attainment of a professional degree. We analyze it from individual, institutional, and state or national perspectives, leading to initial or early dropout, early dropout, late dropout, and academic delay.
University dropout in Italy has been presented as a complex process involving various factors and is explored through different approaches and models, with key factors including family income, grades achieved at the secondary level, and the type of school the students come from Delogu et al. (2024). Similarly, Martelo et al. (2017) highlight that, in the case study of the University of Cartagena in Colombia, the factors driving dropout were the lack of study outside the classroom, poor interpretation skills, economic problems, and vocational guidance. In a specific case, the study conducted in Ecuador at the Technical University of Ambato by Ethington (1990) found that between 2011 and 2017, the data collected showed a 39% dropout rate in the engineering program, which was associated with an academic factor, stemming from low performance in subjects such as mathematics, physics, or chemistry. Finally, Salgado-Orellana et al. (2019) used a model to predict dropout rates in the leveling course at the National Polytechnic School, a public university in Ecuador. The relevant finding was observed using a logistic regression model and an artificial neural network model, utilizing four variables that incorporated students’ academic and socioeconomic information. Ultimately, the students at the highest risk of dropping out were those in both economic and social vulnerability, in low-demand careers, from the coastal region, enrolled in the leveling course for technical programs.
The Psychological Approach focuses on the analysis of students’ behaviors and conduct, distinguishing between those who complete their studies and those who do not. A relevant model is proposed by Fishbein and Ajzen in 1975, which demonstrates that prior behaviors, behavior, and subjective norms generate a behavioral intention to stay or dropout. According to this model, university dropout is considered the weakening of initial intentions, and retention is seen as the strengthening of these intentions. In 1986, Atináis introduced the concept of persistence and related it to the evaluation that students make upon entering university. Ethington integrates these models with Eccles’ 1983 theory and creates the achievement theory, focusing on personality traits (Ethington, 1990), and establishes that previous academic performance is a decisive factor in current performance, goals, purposes, and expectations, relating these elements to family backgrounds. In this approach, personal variables are considered attributes of the individual that influence both academic and social integration.
The Sociological Approach emphasizes the attributes of the university student and the institutional environment, without considering academic variables such as grade point average as a guarantee of retention or dropout. It is associated with Durkheim’s suicide theory in 1952, which links dropout to problems of social integration, impact on interests, skills, dispositions, and attitudes. It also relates individual variables, such as motivation, attitude, and disposition for study, with social variables within the university environment (Spady, 1970). This model is known as the “suicide theory,” where social-institutional interaction is crucial in deciding whether to face academic and social demands, potentially increasing dropout. Factors such as family and life situations also influence individual integration into the university environment and can lead to dropout.
The Economic Approach examines the cost–benefit relationship of university education (Wild et al., 2023). Students who choose to stay in university perceive that the benefits outweigh the economic costs and time invested, leading them to seek scholarships, tuition discounts, and student loans (Caire and Becker, 1967). This approach is based on the theory of human capital, which holds that individuals invest time and economic resources when they anticipate long-term economic and social benefits. Dropout occurs when educational investment yields lower income or unforeseen additional expenses (Cabrera et al., 1992; Donoso and Schiefelbein, 2007; Ishitani and DesJardins, 2002).
The Organizational Approach analyzes dropout from the perspective of institutional organization and the student experience in relation to expectations. Aspects such as teaching quality, infrastructure, class schedules, and extracurricular activities such as sports, cultural events, and additional services like medical and dental care are considered (Bean and Eaton, 2001; Bean, 1982). This model, associated with Bean, integrates social, familial, academic, and institutional variables and is based on productivity theory in a workplace context. It states that dropout is related to external factors affecting university students’ attitudes and decisions. The author compares academic dissatisfaction with job dissatisfaction. Another relevant model is Pascarella and Terenzini’s quality of effort, which groups the causes of dropout into academic, psychosocial, and institutional factors, including family backgrounds, institutional characteristics, the university environment, and the quality of the student’s effort (Braxton et al., 2000; Terenzini and Pascarella, 1980). This model is considered interactive and organizational, as dropout depends on the quality of the student’s effort to achieve their goals and their interaction with the university environment.
The Interactionist Approach refers to dropout as a product of the interaction between the institution and students (academic-personal). The greater the student’s commitment to the institution, the higher the probability of retention instead of dropout. Social integration occurs in the classroom, fostering participation in learning communities. This interaction results in benefits in terms of relationships, emotions, or rewards of some kind. Economic, familial, cultural attributes, and certain individual characteristics influence the decision to stay or leave (Braxton et al., 2000). Tinto’s model, based on studies by Durkheim and Spady, argues that students who associate with groups or have friends in the university context are less likely to drop out and compares dropout to suicide, differentiating the consequences of these two situations (Halpin, 1990). Tinto formulates a theoretical model that explains the individual versus institutional process and the interaction between them from a longitudinal perspective associated with social, familial, and personal characteristics.
In related studies, various strategies have been proposed to address university dropout, as described below. In a quantitative study by García Ochoa et al. (2021), who conducted a categorical analysis of 3,289 students, revealing a dropout rate of 25.3% in the first level. It was suggested to implement economic policies to reduce dropout by 7%, especially in relation to economic variables. In another study by Núñez-Hernández and Buele (2023), who used action research and administered surveys to 260 students. Economic factors were associated with dropout, and it was concluded that poor performance, insufficient time dedicated to studies, and economic circumstances influenced dropout. Nurmalitasari et al. (2023) employed qualitative and quantitative approaches to observe students who dropped out of private universities in Central Java, Indonesia. Findings indicated that personal economic factors, academic satisfaction, academic performance, and family economic situations were the most influential in dropout. Finally, Gonzalez-Nucamendi et al. (2023) employed quantitative variables using clustering and classification methods. Key variables associated with dropout were academic performance in the first semesters, entrance exam scores, number of attended class hours, student age, scholarship funding status, and English proficiency. In a predictive context, Hernández-Jácquez and Montes-Ramos (2020) established a model to predict the risk of dropout in upper secondary school students in Mexico. The independent variable, dropout risk, was assessed through the School Dropout Questionnaire, while the predictor variables were study habits, self-regulated learning, and learning styles (as deemed appropriate by the institution). A model was obtained that includes dimensions of study planning strategies and note-taking strategies, related to study habits; and self-efficacy for learning, related to self-regulation, explaining 37.0% of the phenomenon. Similarly, Pérez et al. (2018) presented a Model for predicting first-year student dropout at the Universidad Bernardo O’Higgins. They consolidated a tripartite matrix with data associated with the variables that emerged from the analysis of previous studies, and then analyzed the relationship of each of the explanatory variables of the study with the control variable of student dropout. A bivariate analysis was applied that allowed identifying seventeen variables significantly associated with student dropout and specifying the dependency relationships with dropout. The multivariate model predicted 86.4% of the dropout behavior, indicating seven independent categorical variables that ultimately emerged as relevant factors in the prediction model. These studies and proposals underline the importance of addressing university dropout from various perspectives and using multiple approaches to better understand this complex issue.
The importance of studying university dropout lies in its direct impact on the professional and personal development of individuals. Those who abandon their studies may face greater challenges in accessing job opportunities and improving their quality of life. Additionally, it can have repercussions on the socioeconomic level, posing challenges in terms of competitiveness and sustainable development.
From the progress made so far, the variables for analyzing dropout are based on socio-demographic factors such as gender, socioeconomic status, age, employment status, technology and health, gender, marital status, race, ethnicity, area, region, academic level, and family responsibilities, parents’ economic income, and institutional factors. In order to conduct a more comprehensive study, personal, academic, familial, economic, and institutional variables were analyzed. The guiding question of this study was: What are the main factors causing university dropout? This sets the general objective of the study as analyzing the determining factors causing university dropout, a case study of Ecuador.
3 Methodology
This research employs a quantitative approach methodology grounded in the post-positivist paradigm. This is a case study on student dropout in a private university in Ecuador, considering the available digitalized information from the institution on categorized and coded variables such as: age at entry, marital status, gender, ethnicity, region of origin, type of school, type of family, income quintile, level of study, selected major, campus, and study modality. These variables are associated with five specific factors: familial, personal, economic, academic, and institutional, as shown in Table 1.
3.1 Data collection and organization
For data collection, the technique of document review was employed, focusing on the available digital historical records of 1,078 students who were admitted and enrolled during the 2014–2019 period. This information was obtained from the Academic Student Information System (SIAE) and an institutional survey sent via email to a sample of 484 students who dropped out. The instruments used included tabulated data records and a questionnaire that asked for the reasons for dropping out based on the aforementioned factors. These students (both men and women) were aged between 17 and 58 years and graduated from both public and private secondary education institutions located in various regions of the country. A noteworthy aspect is that these students pursued their studies either in-person or semi-presential at the Main Campus/Headquarters or the Extension Branches.
The detailed information from the SIAE facilitated the distribution of the population, which was divided into five categories: A (graduates), B (active students), C (students who changed majors), D (re-enrolled students), and E (students who dropped out).
Category A refers to graduates, who are students who have completed their studies and graduated, totaling 186 students. Category B includes students who are still in university without having graduated, despite repeating one or more levels, and this group consists of 279 students. Category C encompasses those who have decided to change majors within the same university, with 23 students. Category D refers to re-entered students, totaling 106. Finally, Category E represents the dropouts, which includes students who permanently withdrew from the university. This group comprises 484 students and was considered the sample for the in-depth descriptive study. The distribution is shown in Table 2.
For the interpretation of the results, a descriptive analysis was first applied, and for greater consistency in predictive analysis, tabulation, diagramming, and application of the Multilevel Logistic Regression model Constate-Amores et al. (2020) were used. This study covered both the “Main Campus” and the “Extension/Branch” locations of the institution, considering the student population enrolled in both face-to-face and semi-face-to-face modalities. For the initial comparative descriptive analysis, information associated with personal, familial, economic, academic, and institutional conditions was extracted, compiled based on the references consulted that have addressed the issue of dropout based on student decision and/or persistence or retention, seen from the institution’s perspective. The selected factors are detailed in Table 2.
In the second phase, a predictive model was configured supported by determining the significant variables related to the characteristics and entry conditions of the students entering the university. Prior to this, an inquiry was conducted into the relationship of each of the explanatory variables (independent variables) with the student retention variable (dependent variable) of the students at the university under study, by applying the Chi-square Test and Fischer’s Exact Test (categorized qualitative variables) based on the frequency reflected in the contingency tables generated using the IBM SPSS v29 program.
The main condition of the model is that the binary dependent variable Y takes the code values of 1 if the student drops out and 0 if they remain (retained).
The independent variables of the study will be identified as X1, X2, X3, …, Xi. In this context, the model designed to fulfill the objective of determining the probability that the variable Y is 1, given X1, X2, …, Xi (P(Y | X1, X2, …, Xi)) is:
P(Y | X1, X2, X3, …, Xi) = g(X1, X2, X3, …, Xi; β) where the probabilistic function g is the link function with values between [0,1], and its value depends on a vector of parameters β, which in the case of binary logistic regression is formulated as shown in Equation (1):
The explanatory variables of entry characteristics and conditions (independent variables selected for this study) are extracted from the record that was structured at the beginning of the admission and enrollment processes of the cohorts and the annual student characterization survey conducted by the institution’s admissions department. The dependent variable is specified in Table 3, with a value of 0 assigned to students who drop out and 1 assigned to students who do not drop out (retained) in the regression model.
4 Results
4.1 Descriptive analysis of historical records
According to the proposed methodology, a summary of the collected information on student data registered during the study period is presented to make a comparison of the previously identified categories. Despite the high dropout rate, a significant percentage of graduates and continuing students are observed. However, the majority of those who enroll fail to graduate, indicating that dropout is a significant issue. The following categorical analysis is presented by campus, modality, major, age, ethnicity, marital status, gender, region, type of school, type of family, quintile, and level.
4.2 Pre-entry
4.2.1 Age-based
This category refers to the distribution by age, divided into two groups. Young students aged 17–19 represent 26%, while those aged 20–58 constitute 74%. Those who entered university at a young age make up the majority of graduates and continuing students (24 and 30%, respectively). Meanwhile, students in categories D and E belong to the older age group (10 and 49%, respectively). There is significant diversity in terms of ages.
In Category E, which constitutes the study sample, 65 students showed early or initial dropout upon admission but did not complete their enrollment. Of these, 77% were over 30 years old, indicating that retention challenges are more significant for this group. These data provide a better understanding of the needs of students at different stages of their academic life and the development of effective retention and graduation strategies based on older age groups.
4.2.2 Ethnicity-based
The Afro-Ecuadorian population represents 0.28%, with the majority of them belonging to Category D, showing a trend towards withdrawal. White people constitute 1.11% and are more prevalent in Category C. Indigenous people represent 1.86%. Mestizos represent 96.66%, and Montuvians represent 0.09%, with the majority of them belonging to Category D.
Comparing Categories A, B, C, D, E reveals significant variations in relation to ethnicity, with mestizos being the largest group in all categories. Additionally, mestizos are the most successful group in terms of graduation, while indigenous people manage to graduate despite challenges and have a high representation in changing majors. White people have a significant presence in Category B, indicating their commitment to achieving their goals without dropping out. Regarding reentries, Afro-Ecuadorians and Montuvians have low proportions. These data highlight notable differences in educational patterns among different ethnicities. Specifically analyzing Group E, of the 65 students who dropped out early, the highest percentage of dropout was reflected in the Montuvio ethnicity, with 48%.
4.2.3 According to marital status
The marital status “Single” is the most representative, covering 86.4% of the total population. In Category A, there is a relatively even distribution in terms of marital status, with “Married” being the most common at 19.1% and “Divorced” the least common at 10.0%. Category B exhibits a more varied distribution, with “Married” being predominant at 33.0%. In contrast, Category C shows generally low representation.
Category D presents a more even distribution, and Category E reveals a higher representation of “Single” individuals, followed by “Married” individuals (45.5 and 41.7% respectively). Categories A and C show more uniform distributions in terms of marital status, suggesting that these categories may depend less on individuals’ marital status. On the other hand, Categories B and E demonstrate more diverse distributions in terms of marital status. It appears that marital status influences academic trajectories, as “Single” individuals achieve higher academic outcomes in all categories. Although the population of “Divorced” and “Widowed” individuals is small, they are present in all categories. Among the 65 students who dropped out early, 64.61% were married, 26.15% were divorced, and the remainder were single.
4.2.4 According to gender
The male gender had higher enrollment in 2014, representing 62.2%, compared to the female gender, which represented 37.8%. This particularity emphasizes that for every woman entering university, two men do so. In this category, a notable characteristic is presented, as the dropout rate is higher among men than among women (48.9 and 38.3%, respectively), indicating that for every two male students enrolled, at least one drops out, while for every three female students enrolled, one drops out.
Despite the male gender having greater access to university, it is women who graduate in greater numbers, stay longer in the institution, and have lower dropout rates. This situation may be influenced by robust trends. On the other hand, the male gender shows a high dropout rate, a situation that could be attributed not only to the process of adaptation and integration into the private university environment but also to factors related to economics and other personal, social, economic, institutional, and academic variables. Regarding the early dropout of the 65 students who did not enroll, 70.76% were male, which aligns with the previously mentioned trend.
4.2.5 According to region
An unequal distribution of categories was detected across different regions. The Sierra region has the largest population, followed by the Oriente and the Costa. In Category A, the Costa region has the highest proportion of graduates, followed by the Sierra and the Oriente (21.1, 17.9, and 7.5%, respectively). On the other hand, Category E shows a higher incidence of students from the Sierra, followed by Categories B, A, D, and C (43.6, 26.7, 17.9, 9.6, and 2.2%, respectively). Dropout and retention rates are notable in all three regions. These data reflect the influence of students’ geographical location on their academic outcomes.
4.2.6 By type of school
Figure 1 shows that the majority of students enrolled in the first level in 2014 primarily come from public schools, followed by private, municipal, and state schools (48.9, 40.4, 5.6, and 5.1%, respectively). In categories A, B, and D, students from public schools lead, followed by students from private schools, while those from municipal and state schools have relatively low percentages. In category E, dropout rates are high among students from public schools and low among those from private schools (51.7 and 38.8%, respectively). The type of school attended by students has an impact on retention and dropout rates, as high dropout rates are observed among students from public schools.
4.2.7 By type of family
Students who graduate most frequently primarily come from nuclear and extended families (26.6 and 22.5%), the same is true in category B (35.5 and 50.5%), and category D (13.6 and 16.2%), while those with the lowest graduation and retention rates come from reconstructed families (1.1 and 2.7%). The population in category C is not significant compared to the other categories but shows similar trends to the previous categories.
In category E, there is a marked difference as dropouts primarily come from reconstructed families, followed by separated families (92.6 and 62.4%). Students from nuclear and extended families have higher rates of graduation and retention, suggesting that a stable family environment is important in the academic process. On the other hand, those from separated and single-parent families show higher rates of changing majors and re-entry, which may be related to additional challenges they face within their families.
4.2.8 According to the quintile (quartile)
Students from the “High,” “Upper Middle,” and “Middle Typical” quintiles seem to be associated as they tend to graduate (25, 21.4, and 24.4%) and remain in higher proportions (41.7, 44.6, and 39.3%). Students from the “Low” quintile have higher rates in categories C and D (40.0 and 60%). In Category E, there is a complex relationship with the “Lower Middle,” “Middle Typical,” and “High” quintiles, as they present relatively significant dropout rates (56.5, 14.3, and 33.3%). These findings suggest that quintiles may be associated with academic performance and students’ academic decisions.
This identification of levels of wealth or poverty allows us to see that the majority of students entering the institution do not have the financial resources to sustain themselves and cover expenses at a private university. This is primarily due to two reasons: first, students who progress from one level to another often apply for admission to other public institutions of higher education, and second, when they do not pass a subject or semester, they must pay fees for credits that are not budgeted, making it difficult to continue their higher education and increasing the likelihood of dropping out.
4.3 Characteristics and behavior of data for the post-admission stage
This section systematically presents information on students who have passed the admission process and completed formal enrollment at the university, totaling 419 students. Both frequency and percentage distribution are specified for each segmented category of the study, according to each factor and study variables. Among personal factors, entry age stands out as more than 60% of students were over 20 years old. Marital status is predominantly single at over 77%, and gender is predominantly male at 60.38%. Additionally, 46.30% of these dropouts belonged to the Montuvios ethnicity.
Regarding family information, 49.16% came from the coastal region, with 74.22% having graduated from public secondary education institutions and 40.81% belonging to extended families. In terms of economic factors, the majority, 58.95%, were from the lower quintile, while academic factors highlight that most dropouts, 52.98%, occurred in the first cycle of the career. Finally, institutional factors showed that the career with the highest dropout rate was Industrial Engineering at 22.91%, with 57.52% belonging to the Main Campus, where 72.08% studied in person.
The following table details all specific values by factor and variables:
From Table 4, it can be observed that the factor with the highest average percentage was personal type at 61.10%. The second highest was the economic factor at 58.61%, while the family factor was third with 54.63%, followed by the institutional factor at 50.84%, and lastly, the academic factor with 10%. This suggests that dropout in the early cycles of the career is attributed to factors related to prior education before university entry.
In addition, the information received to date from students who responded to the email survey was processed. The questionnaire contained a single question asking students to indicate the factor associated with their decision to abandon their university studies, with personal, family, and economic problems ranking as the top three. Figure 1 displays the percentage distribution of these factors.
4.4 Predictive analysis
Before conducting the multivariate analysis, the relationship between the explanatory variables and university dropout or retention was established by testing hypotheses for each case. Given that the dependent variable Y (dropout or retention) is considered, 12 specific variables were analyzed in the statistical process. These variables were categorized into 12 data groups: age, ethnicity, marital status, gender, region of origin, type of school, type of family, income quintile, campus, mode of study, career, and level achieved. Table 5 displays the results of the bivariate analysis with their respective statistical tests, including Pearson’s Chi-square and Fisher’s exact test. The hypothesis was based on testing the association of each variable with the dependent variable Y. It is worth noting that for significance values less than 0.05, the hypothesis of each test is accepted, indicating a relationship between the variables under study.
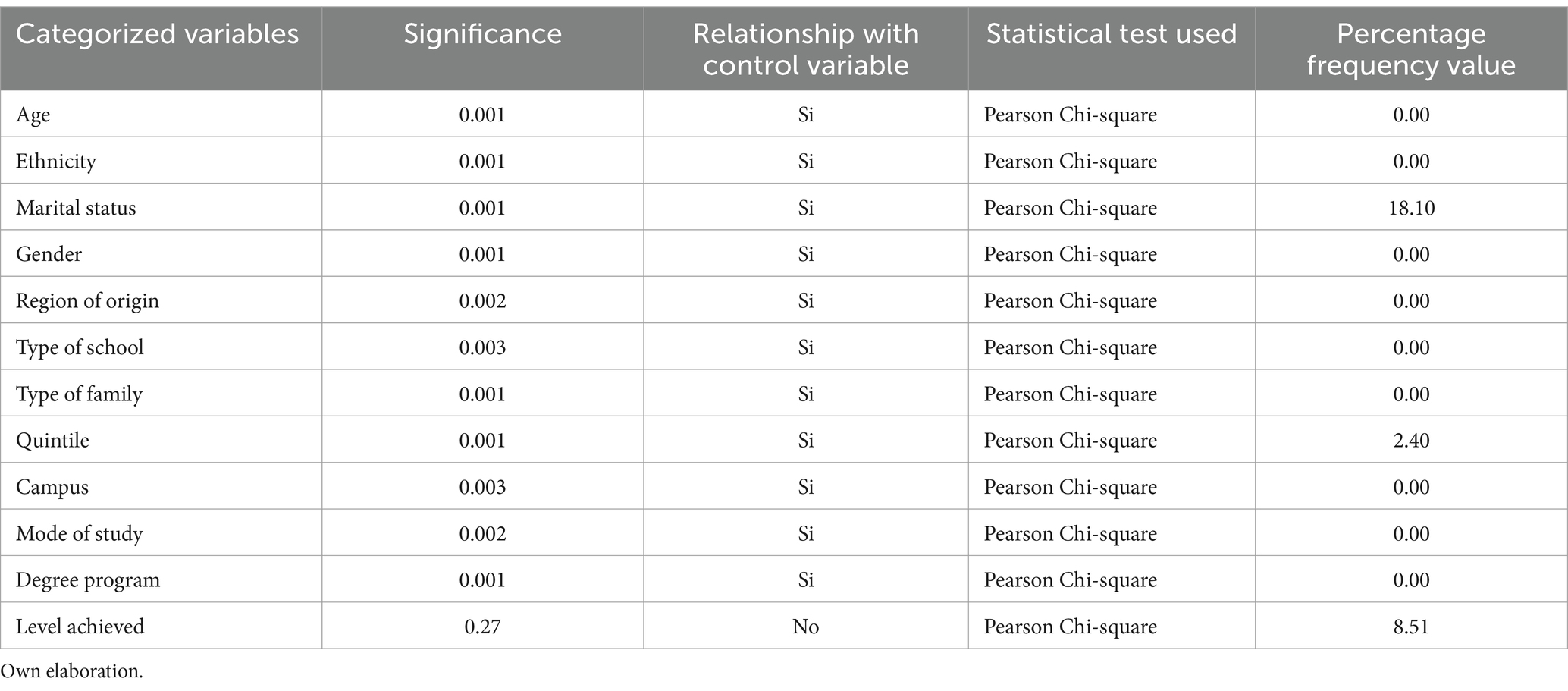
Table 5. Significance of explanatory variables in bivariate analysis against the control variable retention.
Table 5 shows that for significance values less than 0.005, there is an inferred relationship between the categorized variable and the dependent variable Y (dropout). Specifically, the variables age, ethnicity, marital status, student’s gender, region of origin, type of school, type of family, income quintile, campus, mode of study, and career are all related to the dependent variable.
The specific relational analysis is presented according to the percentage distribution of each independent variable against the dependent variable Y, as shown in Table 6. The collected information reveals that the variables associated with a lower percentage of school dropout are as follows: for the age variable, students aged 19 to 20 years showed a dropout rate of 10%; for ethnicity, mestizos reflected a 23.34% dropout rate; for marital status, married individuals exhibited a 31.70% dropout rate. Regarding gender, females had a dropout rate of 37.40%. For region of origin, students from the Sierra region had a 36.70% dropout rate, while students from private institutions showed a 31.80% dropout rate.
In terms of family type, students from extended family structures had a dropout rate of 26.50%, and according to income quintile, those from the middle quintile had a 14.30% dropout rate. Concerning the campus, students studying at the Extension/satellite campus had a 15.10% dropout rate, and those in the semi-presential study mode had a 22.63% dropout rate. For the career variable, dropout rates were quite similar, with Architecture having the lowest percentage at 45.00%. Finally, according to the level achieved or year completed, the tenth year had the lowest dropout rate at 0.40%. However, it is noteworthy that with a 52.90% dropout rate in the first year, which decreases year after year, this figure appears almost negligible.
4.5 Multivariate analysis of logistical regression of student retention
Processing the data using the SPSS program, considering the encoding of the managed independent variables, the Hossmer-Lemeshow test is first developed which allows to obtain the classification table, the information of which is appreciated in Table 7.
Table 7 makes it possible to interpret that 74.60% of the data has been accepted and processed correctly, providing the variables of the model equation with the values observed in Table 8.
The following is the explanation of the summary of the model which highlights the probability of the same in the Table 9.
To measure the overall fit of the proposed model, the results of the Hossmer-Lemeshow test are presented which must generate a significance greater than 0.05. Table 10 confirms this condition.
The value of significance was placed at 0.392 above the value of 0.05, which ensures that the model has a high level of reliability and can be of great use in predicting the behavior of the variables under study. The correctly adjusted classification table is presented below, as shown in Table 11.
The value of 76.44% allows us to infer that in future events applying the current model, there will be a 76.44% success rate in predicting the behavior of the dependent variables. Therefore, this model can be considered to have acceptable efficiency. In this regard, the conditions to be verified are as follows:
Ho: Bi = 0, then the variable Xi is not significant H1: Bi ≠ 0, then the variable Xi is significant.
Finally, the variables (Xi) that should be present in the final model equation are presented. The results show that statistically, the variables region of origin and the career do not form part of the final model equation according to the value of Bi, in addition to the significance of each, as shown in Table 12.
Based on the results achieved, it can be inferred that the determinants for this study associated with university student dropout are of a personal nature (age, marital status, gender, and ethnicity), family-related (region of origin, type of school, and type of family), economic (income quintile), academic (level achieved), and institutional (campus and mode of study). The model in general shows a special relationship in terms of dropout or permanence, that is, EXP (B) of 0.87 indicates that (1–0.870) x 100 = 13%, which indicates that a student between 17 and 19 years of age has a probability of dropping out, while those between 20 and 58 years of age can drop out of university with a probability of 87%.
5 Discussion
In the first phase of this work, a categorical analysis of attrition is carried out using data from university students during a 5-year study period. Subsequently, a comparison is made with other research to identify the most significant findings. It was found that the population in this study, although substantial in size, was smaller than that of other research, such as García Ochoa et al. (2021), which focused on the economic factor of students and reported a first-level dropout rate of 25.3%. This is comparable to the first level dropout rate of this study, which reached 23.7%. It should be noted that both studies were conducted in private institutions and shared a quantitative approach and a categorical analysis.
In the study by Núñez-Hernández and Buele (2023), although their representative sample was smaller than that of this study, the authors analyzed sociodemographic and technological variables specific to distance education, using information from students enrolled in the second, fourth and seventh levels. This study also highlighted the economic factor as the main driver of attrition. There were similarities with the current study, such as the fact that the majority of students were from public secondary institutions. However, Núñez-Hernández and Buele (2023) reported a dropout rate of 60%, in contrast to the results of this study and García Ochoa et al. (2021), where the dropout rate was lower. In addition, Núñez-Hernández and Buele (2023) mentioned that no student over 40 years of age dropped out, whereas in this study, ages 20–58 years showed a dropout rate of 49%.
The study by Nurmalitasari et al. (2023) focused on private institutions, similar to García Ochoa et al. (2021) and Núñez-Hernández and Buele (2023). Their findings emphasized that economic, personal, academic satisfaction, academic performance, and family economic status factors were the most influential in attrition. Compared to this study, personal and socio-family factors were more prominent in attrition. In addition, Nurmalitasari et al. (2023) reported that 56% of their students did not enter higher education immediately after high school, a value similar to this study, which corresponded to 74%. Reference was also made to income levels, as 53% of the study population had below-average income, as did this study, which showed 72.4% with below-average income. Furthermore, in Nurmalitasari et al. (2023), engineering students had an attrition rate of 79%, while in this study, engineering majors had an attrition rate of 29.1%.
Gonzalez-Nucamendi et al. (2023), analyzed a significantly larger population, and the reported attrition rate was 8.5%. This value is much lower than that observed in this study, despite the fact that both institutions are private and located in developing countries, which could be considered atypical. Gonzalez-Nucamendi et al. (2023) used quantitative variables and ranking methods, highlighting that academic performance during the first weeks of the first semester, entrance exam scores, number of class hours taken, student age, scholarship funding status, and English proficiency were the main variables associated with attrition.
In relation to Hernández-Jácquez and Montes-Ramos (2020) together with Pérez et al. (2018) who used the principles of predictive and inferential statistics to address the determinants of dropout or dropout at the university level. Their contributions show the importance of knowing the behavior of the independent variables of an economic, social, academic, personal and cultural nature to establish a relationship with the dependent variable dropout or permanence within the course of a university career. In this sense, the multivariate analysis, using the theory of the Multilevel Logistic Regression model.
In comparison with previous studies conducted in Ecuador by Buenaño et al. (14), their results are not fully aligned with the findings of this study, as they only highlighted institutional and academic factors. However, they do agree that retention or non-dropout can be associated with the type of private institution of origin. On the other hand, Mena et al. (22) determined that academic factors were the primary cause of dropout in the Faculty of Engineering at the Technical University of Ambato, while Sandoval-Palis et al. (23) highlighted student dropout related to factors associated with both economic and social vulnerability of the students, which corresponds with the findings of the present study.
5.1 Principio del formulario
This work identified significant differences in several factors that influence college dropout, including institutional, personal, socio-familial and academic factors. These findings highlighted that dropout and graduation is a function of the behavior of explanatory variables such as campus, modality, age, gender, region, type of school, type of family, socioeconomic quintile, and level of study. Retention and graduation policies and actions should take these factors into account to improve graduation rates and reduce dropout. Finally, it is important to mention that this study had an important limitation in that it relied on a purely quantitative methodology. Therefore, future work could consider mixed approaches to analyze the reasons for college dropout from a broader perspective.
Similarly, future researchers are encouraged to address this issue from a longitudinal perspective, delving deeper through qualitative or mixed methods approaches, including in-depth literature reviews or systematic reviews with meta-analyses. This will help to highlight the contributions of various authors and encourage the international scientific community to tackle this truly concerning problem that can impact the normal development of educational plans in both public and private higher education institutions. It is also suggested that these institutions review and update their comprehensive student support policies, extending beyond an academic perspective. The goal is to enhance both the internal and external environments where students can achieve a harmonious balance and contribute to the reduction of dropout rates in higher education.
It is important to mention that this study had a significant limitation by relying on a quantitative methodology based on historical records of students who left their studies during the given period. Therefore, future work could consider mixed-method approaches to predict the reasons for university dropout from a broader perspective.
6 Conclusion
This study highlights significant differences in various factors influencing school dropout. Through the initial study and descriptive statistical analysis, it was found that early dropout before enrollment among 65 students was primarily associated with personal, economic, and family factors, with most being over 30 years old.
In the post-admission study of the group consisting of 419 students, the prominent factors were personal, categorized by marital status (divorced), gender (male), and ethnicity (Montubio). Economic factors were the second most significant, categorized by low-income quintiles. Family factors ranked third, categorized by coastal origin region, public school type, and reconstructed family type.
Institutionally, it was observed that the Extension site has a lower dropout rate and a higher graduation rate compared to the Main Campus. Regarding the mode of study, face-to-face education shows a higher graduation rate, while blended learning experiences a higher dropout rate. Concerning personal factors, the age at which students enter higher education influences dropout, with those entering immediately (ages 17 to 20) showing greater retention.
Gender is also a relevant variable; despite higher enrollment of male students, they exhibit a higher dropout rate and lower graduation rate. Conversely, female students show a lower dropout rate. These results underscore the need for specific policies and strategies to address these differences in institutional, personal, social, and academic factors. Additionally, it is imperative to suggest that future research using quantitative or mixed methods could provide a deeper understanding of university dropout.
Given the study results, it can be noted that dropout in the case study is marked by personal, family, and economic factors, with high dropout rates in the early cycles of the degree possibly associated with prior inadequate education of students from public institutions, mainly located in coastal areas, which may represent a current gap for future specific studies.
Finally, it can be suggested that by using the principles of the Multilevel Logistic Regression model, universities could periodically analyze dropout behavior and thereby establish strategies to better integrate students into the university environment.
Data availability statement
The raw data supporting the conclusions of this article will be made available by the author, without undue reservation.
Ethics statement
Ethical review and approval was not required for the study on human participants in accordance with the local legislation and institutional requirements. Written informed consent from the participants was not required to participate in this study in accordance with the national legislation and the institutional requirements.
Author contributions
AN-N: Writing – review & editing, Writing – original draft, Visualization, Validation, Supervision, Software, Resources, Project administration, Methodology, Investigation, Funding acquisition, Formal analysis, Data curation, Conceptualization.
Funding
The author(s) declare financial support was received for the research, authorship, and/or publication of this article. This work was funded by the employer, Universidad Indoamérica. For its support in the development of this research under the project IIDI-001-23.
Conflict of interest
The author declares that the research was conducted in the absence of any commercial or financial relationships that could be construed as a potential conflict of interest.
Publisher’s note
All claims expressed in this article are solely those of the authors and do not necessarily represent those of their affiliated organizations, or those of the publisher, the editors and the reviewers. Any product that may be evaluated in this article, or claim that may be made by its manufacturer, is not guaranteed or endorsed by the publisher.
References
Al-hawamdeh, B. O. S., and Alam, S. (2022). Praxis and effectiveness of pedagogy during pandemic: an investigation of learners’ perspective. Educ. Res. Int. 2022, 1–9. doi: 10.1155/2022/3671478
Alvarado-Uribe, J., Mejía-Almada, P., Masetto Herrera, A. L., Molontay, R., Hilliger, I., Hegde, V., et al. (2022). Student dataset from Tecnologico de Monterrey in Mexico to predict dropout in higher education. Data 7:119. doi: 10.3390/data7090119
Álvarez Ferrandiz, D. (2021). Análisis del abandono universitario en España: un estudio bibliométrico. publicaciones 52, 241–261. doi: 10.30827/publicaciones.v52i2.23843
Bean, J. P. (1982). Conceptual models of student attrition: how theory can help the institutional researcher. New Dir. Inst. Res. 1982, 17–33. doi: 10.1002/ir.37019823604
Bean, J., and Eaton, S. B. (2001). The psychology underlying successful retention practices. J. Coll. Stud. Retent. 3, 73–89. doi: 10.2190/6r55-4b30-28xg-l8u0
Behr, A., Giese, M., Teguim Kamdjou, H. D., and Theune, K. (2020). Dropping out of university: a literature review. Rev. Educ. 8, 614–652. doi: 10.1002/rev3.3202
Braxton, J. M., Milem, J. F., and Sullivan, A. S. (2000). The influence of active learning on the college student departure process: toward a revision of Tinto’s theory. J. High. Educ. 71:569. doi: 10.2307/2649260
Buenaño, E., Beletanga, M. J., and Mancheno, M. (2024). What factors are relevant to understanding dropout? Analysis at a co-financed University in Ecuador and Policy Implications, using survival cox models. J. Latinos Educ. 23, 1400–1415. doi: 10.1080/15348431.2023.2271570
Cabrera, A. F., Nora, A., and Castaneda, M. B. (1992). The role of finances in the persistence process: a structural model. Res. High. Educ. 33, 571–593. doi: 10.1007/BF00973759
Caire, G., and Becker, G. S. (1967). Human capital, a theoretical and empirical analysis with special reference to education. Revue Économique 18:132. doi: 10.2307/3499575
Constate-Amores, A., Florenciano Martínez, E., Navarro Asencio, E., and Fernández-Mellizo, M. (2020). Factores asociados al abandono universitario. Educación XX1 24, 17–44. doi: 10.5944/educxx1.26889
De La Cruz-Campos, J.-C., Victoria-Maldonado, J.-J., Martínez-Domingo, J.-A., and Campos-Soto, M.-N. (2023). Causes of academic dropout in higher education in Andalusia and proposals for its prevention at university: a systematic review. Front. Educ. 8:1130952. doi: 10.3389/feduc.2023.1130952
Delogu, M., Lagravinese, R., Paolini, D., and Resce, G. (2024). Predicting dropout from higher education: evidence from Italy. Econ. Model. 130:106583. doi: 10.1016/j.econmod.2023.106583
Donoso, S., and Schiefelbein, E. (2007). Análisis de los modelos explicativos de retención de estudiantes en la universidad: una visión desde la desigualdad social. Clin. Pract. Guide. 33, 7–27. doi: 10.4067/s0718-07052007000100001
Enguídanos, D., Aroztegui, J., Iglesias-Soilán, M., Sánchez-San-José, I., and Fernández, J. (2023). Academic emotions and regulation strategies: interaction with higher education dropout ideation. Educ. Sci. 13:1152. doi: 10.3390/educsci13111152
Ethington, C. A. (1990). A psychological model of student persistence. Res. High. Educ. 31, 279–293. doi: 10.1007/BF00992313
Fishbein, M., and Ajzen, I. (1975). Belief, Attitude, Intention, and Behavior: An Introduction to Theory and Research. Reading, MA: Addison-Wesley.
García Ochoa, J. J., Mendoza León, J., Serna Gómez, H., and Sánchez Arenas, R. (2021). Identificación de variables que inciden en la deserción de estudiantes de educación superior en un contexto de pandemia: un estudio de caso en Colombia. Revista de Investigación Académica Sin Frontera: División de Ciencias Económicas y Sociales 36:154. doi: 10.46589/rdiasf.vi36.425
Gonzalez-Nucamendi, A., Noguez, J., Neri, L., Robledo-Rella, V., and García-Castelán, R. M. G. (2023). Predictive analytics study to determine undergraduate students at risk of dropout. Front. Educ. 8:1244686. doi: 10.3389/feduc.2023.1244686
Gutierrez-Pachas, D. A., Garcia-Zanabria, G., Cuadros-Vargas, E., Camara-Chavez, G., and Gomez-Nieto, E. (2023). Supporting decision-making process on higher education dropout by analyzing academic, socioeconomic, and equity factors through machine learning and survival analysis methods in the Latin American context. Educ. Sci. 13:154. doi: 10.3390/educsci13020154
Halpin, R. L. (1990). An application of the Tinto model to the analysis of freshman persistence in a community college. Community Coll. Rev. 17, 22–32. doi: 10.1177/009155219001700405
Hernández-Jácquez, L. F., and Montes-Ramos, F. V. (2020). Modelo predictivo del riesgo de abandono escolar en educación media superior en México. Ciencia UAT, 15, 75–85. doi: 10.29059/cienciauat.v15i1.1349
Horstschräer, J., and Sprietsma, M. (2015). The effects of the introduction of bachelor degrees on college enrollment and dropout rates. Educ. Econ. 23, 296–317. doi: 10.1080/09645292.2013.823908
Humphrey, K. B. (2008). Bottom line: new sails for the recruitment, retention, and learning ship. About Campus 13, 2–3. doi: 10.1002/abc.239
Ishitani, T. T., and DesJardins, S. L. (2002). A longitudinal investigation of Dropout from college in the United States. J. Coll. Stud. Retent. 4, 173–201. doi: 10.2190/V4EN-NW42-742Q-2NTL
Lorenzo-Quiles, O., Galdón-López, S., and Lendínez-Turón, A. (2023). Factors contributing to university dropout: a review. Front. Educ. 8:1159864. doi: 10.3389/feduc.2023.1159864
Martelo, R. J., Jimenez-Pitre, I., and Villabona-Gómez, N. (2017). Determinación de factores para deserción de estudiantes en pregrado a través de las técnicas lluvia de ideas y MICMAC. Espacios 38, 2–9. Available at: https://www.revistaespacios.com/a17v38n20/a17v38n20p24.pdf#:~:text=El%20objetivo%20del%20presente%20estudio%20es%20la%20determinaci%C3%B3n%20de%20factores
Mascia, M. L., Agus, M., Cabras, C., Bellini, D., Renati, R., and Penna, M. P. (2023). Present and future undergraduate students’ well-being: role of time perspective, self-efficacy, self-regulation and intention to drop-out. Educ. Sci. 13:202. doi: 10.3390/educsci13020202
Mundial, B. (2017). La educación superior se expande en América Latina y el Caribe, pero aún no desarrolla todo su potencial : Comunicado de Prensa. Available at: https://www.bancomundial.org/es/news/press-release/2017/05/17/higher-education-expanding-in-latin-america-and-the-caribbean-but-falling-short-of-potential
Núñez-Hernández, C., and Buele, J. (2023). Factors influencing university dropout in distance learning: a case study. J. High. Educ. Theory Pract. 23, 29–38. doi: 10.33423/jhetp.v23i14.6379
Núñez-Naranjo, A. F., Ayala-Chauvin, M., and Riba-Sanmartí, G. (2021). Prediction of university dropout using machine learning. Inform. Technol. Syst. 1, 396–406. doi: 10.1007/978-3-030-68285-9_38
Nurmalitasari, A. L., Awang Long, Z., and Faizuddin Mohd Noor, M. (2023). Factors influencing dropout students in higher education. Educ. Res. Int. 2023, 1–13. doi: 10.1155/2023/7704142
Pérez, A. M., Escobar, C. R., Toledo, M. R., Gutierrez, L. B., and Reyes, G. M. (2018). Modelo de predicción de la deserción estudiantil de primer año en la Universidad Bernardo O’Higgins. Educ. Pesqui. 44:94. doi: 10.1590/s1678-4634201844172094
Salgado-Orellana, N., Berrocal de Luna, E., and Sánchez-Núñez, C. A. (2019). Intercultural education for sustainability in the educational interventions targeting the Roma student: a systematic review. Sustain. For. 11:3238. doi: 10.3390/su11123238
Segura, M., Mello, J., and Hernández, A. (2022). Machine learning prediction of university student dropout: does preference play a key role? Mathematics 10:3359. doi: 10.3390/math10183359
SIAE (2019). Academic Student Information System. Available at: https://sga.uti.edu.ec/login?next=/
Spady, W. G. (1970). Dropouts from higher education: an interdisciplinary review and synthesis. Interchange 1, 64–85. doi: 10.1007/BF02214313
Terenzini, P. T., and Pascarella, E. T. (1980). Toward the validation of Tinto’s model of college student attrition: a review of recent studies. Res. High. Educ. 12, 271–282. doi: 10.1007/BF00976097
Vadivel, B., Alam, S., Nikpoo, I., and Ajanil, B. (2023). The impact of low socioeconomic background on a Child’s educational achievements. Educ. Res. Int. 2023, 1–11. doi: 10.1155/2023/6565088
Wang, Z., Huang, J., Chen, C., and Fukushima, S. (2022). Design of Prediction-Based Controller for networked control systems with packet dropouts and time-delay. Math. Probl. Eng. 2022, 1–12. doi: 10.1155/2022/9437955
Keywords: university dropout, determinants of dropout, Ecuadorian higher education, student retention, multifactorial analysis
Citation: Núñez-Naranjo AF (2024) Analysis of the determinant factors in university dropout: a case study of Ecuador. Front. Educ. 9:1444534. doi: 10.3389/feduc.2024.1444534
Edited by:
Ana B. Bernardo, University of Oviedo, SpainReviewed by:
Rubia Cobo-Rendon, University for Development, ChileKarla Andrea Lobos, University of Concepcion, Chile
Copyright © 2024 Núñez-Naranjo. This is an open-access article distributed under the terms of the Creative Commons Attribution License (CC BY). The use, distribution or reproduction in other forums is permitted, provided the original author(s) and the copyright owner(s) are credited and that the original publication in this journal is cited, in accordance with accepted academic practice. No use, distribution or reproduction is permitted which does not comply with these terms.
*Correspondence: Aracelly Fernanda Núñez-Naranjo, ZmVybmFuZGFudW5lekB1dGkuZWR1LmVj