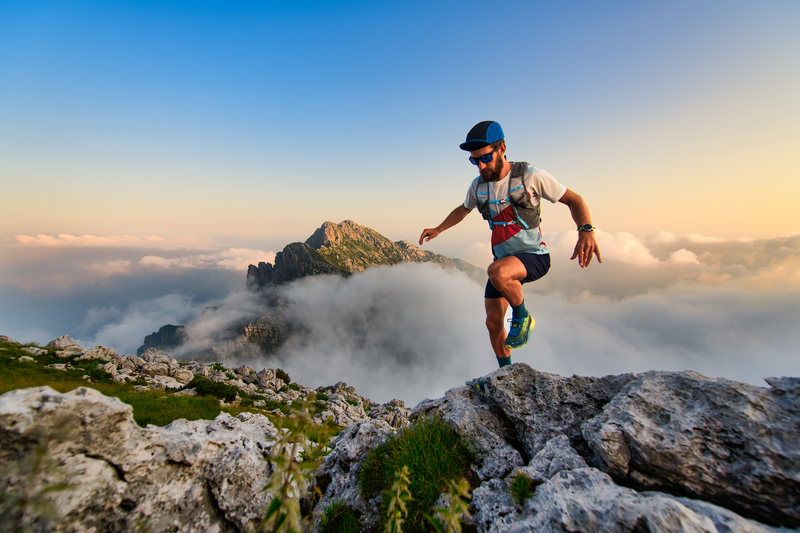
95% of researchers rate our articles as excellent or good
Learn more about the work of our research integrity team to safeguard the quality of each article we publish.
Find out more
ORIGINAL RESEARCH article
Front. Educ. , 21 November 2024
Sec. Higher Education
Volume 9 - 2024 | https://doi.org/10.3389/feduc.2024.1433021
Studying the factors influencing the academic performance of higher education students has been a major focus of attention for research scholars across the world. However, empirical research literature specifically addressing this topic in the context of Chinese higher vocational colleges remains limited. This study aims to construct a novel indicator that hints at the academic performance of Chinese college students. Using approximately 2,270 records of students majoring in economics and management from the 2016 to 2020 academic years, this comprehensive study analyzed various aspects of their academic and extracurricular performance, encompassing 33 variables. These variables ranged from fundamental demographic characteristics to essential academic metrics like Gaokao Scores (GKs), College English Test 4 (CET4), academic achievements, participation and outcomes in skill competitions, student honors received, challenges faced, and involvement in volunteer services. This study found that (1) GKs, CET4, National Computer Rank Examination (NCRE), Total Scholarship (TSS), Outstanding Student (OS), Outstanding Student Leader (OSL), General Difficulty Student (GDS), and Volunteer Service Hours (VSHs) all show a significant positive correlation with academic performance and (2) age was negatively correlated with academic performance. In addition, the study also found that the effect of volunteer service on academic performance showed a folded relationship between “valley” and “peak.” These findings provide a source of ideas and empirical references for college education administrators and educational policymakers when revising or developing policy documents.
Every year, millions of young students graduate from school, which provides a constant supply of labor for the high-quality and rapid development of human society, especially those who have higher education or possess a skill have become the focus of society and the important human resources every industry competes to hire (Berger and Fisher, 2013). Students are regarded as the internal driving force for the rapid growth of the economy (Romer, 1990). Such high expectations of this group or human resources arise from their education, which is seen to play an important role in improving the productivity of enterprises, increasing the wellbeing of society, and enhancing the survival skills and living standards of individuals. It is well known that well-educated groups will have the opportunity to earn higher incomes, better working conditions, and more room for development than those without good education or the less educated (Berger and Fisher, 2013). However, acquiring knowledge and skills through education is a difficult and lengthy process full of opportunities and challenges, achievements, and hardships. Therefore, quality educational resources are a necessity and a prerequisite for producing a workforce that meets the needs of economic development and is suitable for social development (Owings et al., 2012).
Schools attach great importance to the quality of education and are very concerned about students’ academic performance and the quality of student training (Chen et al., 2024). By formulating policies on incentives, organizing skill competitions, and holding different types of lectures and salon activities, they guide students to make good career choices and help them improve their academic performance and employability skills and enhance their graduation strength. The majority of the previous empirical research that has been done indicates that there are many factors affecting students’ academic performance, including individual and external factors. Individual factors include gender (Abbey and Adu-Danso, 2022; Alghamdi et al., 2020; Atas, 2022; Burns et al., 2008; Cheng and Nguyen, 2023; Ibarra-Vazquez et al., 2023), ethnicity (Van Es and Weaver, 2018), age (Alhajraf and Alasfour, 2014; Guney, 2009; Kim et al., 2023), and personal characteristics (Akimov et al., 2024), whereas extrinsic factors include the school’s level of operation, level of education, social status, and the level of teachers’ teaching. In addition, there are other influencing factors such as English proficiency, computer (Simoes et al., 2022), parents’ educational background (Aktaç et al., 2019), family economic status (Chui et al., 2020; Gao et al., 2021; Gil et al., 2022), part-time work (Beauchamp et al., 2016), high school entrance exam scores (Akimov et al., 2024), academic engagement (Jawad et al., 2022; Luo et al., 2023; Rao, 2020), efforts for study (Sothan, 2019), and class attendance (Akimov et al., 2024; Sothan, 2019). In other cases, there are research scholars who focus on student characteristics (Beauchamp et al., 2016), playing video games (Savić Tot et al., 2023), peer group stability (Herbst et al., 2023), learning stress (Muhonen et al., 2023; Pascoe et al., 2020; Zheng et al., 2023), study strategies (Bergey et al., 2019), academic self-efficacy (Alghamdi et al., 2020; Alhadabi and Karpinski, 2020; Bergey et al., 2019), living arrangement (Forster and Reuter, 2022), course load (Huntington-Klein and Gill, 2021; Phipps and Amaya, 2023), academic stress and lifestyle factors (Han et al., 2024), college teachers’ frustration tolerance, and academic performance (Shi et al., 2021). Of course, student performance is also related to the quality of experimental data collection, assessment methods, and technological tools.
Although there have been previous empirical studies on the factors influencing academic performance, these studies are not without limitations. Differences in estimation techniques, data measurements, and data quality across studies have led to a lack of consensus regarding the impact on college academic performance, especially within the context of Chinese college students. Educational systems vary among different countries, with each country’s schools having their own distinct talent cultivation modes and paths. In China, while some researchers have explored certain factors influencing higher vocational college students’ academic performance (Han et al., 2024; Zhai et al., 2022), aspects such as the relationship between skill competitions or volunteer service and academic performance have received relatively less attention. However, studies outside of China have indicated the potential impact of extracurricular activities on academic achievement. For example, participation in skills competitions has been linked to improved problem-solving abilities, teamwork, and time management, all of which can positively affect academic performance (Anjum, 2021). Similarly, volunteer service is often associated with higher levels of civic engagement, social responsibility, and the development of soft skills, which are crucial for success in both academic and professional settings (Astin and Sax, 1998).
Moreover, evaluation criteria for student academic performance and the data used in research studies can vary. For example, research by Han et al. (2024) and Zhai et al. (2022) focus on different indicators of academic success, including grade point averages (GPA), learning attitudes, and participation in academic activities, leading to varying outcomes. This suggests that empirical research results from other educational contexts, such as Western countries, may not always apply to Chinese vocational colleges without careful adaptation. This implies that directly applying empirical research results that do not align with the actual situation of Chinese college education may pose potential risks to Chinese colleges. Given this background, the specific goal of this study is to enrich and validate the factors that influence students’ performance in Chinese colleges. By constructing a novel indicator for the academic performance of Chinese college students based on stepwise regression technique and correlation analysis, this study aims to explore the influencing factors and determine their specific coefficients. Through this research, we hope to bridge the gaps found in the existing literature and provide valuable ideas and empirical evidence for educational administrators and policymakers in China. This study not only contributes to a more comprehensive understanding of the factors influencing Chinese college student’s academic performance but also offers practical implications for educational policymakers in the Chinese higher education context.
Some studies present their findings based on the analysis of empirical literature on the factors influencing student performance internationally, both in primary and tertiary education. Generally speaking, literature studies have addressed various factors that affect student performance. For example, academic stress, living arrangements, student characteristics, self-efficacy, learning strategies, peer guidance, and even physical education were also addressed by some researchers.
The results revealed that basic demographic attributes such as age, gender, and ethnicity have significant effects on academic performance, but the findings are often mixed or contradictory. For example, some studies show that younger students perform better than older students (Da Wan and Cheo, 2012). However, other researchers have found that older students perform better than younger students (Alhajraf and Alasfour, 2014; Guney, 2009; Kim et al., 2023). In addition, the effect of gender as one of the basic demographic attributes on students’ performance has also been tested (Kaighobadi and Allen, 2008). Studies on the effect of gender in statistics courses have yielded mixed results (Haley et al., 2007; Scheaffer and Stasny, 2004). Some studies show that female students outperform male students in certain courses. For example, Johnson and Kuennen (2006) found this in statistics courses, and Orlov and Roufagalas (2012) and Alfan and Othman (2005) in economics courses where female students outperformed male students by about 3%. Kaighobadi and Allen’s (2008) empirical studies have shown a gap between male and female students’ academic performance, with female students outperforming male students in course works, language learning, and various exams. These studies show a gap between male and female students’ academic performance. Female students outperform male students in coursework, language learning, and various exams. This contrasts with the belief of some that male students perform much better than female students (Alyoussef et al., 2016). Regarding ethnicity attributes facilitation, Van Es and Weaver (2018) found that students’ ethnicity played a significant role in their performance, expectations, and learning styles. Asian students outperformed all other groups in both meeting personal expectations and achieving significantly higher final grades.
Students’ Gaokao Scores (entrance examination scores), computer proficiency, English language proficiency, and grade point average (GPA) were usually found to be positively correlated with academic performance (Asarta and Schmidt, 2017; Alturki et al., 2022). Gaokao Scores were always considered one of the main variables predicting the students’ academic performance in higher education. For instance, Cyrenne and Chan (2012) found high school grades were a significant predictor of academic performance using least squares dummy variable models and hierarchical linear models. Danilowicz-Gösele et al. (2017) examined the determinants of student success in German universities and found that high school grades were highly correlated with the probability of graduation and final grades. Asarta and Schmidt (2017) confirmed that GPA was the strongest determinant of performance in statistics courses. In addition, Simoes et al. (2022) surveyed 286 students aged 16–18 years old through a questionnaire, and their study found that computer use positively affected students’ academic performance, and also found that enjoyment attitudes, school environment, interest motivation, and loneliness negatively affected academic performance. Hovdhaugen (2013) found that students from non-English speaking backgrounds performed poorly in two of the investigated courses.
Students engaging in playing games, video games, and after-school part-time jobs have also been studied as influencing academic performance. The study results of Savić Tot et al. (2023) showed that students who played more games may have slightly lower grades than those who did not play games. Meanwhile, the research results showed a statistically insignificant correlation between perceived GPA and time spent on all fun activities. Similar findings were found in the study by Zhang et al. (2021). Johnson and Kuennen (2006) observed that grades in statistics courses declined as the amount of time spent working outside of the classroom increased. Salamonson and Andrew (2006) showed that working more than 16 h per week had a negative impact on the academic performance of Australian nursing students. These studies suggest that both excessive gaming and a significant amount of part-time work can potentially have a negative impact on academic performance. However, more research is needed to understand the underlying mechanisms and to determine the optimal balance between leisure activities and work, on the one hand, and academic pursuits, on the other.
In another research, Rochford et al. (2009) found that students who worked longer hours reported negative outcomes. Greenbank et al. (2009) argued that part-time extracurricular work limited students’ participation in a variety of extracurricular activities, including group activities and career planning and preparation. Not surprisingly, in addition to its impact on academic performance, part-time extracurricular work may also contribute to high dropout rates during college. For instance, Hovdhaugen (2013) revealed that students who work full-time during their full-time studies were less likely to complete their studies than those who work part-time or not at all. These findings suggest that part-time extracurricular work needs to be carefully managed to ensure it does not have a detrimental impact on student’s academic progress and completion of studies.
In addition to the above-mentioned factors affecting academic performance, within the context of Chinese current vocational education focusing on enhancing students’ skills and cultivating the spirit of craftsmanship, this study also examines the relationship between students’ participation in vocational skills competitions, volunteer service, leadership roles, and their academic performance. Existing research highlights that participation in skill competitions not only improves students’ technical abilities but also enhances their problem-solving skills and teamwork, which are essential for academic success (Liang et al., 2023). Similarly, students who engage in volunteer service tend to develop soft skills such as communication, empathy, and social responsibility, all of which positively influence their academic achievements (Astin and Sax, 1998). Furthermore, leadership roles, such as being an outstanding student leader, have been linked to improved time management, self-discipline, and academic performance (Kuh, 2009). This study reflects the characteristics of higher vocational education in China and aligns with societal demands for improving the employability and employment quality of vocational college students. By focusing on the intersection of vocational skills training and extracurricular involvement, the research deepens the theoretical understanding of the factors influencing academic performance.
The study was based on student academic performance data from a Chinese college. It aimed to investigate the factors impacting academic performance and construct a novel indicator. This indicator is designed to indicate the students’ academic performance. In this study, we utilized stepwise regression analysis and correlation analysis to examine the existence and magnitude of the effect of the independent variables on the OGPA. The flow chart of this study is shown in Figure 1.
To clearly understand the academic performance of students in Chinese vocational colleges, 2,270 students’ historical data were collected from a vocational college in China for the 2016 to 2020 academic years. In the college chosen for this study, five departments appointed a teacher to supply related data for the research. Among the collected records, the participants in the 2016 academic year accounted for 29.4%, the 2017 academic year for 15.5%, the 2018 academic year for 19.8%, and the 2019 academic year for 35.5%. In addition, male students constituted 19.2% and female students 80.8% of the participants. The mean age was 19.02 (SD = 1.047,) with both the median and the mode being 20. The Han ethnicity students made up 72.2% and non--Han ethnicity students 27.8% of the study population. Due to their high school backgrounds, the participants taking part in the study had different major subjects as part of their study. The participants from the majors in literature and history (MLH) were 41.3% and those from the majors in science and engineering (MSE) were 48.7%. The participants who were communist youth league members accounted for 17.8%, and the others 82.2%. In the college where this study was performed, the participants came from different regions throughout China. The participants from rural regions were 62.7% and others from urban regions were 37.3%. In addition, the participants who were recognized as particularly difficult students (PDS) constituted 23.3%, and the others 76.7% of the study population. Those recognized as generally difficult students (GDS) were 29.4% and others 70.6% in this study. As shown in Table 1, a few of the participants who voluntarily joined volunteer services would get hours and participate in a variety of vocational skill competitions, which helped them obtain various honors.
In the present research, developing an Excel sheet for collecting data was the main instrument. The instrument was primarily used to gather student data regarding demographics, academic performance, skill competitions, student difficulty status, and volunteer services that were hypothesized to be the impact factors for students’ academic performance. So, for gathering the related data in response to the hypothesized research object in the research, the Excel sheet was divided into three parts. Part 1 covered the demographic profiles [Student ID (SID), entrance year, gender, ethnicity, age, Communist Youth League Member (CYLM), profession name (PN), discipline type (DT), and regional type (RT)]. Part 2 covered academic performance [Gaokao scores (GKs), College English Test Band 4 (CET4), College English Test Band 6 (CET6), National Computer Rank Examination (NCRE), First Semester GPA (S1GPA), Second Semester GPA (S2GPA), Third Semester GPA (S3GPA), Fourth Semester GPA (S4GPA), Fifth Semester GPA (S5GPA), Sixth Semester GPA (S6GPA), Overall Semester GPA (OGPA)]. Part 3 covered the extracurricular activities of the participants [School Skills Competitions (SSC), School Skill Rewards (SSRs), Province Skills Competitions (PSC), Province Skill Rewards (PSRs), Country Skills Competitions (CSC), Country Skill Rewards (CSRs), Total Scholarship (TSS), Outstanding Student Leader (OSL), Outstanding Student (OS), General Difficulty Student (GDS), Special Difficulty Student (SDS), Amount of Assistance (AoA), Times of Assistance (ToA), Source of Student (SoS), and Volunteer Service Hours (VSHs)]. Considering that SID and entrance year did not have a direct impact on student’s learning process and outcomes, they were not included in the regression experiment field. The final variables used for investigation are shown in Table 2. These variables were carefully selected based on their theoretical and practical significance with academic performance. They include factors such as participation in extracurricular activities, and previous academic achievements, which are expected to have a more direct and substantial impact on the student’s academic performance.
Data gathering for this study was done over approximately 3 weeks in January 2024. The methods of data gathering were downloading necessary Excel documents uploaded by administrators from the web pages of each department or exporting related data supporting the Excel format with the support of system administrators from management information systems. Then, we used the SID as the unique matching field to integrate the gathered data and obtained a final Excel document.
The collected samples need to be processed to facilitate the subsequent work and data analysis performed to construct a model.
To improve the stability of the regression model, the research did not intend to adopt all the variables but reduce the number of variables. To choose the objective variable from among several related variables, stepwise regression analysis was performed as a variable reduction tool. Stepwise regression analysis is a method of selecting independent variables in a linear regression model. Its basic idea is to introduce variables one by one, provided that their partial regression sum of squares is statistically significant. This study used SPSS software for stepwise regression analysis. The variable inclusion criteria probability of F-to-enter <=0.050 and exclusion criteria probability of F-to-remove >= 0.100 provided by SPSS software are widely used in various statistical analyses. This standard combination is based on traditional statistical principles and aims to construct a regression model that can effectively screen for significant variables while maintaining model stability.
In the stepwise regression experiment’s variable entry/exclusion operation, nine variables were retained and the remaining 24 variables were excluded. This study concluded that there are two main reasons for the above results. The two reasons are as follows.
First, variables have both direct and indirect effects on academic performance. For example, age and GKs can reflect students’ learning ability and basic knowledge level (Asarta and Schmidt, 2017; Alturki et al., 2022). Excellent students (such as OS, OSL, and TSS) have comprehensive qualities (Hensel, 2016). In vocational schools, obtaining the certificates of CET4 and NCRE helps students gain an advantage in academic and career development, which is reflected in their academic performance (Simoes et al., 2022). These variables have a direct or indirect positive impact on academic performance. Additionally, the support from schools and the coping abilities of students facing general difficulties may also affect their academic performance (Densley et al., 2021). Moreover, students who participate in volunteer services may have a broader perspective, a higher sense of social responsibility, and stronger interpersonal skills, which can be transferred to their studies and have a positive impact on academic performance (Qiao and Zhang, 2020).
Second, the relationship between data features and variables are explained here. In vocational colleges, due to factors like curriculum design, men and women enjoy equal opportunities; so gender has little impact on academic performance (Abbey and Adu-Danso, 2022). Under a unified education standard, ethnicity and the province to which the student belongs do not show clear distinctions in academic performance (Van Es and Weaver, 2018). Being a member of the CYLM is manifestation of identity, and there is no direct causal relationship between the membership and academic performance. It is difficult to measure the impact of professional names and subject classifications on academic performance using a unified standard. In the same school, the urban–rural categories do not have a significant impact on academic performance (Zhao, 2022). In this dataset, the number of students receiving special hardship support is small, and there is no direct logical connection between their academic performance and this number. Although the relationship between CET6 and academic performance is relatively close, relatively few vocational college students pass CET6 and obtain the certificate, so it does not universally impact academic performance (Simoes et al., 2022). The GPA of each semester can reflect the overall performance of students this semester, but considering it may easily lead to logical confusion (Beauchamp et al., 2016). Students who received rewards for individual skill competitions were originally expected to achieve higher academic performance (Berger and Fisher, 2013). Still, the actual results showed no significant relationship between this variable and academic performance. It was expected that students who took part in individual skill competitions would achieve higher academic performance (Berger and Fisher, 2013). However, the research showed no significant relationship between this variable and academic performance. The possible reason for this is that students who experienced skill competitions did not maintain good study habits and relaxed their requirements. Therefore, these variables were deleted to improve the stability and accuracy of the model.
Through stepwise regression analysis, the researcher obtained nine variables, such as Age, GKs, CET4, NCRE, TSS, OS, OSL, GDS, and VSHs. The results of the regression analysis based on the dependent variable OGPA are shown in Table 3. Based on these results from the stepwise regression analysis, the overall R-squared was 0.0475, and the Adj. R-squared was 0. 222, meaning that the dependent variable, OGPA, was predicted by the independent variables to the extent of 22.2%. The results of the variance inflation factor (VIF) in Table 3 showed that the maximum value of VIF was 1.119(<5), meaning that no multicollinearity was detected in stepwise regression. The Durbin–Watson (U) value of the model is 1.762 (belonging to the value interval of 1.7 to 2.3), which indicates that there is no autocorrelation between the independent variables and the model is better constructed.
As seen in Table 3, the value of Adj. R-squared is 0.222, which is not satisfactory, but Figure 2 shows that the standardized residuals of regression are uniformly distributed on both sides of the Y line (Y = 0) and were located between Y = 0 ± 4, which indicates that the data meets the requirements of linear regression analysis and the linear relationship is well established. The independent variables are normally distributed. In Figure 3, the residual conforms to normal analysis.
Figure 2. Scatterplot of regression standardized residual predicted value on dependent variable OGPA.
As shown in Table 3, although statistically insignificant (B = -0.026, p < 0.05), the coefficient of age is negative, suggesting that age does not have predictive power on student academic performance. In this study, the variable GKs is incorporated into the analysis. The coefficient of GKs is positive and statistically insignificant (B = 0.001, p < 0.01). Hence, GKs have a positive predictive power on academic performance. The variable CET4 is positive and statistically insignificant at the 1% level (B = 0.0001, p < 0.01). So, CET4 can be a predictor of academic performance. Based on the findings in Table 3, the coefficient of NCRE is the same as CET4. This variable also shows a positive and statistically insignificant relationship at the 1% level (B = 0.154, p < 0.01). Therefore, English ability and computer ability have a positive impact on academic performance.
Turning to student extracurricular factors, TSS has a positive and statistically significant correlation at the 1% level (B = 0.0001, p < 0.01), suggesting that TSS has a predictive power on academic performance. In addition, the regression results also show that both OS (B = 0.326, p < 0.01) and OSL (B = 0.183, p < 0.01) have been corroborated to exert positive effects on academic performance because the coefficient of the two variables is positive and statistically significant. Similarly, GDS is found to have a positive and statistically significant coefficient in the analysis (B = 0.068, p < 0.01). Hence, GDS contributes to the academic performance of the Chinese college students. The findings show that VSHs, one of the extracurricular factors, is a predictor of academic performance as well because its coefficient is statistically significant at the 1% level (B = 0.001, p < 0.01), meaning that VSHs have a positive power on OGPA. Finally, the formula of the stepwise regression model is:
In this research, variables such as CET6, SSC, SSRs, PSC, PSRs, CSC, CSRs, SDS, AoA, and ToA were excluded in stepwise regression. Possible reasons included multicollinearity, insignificant correlation with the dependent variable, the weakest ability to explain the dependent variable, or to improve the model’s simplicity and predictive ability. In other words, they contributed the least to the target variable.
Among the many factors that may affect student performance, this study specifically focuses on VSHs. With the continuous development of educational concepts, the comprehensive development of students is increasingly valued, and volunteer service, as an important form of student participation in social activities, deserves further exploration of its impact on student performance (Tlili et al., 2021). Hence, through stepwise regression, the researchers attempted to find a deeper coefficient between VSHs and OGPA by using the correlation analysis method. Turning to the discussion of the effect of VSR on academic performance, the researchers converted VSHs to VSR (0:0 h, 1: 1–100 h, 2: 101–300 h, 3:301–600 h, and 4: 601–1,000h), and constructed a chart of correlation analysis for OGPA and VSR as shown in Figure 4, in which the graph shows a “valley” and a “peak” phenomenon.
In a vast majority of empirical research, age has been analyzed for decades as one of the usual factors affecting student academic performance in colleges or universities. The findings of the present study indicated that age has a statistically significant but negative correlation and suggested that age has a negative impact on academic performance. In other words, as students get older, their academic performance tends to decline. The finding corresponds to the perspective of Da Wan and Cheo (2012), which indicated that younger students perform better than older students in academic performance. In contrast, in response to this issue, some researchers have found that older students perform better than younger students, such as Guney (2009), Alhajraf and Alasfour (2014), and Kim et al. (2023).
As reported in Table 3, GKs play a significant role in student academic performance and have positive effects. The results revealed that those students who had good habits, high ability, or performed well in high school also performed well when they entered college or university. In other words, students will bring their good habits from high school to college or university until they graduate. The finding shows that GKs have a positive and significant correlation with academic performance. Not surprisingly, the result is corroborated by several results that GKs have predictive power on academic performance in college or university (Cyrenne and Chan, 2012; Asarta and Schmidt, 2017).
Aside from GKs, CET4 (English ability) plays an important role, especially in a non-native English-speaking country. Sufficient English ability enables students to read more textbooks published in English and make new friends speaking English. Then, they have more opportunities and the ability to gain more knowledge or even jobs. Although colleges pay close attention to student’s practice, it is necessary to improve their English ability. As seen in Table 3, CET 4 has a positive and significant impact on academic performance. This finding is corroborated by several studies that propose English ability has predictive power on academic performance in colleges or universities (Asarta and Schmidt, 2017; Alturki et al., 2022).
As seen in Table 3, NCRE also has an important positive effect on OGPA. The explanation is that the majors of these participants mainly include big data and accounting, big data and financial management, and big data and finance, which require students to have high computer skills and abilities to deal with and analyze data. NCRE is a standardized and national certification exam in China that tests students’ computer skills. Those students who have already passed NCRE and obtained certificates demonstrate their computer proficiency. The findings of the research are empirically supported by Simoes et al. (2022) who found that computers influence students’ academic achievement, though few have studied the influence of computers on the prediction of students’ academic achievement.
The findings of the research showed that OS and OSL were significantly and positively associated with student academic performance. OS and OSL indicate a stronger belief in their ability to complete their task and perform well at school. Akimov et al. (2024) explored the drivers of students’ success in a first-year university and found that student engagement with their studies was reflected in class attendance. They also discovered students’ characteristics affect their academic performance, such as age, gender, marital status, and employment. Moreover, how outstanding characteristics, such as study effort and self-effacing behavior, affect academic performance remains unexplored. Although a few studies have examined the relationship between student leadership and academic performance, in medical schools, Barry et al. (2019) analyzed three cohorts of Uniformed Services University medical students (N = 509) using Pearson correlation analysis and a repeated-measures two-level hierarchical linear modeling analysis. They indicated that leader performance could be measured by the assessment tool with the guidance of a conceptual framework. In contrast, as seen in Table 3, this finding, which is different from the previous studies that did not explore student leadership, indicated that student leadership or student leader’s ability was significantly and positively associated with their OGPA.
As illustrated in Table 3, the present research supported the important factor of scholarship. This means that those students supported by scholarships (including TSS and GDS) would obtain good academic performance. Difficulty scholarships help students who are socially or economically disadvantaged to share equally in public education resources and provide students with opportunities to attend classes, obtain lecture information, and participate in class activities, which would later lead to high academic performance (Adhikari, 2023). Mulyaningsih et al. (2022) found that scholarships were positively associated with student performance in terms of GPA. Furthermore, they also found that these positive associations were strongest for the least advantaged group in terms of family income. In contrast, students influenced by their family’s difficult economic status and not supported by scholarship may place less importance on academic performance, have a more negative attitude toward school and classmates, and even have a risk of dropping out (Benner et al., 2016; Merritt and Buboltz, 2015; Jaeger et al., 2017).
A major finding of the present study was that VSHs were positively and statistically significantly associated with student academic performance. As everyone knows, volunteer services are a public welfare activity without monetary compensation for their efforts (Shantz et al., 2016). Why do volunteers continually make sacrifices of their time and energy for individuals or society? Because the motivation along with certain purposes and values energizes volunteers to devote their time (Agostinho and Paço, 2012). As reported in Table 3, this result indicated that VSHs have a significant impact on academic performance. This finding thus verifies Vaikunth et al.'s (2014) results in finding a significant difference in academic performance between students who are volunteers and those who are not. Then an interesting finding is that volunteers spend more time and energy on volunteer service or volunteer activity, and volunteers’ performance has occurred two changes. According to Figure 4, when the volunteer service level increases from 0 to 1 (i.e., the volunteer service hours are 0–100), the OGPA is in a decreasing trend, and the value of OGPA drops from 3.006 to 2.950. When the service hours are between 101 and 300, the OGPA turns into an increasing trend and the highest value of OGPA is 3.149 and currently the volunteer service level is 2. Unfortunately, when the VHS increases again to 1,000 h, the volunteer service level is 2. Though VSHs increase until 1,000 h and the service level reaches 4, the OGPA keeps decreasing until it approaches the initial value (3.010). The results showed that VSHs have a positive effect on academic performance, but we found that there was a curvilinear variation in the effect of this variable on academic performance. Therefore, it should be noted that the schools need to limit the students from spending more time or energy to be involved in the volunteer services during studying at college and encourage them to devote their limited time and energy to learning.
Although all nine variables discussed in this study have significant research significance with academic performance, only age is negatively correlated with academic performance, while all the others are positively correlated, which may be due to the following reasons:
1. From the perspective of cognitive development theory, it is a stage where students undergo significant changes in the educational environment and learning requirements after entering university from high school. High school education mainly focuses on the accumulation of basic knowledge, while university education emphasizes self-directed learning, professional skill development, and career planning (Cyrenne and Chan, 2012; Pascoe et al., 2020). Students will experience a cognitive shift in their learning during this process. The inclusion of age variables in the regression model is because as students age, they experience different stages of learning during their university years. For example, freshmen may be more in the stage of adapting to the university learning mode, and as they age, their learning cognition gradually matures in their sophomore and junior years (Akimov et al., 2024; Bergey et al., 2019). The accumulation of learning experiences accompanies the increase in age. Some students begin to transition from high school-style unilateral cognitive learning to interest-based and skill-based learning. This transition process is closely related to age and can have an impact on academic performance. In modern educational theory, English proficiency, computer operation ability, and professional skills are all important components of students’ comprehensive abilities (Liang et al., 2023; Simoes et al., 2022). The inclusion of these variables in the regression model is because they reflect different aspects of students’ ability performance in achieving this educational goal, and there is a theoretical correlation between the improvement of these abilities and academic performance.
2. To improve students’ comprehensive abilities, Chinese higher vocational colleges have formulated a series of incentive policies based on incentive theory. For example, based on motivation theory, the management department formulates policies to encourage students to participate in skill-based courses and various skill competitions (Liang et al., 2023). From the perspective of incentive compatibility, when schools provide incentives for students to participate in skill activities, such as awarding scholarships to students who have won honors in competitions, students have more motivation to improve their abilities in these areas (Adhikari, 2023; Mulyaningsih et al., 2022). This study incorporates skills-related variables, such as participation in skills competitions, into the regression model because these variables reflect the impact of incentive policies on student behavior and academic performance.
3. From the perspective of the requirements of talent cultivation objectives and social demand, students can only obtain English proficiency certificates, computer operation ability certificates, and professional skills certificates by adopting scientific learning methods and studying diligently, which is conducive to better realizing the students’ personal goals and personal values and achieving the objectives of talent cultivation (Alhadabi and Karpinski, 2020; Liang et al., 2023; Luo et al., 2023). These variables related to talent cultivation goals are added to the regression model because they reflect the impact of social demands on students’ academic performance and skills training. To meet these requirements, students will devote more learning energy and time to relevant learning and practical activities, which will, in turn, affect their academic performance (Alhadabi and Karpinski, 2020; Luo et al., 2023).
Researchers and scholars in many countries have studied the factors influencing academic performance for decades. However, empirical studies on Chinese higher vocational institutions are still limited. The target of this study is one of the 56 institutions of Chinese high-level vocational schools and specialized programs, which makes it only a representative study. Therefore, this article aimed to analyze the relevant factors affecting students’ academic performance in higher vocational colleges. The study incorporated 35 endogenous and exogenous variables.
Variables are significant factors for testing the influence on academic achievement. Although we designed the 35 variables before collecting data, the significant levels and coefficients of Age, GKs, CET4, NCRE, TSS, OS, OSL, GDS, and VSHs were investigated by stepwise regression analysis. All eight variables except age have a positive effect on academic performance. Age has a negative impact on academic achievement. Although VSHs have a positive effect on academic performance, the study found that there is a curvilinear variation in the effect of this variable on academic achievement. Therefore, it should be noted that schools should limit students from spending too much time or energy on volunteering activities during the semester and encourage them to dedicate limited time and energy to learning activities. In addition, schools should be at a higher level to improve students’ enthusiasm for participating in skill competitions and competition projects. At the same time, in improving academic achievement, schools should also improve students’ operational skills and practical abilities, because the practical hands-on ability of students is one of the important factors for the rapid employment of students in higher vocational colleges and universities. It also has become the primary recruitment condition for enterprises to choose talent.
The impact factors impacting students’ academic performance in higher vocational colleges play an absolutely important and significant role and are a major concern for educational administrators, parents, and students themselves. Firstly, the present study incorporates 35 variables related to the influence on academic performance, which are mainly derived from students’ basic personal information and academic performance. Secondly, this study will also add to the literature that has been previously researched only in other countries and not for Chinese higher vocational institutions. Internationally, although a variety of factors are related to academic performance, it is also important to explore the factors influencing students’ academic performance in Chinese higher vocational colleges and universities, which will help enrich the empirical case for research in this area. In addition, it can contribute to the comparison of national and international research findings. Thirdly, this article can provide a source for policy revision for educational administrators and educational policymakers and a guide for students to develop their study plans and efforts, because this study compares and analyzes all the major factors that affect students’ academic performance during their studies. The study reported here provides a basis for further studies, which should deepen our understanding of impact indicators related to the academic performance of Chinese college students. It is necessary to investigate how to use data-mining algorithms, such as decision trees (DT), random forest algorithms (RF), and Long Short-Term Memory (LSTM), in combination with a larger sample to explore the determination indicators of academic performance. During later experiments, we will add interventions like AI-assisted learning. Also, we will focus on time-series data collection for longitudinal studies.
This article selected one of the 26 China high-level vocational schools (Class C) as the research site, and the research data includes information gathered from a sample of 2,270 students between the academic years 2016 and 2020. This study has limitations in the following aspects.
1. Variable selection: Only some variables were included in the construction of the analysis model, without considering various factors such as students’ behavioral data and life data. This may lead to potential biases in the selected factors. Due to the lack of consideration for student behavior data (such as study habits, social activities, etc.) and life data (such as family environment, economic status, etc.), the model may not fully reflect the real factors that affect students’ academic performance.
2. Research methods: Only stepwise regression analysis and correlation analysis methods were used in the experiment, and no other data analysis methods or machine learning algorithms were utilized. Different data analysis methods and algorithms have their own advantages and applicable scenarios, and relying solely on these two methods may limit in-depth data mining and comprehensive understanding. For example, clustering analysis algorithms in machine learning can classify students based on their various characteristics, thereby unraveling differences between different types of students and their relationship with academic performance; Factor analysis can help identify common factors hidden behind numerous variables, further revealing the underlying structure that affects academic performance.
3. Sample selection: Only one vocational college was selected as the research object, which has certain limitations in the representativeness of the sample. This school may have its unique geographical, cultural, and faculty characteristics, which may differ from other vocational colleges. Therefore, the research results based on a single school sample may have applicability issues and poor generalizability when extended to other vocational colleges.
4. Practical significance: Although this study has had an impact on educational practice, there are shortcomings in providing specific strategies to local education management departments and policymakers. The research results have not been fully integrated with the actual work of local education management departments and policymakers, and there is a lack of in-depth exploration of how they can develop specific strategies to improve student performance based on the research results.
The original contributions presented in the study are included in the article/Supplementary material, further inquiries can be directed to the corresponding author.
The studies involving student’s information were approved by Hainan College of Economics and Business. The studies were conducted in accordance with the local legislation and institutional requirements. Written informed consent from the (patients/participants OR patients/participants legal guardian/next of kin) was not required to participate in this study in accordance with the national legislation and the institutional requirements. Written informed consent was obtained from the college, for the publication of any potentially identifiable images or data included in this article.
JC: Conceptualization, Data curation, Methodology, Writing – original draft, Writing – review & editing. SW: Conceptualization, Methodology, Writing – review & editing. XZ: Conceptualization, Writing – review & editing. JY: Supervision, Writing – review & editing. S-KT: Conceptualization, Funding acquisition, Writing – review & editing.
The author(s) declare that financial support was received for the research, authorship, and/or publication of this article. This study was also supported in part by the research grant (No.: RP/FCA-09/2023) offered by Macao Polytechnic University, the Hainan Provincial Natural Science Foundation of China (project number: 622RC723), and the Research and Innovation Team for the Application of HyperAutomation Technology (project number: HNJMT2023-101).
I would first like to acknowledge and thank my colleagues at Hainan College of Economics and Business for patiently providing me with the research data. In particular, I would like to thank my supervisor, Su-Kit Tang, and classmates, Shuling Wei, Xiaodeng Zhou, and Jiahua Yao, who were involved in data collection, organization, thesis conceptualization, and revision, and played an important role in the thesis writing process.
The authors declare that the research was conducted in the absence of any commercial or financial relationships that could be construed as a potential conflict of interest.
All claims expressed in this article are solely those of the authors and do not necessarily represent those of their affiliated organizations, or those of the publisher, the editors and the reviewers. Any product that may be evaluated in this article, or claim that may be made by its manufacturer, is not guaranteed or endorsed by the publisher.
The Supplementary material for this article can be found online at: https://www.frontiersin.org/articles/10.3389/feduc.2024.1433021/full#supplementary-material
Abbey, E., and Adu-Danso, E. (2022). Gender diversity and productivity in manufacturing firms: evidence from six sub-Saharan African (SSA) countries. J. Manag. Organ. 29, 1029–1050. doi: 10.1017/jmo.2022.50
Adhikari, K. P. (2023). Academic performance of fee paying and scholarship students at CTEVT affiliated nursing colleges in Nepal. J. Tech. Vocation. Educ. Train. 17, 130–137. doi: 10.3126/tvet.v17i1.52430
Agostinho, D., and Paço, A. (2012). Analysis of the motivations, generativity and demographics of the food bank volunteer. Int. J. Nonprofit Volunt. Sect. Mark. 17, 249–261. doi: 10.1002/nvsm.1427
Akimov, A., Malin, M., Sargsyan, Y., Suyunov, G., and Turdaliev, S. (2024). Student success in a university first-year statistics course: do students’ characteristics affect their academic performance? J. Stat. Data Sci. Educ. 32, 24–35. doi: 10.1080/26939169.2023.2184435
Aktaç, Ş., Kızıltan, G., and Avcı, S. (2019). The effect of family participation in nutrition education intervention on the nutritional status of preschool age children. Ted Eğitim Bilim. 44, 415–431. doi: 10.15390/EB.2019.7819
Alfan, E., and Othman, N. M. (2005). Undergraduate students’ performance: the case of University of Malaya. Quality Assurance in Education, 13, 329–343. doi: 10.1108/09684880510626593
Alghamdi, A., Karpinski, A. C., Lepp, A., and Barkley, J. (2020). Online and face-to-face classroom multitasking and academic performance: moderated mediation with self-efficacy for self-regulated learning and gender. Comput. Hum. Behav. 102, 214–222. doi: 10.1016/j.chb.2019.08.018
Alhadabi, A., and Karpinski, A. (2020). Grit, self-efficacy, achievement orientation goals, and academic performance in university students. Int. J. Adolesc. Youth 25, 519–535. doi: 10.1080/02673843.2019.1679202
Alhajraf, N. M., and Alasfour, A. M. (2014). The impact of demographic and academic characteristics on academic performance. Int. Bus. Res. 7:p92. doi: 10.5539/ibr.v7n4p92
Alturki, S., Cohausz, L., and Stuckenschmidt, H. (2022). Predicting Master’s students’ academic performance: an empirical study in Germany. Smart Learn. Environ. 9:38. doi: 10.1186/s40561-022-00220-y
Alyoussef, A. A. K., Amirthalingam, P., and Mohammed, O. S. (2016). Reciprocity of the level of parent education and academic performance of the medical students of the University of Tabuk, Kingdom of Saudi Arabia. Ind. J. Med. Specialit. 7, 103–105. doi: 10.1016/j.injms.2016.04.004
Anjum, S. (2021). Impact of extracurricular activities on academic performance of students at secondary level. Int. J. Appl. Guid. Counsel. 2, 7–14. doi: 10.26486/ijagc.v2i2.1869
Asarta, C. J., and Schmidt, J. R. (2017). Comparing student performance in blended and traditional courses: does prior academic achievement matter? Internet High. Educ. 32, 29–38. doi: 10.1016/j.iheduc.2016.08.002
Atas, I. (2022). Human gender prediction based on deep transfer learning from panoramic dental radiograph images. Trait. Du Signal 39, 1585–1595. doi: 10.18280/ts.390515
Barry, E., Dong, T., Durning, S., Schreiber-Gregory, D., Torre, D., and Grunberg, N. (2019). Medical student leader performance in an applied medical field practicum. Mil. Med. 184, 653–660. doi: 10.1093/milmed/usz121
Beauchamp, G., Martineau, M., and Gagnon-, A. (2016). Examining the link between adult attachment style, employment and academic achievement in first semester higher education. Soc. Psychol. Educ. 19, 367–384. doi: 10.1007/s11218-015-9329-3
Benner, A. D., Boyle, A. E., and Sadler, S. (2016). Parental involvement and adolescents’ educational success: the roles of prior achievement and socioeconomic status. J. Youth. Adolesc. 45, 1053–1064. doi: 10.1007/s10964-016-0431-4
Berger, N., and Fisher, P. (2013). A well-educated studyforce is key to state prosperity. Econ. Policy Instit. 22, 1–14.
Bergey, B. W., Parrila, R. K., Laroche, A., and Deacon, S. H. (2019). Effects of peer-led training on academic self-efficacy, study strategies, and academic performance for first-year university students with and without reading difficulties. Contemp. Educ. Psychol. 56, 25–39. doi: 10.1016/j.cedpsych.2018.11.001
Burns, D. J., Reid, J., Toncar, M., Anderson, C., and Wells, C. (2008). The effect of gender on the motivation of members of generation Y college students to volunteer. J. Nonprofit Publ. Sect. Market. 19, 99–118. doi: 10.1300/J054v19n01_05
Chen, J., Zhou, X., Yao, J., and Tang, S.-K. (2024). “Evaluation of student performance based on learning behavior with random Forest model” in 2024 13th International Conference on Educational and Information Technology (ICEIT), 266–272.
Cheng, W., and Nguyen, P. (2023). Gender differences in future time perspectives and risk of being not in employment, education, or training: the mediating role of achievement goal motivations. Curr. Psychol. 42, 28180–28190. doi: 10.1007/s12144-022-03921-2
Chui, K. T., Liu, R. W., Zhao, M., and Ordóñez De Pablos, P. (2020). Predicting students’ performance with school and family tutoring using generative adversarial Netstudy-based deep support vector machine. IEEE Access 8, 86745–86752. doi: 10.1109/ACCESS.2020.2992869
Cyrenne, P., and Chan, A. (2012). High school grades and university performance: a case study. Econ. Educ. Rev. 31, 524–542. doi: 10.1016/j.econedurev.2012.03.005
Da Wan, C., and Cheo, R. (2012). Determinants of Malaysian and Singaporean economics undergraduates’ academic performance. Int. Rev. Econ. Educ. 11, 7–27. doi: 10.1016/S1477-3880(15)30014-1
Danilowicz-Gösele, K., Lerche, K., Meya, J., and Schwager, R. (2017). Determinants of students’ success at university. Educ. Econ. 25, 513–532. doi: 10.1080/09645292.2017.1305329
Densley, B., Calvert, H., Boedeker, P., and Turner, L. (2021). Implementation of physical activity in US elementary schools: the role of administrative support, financial resources, and champions. Int. J. Environ. Res. Public Health 18:4476. doi: 10.3390/ijerph18094476
Forster, B. L., and Reuter, P. R. (2022). Do college students’ living arrangements affect their health behaviors and academic performance? J. Am. Coll. Heal. 72, 1078–1084. doi: 10.1080/07448481.2022.2066978
Gao, H., Ou, Y., Zhang, Z., Ni, M., Zhou, X., and Liao, L. (2021). The relationship between family support and e-learning engagement in college students: the mediating role of e-learning normative consciousness and behaviors and self-efficacy. Front. Psychol. 12:573779. doi: 10.3389/fpsyg.2021.573779
Gil, M., Kim, S., and Min, E. (2022). Machine learning models for predicting risk of depression in Korean college students: identifying family and individual factors. Front. Public Health 10:1023010. doi: 10.3389/fpubh.2022.1023010
Greenbank, P., Hepworth, S., and Mercer, J. (2009). Term-time employment and the student experience. Educ. Train. 51, 43–55. doi: 10.1108/00400910910931823
Guney, Y. (2009). Exogenous and endogenous factors influencing students’ performance in undergraduate accounting modules. Acc. Educ. 18, 51–73. doi: 10.1080/09639280701740142
Haley, M. R., Johnson, M. F., and Kuennen, E. W. (2007). Student and professor gender effects in introductory business statistics. J. Stat. Educ. 15:8. doi: 10.1080/10691898.2007.11889549
Han, W., Altalbe, A., Rehman, N., Rehman, S., and Sharma, S. (2024). Exploring the longitudinal impacts of academic stress and lifestyle factors among Chinese students. J. Pharm. Policy Pract. 17:2398706. doi: 10.1080/20523211.2024.2398706
Hensel, D. (2016). Q-methodology: an alternative design for undergraduate nursing honors research. J. Nurs. Educ. 55, 659–662. doi: 10.3928/01484834-20161011-10
Herbst, M., Sobotka, A., and Wójcik, P. (2023). The effect of peer group stability on achievements: evidence from Poland. Eur. J. Educ. 58, 166–180. doi: 10.1111/ejed.12545
Hovdhaugen, E. (2013). Working while studying: the impact of term-time employment on dropout rates. J. Educ. Work. 28:869311. doi: 10.1080/13639080.2013.869311
Huntington-Klein, N., and Gill, A. (2021). Semester course load and student performance. Res. High. Educ. 62, 623–650. doi: 10.1007/s11162-020-09614-8
Ibarra-Vazquez, G., Rami-rez-Montoya, M., and Terashima, H. (2023). Gender prediction based on university students’ complex thinking competency: an analysis from machine learning approaches. Educ. Inf. Technol. 29, 2721–2739. doi: 10.1007/s10639-023-11831-4
Jaeger, A. J., Mitchall, A., O’Meara, K., Grantham, A., Zhang, J., Eliason, J., et al. (2017). Push and pull: The influence of race/ethnicity on agency in doctoral student career advancement. J. Divers. High. Educ. 10, 232–252. doi: 10.1037/dhe0000018
Jawad, K., Shah, M. A., and Tahir, M. (2022). Students’ academic performance and engagement prediction in a virtual learning environment using random Forest with data balancing. Sustain. For. 14:14795. doi: 10.3390/su142214795
Johnson, M., and Kuennen, E. (2006). Basic math skills and performance in an introductory statistics course. J. Stat. Educ. 14:2. doi: 10.1080/10691898.2006.11910581
Kaighobadi, M., and Allen, M. T. (2008). Investigating academic success factors for undergraduate business students. Decis. Sci. J. Innov. Educ. 6, 427–436. doi: 10.1111/j.1540-4609.2008.00184.x
Kim, S., Choi, E., Jun, Y.-K., and Lee, S. (2023). Student dropout prediction for university with high precision and recall. Appl. Sci. 13:6275. doi: 10.3390/app13106275
Kuh, G. D. (2009). The national survey of student engagement: conceptual and empirical foundations. New Dir. Inst. Res. 2009, 5–20. doi: 10.1002/ir.283
Liang, M., Wang, S., Liang, M., and Zhang, J. (2023). The role of skills competitions in improving professional knowledge learning in vocational education. Sci. Soc. Res. 5, 16–21. doi: 10.26689/ssr.v5i5.4886
Luo, Q., Chen, L., Yu, D., and Zhang, K. (2023). The mediating role of learning engagement between self-efficacy and academic achievement among Chinese college students. Psychol. Res. Behav. Manag. 16, 1533–1543. doi: 10.2147/PRBM.S401145
Merritt, D. L., and Buboltz, W. (2015). Academic Success in College: Socioeconomic Status and Parental Influence as Predictors of Outcome. Open J. Soc. Sci. 3, 127–135. doi: 10.4236/jss.2015.35018
Muhonen, H., Pakarinen, E., Rasku-Puttonen, H., and Lerkkanen, M.-K. (2023). Educational dialogue and teacher occupational stress in relation to student math performance. Scand. J. Educ. Res. Scopus. 68, 539–557. doi: 10.1080/00313831.2023.2175240
Mulyaningsih, T., Dong, S., Miranti, R., Daly, A., and Purwaningsih, Y. (2022). Targeted scholarship for higher education and academic performance: evidence from Indonesia. Int. J. Educ. Dev. 88:102510. doi: 10.1016/j.ijedudev.2021.102510
Orlov, A., and Roufagalas, J. (2012). Performance determinants in undergraduate economics classes: the effect of cognitive reflection. Int. Rev. Econ. Educ. 11, 28–45. doi: 10.1016/S1477-3880(15)30013-X
Owings, W. A., Kaplan, L. S., and Pirim, Z. (2012). Education as an Investment in Turkey’s human capital: a work in progress. Euras. J. Bus. Econ. 5, 45–70.
Pascoe, M., Hetrick, S., and Parker, A. (2020). The impact of stress on students in secondary school and higher education. Int. J. Adolesc. Youth 25, 104–112. doi: 10.1080/02673843.2019.1596823
Phipps, A., and Amaya, A. (2023). Are students time constrained? Course load, GPA, and failing. J. Public Econ. 225:104981. doi: 10.1016/j.jpubeco.2023.104981
Qiao, G., and Zhang, H. (2020). Exploratory research on college students’ motivations to volunteer – a case of international events hosted in China. Tour. Critiq. Pract. Theory 1, 35–46. doi: 10.1108/TRC-03-2020-0003
Rao, A. R. (2020). Interventions for promoting student engagement and predicting performance in an introductory engineering class. AEE J. 8:36019. doi: 10.18260/3-1-1130-36019
Rochford, C., Connolly, M., and Drennan, J. (2009). Paid part-time employment and academic performance of undergraduate nursing students. Nurse Educ. Today 29, 601–606. doi: 10.1016/j.nedt.2009.01.004
Romer, P. M. (1990). Endogenous technological change. J. Polit. Econ. 98, S71–S102. doi: 10.1086/261725
Salamonson, Y., and Andrew, S. (2006). Academic performance in nursing students: influence of part-time employment, age and ethnicity. J. Adv. Nurs. 55, 342–349. doi: 10.1111/j.1365-2648.2006.03863_1.x
Savić Tot, T., Adžić, S., Tot, V., Aleksić, M., and Zakić, N. (2023). The impact of time devoted to video games on student achievement. Educ. Inf. Technol. 28, 5921–5944. doi: 10.1007/s10639-022-11418-5
Scheaffer, R. L., and Stasny, E. A. (2004). The state of undergraduate education in statistics: a report from the CBMS 2000. Am. Stat. 58, 265–271. doi: 10.1198/000313004X5770
Shantz, A., Alfes, K., and Whiley, L. (2016). HRM in healthcare: the role of study engagement. Pers. Rev. 45, 274–295. doi: 10.1108/PR-09-2014-0203
Shi, S., Zhang, Z., Wang, Y., Yue, H., Wang, Z., and Qian, S. (2021). The relationship between college teachers’ frustration tolerance and academic performance. Front. Psychol. 12:564484. doi: 10.3389/fpsyg.2021.564484
Simoes, S., Oliveira, T., and Nunes, C. (2022). Influence of computers in students’ academic achievement. Heliyon 8:e09004. doi: 10.1016/j.heliyon.2022.e09004
Sothan, S. (2019). The determinants of academic performance: evidence from a Cambodian university. Stud. High. Educ. 44, 2096–2111. doi: 10.1080/03075079.2018.1496408
Tlili, A., Zhang, J., Papamitsiou, Z., Manske, S., Huang, R., Kinshuk,, et al. (2021). Towards utilising emerging technologies to address the challenges of using open educational resources: a vision of the future. Educ. Technol. Res. Dev. 69, 515–532. doi: 10.1007/s11423-021-09993-4
Vaikunth, S. S., Cesari, W. A., Norwood, K. V., Satterfield, S., Shreve, R. G., Ryan, J. P., et al. (2014). Academic achievement and primary care specialty selection of volunteers at a student-run free clinic. Teach. Learn. Med. 26, 129–134. doi: 10.1080/10401334.2014.883980
Van Es, C., and Weaver, M. M. (2018). Race, sex, and their influences on introductory statistics education. J. Stat. Educ. 26, 48–54. doi: 10.1080/10691898.2018.1434426
Zhai, X., Ye, M., Gu, Q., Huang, T., Wang, K., Chen, Z., et al. (2022). The relationship between physical fitness and academic performance among Chinese college students. J. Am. Coll. Heal. 70, 395–403. doi: 10.1080/07448481.2020.1751643
Zhang, Q., Cao, Y., and Tian, J. (2021). Effects of violent video games on aggressive cognition and aggressive behavior. Cyberpsychol. Behav. Soc. Netstud. 24, 5–10. doi: 10.1089/cyber.2019.0676
Zhao, K. (2022). Rural-urban gap in academic performance at a highly selective Chinese university: variations and determinants. High. Educ. Res. Dev. 41, 177–192. doi: 10.1080/07294360.2020.1835836
Keywords: academic performance, influencing factors, Chinese vocational college students, stepwise regression model, correlation analysis
Citation: Chen J, Wei S, Zhou X, Yao J and Tang S-K (2024) OGPA: a novel indicator hinting at the academic performance of Chinese vocational college students using stepwise regression. Front. Educ. 9:1433021. doi: 10.3389/feduc.2024.1433021
Received: 15 May 2024; Accepted: 30 October 2024;
Published: 21 November 2024.
Edited by:
Khalida Parveen, Qujing Normal University, ChinaReviewed by:
Phuc Quang Bao Tran, Ho Chi Minh City University of Foreign Languages and Information Technology, VietnamCopyright © 2024 Chen, Wei, Zhou, Yao and Tang. This is an open-access article distributed under the terms of the Creative Commons Attribution License (CC BY). The use, distribution or reproduction in other forums is permitted, provided the original author(s) and the copyright owner(s) are credited and that the original publication in this journal is cited, in accordance with accepted academic practice. No use, distribution or reproduction is permitted which does not comply with these terms.
*Correspondence: Juntao Chen, SnVudGFvQ2hlbkBoY2ViLmVkdS5jbg==
Disclaimer: All claims expressed in this article are solely those of the authors and do not necessarily represent those of their affiliated organizations, or those of the publisher, the editors and the reviewers. Any product that may be evaluated in this article or claim that may be made by its manufacturer is not guaranteed or endorsed by the publisher.
Research integrity at Frontiers
Learn more about the work of our research integrity team to safeguard the quality of each article we publish.