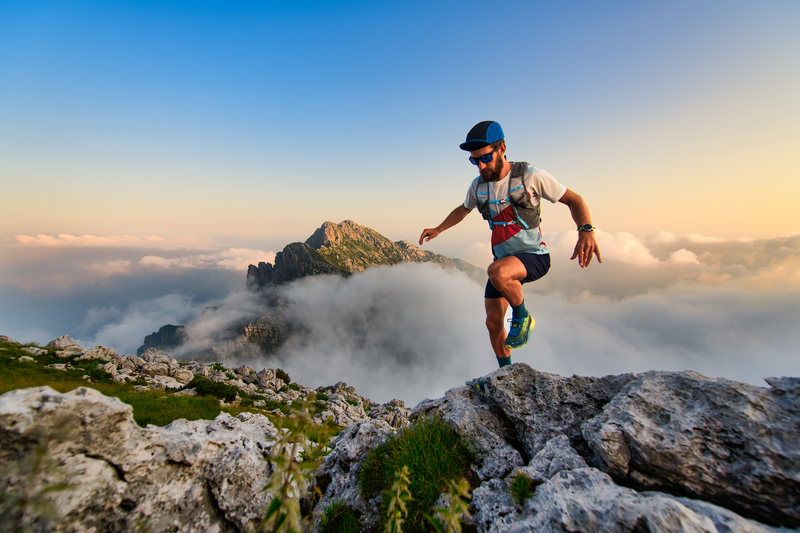
95% of researchers rate our articles as excellent or good
Learn more about the work of our research integrity team to safeguard the quality of each article we publish.
Find out more
ORIGINAL RESEARCH article
Front. Educ. , 07 August 2024
Sec. Digital Education
Volume 9 - 2024 | https://doi.org/10.3389/feduc.2024.1417062
Introduction: This study investigates the digital citizenship behavior (DCB) and behavioral intention (BI) of faculty members at the Public Authority for Applied Education and Training (PAAET) colleges in Kuwait. The research aims to evaluate the effectiveness of technology in teaching and provide a case study of higher educational institutions in Kuwait. The study’s theoretical framework focuses on four key factors: performance expectancy (PE), effort expectancy (EE), social influence (SI), and facilitating conditions (FC), examining their impact on faculty readiness (RED) through the mediation of both BI and DCB. A total of 28 hypotheses were tested, exploring direct and indirect relationships among these variables.
Methods: A survey was conducted among 122 faculty members from PAAET colleges in Kuwait. The questionnaire measured the participants’ perceptions of PE, EE, SI, and FC, along with their BI and DCB. The study employed statistical methods to analyze the data and test the 28 hypotheses, aiming to identify significant paths of relationships that contribute to faculty readiness for technology use in education.
Results: The findings confirmed eight significant paths of relationships, highlighting the importance of inputs such as effort and social influence and their impact on performance as an output in determining faculty readiness. However, the study raised empirical doubts about the remaining 20 hypotheses, providing new evidence that challenges the currently established relationships between technology acceptance and digital citizenship.
Discussion: The study offers valuable insights into the factors influencing the effective use of technology in higher education institutions in Kuwait. It suggests that while some pathways, such as effort and social influence, significantly contribute to faculty readiness, other expected relationships may not hold as previously thought. This new evidence calls for a reevaluation of the existing models of technology acceptance and digital citizenship. The results have important implications for policy and practice, offering guidance on enhancing technology use among faculty members in educational settings. The study contributes to the broader discussion on digital transformation in education, providing a comprehensive understanding of the key factors that affect faculty readiness for adopting new technologies.
The use of technology in higher education institutions has become increasingly important in recent years, leading to a significant digital transformation in the teaching and learning processes. Integrating digital technology has reshaped pedagogical approaches and content delivery to enhance teaching effectiveness. However, the adoption of digital transformation in higher education institutions is often accompanied by challenges related to a lack of readiness (Dehghan et al., 2022).
Understanding the factors that influence the readiness of digital transformation is essential for institutions to effectively leverage technology and maximize its potential. Readiness refers to the degree of preparedness and capability to adopt digital transformation within an organization (Hradecky et al., 2022). In the context of higher education institutions, readiness is related to being prepared for new work situations, fostering an effective learning environment (Dehghan et al., 2022). The complexity of understanding technology readiness requires a comprehensive examination of the associated factors to provide a holistic view of the digital transformation challenges faced by higher education institutions.
The complexity of the technology readiness arises from its determinants, as organizations increasingly integrate both social and digital artifacts, creating a need for new paradigms to effectively manage and utilize these elements (Figueroa-Armijos et al., 2023).
First, social artifacts are social conditions, where social beings are transforming and adapting their behaviors to fit in the new technology in various spheres of life (Al-Mamary, 2022). The emergent spheres of life motivate individuals to form and reform their behavior and intentions when technology is adopted. Such continuous change in behavioral intentions is important to be captured as evidence in various contexts, to assess the level of readiness existing in a social cluster such as universities (Al-Shamali et al., 2022; Purohit et al., 2022; Trivedi et al., 2022).
Second, the spur of technology and digital transformation has also caused the emergence of digital citizenship, thereby providing a way for the readiness to work in a digitally enabled learning environment in universities. The notion of digital citizenship incorporates how digital technologies may be utilized ethically to create a more responsible learning environment (Sussan and Acs, 2017). Readiness is an essential component of digital citizenship, since it encompasses the ethical utilisation of digital technology to cultivate a responsible learning environment. This preparedness not only promotes the utilisation of digital educational technologies but also emphasises the difficulties in controlling their improper use, particularly when educators and students share information electronically. Recent studies have suggested the increase in digital citizenship awareness among the faculty and also the content has changed to incorporate the same (Martin et al., 2022).
In the dichotomous intersection of behavioral intention as the users’ decision to adopt the technology and the growing digital citizenship, it is important to explain the role of emergent determinants and how the same go through the behavioral intention and digital citizenship to inform the new form of readiness for the digitally enabled learning environment. This study, therefore, poses two main questions.
First, what are the determinants of behavioral intention toward the use of technology? This question is important in the context of understanding how the intention to use technology in learning environment happens and how the same leads to the readiness of the faculty (Al-Fahim et al., 2016; Inayatulloh, 2020; Qader et al., 2022).
Second, what are the determinants of digital citizenship and technology readiness? Technology, like a double-edged sword, has two sides. If used constructively, it can create significant performance differences but can also result in the opposite and bring in unique problems to the workplaces at universities (Khechine and Lakhal, 2018). Therefore, it is essential to evaluate how the responsible use of technology demonstrated in digital citizenship may mediate the impacts on overall readiness for the technology adoption. Collectively, both mediators can inform the technology readiness in terms of digital skill preparedness and also preparedness in terms of the ethical use of digital skills.
Although extensive research exists on the adoption of digital technology by educational institutions, limited studies have investigated the factors influencing faculty technology readiness. Therefore, this study aims to address this gap by exploring the factors influencing technology readiness for digital transformation in higher education institutions in Kuwait, particularly at the Public Authority for Applied Education and Training (PAAET) colleges.
The following section reviews the literature on the four prominent determinants of both behavioral intention and digital citizenship and their impact on technology readiness.
This study presents a cohesive review on the core determinants of technology acceptance with the focus on how the determinants lead to dichotomous mediators of behavioral intention and digital citizenship and consequent collective readiness to use technology in higher education context. The complete framework of the Unified Theory of acceptance and Use of Technology (UTAUT), which evaluates technology adoption via the impacts of performance expectation (PE), effort expectancy (EE), social influence (SI), and facilitating conditions (FC), justifies the use of UTAUT in this study. The purpose of this study is to assess the behavioral intention (BI) and digital citizenship behavior (DCB) of faculty members serving as mediators at the Public Authority for Applied Education and Training (PAAET) institutions. By doing this, it aims to assess how well technology is used in the classroom and offers a case study of higher education institutions in Kuwait.
Performance expectancy of a teaching technology is associated with how the teacher expects that the technology will be useful for them in achieving effective and efficient teaching practices. Based on the dichotomous perspective of this research, the literature can provide instances of performance expectancy that can lead to both behavioral intention to use technology and contributes to the very nature of digital citizenship that emerges.
The performance expectancy motivates the teachers to make up their mind about using a particular technology (Anwar and Alviayatun, 2022). It is because performance expectancy is essential because of both internal and systemic measures, and when the technology is believed to be helping in achieving the same, then teachers are more inclined to adopt the technology and thus set the learning of technology as their goals for better performance (Kaluarachchi, 2023).
The performance expectancy also motivates the teachers to establish a digital citizenship. As for example, studies have suggested that when workers are provided with the opportunities to use digital artifacts, they also bring in their ethical self into the practice to establish more trustworthy practices in their routine (Figueroa-Armijos et al., 2023). Such a routine forms a digital citizenship toward the employee’s readiness to adopt technology.
Teachers as efficient human beings try to reduce the cognitive and physical efforts that they are placing in various teaching functions, such as preparation of the content, delivery of a lecture, and evaluation of the examination papers. Consequently, when teachers observe a potential technology, they evaluate if the same will require minimal cognitive and physical efforts to incorporate in their current regime of teaching. They are more likely to establish a behavioral intention to utilize it (Alkuwaiti et al., 2023) and in the government sector institutions (Undi-Phiri and Phiri, 2022). There is, however, a counter thesis to this effort expectancy which do not have a positive effect on the continuance of such an intention once it is preformed (Winata and Tjokrosaputro, 2022; Ramírez-Correa et al., 2023).
The effort expectancy is also theorized to have an effect on the digital citizenship behavior of the teachers. It is because the citizenship of morals and ethics is strongly associated with the legitimate amount of work efforts required to perform a task. Therefore, teachers when performing their duties through technology triggers their responsible citizenship as well. The trigger of such a citizenship is not enough as both “students and faculty in institutions were aware of the digital citizenship concepts but lacked the in-depth knowledge and understanding of concepts such as digital rights, digital security, and digital ethics” (Hawamdeh et al., 2022). It is, nevertheless, important for the faculty to have sufficient knowledge of the technology and associated digital citizenship toward readiness.
Social influence is concerned with how opinions, beliefs, and routines of others around the user can impact the users’ own behavioral intention and also their citizenship behavior. The people in the institutions and organizations make a collective culture and form a basis for more herding behavior and thus influence individuals to form certain behavioral intention and citizenship behavior. “This intangible aspect and power are even more enigmatic and harmful, which can lead to a change in cognitive references and behaviors. Social influence heavily affects the spontaneity of individuals and they become a subject to dominant forces, which must be properly controlled by management” (Gharbi et al., 2022). These influences have been found varying with various demographics, such as gender, age, and education but still social influence is significant in establishing the overall technology adoption (Gumz et al., 2022). The social influence can be both negative and positive in which if the adoption is prevailing in the user’s neighborhood, then the user is more likely to adopt it and the opposite is also true if the adoption is otherwise not popular (Luo et al., 2022).
Facilitating conditions, in the context of technology acceptance, refers to the factors and resources which help individuals adopt the technology and use it for their routine functions. Studies have suggested that facilitating conditions in any organizational settings enable the users’ perceptions of ease and use and consequently leads them to form their behavioral intention (Natasia et al., 2022). The facilitating conditions have also been shown as co-existing with the behavior intention leading toward implementing the technology for enhancing the performance in the learning system (Al-Mamary, 2022). The facilitating conditions provide an overall environment for learning to happen and also trigger digital citizenship in terms of its responsible use and overall learning experience of the users (Purohit et al., 2022).
Behavioral intention is an important construct of the technology adoption models, and it refers to the explicitly expressed potential to perform a certain action related to the technology. Such a behavior is when form is more likely to lead to the adoption of a particular technology. Similarly, studies also suggest that behavioral intention is deeply concerned with the existence of users’ trust, which is observed as a fundamental component of behavioral intention (Hooda et al., 2022). The behavioral intention as output of the antecedents discussed may, however, vary in various cultural contexts (Tseng et al., 2022). The learning context of faculty members, the behavioral intention, is considered an important indication of the faculty experience with technology and can provide bases for the content and related information system developments (Lavidas et al., 2022). Studies suggest significant variations in every determinant of behavioral intention (Al-Sharhan et al., 2024).
Another important mediating variable that this study is considering is digital citizenship, which is the ethical and moral use of technology in learning environments. On one hand, the antecedents such as effort and performance expectancies, social pressure, and facilitating conditions determine the user intention to use or not use a technology, and on the other hand, antecedents also inform their digital citizenship (Almogren and Aljammaz, 2022). This usually happens in the learning context because the faculty knowledge about the technology and its associated citizenship are transferred across the university through content, interactions with students, and other curricular activities (Martin et al., 2022). The more everlasting mediating role of digital citizenship is established through extended and formal development of digital citizenship curriculum to increase knowledge about the phenomenon (Brandau et al., 2022). The users are also found to be making themselves literate about digital citizenship while reading online when they are concerned with technology (Avcı and Yıldız Durak, 2022). There is, however, more in-depth knowledge required about the digital citizenship concepts “such as digital rights, digital security, and digital ethics” (Hawamdeh et al., 2022) for achieving a readiness for the technology use in the classrooms.
The readiness, in general, refers to the condition of being prepared to adapt to specific situation or situation or circumstance (Dehghan et al., 2022; Hradecky et al., 2022; Masso et al., 2022). Readiness has been considered as an output of the model because even if behavioral intention and digital citizenship exist, the technology adoption may still not happen until sufficient readiness is achieved. The readiness is expected to emerge when the learning of the technology is achieved in terms of having a behavioral intention and sufficient digital citizenship. Both of these constructs form a high level of awareness for learning readiness among the users (Almaiah et al., 2022). The users’ readiness, however, can be further enhanced through purposeful training about the courses and the system available so that the overall learning experience is effective (Dehghan et al., 2022). In this regard, the readiness of teachers is the core of overall success of e-learning (Alkandari, 2023). Teachers’ behavioral intentions, along with their ethics and value systems, demonstrate that digital citizenship is present and essential for fostering a thriving environment of readiness (AlMutairi and Alharbi, 2022; Al-Sharhan et al., 2024). Such readiness is important for continue improvement in the learning systems in higher educational institutions of Kuwait (Al-Shamali et al., 2022).
The online questionnaire used in this study consisted of 41 items, which required approximately 20 min to complete. The questionnaire was designed to gather data on various aspects related to the research objective. The introduction section of the questionnaire aimed to provide a clear explanation of the research objective and assure participants of the confidentiality of their responses. To measure the participants’ responses, a five-point Likert scale was utilized for all items, except for the demographic variables, such as gender, education, technology, and experience. The survey was distributed through social networking among the instructors from PAAET. This method of distribution may have been selected for its convenience and accessibility, as well as the potential to reach a larger number of participants within the target population. By targeting instructors, the study may aim to gain insights into their attitudes, beliefs, and practices related to the research objective.
In this study, we surveyed a sample population of 122 faculty members from PAAET colleges in Kuwait. The sample was selected using a convenience sampling technique, where the survey was distributed through social networking and official email addresses. This method ensured accessibility and a higher response rate. The sample size of 122 faculty members, out of 320 members, represents a substantial response rate of approximately 38%, which is adequate for the statistical analyses conducted and supports the reliability and validity of the findings of the study.
The data collection of the study was meticulously executed among faculty members belonging to PAAET colleges. Questionnaires were distributed anonymously via the faculty members’ official email addresses, a strategy that was integral in maintaining confidentiality and promoting the authenticity of the responses. To enhance the response rate, the study used several reminders and direct engagements with the target audience. These concerted efforts resulted in the successful participation of 122 out of 320 faculty members, representing a substantial response that corroborates the diligent approach.
The data analysis process consisted of two steps. Initially, the data were imported into SPSS version 28 for initial data analysis, which involved data cleaning and the generation of descriptive statistics. This step provided an overview of the data. In the second step, the suggested model was assessed using Partial Least Squares Structural Equation Modeling (PLS-SEM) with the SmartPLS 4 software package (Ringle et al., 2015; Hair et al., 2018). PLS-SEM is an advanced statistical method that extends traditional multivariate analysis techniques such as regression, factor analysis, and discriminant analysis. It allows for the simultaneous examination of multiple relationships between independent and dependent variables. The structural equation model enables the investigation of connections between observable variables and latent constructs. It focused on the evaluation of the measurement model and the assessment of the structural model (Qureshi et al., 2023a).
In the measurement model assessment, various statistical measures such as Cronbach’s alpha, composite reliability, and cross-loadings were examined to assess the reliability and validity of the measurement model. These measures were available in SmartPLS software and helped ensure the robustness of the measurement model. Following the measurement model assessment, the proposed hypotheses were examined within the structural model assessment phase. This involved analyzing the relationships between variables and evaluating the significance of these relationships.
Table 1 demonstrates the demographic characteristics of the participants. There are 122 individuals. Out of these, 33.6% were male and 66.4% were female participants. This shows a higher proportion of females to males in the sample. Furthermore, it was noticeable that the majority of the participants (73.8%) had more than 20 years of experience. Educational attainment was notably high, with the majority of participants (57.4%) holding a master’s degree, followed by those with PhD (25.4%), and a smaller fraction holding a bachelor’s degree (17.2%). In relation to technology usage, a sizable portion of the participants reported “always” integrating technology into their work (43.4%), with “very often” (25.4%) and “sometimes” (27.9%) also being prevalent, whereas a negligible 3.3% reported “rarely” using technology.
Table 2 shows the results of the χ2 test that was conducted to find the association of demographic variables with technology used. The provided data offer a breakdown of technology usage patterns by gender, experience, and education level among 122 individuals. The chi-square test for gender differences in technology usage did not reach statistical significance (p = 0.344), suggesting that within this sample, technology usage does not differ between males and females.
Regarding professional experience, there was a statistically significant association with technology usage (p = 0.026). Individuals with more than 20 years of experience were the most likely to use technology “sometimes” (85.3%) and “very often” (83.9%), yet this group was less dominant in the “always” category (64.2%). This might imply that those with more experience may integrate technology in a more flexible or situation-dependent manner.
In terms of educational attainment, a statistically significant difference was also observed (p = 0.011), indicating that educational levels are associated with different patterns of technology use. Interestingly, individuals with PhD were most likely to “rarely” use technology (75.0%), while those with a master’s degree led in the “sometimes” (61.8%) and “very often” (71.0%) categories. Bachelor’s degree holders, although the least represented overall, had a substantial presence in the “always” category (30.2%).
Figure 1 shows the distribution of technology usage among individuals with varying educational qualifications. The frequency of technology usage is classified into four categories: “always,” “very often,” “sometimes,” and “rarely.” The data suggest a higher propensity for technology usage among those with a bachelor’s degree, with a notable 76% reporting that they always use technology, followed by 23% who very often do so, and a combined 1% who either sometimes or rarely use it. Contrastingly, individuals with a master’s degree exhibit a more even distribution of usage frequency: 37% always use technology, 31% very often use technology, 30% sometimes use technology, and a minimal 1% rarely use technology. For those with a PhD, the trend shifts slightly, with 35% always using technology, a smaller 23% doing so very often, 32% sometimes, and 10% rarely. These findings underscore the variability in technology engagement relative to academic attainment, potentially reflecting differing professional demands or personal inclinations toward technology use.
Structural equation modeling (SEM) is a statistical technique used to examine multiple relationships simultaneously (Figure 2). The first step of SEM involves assessing the outer measurement model, which examines the relationships between observed variables and their underlying latent constructs. The second step involves evaluating the inner model, also known as the structural model, which examines the relationships between latent constructs themselves (Al-Shamali et al., 2022; Almakayeel et al., 2023). Figure 1 shows the basic node diagram of the model after removing the items having loading less than 0.6.
Figure 2. Structural equation model (SEM) depicting relationships among variables influencing behavioral intentions and readiness. This model visualizes the hypothesized pathways and their respective loadings between constructs such as Performance Expectancy (PE), Effort Expectancy (EE), Social Influence (SI), Facilitating Conditions (FC), Behavioral Intention (BI), Digital Citizenship Behavior (DCB), and Readiness (RED). Each path’s loading reflects the strength and direction of relationships, informed by the bootstrap results from Table 6, which indicates the statistical support for each pathway. Supported paths include EE → BI, EE → DCB, PE → BI, SI → BI, and SI → DCB, as evidenced by p values of less than 0.05, while other paths such as BI → DCB, BI → RED, and FC → BI did not achieve statistical significance. The solid arrow from DCB to RED represents the highly significant relationship between digital citizenship behavior and readiness.
Measurement models, also known as outer models, explain the relationships that exist between constructs and the indicators that are used to describe them. It defines how constructs are assessed or quantified through various indicators (Hair et al., 2018). Researchers must determine the reliability and validity of the indicators used in multivariate analysis to improve the accuracy of the measurement (Qureshi et al., 2023b).
Confirmatory factor analysis (CFA) is a statistical technique used to assess the validity of measurement instruments by examining the relationships between observed variables and latent constructs. In this study, CFA was used to validate the measurement instruments for the endogenous variables. The aim was to identify and eliminate unreliable items with weak loading values. The items having weak loading (preferably less than 0.6) were eliminated from the study (Hair et al., 2014). The items loading after the deletion of items with weak loadings are presented in Table 3. Furthermore, Cronbach’s alpha measure was used to examine the internal consistency reliability of the constructs. It assesses the extent to which items within a construct are consistent in measuring the underlying construct. Cronbach’s alpha values reported for the constructs in the study indicate varying levels of internal consistency but fall within the acceptable to excellent range.
The study further used the composite reliability measure to evaluate the internal consistency of the measurement instruments for the endogenous variables. Composite reliability is a statistical measure used to assess the internal consistency of measurement instruments in structural equation modeling (SEM). The coefficient values for composite reliability exceeded the recommended threshold of 0.7, as suggested by Gerbing and Anderson (1988), for all variables, thus confirmed the reliability of the constructs being measured.
The assessment of convergent validity was conducted by using the average variance extracted (AVE) measure (Table 4). According to the recommendation put forth by Chin (1998), it is necessary for the average variance extracted (AVE) values to exceed 0.5 in order to confirm the validity of the items. The average variance extracted (AVE) is a measure used to assess the level of variance captured by a construct compared with the variance due to measurement error. In this analysis, the AVE values for the constructs range from 0.556 to 0.918, thus confirmed the convergent validity of the constructs.
Furthermore, Fornell–Larcker criterion was used to examine the discriminant validity across the constructs (Table 4). The Fornell–Larcker criterion compares the square root of AVE values (diagonal values in the provided matrix) with the correlations between the constructs (off-diagonal values in the provided matrix), to assess discriminant validity (Fornell and Larcker, 1981). According to the criterion, the square root of AVE for each construct should be greater than its highest correlation with any other constructs. This condition appears to be met for all constructs. This suggests that each construct is indeed distinct and captures phenomena that are not captured by the other constructs in the model, affirming good discriminant validity across the constructs (Chin, 1998).
The heterotrait–monotrait ratio (HTMT) matrix is utilized to evaluate the discriminant validity of constructs within a structural equation model (Table 5). The majority of HTMT values fall below the commonly accepted threshold of 0.85, suggesting that the constructs (BI, DCB, EE, FC, PE, RED, and SI) are empirically separated from each other, therefore supporting their good discriminant validity. Some values in the range of 0.85 or somewhat higher (Henseler, 2017), such as EE and BI (0.877), may require additional investigation to confirm that the constructs are not excessively comparable.
Table 6 presents the cross-loading results, which is another method for assessing discriminant validity. Discriminant validity requires that items have higher loadings on their designated constructs compared with loadings on other constructs within the model (Henseler, 2017). In simpler terms, each item should have a stronger correlation with its intended construct than with any other constructs in the model. The results of the study indicate that the items exhibit higher loadings on their respective constructs than other constructs, indicating good discriminant validity.
After validating the measurement model, the next step is to assess the structural model, also known as the inner model. This involves examining the relationships between the constructs within the model. The assessment of the structural model includes estimating path coefficients using a bootstrapping procedure (Hair et al., 2017). The results of the path analysis are presented in Table 7. The findings indicate that there is a significant direct effect of digital citizenship behavior (DCB) on readiness (RED) (β = 0.675, t = 9.195, p = 0.000). This suggests that higher levels of digital citizenship behavior are associated with increased readiness. Furthermore, a statistically significant effect of effort expectancy (EE) (β = 0.243, t = 3.256, p = 0.001), performance expectancy (PE) (β = 0.529, t = 8.309, p = 0.000), and social influence (SI) (β = 0.258, t = 3.642, p = 0.000) was observed on behavioral intention (BI). These results indicate that individuals’ perceptions of effort expectancy, perceived ease of use, and social influence significantly influence their behavioral intentions. Additionally, a significant effect of effort expectancy (EE) (β = 0.306, t = 2.251, p = 0.024) and social influence (SI) (β = 0.329, t = 2.431, p = 0.015) was observed on digital citizenship behavior (DCB). This suggests that individuals’ perceptions of effort expectancy and social influence play a role in shaping their digital citizenship behavior.
Mediation analysis is a valuable method for examining and evaluating the indirect effects of independent variables on a dependent variable through one or more mediator variables. It allows researchers to understand the extent to which the impact of the independent variable is transmitted through the mediator variable to influence the dependent variable.
Table 8 presents the results from a bootstrap analysis of mediation model paths, showing regression coefficients, their variability, and statistical significance for each tested pathway. Most paths (indicated by abbreviations such as SI, BI, and DCB) are marked “not supported” due to high p values, suggesting that the effects are not statistically significant. However, two paths, “EE → DCB → RED” and “SI → DCB → RED,” show p values of below 0.05, marking them as “supported,” indicating statistically significant relationships in these specific routes. These findings highlight which specific mediation relationships within the model are valid and which are not valid, providing insights into the influential dynamics among the variables studied.
There are various approaches available for assessing mediation, with bootstrapping being the most contemporary method for mediation analysis (Madhavan, 2018; Rijnhart et al., 2021). In the current study, the researchers investigated the potential mediating effects of behavioral intention (BI) and digital citizenship behavior (DCB) on the exogenous and endogenous variables of interest. The results of the mediation analysis are shown in Table 8. The mediating effect of digital citizenship behavior (DCB) on effort expectancy (EE) and readiness (RED) (β = 0.206; t = 1.989; p = 0.047) was found to be statistically significant. Furthermore, the mediating effect of digital citizenship behavior (DCB) was found to be significant between social influence (SI) and readiness (RED) (β = 0222; t = 2.323; p = 0.020).
The study has developed a distinctive thesis on unifying the notions of creating behavioral intention and digital citizenship toward the readiness of adopting technology in the teaching environment in PAAET colleges in Kuwait. This dichotomous perspective provides originality to the research because most technology acceptance is studied alone using technology acceptance models with a focus on behavior intention only (Al-Fahim et al., 2016; Inayatulloh, 2020; Almaiah et al., 2022; Natasia et al., 2022; Nazir and Khan, 2022; Qader et al., 2022), whereas digital citizenship is a more ethical and responsible use of technology and has remained a separate set of literature in the information system debates related to ethical access, use, restriction, and rights (Sussan and Acs, 2017; Hawamdeh et al., 2022; Martin et al., 2022). Unifying both of these constructs as mediators and studying them through the same antecedents provide a useful and more holistic understanding of determining the readiness of both technological and ethical use of technology in teaching.
The results of the survey offer empirical information to evaluate 28 theories on these connections. The results validate eight noteworthy trajectories, underscoring the significance of elements, such as exertion, interpersonal impact, and output in defining faculty preparedness. On the other hand, the findings also refute 20 theories, which calls into question long-held beliefs about digital citizenship and technological acceptability, as discussed in this section.
First, the hypotheses which are confirmed are H3, H4, H5, H8, H10, H11, H16, and H28. Behavioral intentions are found to impact the faculty readiness to use technology in their teaching. This finding has a profound congruence with the current literature (Almaiah et al., 2022; Al-Shamali et al., 2022; Hradecky et al., 2022; Scherer et al., 2023; Al-Adwan et al., 2024), where behavioral intention has been found to be leading to readiness in multiple contexts and times (H3). Effort expectancy on the part of faculty feeling of how much effort is needed for using a technology is found to be impacting both behavioral intention (H4) and digital citizenship (H5). The effort expectancy also impacts the faculty readiness through their digital citizenship as a mediator (H16). The teaching faculty seems to consider efforts as important consideration because their times are usually tightly allocated into diverse tasks, such as teaching, administration, and evaluation. Therefore, they feel important to consider how much effort an application will need to create a behavioral intention and related digital citizenship.
Like effort expectancy, the social influence is also found to be impacting both behavioral intention (H10) and digital citizenship of the faculty (H11). This finding also has a profound agreement with the current literature (Alkandari et al., 2021; Avcı and Yıldız Durak, 2022; Brandau et al., 2022; Al-Sharhan et al., 2024). Teachers are inspired by their surroundings as they try to adapt to their social circumstances so that their teaching remains relevant and effective and they get influenced to establish a behavioral intention and be digital citizens by using technology. The study has also confirmed that the social influence proceeded further to impact the faculty readiness through digital citizenship as a mediator (H28). Furthermore, the study has also confirmed that the performance expectancy impacts the faculty’s behavioral intention. In connection to other hypotheses, this funding provides insights into the teaching mental focus of considering technology more as input and output considerations.
The study did not find significant evidence to support the behavioral intention of faculty affecting digital citizenship behavior (H1), readiness (H2), and readiness through digital citizenship as mediators (H23). These findings are contradictory to the literature but signify the importance of faculty considering both behavioral intention and digital citizenship as stand-alone constructs toward readiness. Similarly, the study also did not find significant evidence to support the effort expectancy impacting readiness when mediated by the behavioral intention and digital citizenship (H18) or through the mediation of behavioral intention alone (H27) (Al-Rahmi et al., 2022). In the same way, the findings also do not confirm the effort expectancy impacting digital citizenship through behavioral intention as mediation (H26).
The facilitating conditions are also not confirmed supporting the readiness when behavioral intention and digital citizenship are used as mediators (H14), digital citizenship as a mediator (H22), and behavioral intention as a mediator (H24). Similarly, no considerable evidence is found to support the facilitating conditions affecting behavioral intention (H6) and digital citizenship (H7) when using behavioral intention as a mediator (Shen et al., 2023).
The study also does not support the performance expectancy impacting readiness through behavioral intention (H13), behavioral intention, digital citizenship (H15), and digital citizenship (H19) as mediators (Hong et al., 2021). Similarly, no evidence has been found to support the performance expectancy impacting digital citizenship (H9) through behavioral intention as mediator (H17). Furthermore, the study has no evidence to support the social influence impacting digital citizenship through behavioral intention (H12) or readiness through behavioral intention (H20) or behavioral intention and digital citizenship (H25).
This study provides a rigorous evaluation of 28 hypotheses to provide an evaluation of how the technology readiness of faculty members in Kuwait colleges occurs through the dichotomous co-existence of behavioral intention and digital citizenship. The study, however, has a few limitations which open up future research possibilities.
First, behavioral intentions, particularly when combined with digital citizenship, create a limitation of the study in essence as ethics and morality are very much culturally different and may therefore generate differences in the results (AlMutairi and Yen, 2022). The study, however, took a very static causal assumption about digital citizenship.
Second, the context of teachers in PAAET colleges in Kuwait also brings a limitation to the study. The technology in the teaching environment is multi-faceted and usually co-used by both teachers and students. The readiness of one group can influence the other group. As the student’s readiness may need to be taken into consideration together to make sense of the teacher’s readiness as they are both co-creating the learning environment and the usage of technology.
Finally, the study has only accepted eight hypotheses and did not find support for the rest of the 20 hypotheses, even though there is literature that supports these hypotheses. The discussions and conclusions are therefore cautiously drawn as the sample size is not big enough and may be limiting the perspective established through the findings of this study.
The limitations discussed also provide many opportunities for expanding this study. First, in future studies, more contextual interpretations and cultural aspects may be more useful to research particularly in connection to digital citizenship being a more culturally sensitive area. Such cultural instances may expand the technology readiness debates as predominantly this study explains through common determinants with the behavioral intention of using technology. Second, to have a multi-faced perspective, the findings should be correlated with the testing of the hypothesis among the students. In addition, both teachers and students co-exist in a context of learning, and they interact through technology, so the readiness of one will affect the others. The results related to both parties in the learning context will be more comprehensive and realistic to evaluate if both samples are measured together. Third, the sample size in the study is only 122 participants, and larger samples in multiple locations may bring not only more generalizable findings but may also achieve more congruence with the current literature. Such studies can be built among the current results and evaluate if more significant evidence can be gathered to support the 20 hypotheses rejected in this study.
This study has profound implications and can provide a basis for the recommendations related to the crucial factors toward achieving higher effectiveness in terms of using technology by faculty members. The supported hypotheses are mainly related to the effort expectancy and performance expectancy impacting the mediating and dependent variables, thus leading to important recommendations as the faculty seem to be taking a more input and output perspective of the technology readiness.
First, recognizing the considerable impact of faculty members’ behavioral intentions on their preparedness to utilize technology in teaching and create strategies to improve and promote using technology in classrooms. Furthermore, conducting regular training sessions and workshops on the practical aspects of adopting technology into teaching by offering a variety of professional development opportunities, such as online courses, webinars, and self-paced modules. PAAET can also put in place a recognition program that recognizes and honors faculty members who excel in integrating technology. Additionally, they can invest in developing technologically advanced classrooms with access to cutting-edge equipment and software and create an environment in which experimentation with new technology is encouraged and acknowledged.
This study explains the determinants of faculty members’ readiness and how digital citizenship behavior and behavioral intention mediate these relationships to influence the readiness of faculty members in PAAET colleges to use technology. The study is a rare attempt to combine both behavioral intention and digital citizenship to broaden the horizon of faculty readiness in terms of not just their intention to use the technology but also establishing themselves as more ethically and morally aware to have more effective use of technology in teaching. The evidence, however, supports that more input-oriented factors such as effort expectancy and social influence and also more out-oriented factors such as performance expectancy are found to be important for the teachers to achieve readiness, which, in turn, would affect the effectiveness of using technology in higher education. These and other findings thus establish and support a more purposive approach to technology readiness as the faculty considers the effort inputs and performance outputs as linear causal frameworks for the use of technology. Future studies and the course of technology adoption may, however, bring alternate interpretations as the current findings are based on a limited number of teaching faculties in PAAET colleges in Kuwait and therefore cannot be widely generalized.
The raw data supporting the conclusions of this article will be made available by the authors, without undue reservation.
Ethical review and approval was not required for the study on human participants in accordance with the local legislation and institutional requirements. Written informed consent from the patients/participants or patients/participants legal guardian/next of kin was not required to participate in this study in accordance with the national legislation and the institutional requirements.
FA: Conceptualization, Resources, Writing – review & editing. AA: Formal analysis, Software, Supervision, Validation, Visualization, Writing – original draft, Writing – review & editing. AA-K: Investigation, Resources, Supervision, Validation, Writing – original draft, Writing – review & editing. BA: Methodology, Project administration, Resources, Writing – review & editing. SA: Investigation, Resources, Validation, Writing – original draft, Writing – review & editing.
The author(s) declare that no financial support was received for the research, authorship, and/or publication of this article.
The authors acknowledge the American University of Kuwait (AUK) for their support and Kuwait Technical College for ethical oversight, both of which were vital to this research.
The authors declare that the research was conducted in the absence of any commercial or financial relationships that could be construed as a potential conflict of interest.
All claims expressed in this article are solely those of the authors and do not necessarily represent those of their affiliated organizations, or those of the publisher, the editors and the reviewers. Any product that may be evaluated in this article, or claim that may be made by its manufacturer, is not guaranteed or endorsed by the publisher.
Al-Adwan, A. S., Meet, R. K., Anand, S., Shukla, G. P., Alsharif, R., and Dabbaghia, M. (2024). Understanding continuous use intention of technology among higher education teachers in emerging economy: evidence from integrated TAM, TPACK, and UTAUT model. Stud. High. Educ. 28, 1–20. doi: 10.1080/03075079.2024.2343955
Al-Fahim, N. H., Jusoh, W. J. W., and Abideen, A. (2016). An examination factors influencing the intention to adopt internet banking among SMES in Yemen: using an extension of the technology acceptance model (TAM). J. Internet Bank. Commer. 21, 1–23.
Alkandari, K. (2023). Transformation to competency-based curriculum: readiness and self-efficacy among Islamic studies teachers in Kuwait. Curric. Perspect. 43, 67–79. doi: 10.1007/s41297-022-00179-3
Alkandari, A., Alseddiqi, A., and Alsaber, A. A. R. (2021). Measuring instructor’s readiness on accepting the use of e-learning system during Covid-19 pandemic in higher education of Kuwait. J. Acad. Bus. Econ. 21, 29–40. doi: 10.18374/JABE-21-2.3
Alkuwaiti, S. A. A., Ahmad, A. N. A., and Mosali, N. A. (2023). Service quality factors influencing the use of artificial intelligent security technology in UAE. IJSCET 14, 76–87. doi: 10.30880/ijscet.2023.14.02.005
Almaiah, M. A., Al-Otaibi, S., Lutfi, A., Almomani, O., Awajan, A., Alsaaidah, A., et al. (2022). Employing the TAM model to investigate the readiness of M-learning system usage using SEM technique. Electronics 11:1259. doi: 10.3390/electronics11081259
Almakayeel, N., Buniya, M. K., Abubakar, A. S., Kamil, S. M., Qureshi, K. M., and Qureshi, M. R. N. M. (2023). Modelling the construction projects implementation barriers: a structure equation modelling approach. Buildings 13:1223. doi: 10.3390/buildings13051223
Al-Mamary, Y. H. S. (2022). Understanding the use of learning management systems by undergraduate university students using the UTAUT model: credible evidence from Saudi Arabia. Int. J. Info. Manag. Data Insights 2:100092. doi: 10.1016/j.jjimei.2022.100092
Almogren, A. S., and Aljammaz, N. A. (2022). The integrated social cognitive theory with the TAM model: the impact of M-learning in King Saud University art education. Front. Psychol. 13:1050532. doi: 10.3389/fpsyg.2022.1050532
AlMutairi, N., and Alharbi, E. (2022). The readiness of teachers in secondary schools in Kuwait to employ the ethics and values of the fourth industrial revolution. Education 49, 161–174. doi: 10.35516/edu.v49i1.711
AlMutairi, S., and Yen, D. (2022). An exploratory examination of the influence of national culture on cross national diffusion: a case study on the MENA region. Cog. Bus. Manag. 9:2016554. doi: 10.1080/23311975.2021.2016554
Al-Rahmi, A. M., Shamsuddin, A., Wahab, E., Al-Rahmi, W. M., Alturki, U., Aldraiweesh, A., et al. (2022). Integrating the role of UTAUT and TTF model to evaluate social media use for teaching and learning in higher education. Front. Public Health 10:905968. doi: 10.3389/fpubh.2022.905968
Al-Shamali, S., Al-Shamali, A., Alsaber, A., Al-Kandari, A., AlMutairi, S., and Alaya, A. (2022). Impact of organizational culture on academics’ readiness and behavioral intention to implement eLearning changes in Kuwaiti universities during COVID-19. Sustain. For. 14, 1–16. doi: 10.3390/su142315824
Al-Sharhan, A., Alsaber, A., Al Khasham, Y., Al Kandari, A., Nafea, R., and Setiya, P. (2024). The influence of governmental support on cyber-security adoption and performance: the mediation of cyber security and technological readiness. Int. J. Bus. Data Commun. Netw. 19, 1–16. doi: 10.4018/IJBDCN.341264
Anwar, R. N., and Alviayatun, T. (2022). The effect of performance expectancy, effort expectancy, social influence, facilitating conditions on mobile wallet adoption. Fair Value J. Ilmiah Akunt. Dan Keuang. 4, 2211–2223. doi: 10.32670/fairvalue.v4iSpesial%20Issue%205.2178
Avcı, Ü., and Yıldız Durak, H. (2022). Examination of digital citizenship, online information searching strategy and information literacy depending on changing state of experience in using digital technologies during COVID-19 pandemic. J. Inf. Sci. Eng. 01655515221114455:016555152211144. doi: 10.1177/01655515221114455
Brandau, M., Dilley, T., Schaumleffel, C., and Himawan, L. (2022). Digital citizenship among Appalachian middle schoolers: the common sense digital citizenship curriculum. Health Educ. J. 81, 157–169. doi: 10.1177/00178969211056429
Chin, W. W. (1998). “The partial least squares approach to structural equation modeling” in Modern Methods for Business Research. ed. G. A. Marcoulides (Mahwah, NJ: Lawrence Erlbaum).
Dehghan, H., Esmaeili, S. V., Paridokht, F., Javadzade, N., and Jalali, M. (2022). Assessing the students’ readiness for E-learning during the Covid-19 pandemic: a case study. Heliyon 8:e10219. doi: 10.1016/j.heliyon.2022.e10219
Figueroa-Armijos, M., Clark, B. B., and da Motta Veiga, S. P. (2023). Ethical perceptions of AI in hiring and organizational trust: the role of performance expectancy and social influence. J. Bus. Ethics 186, 179–197. doi: 10.1007/s10551-022-05166-2
Fornell, C., and Larcker, D. F. (1981). Evaluating structural equation models with unobservable variables and measurement error. J. Mark. Res. 18, 39–50. doi: 10.1177/002224378101800104
Gerbing, D. W., and Anderson, J. C. (1988). An updated paradigm for scale development incorporating Unidimensionality and its assessment. J. Mark. Res. 25, 186–192. doi: 10.1177/002224378802500207
Gharbi, H., Aliane, N., Al Falah, K. A., and Sobaih, A. E. E. (2022). You really affect me: the role of social influence in the relationship between procedural justice and turnover intention. Int. J. Environ. Res. Public Health 19, 51–62. doi: 10.3390/ijerph19095162
Gumz, J., Fettermann, D. C., Sant’Anna, Â. M. O., and Tortorella, G. L. (2022). Social influence as a major factor in smart meters’ acceptance: findings from Brazil. Results Eng. 15:100510. doi: 10.1016/j.rineng.2022.100510
Hair, J., Hollingsworth, C. L., Randolph, A. B., and Chong, A. Y. L. (2017). An updated and expanded assessment of PLS-SEM in information systems research. Ind. Manag. Data Syst. 117, 442–458. doi: 10.1108/IMDS-04-2016-0130
Hair, M., Ringle, C. M., Gudergan, S. P., and Sarstedt, M. (2018). Advanced Issues in Partial Least Squares Structural Equation Modeling. Thousand Oaks, CA: SAGE Publications, Inc.
Hair, J. F., Sarstedt, M., Hopkins, L., and Kuppelwieser, V. G. (2014). Partial least squares structural equation modeling (PLS-SEM): an emerging tool in business research. Eur. Bus. Rev. 26, 106–121. doi: 10.1108/EBR-10-2013-0128
Hawamdeh, M., Altınay, Z., Altınay, F., Arnavut, A., Ozansoy, K., and Adamu, I. (2022). Comparative analysis of students and faculty level of awareness and knowledge of digital citizenship practices in a distance learning environment: case study. Educ. Info. Technol. 27, 6037–6068. doi: 10.1007/s10639-021-10868-7
Hong, X., Zhang, M., and Liu, Q. (2021). Preschool teachers’ technology acceptance during the COVID-19: an adapted technology acceptance model. Front. Psychol. 12:691492. doi: 10.3389/fpsyg.2021.691492
Hooda, A., Gupta, P., Jeyaraj, A., Giannakis, M., and Dwivedi, Y. K. (2022). The effects of trust on behavioral intention and use behavior within e-government contexts. Int. J. Inf. Manag. 67:102553. doi: 10.1016/j.ijinfomgt.2022.102553
Hradecky, D., Kennell, J., Cai, W., and Davidson, R. (2022). Organizational readiness to adopt artificial intelligence in the exhibition sector in Western Europe. Int. J. Inf. Manag. 65:102497. doi: 10.1016/j.ijinfomgt.2022.102497
Inayatulloh, I. (2020). “Technology acceptance model (TAM) for the implementation of knowledge acquired model for SME” in 2020 International Conference on Information Management and Technology (ICIMTech), 767–770.
Kaluarachchi, S. (2023). Triangulating moderate impact of social media marketing communication between performance expectancy, effort expectancy and social influence on business performance in cashew industry in Sri Lanka. Research Square [Preprint]. doi: 10.21203/rs.3.rs-2838965/v1
Khechine, H., and Lakhal, S. (2018). Technology as a double-edged sword: from behavior prediction with UTAUT to students’ outcomes considering personal characteristics. Int. J. Inf. Commun. Technol. Educ. 17, 063–102. doi: 10.28945/4022
Lavidas, K., Komis, V., and Achriani, A. (2022). Explaining faculty members’ behavioral intention to use learning management systems. J. Comput. Educ. 9, 707–725. doi: 10.1007/s40692-021-00217-5
Luo, P., Guo, G., and Zhang, W. (2022). The role of social influence in green travel behavior in rural China. Transp. Res. Part D: Trans. Environ. 107:103284. doi: 10.1016/j.trd.2022.103284
Madhavan, M. (2018). A basic understanding to mediation analysis and statistical procedures in management research. J. Asia Pac. Stud. 5, 1–61.
Martin, F., Gezer, T., Wang, W. C., Petty, T., and Wang, C. (2022). Examining K-12 educator experiences from digital citizenship professional development. J. Res. Technol. Educ. 54, 143–160. doi: 10.1080/15391523.2020.1815611
Masso, M., Sim, J., Halcomb, E., and Thompson, C. (2022). Practice readiness of new graduate nurses and factors influencing practice readiness: a scoping review of reviews. Int. J. Nurs. Stud. 129:104208. doi: 10.1016/j.ijnurstu.2022.104208
Natasia, S. R., Wiranti, Y. T., and Parastika, A. (2022). Acceptance analysis of NUADU as e-learning platform using the technology acceptance model (TAM) approach. Proced. Comput. Sci. 197, 512–520. doi: 10.1016/j.procs.2021.12.168
Nazir, M. A., and Khan, M. R. (2022). Identification of roles and factors influencing the adoption of ICTs in the SMEs of Pakistan by using an extended technology acceptance model (TAM). Innov. Dev. 14, 189–215. doi: 10.1080/2157930X.2022.2116785
Purohit, S., Arora, R., and Paul, J. (2022). The bright side of online consumer behavior: continuance intention for mobile payments. J. Consum. Behav. 21, 523–542. doi: 10.1002/cb.2017
Qader, K. S., Jamil, D. A., Sabah, K. K., Anwer, S. A., Mohammad, A. J., Gardi, B., et al. (2022). The impact of technological acceptance model (TAM) outcome on implementing accounting software. Int. J. Eng. Bus. Manag. 6, 14–24. doi: 10.22161/ijebm.6.6.3
Qureshi, K. M., Mewada, B. G., Buniya, M. K., and Qureshi, M. R. N. M. (2023a). Analyzing critical success factors of lean 4.0 implementation in small and medium enterprises for sustainable manufacturing supply chain for industry 4.0 using PLS-SEM. Sustain. For. 15:5528. doi: 10.3390/su15065528
Qureshi, K. M., Mewada, B. G., Kaur, S., Alghamdi, S. Y., Almakayeel, N., Almuflih, A. S., et al. (2023b). Sustainable manufacturing supply chain performance enhancement through technology utilization and process innovation in industry 4.0: a SEM-PLS approach. Sustain. For. 15:15388. doi: 10.3390/su152115388
Ramírez-Correa, P., Grandón, E. E., Ramírez-Santana, M., Arenas-Gaitán, J., and Rondán-Cataluña, F. J. (2023). Explaining the consumption technology acceptance in the elderly post-pandemic: effort expectancy does not matter. Behav. Sci. 13:87. doi: 10.3390/bs13020087
Rijnhart, J. J. M., Lamp, S. J., Valente, M. J., MacKinnon, D. P., Twisk, J. W. R., and Heymans, M. W. (2021). Mediation analysis methods used in observational research: a scoping review and recommendations. BMC Med. Res. Methodol. 21:226. doi: 10.1186/s12874-021-01426-3
Scherer, R., Siddiq, F., Howard, S. K., and Tondeur, J. (2023). The more experienced, the better prepared? New evidence on the relation between teachers’ experience and their readiness for online teaching and learning. Comput. Hum. Behav. 139:107530. doi: 10.1016/j.chb.2022.107530
Shen, F., Liang, L., and Feng, Y. (2023). A study on factors influencing Chinese undergraduate EFL learners’ self-directed use of mobile English learning resources. Front. Psychol. 14:1189055. doi: 10.3389/fpsyg.2023.1189055
Sussan, F., and Acs, Z. J. (2017). The digital entrepreneurial ecosystem. Small Bus. Econ. 49, 55–73. doi: 10.1007/s11187-017-9867-5
Trivedi, S. K., Patra, P., Srivastava, P. R., Kumar, A., and Ye, F. (2022). Exploring factors affecting users’ behavioral intention to adopt digital technologies: the mediating effect of social influence. IEEE Trans. Eng. Manag. 99, 1–13. doi: 10.1109/TEM.2022.3182361
Tseng, T. H., Lin, S., Wang, Y.-S., and Liu, H.-X. (2022). Investigating teachers’ adoption of MOOCs: the perspective of UTAUT2. Interact. Learn. Environ. 30, 635–650. doi: 10.1080/10494820.2019.1674888
Undi-Phiri, B., and Phiri, J. (2022). Assessing factors affecting the adoption of E-government services in developing countries for transport sector, amidst the covid-19 pandemic. Commun. Netw. 14, 69–90. doi: 10.4236/cn.2022.142006
Keywords: digital citizenship, faculty, readiness, performance, Kuwait
Citation: Alkandari F, Alsaber A, Al-Kandari A, Alboloushi B and AlMutairi S (2024) Enhancing the effectiveness of digital transformation on teaching in higher education in Kuwait. Front. Educ. 9:1417062. doi: 10.3389/feduc.2024.1417062
Received: 13 April 2024; Accepted: 25 July 2024;
Published: 07 August 2024.
Edited by:
Cucuk Wawan Budiyanto, Sebelas Maret University, IndonesiaReviewed by:
Aris Budianto, Sebelas Maret University, IndonesiaCopyright © 2024 Alkandari, Alsaber, Al-Kandari, Alboloushi and AlMutairi. This is an open-access article distributed under the terms of the Creative Commons Attribution License (CC BY). The use, distribution or reproduction in other forums is permitted, provided the original author(s) and the copyright owner(s) are credited and that the original publication in this journal is cited, in accordance with accepted academic practice. No use, distribution or reproduction is permitted which does not comply with these terms.
*Correspondence: Ahmad Alsaber, YWFsc2FiZXJAYXVrLmVkdS5rdw==
Disclaimer: All claims expressed in this article are solely those of the authors and do not necessarily represent those of their affiliated organizations, or those of the publisher, the editors and the reviewers. Any product that may be evaluated in this article or claim that may be made by its manufacturer is not guaranteed or endorsed by the publisher.
Research integrity at Frontiers
Learn more about the work of our research integrity team to safeguard the quality of each article we publish.