- Johann Bernoulli Institute for Mathematics and Computer Science, Artificial Intelligence, University of Groningen, Groningen, Netherlands
This study investigates the role of statistical learning in the learning of lexical tones by non-tone language speakers. Over two experiments, participants were exposed to tone-syllable combinations with conditioned patterns. Experiment 1 used a typical statistical learning paradigm without feedback to assess participants' ability to discriminate tone-syllable combinations. The results revealed significant syllable learning but not tone learning. Experiment 2 controlled for syllable occurrence to isolate the learning of tonal patterns and demonstrated above-chance learning accuracy from the first training day, indicating successful lexical tone learning through the statistical learning mechanisms. The findings suggest that statistical learning without feedback facilitates lexical tone learning. Our study not only supports the universality of statistical learning in language acquisition but also prompts further research into its application in educational settings for teaching tonal languages.
1 Introduction
Variations in pitch provide critical lexical information in tonal languages. The concept of lexical tone in linguistics refers to the fundamental frequency (F0) patterns that are perceived as pitch variation by listeners to distinguish meaning. Unlike intonation which adds expressive meanings in non-tonal languages, lexical tones in tonal languages act as the fundamental and critical cues with different F0 patterns being used to create lexical contrast on words and syllables that share the same phoneme, thereby allowing a broader vocabulary within a relatively compact phonemic space (Yip, 2002).
Despite extensive research into the acquisition of phonetic and phonological aspects of language, how tonal patterns are learned and integrated into the mental lexicon remains unclear, especially for non-tonal language speakers whose first language lacks lexical contrasts between tones. To address this gap, we will explore the role of statistical learning in tonal acquisition. Statistical learning refers to the process by which individuals acquire knowledge about the statistical properties of sensory input, enabling them to understand and learn the sensory information. It is considered domain-general (Frost et al., 2015) and fundamental to language acquisition (Romberg and Saffran, 2010). The research by Saffran et al. (1996a) gives an example of statistical learning: Infants can segment words from a continuous speech stream based on transitional probabilities. After 2 min of listening, they distinguished words from nonwords, demonstrating statistical learning in language acquisition. Other than word segmentation, statistical learning is widely seen in learning various linguistic properties like phonemes (Kuhl et al., 2003) and phonotactic structures (Jusczyk et al., 1994). Of course, statistical learning is not the only mechanism that explains language acquisition. Implicit learning also plays a role, as people absorb linguistic patterns without being aware of them (Williams and Rebuschat, 2016). Error-driven learning that involves refining language skills through the updates of predictive errors enhances language acquisition as well (Bovolenta and Marsden, 2022).
The robustness of statistical language learning implies that this mechanism could be universal to non-native language acquisition, but empirical evidence remains inconclusive. While studies have shown statistical learning contributes positively to second-language acquisition (Onnis, 2011; Kerz and Wiechmann, 2019), other research found only partial correlations (Godfroid and Kim, 2021) or no significant benefits (Treffers-Daller and Calude, 2015). In particular, on the topic of lexical tone learning by non-tone speakers, the limited relevant research reveals mixed evidence: Wang and Saffran (2014) found that English speakers could not track statistical regularities between lexical tones. Liu and Kager (2012) did observe learning of tones from exposure in a preceding task but this was an incidental finding as the experiment was not designed with specific training phases. Ong et al. (2015) also found English speakers failed to learn Thai tones through statistical learning unless attention was engaged, indicating attention is necessary for statistical tone learning. However, other recent evidence suggests that attention is not necessary. Mismatch Negativity, a pre-attentive brain response to deviations in the rules of sensory input, showed that learners distinguished tonal contrasts after exposure to a bimodal distribution of tonal stimuli (Liu et al., 2018, 2022). These mixed findings raise the critical research question of whether statistical learning is truly universal and extends to non-native linguistic units.
It should be noted that the findings mentioned above about non-tone speakers learning lexical tones came from distributional learning, where the lack of variability in training input might limit broader inferences from the findings. Wiener et al. (2021) explored statistical tone learning in non-tone speakers in an eye-tracking study using multivariate training stimuli. The artificial language in their study closely resembled Mandarin with variability in tone-syllable co-occurrence probabilities and mapping of these combinations to visual symbols. The study showed that after 4 days of learning, adult learners could identify syllables using tonal probability and looked more frequently at items whose tones appeared more often during training. However, the exact mechanism of such learning remains unclear. It could be statistical learning, as they hypothesized, or error-driven learning. This conflation between the two types of learning was evident in how the sequence of tone-syllable stimuli (the cues) and the symbols (the outcomes) affected the learning process. Nixon (2020) found that an error-driven learning sequence, where cues were presented before outcomes, facilitated better learning of the cues. In one of the training tasks by Wiener, participants produced the syllable-tone cues based on the symbols they saw. The symbols stayed on the screen until the correct audio sounds for the symbols were presented. In their perceptual identification task, feedback was given after each response, making it unclear how much error-driven learning contributed to the learning results. These presentations of predictive cues and feedback likely indicate that the learning observed by Wiener et al. resulted from predictive error updates brought by feedback rather than tracking statistical cues of tonal probabilities on syllables. Furthermore, the training tasks in their study that involved production required active attention. Although they manipulated the explicitness of instructions on lexical tone, their production task designs deviated from the implicit nature of statistical learning.
The present study aims to clarify the role of statistical learning in non-tone speakers learning lexical tones. We used an artificial language learning paradigm with variations of tone and syllable included in the training input. In a subsequent experiment, we controlled the frequency of syllable occurrence to focus exclusively on observing the learning of lexical tones. In both experiments, neither feedback nor explicit learning cues were presented to participants, ensuring that the statistical learning tasks were passive and implicit. Although we acknowledge some overlap between the concepts of statistical learning and implicit learning (Perruchet and Pacton, 2006; Hamrick and Rebuschat, 2011), our study focuses on examining statistical abstraction from language input. A more detailed discussion of the relationship between statistical learning and implicit learning follows in Section 4. The two experiments were conducted following the Declaration of Helsinki and approved by the Research Ethics Committee (CETO) of the University of Groningen.
2 Experiment 1
2.1 Method
In experiment 1, we examined how well participants could learn tone-syllable regularities from artificial language input. An unsupervised categorical learning procedure was used. Learners were first familiarized with the language (referred to as “training” below), followed by a test phase to identify legal (i.e., pattern-conforming) and illegal items (i.e., non-conforming), with no feedback provided. This paradigm is common in statistical learning studies (e.g., Saffran and Thiessen, 2003; Cristiá and Seidl, 2008; Moreton and Pater, 2012). If learners managed to abstract patterns from the input, they should mostly categorize legal and illegal test items correctly. After 3 days of training, we anticipated participants would identify the legal and the illegal syllable-tone combinations.
2.1.1 Participants
We recruited 20 monolingual English speakers via Prolific. They were paid 4.88–5.88 GBP for participation. The rewards vary because more money was paid to those who had higher average accuracy across the 3 days. A total of 14 participants (Mean age: 26.36, Range: 19–34, four male) completed the experiment and their results are reported here.
2.1.2 Stimuli
We arbitrarily selected four consonant-vowel syllables (/ge/, /bi/, /du/, /kou/) from a Chinese dictionary, combining them with the four Mandarin tones (flat, rising, low-dipping, falling) to create 16 tone-syllable combinations. We selected eight of these as the legal items of the artificial language for participants to learn. The remaining eight combinations were illegal items. The difference between legal and illegal items depended on specific syllable-tone combinations. In other words, to distinguish which are legal items and which are not, a participant has to encode both the syllable and the tone dimensions. The legal and illegal designations were counterbalanced across participants: the legal items for one participant were the illegal items for another, and vice versa.
To determine if learning could be attributed to the syllable-tone combinations rather than just syllables, we introduced eight new combinations using syllables (/pei/ and /tai/) crossed with four lexical tones as additional illegal items. These were termed “illegal items with new syllables” to differentiate them from those made with /ge/, /bi/, /du/, and /kou/, which were termed “illegal items with old syllables.” In a post-hoc analysis using syllables as a predictor of participants' responses, we confirmed that participants did not show sensitivity to any particular syllable, as no significant variations were observed between the four old syllables or the two new syllables.
In sum, there are three types of items: (1) legal items, (2) illegal items with old syllables, and (3) illegal items with new syllables. Figure 1 shows an exemplar set of the materials. The 24 syllable-tone combinations were recorded by a voice actor and actress, resulting in 48 sound files. The actors were 25–30 years old and originally from Shandong Province, where local dialects form part of the standard Mandarin group. The recording was done in a studio in China, using an Audio-Technica AT2020 microphone. All sound files were normalized to an average of 68.92 (standard deviation: 4.79) dB SPL and an average of 416.88 (standard deviation: 111.34) ms in duration using Adobe Audition CS6.
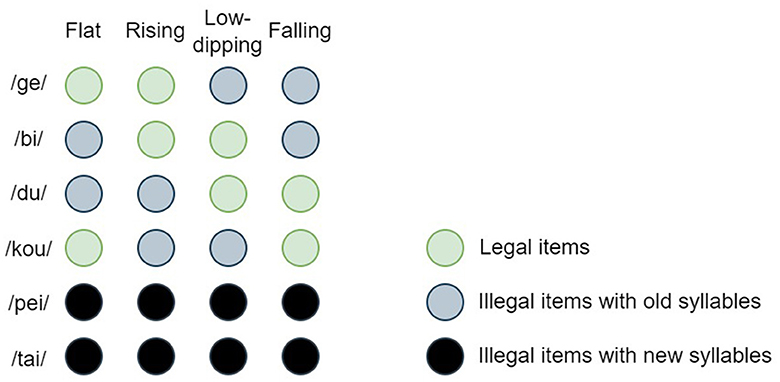
Figure 1. An example of the three item types in experiment 1. The green dots denote legal items. The light gray and the black dots respectively denote illegal items with old syllables and illegal items with new syllables.
2.1.3 Procedure
The experiment was conducted online using a website created with PsyToolkit (Stoet, 2010, 2017). It was hosted on the Psytoolkit server and accessed by participants via Prolific. In the training phase, participants were introduced to a new “language” through a 1-min audio stream that concatenated the eight legal items with a randomized order, each repeated 7–8 times by one speaker (e.g., the green dots in Figure 1). Participants heard 60 sound stimuli in total and were required to do nothing but stay focused on the audio stream for the 1 min when it was playing.
In the subsequent test phase, participants responded to 48 items (i.e., all dots in Figure 1 × 2 speakers)—legal items, illegal items with old syllables, and illegal items with new syllables—to decide if they belonged to the learned “language.” Each item was presented once, and participants had 5 s to respond by pressing “y” for acceptance or “n” for rejection. The test phase lasted 3–4 min.
Each day, participants completed two sessions, each session comprising a training phase and a test phase and lasting ~10 min. The entire experiment was conducted over three consecutive days. After the final day, participants were informed of their learning accuracy and payment.
2.2 Results
If participants had implicitly learned the conditional tone-syllable patterns, they should have accepted more legal items and rejected more illegal items with old syllables as the training progressed. However, we did not observe such results. As Figure 2A shows, regardless of training days, the acceptance rates for legal items and illegal items with old syllables are comparable.
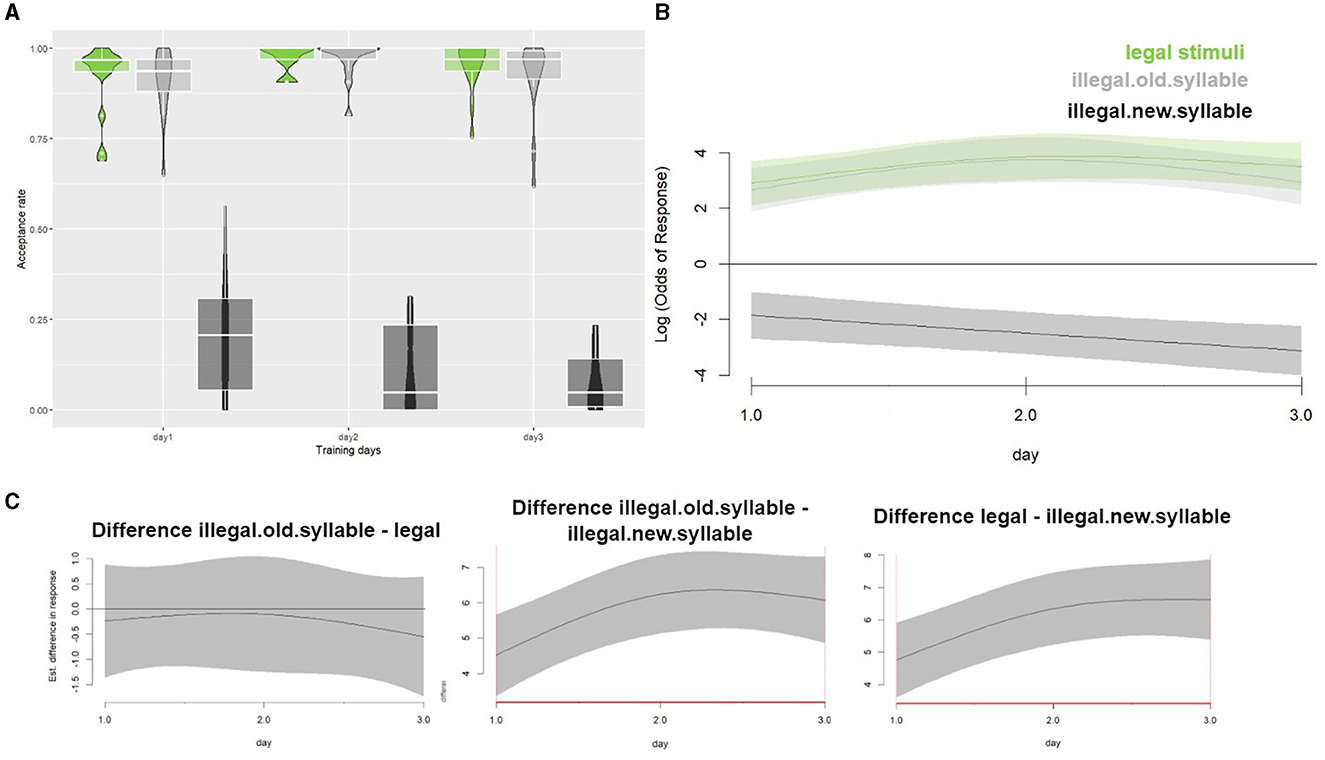
Figure 2. (A) A violin plot of the acceptance rate for the legal items (green), the illegal items with old syllables (light gray), and the illegal items with new syllables (black) in experiment 1 across the three training days. (B) The model plot of the predictor “item types” in the best fit GAM model. The three lines in the plot show the relationship between the binomial response and the legal items (green line labeled with “legal”), the illegal items with old syllables (light gray line labeled with “illegal.old.syllable”), and the illegal items with new syllables (black line labeled with “illegal.new.syllable”), respectively. The horizontal line at 0 denotes the chance level. Lines away from the chance level indicate significant effects on the conditions represented by the lines. (C) Difference plots for each two levels of “item types” on “training days.” From left to right, the plots respectively show the difference between the response to illegal items with old syllables and that to legal items, the difference between the response to illegal items with old syllables and that to illegal items with new syllables, and the difference between the response to legal items and that to illegal items with old syllables. Significant differences between conditions are marked as red on the x-axis. The horizontal line at 0 denotes the chance of no difference between the conditions compared.
To examine differences in responses to item types over training days, data were analyzed using a Generalized Additive Mixed (GAM) model, implemented in R (R Core Team, 2022) with the package mgcv (Wood, 2011). We chose the GAM model because of its flexibility in handling complex data patterns and uncovering subtle relations, and because our data include repeated measures. We also tried to implement similar model structures in a linear model, but it failed to converge.
The dependent variable of the model was binomial, consisting of participants' acceptance or rejection of an item. The acceptance rates were derived from the dependent variable, and the log-odds of these were modeled onto the predictors of training days (continuous), item types (categorical: legal items, illegal items with old syllables, and illegal items with new syllables), and the non-linear interaction between training days and item type. The model also included two random effects: the interaction between training days and participants per item type, and the effect of item variance per item type. No interaction was found between training days and items. The formula for the model is [response~item types+s(training days, k = 3, by = item types)+s(training days, participants, by = item types, bs = “fs, ” k = 3, m = 1)+s(item, by = item types, bs = “re”)].
Using a χ2 test of fREML score (itsadug package, van Rij et al., 2022), backward model comparisons confirmed the significant contribution of the interaction of training days and item types to the model (p < 0.01 for all comparisons). The model retaining all the above-mentioned predictors and random structures was therefore chosen as the best-fit model. In the model plot (Figure 2B), we observed a significant decline in acceptance rates for illegal items with new syllables over training days (χ2 = 8.965, edf = 1.002, p < 0.01), indicating that participants increasingly rejected these items with more training received. No significant trend along training days was observed for other item types. We further plotted the difference between each possible pairing of item types in relation to training days. As we already observed in Figure 2A, the difference plots further clarify that there is no difference between legal items and illegal items with old syllables (left panel in Figure 2C), but there is a clear difference when the comparisons are against illegal items with new syllables (middle and right panels in Figure 2C). This evidence together shows that new syllables are more likely to be rejected compared to the other items. This means that participants learned to be more aware of the syllabic differences among the test items, but no learning effect was seen that allowed discrimination of the tone-syllable combinations.
2.3 Discussion
If lexical tone learning had occurred, there would be a significantly higher acceptance rate for legal items than illegal items. Given previous evidence showing that non-tone language speakers can use statistical learning to discriminate syllables (Saffran et al., 1996b; Mirman et al., 2008; Palmer et al., 2018), we predicted a lower acceptance rate for stimuli that did not fit the learned patterns of segmentation, which were the illegal items with new syllables in the current experiment, and this was indeed observed.
However, we found no conclusive evidence of successful tone learning. Participants did not show enhanced recognition of conditioned tonal patterns. Progress was only made in rejecting the illegal items with new syllables, which signifies syllable learning instead of lexical tone learning.
Despite these findings, we cannot infer that statistical learning is completely ineffective for tone. Given the experimental design, syllable learning may have overshadowed the learning of lexical tones. As any lexical tone has to be carried by a syllable, the overlapping of tone and syllable in the current design prevents independent assessment of tone learning. Accordingly, we carried out a second experiment to address this issue by exclusively focusing on lexical tone learning while keeping syllabic information constant.
3 Experiment 2
3.1 Method
Referencing the experimental design of Wiener et al. (2021), experiment 2 used the same stimuli and absence of feedback during the test phase as in experiment 1, but specifically varied the co-occurrence frequencies of tone-syllable combinations during the training phase while ensuring consistent overall occurrences of each tone and syllable. Unlike Wiener, we chose not to manipulate syllable token frequency to avoid presenting syllables with low frequency. Nixon (2020) highlighted that error-driven learning induced by learning from discriminative order particularly works for items with low frequency. By ensuring no frequency variations between syllables, we intended to avoid a source of variance that could allow for error-driven learning. As in experiment 1, tone learning was tested using a discrimination task with no feedback provided. Participants were instructed to judge which of the two tone-syllable combinations in each pair was more familiar.
If our participants managed to learn the tone distinctions through statistical learning, we would expect higher task accuracy, as the tone-syllable combinations are the only cues for participants to make responses. Otherwise, if there was no learning of the tone distinctions, we would anticipate near-chance level performance and no improvement in accuracy during training days, because the overall occurrence frequencies for syllables and tones were kept constant.
3.1.1 Participants
Twenty non-tonal language speakers participated in experiment 2. They were recruited online and participated in the experiment on-site. The final data were from 19 participants (mean age: 22.97; range: 19–27; 2 male) after excluding one participant who did not attend all sessions. All participants reported no hearing or language deficits and were paid 14.58–16.36 Euros on completion of all sessions. Those who reached higher average accuracy got higher payments.
3.1.2 Stimuli
The 16 tone-syllable combinations from experiment 1, made by fully crossing the 4 syllables (/ge/, /bi/, /du/, /kou/) and the four Mandarin tones (flat, rising, low-dipping, falling), were used again. These stimuli show an average sound pressure level of 68.78 (standard deviation: 4.64) dB SPL and an average duration of 374.75 (standard deviation: 98.81) ms. The averaged sound pressure and duration are different from those in experiment 1 due to the exclusion of /pei/ and /tai/ stimuli. The 16 stimuli were organized into a set of training items and a set of test items in the following manner.
3.1.2.1 Training items
The training phase presented all 16 combinations. We chose four stimuli as the high-frequency (HF) items, with each item presented with equal frequency and each of the four tones represented in the selection. The HF items together make up 90% of the presentations. The remaining combinations were designated as medium-frequency (MF) items (9% of presentations) and low-frequency (LF) items (1% of presentations). The selection of the 4 HF items and the proportion of presentation were aimed to ensure a pronounced contrast in the occurrence rates between HF and LF and strengthen the learning impact of HF exposure. This setup led to 600 stimuli in the training phase (540 HF, 54 MF, and 6 LF). Each individual tone and syllable was presented 150 times in total, to ensure that the only contrast in frequency was on the co-occurrence of tone and syllable. Figure 3 illustrates how the training stimuli were composed. HF and LF items were counterbalanced between participants to control for arbitrary item preferences during learning, while MF items remained the same across participants.
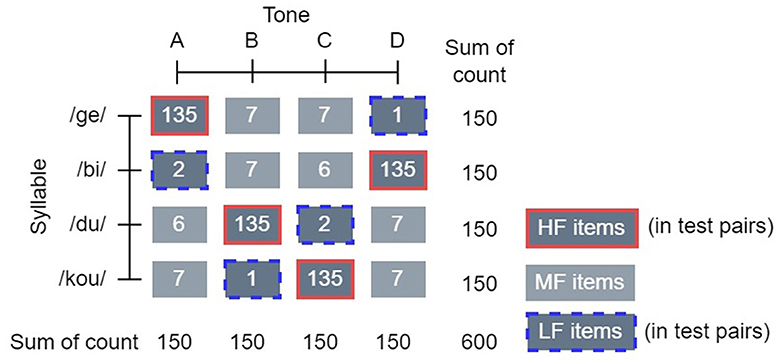
Figure 3. The structure of training stimuli and test pairs in experiment 2. Training stimuli: The four syllables are illustrated as the rows, and the four tones are illustrated as the columns in the figure. Because of the between-participants counterbalancing design on training stimuli, instead of denoting the specific tones in the figure, we code them as A, B, C, and D to ensure this structure applies to all counterbalanced conditions. For example, to one participant, the tones can be flat, rising, low-dipping, and falling from A to D. To another participant, in the same order from A to D, the tones can be falling, low-dipping, rising, and flat. Numbers inside the gray blocks denote how many times the tone-syllable combinations occurred in the training phase. The HF items are highlighted by solid red borders. They together make up 90% of the total presentations of training stimuli. The MF items are shown as gray blocks without borders, making up 9% of the presentations, and the LF items, which are highlighted by dashed blue borders, make up 1% of the presentations. Test pairs: Only the HF items and the LF items were presented in test pairs, as denoted in the legend. The order of whether an HF item or an LF item appeared first in a test pair was counterbalanced within the test phase for each participant.
3.1.2.2 Test items
In the test phase, sound files were presented in pairs that differed in the occurrence frequencies of tone and syllables. To be specific, each test pair always consisted of an LF and an HF item, as denoted in the legend of Figure 3. To focus on the contrast of frequency, we only present the HF items and the LF items in the test phase. Each HF-LF combination was presented twice, once with each speaker, resulting in 32 test items.
3.1.3 Procedure
Participants took part in a 20-min training-test session on four consecutive days on site. Each session began with a 10-min training phase framed as a counting task, where participants counted beeps that randomly occurred among speech stimuli. This counting task was included to maintain participants' attention throughout training because the current experiment has a longer training duration than that in experiment 1. The 600 training items were randomly and evenly distributed among five blocks.
Following the training, a 10-min test phase presented 64 trials (32 test pairs × 2 orders of presenting stimuli) where participants compared two tone-syllable combinations of differing frequencies, selecting the more familiar one based on training.
Sound files were delivered binaurally via headphones (Sennheiser HD 201) at a volume that participants found suitable. The experiment was presented using OpenSesame (Mathôt et al., 2012) with the Xpyriment back-end (Krause and Lindemann, 2014). The inter-trial interval was 500 ms throughout the experiment. Participants had up to 5 s to make a response. At the end of each day's training, participants were shown their accuracy in the counting task and the test phase.
3.2 Results
Given that syllable and tone occurrences were equally manipulated, any above-chance accuracy (>0.5) and observed learning progress must be due to the learning of tone-syllable combinations. Figure 4A illustrates the changes in averaged accuracy across the training days. We noticed above-chance performance on the first day and a clear increasing trend in day-to-day learning progress.
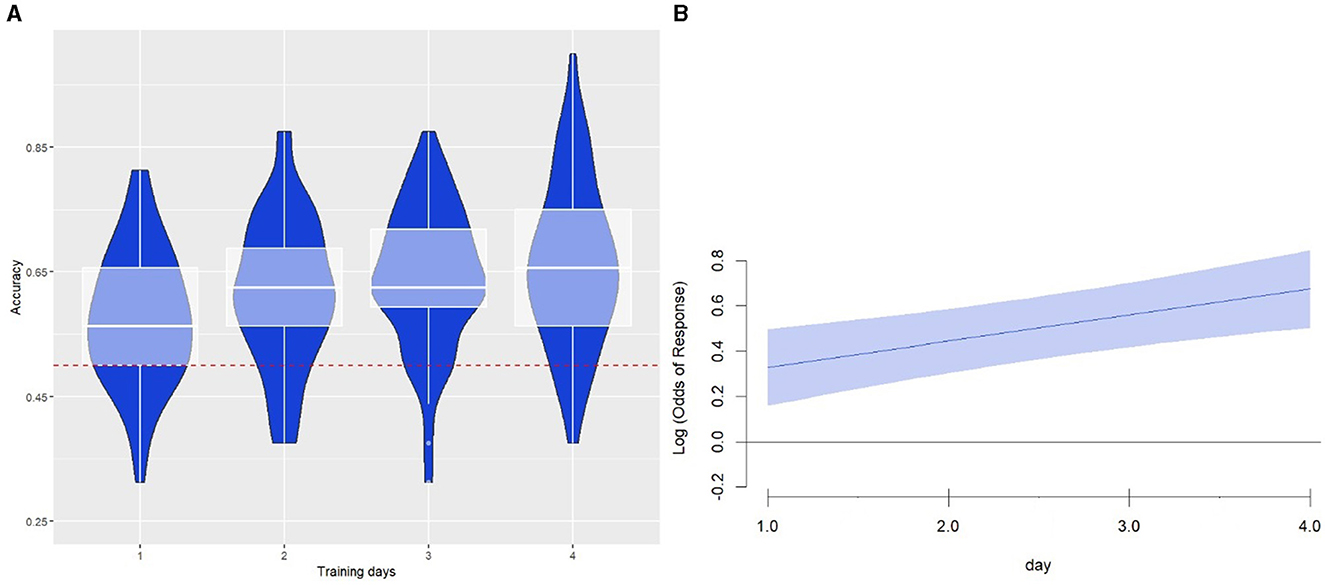
Figure 4. (A) The learning outcomes across the four training days. Accuracy was calculated as the proportion of responses in which participants managed to recognize the HF tone-syllable combination from a test stimuli pair. The horizontal line at 0.5 denotes the chance level. (B) The plot of the predictor “training days” in the GAM model of experiment 2. The horizontal line at 0 denotes the chance level. The effect of “training days” is significant because it is above the chance level.
To assess the statistical significance of the observed learning, we used a GAM model to examine the effect of training days on response accuracy. The log odds of binomial responses were modeled on a continuous predictor of training days. The random structure retained the effect of training days on participants and the random intercept for test pairs. As in experiment 1, we omitted the effect of training days on test pairs [response~s(training days)+s(training days, participants, bs = “fs, ” k = 3, m = 1)+s(test pairs, bs = “re”)]. This model significantly outperformed a reduced model that only contained the random structure (p < 0.01). Confirming our observation in Figure 4A, the smooth term of training days in this model indicated significant learning across the days (χ2 = 11.5, edf = 1.018, p < 0.001). From the model plot (Figure 4B), it is clear that response accuracy is consistently above chance from the start.
3.3 Discussion
Experiment 2 is a further investigation of the same research question in experiment 1, with a refined experimental design to control for syllable learning. It refers to the design in Wiener et al. (2021) but has no item-level feedback provided to participants, thus excluding the possibility of error-driven learning. Because of this, we inferred that any observed learning progress would arise from statistical learning of tone-syllable co-occurrence.
Results in experiment 2 showed learning above chance from the first day, increasing with each training day. This indicated that participants learned the conditioned tone-syllable patterns, even after just 10 min of exposure.
In summary, our results align with the statistical learning explanation of the findings by Wiener et al. (2021), suggesting an underlying statistical mechanism for lexical tone learning. Even without feedback, learners who have no prior tonal language knowledge can still detect the patterns of tone-syllable co-occurrence. The above-chance learning accuracy we found on the first training day is also in line with the previous statistical learning studies that found rapid learning after a brief exposure (e.g., Mirman et al., 2008).
4 General discussion
The research aimed to explore how non-tone language speakers use statistical learning for lexical tone learning. Experiment 1, using a standard statistical learning paradigm, did not demonstrate tone learning but indicated significant syllable learning, as shown by the increased rejection of illegal items with new syllables. With a design that controlled for syllable occurrence, experiment 2 revealed above-chance accuracy from the first day and increased accuracy with each subsequent training day. Our findings support statistical learning as an available mechanism for learning lexical tones by non-tone speakers.
In contrast to the findings of Wiener et al. (2021), our results excluded the alternative error-driven learning explanation for the successful lexical tone learning effect in their study: we also observed significant learning but provided no feedback during the tasks. However, the presentation sequence in Wiener et al. (2021)'s study, involving visual symbols and feedback after responses, allows the conflation of error-driven learning and statistical learning. In the present study, neither visual symbols nor feedback were presented in the training and test tasks. By excluding outcomes in stimuli presentation, we were able to ensure that any observed effect should not stem from error-driven learning but pure statistical learning.
The results we found in experiment 2 might also be interpreted as implicit learning. By choosing not to provide feedback, we characterized the statistical learning in experiment 2 as implicit. However, this does not imply that the learning is implicit learning in the sense used by Perruchet and others, rather than statistical learning. We define experiment 2 as statistical learning due to its focus on discriminating tone-syllable combinations in the training input. This distinguishes the task from implicit learning, which often involves memorizing chunks rather than computing statistics (Perruchet et al., 2002; Perruchet and Pacton, 2006). Nevertheless, we acknowledge that implicit learning could not be entirely ruled out from our study, as statistical learning and implicit learning are often intertwined (Perruchet and Pacton, 2006; Hamrick and Rebuschat, 2011). Further exploration is needed to clarify the contributions of chunk formation in lexical tone learning.
Alongside Wiener et al., other studies have also found successful statistical tone learning in non-tone language speakers. Liu et al. (2018) used electroencephalography (EEG) recording in a passive oddball paradigm and showed that listeners discriminated the non-native tonal contrast after exposure to a block of tonal stimuli. Further, Liu et al. (2022) found that exposure to a bimodal, rather than unimodal, distribution led to more learning revealed in the EEG results. It is important to note that the bimodal lexical tone continuum used in those research uses a narrower interpretation of learning—the distributional learning of acoustic features—which may not fully capture the natural variability in lexical tones. In contrast, our study employed a diverse range of naturally produced monosyllabic tonal words. By exposing participants to a broader spectrum of tonal variations, our study allows for a more comprehensive exploration of how learners extract patterns from the training input. Therefore, our approach provides a more ecologically valid understanding of the statistical learning processes in language acquisition.
While the current findings indicate that statistical learning without feedback facilitates lexical tone learning in non-tone speakers, concerns regarding the small sample size and limited statistical power must be acknowledged. Future studies should address these limitations by performing an a priori power analysis to ensure an appropriate sample size. Incorporating a more rigorous design that matches participants based on their pitch-related experience is also recommended. It would also be valuable for future research to explore how learning develops from the first training day with naive participants, perhaps by segmenting the training-test procedure into blocks to observe early learning patterns. Investigating statistical tone learning in a classroom setting could also validate the educational potential of these findings, thereby translating statistical learning theory into effective pedagogical strategies.
Data availability statement
The raw data supporting the conclusions of this article will be made available by the authors, without undue reservation.
Ethics statement
The studies involving humans were approved by Research Ethics Committee (CETO) at the University of Groningen. The studies were conducted in accordance with the local legislation and institutional requirements. The participants provided their written informed consent to participate in this study.
Author contributions
MT: Conceptualization, Data curation, Formal analysis, Investigation, Methodology, Project administration, Software, Visualization, Writing – original draft, Writing – review & editing. JS: Conceptualization, Methodology, Project administration, Supervision, Writing – review & editing. SJ: Project administration, Supervision, Writing – review & editing.
Funding
The author(s) declare that no financial support was received for the research, authorship, and/or publication of this article.
Acknowledgments
This research was supported by the PhD program of China Scholarship Council. We sincerely thank Jacolien van Rij for her invaluable help with data analysis. We also thank the editor for guiding the publication process and the reviewers for their insightful feedback that strengthened the present article. Additionally, we are thankful to all the participants whose contributions made this research possible.
Conflict of interest
The authors declare that the research was conducted in the absence of any commercial or financial relationships that could be construed as a potential conflict of interest.
Publisher's note
All claims expressed in this article are solely those of the authors and do not necessarily represent those of their affiliated organizations, or those of the publisher, the editors and the reviewers. Any product that may be evaluated in this article, or claim that may be made by its manufacturer, is not guaranteed or endorsed by the publisher.
References
Bovolenta, G., and Marsden, E. (2022). Prediction and error-based learning in l2 processing and acquisition: a conceptual review. Stud. Second Lang. Acquis. 44, 1384–1409. doi: 10.1017/S0272263121000723
Cristiá, A., and Seidl, A. (2008). Is infants' learning of sound patterns constrained by phonological features? Lang. Learn. Dev. 4, 203–227. doi: 10.1080/15475440802143109
Frost, R., Armstrong, B. C., Siegelman, N., and Christiansen, M. H. (2015). Domain generality vs. modality specificity: the paradox of statistical learning. Trends Cogn. Sci. 19, 117–125. doi: 10.1016/j.tics.2014.12.010
Godfroid, A., and Kim, K. M. (2021). The contributions of implicit-statistical learning aptitude to implicit second-language knowledge. Stud. Second Lang. Acquis. 43, 606–634. doi: 10.1017/S0272263121000085
Hamrick, P., and Rebuschat, P. (2011). “How implicit is statistical learning?” in Statistical Learning and Language Acquisition, eds. P. Rebuschat, and J. N. Williams (Berlin: De Gruyter), 365–382. doi: 10.1515/9781934078242.365
Jusczyk, P. W., Luce, P. A., and Charles-Luce, J. (1994). Infants' sensitivity to phonotactic patterns in the native language. J. Mem. Lang. 33, 630–645. doi: 10.1006/jmla.1994.1030
Kerz, E., and Wiechmann, D. (2019). “Effects of statistical learning ability on the second language processing of multiword sequences,” in Computational and Corpus-Based Phraseology: Third International Conference, Europhras 2019, Malaga, Spain, September 25-27, 2019, Proceedings 3 (Cham: Springer), 200–214. doi: 10.1007/978-3-030-30135-4_15
Krause, F., and Lindemann, O. (2014). Expyriment: a python library for cognitive and neuroscientific experiments. Behav. Res. Methods 46, 416–428. doi: 10.3758/s13428-013-0390-6
Kuhl, P. K., Tsao, F. M., and Liu, H. M. (2003). Foreign-language experience in infancy: effects of short-term exposure and social interaction on phonetic learning. Proc. Natl. Acad. Sci. USA. 100, 9096–9101. doi: 10.1073/pnas.1532872100
Liu, L., and Kager, R. (2012). “Non-native tone perception from infant to adult: how consistent and flexible is it?” in Proceedings of the 6th International Conference on Speech Prosody, SP 1 (Shanghai), 346–349. doi: 10.21437/SpeechProsody.2012-88
Liu, L., Ong, J. H., Tuninetti, A., and Escudero, P. (2018). One way or another: evidence for perceptual asymmetry in pre-attentive learning of non-native contrasts. Front. Psychol. 9, 1–13. doi: 10.3389/fpsyg.2018.00162
Liu, L., Yuan, C., Ong, J. H., Tuninetti, A., Antoniou, M., Cutler, A., et al. (2022). Learning to perceive non-native tones via distributional training: effects of task and acoustic cue weighting. Brain Sci. 12:559. doi: 10.3390/brainsci12050559
Mathôt, S., Schreij, D., and Theeuwes, J. (2012). Opensesame: an open-source, graphical experiment builder for the social sciences. Behav. Res. Methods 44, 314–324. doi: 10.3758/s13428-011-0168-7
Mirman, D., Magnuson, J. S., Estes, K. G., and Dixon, J. A. (2008). The link between statistical segmentation and word learning in adults. Cognition 108, 271–280. doi: 10.1016/j.cognition.2008.02.003
Moreton, E., and Pater, J. (2012). Structure and substance in artificial-phonology learning, part I: structure. Lang. Linguist. Compass 6, 702–718. doi: 10.1002/lnc3.366
Nixon, J. S. (2020). Of mice and men: speech sound acquisition as discriminative learning from prediction error, not just statistical tracking. Cognition 197:104081. doi: 10.1016/j.cognition.2019.104081
Ong, J. H., Burnham, D., and Escudero, P. (2015). Distributional learning of lexical tones: a comparison of attended vs. unattended listening. PLoS ONE 10:e0133446. doi: 10.1371/journal.pone.0133446
Onnis, L. (2011). “The potential contribution of statistical learning to second language acquisition,” in Statistical Learning and Language Acquisition, eds. P. Rebuschat, and J. N. Williams (Berlin: De Gruyter), 203–236. doi: 10.1515/9781934078242.203
Palmer, S. D., Hutson, J., and Mattys, S. L. (2018). Statistical learning for speech segmentation: age-related changes and underlying mechanisms. Psychol. Aging 33, 1035. doi: 10.1037/pag0000292
Perruchet, P., and Pacton, S. (2006). Implicit learning and statistical learning: one phenomenon, two approaches. Trends Cogn. Sci. 10, 233–238. doi: 10.1016/j.tics.2006.03.006
Perruchet, P., Vinter, A., Pacteau, C., and Gallego, J. (2002). The formation of structurally relevant units in artificial grammar learning. Quart. J. Exp. Psychol. A 55, 485–503. doi: 10.1080/02724980143000451
R Core Team (2022). R: A Language and Environment for Statistical Computing. Vienna: R Foundation for Statistical Computing.
Romberg, A. R., and Saffran, J. R. (2010). Statistical learning and language acquisition. Wiley Interdiscip. Rev. Cogn. Sci. 1, 906–914. doi: 10.1002/wcs.78
Saffran, J. R., Aslin, R. N., and Newport, E. L. (1996a). Statistical learning by 8-month-old infants. Science 274, 1926–1928. doi: 10.1126/science.274.5294.1926
Saffran, J. R., Newport, E. L., and Aslin, R. N. (1996b). Word segmentation: the role of distributional cues. J. Mem. Lang. 35, 606–621. doi: 10.1006/jmla.1996.0032
Saffran, J. R., and Thiessen, E. D. (2003). Pattern induction by infant language learners. Dev. Psychol. 39, 484–494. doi: 10.1037/0012-1649.39.3.484
Stoet, G. (2010). Psytoolkit: a software package for programming psychological experiments using linux. Behav. Res. Methods 42, 1096–1104. doi: 10.3758/BRM.42.4.1096
Stoet, G. (2017). Psytoolkit: a novel web-based method for running online questionnaires and reaction-time experiments. Teach. Psychol. 44, 24–31. doi: 10.1177/0098628316677643
Treffers-Daller, J., and Calude, A. (2015). The role of statistical learning in the acquisition of motion event construal in a second language. Int. J. Biling. Educ. Biling. 18, 602–623. doi: 10.1080/13670050.2015.1027146
van Rij, J., Wieling, M., Baayen, R. H., and van Rijn, H. (2022). itsadug: interpreting time series and autocorrelated data using gamms. R package version 2.4.1. Available at: https://cran.r-project.org/web/packages/itsadug/citation.html
Wang, T., and Saffran, J. R. (2014). Statistical learning of a tonal language: the influence of bilingualism and previous linguistic experience. Front. Psychol. 5:953. doi: 10.3389/fpsyg.2014.00953
Wiener, S., Ito, K., and Speer, S. R. (2021). Effects of multitalker input and instructional method on the dimension-based statistical learning of syllable-tone combinations. Stud. Second Lang. Acquis. 43, 155–180. doi: 10.1017/S0272263120000418
Williams, J., and Rebuschat, P. (2016). Implicit Learning and Second Language Acquisition. Evanston, IL: Routledge.
Wood, S. N. (2011). Fast stable restricted maximum likelihood and marginal likelihood estimation of semiparametric generalized linear models. J. R. Stat. Soc. B 73, 3–36. doi: 10.1111/j.1467-9868.2010.00749.x
Keywords: lexical tone learning, statistical learning, lexical tone, non-native phonetic learning, tonal language
Citation: Tang M, Spenader J and Jones S (2024) Learning lexical tone through statistical learning in non-tone language speakers. Front. Educ. 9:1393379. doi: 10.3389/feduc.2024.1393379
Received: 29 February 2024; Accepted: 24 July 2024;
Published: 08 August 2024.
Edited by:
Xin Wang, Macquarie University, AustraliaReviewed by:
Jiaqiang Zhu, Hong Kong Polytechnic University, Hong Kong SAR, ChinaYang Zhao, Shanghai International Studies University, China
Copyright © 2024 Tang, Spenader and Jones. This is an open-access article distributed under the terms of the Creative Commons Attribution License (CC BY). The use, distribution or reproduction in other forums is permitted, provided the original author(s) and the copyright owner(s) are credited and that the original publication in this journal is cited, in accordance with accepted academic practice. No use, distribution or reproduction is permitted which does not comply with these terms.
*Correspondence: Mi Tang, m.tang@rug.nl