- 1Department of Linguistics, Center of Language Sciences, Faculty of Medicine, Health and Human Sciences, Macquarie University, Sydney, NSW, Australia
- 2Department of Psychological and Brain Sciences, University of Iowa, Iowa, IA, United States
- 3Department of Linguistics, University of Iowa, Iowa, IA, United States
In the literature, we encounter papers reporting manipulating pitch contours in speech tokens for a specific problem to be addressed in experiments (e.g., learning pitch patterns superimposed onto a pseudo-syllable), usually in the field of Speech Perception and Spoken Word Recognition. This type of research often tests listeners’ perceptual and processing skills in tonal languages (e.g., Mandarin, Thai, etc.), and requires superimposing a pitch contour onto a spoken syllable. However, very few studies reported in detail how this critical manipulation was done to meet specific experimental needs. In addition, there was neither specific guideline or description of the techniques being used, nor how ‘natural’ these manipulated tokens sounded in a particular language upon speech synthesis. Because this technique is crucial in establishing the conclusions in various studies, here, we will demonstrate our method of establishing this technique of tone superimposition (i.e., lexical tones in Mandarin) onto English syllables. In line with the open science model, we will also show our stimuli and procedures via OSF for readers to evaluate the validity of this technique. Manipulating the pitch contour in a spoken syllable can be complicated and change the perception of the spoken syllable in a significant way. Thus, we will also show the important factors to be considered in this process for doing research in Speech Sciences.
1 Introduction
Lexical tone is a highly prevalent phonetic cue in human languages, featured in about 40% of languages (Dryer and Haspelmath, 2013). It is distinct from phonemic contrasts that distinguish words, in that the pattern of the pitch, which spans the whole syllable, contrasts distinct lexical items. For example, Mandarin has four lexical tones corresponding to four distinct pitch contours. These are typically represented numerically (Tone 1–4) (Chao, 1968; Howie, 1976). Critically, these four tones operate similarly to any phonemic distinction: for example, ma1 ‘mother’, ma2 ‘hemp’, ma3 ‘horse’, ma4 ‘scold’ mean four distinct as in Figure 1.
From the perspective of speech perception, tone is unique. Tone is predominantly cued by the pitch or F0 (or rather, the change in F0) across the syllable. In non-tonal languages, like English, pitch movements are generally used to differentiate emotions, contrast questions and statements, or to indicate stress and focus (Gussenhoven, 2004). That is, pitch serves as suprasegmental or indexical role. In contrast, in a tonal language such as Mandarin Chinese, systematic variation in pitch contours must be integrated with segmental information to disambiguate lexical items. Thus, tone raises complex questions as it requires listeners to parse the tone from a background signal that may contain significant variation due to these suprasegmental properties, and it requires listeners to use a much larger span of the syllable (than most other phonemically contrastive cues).
Given this complexity, as well as the importance of tonal languages more broadly, there has been an explosion of work on the role of tone in speech perception and spoken word recognition in the monolingual speakers (particularly Mandarin) (e.g., Chandrasekaran et al., 2010; Chen and Peng, 2018; Francis et al., 2003, 2008; Gandour, 1983; Liu et al., 2023; Liu et al., 2022; Liu and Samuel, 2007; Maggu et al., 2021; Malins and Joanisse, 2010; Mitterer et al., 2011; Peng et al., 2010; Shuai and Malins, 2017; Wiener and Turnbull, 2016; Wu et al., 2019; Wu and Ortega-Llebaria, 2017; Xu et al., 2006). Moreover, because of this difference in the status of supra-segmental information between languages, bilinguals of one tonal language and one non-tonal language (e.g., Mandarin-English) offer a unique window to understand the interplay between linguistic processing and representation at the supra-segmental level, which is not used to contrast words in the non-tonal languages, versus the segmental level in bilingual individuals. Thus, in recent years, a number of studies have begun investigating tone in the bilingual context and its role in bilingual language processing (e.g., Wang, 2021; Wang et al., 2017, 2020).
These kinds of investigation often require sophisticated techniques for manipulating the pitch pattern of a whole stimulus, to generate a pitch that captures the relevant tone. In most studies, pitch contours are manipulated using the Pitch Synchronous Overlap and Add (PSOLA) technique (Charpentier and Stella, 1986; Boersma and Weenink, 2024) to modify the pitch patterns of naturally recorded stimuli (e.g., Chen et al., 2018; Chien et al., 2020; Moulines and Laroche, 1995). However, PSOLA offers essentially a blank slate – allowing the user to specify the F0 of the manipulated token at each pitch pulse. Given the fact that naturally recorded syllables will vary in duration, this leaves open the question of how systematically to set the F0 to create the same tone (e.g., Tone 1) across different tokens. This is particularly challenging in the case of bilingualism work where the goal may be to superimpose a tone (e.g., from Mandarin) on a word from a non-tonal language (e.g., English), where the synthesized token may be unnatural.
Thus, while PSOLA offers a good base, there is a need for systematic and replicable procedures that can yield appropriate tones that are tightly controlled, but naturally sounding to a tonal language listener, and replicable across a variety of stimulus types. Here, we introduce the steps of applying PSOLA in modifying pitches in a specific experimental context. We do this as a tutorial, which was developed as part of a larger study on bilingual Mandarin-English listeners. Thus, we start with a brief overview of that study and its goals before turning to the tutorial.
1.1 The context
Over the last two decades, most studies on cognitive science of bilingualism have consistently demonstrated the parallel activation of a bilingual’s two languages even when the task was only conducted in one language (e.g., Weber and Cutler, 2004; Costa et al., 2000). This discovery is established on empirical findings showing that even partial cross-language overlap either in phonology or orthography drives the activation of words from both languages (e.g., deksel is activated by a spoken word desk in Dutch-English listeners). This is characterized as cross-language lexical activation and competition. However, some studies have also shown that language-specific acoustic-phonetic cues constrain bilingual spoken word recognition. For example, Ju and Luce (2004) showed that VOT (Voice Onset Time) cues can heavily favor words from a single language such that cross-language lexical competition was only observed in Spanish-English bilinguals when the Spanish targets were altered to English-like VOT.
Our ongoing project that motivated the development of these techniques (Wang and McMurray, in progress), set out to investigate the role of lexical tone, as a crucial phonetic cue in tonal languages, in cross-language competition. Our study asked how Mandarin-English listeners interpret English prosody of English words, when it resembles Mandarin tones. Do Mandarin-English listeners use pitch patterns when processing English words where pitch patterns are not meaningful? Is tone required as part of shared phonology to drive cross-language competition? To answer these questions, we needed to manipulate suprasegmental information across Mandarin and English. For example, an English word, bay, can be manipulated at the supra-segmental level such that bay carries tonal information of Mandarin to sound like Mandarin bei4 (‘quilt’) or bei1 (‘cup’). This way, we can test how phonological information is processed cross-linguistically. Thus, our experimental manipulation of the auditory stimuli sought to modify the prosody of native English syllables (words) to match the forms of lexical tones in Mandarin.
The goal of this tutorial is to walk through the steps with considerably more details, as well as observations, that would not typically be offered in a methods section of a research paper. This way, we offer researchers to take advantage of this technique and build on it.
As part of our ongoing project, this study was approved by Macquarie University Ethics Committee (Project ID: 11189). All research participants, including speakers and raters, were recruited through flyers on campus or email communications.
2 Materials
To achieve the experimental goal, our approach sought to integrate acoustic features of Mandarin tones to English spoken words such that prosodic information was interpretable at the lexical level for Mandarin-English listeners as a Mandarin tone. In a sense we sought to superimpose a Mandarin tone on an English word.
In this section, we present the stimuli for the tone superimposition procedure. These comprised a list of 28 English monosyllabic spoken words on which we superimposed Mandarin tones. To maintain F0 information as similar as native tones, we extracted F0 data from Mandarin words that sound similar or the same to their English counterparts (e.g., bay vs. bei4).
2.1 Selecting spoken words
We selected 28 pairs of English and Mandarin words that are phonologically overlapped either in the whole syllable or the first two phonemes of the syllable (see Table 1). These pairs were rated by 5 naïve Mandarin-English listeners on a Likert scale of 1–5 as to how similar each pair sound cross-linguistically, 1 being the least similar and 5 being the most similar. Among the 50 pairs of English and Mandarin words presented, only those rated above 4 out of 5 were chosen as our target stimuli. These include 13 open syllables (e.g., bay), which broadly speaking are phono-tactically legal in Mandarin, and 15 closed syllables (e.g., ball), many of which are not legal in Mandarin, which only allows /n/ or /ŋ/ in syllable final position. These words were chosen to span a broad sample of the phonologies of both languages, with enough English-specific forms (e.g., closed syllables ending in /d/) to reinforce an English “mode” for the participants. The onsets of the stimuli vary in manner and place of articulation, which are [b, kʰ, d, f, dʒ, n, l, m, w, pʰ, ɹ, ʃ, tʰ, w, j]. In line with the sonority hierarchy, they could be grouped as the obstruents ([b, d, pʰ, kʰ, tʰ, f, ʃ, dʒ]), nasals ([m, n]), liquids ([l, ɹ]) and glides ([j, w]). For the syllable finals, the consonantal coda comprises nasals ([n, ŋ]) and liquids ([l, ɹ]). If described in phonetic terms of Mandarin, the onsets are [p, t, pʰ, kʰ, tɕ, f, ʂ, ʐ] (obstruents), [n, m] (nasals), [l] (liquids) and [j, w] (glides). The consonantal codas are [n, ŋ].
Our goal was to superimpose one of two Mandarin tones on each English word. Because we needed to manipulate/synthesize the English stimuli such that they carry prosody like that in a different language, namely, their counterparts (i.e., syllables) in Mandarin. Each word was matched with two Mandarin counterparts: one which had Tone 4, and the other which had Tone 1, 2, or 3. Namely, the Mandarin counterparts are the same or similar sounding syllables to English but have different tones for a given English syllable. As a result, all English words/syllables would receive a Tone 4. In addition, each word would also have a Tone 1, 2, or 3 for a separate condition (shown as in Table 1).
Although the selected English words and their Mandarin counterparts sound similar (or even the same), we summarize their differences here. First, 13 English words differ in syllabic structure from their Mandarin counterparts (the English words are closed syllables, and the Mandarin homophones are open syllables as listed in Table 2). Second, 18 English words share the same onsets with their Mandarin counterparts (e.g., labiodental fricative [f] in face and fei1 ‘fly’), for the other 10 this is not possible, and they share similar onsets (e.g., voiced bilabial stop [b] in ball and voiceless bilabial stop [p] in bao1 ‘bag’). Third, the vowel qualities of the English words and their Mandarin counterparts are phonetically similar (i.e., frontness/backness and height), but also bear subtle differences, for instance, [ɪ] in inn and [i] in yin1 ‘music’.
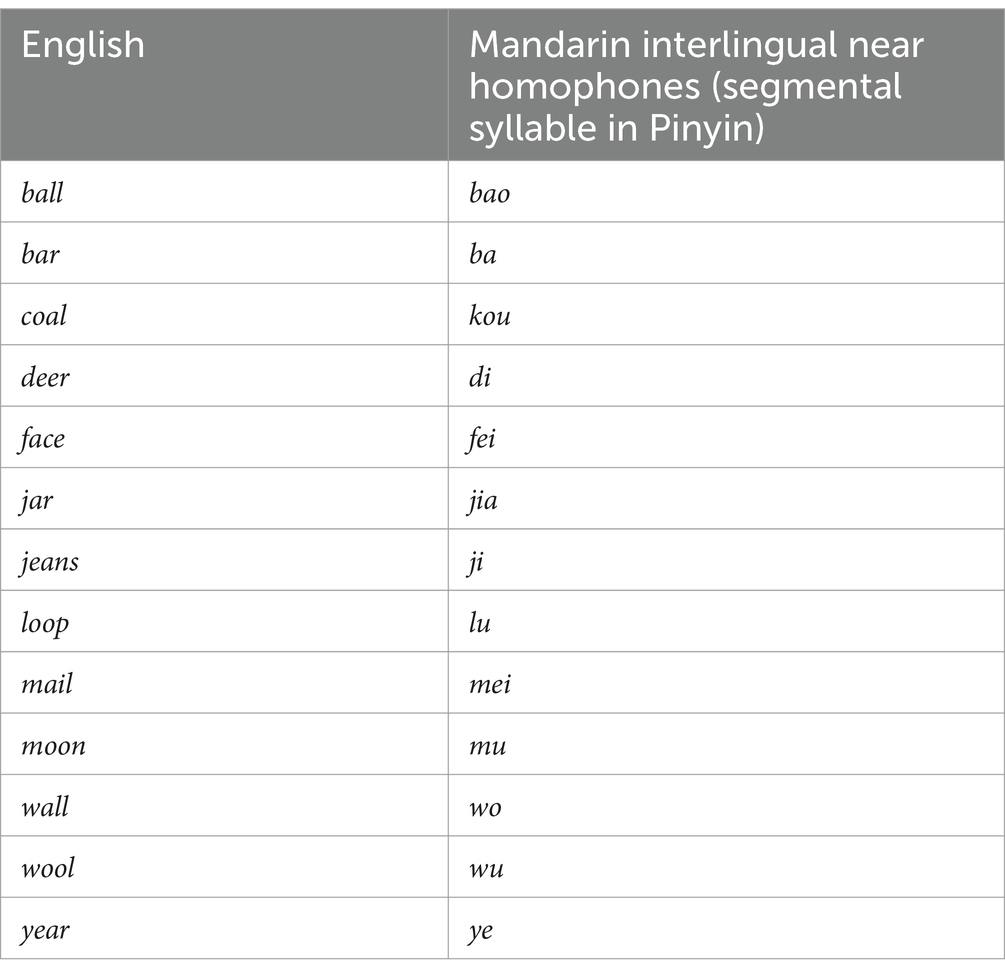
Table 2. Syllabic difference between English words (closed) and their Mandarin interlingual near homophones (open).
2.2 Stimulus development
We started by recording native speech tokens, including both English words and their counterparts in Mandarin. Then we preprocessed the stimuli by employing the following techniques and steps, commonly practiced in speech science.
2.2.1 Speech recording
A native male monolingual speaker of English from Melbourne, Australia, 26 years old, was presented with a randomized list of 28 English words and instructed to pronounce each word with 6 repetitions in a carrier sentence with a statement intonation, “He said X” (X refers to a given target word). The choice of using a carrier sentence is to obtain statement intonation at the end of the sentence, which roughly resembles Tone 4 in Mandarin Chinese. These natural tokens are part of the critical stimuli in our ongoing project. In addition, the choice of a male speaker of English is due to the observation that young female speakers appeared to more likely use creaky voice in speech than male speakers in the western culture (e.g., Loakes and Gregory, 2022). This recording session was conducted at the speech perception lab at Macquarie University (MQ) and lasted about an hour and a half.
As for the Mandarin counterparts, namely, 56 Mandarin words in random order, a male native speaker of Mandarin from Beijing, 28 years old, was instructed to pronounce each word in isolation (to protect the original F0 information in each syllable) three times. Note that we were only interested in the F0 contours of these Mandarin words in isolation, therefore, we took a different approach in recording such that the prosody at the sentence level does not confound the pitch contours of these Mandarin words. In addition, prior to selecting the talker to produce the target tokens, we found and compared a few native Mandarin speakers to avoid creaky voice, esp. in Mandarin Tone 3 and Tone 4 where F0 can be quite low at times. This session lasted about an hour and a half.
The two recordings of English and Mandarin were both conducted in a sound-proof booth at MQ, with an all-in-one computer (HP EliteOne 800 G6) and an external microphone (Rode NT1-A), placed approximately 12 cm from the informants/speakers. All tokens were recorded on mono channel, at the sampling rate of 44,100 Hz with 16-bit depth, by Audacity (version 3.4.2, Audacity Team Members, 2023), a great option for anyone seeking free and easily accessible software for audio recording and editing.
For those new to this step, we present a few practical but important tips here. First, it is important to attempt to record all the experimental tokens/stimuli in one session because the acoustic environment might differ depending on who, when, where and how the speech is recorded. Even in the same booth, small differences in microphone placement, background noise, or the speaker’s general mood could create detectable differences in the sound quality of the recorded words.
Second, prior to recording, it is important to ensure the recording (input) volume on the computer is high enough such that the waves should peak at 0.3 to 0.6, but never exceed 0.9.
Third, it is important to listen to sample recordings to ensure that there is no reverberation in the recordings as this is quite difficult to remove from the signal after the fact (one of the reasons it is crucial to record speech in a sound booth or at least a room with reverberation controlling panels).
Finally, for efficiency, we recorded the words in a batch of 10, saving all of them in a single wav file. This allowed the speaker to record 10 words in a row, without any interruption, as there was no need to pause and save a file every 30 s. Then we later split up the files for individual words.
2.2.2 Noise reduction
Even in a sound booth, there are often low levels of background noise (ventilation, computer fans). This can be eliminated from the recordings, using the noise reduction filter in Audacity.
Specifically, we followed the following steps to remove noise: (1) we selected a period of the waveform in which there was no speech to estimate the properties of the noise in the recording; (2) we navigated to the “Noise Removal and Repair” option under the “Effect” menu, followed by selecting “Noise Reduction”; (3) we then clicked on the “Get Noise Profile” button in the “Noise Reduction” dialog box to obtain the sample for noise reduction; this estimates spectral properties of the noise such that they can be eliminated later; (4) we then selected the whole recording, where we would like to reduce noise, opened the “Noise Reduction” dialog box again, and changed the preferences (we used the default settings), and clicked “OK” to remove the noise. This procedure works best on large single recordings, as that way the specific noise reduction parameters are identical across all the words – this is a second reason why recording in large blocks is important, and why it is important to do any noise reduction before segmenting the individual words. See Supplementary materials for a demo of these steps.1
2.2.3 Segmentation
Next, six tokens for each English word, 168 tokens in total, were extracted from the carrier sentences. Prior to cutting the tokens, we meticulously selected audio at the zero crossing points.2 This technique minimizes undesirable clicks by precisely aligning edits at points where the audio waveform crosses the zero-amplitude threshold. In addition, we selected 100 msec non-speech signals from the source recordings and added before and after each segmented token. Alternatively, one can choose to have a silence of 100 ms to add to the beginning or end of the target tokens using a script for batch processing.3
2.2.4 Rating and token selection
Once we obtained the individual speech tokens, we assigned four native speakers of English (two males and two females) to listen to each token, identify the word, and rate whether they were natural or not on a 1–5 Likert scale, 1 being unnatural and 5 being the most natural. Out of the 28 English words, only shoe was misidentified as chew by three listeners, resulting from the ambiguity of the onset. Given that the experimental paradigm in which we will be using the stimuli for was a closed set task (listeners would select from a small set of options), this ambiguity could be ignored (chew was not one of the options). Thus, a new recording was not actioned. Based on the ratings, four best tokens/variants out of six were selected for each word for experimental purpose. This rating session lasted about an hour for each rater.
2.2.5 Modification and editing
Every recording is likely to have minor artifacts that can be annoying for participants or simply detract from the overall naturalness of the experiments. We thus adopted two approaches and applied them to only those tokens that contain unusual elements that were irrelevant to the phonetic properties of the word. First, we used a series of filters, including high-pass filters to decrease electrical noise, and band stop filters to eliminate the puff sounds occurring in certain stop consonants (the latter was applied to sections of a speech file, not the whole file). Second, we removed mouth clicks generated by tongue movements that created a sudden release of air in the speech signal. They were cleaned up by using the spot healing brush tool in Adobe Audition (version CC 2021, Adobe Inc, 2021).
2.2.6 Intensity scaling
Even with careful control in the sound booth some tokens might sound softer or louder than others as the microphone shifts across the session, or the speaker’s vocal effort changes. Thus, the final step is to apply Intensity Scaling to the stimuli such that all the tokens in one experiment sound similarly in loudness.4
Intensity scaling is an extremely inexact process because the scaling between the actual intensity of the sound file and the percept of loudness is heavily shaped by the composition of syllables and their phonetic properties. Different speech sounds have dramatically different spectra of loudness contours. Thus, we first used automatic intensity normalization to all the tokens, setting a goal of 65 dB. We then adjusted each manually from 60 dB to 70 dB in Praat (version 6.4.23, Boersma and Weenink, 2024). The important criterion here is to ensure that all the words sounded at a similar level of loudness, and that each variant of the same word sounded the same level of loudness.
2.2.7 Summary
The techniques and steps described above prepare the raw records for manipulation of the tone. These serve the foundation to produce synthesized English tokens which preserve speech naturalness, as well as to minimize artifacts but accurately represent Mandarin tones with clarity and consistency. Again, we expect the superimposed speech tokens to closely resemble their Mandarin counterparts in pitch patterns without compromising the naturalness of English. The processed speech tokens in English for the 28 target words can be found at OSF.5
3 Procedure
In Mandarin, syllables are the tone-bearing units (TBUs), carrying lexical tones through their voiced components (Chao, 1968). Accordingly, when the onsets are voiced, lexical tones originate from the onsets and extend across the entire syllables; otherwise, lexical tones begin with the rimes.
Based on this understanding, we superimposed F0 contours extracted from the voiced segments in the Mandarin counterparts on the corresponding voiced segments of the English stimuli. For example, knee [ni], whose interlingual homophone/counterpart (ni2 [ni35] ‘mud’) also initiates with a voiced onset [n]. In this case, we superimposed the F0 values, collected from both onset and rime of ni2, on the corresponding [n] and [i] in knee. In contrast, for ball, whose counterpart bao1 ([paw55] ‘bag’) does not begin with a voiced onset, we superimposed the F0 values, extracted only from the rime [aw], on the corresponding rime of ball.
In this section, step by step, we will demonstrate how to manipulate F0 contours in Praat and superimpose Mandarin lexical tones on English words. Our approach is to extract pitch contours from Mandarin words and use the values from these pitch contours to superimpose on English words.
3.1 How to manipulate F0 in Praat?
Praat can manipulate F0 by using the PSOLA (Moulines and Laroche, 1995) technique as a built-in function. Here, we take Mandarin ba1 ‘scar’ as an example. We start with the overview of the typical procedure for altering pitch manually, before turning to our more systematic approach for tone superimposition.
First, we opened the sound file (e.g., ba1.wav) in Praat, and selected the sound object.
Second, we selected “To Manipulation…” under the “Manipulate -” label on the panel (see Figure 2).
Third, we chose the settings as required in the “Sound: To Manipulation” dialog box (see Figure 3). In the example, we used the standard settings, which include the pitch range of our recordings.
Then, we selected the manipulation object, “Manipulation ba1” in this case, and clicked “View & Edit” to open the editor window. As shown in Figure 4, the blue dots in the middle plot refer to the pitch points of the existing sound. For typical PSOLA use, these points can be manually dragged to manipulate the pitch at that point in time. There are also options for manipulating them as a batch. For example, instead of dragging individual pitch points, one can also shift several pitch points in a selection by a certain value, using “Shift pitch frequencies...” under the “Pitch” menu, or add pitch points at precise time with “Add pitch point at...” under the “Pitch” menu.
After manipulating, we can close the editor window and select the manipulation object again, followed by clicking “Get resynthesis (overlap-add)” to generate the manipulated sound.
Lastly, save the manipulated sound as a wav file to complete the process.6
3.2 How did we superimpose lexical tones onto English words?
This procedure is fine for single stimulus manipulations, but it is laborious for many files, and it is also highly unsystematic. We thus developed a more systematic approach for superimposing lexical tones onto English words by manipulating their F0 contours according to the pitch patterns in their Mandarin counterparts. That is, we altered the pitch values of the English words to match those extracted pitch values from the corresponding Mandarin words.
As described above, we obtained three pitch tracks from Mandarin for each English word. We then evaluated the quality of each pitch track and excluded the obviously bad ones (e.g., missing 3 or more than 3 F0 values out of 10). For those pitch tracks missing less than 3 F0 values, we fitted either a line or quadratic to fill in the missing values. Finally, given the quality of that fit, we selected the best pitch track to apply to each English token, as in word-by-word match between Mandarin and English. Worth to note, because the word durations of English-Mandarin pairs are close enough to transfer the pitch track from Mandarin to English, we chose not to manipulate the word durations to maximize the naturalness of English tokens.
If both English and Mandarin onsets were voiced (e.g., ni2 [ni35] ‘mud’), we applied F0 values from both onsets and rimes because voiced onsets carry additional F0 signals. If Mandarin onsets were voiceless (e.g., bi2 [pi35] ‘nose’), we only applied F0 values from the rimes to English words. The tone superimposition process can be described as the following steps, using two English tokens as examples: knee and bee, as well as their Mandarin counterparts, ni2 ‘mud’ and bi2 ‘nose’.
3.2.1 Step 1
We extracted F0 contours from the recorded Mandarin tokens, including all three repetitions for each word, using a Praat script (Arnhold, 2018). Critically, because words inherently vary in duration, rather than extracting the F0 contour at fixed temporal intervals (e.g., every 10 msec), we extracted them as 10 equidistant points. For words with voiced onsets, such as ni2 ‘mud’, or the other sonorants (see Table 3), these 10 points were extracted from both onsets and vocoids. For words with voiceless onsets, like bi2 ‘nose’ (see Table 4), the 10 points were extracted to only reflect the vocoid (the voiced portion) of the syllable.
3.2.2 Step 2
For each set of Mandarin exemplars (e.g., the three exemplars of bi2), we first excluded those where the F0 contours were missing 3 or more F0 values out of 10. Then we interpolated the missing data (F0 values) on those remaining pitch tracks and then selected the most representative F0 contours for tone superimposition. To interpolate missing F0, polynomial regression (degree = 2) was applied to Tone 3 contours. Tone 1, 2 and 4 contours were applied with linear regression. The choice of different regressions is based on the normalized tone contours in Mandarin (see Figure 1).
Additionally, R-squared value, widely employed for evaluating the accuracy of models, was used for determining the most representative F0 contour for tone superimposition. Thus, a value closer to 1 is a better fit for the data and we used this measure to pick the best fit model (pitch contour).
When handling words with voiceless onsets, the best fit F0 contours were chosen based on those extracted from the vocoids instead of the onsets. In the given examples (as in Tables 3, 4), Token (1) of ni2 and bi2, of the highest R-squared values among three, were therefore chosen as the source data utilized to manipulate the F0 values of English stimuli knee (voiced onset) and bee (voiceless onset).
3.2.3 Step 3
We superimposed lexical tones onto the English words with a script, using the built-in PSOLA in Praat.7 We first removed the original F0 contours of the English words and then added the given F0 values from their Mandarin counterparts following Step 1 and 2. Take knee [ni] for example, pitch points in both [n] and [i] were first removed. Then, based on the most representative F0 contour of the Mandarin counterpart ni2, 10 new pitch points with the values extracted from [n] and another 10 from [i] were equidistantly added to its English counterpart knee, so that knee would have a similar F0 contour as in ni2. Similarly, for bee, pitch points in the vocoids were adjusted to match the extracted values from Mandarin, by removing the existing points and adding the new ones.
4 Revisions
We asked two naïve Mandarin-English bilinguals to listen to the synthesized stimuli to judge whether they were natural or unnatural English tokens. We then asked them to identify the pitch patterns of each token. Both were very confident in tokens of Tone 4. This is predictable, as Tone 4 appears to be the most similar to English word prosody pronounced in statement intonation. However, they showed less confidence about the naturalness of tokens of Tone 1, 2 and 3. Due to this uncertainty, several revisions were made. This rating session lasted about an hour.
As Mandarin-English listeners, we inspected the synthesized tokens visually in Praat for abnormal pitch contours and manually adjusted to fix the F0 values of the “deficient” stimuli to improve their quality such that they sound more natural as English tokens. As follows, we present some examples we modified to improve the token quality. To ensure the validity of this procedure, we also asked naïve Mandarin-English listeners to judge these stimuli (see Section 5). At OSF, we present the 28 tokens resulted from tone superimposition and revision (see text footnote 5).
Some tokens superimposed with Mandarin Tone 3 (the dipping and rising tone) sounded unnatural and hard to identify. To our surprise, they were misperceived as Tone 2 (rising) words by naïve listeners. To address this problem, we manually lowered the overall F0 of the superimposed stimuli, especially the turning points of the contours. We also added a final short falling to each stimulus such that they sounded more like Mandarin Tone 3 (demonstrated as in Figure 5). Note that modifications only applied to the superimposed stimuli which were rated poorly.
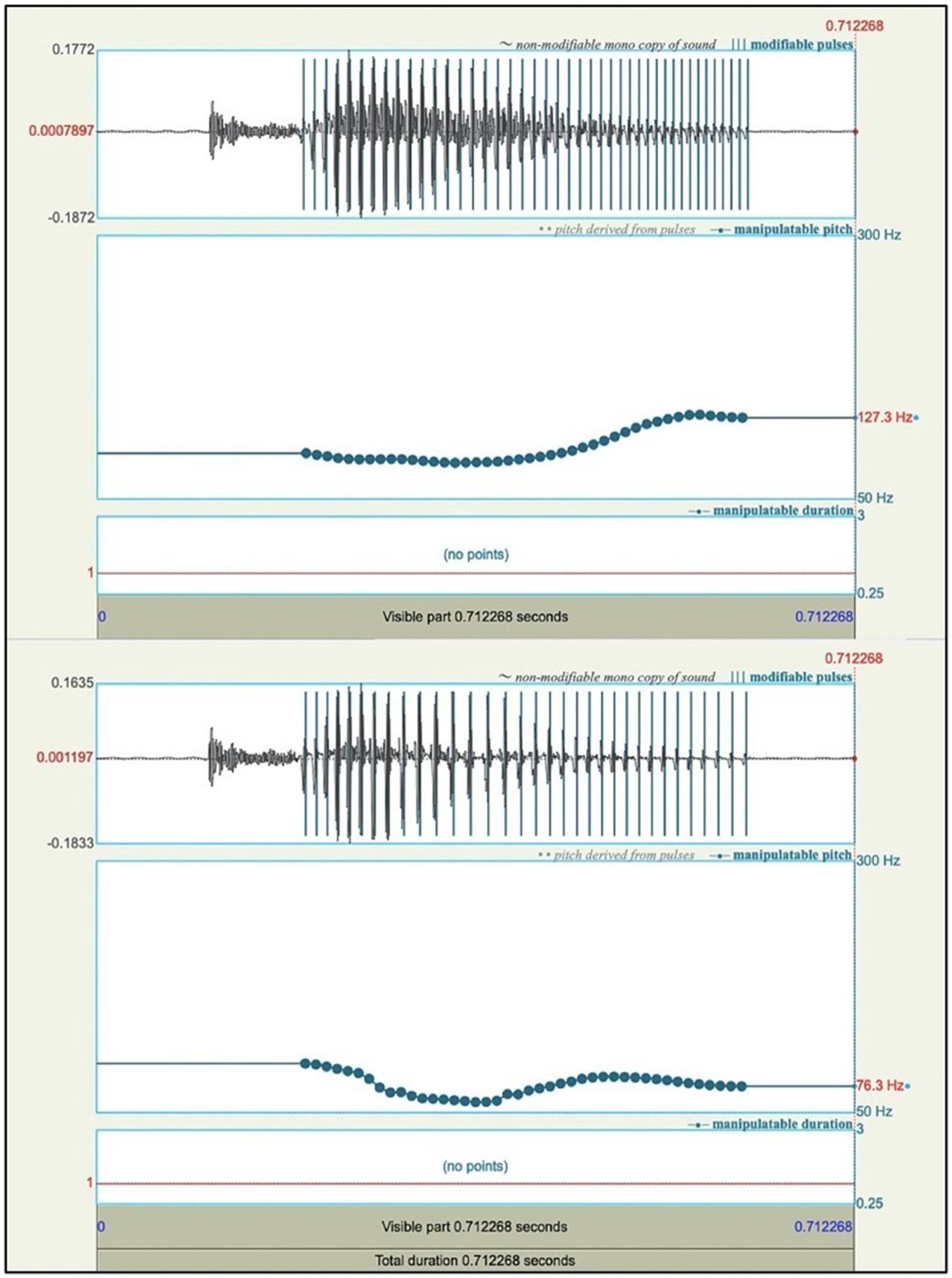
Figure 5. Example of coal superimposed with Mandarin Tone 3. The upper part of the figure showed the extracted contours to coal, based on values from kou3 ‘mouth’. The lower part presented the manually modified pitch contour.
Words with high vowels and syllable-final glides tended to be perceived less like typical Tone 4 words by native listeners, e.g., bi4. For these types of stimuli, we manually shifted the last few pitch points to lower values and the final pitch point was lowered to around 70 Hz (Figure 6). This value matched the lowest pitch of the English informant sampled from the recordings.
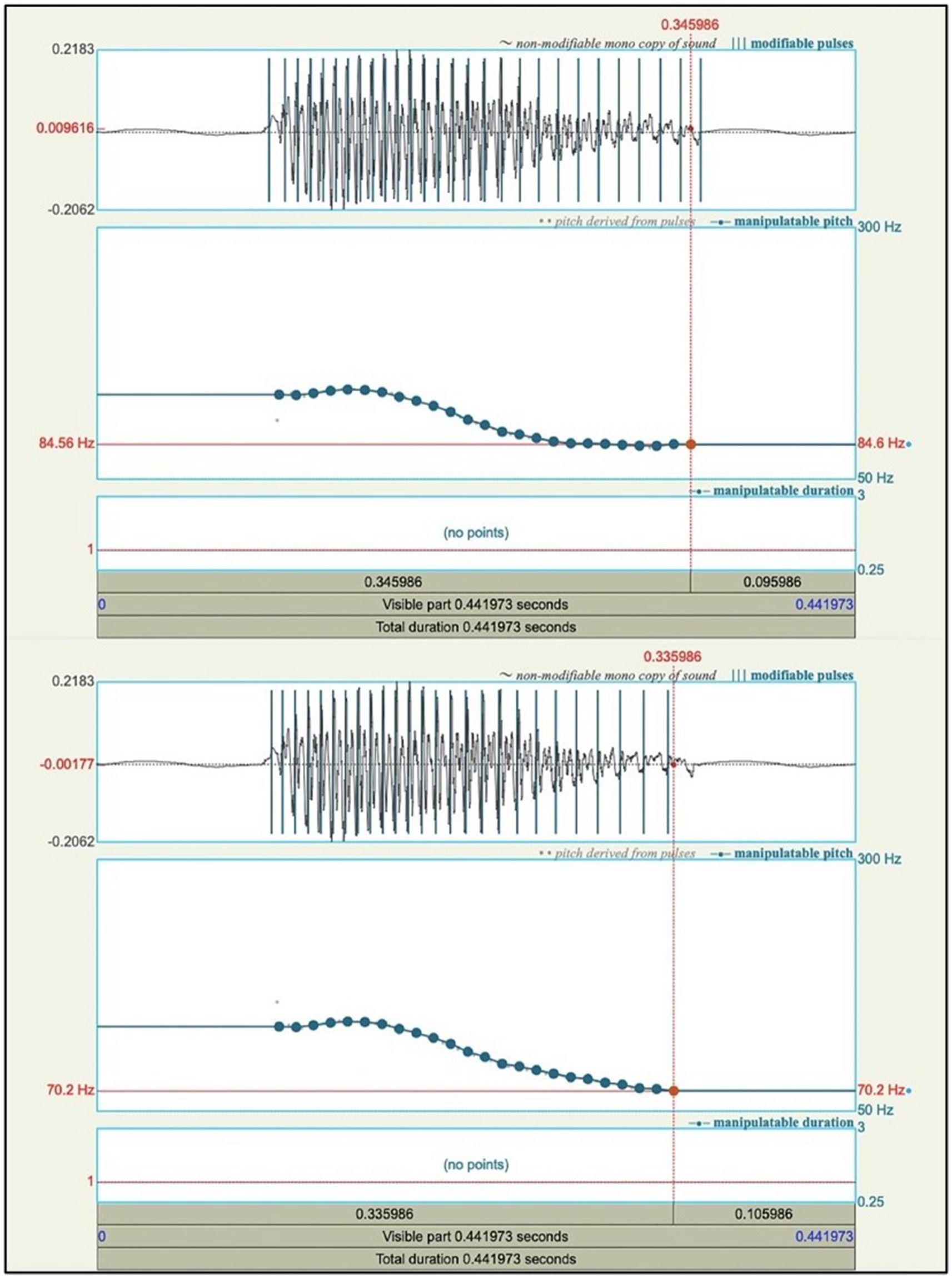
Figure 6. Example of bee superimposed with Mandarin Tone 4. The upper part of the figure showed a mild falling slope, where the last pitch points are about 85 Hz. The lower part of the figure showed a modified slope by lowering the last three pitch points.
We also observed some unpredicted perceptual pitch shifts accompanied by syllable-final approximants (i.e., liquids and glides), which may distort the pitch contours. These tokens were usually associated with Tone 2 superimposed. As Figure 7 shown, the final gliding of [u] occurred in some tokens of two and their offsets created lower F0 values at the end of the tokens. This led to a slight falling at the end of Tone 2 contours. In this case, the offsets were removed to solve the issue.
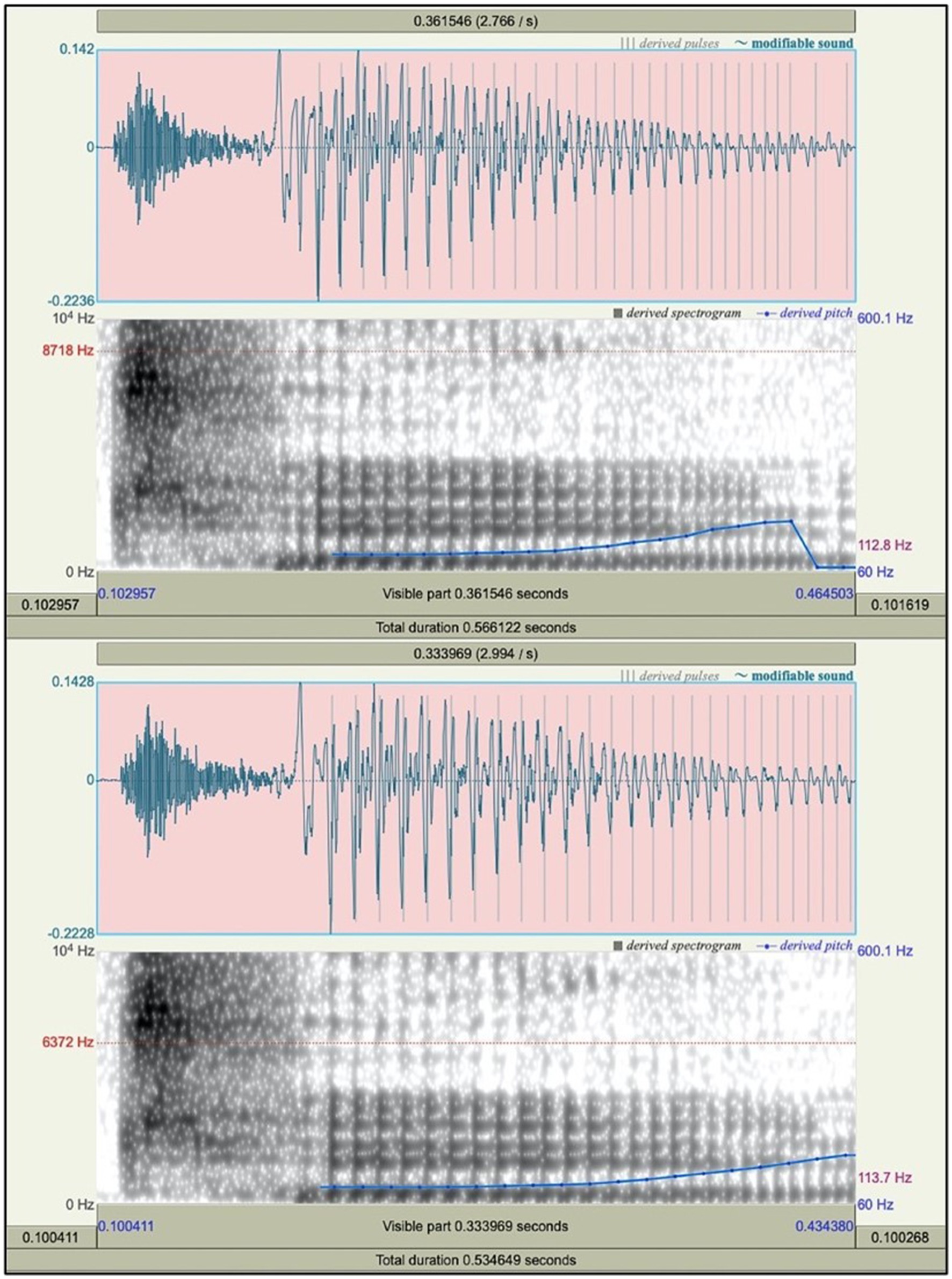
Figure 7. Example of two superimposed with Mandarin Tone 2. The upper part of the figure was the original superimposed token; the lower part showed the modified one, in which the offset of the syllable-final was removed.
The unequal syllable durations between English and Mandarin words can create some distortion of the source data. To illustrate, the F0 contours in weigh, superimposed with Tone 2, produced with a slight falling at the end of the pitch in our case, did not perfectly fulfill our anticipation because of the final falling of the pitch. See Figure 8, there was a slight F0 decline at the end of the superimposed weigh, resulting in an atypically sounding Tone 2. Therefore, we manually adjusted the F0 values of the token to make Tone 2 more typical.
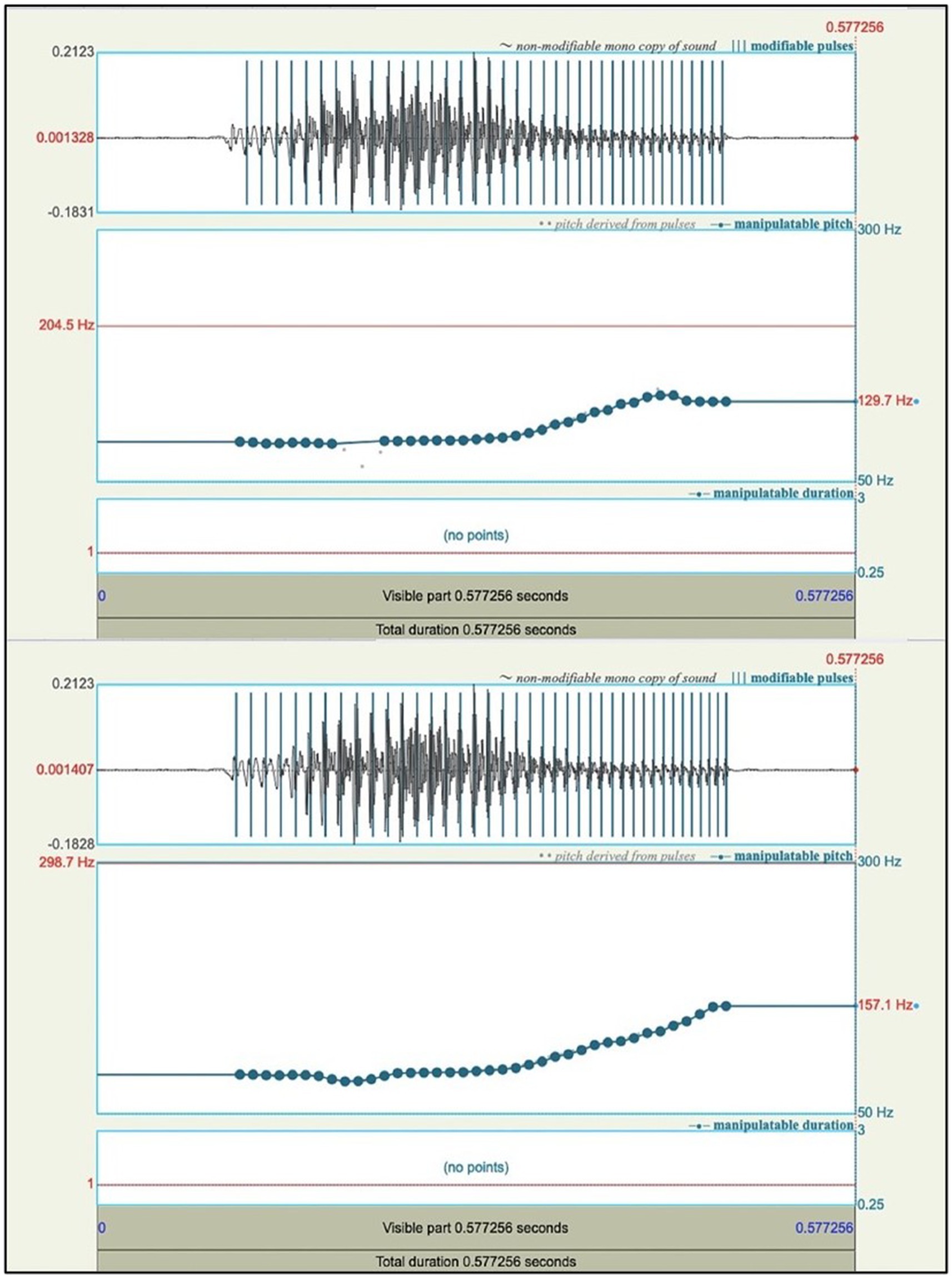
Figure 8. Example of weigh superimposed with Mandarin Tone 2. The upper part of the figure showed the originally superimposed weigh. In the lower part, the declining proportion was tuned as a rising one, and missing pitch points were added to the preceding blank.
5 Results and discussion
We asked three native English listeners to rate our synthesized tokens. They all reported the stimuli were of good quality but held some hesitation on words superimposed with Tone 3. Ultimately, our goal is to test these stimuli in Mandarin-English bilingual listeners to understand how the pitch patterns are interpreted on English words. Therefore, feedback from our target population was sought to evaluate the results and validate our method.
We conducted an online survey to understand how Mandarin-English listeners interpreted our synthesized tokens as either more Mandarin-like or English-like, because they were recorded by a native English speaker but superimposed with Mandarin tones. In random order, we presented each participant with 28 target tokens that were superimposed with Tone 1, 2 or 3 (words sounding similar across Mandarin and English, as listed in Table 1) one by one, as well as 14 filler tokens that were not synthesized but recorded at the same session with the same speaker. We recruited and asked 40 naïve Mandarin-English participants to listen to each token and rate on a 1–7 Likert scale, 7 being the most English-like and 1 being the most Mandarin-like. This rating took about 15 min. These bilingual listeners were native speakers of Mandarin, studying at MQ for a degree. They learned English as their second language at formal educational settings for specific purposes (e.g., degree). We recruited them for this rating because our upcoming experiment using these stimuli would recruit participants from the same pool.
Table 5 is the rating summary: average rating for each word.
Note that we only surveyed words superimposed with Tone 1, 2 and 3, because words superimposed with Tone 4 were of good quality and rated as natural consistently by a few bilingual listeners.
The filler items, namely, words without any synthesis, were rated very consistently as the most English-like among listeners. This result confirmed the quality of our stimuli, in line with our expectations.
The target words, which were synthesized at the supra-segmental level, carried prosodic cues from Mandarin Chinese, showed significant variabilities across items. The mean rating across words was 3.41 but with a large SD of 2.02. In fact, four words received a mean rating of 1.0, and two had a mean rating of 7.0.
Overall, the toned English words showed variabilities across the 28 items (i.e., 28 ×4 = 112 variants). Words superimposed with Tone 1 appeared to be more likely to be interpreted as Mandarin-like, while words superimposed with Tone 2 and 3 appeared to have equal preference to both language memberships. If we consider the average ratings between 3 and 5 (excluding 3 and 5) show the uncertainty of language membership (i.e., fun, jar, low, one, coal, moon), we are left with 22 items that bilinguals interpreted with strong preference as either Mandarin (n = 15) or English (n = 7).
So, what makes a given English token strongly Mandarin? We ran further analyses based on a few other factors, including the Mandarin-like ratings, the phonotactic legality of the word in Mandarin, word frequency in English and Mandarin. Separately, we ran T tests for each factor between groups when evaluating the ratings. Phototactically legal words received lower ratings (M = 2.84) than illegal words (M = 4.04) though this was not significant (p = 0.12). Tone 1 had a lower rating (M = 2.93) than the others (Tone 2: M = 3.88, Tone 3: M = 3.48), though none pairwise comparison was significant (Tone 1 vs. Tone 2: p = 0.31, Tone 1 vs. Tone 3: p = 0.58, Tone 2 vs. Tone 3: p = 0.72). Finally, rating was not correlated with the frequency of the English word (r = 0.14) or the frequency of the Mandarin (r = 0.04). Thus, Mandarin-English listeners’ perceptions of the naturalness of the stimuli may be a product of multiple factors.
6 Conclusion
Along the way demonstrated above, we have learned much through the development of this technique to create hybrid stimuli which combine the acoustic features of two different languages, namely, superimposing lexical tones onto English syllables. This technique is particularly useful to understand speech perception with bilingual populations. Here, we highlight a few key points in this procedure such that researchers who need to create well-controlled speech stimuli for experimental purposes can benefit. First, selecting a good speaker to record tokens of high voice quality is essential, prior to synthesis. For example, if Tone 3 is easy to elicit creaky voice, it is of the researchers’ interest to find a speaker to avoid this. Second, Token rating is heavily involved in this procedure to ensure the quality of speech before and after synthesis. Thus, patience is critical in conducting this type of work. Third, we presented our logic and method of superimposing lexical tones onto English syllables, namely, extracting pitch tracks from Chinese syllables to superimpose them onto their counterparts in English. There are other ways to achieve the same goal. We hope other researchers can also share their methods and/or build on our current technique.
In summary, our revisions and results show that auditory stimuli superimposed with lexical tones are of good quality to process and evaluate by bilingual listeners. This also validates our procedure and method of tone superimposition. However, these synthesized speech tokens of acoustic features from two different languages were perceived with substantial variabilities due to a few item-level and stimulus-level factors. Future research should explore each factor to elucidate their relative contribution in speech perception.
Data availability statement
The datasets presented in this study can be found in online repositories. The names of the repository/repositories and accession number(s) can be found in the article/Supplementary material.
Ethics statement
The studies involving humans were approved by Macquarie University Human Science Subcommittee. The studies were conducted in accordance with the local legislation and institutional requirements. The participants provided their written informed consent to participate in this study.
Author contributions
XW: Conceptualization, Formal analysis, Funding acquisition, Investigation, Project administration, Resources, Supervision, Validation, Writing – original draft, Writing – review & editing. J-YJ: Data curation, Formal analysis, Methodology, Validation, Visualization, Writing – review & editing. BM: Formal analysis, Methodology, Supervision, Writing – review & editing.
Funding
The author(s) declare that financial support was received for the research, authorship, and/or publication of this article. This project was funded by Australia Research Council Discovery Award to Xin Wang and Bob McMurray (DP 210102789).
Acknowledgments
We thank ZiXia Fan’s assistance in recording and editing the auditory stimuli.
Conflict of interest
The authors declare that the research was conducted in the absence of any commercial or financial relationships that could be construed as a potential conflict of interest.
Publisher’s note
All claims expressed in this article are solely those of the authors and do not necessarily represent those of their affiliated organizations, or those of the publisher, the editors and the reviewers. Any product that may be evaluated in this article, or claim that may be made by its manufacturer, is not guaranteed or endorsed by the publisher.
Supplementary material
The Supplementary material for this article can be found online at: https://www.frontiersin.org/articles/10.3389/feduc.2024.1439014/full#supplementary-material
Supplementary Video 1 | Noise Reduction Demonstration.
Supplementary Video 2 | Zero Crossing Demonstration.
Supplementary Video 3 | Intensity Scaling Demonstration.
Supplementary Video 4 | Pitch Manipulation Demonstration.
Footnotes
2. ^see a video demo at https://osf.io/qpcx8
3. ^a MATLAB script is provided as https://osf.io/nkmuw
4. ^see video demo for this step in https://osf.io/6rvwx
6. ^see a video demo of this procedure: https://osf.io/37fh5
References
Arnhold, Anja. (2018). MeasureIntensityDurationF0minF0maxF0contourpoints.Praat [Praat script]. Available at: https://sites.ualberta.ca/~arnhold/scripts/OnlyMeasurements/MeasureIntensityDurationF0minF0maxF0contourpoints.praat
Audacity Team Members. (2023). Audacity (version 3.4.2). Available at: https://www.audacityteam.org
Boersma, Paul, and Weenink, David. (2024). Praat: Doing phonetics by computer [Computer program]. Version 6.4.23. Available at: http://www.praat.org/ (Accessed October 27, 2024).
Chandrasekaran, B., Sampath, P. D., and Wong, P. C. (2010). Individual variability in cue-weighting and lexical tone learning. J. Acoust. Soc. Am. 128, 456–465. doi: 10.1121/1.3445785
Charpentier, F., and Stella, M. (1986). "Diphone synthesis using an overlap-add technique for speech waveforms concatenation." ICASSP '86. IEEE International Conference on Acoustics, Speech, and Signal Processing. Vol. 11. pp. 2015–2018.
Chen, F., and Peng, G. (2018). Lower-level acoustics underlie higher-level phonological categories in lexical tone perception. J. Acoust. Soc. Am. 144:EL158–EL164. doi: 10.1121/1.5052205
Chen, A., Peter, V., Wijnen, F., Schnack, H., and Burnham, D. (2018). Are lexical tones musical? Native language’s influence on neural response to pitch in different domains. Brain Lang. 180-182, 31–41. doi: 10.1016/j.bandl.2018.04.006
Chien, P., Friederici, A. D., Hartwigsen, G., and Sammler, D. (2020). Neural correlates of intonation and lexical tone in tonal and non-tonal language speakers. Hum. Brain Mapp. 41, 1842–1858. doi: 10.1002/hbm.24916
Costa, A., Caramazza, A., and Sebastian-Galles, N. (2000). The cognate facilitation effect: implications for models of lexical access. J. Exp. Psychol. Learn. Mem. Cogn. 26, 1283–1296. doi: 10.1037//0278-7393.26.5.1283
Dryer, M. S., and Haspelmath, M. (2013). WALS Online (v2020.3) [Data set]. Zenodo. doi: 10.5281/zenodo.7385533
Francis, A. L., Ciocca, V., and Chit Ng, B. K. (2003). On the (non)categorical perception of lexical tones. Percept. Psychophys. 65, 1029–1044. doi: 10.3758/BF03194832
Francis, A. L., Ciocca, V., Ma, L., and Fenn, K. (2008). Perceptual learning of Cantonese lexical tones by tone and non-tone language speakers. J. Phon. 36, 268–294. doi: 10.1016/j.wocn.2007.06.005
Gandour, J. (1983). Tone perception in far eastern languages. J. Phon. 11, 149–175. doi: 10.1016/S0095-4470(19)30813-7
Gussenhoven, C. (2004). The phonology of tone and intonation. Cambridge, UK: Cambridge University Press.
Howie, J. (1976). An acoustic study of mandarin tones and vowels. Cambridge, UK: Cambridge University Press.
Ju, M., and Luce, P. (2004). Falling on sensitive ears constraints on bilingual lexical activation. Psychol. Sci. 15, 314–318. doi: 10.1111/j.0956-7976.2004.00675.x
Liu, J., Hilton, C. B., Bergelson, E., and Mehr, S. A. (2023). Language experience predicts music processing in a half-million speakers of fifty-four languages. Curr. Biol. 33, 1916–1925.e4. doi: 10.1016/j.cub.2023.03.067
Liu, L., Lai, R., Singh, L., Kalashnikova, M., Wong, P. C. M., Kasisopa, B., et al. (2022). The tone atlas of perceptual discriminability and perceptual distance: four tone languages and five language groups. Brain Lang. 229:105106. doi: 10.1016/j.bandl.2022.105106
Liu, S., and Samuel, A. G. (2007). The role of mandarin lexical tones in lexical access under different contextual conditions. Lang. Cog. Proc. 22, 566–594. doi: 10.1080/01690960600989600
Loakes, D., and Gregory, A. (2022). Voice quality in Australian English. JASA Express Lett. 2:085201. doi: 10.1121/10.0012994
Maggu, A. R., Lau, J. C. Y., Waye, M. M. Y., and Wong, P. C. M. (2021). Combination of absolute pitch and tone language experience enhances lexical tone perception. Sci. Rep. 11:1485. doi: 10.1038/s41598-020-80260-x
Malins, J. G., and Joanisse, M. F. (2010). The roles of tonal and segmental information in mandarin spoken word recognition: an eyetracking study. J. Mem. Lang. 62, 407–420. doi: 10.1016/j.jml.2010.02.004
Mitterer, H., Chen, Y., and Zhou, X. (2011). Phonological abstraction in processing lexical-tone variation: evidence from a learning paradigm. Cogn. Sci. 35, 184–197. doi: 10.1111/j.1551-6709.2010.01140.x
Moulines, E., and Laroche, J. (1995). Non-parametric techniques for pitch-scale and time-scale modification of speech. Speech Comm. 16, 175–205. doi: 10.1016/0167-6393(94)00054-E
Peng, G., Zheng, H.-Y., Gong, T., Yang, R.-X., Kong, J.-P., and Wang, W. S.-Y. (2010). The influence of language experience on categorical perception of pitch contours. J. Phon. 38, 616–624. doi: 10.1016/j.wocn.2010.09.003
Shuai, L., and Malins, J. G. (2017). Encoding lexical tones in jTRACE: a simulation of monosyllabic spoken word recognition in mandarin Chinese. Behav. Res. Methods 49, 230–241. doi: 10.3758/s13428-015-0690-0
Wang, X. (2021). Beyond segments: towards a lexical model for tonal bilinguals. J. Sec. Lang. Stud. 4, 245–267. doi: 10.1075/jsls.21011.wan
Wang, X., Hui, B., and Chen, S. (2020). Language selective or non-selective in bilingual lexical access? It depends on lexical tones! PLoS One 15:e0230412. doi: 10.1371/journal.pone.0230412
Wang, X., Wang, J., and Malins, J. G. (2017). Do you hear ‘feather’ when listening to ‘rain’? Lexical tone activation during unconscious translation: evidence from mandarin-English bilinguals. Cognition 169, 15–24. doi: 10.1016/j.cognition.2017.07.013
Weber, A., and Cutler, A. (2004). Lexical competition in non-native spoken-word recognition. J. Mem. Lang. 50, 1–25. doi: 10.1016/S0749-596X(03)00105-0
Wiener, S., and Turnbull, R. (2016). Constraints of tones, vowels and consonants on lexical selection in mandarin Chinese. Lang. Speech 59, 59–82. doi: 10.1177/0023830915578000
Wu, J., Chen, Y., van Heuven, V. J., and Schiller, N. O. (2019). Dynamic effect of tonal similarity in bilingual auditory lexical processing. Lang. Cog. Neurosci. 34, 580–598. doi: 10.1080/23273798.2018.1550206
Wu, Z., and Ortega-Llebaria, M. (2017). Pitch shape modulates the time course of tone vs pitch-accent identification in mandarin Chinese. J. Acoust. Soc. Am. 141, 2263–2276. doi: 10.1121/1.4979052
Keywords: lexical tone, tone superimposition, interlingual (near) homophones, Mandarin Chinese, bilingualism, multilingualism
Citation: Wang X, Jheng J-Y and McMurray B (2024) Tone superimposition technique in Speech Sciences: a tutorial. Front. Educ. 9:1439014. doi: 10.3389/feduc.2024.1439014
Edited by:
Kaile Zhang, Hong Kong Polytechnic University, Hong Kong SAR, ChinaReviewed by:
Chenggang Wu, Shanghai International Studies University, ChinaXiaocong Chen, Hong Kong Polytechnic University, Hong Kong SAR, China
Xinran Ren, Sun Yat-sen University, China
Copyright © 2024 Wang, Jheng and McMurray. This is an open-access article distributed under the terms of the Creative Commons Attribution License (CC BY). The use, distribution or reproduction in other forums is permitted, provided the original author(s) and the copyright owner(s) are credited and that the original publication in this journal is cited, in accordance with accepted academic practice. No use, distribution or reproduction is permitted which does not comply with these terms.
*Correspondence: Xin Wang, eC53YW5nMUBtcS5lZHUuYXU=; eGlud2FuZzI2N0BnbWFpbC5jb20=