- 1Department of Pathology, Microbiology and Forensic Medicine, School of Medicine, The University of Jordan, Amman, Jordan
- 2Department of Clinical Laboratories and Forensic Medicine, Jordan University Hospital, Amman, Jordan
- 3College of Dentistry, Gulf Medical University, Ajman, United Arab Emirates
- 4Department of Pharmaceutical Sciences, College of Pharmacy, Gulf Medical University, Ajman, United Arab Emirates
- 5Department of Pharmacology and Toxicology, Faculty of Pharmacy, Cairo University, Cairo, Egypt
- 6Department of Clinical Pharmacy and Therapeutics, Faculty of Pharmacy, Applied Science Private University, Amman, Jordan
- 7Department of Biomedical Sciences, School of Arts and Sciences, Lebanese International University, Bekaa, Lebanon
- 8Center for Applied Mathematics and Bioinformatics (CAMB), Gulf University for Science and Technology (GUST), Hawally, Kuwait
- 9Department of Public Health, Faculty of Communication, Arts and Sciences, Canadian University Dubai, Dubai, United Arab Emirates
- 10College of Health Sciences, Abu Dhabi University, Abu Dhabi, United Arab Emirates
- 11School of Medicine and Medical Sciences, Holy Spirit University of Kaslik, Jounieh, Lebanon
- 12Department of Psychology, College of Humanities, Effat University, Jeddah, Saudi Arabia
- 13Applied Science Research Center, Applied Science Private University, Amman, Jordan
- 14Department of Pharmacy Practice, College of Pharmacy, Gulf Medical University, Ajman, United Arab Emirates
Background: The use of ChatGPT among university students has gained a recent popularity. The current study aimed to assess the factors driving the attitude and usage of ChatGPT as an example of generative artificial intelligence (genAI) among university students in the United Arab Emirates (UAE).
Methods: This cross-sectional study was based on a previously validated Technology Acceptance Model (TAM)-based survey instrument termed TAME-ChatGPT. The self-administered e-survey was distributed by emails for students enrolled in UAE universities during September–December 2023 using a convenience-based approach. Assessment of the demographic and academic variables, and the TAME-ChatGPT constructs’ roles in ChatGPT attitude and usage was conducted using univariate followed by multivariate analyses.
Results: The final study sample comprised 608 participants, 91.0% of whom heard of ChatGPT while 85.4% used ChatGPT before the study. Univariate analysis indicated that a positive attitude to ChatGPT was associated with the three TAME-ChatGPT attitude constructs namely, lower perceived risks, lower anxiety, and higher scores on the attitude to technology/social influence. For the ChatGPT usage, univariate analysis indicated that positive attitude to ChatGPT use was associated with being male, Arab in nationality, and lower point grade average (GPA) as well as the four ChatGPT usage constructs namely, higher perceived usefulness, lower perceived risks of use, higher scores on the behavior/cognitive construct and higher scores on the ease-of-use construct. In multivariate analysis, only TAME-ChatGPT constructs explained the variance in attitude towards ChatGPT (80.8%) and its usage (76.9%).
Conclusion: The findings indicated that ChatGPT usage is commonplace among university students in the UAE. The determinants of use included the perceived usefulness, lower perceived risks, cognitive and behavioral factors, perceived ease of use, while the attitude was determined by lower perceived risks, lower anxiety, and higher scores for attitude to technology/social influence. These factors should be considered for understanding the motivators for successful adoption of genAI including ChatGPT in higher education.
1 Introduction
The integration of technology is becoming an indispensable component to improve the quality of higher education (Haleem et al., 2022; Criollo-C et al., 2023; Okoye et al., 2023). Recently, the incorporation of various generative artificial intelligence (genAI) models in education received a significant attention (Kamalov et al., 2023; King and Prasetyo, 2023; Mijwil et al., 2023; Yu and Guo, 2023). The genAI role in higher education represents a paradigm shift which could redefine the fundamental aspects of teaching and learning methodologies (Ouyang and Jiao, 2021; Yu, 2024).
The emergence of genAI exemplified by popular tools such as ChatGPT (OpenAI, San Francisco, CA), could mark a revolution rather than an evolution which could reshape the entire educational landscape (Caleb et al., 2023; Fütterer et al., 2023; Johnson, 2023). The potential educational benefits of genAI including ChatGPT especially in health education attracted significant research attention within a short time span (Ogunleye et al., 2024; Sallam, 2024; Sallam et al., 2024). Generative AI tools are characterized by a remarkable ability to understand and respond to natural language queries (Bandi et al., 2023). On one hand, these capabilities of genAI models offer innovative educational benefits. These benefits include enhancing personalized learning experiences and providing realistic simulations which would help to create an engaging educational content; thus, improving student engagement and learning outcomes (Kurtz et al., 2024; Salinas-Navarro et al., 2024a,b). For example, (Kıyak and Emekli, 2024) showed the efficiency of ChatGPT in generating medical multiple-choice questions (MCQs) in a recent review. However, the same tools, including ChatGPT, pose valid challenges and ethical concerns igniting controversy in aspects such as bias, cybersecurity, plagiarism and academic dishonesty (Michel-Villarreal et al., 2023; Sallam, 2023; Salazar et al., 2024; Williams, 2024).
Specifically, the concerns regarding genAI include but are not limited to the following aspects. First, a decline in the critical thinking and problem-solving skills can occur among students due to the over-reliance on technology (Sallam et al., 2023b). Second, variability in the ability to get access to novel technologies within and between different societies can put students lacking such an ability at a disadvantage creating a digital divide (Ragnedda and Muschert, 2013; Kitsara, 2022). Third, genAI integration into educational practices requires adaptation from the educators, who may display hesitancy or lack of the needed support due to perceived barriers or misconceptions (Karen et al., 2023; Ng et al., 2023; Barakat et al., 2024). Fourth, the rapid emergence and evolution of genAI models could surpass the pace of developing policies and regulations for successful implementation and responsible use of these tools (Chan, 2023; Dempere et al., 2023; Lim et al., 2023). In turn, this could potentially create significant challenges in establishing standardized practices for governing higher education. Fifth, the impact of genAI on the job market necessitates a thorough re-evaluation of the competencies and skills acquired during higher education to ensure the preparation of graduates capable to adapt in a rapidly changing work environment (Bukartaite and Hooper, 2023; Gupta, 2024; Tayan et al., 2024).
Educators and students have been shown to increasingly utilize genAI models with ChatGPT being among the most popular of these tools (Ansari et al., 2023; Ibrahim et al., 2023; von Garrel and Mayer, 2023; Abdaljaleel et al., 2024). Therefore, it is important to understand how these genAI models are perceived and utilized especially among students. Such an inquiry could be viewed as a critical factor for successful implementation of genAI models including ChatGPT into the educational framework (Chan and Hu, 2023; Sallam et al., 2024).
The implications of this research area are far-reaching. Understanding the factors driving the genAI adoption in higher education can inform the development of effective implementation strategies (Kamalov et al., 2023). Additionally, this area of research could shed light on the broader implications of genAI for the future of higher education and the job market (Michel-Villarreal et al., 2023). Such a quest involves the assessment of demographic, academic, psychological, social, and economic aspects driving the attitude towards this novel technology (Farina and Lavazza, 2023; Ibrahim et al., 2023; Zarifhonarvar, 2023; Abdaljaleel et al., 2024). In addition, this investigation can help to embrace genAI tools as constructive assets within educational settings, rather than viewing this inevitable technology as a challenge (de Winter et al., 2023).
A comprehensive framework for assessing the determinants of adopting a novel technology is the Technology Acceptance Model (TAM) (Davis, 1989; Bagozzi et al., 1992; Marangunić and Granić, 2015). Based on the TAM framework, a recently developed and validated instrument termed “TAME-ChatGPT” described several factors as drivers of the attitude to ChatGPT and its usage among university students (Sallam et al., 2023a). These factors include the perceived usefulness, behavioral and cognitive factors, general perceived risks and the perceived risks of use, the perceived ease of use, anxiety, attitude to technology and social influence (Sallam et al., 2023a).
Based on the TAME-ChatGPT tool, the current study aimed to unravel the factors driving the adoption of ChatGPT among university students in the United Arab Emirates (UAE). The UAE presents a unique setting for this investigation, given its diverse cultural composition and rapidly evolving higher education landscape with aspiration to achieve top tier quality in education (Badry, 2019). The UAE has placed AI at the core of its national agenda, establishing a deep commitment to embedding AI within its economic and technological strategies (Alkhaldi and Altaei, 2021; Shwedeh et al., 2024). A pioneering step in this direction was the early establishment of the Ministry of State for Artificial Intelligence, Digital Economy & Remote Work Applications. As the first entity of its kind worldwide, this ministry coordinates AI governance and policy, positioning the UAE as a leader in the global AI landscape (Dahabreh, 2023). Furthermore, the UAE hosts the world’s first dedicated AI research university at the graduate level, highlighting its strategic educational initiatives aimed at developing a proficient workforce to meet the demands of an AI-driven future (Science/AAAS Custom Publishing Office, 2023). The current study implications could help in contributing to the growing literature assessing the determinants of generative AI implementation in higher education. Additionally, this study sought to provide insights that can guide educators and academic policymakers regarding the students’ perspectives on ChatGPT which can consequently help to enrich their educational experience.
2 Methods
2.1 Study design
This study adopted a validated survey instrument based on the technology acceptance model (TAM) and specifically tailored to measure the attitude towards ChatGPT among university students (Sallam et al., 2023a). The survey instrument validity was confirmed in a recently published multinational study among university students in five Arab countries (Abdaljaleel et al., 2024).
This study utilized a self-administered electronic survey, distributed via email to university students in the UAE. The survey employed a non-probability, convenience sampling approach, hosted using Google Forms, and distributed by the authors based in the UAE (W.E., M.A.-S., W.G., N.A., and D.M.). The questionnaire was offered simultaneously in both Arabic and English languages to accommodate the linguistic preferences and cultural diversity among university students in the UAE. The survey was accessible from 20 September 2023 to 8 December 2023. Participation in the study was entirely voluntary, with no incentives for participation. To reduce the effect of non-response bias, responding to all items were mandatory for successful completion of the questionnaire with the exception of self-reported latest cumulative grade point average (GPA).
The minimum required sample size was calculated at 384 based on the formula: n = (Z2 × P × (1 − P))/e2, where: Z = 1.96 for 95% confidence interval (CI), P as the expected true proportion (set at 50%), and e as the desired precision (set at ±0.05), and the latest estimate of the total number of university students in the UAE as retrieved from the UAE Ministry of Education official website in the academic year 2019/2020 (The UAE Ministry of Education, 2024). Calculation of the minimum sample size was done using the EPITOOLS sample size to estimate a proportion or apparent prevalence with specified precision available from Epitools – Epidemiological Calculators (2024).
2.2 Ethical considerations
This study was approved by the Institutional Review Board (IRB) at Gulf Medical University (Reference number: IRB-COD-FAC-49-APRIL-2023). Obtaining the informed consent to participation was ensured by the inclusion of a mandatory item at the beginning of the electronic survey to explicitly indicate consent for participation.
2.3 Survey instrument
The electronic survey started with an introductory section which outlined the study objectives. This was followed by the mandatory informed consent item: “Do you agree to participate in this study?” Agreement with “yes” as an answer allowed the participant to proceed to the subsequent survey sections, whereas disagreement as indicated by “no” response resulted in closure of the survey.
The following section assessed the socio-demographic and academic data including the following variables: (1) age (as a scale variable); (2) sex (male vs. female); (3) nationality (Arab vs. non-Arab); (4) college/faculty affiliation (health-related (Health Sciences and Public Health colleges), vs. non-health-related (Art and Sciences, Law, Business, Engineering, Military, Electrical Engineering, Communication, Arts, and Sciences, and Social Sciences colleges)); (5) current educational level (undergraduate vs. postgraduate); and (6) the latest self-reported GPA (optional item), later classified into four categories as follows: <2.50, 2.50–2.99, 3.00–3.49, and 3.50–4.00.
The next section comprised two preliminary questions: first, “Have you heard of ChatGPT before the study?” (Yes vs. No), where a “No” response led to the survey submission. A “Yes” response led to the second question, “Have you used ChatGPT before the study?” (Yes vs. No). Respondents who had not used ChatGPT were directed to a set of 13 attitude TAME-ChatGPT scale questions, whereas those who had used ChatGPT proceeded to a comprehensive set of 25 TAME-ChatGPT items addressing both attitude to ChatGPT and its usage.
The survey items comprising the constructs of the TAME-ChatGPT, are outlined in Appendix. Each item was evaluated using a 5-point Likert scale, where “strongly agree” was scored as 5, “agree” as 4, “neutral/no opinion” as 3, “disagree” as 2, and “strongly disagree” as 1. For the TAME-ChatGPT items indicative of a negative attitude (perceived risk, anxiety, and perceived risk of use), the scoring was reversed.
The attitude scale encompassed three constructs: a perceived risk sub-scale with 5 items, an anxiety sub-scale with 3 items, and an attitude to technology/social influence sub-scale with 5 items. The usage scale comprised four constructs: perceived usefulness sub-scale with 6 items, behavior/cognitive factors sub-scale with 3 items, perceived risk of ChatGPT use sub-scale with 3 items, two of which were also present in the perceived risk construct, and perceived ease of use subs-scale with 2 items (Appendix).
2.4 Statistical and data analyses
Statistical analyses were conducted using IBM SPSS Statistics Version 26.0 (Armonk, NY: IBM Corp). The association between categorical variables was evaluated using the Chi-squared test (χ2). The Chi-squared test was selected for its effectiveness in determining statistical significance between categorical variables in a contingency table. This choice was supported by the sufficient sample size, which ensured that the expected frequency in each cell of the table was adequate. For the analysis involving categorical and scale variables, the Mann–Whitney U (M-W) and Kruskal-Wallis H (K-W) tests were employed. These non-parametric tests are appropriate for datasets where a normal distribution cannot be assumed. Specifically, the M-W test was utilized to compare two independent groups when the dependent variable was a scale variable that is not normally distributed. For comparisons involving more than two groups, the K-W test, which extends the M-W test, was applied. The selection of these tests was done following the determination of non-normal distribution of the scale variables via the Kolmogorov–Smirnov test (p < 0.001 for all). The level of statistical significance was determined at p < 0.050.
For the multivariate analysis, predictor variables were included based on p < 0.100 in univariate analysis. The selection of a less stringent threshold allowed an exploratory approach in model building for the identification of potentially important variables which could have been overlooked with a more conservative p value cutoff.
Multivariate regression analysis was employed to assess the influence of multiple predictors simultaneously, accounting for their interdependencies. The overall significance of the regression model was evaluated, which was crucial to determine whether the set of variables in the model significantly predicted the outcome variable, compared to a model with no predictors. This evaluation was reported as an F-test in the Analysis of Variance (ANOVA) table provided in regression analysis output, testing the null hypothesis that no relationship existed between the dependent and independent variables. To ensure the reliability of the regression analysis, the Variance Inflation Factor (VIF) was used to assess multicollinearity among predictors. A VIF value >3.0 was used as a conservative threshold to flag variables that might excessively inflate variances, which helped to prevent the inclusion of highly correlated variables that could distort the true relationship between predictors and the outcome.
Scores for each construct of the TAME-ChatGPT scale were calculated by dividing the total scores by the number of items within that construct, resulting in a score range of 1–5. The overall TAME-ChatGPT scores were based on the mean of the scores for scale items divided by the number of items in each scale. The scoring classification for both the TAME-ChatGPT and its individual constructs was categorized as follows: a score range of 1.00 to 2.33 indicated disagreement (negative), 2.34 to 3.67 indicated neutral position, and 3.68 to 5.00 indicated agreement (positive). The internal consistency of the seven TAME-ChatGPT constructs were ensured by the following Cronbach’s α values: perceived usefulness = 0.888, behavior/cognitive factors = 0.796, perceived risk of use = 0.638, perceived ease of use = 0.779, perceived risk = 0.846, anxiety = 0.867, and attitude to technology/social influence = 0.904. The Cronbach’s α value for the overall TAME-ChatGPT usage scale was 0.797, while the value for the overall attitude scale was 0.736. The calculated Cronbach’s α values indicated an acceptable level of consistency within the TAME-ChatGPT constructs (Tavakol and Dennick, 2011).
3 Results
3.1 General features of the study sample
A total of 608 responses were collected over the period 20 September 2023 to 8 December 2023. Most of the participants were females, less than 21 years in age, Arabs, enrolled in non-health-related colleges, and undergraduates. For age as a scale variable, the overall mean age of the participants was 20.9 ± 3.5 (median: 20, interquartile range (IQR): 19–22). The latest self-reported cumulative GPA data were available from 520 participants out of the 608 participants (85.5%). The vast majority of participants heard of ChatGPT (91.0%) or used ChatGPT before the study (85.4%, Table 1). Subsequent analysis was conducted among those who heard of ChatGPT for the attitude constructs (n = 553), and among those who indicated ChatGPT usage before the study for the usage constructs (n = 472).
3.2 Analysis of TAME-ChatGPT usage constructs
The highest average score for the TAME-ChatGPT usage constructs was observed for the ease of use construct with a mean score of 4.36 ± 0.74 followed by the perceived usefulness construct with a mean score of 3.97 ± 0.80, behavior/cognitive construct with a mean score of 3.73 ± 0.97, and finally the perceived risk of use construct with a mean score of 2.06 ± 0.77 (Figure 1).
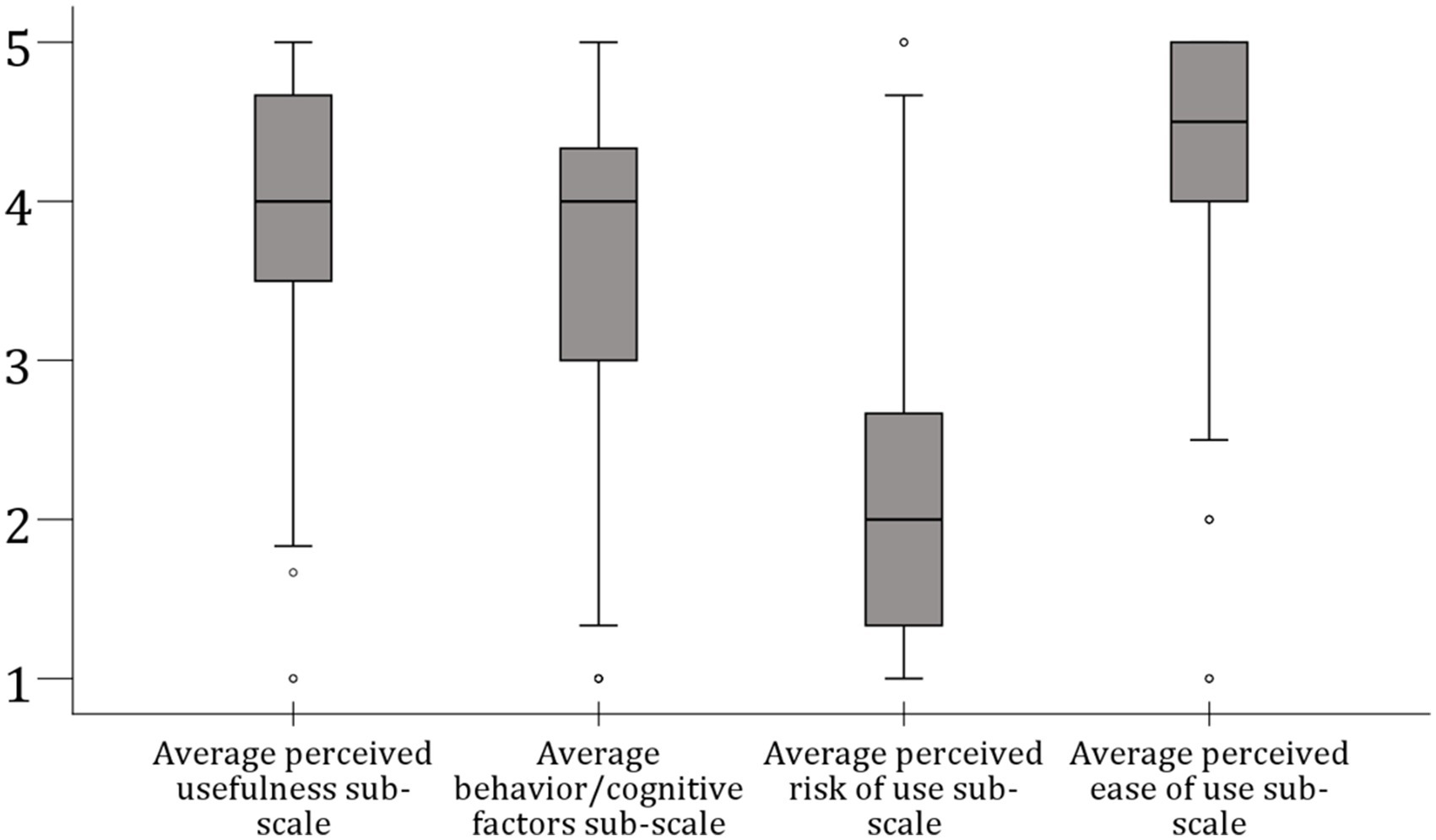
Figure 1. Box plots showing the distribution of scores across the four TAME-ChatGPT usage constructs.
Univariate analysis of the demographic factors associated with each TAME-ChatGPT usage construct revealed statistically significant higher scores among males, Arabs, participants in non-health-related colleges, and postgraduates that for both the perceived usefulness construct and the behavior/cognitive construct (Table 2).
3.3 Attitude towards ChatGPT based on TAME-ChatGPT constructs
The highest average score for the TAME-ChatGPT attitude sub-scales was observed for the technology/social influence construct with a mean score of 3.95 ± 0.82 followed by the perceived risk construct with a mean score of 2.08 ± 0.79, and finally the anxiety construct with a mean score of 2.07 ± 0.92 (Figure 2).
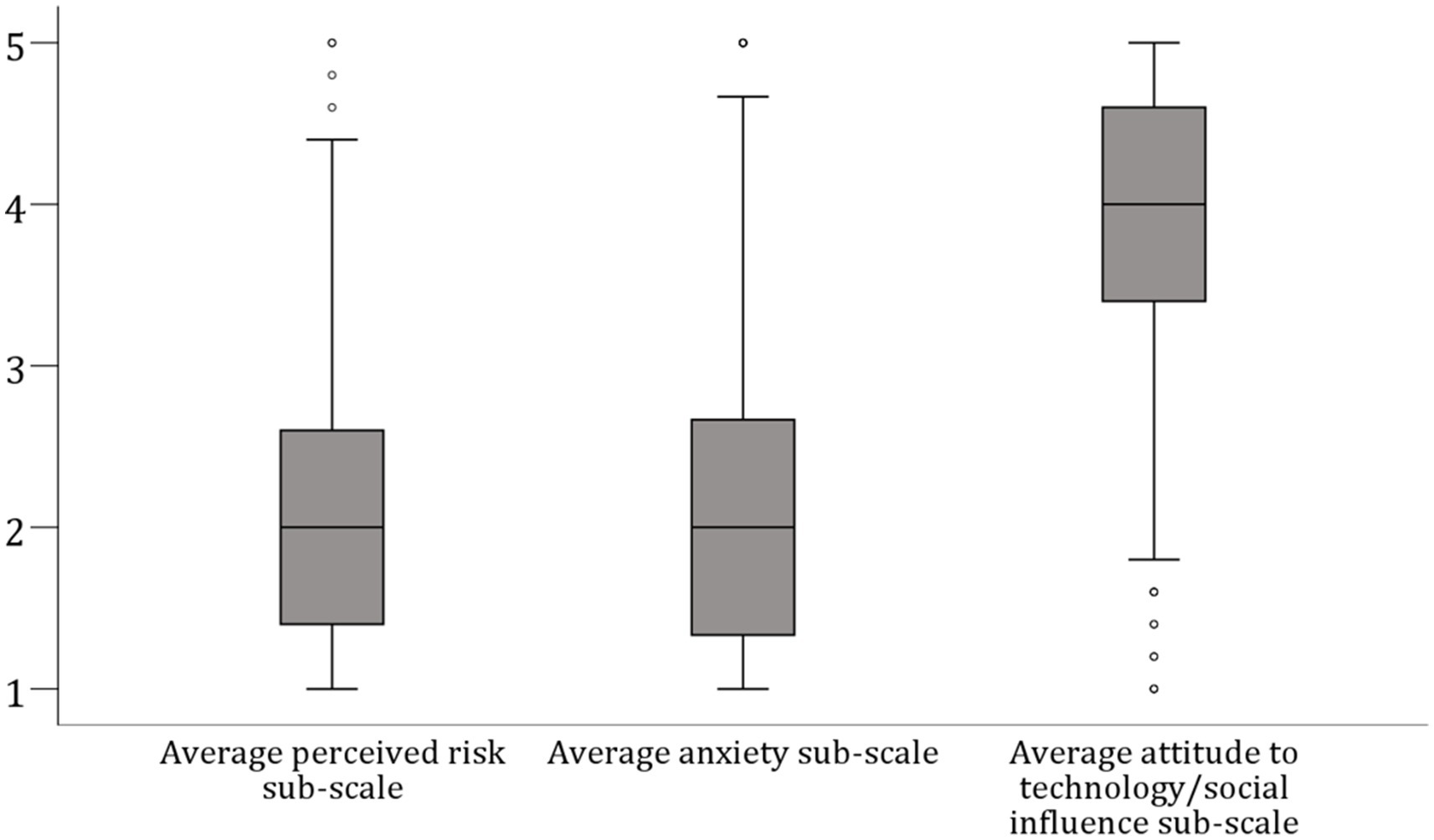
Figure 2. Box plots showing the distribution of scores across the three TAME-ChatGPT attitude constructs.
Univariate analysis of the demographic factors associated with each TAME-ChatGPT attitude constructs revealed statistically significant higher scores among males, Arabs, and participants in non-Health-related colleges for attitude to technology/social influence construct (Table 3).
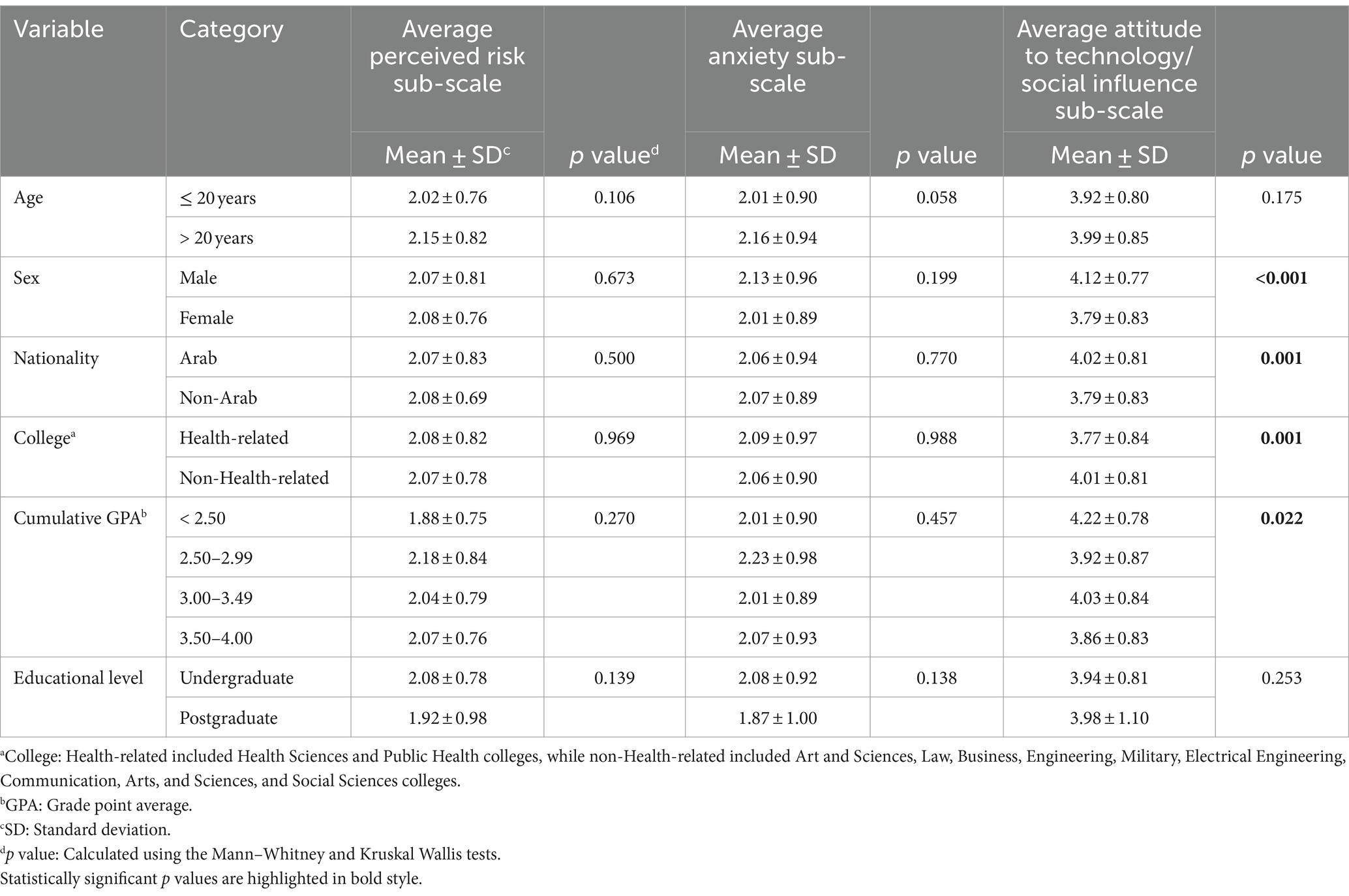
Table 3. The demographic determinants of attitude to ChatGPT based on TAME-ChatGPT attitude constructs.
3.4 Univariate analysis of the attitude and usage of ChatGPT based on TAME-ChatGPT constructs
To assess the overall factors influencing the usage of ChatGPT, univariate analysis revealed that the following demographic variables were significantly associated with higher ChatGPT usage scores: being a male, an Arab in nationality, and lower self-reported latest GPA (Table 4). Additionally, the four TAME-ChatGPT constructs were significantly associated with higher ChatGPT usage scores as follows: higher perceived usefulness, higher scores of the behavior/cognitive factors, lower perceived risk of use, and higher perceived ease of use (Table 4).
For the attitude towards ChatGPT, univariate analysis showed that the three TAME-ChatGPT constructs were the only variables having significant associations with the overall TAME-ChatGPT attitude score with lower perceived ChatGPT risk, lower anxiety, and higher scores on attitude to technology/social influence being linked with higher attitude scores (Table 5).
3.5 Multivariate analysis of the determinants of ChatGPT usage
The regression model for the predictors of ChatGPT usage showed a high degree of explanatory power with an R2 value of 0.774 indicating that 77.4% of the variation in the usage of ChatGPT were accounted for by the predictors included in the model. Nationality was the only demographic variable with a significant association with TAME-ChatGPT usage score (p = 0.025), suggesting that being Arab in nationality was linked with higher usage scores.
On the other hand, the four TAME-ChatGPT usage constructs were associated with the overall TAME-ChatGPT usage scores as follows: higher perceived usefulness (B = 0.398, p < 0.001), higher scores on the behavior/cognitive factors (B = 0.276, p < 0.001), lower perceived risk of use (B = 0.265, p < 0.001), and higher perceived ease of use scores (B = 0.368, p < 0.001, Table 6).
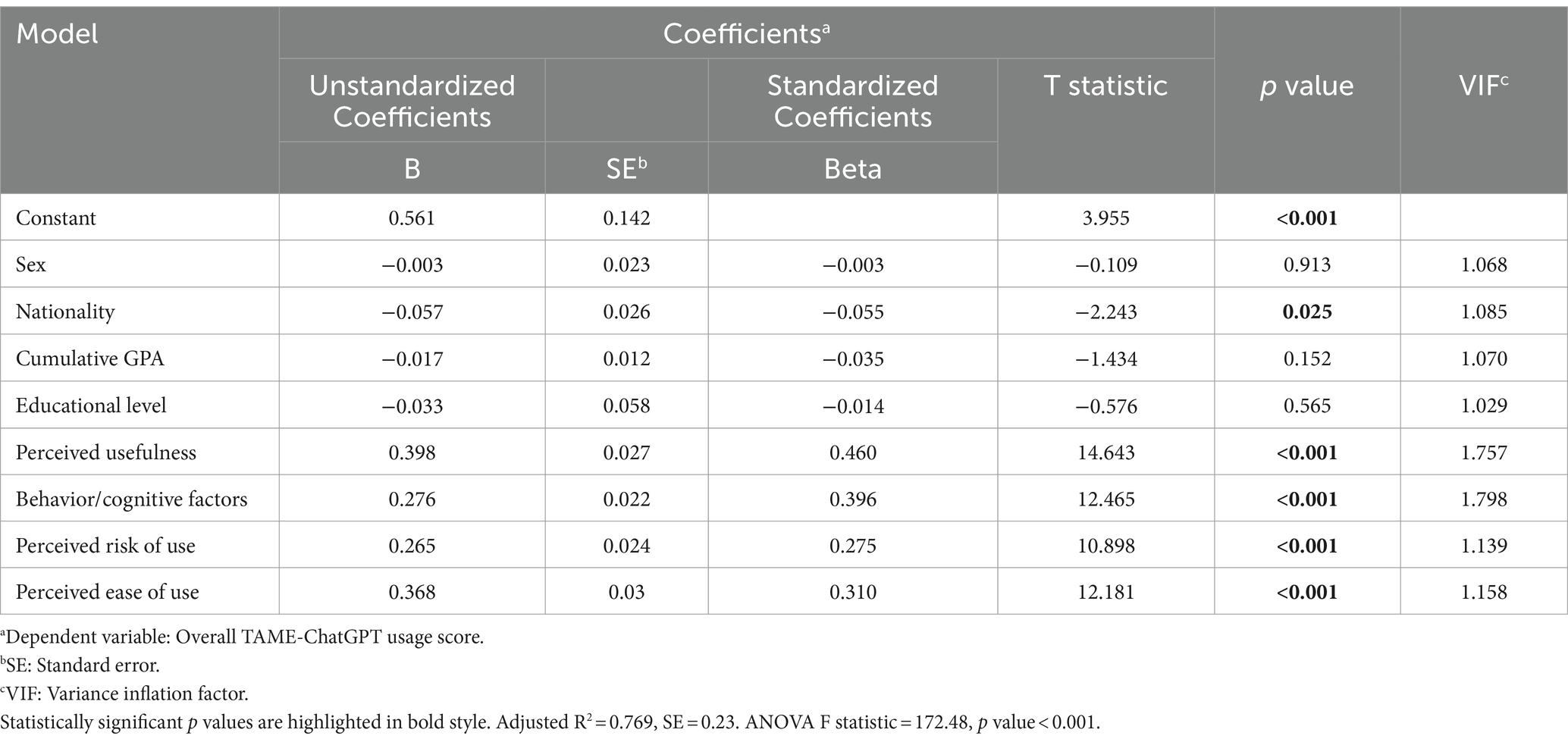
Table 6. Regression analysis of the predictors influencing ChatGPT usage based on the TAME-ChatGPT constructs.
3.6 Multivariate analysis of the determinants of attitude towards ChatGPT
For the predictors of the attitude towards ChatGPT, the regression model showed a high degree of explanatory power with an R2 value of 0.808. Using this model, the three TAME-ChatGPT attitude constructs were associated with the attitude scores as follows: lower perceived risk (B = 0.418, p < 0.001), lower anxiety scores (B = 0.479, p < 0.001), and higher scores in the attitude to technology/social influence construct (B = 0.413, p < 0.001, Table 7).
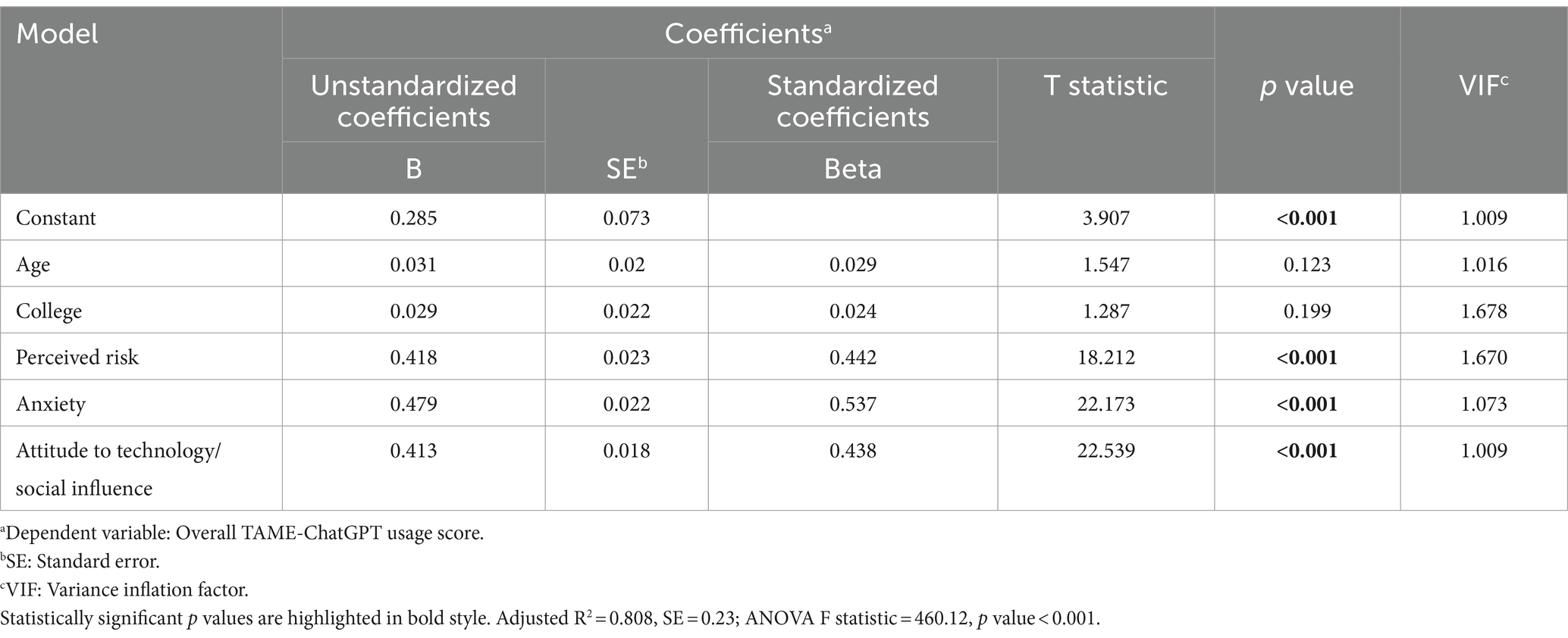
Table 7. Regression analysis of the predictors influencing the attitude towards ChatGPT based on the TAME-ChatGPT constructs.
4 Discussion
The current study highlighted a notable increase in the usage of ChatGPT among university students, with a substantial increase compared to earlier studies from different world regions. The findings of this study revealed that 85% of the participating university students in the UAE have already used ChatGPT. An early study conducted during February–March 2023 among university students in health schools in Jordan reported that only 11% used ChatGPT at the time (Sallam et al., 2023a). A subsequent multinational study that involved university students in five Arab countries (Iraq, Kuwait, Egypt, Lebanon, and Jordan) during April–August 2023 reported ChatGPT usage at a rate of 25% (Abdaljaleel et al., 2024).
In a culturally different context, a study that was conducted during June–July 2023 revealed that 39% of medical students across Germany, Austria, and Switzerland previously engaged with AI-based chatbots including ChatGPT (Weidener and Fischer, 2024). Another multinational study among academics and university students in Brazil, India, Japan, United Kingdom, and United States, which was conducted in January 2023 indicated that a majority of students intend to use ChatGPT for support in university assignments and anticipate that their peers would endorse its usage (Ibrahim et al., 2023). A recently published study among marketing students in India indicated that 309 out of 425 students were aware of ChatGPT (73%), with daily usage among 19% of the participants (Gulati et al., 2024). Taken together, these results highlight a noticeable rise in the adoption of ChatGPT among university students and its evolving status to become a normal practice within this demographic group.
In this study, the univariate analysis identified a positive correlation between the attitude to technology/social influence in the context of general attitude to ChatGPT and the following demographic groups: being an Arab student, being a male participant, and affiliation in non-health colleges. This association might indicate the interplay of cultural and demographic factors in the adoption of new technologies such as ChatGPT. For example, Arab students might exhibit more positive attitude to innovative AI technologies possibly due to its utility in overcoming language barriers (Chen, 2023; Mijwil et al., 2023b; Barwise et al., 2024). Nevertheless, this justification remains tentative considering the studies showing inferior performance of ChatGPT in non-English languages (Żammit, 2023; Liu et al., 2024; Sallam and Mousa, 2024). Sex also appeared to play a role in ChatGPT acceptance, with previous evidence suggesting that females may face more technical challenges and perceive greater risks when using technology compared to males (Goswami and Dutta, 2016; Cai et al., 2017). The more positive attitude observed among students from non-health disciplines can be attributed to their specific academic fields. University students in technology-related disciplines might be more inclined towards embracing new technologies such as ChatGPT which is influenced by both the curricular content and personal interests. This notion is supported by a study by Margaryan et al. (2011), which found that engineering students were more likely to use technology tools for various purposes compared to social work students.
The univariate analysis of the usage constructs of TAME-ChatGPT in this study revealed the following findings. The lower perceived risk of ChatGPT use and higher agreement with behavior/cognitive factors, indicative of an instinctive impulse to utilize this novel technology, were associated with being male, Arab, enrolled in non-health colleges, and having a lower GPA. These associations may point to a greater tendency among these groups to embrace new technologies, likely influenced by a combination of cultural/societal norms and prior educational experiences as well as the attitudes towards the perceived risk.
The propensity of postgraduate students to exhibit these characteristics could be related to their extended experience with a variety of technologies. Consequently, this extended exposure could result in a more readiness to accept and engage with innovative technological tools such as ChatGPT. Likewise, students in non-health disciplines especially in technology-related colleges might be more regularly exposed to emerging technologies. Consequently, this exposure would lead to increased familiarity with novel technologies and a lower level of perceived risks. Additionally, the previous academic experience with novel technologies among these students could result in an innate readiness to engage with technological advancements such as ChatGPT.
The correlation of lower perceived risk from ChatGPT and more agreement with cognitive/behavioral factors with lower GPA categories was an interesting finding in this study. One possible explanation is that university students with lower academic performance are more inclined to experiment with novel technologies such as ChatGPT as a compensatory mechanism to improve their academic achievements. Another explanation could be related to the higher propensity to explore innovative tools including ChatGPT as a result of the lower level of perceived constraints of academic rigor. In all cases, this particular observation warrants further investigation to understand the underlying motivations and implications of ChatGPT adoption among university students with varying academic performances which may require tailoring the adoption of ChatGPT to the individual student needs.
In this study, the perceived usefulness of ChatGPT, behavioral/cognitive factors, perceived risk associated with ChatGPT usage, and the perceived ease of using ChatGPT were all significantly correlated with the overall ChatGPT usage score. Concerning the overall TAME-ChatGPT attitude score, lower perceived risk and anxiety were associated with more favorable attitudes towards ChatGPT, alongside a positive attitude towards technology and social influence. These findings contribute an additional evidence to the growing literature emphasizing the significance of various constructs in technology acceptance assessment tools, such as the TAM and the Unified Theory of Acceptance and Use of Technology (UTAUT2) in the adoption of ChatGPT in various settings (Foroughi et al., 2023; Habibi et al., 2023; Jo and Bang, 2023; Yilmaz et al., 2023). In turn, this can help to guide evidence-based strategies to govern ChatGPT use among other genAI models in higher education (Veras et al., 2023; Grájeda et al., 2024).
For example, a study among Polish university students using UTAUT2 found that habit was the most influential factor on behavioral intention in the context of ChatGPT use, followed by performance expectancy and hedonic motivation (Strzelecki, 2023). Moreover, behavioral intention had the most substantial effect on ChatGPT usage behavior, followed by habit and facilitating conditions (Strzelecki, 2023). Our results align with these findings, particularly regarding behavioral/cognitive factors, which are reflected in items indicating previous use of similar tools and frequent utilization of ChatGPT in university assignments. Performance expectations in our study were analogous to the perceived usefulness construct, while facilitating conditions are comparable to our perceived ease of use construct highlighting recurrence of similar themes for the factors driving the acceptance of ChatGPT as an innovative tool in higher education (Gupta and Yang, 2024). The perceived ease of use is particularly an important driver for the wide popularity of ChatGPT with a user-friendly interface and little technical requirements (Shaikh et al., 2023; Albayati, 2024). In turn, this perceived ease of use would render ChatGPT more appealing for students to try and continue using.
In line with our findings, the importance of usefulness and ease of use has been shown in a recent study by Almogren et al. (2024) among a group of undergraduate and postgraduate university students. Additionally, the effectiveness of AI tools was an important predictor of its acceptance and use among university students in a recent study conducted in Malaysia and Pakistan (Dahri et al., 2024). Moreover, effectiveness has been shown to positively influence ChatGPT usage frequency as shown in a recent study in a different context by de Winter et al. (2024). The importance of usefulness has also been shown through its influence on user satisfaction in a recent study addressing AI chatbots user experience (Xing and Jiang, 2024). Furthermore, the central role of perceived usefulness and ease of use of ChatGPT in learning has been demonstrated in a study involving nursing students (Savellon et al., 2024).
The significance of perceived risks in ChatGPT use, which includes concerns about cybersecurity (Mijwil et al., 2023a), bias (Ray, 2023), and inaccuracies (Borji, 2023; Sallam, 2023), was a critical determinant of both attitude to ChatGPT and its usage in this study. This finding was consistent with a recent qualitative study which utilized the UTAUT model and highlighted the role of privacy concerns, performance expectancy, effort expectancy, social influence, and facilitating conditions in driving engagement with ChatGPT (Menon and Shilpa, 2023). This suggests that university students’ collective perceptions of both benefits and risks posed by technologies such as ChatGPT play a key role to shape their engagement and adoption of this novel technology (Chan and Hu, 2023; Abdaljaleel et al., 2024).
In this study, multivariate regression analyses provided comprehensive insights into the predictors of ChatGPT usage, and attitudes as modeled by the TAME-ChatGPT constructs. The complex nature of ChatGPT perceptions and adoption were determined by several factors including the psycho-social determinants manifested in anxiety, behavior/cognitive and social influence constructs besides the individual perception of usefulness, usability, and perception of risks. Notably, the demographic and academic variables (e.g., age, sex, college, GPA) were not significant predictors of ChatGPT attitude or usage. This result suggests that practical aspects of this novel technology such as the usefulness, user-friendly nature, and potential risks were more impactful in determining usage than demographic attributes. Thus, the primary drivers of ChatGPT usage and attitudes are mainly rooted in students’ psycho-social predispositions. These findings suggest that if ChatGPT among other genAI models are to be integrated in higher education, there is a necessity for strategies to enhance the perceived usefulness and ease of use for these models. Additionally, addressing the students’ anxiety and perceived risks is required for positive engagement with genAI technology in education. Nevertheless, the demographic and academic variables can also be considered to achieve an intricate understanding of attitude and use of ChatGPT among university students.
Based on the results of this study, it is crucial for higher education institutions, policymakers, and educators to formulate new educational strategies to accommodate the transformative AI changes. These strategies should highlight the utility and accessibility of genAI tools and proactively address the potential apprehensions that students might encounter as shown recently by Oluwadiya et al. (2023). To enhance the perception of ease and usefulness of genAI models, higher education institutions are advised to launch AI integration initiatives that include raising awareness regarding the benefits of these tools in education (Ivanov et al., 2024). These initiatives should also focus on providing training sessions, tutorials, and practical, hands-on experiences for students and faculty alike to explore the full potential of genAI in education (Chiu, 2024). Furthermore, integrating these genAI tools into the curriculum can directly benefit learning and research by demonstrating their real-world applications (Sheikh Faisal et al., 2024). These applications include generating content for study materials, or simulating complex concepts, thereby enriching the educational experience (Yu, 2024). The better usability of ChatGPT compared to web-based tools in health education has been suggested in a recent research protocol by Veras et al. (2023). In another aspect, addressing psychological barriers such as anxiety and perceived risks associated with genAI technology is essential and can be effectively managed by shifting the cultural dynamics within educational institutions (Yusuf et al., 2024). Programs designed to familiarize students and faculties with genAI tools can facilitate the integration of this novel technology into higher education and alleviate fears, similar to initiatives seen with the introduction of digital learning tools in the past decade as reviewed recently by Fernández et al. (2023).
Although demographic and academic variables may not emerge as primary drivers in genAI adoption, their influence on technology interaction should not be overlooked. Tailored educational practices that consider these factors can significantly enhance genAI adoption rates. By acknowledging these subtle differences, educational policies can better accommodate a diverse students’ strata, ensuring that the benefits of genAI are accessible to all students, regardless of their background (Sheikh Faisal et al., 2024). These strategic approaches can facilitate the adoption of genAI technologies and maximize their potential to enrich learning experiences and outcomes. Subsequently, this can help to prepare students to operate effectively in an increasingly digital AI-driven world (George, 2023).
The results of this study highlighted several areas for future research to enhance the collective understanding of genAI effects in different educational contexts. For example, longitudinal studies are essential to assess the long-term impact of genAI on learning outcomes and experiences, tracking changes in students’ perceptions and academic performance over extended periods. Additionally, experimental designs like randomized controlled trials (RCTs) are recommended to establish causal links between genAI usage and educational outcomes, building on recent protocols such as the one conceived by Veras et al. (2023). Investigating genAI influence across different academic disciplines would also be beneficial, which would help to develop tailored integration strategies that address the specific needs and challenges for various academic disciplines. Moreover, considering the significant role of cultural context in technology adoption, cross-cultural studies could examine how different settings influence genAI acceptance and effectiveness. Lastly, with ongoing concerns about the ethical implications and risks of genAI, further research should focus on these areas, particularly privacy, data security, and bias, to ensure responsible and ethical use of genAI for students and educational institutions.
Finally, it is important to consider the findings of this study in light of several limitations as follows. The convenience sampling approach utilized with the inherent selection bias could limit the representativeness of the sample and generalizability of the findings. The selection bias is also expected considering the electronic distribution of the survey among students. An element of bias should be considered as well in light of more inclination of the students who previously engaged with ChatGPT or heard of it to participate in the study and express their opinions. Finally, the reliance on self-reported data could result in self-reporting bias.
5 Conclusion
This study elucidated the determinants of ChatGPT adoption among university students in the UAE. Addressing these factors could help to exploit ChatGPT potential for better learning experience and to help equip university students to responsibly use the current and future technological innovations. Students’ familiarity with ChatGPT can provide an opportunity for genAI integration in higher education curricula and teaching methods.
The study highlighted the central role of individual and psycho-social factors as modeled in the TAME-ChatGPT constructs as significant factors driving the attitude towards ChatGPT and its usage. These insights can help higher education institutions, policymakers, and educators to formulate clear initiatives and guidelines that would help students in circumventing the ethical and practical aspects of genAI tool adoption in higher education.
Data availability statement
The original contributions presented in the study are included in the article/supplementary material, further inquiries can be directed to the corresponding authors.
Ethics statement
This study was approved by the Institutional Review Board (IRB) at Gulf Medical University (Reference number: IRB-COD-FAC-49-APRIL-2023). Obtaining the informed consent to participation was ensured by the inclusion of a mandatory item at the beginning of the electronic survey to explicitly indicate consent for participation. The studies were conducted in accordance with the local legislation and institutional requirements. The participants provided their written informed consent to participate in this study.
Author contributions
MS: Conceptualization, Data curation, Formal analysis, Investigation, Methodology, Resources, Software, Supervision, Validation, Writing – original draft, Writing – review & editing. WE: Data curation, Investigation, Methodology, Writing – review & editing. MA-S: Data curation, Investigation, Methodology, Writing – review & editing. MB: Data curation, Investigation, Methodology, Writing – review & editing. SE: Data curation, Investigation, Methodology, Writing – review & editing. WG: Data curation, Investigation, Methodology, Writing – review & editing. NA: Data curation, Investigation, Methodology, Writing – review & editing. SH: Data curation, Investigation, Methodology, Writing – review & editing. DM: Data curation, Investigation, Methodology, Project administration, Supervision, Validation, Writing – review & editing.
Funding
The author(s) declare that no financial support was received for the research, authorship, and/or publication of this article.
Conflict of interest
The authors declare that the research was conducted in the absence of any commercial or financial relationships that could be construed as a potential conflict of interest.
The author(s) declared that they were an editorial board member of Frontiers, at the time of submission. This had no impact on the peer review process and the final decision.
Publisher’s note
All claims expressed in this article are solely those of the authors and do not necessarily represent those of their affiliated organizations, or those of the publisher, the editors and the reviewers. Any product that may be evaluated in this article, or claim that may be made by its manufacturer, is not guaranteed or endorsed by the publisher.
References
Abdaljaleel, M., Barakat, M., Alsanafi, M., Salim, N. A., Abazid, H., Malaeb, D., et al. (2024). A multinational study on the factors influencing university students’ attitudes and usage of ChatGPT. Sci. Rep. 14:1983. doi: 10.1038/s41598-024-52549-8
Albayati, H. (2024). Investigating undergraduate students' perceptions and awareness of using ChatGPT as a regular assistance tool: a user acceptance perspective study. Comput. Educ.: Artif. Intell. 6:100203. doi: 10.1016/j.caeai.2024.100203
Alkhaldi, F. K., and Altaei, S. (2021). “Emirates leading experience in employing artificial intelligence” in The fourth industrial revolution: Implementation of artificial intelligence for growing business success. eds. A. Hamdan, A. E. Hassanien, A. Razzaque, and B. Alareeni (Cham: Springer International Publishing), 241–251.
Almogren, A. S., Al-Rahmi, W. M., and Dahri, N. A. (2024). Exploring factors influencing the acceptance of ChatGPT in higher education: a smart education perspective. Heliyon 10:e31887. doi: 10.1016/j.heliyon.2024.e31887
Ansari, A. N., Ahmad, S., and Bhutta, S. M. (2023). Mapping the global evidence around the use of ChatGPT in higher education: a systematic scoping review. Educ. Inf. Technol. 29, 11281–11321. doi: 10.1007/s10639-023-12223-4
Badry, F. (2019). “Expanding the UAE’s higher education horizon: path toward a sustainable future” in Education in the United Arab Emirates: Innovation and transformation. ed. K. Gallagher (Singapore: Springer Singapore), 57–73.
Bagozzi, R. P., Davis, F. D., and Warshaw, P. R. (1992). Development and test of a theory of technological learning and usage. Hum. Relat. 45, 659–686. doi: 10.1177/001872679204500702
Bandi, A., Adapa, P. V., and Kuchi, Y. E. (2023). The power of generative AI: a review of requirements, models, input–output formats, evaluation metrics, and challenges. Future Internet 15:260. doi: 10.3390/fi15080260
Barakat, M., Salim, N. A., and Sallam, M. (2024). Perspectives of university educators regarding ChatGPT: a validation study based on the technology acceptance model. Res. Sq. doi: 10.21203/rs.3.rs-3919524/v1
Barwise, A. K., Curtis, S., Diedrich, D. A., and Pickering, B. W. (2024). Using artificial intelligence to promote equitable care for inpatients with language barriers and complex medical needs: clinical stakeholder perspectives. J. Am. Med. Inf. Assoc. 31, 611–621. doi: 10.1093/jamia/ocad224
Borji, A. (2023). A categorical archive of ChatGPT failures. Res. Sq. doi: 10.21203/rs.3.rs-2895792/v1
Bukartaite, R., and Hooper, D. (2023). Automation, artificial intelligence and future skills needs: an Irish perspective. Eur. J. Train. Dev. 47, 163–185. doi: 10.1108/EJTD-03-2023-0045
Cai, Z., Fan, X., and Du, J. (2017). Gender and attitudes toward technology use: a meta-analysis. Comput. Educ. 105, 1–13. doi: 10.1016/j.compedu.2016.11.003
Caleb, K., Lenny, W., Dazzy, I., and Azra, E. (2023). The impact of AI on teaching and learning. London J. Soc. Sci. 6, 124–129. doi: 10.31039/ljss.2023.6.111
Chan, C. K. Y. (2023). A comprehensive AI policy education framework for university teaching and learning. Int. J. Educ. Technol. High. Educ. 20:38. doi: 10.1186/s41239-023-00408-3
Chan, C. K. Y., and Hu, W. (2023). Students’ voices on generative AI: perceptions, benefits, and challenges in higher education. Int. J. Educ. Technol. High. Educ. 20:43. doi: 10.1186/s41239-023-00411-8
Chen, T. J. (2023). ChatGPT and other artificial intelligence applications speed up scientific writing. J. Chin. Med. Assoc. 86, 351–353. doi: 10.1097/jcma.0000000000000900
Chiu, T. K. F. (2024). Future research recommendations for transforming higher education with generative AI. Comput. Educ.: Artif. Intell. 6:100197. doi: 10.1016/j.caeai.2023.100197
Criollo-C, S., Govea, J., Játiva, W., Pierrottet, J., Guerrero-Arias, A., Jaramillo-Alcázar, Á., et al. (2023). Towards the integration of emerging technologies as support for the teaching and learning model in higher education. Sustain. For. 15:6055. doi: 10.3390/su15076055
Dahabreh, F. (2023) The continued usage of artificial intelligence in the United Arab Emirates public sector organisations: An extended information system success model. Northumbria University.
Dahri, N. A., Yahaya, N., Al-Rahmi, W. M., Vighio, M. S., Alblehai, F., Soomro, R. B., et al. (2024). Investigating AI-based academic support acceptance and its impact on students’ performance in Malaysian and Pakistani higher education institutions. Educ. Inf. Technol. doi: 10.1007/s10639-024-12599-x
Davis, F. D. (1989). Perceived usefulness, perceived ease of use, and user acceptance of information technology. MIS Q. 13, 319–340. doi: 10.2307/249008
de Winter, J., Dodou, D., and Eisma, Y. B. (2024). Personality and acceptance as predictors of ChatGPT use. Discov. Psychol. 4:57. doi: 10.1007/s44202-024-00161-2
de Winter, J. C. F., Dodou, D., and Stienen, A. H. A. (2023). ChatGPT in education: empowering educators through methods for recognition and assessment. Informatics 10:87. doi: 10.3390/informatics10040087
Dempere, J., Modugu, K., Hesham, A., and Ramasamy, L. K. (2023). The impact of ChatGPT on higher education. Front. Educ. 8:1206936. doi: 10.3389/feduc.2023.1206936
Epitools – Epidemiological Calculators (2024) Sample size to estimate a proportion or apparent prevalence with specified precision. Available at: https://epitools.ausvet.com.au/oneproportion (Accessed January 26, 2024)
Farina, M., and Lavazza, A. (2023). ChatGPT in society: emerging issues. Front. Artif. Intell. 6:1130913. doi: 10.3389/frai.2023.1130913
Fernández, A., Gómez, B., Binjaku, K., and Meçe, E. K. (2023). Digital transformation initiatives in higher education institutions: a multivocal literature review. Educ. Inf. Technol. 28, 12351–12382. doi: 10.1007/s10639-022-11544-0
Foroughi, B., Senali, M. G., Iranmanesh, M., Khanfar, A., Ghobakhloo, M., Annamalai, N., et al. (2023). Determinants of intention to use ChatGPT for educational purposes: findings from PLS-SEM and fsQCA. Int. J. Hum.-Comput. Interact., 1–20. doi: 10.1080/10447318.2023.2226495
Fütterer, T., Fischer, C., Alekseeva, A., Chen, X., Tate, T., Warschauer, M., et al. (2023). ChatGPT in education: global reactions to AI innovations. Sci. Rep. 13:15310. doi: 10.1038/s41598-023-42227-6
George, A. S. (2023). Preparing students for an AI-driven world: rethinking curriculum and pedagogy in the age of artificial intelligence. Partners Univers. Innovative Res. Publ. 1, 112–136. doi: 10.5281/zenodo.10245675
Goswami, A., and Dutta, S. (2016). Gender differences in technology usage—a literature review. Open J. Bus. Manage. 4, 51–59. doi: 10.4236/ojbm.2016.41006
Grájeda, A., Burgos, J., Córdova, P., and Sanjinés, A. (2024). Assessing student-perceived impact of using artificial intelligence tools: construction of a synthetic index of application in higher education. Cogent Educ. 11:2287917. doi: 10.1080/2331186X.2023.2287917
Gulati, A., Saini, H., Singh, S., and Kumar, V. (2024). Enhancing learning potential: investigating marketing students’ behavioral intentions to adopt ChatGPT. Mark. Educ. Rev. 1-34, 1–34. doi: 10.1080/10528008.2023.2300139
Gupta, V. P. (2024). “AI-driven skill development: bridging students with industry 5.0” in Infrastructure possibilities and human-centered approaches with industry 5.0. eds. M. A. Khan, R. Khan, P. Praveen, A. R. Verma, and M. K. Panda (Hershey, PA, USA: IGI Global), 56–68.
Gupta, V., and Yang, H. (2024). Generative artificial intelligence (AI) technology adoption model for entrepreneurs: case of ChatGPT. Internet Ref. Serv. Q. 28, 223–242. doi: 10.1080/10875301.2023.2300114
Habibi, A., Muhaimin, M., Danibao, B. K., Wibowo, Y. G., Wahyuni, S., and Octavia, A. (2023). ChatGPT in higher education learning: acceptance and use. Comput. Educ.: Artif. Intell. 5:100190. doi: 10.1016/j.caeai.2023.100190
Haleem, A., Javaid, M., Qadri, M. A., and Suman, R. (2022). Understanding the role of digital technologies in education: a review. Sustainable Oper. Comput. 3, 275–285. doi: 10.1016/j.susoc.2022.05.004
Ibrahim, H., Liu, F., Asim, R., Battu, B., Benabderrahmane, S., Alhafni, B., et al. (2023). Perception, performance, and detectability of conversational artificial intelligence across 32 university courses. Sci. Rep. 13:12187. doi: 10.1038/s41598-023-38964-3
Ivanov, S., Soliman, M., Tuomi, A., Alkathiri, N. A., and Al-Alawi, A. N. (2024). Drivers of generative AI adoption in higher education through the lens of the theory of planned behaviour. Technol. Soc. 77:102521. doi: 10.1016/j.techsoc.2024.102521
Jo, H., and Bang, Y. (2023). Analyzing ChatGPT adoption drivers with the TOEK framework. Sci. Rep. 13:22606. doi: 10.1038/s41598-023-49710-0
Johnson, W. L. (2023). How to harness generative AI to accelerate human learning. Int. J. Artif. Intell. Educ. doi: 10.1007/s40593-023-00367-w
Kamalov, F., Santandreu Calonge, D., and Gurrib, I. (2023). New era of artificial intelligence in education: towards a sustainable multifaceted revolution. Sustain. For. 15:12451. doi: 10.3390/su151612451
Karen, W., James, H., and Kathryn, A. (2023). “Perceptions and barriers to adopting artificial intelligence in K-12 education: a survey of educators in fifty states” in Reimagining education. ed. M. Sharon (Rijeka: IntechOpen).
King, S., and Prasetyo, J. (2023). Assessing generative A.I. Through the lens of the 2023 Gartner hype cycle for emerging technologies: a collaborative autoethnography. Front. Educ. 8:1300391. doi: 10.3389/feduc.2023.1300391
Kitsara, I. (2022). “Artificial intelligence and the digital divide: from an innovation perspective” in Platforms and artificial intelligence: The next generation of competences. ed. A. Bounfour (Cham: Springer International Publishing), 245–265.
Kıyak, Y. S., and Emekli, E. (2024). ChatGPT prompts for generating multiple-choice questions in medical education and evidence on their validity: a literature review. Postgrad. Med. J. :qgae065. doi: 10.1093/postmj/qgae065
Kurtz, G., Amzalag, M., Shaked, N., Zaguri, Y., Kohen-Vacs, D., Gal, E., et al. (2024). Strategies for integrating generative AI into higher education: navigating challenges and leveraging opportunities. Educ. Sci. 14:503. doi: 10.3390/educsci14050503
Lim, W. M., Gunasekara, A., Pallant, J. L., Pallant, J. I., and Pechenkina, E. (2023). Generative AI and the future of education: Ragnarök or reformation? A paradoxical perspective from management educators. Int. J. Manage. Educ. 21:100790. doi: 10.1016/j.ijme.2023.100790
Liu, X., Wu, J., Shao, A., Shen, W., Ye, P., Wang, Y., et al. (2024). Uncovering language disparity of ChatGPT on retinal vascular disease classification: cross-sectional study. J. Med. Internet Res. 26:e51926. doi: 10.2196/51926
Marangunić, N., and Granić, A. (2015). Technology acceptance model: a literature review from 1986 to 2013. Univ. Access Inf. Soc. 14, 81–95. doi: 10.1007/s10209-014-0348-1
Margaryan, A., Littlejohn, A., and Vojt, G. (2011). Are digital natives a myth or reality? University students’ use of digital technologies. Comput. Educ. 56, 429–440. doi: 10.1016/j.compedu.2010.09.004
Menon, D., and Shilpa, K. (2023). “Chatting with ChatGPT”: analyzing the factors influencing users' intention to use the open AI's ChatGPT using the UTAUT model. Heliyon 9:e20962. doi: 10.1016/j.heliyon.2023.e20962
Michel-Villarreal, R., Vilalta-Perdomo, E., Salinas-Navarro, D. E., Thierry-Aguilera, R., and Gerardou, F. S. (2023). Challenges and opportunities of generative AI for higher education as explained by ChatGPT. Educ. Sci. 13:856. doi: 10.3390/educsci13090856
Mijwil, M. M., Abdulrhman, S. H., Abttan, R. A., Faieq, A. K., and Alkhazraji, A. (2023b). Artificial intelligence applications in English language teaching: a short survey. Asian J. Appl. Sci. 10. doi: 10.24203/ajas.v10i6.7111
Mijwil, M., Ali, G., Sadıkoğlu, E., Guma, A., and Emre, S. (2023). The evolving role of artificial intelligence in the future of distance learning: exploring the next frontier. Mesopotamian J. Comput. Sci. 2023, 98–105. doi: 10.58496/MJCSC/2023/012
Mijwil, M. M., Aljanabi, M., and Ali, A. H. (2023a). ChatGPT: exploring the role of cybersecurity in the protection of medical information. Mesopotamian J. CyberSecur. 2023, 18–21. doi: 10.58496/MJCS/2023/004
Ng, D. T. K., Leung, J. K. L., Su, J., Ng, R. C. W., and Chu, S. K. W. (2023). Teachers’ AI digital competencies and twenty-first century skills in the post-pandemic world. Educ. Technol. Res. Dev. 71, 137–161. doi: 10.1007/s11423-023-10203-6
Ogunleye, B., Zakariyyah, K. I., Ajao, O., Olayinka, O., and Sharma, H. (2024). A systematic review of generative AI for teaching and learning practice. Educ. Sci. 14:636. doi: 10.3390/educsci14060636
Okoye, K., Hussein, H., Arrona-Palacios, A., Quintero, H. N., Ortega, L. O. P., Sanchez, A. L., et al. (2023). Impact of digital technologies upon teaching and learning in higher education in Latin America: an outlook on the reach, barriers, and bottlenecks. Educ. Inf. Technol. 28, 2291–2360. doi: 10.1007/s10639-022-11214-1
Oluwadiya, K. S., Adeoti, A. O., Agodirin, S. O., Nottidge, T. E., Usman, M. I., Gali, M. B., et al. (2023). Exploring artificial intelligence in the Nigerian medical educational space: an online cross-sectional study of perceptions, risks and benefits among students and lecturers from ten universities. Niger. Postgrad. Med. J. 30, 285–292. doi: 10.4103/npmj.npmj_186_23
Ouyang, F., and Jiao, P. (2021). Artificial intelligence in education: the three paradigms. Comput. Educ.: Artif. Intell. 2:100020. doi: 10.1016/j.caeai.2021.100020
Ragnedda, M., and Muschert, G. (2013). The digital divide: the internet and social inequality in international perspective. 1st Edn. London: Routledge.
Ray, P. P. (2023). ChatGPT: a comprehensive review on background, applications, key challenges, bias, ethics, limitations and future scope. Internet Things Cyber-Phys. Syst. 3, 121–154. doi: 10.1016/j.iotcps.2023.04.003
Salazar, L. R., Peeples, S. F., and Brooks, M. E. (2024). “Generative AI ethical considerations and discriminatory biases on diverse students within the classroom” in The role of generative AI in the communication classroom. eds. S. Elmoudden and J. S. Wrench (Hershey, PA, USA: IGI Global), 191–213.
Salinas-Navarro, D. E., Vilalta-Perdomo, E., Michel-Villarreal, R., and Montesinos, L. (2024a). Designing experiential learning activities with generative artificial intelligence tools for authentic assessment. Interact. Technol. Smart Educ. doi: 10.1108/ITSE-12-2023-0236
Salinas-Navarro, D. E., Vilalta-Perdomo, E., Michel-Villarreal, R., and Montesinos, L. (2024b). Using generative artificial intelligence tools to explain and enhance experiential learning for authentic assessment. Educ. Sci. 14:83. doi: 10.3390/educsci14010083
Sallam, M. (2023). ChatGPT utility in healthcare education, research, and practice: systematic review on the promising perspectives and valid concerns. Healthcare (Basel) 11:887. doi: 10.3390/healthcare11060887
Sallam, M. (2024). Bibliometric top ten healthcare-related ChatGPT publications in the first ChatGPT anniversary. Narra J. 4:e917. doi: 10.52225/narra.v4i2.917
Sallam, M., Al-Farajat, A., and Egger, J. (2024). Envisioning the future of ChatGPT in healthcare: insights and recommendations from a systematic identification of influential research and a call for papers. Jordan Med. J. 58, 236–249. doi: 10.35516/jmj.v58i1.2285
Sallam, M., and Mousa, D. (2024). Evaluating ChatGPT performance in Arabic dialects: a comparative study showing defects in responding to Jordanian and Tunisian general health prompts. Mesopotamian J. Artif. Intell. Healthcare 2024, 1–7. doi: 10.58496/MJAIH/2024/001
Sallam, M., Salim, N. A., Barakat, M., Al-Mahzoum, K., Al-Tammemi, A. B., Malaeb, D., et al. (2023a). Assessing health Students' attitudes and usage of ChatGPT in Jordan: validation study. JMIR Med. Educ. 9:e48254. doi: 10.2196/48254
Sallam, M., Salim, N. A., Barakat, M., and Al-Tammemi, A. B. (2023b). ChatGPT applications in medical, dental, pharmacy, and public health education: a descriptive study highlighting the advantages and limitations. Narra J. 3:e103. doi: 10.52225/narra.v3i1.103
Savellon, M. U., Baybayan, S. B., and Asiri, M. S. (2024). Learning satisfaction on the use of chatgpt among nursing students in selected higher education institutions in Sulu. J. Educ. Acad. Settings 1, 1–16. doi: 10.62596/t3wgsm55
Science/AAAS Custom Publishing Office (2023) The world’s first AI university pairs machine learning’s great power with great responsibility. Available at: https://www.science.org/content/resource/worlds-first-ai-university-pairs-machine-learnings-great-power-great-responsibility (Accessed June 23, 2024)
Shaikh, S., Yayilgan, S. Y., Klimova, B., and Pikhart, M. (2023). Assessing the usability of ChatGPT for formal English language learning. Eur. J. Investig. Health Psychol. 13, 1937–1960. doi: 10.3390/ejihpe13090140
Sheikh Faisal, R., Nghia, D.-T., and Niels, P. (2024). “Generative AI in education: technical foundations, applications, and challenges” in Artificial intelligence for quality education. ed. K. Seifedine (Rijeka: IntechOpen).
Shwedeh, F., Salloum, S. A., Aburayya, A., Fatin, B., Elbadawi, M. A., Al Ghurabli, Z., et al. (2024). “AI adoption and educational sustainability in higher education in the UAE” in Artificial intelligence in education: The power and dangers of ChatGPT in the classroom. eds. A. Al-Marzouqi, S. A. Salloum, M. Al-Saidat, A. Aburayya, and B. Gupta (Switzerland, Cham: Springer Nature), 201–229.
Strzelecki, A. (2023). Students’ acceptance of ChatGPT in higher education: an extended unified theory of acceptance and use of technology. Innov. High. Educ. 49, 223–245. doi: 10.1007/s10755-023-09686-1
Tavakol, M., and Dennick, R. (2011). Making sense of Cronbach's alpha. Int. J. Med. Educ. 2, 53–55. doi: 10.5116/ijme.4dfb.8dfd
Tayan, O., Hassan, A., Khankan, K., and Askool, S. (2024). Considerations for adapting higher education technology courses for AI large language models: a critical review of the impact of ChatGPT. Mach. Learn. Appl. 15:100513. doi: 10.1016/j.mlwa.2023.100513
The UAE Ministry of Education (2024) Open Data. Available at: https://www.moe.gov.ae/En/OpenData/pages/home.aspx (Accessed January 26, 2024)
Veras, M., Dyer, J. O., Rooney, M., Barros Silva, P. G., Rutherford, D., and Kairy, D. (2023). Usability and efficacy of artificial intelligence Chatbots (ChatGPT) for health sciences students: protocol for a crossover randomized controlled trial. JMIR Res. Protoc. 12:e51873. doi: 10.2196/51873
von Garrel, J., and Mayer, J. (2023). Artificial intelligence in studies—use of ChatGPT and AI-based tools among students in Germany. Humanit. Soc. Sci. Commun. 10:799. doi: 10.1057/s41599-023-02304-7
Weidener, L., and Fischer, M. (2024). Artificial intelligence in medicine: cross-sectional study among medical students on application, education, and ethical aspects. JMIR Med. Educ. 10:e51247. doi: 10.2196/51247
Williams, R. T. (2024). The ethical implications of using generative chatbots in higher education. Front. Educ. 8:1331607. doi: 10.3389/feduc.2023.1331607
Xing, J., and Jiang, Q. (2024). Factors influencing user experience in AI chat systems – a satisfaction study based on factor analysis and linear regression. Kybernetes. doi: 10.1108/K-10-2023-2237
Yilmaz, F. G. K., Yilmaz, R., and Ceylan, M. (2023). Generative artificial intelligence acceptance scale: a validity and reliability study. Int. J. Hum.-Comput. Interact., 1–13. doi: 10.1080/10447318.2023.2288730
Yu, H. (2024). The application and challenges of ChatGPT in educational transformation: new demands for teachers' roles. Heliyon 10:e24289. doi: 10.1016/j.heliyon.2024.e24289
Yu, H., and Guo, Y. (2023). Generative artificial intelligence empowers educational reform: current status, issues, and prospects. Front. Educ. 8:1183162. doi: 10.3389/feduc.2023.1183162
Yusuf, A., Pervin, N., and Román-González, M. (2024). Generative AI and the future of higher education: a threat to academic integrity or reformation? Evidence from multicultural perspectives. Int. J. Educ. Technol. High. Educ. 21:21. doi: 10.1186/s41239-024-00453-6
Żammit, J. (2023). “Harnessing the power of ChatGPT for mastering the Maltese language: a journey of breaking barriers and charting new paths” in Machine intelligence for smart applications: Opportunities and risks. eds. A. Adadi and S. Motahhir (Switzerland, Cham: Springer Nature), 161–178.
Zarifhonarvar, A. (2023). Economics of ChatGPT: a labor market view on the occupational impact of artificial intelligence. J. Electron. Bus. Digit. Econ. 3, 100–116. doi: 10.1108/JEBDE-10-2023-0021
Appendix A1
Keywords: AI in education, higher education, large language models, attitude, ChatGPT
Citation: Sallam M, Elsayed W, Al-Shorbagy M, Barakat M, El Khatib S, Ghach W, Alwan N, Hallit S and Malaeb D (2024) ChatGPT usage and attitudes are driven by perceptions of usefulness, ease of use, risks, and psycho-social impact: a study among university students in the UAE. Front. Educ. 9:1414758. doi: 10.3389/feduc.2024.1414758
Edited by:
Hongbiao Yin, The Chinese University of Hong Kong, ChinaReviewed by:
Xindong Ye, Wenzhou University, ChinaIjeoma John-Adubasim, University of Plymouth, United Kingdom
Copyright © 2024 Sallam, Elsayed, Al-Shorbagy, Barakat, El Khatib, Ghach, Alwan, Hallit and Malaeb. This is an open-access article distributed under the terms of the Creative Commons Attribution License (CC BY). The use, distribution or reproduction in other forums is permitted, provided the original author(s) and the copyright owner(s) are credited and that the original publication in this journal is cited, in accordance with accepted academic practice. No use, distribution or reproduction is permitted which does not comply with these terms.
*Correspondence: Malik Sallam, bWFsaWsuc2FsbGFtQGp1LmVkdS5qbw==; Diana Malaeb, ZHIuZGlhbmFAZ211LmFjLmFl
†These authors share last authorship