- 1Kazan State Institute of Culture, Kazan, Russia
- 2Foreign Languages Department, Peoples' Friendship University of Russia, Moscow, Russia
- 3Department of Development and Operation of Oil and Gas Fields, Almetyevsk State Technological University “Petroleum High School”, Almetyevsk, Russia
- 4Department of State and Legal Discipline, Plekhanov Russian University of Economics, Moscow, Russia
- 5Department of Biology and General Genetics, I.M. Sechenov First Moscow State Medical University, Moscow, Russia
- 6Institute of Linguistics and Intercultural Communication, I.M. Sechenov First Moscow State Medical University, Moscow, Russia
The latest technological advancements have greatly interested researchers in artificial intelligence (AI) in education. In parallel, researchers have expressed concern about using and applying AI in education. However, there is a shortage of research that comprehensively and holistically examines trends in the use of AI in higher education. Hence, this study aimed to comprehensively analyze and assess AI research trends in higher education. In the SCOPUS database, we conducted a bibliometric analysis of 1,563 articles on research on AI in education. Our results revealed that the use of AI in education has increased dramatically from 2004 to 2023. In particular, a dramatic increase and peak exist after 2019. We also found limited interaction among scholars studying AI. Furthermore, our findings indicate that most of the most influential institutions are located in developed countries. Moreover, our findings demonstrated that AI research primarily concentrated on comprehending the impact of AI-based instruction, with the majority of these studies taking place in engineering education between 2017 and 2020. We also noticed that research on medical education in higher education occurred between 2015 and 2017. In addition, before 2015, research used AI in medical education as a teaching method to implement problem-based learning in higher education.
Introduction
With the recent development of artificial intelligence (AI), its characteristics attract people’s attention, and many people have begun using artificial intelligence in their work and daily lives. In this parallel, it has been increasingly integrated into all levels of education to improve teaching and learning processes (Luan et al., 2020; Schiff, 2020; Zawacki-Richter et al., 2019). In this parallel, scholars have focused on examining the potential effects of the use of AI on instruction (e.g., Chen et al., 2020; Kuleto et al., 2021; Toapanta et al., 2022; Wang et al., 2023). Activities and applications based on AI algorithms have been designed and implemented to increase personalized and interactive learning experiences (Bozkurt et al., 2021; Rebolledo Font De La Vall and González Araya, 2023). The results of previous research have suggested that these activities and applications have contributed to increasing learning outcomes (Luan et al., 2020; Ng et al., 2023; Ouyang et al., 2023). The positive results of using AI in educational research have helped to understand the importance of AI in depth for student learning in higher education.
The contribution of AI to education also includes benefits for instructors to assess student performance and present personalized learning opportunities to students (Ng et al., 2023). Hence, using AI in higher education is essential for examining the contribution of new technologies to instruction. Furthermore, researchers using AI technologies in education utilize machine learning techniques to improve students’ and lecturers’ learning and teaching processes. Depending on the use of AI in education, researchers have conducted research studies to incorporate AI in higher and have examined the effects of AI on instruction in higher education. To this parallel, scholars have conducted some review studies to understand the situation of AI at all levels of education (Bond et al., 2024; Guo et al., 2024; Kim and Kwon, 2024; Nagaraj et al., 2023; Ouyang et al., 2022; Prahani et al., 2022; Shamkuwar et al., 2023).
However, to our knowledge, a few studies (López-Chila et al., 2024; Shamkuwar et al., 2023) analyzed the research trends in using and implementing AI in higher education. Although numerous studies have been conducted on using AI in higher education, only a few systematic studies have reflected the state and evolution of international research and identified research trends. From this perspective, more detailed studies are needed to clarify research trends, highlight the current state and evolution of research and potential gaps, and predict future directions to inform researchers and policymakers on the use of AI in the higher education context. In this way, future research can be conducted more effectively. A key benefit of identifying research trends is that it provides a comprehensive and systematic overview of the contributions of authors, institutions, and countries to the use of AI in higher education. This analysis’s results help promote international collaboration, improve the visibility of research findings, and focus on potential gaps that require further investigation. This is particularly important in the rapidly evolving AI field in the context of higher education, where timely findings can influence the direction of research (Murillo et al., 2021). In addition, there is a large body of research on the use of AI, and analyzing research trends through bibliometric analysis enriches the analysis process and makes complex data more accessible and interpretable for scientists to use in future studies. Thus, this research aims to fill this gap by providing an overall picture of the state and development of AI research in higher education by identifying trends based on publications from the SCOPUS database from 2004 to 2023. Hence, examining and evaluating current research trends using AI in higher education worldwide is necessary. There is a need to discuss the research trends in the use of AI in higher education. To address this research need, this study aims to conduct a comprehensive analysis to analyze and assess research trends in the use of AI in higher education. Thus, this study aims to provide a holistic analysis of the use of AI. In this study, we strive to offer a comprehensive and holistic overview of research on AI in higher education based on the published articles using a bibliometric analysis method. We believe that the results of this study will contribute to the knowledge base of researchers to prepare future generations for an AI-driven society.
We used bibliometric analysis in this research because it is a statistical method for analyzing different aspects of publications in a field of study. It includes various analyses to provide insights into research trends in a particular field and understand the thematic development within a specific field (e.g., Zhang et al., 2022). VOSviewer is a widely used software tool that enables researchers to create and display bibliometric maps illustrating the relationships between publications, authors, and keywords. Its use enables researchers to understand better the underlying structures in the literature (Van Eck and Waltman, 2010). VOSviewer’s various visualization techniques allow researchers to identify research clusters on a given topic and track the evolution of issues or questions over time (Waltman et al., 2010). The user-friendly interface and compatibility of VOSviewer make it a valuable tool for researchers conducting bibliometric analyses (Özdemir, 2023). In light of this information about bibliometric analysis, it can be concluded that it is a powerful method to assess the existing literature on the use of AI in higher education and to gain insights into research trends.
Related literature
In recent years, researchers have studied the use of AI in education. Upon this line of research, scholars have conducted review studies to examine the status of using AI in higher education research. However, the existing literature reviews have mainly focused on systematic reviews of AI in the higher education context (Bond et al., 2024; Guo et al., 2024; Kim and Kwon, 2024; Nagaraj et al., 2023; Ouyang et al., 2022; Prahani et al., 2022; Shamkuwar et al., 2023). We detected that only two research focused on analyzing publications on AI in higher education using bibliometric analysis. For example, a study by López-Chila et al. (2024) examined the status of AI in the context of higher education. They searched the SCOPUS database for publications from 2017 to 2023. Their research demonstrated a consistent increase in AI studies in higher education. They found that China and the United States were leaders regarding production and citations. Similarly, Shamkuwar et al. (2023) analyzed the research patterns in bibliometric analysis in applying AI in higher education published during the last two decades. They examined 314 publications indexed in the Scopus database, and their findings demonstrated an increase in the yearly publishing rate of AI articles and their geographical distribution. Their findings suggested that computer science and engineering have a strong influence on interdisciplinary research in the field of AI. In addition, they found that 78% of articles published in the last 5 years, compared to only 22% in the first 15 years. China and the United States were the first ranks in AI.
Other studies in the literature on AI and higher education have been conducted to examine the use of AI in general educational research. For example, the study of Guo et al. (2024) performed a bibliometric analysis on 6,843 publications published in general educational research. They did not focus on higher education. Their findings indicated that China, the United States, India, Spain, and Germany were at the forefront of the research effort, and AI research in education prioritizes higher education over K–12 education. Their research revealed 15 themes for research topics, including instructional robotics and big data analytics. They found that AI was used in various educational research. Research by Prahani et al. (2022) analyzed the trends in 457 publications on AI in general educational research in the last decade using a bibliometric analysis. Their findings indicated that the advancement of AI education research has grown rapidly in the past 5 years. Articles and journal sources were the most prevalent documents, and China had the highest distribution rate. The study revealed that AI research over the past decade focused on student-oriented applications, engineering education, pedagogical approaches, e-learning-focused education, educational frameworks, and AI integration in curriculums.
Among systematics review studies focused on AI in higher education, Ouyang et al. (2022) conducted a systematic review of AI in higher education and reviewed publications from 2011 to 2020. Their research revealed that AI applications in online higher education serve several functions, including predicting learning status, performance, and satisfaction, recommending resources, automatically assessing students, and enhancing the learning experience. They found that the impact of AI applications in this context includes the provision of high-quality predictions. In another research, Flores-Velásquez et al. (2024) examined the studies examined the acceptability of AI technologies in higher education. However, their research focused on students’ acceptance of AI technologies. They reviewed 56 publications from Scopus, and their results revealed an increasing pattern in scientific output from 2021. The researchers determined that the research studies on AI in higher education demonstrate an ongoing interest in studying the acceptance of AI technologies. Research by Bond et al. (2024) conducted a meta-study and systematic review to investigate studies on artificial intelligence in higher education. They assessed articles between 2018 and July 2023. The study’s outcomes revealed that most reviews examined AI broadly (47.0%) or focused on profiling and prediction (28.8%). However, the main results indicated that adaptive systems and personalization were the most commonly employed approaches in higher education. In addition, Nagaraj et al. (2023) reviewed AI research in higher education programs focused on science, technology, engineering, and mathematics (STEM). The researchers analyzed the influence of AI. They emphasized the possible advantages and difficulties of incorporating AI into STEM education for future investigation and advancement. Considering the above information, the lack of research on AI and higher education using a bibliometric methodology shows a significant gap in the existing literature to investigate the implementation of AI in higher education and to understand research trends related to the use of AI in higher education. Table 1 presents a review of studies conducted on AI in higher education.
Only two studies (López-Chila et al., 2024; Shamkuwar et al., 2023) analyzed the research trends in using and implementing AI in higher education. Based on the weaknesses of previous studies, therefore, there is a need to address this research gap to explore and understand the research trends on AI in higher education by conducting a thorough bibliometric analysis. However, limited studies (López-Chila et al., 2024) have specifically focused on examining research trends in AI in higher education using bibliometric analytic techniques. To address this void, our study seeks to analyze and explore research trends in AI research in higher education. The results offer significant insights into the utilization of AI in educational research within the higher education setting and reveal research trends for learning and teaching in AI.
Method
Data collection
We selected the SCOPUS databases for data collection. We searched for AI and higher education using the keywords in Table 2 in the SCOPUS database. Because AI covers a wide range of applications, we included many alternative keywords for AI in the literature. Table 2 shows a list of keywords we used during the database search.
The search yielded 1,563 articles after we used the filter options based on inclusion and exclusion criteria in Table 3. The analysis of relevant articles in the database was conducted in May 2024. The publications published in 2024 were not considered in the database search analysis. We searched the publications between 2004 and 2023. We used a bibliometric analysis approach that researchers commonly use to examine research trends and hotspots and comprehensively understand publications on a particular topic or research area. We defined inclusion and exclusion criteria for determining the publications in this study. To this end, we conducted an advanced database search and used Boolean operators to apply and utilize filtering options. The initial analysis revealed 13.373 documents in the database; we used the filtering options to limit the results to social sciences. Thus, the results decreased to 3,251. Later, we selected articles, and the results decreased to 1,621. Later, we chose journal articles and found 1,613 results. Finally, we limited the articles published to English and found 1,563 results. Among 1,563 articles, 27 were in the press, and the rest (n = 1,536) were published. In addition, we used bibliometric indicators to analyze the scientific production, such as the distribution of articles by year of publication and the main sources used.
The bibliometric analysis approach allowed us to provide relevant information about the research trends and their development in the field of AI in the higher education context. VOSviewer is a powerful software tool commonly used for bibliometric analysis (Van Eck and Waltman, 2010). This software facilitates the construction and visualization of bibliometric networks and enables researchers to analyze the relationships between scholarly elements such as publications, authors, and keywords (Van Eck and Waltman, 2010; Zhang et al., 2022). It is important because it transforms complex bibliometric data into understandable visual representations and enables an understanding of research trends in a particular research field or topic. The main reason for using VOSviewer in this research is its user-friendly interface that simplifies the process of data visualization (Özdemir, 2023). In addition, the software makes it possible to uncover complex patterns and relationships in the dataset (Van Eck and Waltman, 2010). This makes it easier for researchers to gain insights into research trends and their development. In addition, VOSviewer is particularly effective in exploring research trends by identifying clusters of research activities and key authors. The findings from previous studies support this idea (e.g., Pradana et al., 2023; Zhang et al., 2022).
Data analysis
We used the Vosviewer software (Van Eck and Waltman, 2010) for this research. The software employs a bibliometric analysis methodology and was created to visualize bibliographic data. The software primarily aims to investigate prominent research areas and cutting-edge advancements in a specific field or research topic. It achieves this by analyzing high-frequency keywords and examining the co-occurrence of keywords in the literature. Consequently, the software can evaluate and predict the research hotspots, historical development, emerging trends, and research clusters in a given field. We uploaded a CSV file containing 1,563 specifically chosen articles into the VOSviewer application. Data visualizations mostly employ nodes and lines as principal representations. The size of the nodes corresponds to the frequency of the relevant data references or instances. This study primarily focused on assessing the fundamental information regarding the status of AI research in higher education to examine its current state and future trends. In addition, we analyzed the co-occurrence mapping of keywords to get insights into the primary domains, fundamental subject matter, and current areas of focus in the field of AI within the context of higher education, namely from 2004 to 2023. This analysis also aimed to identify the prominent research topics in using AI in higher education.
Results
Trends in the number of articles and citations
Analyzing the year of publication allows researchers to track the development of a particular area over time. This analysis can reveal patterns such as the growth or decline of research interest, the emergence of new topics, and shifts in methodological approaches. The number of publications and citations show a growing interest among researchers in AI and its use in education. As shown in Figure 1, the number of publications in AI research grew dynamically during the last 20 years of research. The results regarding the number of articles on AI showed that AI research for educational purposes maintained a high growth level from 2004 to 2023 regarding the number of articles. However, the results also showed a flat number of articles from 2004 to 2012. Additionally, between 2013 and 2018, researchers’ interest in AI in education grew. Since 2019, interest in AI research has grown dramatically, with a significant increase in publications. In 2021, there has been a decrease in the number of articles. However, the number of articles published in 2021 is close to those of 2020 and 2022. The results demonstrate a dramatic increase in articles from 2022 to 2023. The number of articles (n = 279) published in 2023 showed a 50% increase compared to 2022 (n = 183). In general, the results regarding the number of articles during the last two decades showed a tendency in AI education research that there are growth peaks. This tendency shows that AI education research has received more and more attention since 2019.
Figure 2 shows the number of citations according to years. As shown in Figure 2, the number of citations in AI research grew dynamically during the last 20 years of study. A dramatic increase in citations shows the researchers’ interest in AI. Between 2004 and 2023, the 1,563 articles received a total of 29,692 citations in total. The results regarding the number of citations during the last two decades showed a growing tendency and significant increase in AI education research. This tendency and increase shows that AI education research has received more and more attention during the last 20 years.
Prominent authors
Table 4 lists the top 10 authors according to the number of articles and citations. The top authors are Dragan Gašević, Kyle M.L. Jones, Yi-Shan Tsai, Shane Dawson, Dirk Ifenthaler, Abelardo Pardo, Hendrik Drachsler, Martin R. Fischer, Fan Ouyang, and Bart Rienties, respectively. These authors are more prolific authors who have published on AI education research. According to the number of citations. Accordingly, Sebastian Ventura, Chrisbal Romero, Dragan Gašević, Dirk Ifenthaler, Jurgen Rudolph, Shannon Tan, Paul Prinsloo, Samson Tan, Sharon Slade, and Pu-Shih Daniel Chen are the authors with the most citations. This means the authors with the most citations have contributed to developing AI research through their articles.
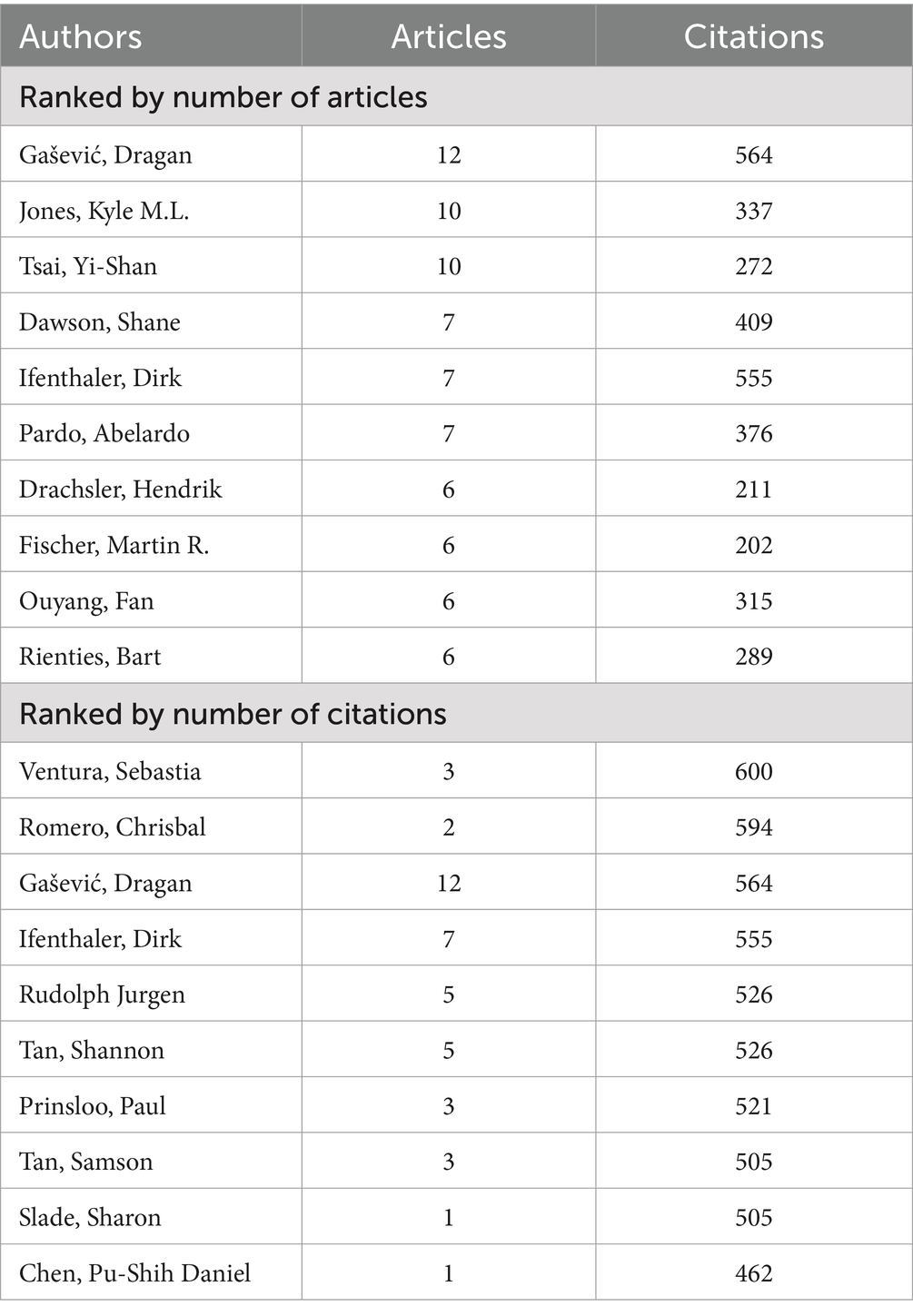
Table 4. A list of the 10 most important authors according to the number of articles and the number of citations.
Figure 3 shows the collaborative networks between the authors who published more than two articles. The results show 32 collaborations between authors with two or more articles (n = 32). The nodes in Figure 3 show the magnitude of collaboration among the authors, and links show close cooperation among the authors when there was a collaboration between the authors. The results show that some lead authors (e.g., Gasevic, Dragan) collaborate closely with other researchers. In addition, the results showed weak collaboration between researchers and different research groups. This result suggests that interactions for research in the field of AI are limited.
Prominent institutions
Table 5 analyzes the top 10 institutions contributing to developing an AI knowledge base. Monash University was the institution with the highest number of articles. The Institute of Tecnológico de Monterrey had 20 articles. The following institutions are Universiteit Maastricht, University of Michigan, Ann Arbor, Universitat Oberta de Catalunya, The University of Sydney, The University of Edinburgh, The University of British Columbia, King Abdulaziz University, and Indiana University-Purdue University Indianapolis. The results demonstrated that most of the institutions, according to the number of articles, were from Australia and the United States. The other institutions were from Mexico, the Netherlands, Spain, Scotland, Canada, and Saudi Arabia.
Prominent countries
The analysis of the authors’ country provides a perspective on understanding the global research background, allows us to identify the leading countries, and shows how research productivity is distributed across different countries and regions. This analysis can show the cooperation networks between countries and the influence of policy on research performance. Table 6 shows the leading countries that have contributed to AI research. We found authors from 101 countries published on AI. The results revealed 41 countries that published more than 10 articles. Our results showed that the United States, UK, China, Australia, and Spain are ranked in the top five in the number of published articles. Canada, Germany, the Netherlands, Mexico, and Saudi Arabia are the following countries. These results show that more than half of the articles on AI published by researchers are located in developed countries. The other countries, including China, Mexico, and Saudi Arabia, were developing categories.
Results regarding the number of citations show that the United States, UK, Australia, Spain, and Germany are ranked in the top five. China, Netherlands, Canada, Malaysia, and South Africa are the following countries. These results show that researchers in developed countries received the most citations. The other countries, including China, Malaysia, and South Africa, were developing categories.
Later, we searched for research collaborations among the countries that have published more than 10 articles (see Figure 4). The findings indicate that the United States was the primary center for AI research in education and maintained strong connections with numerous countries, especially Canada, Israel, South Korea, the United Arab Emirates, and Turkey. In addition, the United Kingdom was closely connected with Australia, Germany, the Netherlands, Switzerland, and South Africa. The other countries have a few links with others and demonstrated weak connections with other countries.
Most influential studies
We used co-citation analysis to identify the most influential studies on AI research in higher education. This analysis helps to uncover co-cited documents on a particular topic or area. The co-citation analysis is an important contribution to knowledge building for researchers. Table 7 shows the 10 most influential studies cited in the articles on AI in higher education. The results regarding most influential studies demonstrate that nearly all of these studies are not from publications published during the last 5 years. Also, two books were published by Cohen (1988) and Lave and Wenger (1991). The other 8 publications are peer-reviewed articles. These articles were published between 2013 and 2018.
Hot research topics and trends in AI research
Exploring hot topics helps to uncover the core knowledge nodes in AI education research. VOSviewer can analyze the keywords used in the study according to a specific timeline. Figure 5 shows the results from a keyword analysis. In this analysis, we included the keywords used more than 30 times. Thus, our results yielded 102 keywords. The program categorized all these keywords into 4 clusters. In Figure 5, nodes represent the number of keywords, and the colors in Figure 5 represent the different clusters. The cluster analysis focused on determining keywords with the most strengths and links on the dataset. The results show that the time indicator of years at the bottom right of Figure 5 shows the time interval in which the hot topics keywords in the studies were used.
The results in Figure 5 show that after 2020 (between 2020 and 2023), research (see nodes in yellow, cluster 1) has focused on higher education, learning analytics, machine learning, academic performance, forecasting, online learning, COVID-19, and college students. This result means that AI research has focused on examining the effects of learning analytics, machine learning, academic performance, forecasting, online learning, and COVID-19 on academic performance after 2020. In addition, light green nodes in Figure 5 show the hot topics in AI research between 2017 and 2020. Accordingly, research between 2017 and 2020 has focused on keywords (see nodes in light green, cluster 1) related to students, curricula, engineering education, deep learning, computer-aided instruction, education computing, e-learning, learning systems, active learning, learning algorithms, motivation, knowledge, decision-making, algorithms, surveys, and questionnaires. All of these keywords are placed in cluster 1. This result means that AI research has focused on understanding the effects of instruction based on AI. In addition, the AI research between 2017 and 2020 has focused mostly on engineering education. In summary, the studies in cluster 1 have focused on understanding the effects of instruction based on AI in general.
Between 2015 and 2017, hot topics in Figure 5 (see nodes in darker blue or turquoise, cluster 2) focused on feedback systems, feedback, humans/humans, medical education, medical students, curriculum, educational measurement, questionnaires, and clinical competence. Based on this result, we can conclude that AI research mainly was conducted on medical education in higher education between 2015 and 2017. In addition, cluster 2 included problem-based learning, program evaluation, psychological aspect, clinical education, clinical clerkship, medical school, standard/standards, and attitude of health personnel. These keywords refer to hot topics of research conducted before 2015 (see nodes in purple, cluster 2). This result suggests that AI was used in medical education as a teaching method to implement problem-based learning in higher education before 2015.
Cluster 3 included male, female, adult, clinical competence, controlled study, and skill. Figure 5 shows that these keywords were used in research conducted between 2015 and 2017. Thus, these results prove that the research on AI focused on medical education in higher education. Cluster 4 included only the keyword “assessment.” We found that this keyword was used in research between 2017 and 2020. Thus, we can conclude that research also focused on assessing the outcomes of AI-based instruction.
Table 8 shows the most frequently used keywords for hot research topics by year. It helps to show an evolutionary model of AI research in light of the most frequently used keywords in college research. These most frequently used keywords show the characteristics of research to understand the development time of research by years. Based on the results we obtained using the VOSviewer program, we divided the research period into four time periods (see Table 8).
Discussion
This study aimed to comprehensively analyze research trends in the use of AI in higher education. Thus, this study provides a holistic analysis of AI using a bibliometric analysis method based on published articles. Our results showed that the use of AI in education has increased dramatically. This research revealed that the number of publications in AI education research grew dynamically during the last 20 years of research. Since 2019, interest in AI research has grown dramatically, with a significant increase in publications. In addition, the results demonstrate a dramatic rise in articles from 2022 to 2023. Our results revealed a peak of research on the use of AI in higher education after 2019. These results confirm the findings of previous studies indicating that AI has been one of the hot topics in educational research and that AI research has shown an increase in higher education (Akhmadieva et al., 2023; Bond et al., 2024; López-Chila et al., 2024; Prahani et al., 2022; Shamkuwar et al., 2023). One reason is that AI is a rapidly developing field that can improve the quality of learning and teaching at all levels (Popenici and Kerr, 2017). Several factors can explain this result. The first reason is that companies and universities have been working on important AI projects, and significant investments in the development of AI have been implemented (Zawacki-Richter et al., 2019). These substantial university research funds are being channeled into the development of AI because of its potential to transform various dimensions of education and the need to integrate AI technologies into higher education to improve educational outcomes and overall institutional efficiency. One of the primary motivations for higher education institutions to invest in AI is the potential for enhanced teaching and learning experiences. For instance, AI applications can personalize education by analyzing large amounts of student data to tailor learning experiences to individual needs to improve engagement and knowledge retention (Zawacki-Richter et al., 2019; Abbas et al., 2023). This enables universities to cater to students with different learning styles and teaching approaches (Thomas, 2024). In addition, the rapid development of AI technologies has led to significant investment from both the private and public areas, with large companies contributing significantly to AI research (Zawacki-Richter et al., 2019). In light of these efforts, universities have adopted AI research and development studies as an integral part of their strategic missions (Sungkono and Widana, 2024).
These technological advances and new technologies, including AI in higher education, have driven research into how artificial intelligence can enhance teaching and learning processes (Popenici and Kerr, 2017; Zawacki-Richter et al., 2019). Thus, the use of AI in higher education has become an increasingly important topic in higher education (Wang and Zheng, 2020). The second reason could be that research has shown that AI can be used in various ways to revolutionize learning and teaching in higher education. These include adaptive learning, personalized education, machine learning algorithms for online learning, human-AI interaction, and the pedagogical use of AI-generated data (Bozkurt et al., 2021). In addition, the positive learning outcomes regarding the implementation of AI may have engaged researchers to improve students’ learning outcomes in new research studies (Guo et al., 2023; Urban et al., 2024). The third reason may be that the impact of AI on higher education is also evident in research focused on predicting students’ needs, analyzing learning outcomes, and evaluating teaching processes in online higher education environments (Akinwalere and Ivanov, 2022). Findings from research on AI show that this period includes evidence regarding the use of AI in non-instructional applications. For example, the study of Akiba and Fraboni (2023) investigated how AI-supported tools can provide students with academic advice using a free version of ChatGPT. They found that ChatGPT generated surprisingly high-quality responses in an authoritative yet supportive tone and was particularly good at answering general and open-ended career-related questions, such as career prospects, in a clear, comprehensive, and supportive manner using simple language. The research of Ruwe and Mayweg-Paus (2023) demonstrated that participants attributed more significant trustworthiness to AI over educators and peers, particularly in terms of expertise, as feedback was provided.
Furthermore, the transformative potential of AI for teaching, learning, and research in higher education is recognized. The fourth reason is that since 2019, AI technology has made significant advances, including in the sophistication of algorithms and the increasing availability of data. In particular, the most notable leaps in AI technology in deep learning and natural language processing have led to significant advances in the use of AI. Introducing models such as OpenAI’s GPT-3 in 2022 is an example of these advances and has shown that they can generate human-like text and understand context. The rapid increase in patent filings related to AI technologies across various industries supports a robust trend toward innovation and application of AI solutions in all areas of life (Park and Kwon, 2023). These developments have led to transformative applications in the field of education.
In addition to the number of articles, we found that the results regarding the number of citations over the last two decades showed a growing trend and a significant increase from 2004 to 2023. This trend and increase show that AI education research has received more and more attention from researchers in the last 20 years. This result could be because the inclusion of AI tools in education has sparked researchers’ interest in exploring the impact of AI (Popenici and Kerr, 2017; Tapalova and Zhiyenbayeva, 2022). In addition, the potential of AI has attracted much attention (Tapalova and Zhiyenbayeva, 2022). Researchers have emphasized that AI applications have benefits on learning outcomes and improving the educational environment (Aldosari, 2020). In addition, there is a need to adapt AI-driven e-learning systems after COVID-19 (Aldosari, 2020; Khan et al., 2022).
On the other hand, the results also revealed that the number of publications on AI in higher education research was flat from 2004 to 2012. The number of articles during this period suggests that the early integration of AI into higher education and the limited awareness and understanding of AI technologies among faculty may have caused this result. It is also essential to indicate that, during this period, AI was still in its infancy to be used within educational technology. The literature suggests that technology integration in higher education primarily focuses on e-learning and digital resources rather than AI (Cotton and Gresty, 2005). Furthermore, AI was not recognized as a critical component of educational strategies during this period. These factors may have contributed to the slow progress of research on AI in higher education during this period.
In addition, our analyses revealed weak collaboration between researchers and different research groups. This result revealed limited interaction among scholars studying AI in higher education. This result can be explained by a lack of understanding of how researchers should effectively collaborate with AI in teaching (Järvelä et al., 2023). Furthermore, our results regarding the top 10 institutions revealed that most of the institutions, according to the number of articles, were from Australia and the United States. The other institutions were from Mexico, the Netherlands, Spain, Scotland, Canada, and Saudi Arabia. This finding can be explained by the great success of researchers in countries such as Australia and the United States in using AI technologies in higher education. This means these countries are actively preparing and planning to use AI in education. Another reason may stem from the COVID-19 pandemic has also accelerated the adoption of AI in education due to the emphasis on online or distance learning. Our findings are consistent with other research that has found that developed countries have significantly contributed to the development of AI in education (Akhmadieva et al., 2023; López-Chila et al., 2024). In parallel with advances in AI technologies in developed countries, policymakers in these countries may have been very active in promoting research on the use of AI in education.
Furthermore, our results showed that the most influential studies were published between 2013 and 2018, and all the most influential articles focused on learning analytics. This finding has significantly influenced AI education research, as the results show that they were co-cited considerably by researchers who were also the authors of the analyzed articles in this study. Our results also included the hot topics in AI education research. They showed that AI research has focused on understanding the effects of instruction based on AI, and most studies were conducted in engineering education. The research between 2015 and 2017 was conducted on medical education in higher education. In addition, before 2015, research focused on using AI in medical education as a teaching method to implement problem-based learning in higher education. These findings, evident from previous studies, indicate that adopting AI as a teaching aid in online education is a significant development supporting traditional teaching methods, automatically analyzing teaching processes, and promoting effective teaching practices (Xiao and Yi, 2020).
The findings about research trends and topics after 2020 (between 2020 and 2023) have shown that research has focused on examining the effects of learning analytics, machine learning, academic performance, forecasting, online learning, and COVID-19 on academic performance in higher education. This result parallels the launch and development of ChatGPT. Before the introduction of ChatGPT in 2022, research on AI in higher education focused mainly on personalized learning environments, intelligent tutoring systems, and adaptive learning platforms. For example, Luckin and Holmes (2016) emphasized AI’s potential for customized learning, including machine learning algorithms that could adapt educational content based on student needs. Following the release of ChatGPT in November 2022, an AI model has dramatically changed the use of AI in higher education. The existence of ChatGPT has shifted to the potential of generative AI to help students with academic writing, provide real-time tutoring, and support research activities. Research has shown that AI, such as ChatGPT, is now directly integrated into educational practice to help create essay drafts, answer complex questions, and assist with literature searches. In the post-ChatGPT era, research has focused on using AI by students in higher education. In particular, the post-ChatGPT era has shifted to content creation, academic support, and various aspects of AI-enhanced learning in the higher education context. Similarly, we found in this research that AI has been used to examine the effects of learning analytics, machine learning, and online learning on academic performance.
Conclusion
The results of this research yielded several conclusions. Firstly, it is evident that the use of artificial intelligence in higher education has significantly grown in the past few years. This is evident in our findings, which indicated that research has increased, particularly after 2019. And a significant increase in the number of articles and citations exists. Furthermore, the results of research trends demonstrated that most papers centered around understanding the effects of AI-based instruction between 2017 and 2020. Studies between 2015 and 2017 were conducted on medical higher education. In addition, before 2015, scholars studied the use of AI in medical education to teach and implement problem-based learning in higher education. Thus, this study has revealed that AI has the potential to transform higher education by providing new teaching methods to involve students actively. Based on the results of this research, we can conclude that higher education educators should include AI technology in their curricula to learn and teach their course content. Nevertheless, educators must consider the ethical concerns of using artificial intelligence in education. To summarize our findings, the bibliometric analysis in this paper underscores the increasing significance of AI in higher education. Our results emphasize the research trends on AI. Our findings have implications for all researchers and educators in higher education. According to the researchers, this review has indicated the necessity for additional studies on artificial intelligence in higher education due to the existing shortage of research in this domain. Researchers must determine the extent to which AI may enhance higher education and identify the issues that must be addressed for its successful adoption.
Recommendations
First, to further our understanding of research trends regarding the use of AI in higher education, more in-depth studies are needed to explore future research trends. This study could not systematically review articles on AI in higher education due to the many articles. Second, future research could bridge this gap by conducting a systematic review of AI research on the effectiveness of publications on learning and teaching. Third, since our analysis focused solely on journal articles, future research should consider including other publication types, such as conference proceedings and book chapters, in future studies. Fourth, an additional recommendation is that a comparative analysis of the status of research in developed and developing countries can add new information to scholars’ knowledge. Fifth, because AI generates sophisticated content that raises questions, future research should focus on the ethical aspects of AI-generated content. Sixth, regarding the use of AI as personalized tutoring in real-time, future studies should investigate the effectiveness of AI-driven tutoring systems in different disciplines of higher education in terms of student engagement and learning. Seventh, the potential of AI tools such as ChatGPT to assist students with research tasks such as literature searches, writing assignments, and analyzing data is well known. Future studies should investigate how AI can be integrated into higher education. Finally, longitudinal studies that examine the long-term impact of integrating AI into higher education are necessary. Research to date has focused primarily on short-term studies in general. Hence, long-term studies are required to understand the use of AI in teaching and student achievement in higher education.
Limitations
This study analyzed research trends in higher education, and our research has some limitations. First, we only analyzed a limited number of publications. Our analysis was based on the SCOPUS database, and the publications analyzed were written in English. Therefore, we neglected articles in other languages and could not consider publications published in other databases. Second, we only used the VOSviewer software to identify research trends. Further studies should consider these limitations to gain further insights into AI research trends in higher education. Finally, more in-depth studies using the findings of this research are required.
Author contributions
RA: Writing – original draft, Writing – review & editing. NK: Writing – original draft, Writing – review & editing. TB: Writing – original draft, Writing – review & editing. AP: Writing – original draft, Writing – review & editing. NM: Writing – original draft, Writing – review & editing. VS: Writing – original draft, Writing – review & editing.
Funding
The author(s) declare that no financial support was received for the research, authorship, and/or publication of this article.
Conflict of interest
The authors declare that the research was conducted in the absence of any commercial or financial relationships that could be construed as a potential conflict of interest.
Publisher’s note
All claims expressed in this article are solely those of the authors and do not necessarily represent those of their affiliated organizations, or those of the publisher, the editors and the reviewers. Any product that may be evaluated in this article, or claim that may be made by its manufacturer, is not guaranteed or endorsed by the publisher.
References
Abbas, N., Ali, I., Manzoor, R., Hussain, T., and Hussain, M. H. A. I. (2023). Role of artificial intelligence tools in enhancing students’ educational performance at higher levels. J. Artif. Intell. Mach. Learn. Neural Netw. 35, 36–49. doi: 10.55529/jaimlnn.35.36.49
Akhmadieva, R. S., Udina, N. N., Kosheleva, Y. P., Zhdanov, S. P., Timofeeva, M. O., and Budkevich, R. L. (2023). Artificial intelligence in science education: a bibliometric review. Contemp. Educ. Technol. 15:ep460. doi: 10.30935/cedtech/13587
Akiba, D., and Fraboni, M. C. (2023). AI-supported academic advising: exploring ChatGPT’s current state and future potential toward student empowerment. Educ. Sci. 13:885. doi: 10.3390/educsci13090885
Akinwalere, S. N., and Ivanov, V. (2022). Artificial intelligence in higher education: challenges and opportunities. Border Crossing 12, 1–15. doi: 10.33182/bc.v12i1.2015
Aldosari, S. A. M. (2020). The future of higher education in the light of artificial intelligence transformations. Int. J. High. Educ. 9:145. doi: 10.5430/ijhe.v9n3p145
Bond, M., Khosravi, H., De Laat, M., Bergdahl, N., Negrea, V., Oxley, E., et al. (2024). A meta systematic review of artificial intelligence in higher education: a call for increased ethics, collaboration, and rigour. Int. J. Educ. Technol. High. Educ. 21, 1–41. doi: 10.1186/s41239-023-00436-z
Bozkurt, A., Karadeniz, A., Baneres, D., Guerrero-Roldán, A. E., and Rodríguez, M. E. (2021). Artificial intelligence and reflections from educational landscape: a review of AI studies in half a century. Sustain. For. 13:800. doi: 10.3390/su13020800
Chen, L., Chen, P., and Lin, Z. (2020). Artificial intelligence in education: a review. IEEE Access 8, 75264–75278. doi: 10.1109/access.2020.2988510
Cotton, D., and Gresty, K. A. (2005). Reflecting on the think-aloud method for evaluating e-learning. Br. J. Educ. Technol. 37, 45–54. doi: 10.1111/j.1467-8535.2005.00521.x
Ferguson, R. (2012). Learning analytics: drivers, developments and challenges. Int. J. Technol. Enhanced Learn. 4:304. doi: 10.1504/ijtel.2012.051816
Flores-Velásquez, C. H., Olivares-Zegarra, S., Davila-Ignacio, C., Arévalo-Tuesta, J. A., Morales-Romero, G., Trinidad-Loli, N., et al. (2024). A bibliometric review of studies about the acceptance of artificial intelligence technologies in teaching and learning in higher education. Int. J. Learn. Teach. Educ. Res. 23, 275–292. doi: 10.26803/ijlter.23.3.14
Gašević, D., Dawson, S., Rogers, T., and Gasevic, D. (2016). Learning analytics should not promote one size fits all: the effects of instructional conditions in predicting academic success. Internet High. Educ. 28, 68–84. doi: 10.1016/j.iheduc.2015.10.002
Gašević, D., Dawson, S., and Siemens, G. (2014). Let’s not forget: learning analytics are about learning. TechTrends 59, 64–71. doi: 10.1007/s11528-014-0822-x
Guo, S., Zheng, Y., and Zhai, X. (2024). Artificial intelligence in education research during 2013–2023: a review based on bibliometric analysis. Educ. Inf. Technol. 29, 16387–16409. doi: 10.1007/s10639-024-12491-8
Guo, K., Zhong, Y., Li, D., and Chu, S. K. W. (2023). Effects of chatbot-assisted in-class debates on students’ argumentation skills and task motivation. Comput. Educ. 203:104862. doi: 10.1016/j.compedu.2023.104862
Järvelä, S., Nguyen, A., and Hadwin, A. (2023). Human and artificial intelligence collaboration for socially shared regulation in learning. Br. J. Educ. Technol. 54, 1057–1076. doi: 10.1111/bjet.13325
Khan, M. A., Khojah, M., and Vivek, V. (2022). Artificial intelligence and big data: the advent of new pedagogy in the adaptive e-learning system in the higher educational institutions of Saudi Arabia. Educ. Res. Int. 2022, 1–10. doi: 10.1155/2022/1263555
Kim, K., and Kwon, K. (2024). A systematic review of the evaluation in K-12 artificial intelligence education from 2013 to 2022. Interact. Learn. Environ. 1–29, 1–29. doi: 10.1080/10494820.2024.2335499
Kuleto, V., Ilić, M., Dumangiu, M., Ranković, M., Martins, O. M. D., Păun, D., et al. (2021). Exploring opportunities and challenges of artificial intelligence and machine learning in higher education institutions. Sustain. For. 13:10424. doi: 10.3390/su131810424
Lave, J., and Wenger, E. (1991). Situated learning: Legitimate peripheral participation. Cambridge: Cambridge University Press.
López-Chila, R., Llerena-Izquierdo, J., Sumba-Nacipucha, N., and Cueva-Estrada, J. (2024). Artificial intelligence in higher education: an analysis of existing bibliometrics. Educ. Sci. 14:47. doi: 10.3390/educsci14010047
Luan, H., Geczy, P., Lai, H., Gobert, J., Yang, S. J. H., Ogata, H., et al. (2020). Challenges and future directions of big data and artificial intelligence in education. Front. Psychol. 11:580820. doi: 10.3389/fpsyg.2020.580820
Luckin, R., and Holmes, W. (2016). Intelligence Unleashed: An argument for AI in Education. Available at: http://oro.open.ac.uk/50104/.
Murillo, J., Villegas, L. M., Ulloa-Murillo, L. M., and Rodríguez, A. R. (2021). Recent trends on omics and bioinformatics approaches to study SARS-CoV-2: a bibliometric analysis and mini-review. Comput. Biol. Med. 128:104162. doi: 10.1016/j.compbiomed.2020.104162
Nagaraj, B. K., Easwaramoorthi, K., Begum, S. R., Akila, S., Sachdev, H. K., and Kumar, S. N. (2023). The emerging role of artificial intelligence in STEM higher education: a critical review. Int. Res. J. Multidiscip. Technovat. 5, 1–19. doi: 10.54392/irjmt2351
Ng, D. T. K., Leung, J. K. L., Su, J., Ng, R. C. W., and Chu, S. K. W. (2023). Teachers’ AI digital competencies and twenty-first century skills in the post-pandemic world. Educ. Technol. Res. Dev. 71, 137–161. doi: 10.1007/s11423-023-10203-6
Ouyang, F., Wu, M., Zheng, L., Zhang, L., and Jiao, P. (2023). Integration of artificial intelligence performance prediction and learning analytics to improve student learning in online engineering course. Int. J. Educ. Technol. High. Educ. 20:4. doi: 10.1186/s41239-022-00372-4
Ouyang, F., Zheng, L., and Jiao, P. (2022). Artificial intelligence in online higher education: a systematic review of empirical research from 2011 to 2020. Educ. Inf. Technol. 27, 7893–7925. doi: 10.1007/s10639-022-10925-9
Özdemir, A. Y. (2023). Publications on Germanistics in the Scopus database: a science mapping study. Int. J. Educ. Literacy Stud. 11, 84–94. doi: 10.7575/aiac.ijels.v.11n.3p.84
Pardo, A., and Siemens, G. (2014). Ethical and privacy principles for learning analytics. Br. J. Educ. Technol. 45, 438–450. doi: 10.1111/bjet.12152
Park, W., and Kwon, H. (2023). Implementing artificial intelligence education for middle school technology education in Republic of Korea. Int. J. Technol. Des. Educ. 34, 109–135. doi: 10.1007/s10798-023-09812-2
Popenici, S. A. D., and Kerr, S. (2017). Exploring the impact of artificial intelligence on teaching and learning in higher education. Res. Pract. Technol. Enhanc. Learn. 12:22. doi: 10.1186/s41039-017-0062-8
Pradana, M., Elisa, H. P., and Syarifuddin, S. (2023). Discussing ChatGPT in education: a literature review and bibliometric analysis. Cogent Educ. 10:2243134. doi: 10.1080/2331186x.2023.2243134
Prahani, B. K., Rizki, I. A., Jatmiko, B., Suprapto, N., and Tan, A. (2022). Artificial intelligence in education research during the last ten years: a review and bibliometric study. Int. J. Emerg. Technol. Learn. 17, 169–188. doi: 10.3991/ijet.v17i08.29833
Rebolledo Font De La Vall, R., and González Araya, F. (2023). Exploring the benefits and challenges of ai-language learning tools. Int. J. Soc. Sci. Hum. Invent. 10, 7569–7576. doi: 10.18535/ijsshi/v10i01.02
Ruwe, T., and Mayweg-Paus, E. (2023). “Your argumentation is good”, says the AI vs humans – the role of feedback providers and personalised language for feedback effectiveness. Comput. Educ. Artif. Intell. 5:100189. doi: 10.1016/j.caeai.2023.100189
Schiff, D. (2020). Out of the laboratory and into the classroom: the future of artificial intelligence in education. AI & Soc. 36, 331–348. doi: 10.1007/s00146-020-01033-8
Schumacher, C., and Ifenthaler, D. (2018). Features students really expect from learning analytics. Comput. Hum. Behav. 78, 397–407. doi: 10.1016/j.chb.2017.06.030
Shamkuwar, M., Jindal, P., More, R., Patil, P., and Mahamuni, P. (2023). Artificial intelligence and higher education: a systematic visualizations based review. J. e-Learn. Knowl. Soc. 19, 36–42. doi: 10.20368/1971-8829/1135857
Siemens, G. (2013). Learning analytics. Am. Behav. Sci. 57, 1380–1400. doi: 10.1177/0002764213498851
Slade, S., and Prinsloo, P. (2013). Learning analytics. Am. Behav. Sci. 57, 1510–1529. doi: 10.1177/0002764213479366
Sungkono, N., and Widana, N. I. D. K. K. (2024). Optimizing artificial intelligence (AI) as a catalyst for digital economic transformation to increase National Economic Growth. Tech. Soc. Sci. J. 58, 235–247. doi: 10.47577/tssj.v58i1.11174
Tapalova, O., and Zhiyenbayeva, N. (2022). Artificial intelligence in education: AIEd for personalised learning pathways. Electr. J e-Learn. 20, 639–653. doi: 10.34190/ejel.20.5.2597
Thomas, T. (2024). The role of artificial intelligence in formal and informal education for students. Int. J. Res. Appl. Sci. Eng. Technol. 12, 69–71. doi: 10.22214/ijraset.2024.58738
Toapanta, S. M. T., Díaz, E. Z. G., Vizuete, O. M. Z., and Chávez, E. E. O. (2022). Analysis of artificial intelligence applied in virtual learning environments in higher education for Ecuador. Front. Artif. Intell. Appl. 363, 436–443. doi: 10.3233/faia220563
Urban, M., Děchtěrenko, F., Lukavský, J., Hrabalová, V., Svacha, F., Brom, C., et al. (2024). ChatGPT improves creative problem-solving performance in university students: an experimental study. Comput. Educ. 215:105031. doi: 10.1016/j.compedu.2024.105031
Van Eck, N. J., and Waltman, L. (2010). Software survey: VOSviewer, a computer program for bibliometric mapping. Scientometrics 84, 523–538. doi: 10.1007/s11192-009-0146-3
Viberg, O., Hatakka, M., Bälter, O., and Mavroudi, A. (2018). The current landscape of learning analytics in higher education. Comput. Hum. Behav. 89, 98–110. doi: 10.1016/j.chb.2018.07.027
Waltman, L., Van Eck, N. J., and Noyons, E. C. (2010). A unified approach to mapping and clustering of bibliometric networks. J. Informet. 4, 629–635. doi: 10.1016/j.joi.2010.07.002
Wang, S., Sun, Z., and Chen, Y. (2023). Effects of higher education institutes’ artificial intelligence capability on students’ self-efficacy, creativity and learning performance. Educ. Inf. Technol. 28, 4919–4939. doi: 10.1007/s10639-022-11338-4
Wang, Y., and Zheng, G. (2020). Application of artificial intelligence in college dance teaching and its performance analysis. Int. J. Emerg. Technol. Learn. 15:178. doi: 10.3991/ijet.v15i16.15939
Xiao, M., and Yi, H. (2020). Building an efficient artificial intelligence model for personalized training in colleges and universities. Comput. Appl. Eng. Educ. 29, 350–358. doi: 10.1002/cae.22235
Zawacki-Richter, O., Marín, V. I., Bond, M., and Gouverneur, F. (2019). Systematic review of research on artificial intelligence applications in higher education – where are the educators? Int. J. Educ. Technol. High. Educ. 16:39. doi: 10.1186/s41239-019-0171-0
Keywords: higher education, bibliometric analysis, research trend, artificial intelligence, AI
Citation: Akhmadieva RS, Kalmazova NA, Belova T, Prokopyev A, Molodozhnikova NM and Spichak VY (2024) Research trends in the use of artificial intelligence in higher education. Front. Educ. 9:1438715. doi: 10.3389/feduc.2024.1438715
Edited by:
Aleksander Aristovnik, University of Ljubljana, SloveniaReviewed by:
Denice Hood, University of Illinois at Urbana-Champaign, United StatesJason S. Wrench, State University of New York at New Paltz, United States
Copyright © 2024 Akhmadieva, Kalmazova, Belova, Prokopyev, Molodozhnikova and Spichak. This is an open-access article distributed under the terms of the Creative Commons Attribution License (CC BY). The use, distribution or reproduction in other forums is permitted, provided the original author(s) and the copyright owner(s) are credited and that the original publication in this journal is cited, in accordance with accepted academic practice. No use, distribution or reproduction is permitted which does not comply with these terms.
*Correspondence: Tatyana Belova, YmVsb3ZhLnRhdGlhbmE5MkBtYWlsLnJ1