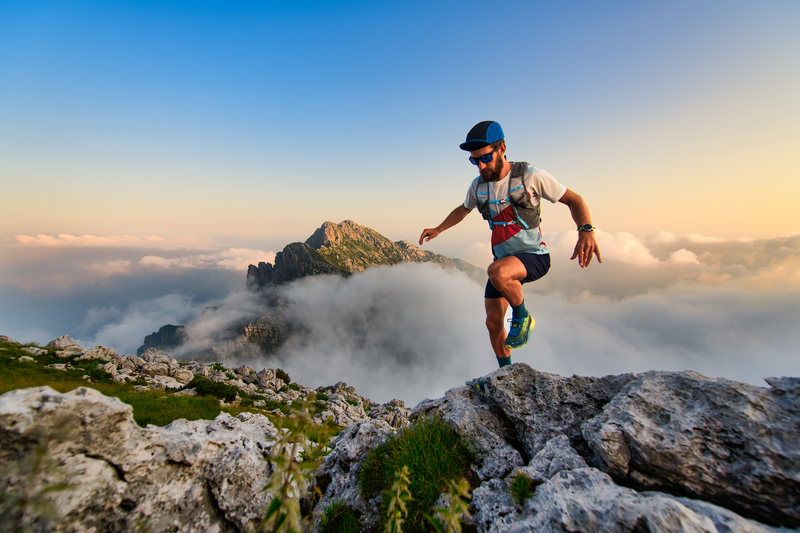
95% of researchers rate our articles as excellent or good
Learn more about the work of our research integrity team to safeguard the quality of each article we publish.
Find out more
ORIGINAL RESEARCH article
Front. Educ. , 17 December 2024
Sec. Digital Learning Innovations
Volume 9 - 2024 | https://doi.org/10.3389/feduc.2024.1435483
This article is part of the Research Topic Designing, implementing and evaluating self-regulated learning experiences in online and innovative learning environments View all 5 articles
Introduction: Monitoring and controlling learning is often difficult for primary school students. This issue is partially resolved when Adaptive Learning Technologies (ALTs) take over part of these self-regulated learning (SRL) processes. Trace data in ALTs provides elaborate information on students' learning process, which can be translated into monitoring support. However, this data does not provide insight into students' goal-setting behavior, which is a crucial part of the monitoring loop. Therefore, we developed a form of co-regulation between the student and the ALT with goal-setting prompts and monitoring support.
Method: This experimental study compared an experimental condition in which primary school students worked with the co-regulation intervention while practicing mathematics problems in the ALT to a control condition where they only practiced with the ALT. Firstly, we examined the effects of co-regulation with ALTs on regulation of practice behavior and learning outcomes by comparing the experimental and control conditions. Secondly, to gain further insight into students' goal-setting behavior in co-regulation, we examined how the experimental condition set their goals and attained them.
Results: Results showed that students in the experimental condition were more effective in regulating their practice behavior, shown by more problems solved and higher accuracy than the control condition. Similar learning gain was found in both conditions on the easy and intermediate learning topics. For the hard learning topic, the control condition showed a higher learning gain. Higher week goals than day goals on all three learning topics and similar goals were set between the learning topics. Students' goal attainment was less for the easy compared to the hard learning topic.
Discussion: Combined, these results indicate that co-regulation with an ALT positively affects students' regulation of practice behavior and goal- setting behavior but does not yet increase learning gain. A practical implication is that teachers could support students by explicitly providing students with goal-setting opportunities.
Research has indicated the importance of self-regulated learning (SRL) for learning, both for the development of knowledge that is well integrated into students' existing knowledge as well as for the development of SRL skills to support lifelong learning (Azevedo et al., 2008; Järvelä and Bannert, 2019). At the same time, monitoring and controlling learning is often difficult for primary school students: they struggle to accurately make monitoring judgments and subsequently control their learning (Azevedo et al., 2008; van Loon and Roebers, 2021). This issue is partially resolved when students work with Adaptive Learning Technologies (ALTs), which are widely used in upper primary education, as the technology takes over part of their monitoring and control (Molenaar et al., 2021). Hence, to develop SRL skills, this should not always be taken over from the student (Molenaar, 2022a; Greene and Azevedo, 2007; Usher and Schunk, 2017; Winne and Hadwin, 1998).
Therefore, we need to think about ways in which ALTs can support students in learning to successfully monitor and control their own learning instead of taking this away from the student. A gradual shift from external to internal control can be helpful to establish this (Azevedo et al., 2008; Dignath and Büttner, 2018; Molenaar, 2022a). This shift can be guided by co-regulation, which can be defined as support for (self-regulated) learning by the environment or others in the environment of the student (Hadwin et al., 2017; McCaslin, 2004). Not only can fellow students or teachers aid co-regulation, but technology can also play a role (Lajoie and Lu, 2012). Dashboards can support co-regulation between the ALT and student by, for example, showing students the adjustments of the ALT on the difficulty of tasks (Jivet et al., 2018; Molenaar, 2022b). Moreover, students can be shown examples on how to monitor their learning by showing them their learning progress (Dignath and Büttner, 2008; Molenaar, 2022a). Co-regulation can, therefore, be implemented with different foci. As little is known about co-regulation with ALTs in the literature, this study tries to fill this gap. Therefore, in this study, the co-regulation intervention consisted of goal-setting prompts and monitoring support by showing students their learning progress.
Trace data in ALTs provide elaborate information on learning processes, which can be translated into monitoring support that provides students with real-time insight into their learning progress (Jivet et al., 2018; Molenaar, 2022a; Molenaar et al., 2020). However, this data does not provide insight into students' intentions toward learning, such as their goal-setting behavior, which is a crucial part of monitoring (Panadero, 2017; Winne, 2010). Setting goals is a prerequisite to evaluating students' performance and diagnosing their progress toward the goals set (Winne and Hadwin, 1998). Therefore, prompting students' goal setting is a crucial aspect of providing this monitoring support (Dignath and Büttner, 2008).
A co-regulation intervention could potentially support students with goal-setting and subsequent monitoring processes during learning (Hadwin et al., 2017; Lajoie and Lu, 2012; McCaslin, 2004). Yet, it is currently unknown how this form of co-regulation affects students' goal-setting behavior and learning outcomes when learning with ALTs in primary education. Therefore, the effect of co-regulation with an ALT on goal-setting behavior, the regulation of practice behavior and learning outcomes of primary school students was investigated by comparing the experimental (co-regulation with an ALT) and control conditions (only ALT, no co-regulation). Moreover, to understand how this co-regulation intervention plays a role in the actual goal-setting behavior of students, we examined how students in the co-regulation condition set their goals and if they attain them.
In educational research, SRL is widely used as a framework to understand cognitive, emotional, and motivational factors that influence learning (Greene and Azevedo, 2007; Panadero, 2017). The COPES model (Winne and Hadwin, 1998) focuses on students' internal regulation processes during learning. In this model, SRL unfolds in four linked phases during learning. The first phase entails task definition, in which students generate an understanding of the task at hand and go over factors that influence the task (Winne, 2010). The second phase involves goal setting and planning, where students set learning goals and plan their actions to achieve these goals. These goals are reached when a specific criterion is met (Winne, 2010). Hence, the first two phases, in which orientation on the task and goal setting take place, are prerequisites for sound monitoring and control in the subsequent execution phase. This third phase includes ongoing monitoring while enacting study tactics and strategies while students execute actions needed to reach their goals. This is the phase where students solve problems, monitor progress, and constantly make judgments of their learning (JOLs) (Koriat, 1997). When needed, they enact control by adjusting their strategies in so-called small-scale adaptations (Winne, 2010; Winne and Hadwin, 1998). To determine whether there is a need for these adaptations, students make JOLs comparing their actual performance to their set goals and consequently cognitively evaluate their progress (Molenaar et al., 2020; Winne and Hadwin, 1998). Finally, in the fourth phase, the adaptation phase, students use their JOLs to reflect on the three previous phases and determine if their progress was according to plan. If not, students adjust their goals, strategies, or tactics for the next lesson (large-scale adaptations; Winne, 2010).
Hence, SRL can be seen as a goal-oriented process in which students make conscious choices while working toward their goals (Winne and Hadwin, 1998; Zimmerman, 2000). To reach their learning goals, students engage in various cognitive activities, such as reading and practicing, and metacognitive activities, which entail orientation, planning, executing, monitoring, and evaluation (e.g., Boekaerts, 1999). Students can use these metacognitive activities to monitor and control their learning (e.g., Veenman, 2013), which are two central processes in SRL (e.g., Panadero, 2017; van Merriënboer and de Bruin, 2019). As students work toward their goals (e.g., mastery of a skill), they rely on monitoring judgments to regulate their learning (De Bruin et al., 2017; Koriat, 1997). Students check available information about their performance and consequently judge their knowledge development via JOLs (De Bruin et al., 2017; Koriat, 1997). This information can inform monitoring and drive control over practice behavior in relation to the goals a student has set, for instance, by adjusting the effort they invest in the task (De Bruin et al., 2017; Molenaar et al., 2020, 2019a). Hence, goal setting, monitoring, and control are closely linked in a cycle and have reciprocal influence on each other (van Merriënboer and de Bruin, 2019).
Currently, when students work with ALTs, part of their monitoring and control is taken over by the ALT. Many upper primary school students in Dutch primary education use ALTs on a daily basis to learn mathematics, spelling, and grammar (Karssen et al., 2023). The adaptive problem selection process in ALTs was originally developed as support for cognitive activities (Klinkenberg et al., 2011), but also offloads regulation from students (Molenaar et al., 2019a). ALTs support students in monitoring by providing immediate feedback after each completed task (Aleven et al., 2016; Pardo et al., 2019; VanLehn, 2011). At the same time, this takes away the need for students to actively monitor themselves, for example by checking their answers themselves before proceeding (Molenaar, 2022a). Control is taken over from the student by ALTs by selecting problems that are adapted to the ability level of students(Molenaar et al., 2019a). This reduces the need for students to make accurate monitoring judgments when practicing, adjust their effort, or change strategies and tactics (Molenaar et al., 2019b). This diminished the opportunities to engage in active monitoring and control and therefore develop their SRL skills (Greene and Azevedo, 2007; Usher and Schunk, 2017; Winne and Hadwin, 1998).
Instead of taking over monitoring and control from the student, a gradual shift from external to internal control is needed to enhance the development of students' SRL skills (Azevedo et al., 2008; Dignath and Büttner, 2008; Molenaar, 2022a,b). One step within this process is described as co-regulation, which addresses the process of developing SRL skills during learning through interactions with others (Hadwin et al., 2017). In the formal definition, teachers or peers engage the student in different SRL challenges and provide expertise on the topic, resulting in the prompting of regulation processes. The idea would be that co-regulation with others over time can lead to internalization of SRL skills in individual students (e.g., Baars et al., 2020). Previous research on co-regulation mostly describes peers and teachers to be in interaction with the student (e.g., Hadwin et al., 2017). In our case, the ALT will function as their interaction partner, resulting in co-regulation with ALTs. Continuous interaction takes place between the student and the ALT in which the student is actively prompted to set goals, informed by the ALT about progress toward the goals. In this way regulation is transferred between the ALT and the student.
An essential element in co-regulation is active goal setting which is crucial for monitoring during (self-regulated) learning (Panadero, 2017; Winne, 2010). Students often face a utilization deficiency, indicating they fail to activate the control and monitoring loop (Winne and Hadwin, 2013). This activation largely depends on the goals students set (Molenaar et al., 2019b; Winne, 2017). Setting goals is a prerequisite for students to evaluate their performance and diagnose their progress (Winne and Hadwin, 1998). In SRL, students use goals to aid their learning actions (Zimmerman, 2000).
When students effectively engage in goal setting, their understanding of a task increases and students can focus more on relevant strategies to successfully complete the task (Locke and Latham, 1990; Schunk, 1990). Previous studies indeed showed that setting goals can improve learning outcomes (Gardner et al., 2016; Moeller et al., 2012; Morgan, 1985). However, earlier research showed that young students often face challenges while setting their goals (Schunk, 1990). Students often skip setting goals before their learning sessions and do not actively engage in the goal-setting step of SRL (Nedzinskaite-Mačiuniene and Šimiene, 2021). Consequently, this could lead to less SRL skills. Prompting young students to actively set goals could be a way to engage students in goal setting (Dignath and Büttner, 2008) and is therefore found to be a crucial part of co-regulation. By encouraging students to actively set their goals in the ALT and the ALT informing students on goal attainment, a bidirectional interaction between the ALT and student to regulate the learning process would take place.
Research indicates that students do seem to benefit from external support to execute SRL (Azevedo et al., 2008; Molenaar et al., 2019a). Both teachers, as well as technology can provide this support. Technology can help students by explaining and modeling SRL processes via scaffolds or prompts (Wong et al., 2019) and teachers by explicitly teaching SRL strategies (Dignath and Büttner, 2018; Ewijk et al., 2013). External support based on trace data has been shown to improve SRL (Azevedo et al., 2008; Molenaar et al., 2019a) and can be seen as a way to make students understand how the ALT monitors and controls their learning process in co-regulation. For example, progress charts informed by trace data show potential for reflecting on learning progress in previous studies (Arroyo et al., 2007). Offering monitoring support by showing students their learning progress, such as their completed tasks or measures of knowledge is commonly done in previous studies (Bodily et al., 2018b). Student-faced dashboards that use trace data to provide this monitoring support were found to improve SRL skills in students (Bodily et al., 2018a; Molenaar et al., 2020). Therefore, monitoring support is seen as the second crucial aspect of co-regulation in ALTs next to the goal-setting prompts. In this study, therefore, we combine the two above-mentioned crucial aspects of co-regulation by combining goal-setting prompts and monitoring support. With those two features, co-regulation with ALTs encompasses interaction between the student and ALT.
In this experimental study, we examined the effects of a co-regulation intervention while practicing mathematics with an ALT, consisting of goal-setting prompts and monitoring support, on the regulation of practice behavior and learning outcomes. We compared an experimental condition (co-regulation with ALT) with a control condition (only ALT, no co-regulation). Moreover, to gain further insight into students' goal setting in co-regulation, we examined how students set their goals in co-regulation and to which extent they attained them.
The research questions were:
1. What is the effect of co-regulation with an ALT on regulation of practice behavior?
2. What is the effect of co-regulation with an ALT on learning outcomes?
3. How do students set goals and to what extent do they attain these goals (goal-setting behavior) when engaged in co-regulation with an ALT?
In general, co-regulation is expected to support students to monitor their own learning more accurately (Azevedo et al., 2008; Hadwin et al., 2017; McCaslin, 2004). As a consequence of accurate monitoring, they are also expected to execute more effective control and, therefore, to show better practice behavior (De Bruin et al., 2017; van Merriënboer and de Bruin, 2019). Consequently, more effective monitoring and control during learning is expected to increase learning outcomes (e.g., De Bruin et al., 2017). Specifically, prompting students to actively set goals (e.g., Dignath and Büttner, 2008; Gardner et al., 2016; Moeller et al., 2012) and support the process of monitoring their goal attainment via co-regulation with an ALT (e.g., Lajoie and Lu, 2012), is expected to improve monitoring and control during learning math in primary education. Therefore, in our current study, we first expected that students in the co-regulation condition showed better regulation of practice behavior by completing more problems (Hypothesis 1a) and attained higher accuracy (Hypothesis 1b) compared to the control condition. Secondly, we expected that students in the co-regulation condition showed higher learning gains compared to students in the control condition (Hypothesis 2). Due to the explorative nature of the third research question, no specific hypotheses were formulated.
This study was conducted with a quasi-experimental pre-post-test design (Figure 1). Participants in this study were 114 grade 5 students from 4 schools (one class per school) located in the South and East of the Netherlands. Schools were contacted only when they worked with the ALT used in this study in their daily practice, to prevent novelty effects. Fifty-five boys and 59 girls between 10 and 13 years old participated in this study, with a mean age of 10.97 (SD = 0.60).
Each school was randomly assigned to either the experimental condition (co-regulation) or the control condition: all students from two schools were assigned to the control condition and all students from two other schools were assigned to the experimental condition. Students in the control condition were, on average, 6 months older than students in the experimental condition, t(112) = 5.96, p < 0.01, d = 1.12, and there were more girls in the control condition, t(112) = 2.05, p = 0.04, d = 0.39.
Participants were included in the analysis when they participated in at least three out of four lessons. Based on this inclusion criterion, 20 students that missed more than one lesson were excluded from the analyses. The excluded students had a similar age as the included participants [t(125) = 0.665, p = 0.507, d = 0.085], and there were no differences in girl/boy ratio between included and excluded participants [t(125) = 0.292, p = 0.771, d = 0.195].
The experiment took place during 1 week, with four mathematics lessons about fractions. On the 1st day, students completed the pre-test (30 min) and participated in the first instruction lesson on simplifying basic fractions. The two other instruction lessons (simplifying mixed and complex fractions) and the repetition lesson were given on consecutive days following the first lesson. On the 5th day, students completed the post-test (30 min) and transfer test (30 min). The lessons had a duration of 55 min.
The structure of each lesson was comparable. The first 10 min consisted of setting goals in the Learning Path app for the co-regulation condition and a puzzle for the control condition. Next, the teacher used a protocol to give standardized instruction about the learning topic. Consequently, all students worked on individual problems in the ALT for 30 min. In the co-regulation condition, students could monitor their progress toward their goals during practice. The last 5 min of each lesson consisted of a reflection on the lesson. The three learning topics of the previous lessons were repeated and practiced in the repetition lesson. Students could choose which of the three previous learning topics to work on and could switch between them.
The Adaptive Learning Technology (ALT) used in this study is used from grade 1 to grade 6 in primary education in the Netherlands. Students can practice mathematics, spelling, language, and vocabulary with this ALT. This technology is applied in blended classrooms where the teacher gives instruction, after which students practice individually on their tablets. The ALT adjusts the difficulty level of problems to students' needs. It selects problems for students based on an estimate of their current knowledge on a learning topic, also called the ability level (Corbett and Anderson, 1994). This ability level is calculated by a derivative of the Elo algorithm (Elo, 1978; Klinkenberg et al., 2011). Every problem also has a difficulty score, which is adjusted continuously. If a student gives an incorrect answer, their ability level is lowered and the difficulty score of that problem increases (Klinkenberg et al., 2011). The ALT selects problems by using a probability of 75% that the student will solve that problem correctly (Aleven et al., 2016; Elo, 1978; Klinkenberg et al., 2011). When the student solved this problem correctly, their ability level increased, whereas an incorrect answer decreased their ability level (Klinkenberg et al., 2011).
After each problem, the student receives feedback indicating if their answer is correct or incorrect. If their answer is incorrect, the student gets a second attempt. The system repeats problems that are answered incorrectly twice at the end of each set of twelve problems. After each set, students see their number of correct and incorrect answers. The teacher can view students' progress during learning via a dashboard included in the ALT.
Participants worked on three mathematics learning topics in the current study. All learning topics covered simplifying fractions and increased in difficulty over the lessons. The problems related to the first learning topic, “simplifying basic fractions,” were relatively easy. Therefore, this learning topic was classified as the easy learning topic. The problems related to the second one, “simplifying mixed fractions,” were of intermediate difficulty. This learning topic was classified as the intermediate learning topic. The last learning topic, “simplifying complex fractions,” was difficult because of the use of large numbers and thus called the hard learning topic. Therefore, this learning topic was classified as the hard learning topic. Table 1 shows examples of problems within the three learning topics.
In the experimental condition, participants engaged in co-regulation via the Learning Path app. This app consists of goal-setting prompts and monitoring support. This app is developed to gradually guide students through the SRL process by following the four phases of the COPES model: task orientation, goal setting, execution of the task, and making adaptations (Winne and Hadwin, 1998). Students received standardized teacher-guided instruction on the Learning Path app. A poster in the classroom was used as a reminder for the students on the meaning of all information provided by the app.
The Learning Path app consisted of 3 screens that serve as dashboards: the overview screen, goal setting screen, and learning path screen. For task orientation, the overview screen of the app provides insight into the learning topics students are working on during the week (Figure 2A). Three dolphins each represent one of the three mathematics learning topics in this experiment.
Figure 2. (A) Overview screen: task definition phase. (B) Goal-setting: goal-setting phase. (C) Overview screen with prompts: execution phase. (D) Goal setting screen with prompts: execution phase. (E) Learning path screens.
For goal setting, students were asked explicitly to set their goals in the goal-setting screen (Figure 2B). Students were asked to set three types of goals by indicating the proficiency they wanted to reach (1) during the first lesson on the learning topic (day goal), (2) after the repetition lesson, and (3) at the end of the experiment (week goal). By moving the flag on a scale from 0% to 100%, students set their goals, the percentages reflecting the ability level in the ALT they wanted to achieve.
After setting their goals, students started executing the task by individually practicing on fractions tasks. During and after learning, students could view progress toward their learning goals in the overview (Figure 2C) and goal-setting screen (Figure 2D). Different prompts were provided to the student in these screens and a separate learning path screen, see Table 2 for the prompt and Figure 2E for the screens. For a more elaborate description of the learning paths provided to the student (see Molenaar et al., 2019a).
The following student characteristics were measured: age in years and months and gender.
Based on the information from the ALT, the number of problems and accuracy were extracted to indicate regulation of practice behavior. Both are measured by one indicator per learning topic. The number of problems was indicated by the number of unique problems a student solved while practicing one learning topic (not including repeated problems). Accuracy was the percentage of correctly answered problems, calculated by dividing the number of correctly solved problems by the total number of problems students solved.
For learning outcomes, the pre- and post-test scores were used. The pre- and post-tests both consisted of 24 problems, eight items per learning topic. The items in the pre- and post-test were structured similarly, but different fractions were used. The problems all were existing exercises in the ALT, and the problems' difficulty levels were similar between the tests. The Cronbach's alpha for the whole pre-test (α = 0.82) and post-test were both good (α = 0.87). For the first learning topic of simplifying basic fractions, the pre- and post-test had similar Cronbach's alpha's (α = 0.77 and α = 0.76). Learning topic 2 contained mixed fractions and had a lower Cronbach's alpha for the pre-test (α = 0.72) than for the post-test (α = 0.84). For the third learning topic containing complex fractions, the pre-test (α = 0.80) had a higher Cronbach's alpha than the post-test (α = 0.72). The differences in Cronbach's alpha could be explained by the different exercises used in pre- and post-test.
The goals students set per learning topic (easy, intermediate, and hard) were stored in the log files of the Learning Path app. These goals were indicated by a percentage from 0 to 100. These percentages reflect the ability level they wanted to achieve in the ALT. The goal they set at the start of each new lesson on a learning topic (day goal) and the goal they set for the end of the experiment (week goal) were used. Both goals were set when they first started a new learning topic.
Students' goal attainment was based on the extent students obtained their goals for each learning topic. Goal attainment was calculated by the set goal minus the ability level after the lesson and then made absolute (removing the minus sign when negative). A higher score on goal attainment means a larger gap between set goal and ability level, so less goal attainment.
To answer research question 1 on regulation of practice behavior (number of problems solved and accuracy), data was analyzed using GLM Repeated Measures ANOVA with Learning topic (easy/intermediate/hard) as within-subjects-factor and Condition (experimental/control) as between-subjects-factor. This was done for the number of problems solved and accuracy separately. Sphericity was checked with Maulchy's test. Follow-up analyses were conducted with paired-samples t-tests when there was a main effect of Learning topic and independent samples t-tests when there was a main effect of Condition.
For research question 2 on learning outcomes, GLM Repeated Measures ANOVA was conducted with pre- and post-test on the Learning topics (easy/intermediate/hard) as within-subjects factor and Condition (experimental/control) as between-subjects factor. Sphericity was checked with Maulchy's test. Follow-up analyses were conducted with paired-samples t-tests when there was a main effect of Learning topic and independent samples t-tests when there was a main effect of Condition.
To answer research question 3 on goal-setting behavior in the co-regulation condition, the goals that students set and goal attainment were explored. The goals students set were indicated by a percentage from 0 to 100, reflecting the ability level they wanted to achieve in the ALT. For set goals, GLM Repeated Measures ANOVA was conducted with Learning topic (easy/intermediate/hard) and Goal type (day goal/week goal) as within-subjects factors. Sphericity was checked with Maulchy's test. Follow-up analyses were conducted with independent samples t-tests. Goal attainment was calculated by the set goal minus the ability level after the lesson. For goal attainment, GLM Repeated Measures ANOVA was conducted with Learning topic (easy/intermediate/hard) and Goal type (day goal/week goal) as within-subjects factors. Follow-up analyses were conducted with independent samples t-tests.
To answer how the co-regulation intervention influenced students' regulation of practice behavior, the differences between the experimental and control conditions on the number of problems solved and accuracy were analyzed (Table 3).
For the number of problems (see Figure 3), Mauchly's test showed that the assumption for sphericity was violated, χ(2) = 0.79, p < 0.001; therefore, Greenhouse-Geisser correction was used. There was a significant main effect of Learning topic, F(1.65,111) = 9.48, p < 0.001, partial η2 = 0.078. Post-hoc analyses showed that students solved fewer problems for the intermediate than for the easy learning topic, t(113) = 3.33, p = 0.001, d = 0.31, and the hard learning topic, t(113) = 4.507, p < 0.001, d = 0.42. There was a significant main effect of Condition, F(1,111) = 4.26, p = 0.041, partial η2 = 0.037. Students in the co-regulation condition solved more problems than the control condition. There was no significant interaction effect between Learning topic and Condition, F(1,111) = 2.86, p = 0.07, partial η2 = 0.025.
For accuracy (see Figure 4), there was a significant main effect of Learning topic, F(2,224) = 183.43, p < 0.001, partial η2 = 0.621. Post-hoc analyses showed that students solved a lower number of problems correctly for the hard learning topic than for the easy, t(113) = 15.79, p < 0.001, d = 1.48, and for the intermediate learning topic, t(113) = 19.01, p < 0.001, d = 1.78. Students solved more problems correctly for the easy and intermediate learning topics than for the hard topic. There was a significant effect of Condition, F(1,112) = 45.68, p < 0.001, partial η2 = 0.290. Students in the co-regulation condition had a higher accuracy than the control condition. There was no significant interaction effect between Learning topic and Condition, F(2,112) = 1.26, p = 0.29, partial η2 = 0.011.
To answer how the co-regulation intervention affected learning outcomes, the differences between the experimental and control condition's scores on the pre-test and post-test were analyzed (Table 4, Figure 5).
Mauchly's test showed that the assumption for sphericity was violated, χ(2) = 9.677, p = 0.008, therefore, Greenhouse-Geisser correction was used. There was a significant main effect of Time, F(1,107) = 303.26, p < 0.001, partial η2 = 0.739. Students scored higher on the post-test than on the pre-test, indicating learning gain. There was a significant main effect of Learning topic, F(1.84,196.83) = 128.87, p < 0.001, partial η2 = 0.546. Post-hoc analyses showed that students scored higher on the intermediate learning topic than the easy learning topic, t(108) = 5.25, p < 0.001 d = 0.50, and hard learning topic, t(108) = 19.05, p < 0.001, d = 1.82. Students scored also higher on the easy learning topic than on the hard learning topic, t(108) = 9.17, p < 0.001, d = 0.90. There was a significant main effect of Condition, F(1,107) = 31.52, p < 0.001, partial η2 = 0.228. Students in the co-regulation condition scored higher (on pre- and post-test combined) than students in the control condition.
An interaction effect was found between Learning Topic and Condition, F(1.840,196.827) = 6.32, p = 0.003, partial η2 = 0.056. However, follow-up analyses per Learning Topic showed that the experimental condition scored higher than the control condition on all three learning topics [easy: t(107) = 2.36, p = 0.020, d = 0.45, intermediate: t(107) = 5.93, p < 0.001, d = 1.14, hard: t(107) = 6.17, p < 0.001, d = 1.19]. No interaction effect was found between Learning topic and Time, F(1.869,200.027) = 2.34, p = 0.056, partial η2 = 0.021, indicating that the difference between pre- and post-test scores was similar for all learning topics. Also, no interaction effect was found between Condition and Time, F(1,107) = 3.87, p = 0.052, partial η2 = 0.035, indicating that overall learning gain was comparable between the conditions.
A three-way-interaction was found between Time, Learning topic and Condition, F(1.869,200.027) = 4.63, p = 0.012, partial η2 = 0.041 (see Figure 5). Follow-up analyses per Learning topic showed that the control condition had a higher learning gain than the experimental condition for the hard learning topic, F(1,107) = 10.67, p = 0.001, partial η2 = 0.091. Similar learning gain was found for the easy, F(1,107) = 0.90, p = 0.345, partial η2 = 0.008, and intermediate learning topic, F(1,107) = 2.24, p = 0.137, partial η2 = 0.021. Thus, students in both conditions learned equally well on easy and intermediate learning topics, but for the hard topic, the control condition outperformed the co-regulation condition.
To understand the differences we found in the regulation of practice behavior and learning between the students who took part in co-regulation (experimental condition) and the control condition, we examined the goals students set (Table 5) and goal attainment in the experimental condition (Table 6).
At the beginning of each lesson, students in the experimental condition (co-regulation) set their goals for that lesson (day goal) and the end of the week (week goal) for the easy, intermediate, and hard learning topics (see Figure 6). There was a significant main effect of Goal type, F(1,41) = 35.81, p < 0.001, partial η2 = 0.466. Students set significantly higher week goals than day goals. No significant main effect of Learning topic was found, F(2,82) = 0.47, p = 0.626, partial η2 = 0.194. Students set similar goals across learning topics.
There was a significant interaction between Goal type and Learning topic, F(2,82) = 7.66, p < 0.001, partial η2 = 0.157. However, follow-up analyses per Learning topic did not find differences between these goals: students set higher week goals than day goals on all three learning topics [easy: t(46) = 7.74, p < 0.001, d = 1.13, intermediate: t(46) = 3.58, p < 0.001, d = 0.52, and hard: t(48) = 2.95, p = 0.005, d = 0.42]. This indicates that students aimed toward becoming more skilled at the end of the experiment.
Next, we examined students' goal attainment (see Figure 7), the difference between their intended performance (set goal) and actual performance (ability level). Students underestimated themselves for the easy and intermediate learning topics and overestimated for the hard learning topic (Table 6).
There was a significant effect of Goal type, F(1,39) = 14.587, p < 0.001, partial η2 = 0.272. Goal attainment was less for the day goals, indicating a larger gap between the set goal and students' ability levels for the day goal compared to the week goal. A significant main effect of Learning topic was found, F(2,78) = 5.161, p = 0.008, partial η2 = 0.117. Post-hoc analyses showed that goal attainment was higher for the easy learning topic than for the hard learning topic, t(43) = 3.62 p < 0.001, d = 0.55, indicating a larger gap between the set goal and students' ability level for the easy learning topic compared to the hard learning topic. No differences were found regarding the intermediate learning topic. There was no significant interaction between Goal Type and Learning topic, F(2,78) = 1.111, p = 0.334, partial η2 = 0.028.
This experimental study examined the effect of co-regulation in upper primary school students when learning mathematics with an ALT, using goal-setting prompts and monitoring support, on students' regulation of practice behavior and learning outcomes by comparing the experimental condition (co-regulation with an ALT) with a control group (only ALT, no co-regulation). Moreover, to gain further insight into students' goal-setting behavior in co-regulation, we examined how students set their goals and attained these goals in the co-regulation condition.
In line with hypotheses 1a and 1b, students in the co-regulation condition solved more problems and had a higher accuracy than the control condition. These results mirror findings from previous studies, which also found improvement in the regulation of practice behavior when students receive monitoring support when learning with ALTs (Molenaar et al., 2020, 2019b). The results showed that students in the co-regulation condition were more effective in regulating their practice behavior in terms of the number of items practiced and the accuracy of their answers compared to the students in the control condition. This means that engaging in co-regulation is potentially a good way to gradually shift from external to internal control, to support monitoring and control during practice in an ALT (Molenaar, 2022a; Azevedo et al., 2008; Dignath and Büttner, 2018; Panadero and Järvelä, 2015).
In both conditions, the number of problems solved was lower for the intermediate learning topic than for the easy and hard topics. This contrasts previous research that showed a negative relationship between difficulty level and the number of solved problems (Jansen et al., 2013, 2016). This could result in students needing more time to solve the problems at this difficulty level, possibly because the tasks required more steps, which they had to familiarize themselves with during the second lesson. This extra problem-solving step was also required in solving problems in the hard learning topic, which was addressed in the third lesson. Students possibly already had familiarized themselves with this extra step, which could have resulted in a higher pace for the hard learning topic. Future research could investigate whether other learning topics show similar results.
In contrast to hypothesis 2, similar learning gains were found in both conditions on the easy and intermediate learning topics. In addition, on the hard learning topic students in the control condition showed higher learning gain compared to students in the experimental condition. These findings are not in line with earlier findings in which higher learning gain for the group working with the learning path app was found (Molenaar et al., 2020, 2019b). Possibly, the adjustments in the learning path app for this study could influence this result. In addition, as students used the co-regulation intervention for the first time, this may caused a higher cognitive load placing a higher demand on students' cognitive capacity (Skulmowski and Xu, 2022). In addition to this, the problems of the hard learning topic probably caused more cognitive load compared to easier tasks (de Jong, 2010; Sweller, 1988). Hence, the co-regulation intervention combined with more difficult tasks could have led to cognitive overload (Howard et al., 2020; Sweller, 1988). The expectation is that when students become more experienced in engaging in co-regulation, the cognitive demands will decrease, resulting in more cognitive capacity for (self-regulated) learning (Skulmowski and Xu, 2022). Future research could investigate the cognitive demands of a co-regulation intervention and how this could diminish over time considering the effects on self-regulated learning. Even though the co-regulation intervention does not lead to more learning gains than the control condition, we did see a positive influence on students' regulation of practice behavior. As earlier studies showed that changes in the regulation of practice behavior can influence learning (Cheng, 2011; Molenaar et al., 2019b), the co-regulation intervention shows potential to affect goal-setting behavior.
Lastly, we explored students' goal-setting behavior in the co-regulation condition more in-depth. We examined how the students in the co-regulation condition set and attained their goals.
Firstly, students set higher week goals than day goals on all three learning topics and set similar goals between the learning topics. This is comparable with general primary school practices, as students are expected to learn more over time (Aubrey et al., 2006). The fact that students set similar goals between the learning topics could be the result of them perceiving the learning topics as equally difficult (Gerhardt and Luzadis, 2009). Moreover, students' prior experiences could cause them to evaluate their overall knowledge as high, resulting in them setting similar goals for all tasks (Hinsz and Matz, 1997). We think it is most likely that students set similar goals on all three learning topics because of their familiarity with receiving personalized learning materials (ALTs). Within the context of ALTs, students commonly encounter problems adjusted to their needs (Klinkenberg et al., 2011), thereby diminishing the need for adjustment of their goals (Molenaar et al., 2019b). This could have impacted their goal-setting behavior in this experiment, as they did not adjust their goals when the learning topic changed.
Secondly, students' goal attainment was less for the easy learning topic compared to the hard learning topic. They underestimated their performance at the easy and intermediate learning topics, which turned into overestimation at the hard learning topic. The underestimation contradicts van Loon et al. (2013) who showed that young students often overestimate their performance. Despite the increasing difficulty of the learning topics, students did not adjust their goal setting downwards, contradicting previous research that found the opposite (Ilies and Judge, 2005). This could result from the type of problems that students are working on in this study. Even though students turned from underestimating to overestimating when learning topics increased in difficulty, the gap between their set goal and their ability level after the lesson became smaller. This means they did set their goals closer to their actual ability level: they became more accurate in setting their goals. This could suggest that they did account for the difficulty of the tasks when setting goals. This is in line with previous research indicating that students' alignment between the set and attained goals increases with difficulty of the learning topics (Molenaar et al., 2019b). This also indicates that the co-regulation intervention benefits students' goal-setting behavior by helping them to set more calibrated goals.
Some limitations and suggestions for future research can be put forward. First, the number of participants in this study was low, leading to a power issue, which could have caused both conditions to have similar learning outcomes. Future research should focus on getting a bigger sample size, which will circumvent the power issue. Students in the co-regulation condition already had higher scores on the pre-test on all topics, resulting in limited learning possibilities on all topics compared to the control group, possibly causing the similarities in learning outcomes between the conditions. As future research already should include more participants, this could consequently also solve the prior-knowledge imbalance between the conditions due to random assignment.
There was a gender and age imbalance between the conditions as well. For gender, it is not clear how the imbalance might have influenced the outcomes, since research on gender differences in math showed mixed findings (Hamhuis et al., 2020; van den Heuvel-Panhuizen, 2004; van de Weijer-Bergsma et al., 2022). However, balancing gender distribution between the conditions in future research will mitigate possible influences. Relative age effects are found to have an impact on math performance, younger students were found to score lower on standardized math tests than their older classmates (Mavilidi et al., 2022). In this study we found the opposite, students in the control group were on average older than the students in the experimental group, however the control group was found to score lower on the pre-test. How the age difference might have influenced the results therefore is not clear. However, balancing the age between condition in future research again might mitigate possible influences.
A larger sample size would also allow for including different learning topics and possibly even matching on prior knowledge between the conditions. In this study, differences in prior knowledge between the conditions as well as the choice of topics could have influenced the results. The combination of the co-regulation intervention with the problems of the hard learning topic could have caused high cognitive load, diminishing the effects on regulation of practice behavior and learning outcomes (Seufert, 2018). In future research it is therefore important to investigate the impact of co-regulation on cognitive load. This would contribute to more insights into the effects of co-regulation on regulation of practice behavior and learning outcomes and how they come about.
The finding that a lower number of problems was solved for the intermediate learning topic in both conditions could be an indication of this topic to be harder than we initially thought. In future research, it is therefore necessary to examine the difficulty of the learning topics again and make sure that there is an increase in difficulty from easy via intermediate to hard.
Furthermore, the co-regulation intervention only lasted for a few days, yet students might need more time to get fully acquainted with the co-regulation intervention and procedure. This could be one of the explanations for our finding that the learning gain was similar between conditions. That is, possible effects on learning outcomes may occur after a more prolonged exposure to the co-regulation intervention, since students need time to get familiar with the intervention. In the beginning the intervention might have an impact on the cognitive capacity left to focus on the math topics (Skulmowski and Xu, 2022). Therefore, future research should investigate the long-term effects of this co-regulation intervention over time on students' practice behavior, learning outcomes and goal-setting behavior.
Based on the results found in the current study, it seems that providing students with monitoring opportunities and supporting them to set goals, could help students to take control over their own learning, resulting in more effective regulation of practice behavior. It presumably provided them with the opportunity to make their self-regulation more explicit and concrete, by setting their own learning goal, seeing their learning progress, and consequently monitoring and controlling their learning. An implication of these findings could be that it is promising for developers of ALTs to consider including co-regulation options including goal-setting and monitoring support in their systems. Not only for developers of ALTs, but for educational specialists in general, supporting students using co-regulation could be beneficial. For example, co-regulation led by teachers is potentially beneficial for students. When teachers take an expert role on SRL and engage students in different challenges around the topic, regulation processes can be triggered in the students themselves (Baars et al., 2020; Hadwin et al., 2017). Teachers could, for example, provide students with more explicit goal-setting opportunities before they start practicing with problems in the ALT. Future research could further investigate the effect of co-regulation interventions provided by ALTs or teachers on students' practice behavior and learning outcomes in educational practice. Another interesting future line of research would be to explore the support of co-regulation in the transition from external to internal control of SRL for students and, consequently, the impact on the development of their SRL skills.
The findings of this study expand our understanding of how students can be supported in developing their goal setting and monitoring. Combined, our results showed that co-regulation with an ALT positively affects students' regulation of practice behavior and goal-setting behavior, yet no effects on learning outcomes were found. Co-regulation with an ALT helps students to be more conscious of their progress and set more realistic goals. More research is needed to understand the relationship with learning outcomes. Overall, co-regulation with ALTs has the potential to support the gradual shift from external to internal control in SRL.
Data available on request from the authors.
This study involved humans and was approved by the Ethics Committee Faculty of Social Sciences, Radboud University. In this study, written passive informed consent of parents/legal guardians was provided which is in accordance with the national legislation and the institutional requirements.
AH: Conceptualization, Data curation, Formal analysis, Investigation, Methodology, Project administration, Writing – original draft, Writing – review & editing. RK: Conceptualization, Writing – review & editing. CK-vC: Conceptualization, Writing – review & editing. RD: Methodology, Software, Writing – review & editing. MB: Conceptualization, Writing – review & editing. IM: Conceptualization, Funding acquisition, Methodology, Writing – review & editing.
The authors declare financial support was received for the research, authorship, and/or publication of this article. This research was funded by a VENI grant awarded to Inge Molenaar by the Netherlands Organization for Scientific Research [project # 451-16-017, the European Research Council grant (HHAIR-948786)] and the National Education Lab Artificial Intelligence funded by the Dutch National Growth Fund.
The authors declare that the research was conducted in the absence of any commercial or financial relationships that could be construed as a potential conflict of interest.
All claims expressed in this article are solely those of the authors and do not necessarily represent those of their affiliated organizations, or those of the publisher, the editors and the reviewers. Any product that may be evaluated in this article, or claim that may be made by its manufacturer, is not guaranteed or endorsed by the publisher.
Aleven, V., McLaughlin, E. A., Glenn, R. A., and Koedinger, K. R. (2016). “Instruction based on adaptive learning technologies,” in Handbook of research on learning and instruction, eds. R. E. Mayer and P. Alexander (London: Routledge), 522–560.
Arroyo, I., Ferguson, K., Johns, J., Dragon, T., Meheranian, H., Fisher, D., et al. (2007). Repairing disengagement with non-invasive interventions. AIED 2007, 195–202.
Aubrey, C., Godfrey, R., and Dahl, S. (2006). Early mathematics development and later achievement: further evidence. Mathem. Educ. Res. J. 18, 27–46. doi: 10.1007/BF03217428
Azevedo, R., Moos, D. C., Greene, J. A., Winters, F. I., and Cromley, J. G. (2008). Why is externally-facilitated regulated learning more effective than self-regulated learning with hypermedia? Educ. Technol. Res. Dev. 56, 45–72. doi: 10.1007/s11423-007-9067-0
Baars, M., Wijnia, L., Bruin, A., and de, Paas, F. (2020). “Sharing the load: a strategy to improve self-regulated learning,” in Handbook of strategies and strategic processing, eds. D. L. Dinsmore, L. K. Fryer, and M. M. Parkinson (London: Routledge). doi: 10.4324/9780429423635-14
Bodily, R., Ikahihifo, T. K., Mackley, B., and Graham, C. R. (2018a). The design, development, and implementation of student-facing learning analytics dashboards. J. Comput. High. Educ. 30, 572–598. doi: 10.1007/s12528-018-9186-0
Bodily, R., Kay, J., Aleven, V., Jivet, I., Davis, D., Xhakaj, F., et al. (2018b). “Open learner models and learning analytics dashboards: a systematic review,” in Proceedings of the 8th International Conference on Learning Analytics and Knowledge, 41–50. doi: 10.1145/3170358.3170409
Boekaerts, M. (1999). Self-regulated learning: where we are today. Int. J. Educ. Res. 31, 445–457. doi: 10.1016/S0883-0355(99)00014-2
Cheng, C. K. E. (2011). The role of self-regulated learning in enhancing learning performance. Int. J. Res. Rev. 6:1.
Corbett, A. T., and Anderson, J. R. (1994). Knowledge tracing: modeling the acquisition of procedural knowledge. User Model. User-Adapt. Interact. 4, 253–278. doi: 10.1007/BF01099821
De Bruin, A. B. H., Dunlosky, J., and Cavalcanti, R. B. (2017). Monitoring and regulation of learning in medical education: the need for predictive cues. Med. Educ. 51, 575–584. doi: 10.1111/medu.13267
de Jong, T. (2010). Cognitive load theory, educational research, and instructional design: some food for thought. Instruct. Sci. 38, 105–134. doi: 10.1007/s11251-009-9110-0
Dignath, C., and Büttner, G. (2008). Components of fostering self-regulated learning among students. A meta-analysis on intervention studies at primary and secondary school level. Metacogn. Learn. 3, 231–264. doi: 10.1007/s11409-008-9029-x
Dignath, C., and Büttner, G. (2018). Teachers' direct and indirect promotion of self-regulated learning in primary and secondary school mathematics classes – insights from video-based classroom observations and teacher interviews. Metacogn. Lear. 13, 127–157. doi: 10.1007/s11409-018-9181-x
Ewijk, C. D., Dickhäuser, O., and Büttner, G. (2013). Assessing how teachers enhance self-regulated learning: a multiperspective approach. J. Cogn. Educ. Psychol. 12, 338–358. doi: 10.1891/1945-8959.12.3.338
Gardner, A. K., Diesen, D. L., Hogg, D., and Huerta, S. (2016). The impact of goal setting and goal orientation on performance during a clerkship surgical skills training program. Am. J. Surg. 211, 321–325. doi: 10.1016/j.amjsurg.2015.07.028
Gerhardt, M. W., and Luzadis, R. A. (2009). The importance of perceived task difficulty in goal orientation—assigned goal alignment. J. Lead. Organ. Stud. 16, 167–174. doi: 10.1177/1548051809337875
Greene, J. A., and Azevedo, R. (2007). A theoretical review of winne and hadwin's model of self-regulated learning: new perspectives and directions. Rev. Educ. Res. 77, 334–372. doi: 10.3102/003465430303953
Hadwin, A., Järvelä, S., and Miller, M. (2017). “Self-regulation, co-regulation, and shared regulation in collaborative learning environments,” in Handbook of self-regulation of learning and performance, eds. D. H. Schunk and J. A. Greene (London: Routledge), 83–106. doi: 10.4324/9781315697048-6
Hamhuis, E., Glas, C., and Meelissen, M. (2020). Tablet assessment in primary education: are there performance differences between TIMSS' paper-and-pencil test and tablet test among Dutch grade-four students? Br. J. Educ. Technol. 51, 2340–2358. doi: 10.1111/bjet.12914
Hinsz, V. B., and Matz, D. C. (1997). Self-evaluations involved in goal setting and task performance. Soc. Behav. Person. 25, 177–181. doi: 10.2224/sbp.1997.25.2.177
Howard, Z. L., Evans, N. J., Innes, R. J., Brown, S. D., and Eidels, A. (2020). How is multi-tasking different from increased difficulty? Psychon. Bull. Rev. 27, 937–951. doi: 10.3758/s13423-020-01741-8
Ilies, R., and Judge, T. (2005). Goal regulation across time: the effects of feedback and affect. J. Appl. Psychol. 90, 453–467. doi: 10.1037/0021-9010.90.3.453
Jansen, B. R. J., Hofman, A. D., Savi, A., Visser, I., and van der Maas, H. L. J. (2016). Self-adapting the success rate when practicing math. Learn. Individ. Differ. 51, 1–10. doi: 10.1016/j.lindif.2016.08.027
Jansen, B. R. J., Louwerse, J., Straatemeier, M., Van der Ven, S. H. G., Klinkenberg, S., and Van der Maas, H. L. J. (2013). The influence of experiencing success in math on math anxiety, perceived math competence, and math performance. Learn. Individ. Differ. 24, 190–197. doi: 10.1016/j.lindif.2012.12.014
Järvelä, S., and Bannert, M. (2019). Temporal and adaptive processes of regulated learning—What can multimodal data tell? Lear. Instr. 72:101268. doi: 10.1016/j.learninstruc.2019.101268
Jivet, I., Scheffel, M., Specht, M., and Drachsler, H. (2018). “License to evaluate: Preparing learning analytics dashboards for educational practice,” in Proceedings of the 8th International Conference on Learning Analytics and Knowledge, 31–40. doi: 10.1145/3170358.3170421
Karssen, M., Krepel, A., Stronkhorst, E., Lourens, J. M. P., Bruck, S., van Kessel, M., et al. (2023). Monitor digitalisering funderend onderwijs: Onderzoeksrapport primair onderwijs MYRA 2023. Kohnstamm Instituut.
Klinkenberg, S., Straatemeier, M., and van der Maas, H. L. J. (2011). Computer adaptive practice of Maths ability using a new item response model for on the fly ability and difficulty estimation. Comput. Educ. 57, 1813–1824. doi: 10.1016/j.compedu.2011.02.003
Koriat, A. (1997). Monitoring one's own knowledge during study: a cue-utilization approach to judgments of learning. J. Exper. Psychol. 126, 349–370. doi: 10.1037/0096-3445.126.4.349
Lajoie, S. P., and Lu, J. (2012). Supporting collaboration with technology: does shared cognition lead to co-regulation in medicine? Metacogn. Lear. 7, 45–62. doi: 10.1007/s11409-011-9077-5
Locke, E. A., and Latham, G. P. (1990). A Theory of Goal Setting and Task Performance. New Jersey: Prentice-Hall, Inc.
Mavilidi, M. F., Marsh, H. W., Xu, K. M., Parker, P. D., Jansen, P. W., and Paas, F. (2022). Relative age effects on academic achievement in the first ten years of formal schooling: a nationally representative longitudinal prospective study. J. Educ. Psychol. 114:308. doi: 10.1037/edu0000681
McCaslin, M. (2004). Coregulation of opportunity, activity, and identity in student motivation: elaborations on Vygotskian themes. Big Theories Revisited 4, 249–274.
Moeller, A. J., Theiler, J. M., and Wu, C. (2012). Goal setting and student achievement: a longitudinal study. Modern Lang. J. 96, 153–169. doi: 10.1111/j.1540-4781.2011.01231.x
Molenaar, I. (2022a). The concept of hybrid human-AI regulation: exemplifying how to support young learners' self-regulated learning. Comput. Educ. Artif. Intellig. 3:100070. doi: 10.1016/j.caeai.2022.100070
Molenaar, I. (2022b). Towards hybrid human-AI learning technologies. Eur. J. Educ. 57, 632–645. doi: 10.1111/ejed.12527
Molenaar, I., Horvers, A., and Baker, R. S. (2019a). “Towards hybrid human-system regulation: understanding children' SRL support needs in blended classrooms,” in Proceedings of the 9th International Conference on Learning Analytics & Knowledge, 471–480. doi: 10.1145/3303772.3303780
Molenaar, I., Horvers, A., and Baker, R. S. (2021). What can moment-by-moment learning curves tell about students' self-regulated learning? Learn. Instruct. 72:101206. doi: 10.1016/j.learninstruc.2019.05.003
Molenaar, I., Horvers, A., and Dijkstra, R. (2019b). Young learners' regulation of practice behavior in adaptive learning technologies. Front. Psychol. 10:2792. doi: 10.3389/fpsyg.2019.02792
Molenaar, I., Horvers, A., Dijkstra, R., and Baker, R. S. (2020). “Personalized visualizations to promote young learners' SRL: the learning path app,” in Proceedings of the Tenth International Conference on Learning Analytics & Knowledge, 330–339. doi: 10.1145/3375462.3375465
Morgan, M. (1985). Self-monitoring of attained subgoals in private study. J. Educ. Psychol. 77, 623–630. doi: 10.1037/0022-0663.77.6.623
Nedzinskaite-Mačiuniene, R., and Šimiene, G. (2021). “A strategic and goal-directed student: expectations vs. reality,” in Improving Inclusive Education through Universal Design for Learning, 187–215. doi: 10.1007/978-3-030-80658-3_8
Panadero, E. (2017). A review of self-regulated learning: six models and four directions for research. Front. Psychol. 8:422. doi: 10.3389/fpsyg.2017.00422
Panadero, E., and Järvelä, S. (2015). Socially shared regulation of learning: a review. Eur. Psychol. 20, 190–203. doi: 10.1027/1016-9040/a000226
Pardo, A., Jovanovic, J., Dawson, S., Gašević, D., and Mirriahi, N. (2019). Using learning analytics to scale the provision of personalised feedback. Br. J. Educ. Technol. 50, 128–138. doi: 10.1111/bjet.12592
Schunk, D. H. (1990). Goal setting and self-efficacy during self-regulated learning. Educ. Psychol. 25, 71–86. doi: 10.1207/s15326985ep2501_6
Seufert, T. (2018). The interplay between self-regulation in learning and cognitive load. Educ. Res. Rev. 24, 116–129. doi: 10.1016/j.edurev.2018.03.004
Skulmowski, A., and Xu, K. M. (2022). Understanding cognitive load in digital and online learning: a new perspective on extraneous cognitive load. Educ. Psychol. Rev. 34, 171–196. doi: 10.1007/s10648-021-09624-7
Sweller, J. (1988). Cognitive load during problem solving: effects on learning. Cogn. Sci. 12, 257–285. doi: 10.1207/s15516709cog1202_4
Usher, E. L., and Schunk, D. H. (2017). “Social cognitive theoretical perspective of self-regulation,” in Handbook of self-regulation of learning and performance, eds. D. H. Schunk and J. A. Greene (London: Routledge). doi: 10.4324/9781315697048-2
van de Weijer-Bergsma, E., Van Luit, J. E., and Moeller, K. (2022). Sex differences in the association of math achievement with visual-spatial and verbal working memory: does the type of math test matter? Br. J. Psychol. 113, 798–819. doi: 10.1111/bjop.12562
van den Heuvel-Panhuizen, M. H. A. M. (2004). “Girls' and boys' problems: gender differences in solving problems in primary school mathematics in the Netherlands,” in International perspectives on learning and teaching mathematics, eds. B. Clarke, D. M. Clarke, G. Emanuelsson, B. Johansson, D. V. Lambdin, F. K. Lester, et al. (NCM), 237–252.
van Loon, M. H., De Bruin, A. B. H., Van Gog, T., and Van Merriënboer, J. J. G. (2013). The effect of delayed-JOLs and sentence generation on children's monitoring accuracy and regulation of idiom study. Metacogn. Lear. 8, 173–191. doi: 10.1007/s11409-013-9100-0
van Loon, M. H., and Roebers, C. M. (2021). “Using feedback to support children when monitoring and controlling their learning,” in Trends and Prospects in Metacognition Research across the Life Span: A Tribute to Anastasia Efklides, D. Moraitou and P. Metallidou (Cham: Springer International Publishing), 161–184. doi: 10.1007/978-3-030-51673-4_8
van Merriënboer, J. J. G., and de Bruin, A. B. H. (2019). Cue-based facilitation of self-regulated learning: a discussion of multidisciplinary innovations and technologies. Comput. Human Behav. 100, 384–391. doi: 10.1016/j.chb.2019.07.021
VanLehn, K. (2011). The relative effectiveness of human tutoring, intelligent tutoring systems, and other tutoring systems. Educ. Psychol. 46, 197–221. doi: 10.1080/00461520.2011.611369
Veenman, M. V. J. (2013). “Assessing metacognitive skills in computerized learning environments,” in International Handbook of Metacognitive Learning Technologies, eds. R. Azevedo and V. Aleven (Cham: Springer), 157–168. doi: 10.1007/978-1-4419-5546-3_11
Winne, P. H. (2010). Improving measurements of self-regulated learning. Educ. Psychol. 45, 267–276. doi: 10.1080/00461520.2010.517150
Winne, P. H. (2017). “Cognition and metacognition within self-regulated learning,” in Handbook of Self-Regulation of Learning and Performance, eds. D. H. Schunk and J. A. Greene (London: Routledge). doi: 10.4324/9781315697048-3
Winne, P. H., and Hadwin, A. F. (1998). “Studying as self-regulated learning,” in Metacognition in educational theory and practice, eds. D. J. Hacker, J. Dunlosky, and A. Graesser (New Jersey: Lawrence Erlbaum Associates Publishers), 277–304.
Winne, P. H., and Hadwin, A. F. (2013). “nStudy: tracing and supporting self-regulated learning in the internet,” in International Handbook of Metacognitive Learning Technologies, eds. R. Azevedo and V. Aleven (Cham: Springer), 293–308. doi: 10.1007/978-1-4419-5546-3_20
Wong, J., Baars, M., Davis, D., Van Der Zee, T., Houben, G.-J., and Paas, F. (2019). Supporting self-regulated learning in online learning environments and MOOCs: a systematic review. Int. J. Hum. Comput. Inter. 35, 356–373. doi: 10.1080/10447318.2018.1543084
Keywords: co-regulation, adaptive learning technologies, self-regulated learning, primary education, goal-setting prompts, monitoring support
Citation: Horvers A, Kooi R, Knoop-van Campen CAN, Dijkstra R, Baars M and Molenaar I (2024) How does co-regulation with Adaptive Learning Technologies affect primary school students' goal-setting, regulation of practice behavior and learning outcomes? Front. Educ. 9:1435483. doi: 10.3389/feduc.2024.1435483
Received: 20 May 2024; Accepted: 05 November 2024;
Published: 17 December 2024.
Edited by:
Shirley Miedijensky, Oranim Academic College, IsraelCopyright © 2024 Horvers, Kooi, Knoop-van Campen, Dijkstra, Baars and Molenaar. This is an open-access article distributed under the terms of the Creative Commons Attribution License (CC BY). The use, distribution or reproduction in other forums is permitted, provided the original author(s) and the copyright owner(s) are credited and that the original publication in this journal is cited, in accordance with accepted academic practice. No use, distribution or reproduction is permitted which does not comply with these terms.
*Correspondence: Anne Horvers, YW5uZS5ob3J2ZXJzQHJ1Lm5s
Disclaimer: All claims expressed in this article are solely those of the authors and do not necessarily represent those of their affiliated organizations, or those of the publisher, the editors and the reviewers. Any product that may be evaluated in this article or claim that may be made by its manufacturer is not guaranteed or endorsed by the publisher.
Research integrity at Frontiers
Learn more about the work of our research integrity team to safeguard the quality of each article we publish.