- 1Istituto di Linguistica Computazionale “A. Zampolli”, Consiglio Nazionale delle Ricerche (CNR), Pisa, Italy
- 2Centre for Science and Technology Studies (CWTS), Leiden University, Leiden, Netherlands
- 3OpenAIRE AMKE, Athens, Greece
- 4CLARIN ERIC, Utrecht, Netherlands
- 5Health-RI, Utrecht, Netherlands
- 6EIFL, Vilnius, Lithuania
- 7National Institute for Public Health and the Environment, Bilthoven, Netherlands
- 8The Framework for Open and Reproducible Research Training (FORRT), Groningen, Netherlands
- 9Slovenian Social Science Data Archive, Faculty of Social Sciences, University of Ljubljana, Ljubljana, Slovenia
- 10Data Sciences Centre, European Molecular Biology Laboratory (EMBL), Heidelberg, Germany
- 11JISC, Newcastle upon Tyne, United Kingdom
- 12Data Archiving and Networked Services (DANS), The Hague, Netherlands
Since the introduction of the FAIR (Findable, Accessible, Interoperable and Reusable) data principles in 2016, discussions have evolved beyond the original focus on research data to include learning resources. In 2020, a set of simple rules to FAIRify learning resources was proposed, building on existing expertise within the training community. Disciplinary communities have played an important role in advancing FAIR principles for learning resources, although they have approached FAIRification activities in different ways. These communities range from volunteer-led to funded and independent organisations, however commonly include activities such as organising training and capacity building, and coordinated discussions on disciplinary-focused FAIR best practises and standards. Eight disciplinary community case studies are presented and analysed in this paper to examine the motivations, challenges and opportunities towards FAIRification of learning resources, reflecting on how community structure leads to differing responsibilities. The case studies are based on reflections formulated in 2022, the aim is to pull together the experiences of these different communities, focusing on the processes and challenges they encountered, in order to structure this knowledge across different learning platforms, draw attention to the question of sustainability for learning resources and anticipate improvements in future policies and governance.
1 Introduction
The FAIR data principles were first published under FORCE11 in 2016 as a set of guiding principles to make research data and scholarly digital objects Findable, Accessible, Interoperable, and Reusable (Wilkinson et al., 2016). Consisting of 15 statements grouped under the four categories, FAIR is intentionally high-level, and serves to guide disciplinary communities, data publishers, data stewards and other stakeholders in the management of (research) data. In this way, the aspirations outlined in the principles can be contextualised into usable and implementable guidelines for researchers and other data producers/owners.
Central to the concept of FAIR is its applicability to both human- and machine-readable data, with machine-actionability to the highest degree possible (Wilkinson et al., 2016). This aim emphasises the importance of structured metadata, including controlled vocabularies and ontologies,1 and provides an essential tool to implement Open Science (also referred to as Open Research or Open Scholarship) and good practises in research data management (RDM) (Higman et al., 2019).
FAIR has rapidly gained traction and widespread global acceptance leading to many diverse activities, including infrastructure development, new disciplinary standards, and capacity building in data stewardship. Being a relatively new field, it is still evolving and faces challenges to successful implementation, such as requiring infrastructure, expertise and funding. It also requires community buy-in, training, capacity building and support [Directorate-General for Research and Innovation (European Commission), 2018].
In this paper, we take a closer look at eight research communities providing training activities in different research fields. The aim is to explore their motivations and efforts to make learning resources FAIR. We point to the difficulties the communities encountered, and present the strategies they relied on to overcome certain obstacles. Overall, the objective is to gather insights from these communities to improve how educational resources are organised and kept available on various platforms.
2 Materials and methods
2.1 Research communities and FAIR principles
Key to the successful implementation of FAIR principles is their translation into discipline-specific guidelines [Directorate-General for Research and Innovation (European Commission), 2020]. Research disciplines vary considerably in relation to the types of data produced, as well as traditions of data management and sharing (e.g., some fields lack specific repositories, such as earth sciences, whilst others require repositories which can deal with complex outputs as it is the case in the humanities; ORCID identifiers are not equally adopted across disciplines). Disciplinary guidelines can therefore be heterogeneous and may not be transferable between research fields. For this reason, inter-disciplinary discussion, consensus building and standard-setting activities are crucial to the rollout of FAIR in research. A relevant example is seen in the objectives of the European Open Science Cloud (EOSC) to “provide European researchers, innovators, companies and citizens with a federated and open multi-disciplinary environment where they can publish, find and reuse data, tools and services for research, innovation and educational purposes” [Directorate-General for Research and Innovation (European Commission), 2022], and the recently-finished project tasked with its implementation, EOSC Future.
Research communities have played an important role in initiating and coordinating FAIR discussions within the disciplines that they serve. For this paper, we use an intentionally broad definition of “communities” to include any structured organisation dedicated to advancing the interests of a specific group of researchers. Within our definition, research communities are extremely heterogeneous in terms of composition, longevity, funding and objectives. They range from volunteer-led organisations (e.g., FORRT) to short-term externally funded projects (e.g., EOSC Synergy, SSHOC) or longer-term projects funded internally by an institution (e.g., EMBL Bio-IT), e-infrastructures (e.g., OpenAIRE) and discipline-specific research infrastructures (e.g., ELIXIR, CLARIN, CESSDA). Despite this heterogeneity, research communities share the same purpose of uniting researchers and providing a platform for developing common practises.
Whilst several resources exist addressing how to implement FAIR best practises in data and software management, as well as in managing scientific outputs such as publications and other reports, the discussion on how to adopt them in training and capacity building within communities is still open. Research communities tend to engage in training and capacity-building activities, whether internally developed or by collecting external resources, thereby forming a bridge between researchers, learning resources and data collections. Learning resources themselves are diverse. A recent definition outlined them as “a persistent information resource that has one or more physical or digital representations, and that explicitly involves, specifies or entails a learning activity or learning experience. As an information resource, it cannot be, for example, a person, or object, and since it is persistent it cannot be an event (though it can be a record of an event). A learning activity or experience has characteristics that may improve or measure a person’s knowledge, skills or abilities. A learning resource may reference other supporting materials, creative works, tools etc. that do not themselves meet this definition” (Hoebelheinrich et al., 2022). The growing amount of learning resources curated by these communities has highlighted the importance of developing practises for managing these and ensuring their “FAIRness.”
2.2 FAIR learning resources
The Open Science movement has been hugely influential in advocating for the sharing of learning resources. The efforts of the open educational resources2 (OERs) community resulted in a wide range of lecture slides, recordings and related resources placed online for reuse (Roncevic, 2021). These efforts were strengthened during the rapid expansion of online training in response to the COVID-19 pandemic (Ossiannilsson, 2020).
Although there is a wealth of learning resources available online, their lack of standardisation restricts their impact (Atenas and Havemann, 2014; Garcia et al., 2020). The diversity of online storage sites, along with the scarcity of metadata and highly variable annotations of resources, make it difficult to search and confidently reuse the resources available. Long-term reuse of learning resources comes with a range of additional challenges, which include the findability of unique training event resources, updating the content (versioning), and ensuring that it stays relevant (curation). The reuse of learning resources also has distinct legal challenges to address beyond just personal data protection (e.g., the European Union’s GDPR—General Data Protection Regulation), as training could be an income-generating activity, or it could be organised as part of the university curricula, in which case the learning resources may not be available in Open Access. This raises intellectual property issues of learning resources and influences their potential for reuse.
Challenges associated with facilitating effective reuse of learning resources have drawn the attention of the FAIR training community. A set of rules for making training materials FAIR has been suggested (Garcia et al., 2020; Figure 1); they serve as reminders for trainers, as illustrated in Figure 1.
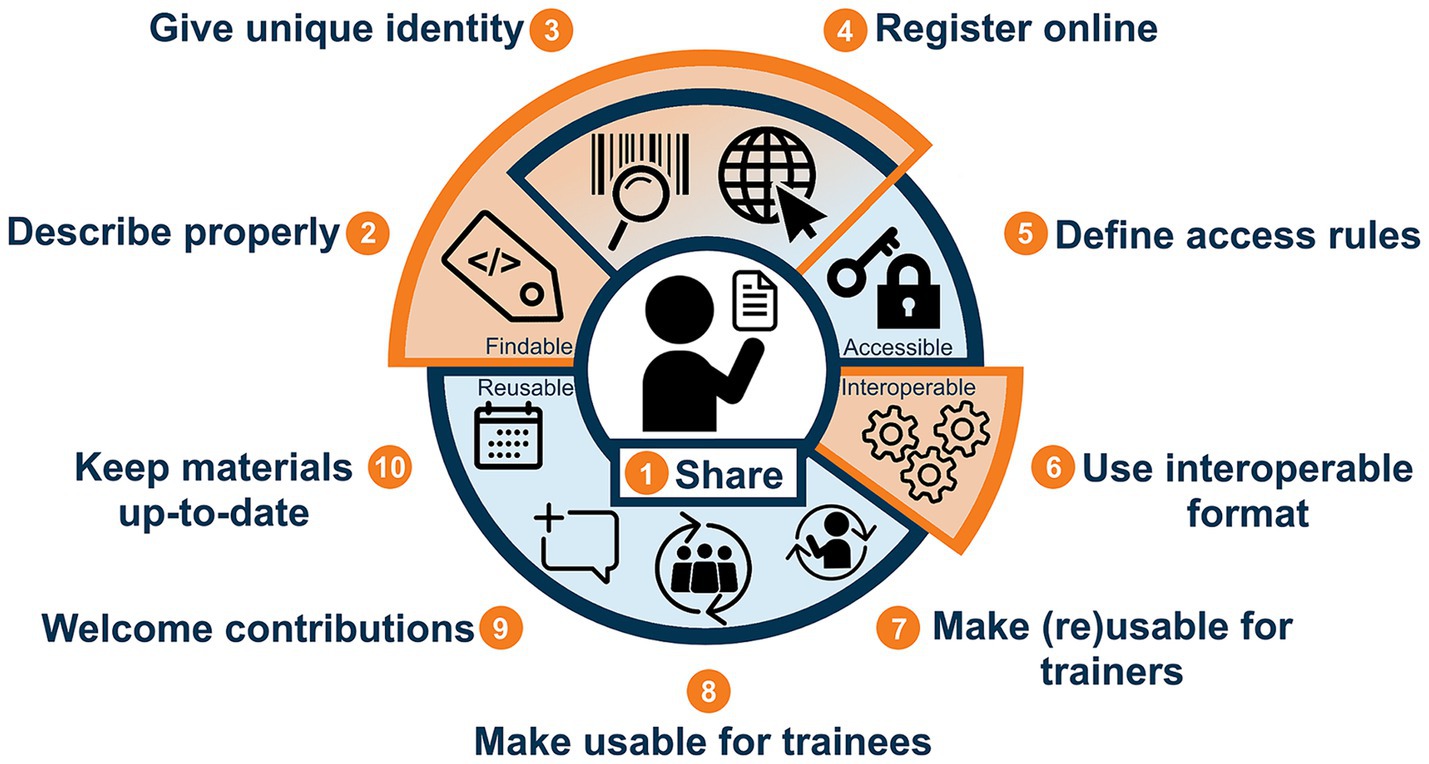
Figure 1. Ten simple rules for making training materials FAIR, taken from Garcia et al. (2020).
The paper in which the 10 rules are presented also provides more specific guidelines to assist FAIRification of learning resources. Importantly, it provides a metadata template outlining the key information needed to facilitate the reuse of any resource. The required metadata is detailed and includes often-overlooked fields such as description, learning outcomes and target audience.
Similarly, the Research Data Alliance (RDA) interest group on Education and Training on Handling Research Data (ETHRD) produced recommendations for a minimal metadata set to aid discovery of learning resources (Hoebelheinrich et al., 2022) as illustrated in Figure 2.
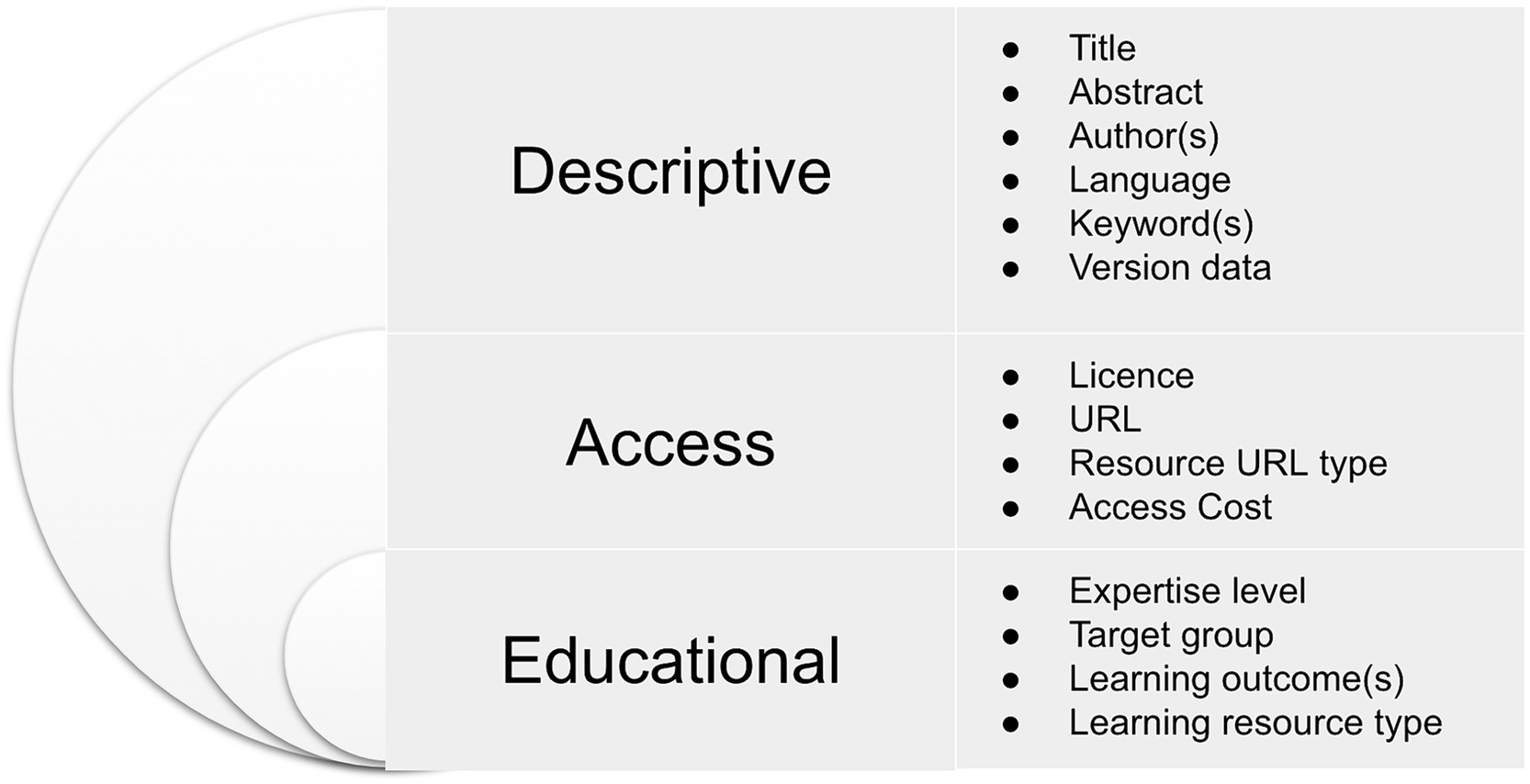
Figure 2. Summary of minimal metadata set for learning resources, adapted from the RDA interest group on Education and Training on Handling Research Data.
The differences between the guidelines proposed by the RDA and those described by Garcia et al. (2020) demonstrate that the field may be advancing rapidly, but consensus on a metadata standard for learning resources remains elusive. Whilst metadata standards provide essential guidance for managing learning resources, it is important to note that they also have limitations. To the best of our knowledge, to date, no learning resources metadata standards address issues related to the quality of learning resources. The complexity of addressing these issues is well-recognised within the community (Gurwitz et al., 2020). It is therefore crucial to make the distinction between the FAIRification of learning resources and their quality, as these are distinct challenges that are currently addressed separately.
2.3 Research communities and FAIR learning resources
Metadata standards are instrumental in advancing discussions about FAIRifying learning resources, but implementing these rules in practise poses certain challenges. A pressing concern is how to retrofit FAIR metadata standards to pre-existing learning resources since many of these are currently associated with little or no metadata. Contacting the original author(s) may not be possible, as training is offered by educators who are sometimes transitory, including contract researchers, postgraduate students and non-academics as well as professional educators. The broader challenges of FAIR are reflected here, too, including a lack of resources to implement FAIR practises, the absence of standardised ontologies, and low levels of community awareness [Directorate-General for Research and Innovation (European Commission), 2020].
As learning resources continue to grow, research communities are taking a more active role in discussions about making these resources FAIR. This reflects their commitment to ensuring that their training outputs have the desired impact, as well as their growing dedication to broader changes in the Open Science landscape, such as the development of interdisciplinary initiatives (e.g., EOSC portal).
Despite the commitment to FAIR learning resources, each research community faces challenges in implementation: their structure, objectives, policies, management, funding, sustainability, and strategies for long-term curation. Moreover, the sources of the learning resources vary greatly, which influences how FAIRness is conceptualised and implemented. The case studies presented in this paper represent a heterogeneous mix of research communities, highlighting how community structure influences the manner in which the FAIRification of learning resources is implemented. The analysis seeks to pull expertise and experience from these diverse communities so as to apply it across different learning platforms.
2.4 Selection of the case studies
Research communities are highly heterogeneous in their structure, focus and funding. The eight case studies selected for this paper represent the heterogeneity of these communities and include representatives from life sciences, social sciences and humanities. The case studies also represent a diversity of funding mechanisms, including independent organisations, funded projects and volunteer communities (see Table 1 in section 2.1 below).
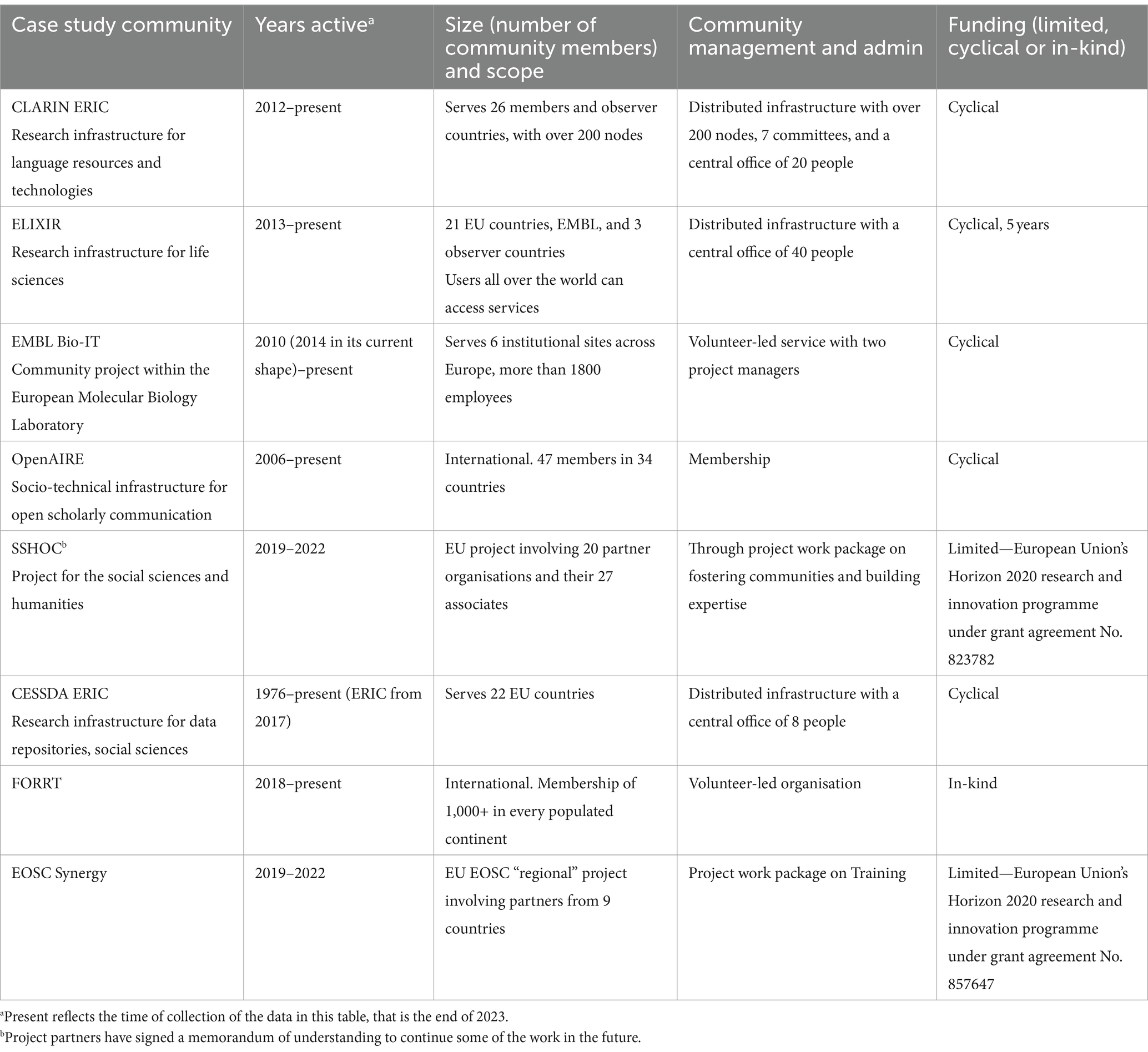
Table 1. Summary of key characteristics of the eight research community case studies analysed in this paper.
The selection of the case studies was purposive, and all authors of the case studies are members of the Community of Practise of Training Coordinators, an informal network of trainers who meet online to share training experiences and expertise. The community was initiated by a group of people coordinating training programmes in research domains and infrastructures, and e-infrastructures. One of its key elements is to strengthen training capacity by improved alignment and cross-infrastructure training activities. The case study authors, who are also authors of this paper, have key roles in coordinating and organising training in their communities.
2.5 Structure of case studies and analysis
Each case study was produced by representatives from the research community, and since these were self-reflections, it is important to highlight that the case studies are self-reported and that there was no desk research to test the FAIRness of the resources. Contributors were asked to provide a narrative reflection3 on the following topics.
• Overview of the research community, scope and objectives.
• Description of learning resources and training activities.
• Activities to FAIRify learning resources (including any metadata standards developed).
• Challenges and opportunities in FAIRifying learning resources.
The data was gathered in 2022 by each community representative based on existing information and ongoing activities within each research community and then reported in a shared document. The narrative reflection from each research community proved a valuable means of associating challenges and opportunities with structural characteristics, including funding, scope, longevity of community and engagement of the research community. These reflections are made available as Appendices.
Rather than providing one-size-fits-all recommendations for all research communities, the critical comparison of case studies we propose here highlights how different communities present different FAIR processes, challenges and opportunities. It is intended that the analysis and discussion sections will offer guidance to research community managers and training coordinators considering implementing FAIR practises, and draw attention to key considerations based on the specific needs of their community. Furthermore, the collection of narratives gathered as Appendices can be used as descriptive examples for FAIRification of training materials at different levels of FAIR maturity.
3 Implementing FAIR standards for learning resources: results
3.1 Case studies overview
This section presents the analysis of the narratives on FAIRification of learning resources from eight case studies drawn from various researcher communities. They include The Common Language Resources and Technology Infrastructure (CLARIN; humanities), the Social Sciences and Humanities Open Cloud (SSHOC; SSH cluster), the Consortium of European Social Science Data Archives (CESSDA; social sciences), the research infrastructure ELIXIR (life sciences), EMBL Bio-IT (computational biology), an infrastructure for Open Scholarly Communication OpenAIRE (multidisciplinary), the Framework of Open and Reproducible Research Training (FORRT; multidisciplinary), and EOSC Synergy (a regional project). Each research community has dedicated training activities and reusable content for their communities, and these have been catalogued and made available by varying methods. Further details from each community are available in the extended Appendices. The case study organisations are heterogeneous in size, focus, age and funding structures as reflected in Table 1.
Whilst some organisations benefited from continuous funding and full-time staff, others are entirely volunteer-led with cyclical or limited funding. The following sub-section aims to show the characteristics that played important roles in these organisations’ efforts to make learning resources as open and FAIR-compliant as possible.
3.2 Organisational strategies to FAIRify learning resources and recurring challenges
The practical strategies implemented within the eight research communities to FAIRify their learning resources are summarised in Table 2, highlighting the variety of approaches they relied on to enact FAIRness. These strategies can be translated into a set of practical recommendations for research communities wishing to do the same.
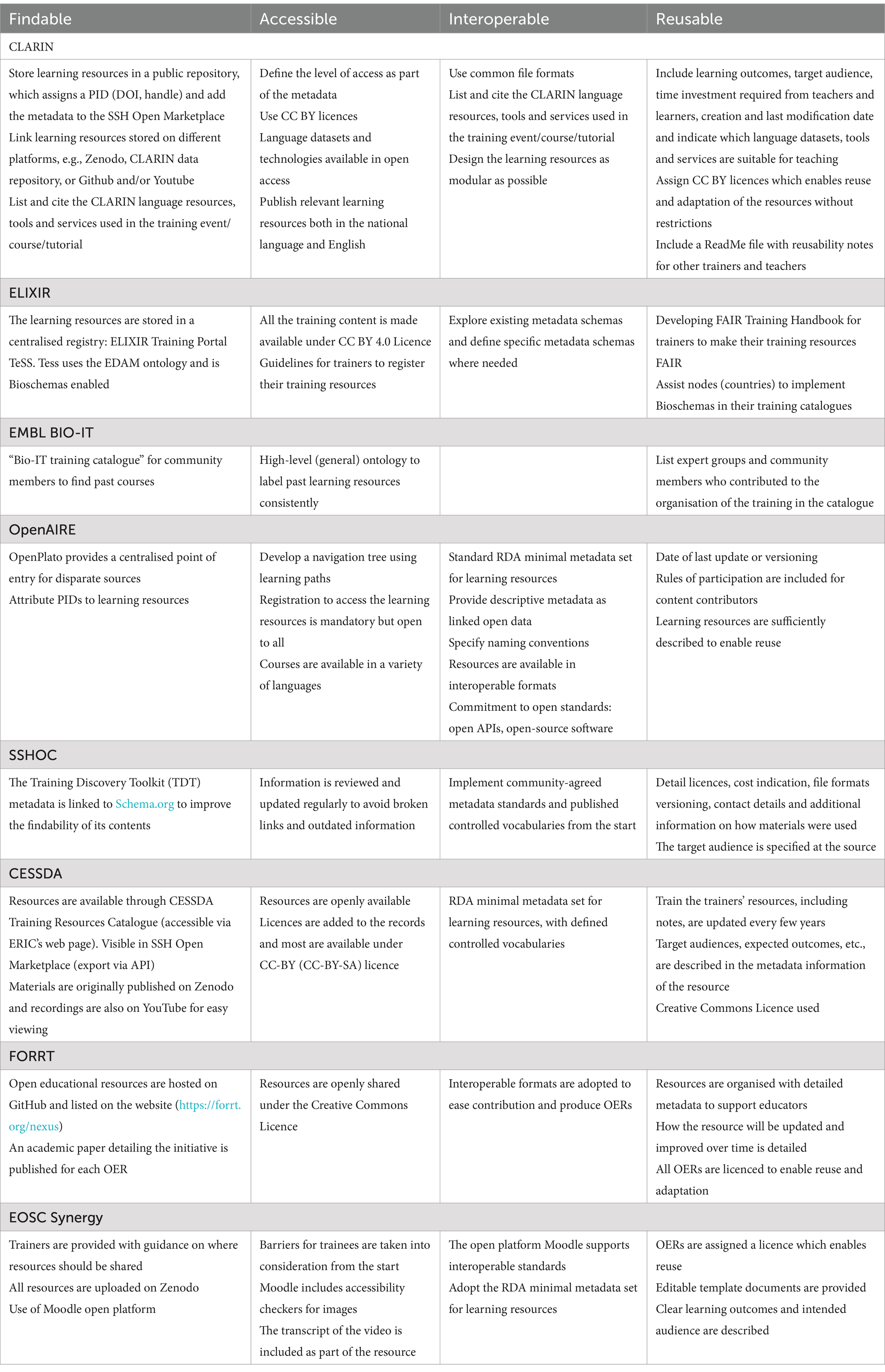
Table 2. Strategies to make learning resources FAIR in practise, as reported by the case study communities.
In addition, the case study narratives in the Appendices show that heterogeneous research communities come across similar challenges. To facilitate navigating the case studies, we grouped the most recurrent challenges into five categories, as follows:
• Hesitance of sharing and trainer buy-in to FAIRification practises.
• Selecting community metadata standards and getting consensus.
• Ensuring funding and sustainability for training catalogues and curation.
• Balancing urgency of FAIRifying learning resources with future changes to FAIR standards.
• Quality assurance and “orphan” learning resources.
Table 3 indicates which challenges were encountered by each case study community in their efforts to FAIRify their learning resources. By highlighting the most recurrent challenges, we aim to help readers navigate the content of the case studies.
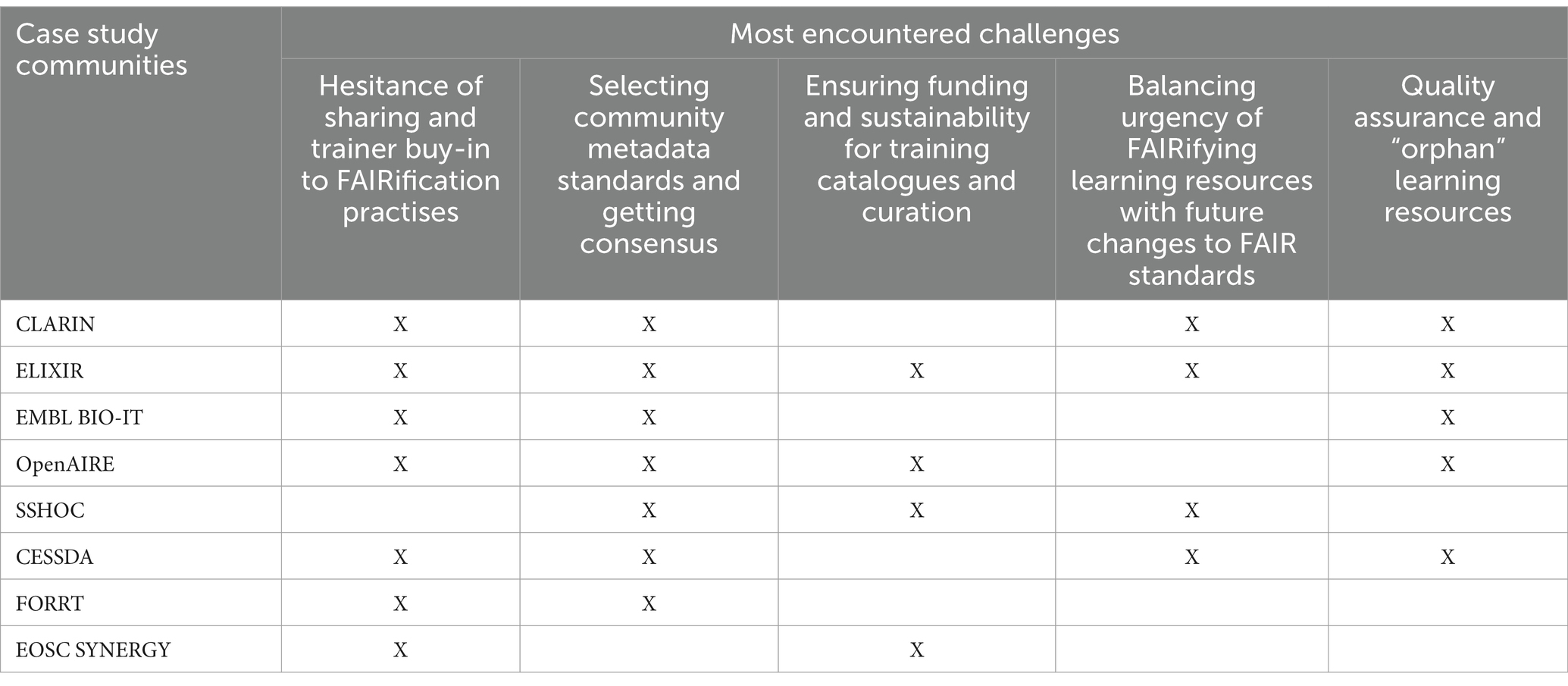
Table 3. Main challenges encountered by the eight case study communities in making their learning resources FAIR.
4 Further analysis and discussion
4.1 Hesitance of sharing and trainer buy-in to FAIRification practises
FORRT, CESSDA and EOSC Synergy noted that contributors had concerns about reuse of their learning resources. In particular, receiving credit for resources in the event they were used—either in their entirety or as part of an extensive training activity. Such concerns are not uncommon, and link to similar issues within Open Science/Open Data discussions (Fecher and Friesike, 2014). Without robust, transparent and widely adopted systems of accreditation and recognition, both for trainers and resources, it is unlikely that the concerns of sharing and reuse will be properly addressed. An example of activities related to this challenge is currently underway at ELIXIR, where a Certification Working Group is working towards the definition of a Training Resource Certification process (i.e., capturing training resources and events) together with the respective criteria, with the ultimate goal of establishing and implementing a community-endorsed Training Resource Certification badge.
In addition, it is likely that many trainers continue to be unaware of credit systems or open licences. This lack of awareness is a current challenge for FAIR data, and it is expected to be more pronounced for learning resources. The case studies highlight the need for further awareness raising amongst teaching communities so that the perceived harms of sharing resources are addressed or ameliorated. In these evolving discussions, it is important to recognise that, for many trainers (and particularly early career individuals), teaching content remains their career “currency” and means of transitioning from contract-focused employment to permanent positions. The importance of this issue means that it must continue to assume a prominent role in discussions.
The CLARIN case study illustrates an additional complication of sharing learning resources, namely institutional permission and licences. Because learning resources are often created for institutional programmes, issues of ownership and access need to be considered on both individual and institutional levels. As many institutions regard their curricula as proprietary content, it is likely that there will not be a “one-size-fits-all” set of guidelines outlining content sharing. Instead, such issues will need to be negotiated on a case-by-case basis. Nevertheless, the learning resources deposited in a CLARIN national research data repository can be assigned an appropriate licence (public, academic, restricted).
The case studies offer two key instances where training communities can offer support in overcoming these challenges. First, the accumulation of positive examples and individual testimonials within trusted communities may encourage hesitant contributors to share their resources. Second, the discussion of institutional complications within these communities could lead to the evolution of advice and policy documents and other means of support that could assist individual researchers in getting institutional buy-in for the sharing of learning resources.
4.2 Selecting community metadata standards and getting consensus
Most case studies highlighted challenges around selecting metadata standards within their communities. The different community structures illustrated the various ways in which the case study networks initiated the discussions and implemented the outcomes. Apart from the challenges involved in setting ontologies and metadata templates, the case studies highlighted several related challenges. For example, CLARIN flagged the difficulty of creating consistent metadata for pre-existing learning resources, whilst SSHOC emphasised the effort it takes to update and curate resources. Both examples demonstrate the need for engaging either volunteer communities or dedicated technical staff in the manual input and curation process of the learning resources.
As learning resource catalogues proliferate, issues of metadata are also associated with a number of technical issues. EMBL Bio-IT highlighted the decisions around DOI attribution, whilst CESSDA outlined the difficulties of dealing with small documents and the utility of preserving them. For the case studies spanning multiple countries, issues of multiple languages and style of resources were additional elements to consider.
The case studies highlight not only the importance of establishing community-endorsed metadata standards, but also the challenges in developing and implementing such tools in their learning resources and programmes. Some of these implementation challenges are of a technical nature. Still, it also has to be realised that it takes considerable human resources to undertake this effort, and this may be very difficult to ensure: the structure of the communities relates to both the challenges and the opportunities of such endeavours. Nevertheless, regardless of community structure, our case studies demonstrate that adopting clear community standards for the documentation of learning resources is key to improving FAIRness.
One common thread that came out of some of the case studies was that the RDA ETHRD’s output on minimal metadata has already been adopted by several communities. This provides evidence of the utility of “off-the-shelf” solutions that can be contextually adapted. This can minimise the input needed—something of particular importance for volunteer communities with limited dedicated time resources. The widespread adoption of these standards could lead to a rapid achievement of critical mass and ease considerations around interoperability. Although retrofitting of metadata has been investigated in some instances, the process has proved time-consuming; hence it is best to adopt a common standard from the outset.
4.3 Ensuring funding and sustainability for training catalogues and curation
Our case studies represent communities that are supported by a diverse range of different funding models. OpenPlato and CESSDA, for instance, have long-term funding in place, as is the case for research infrastructures like CLARIN and ELIXIR, whilst EOSC Synergy and SSHOC were limited-time projects. Funding timescales are intertwined not only with the future availability of learning resources, but also their curation: sustainability and longevity, although central to the FAIR principles, do not feature explicitly in any of the guidelines.
EOSC Synergy and OpenPlato note the importance of selecting an open platform which supports interoperable standards and will enable learning resources from limited-time projects to be adopted and integrated into future projects, thus ensuring long-term sustainability. ELIXIR’s Training Portal TeSS, a registry with training events and resources from training providers in and beyond ELIXIR (Beard et al., 2020), is committed to the FAIR principles, and both the content and the codebase of TeSS is available under appropriate licences. It is clear that sustainability decisions need to become part of the mission and roadmaps of Research Infrastructures (RIs) and institutions to ensure that learning resources are not lost through lack of funding or curation provision.
Moreover, it is important to recognise that the topicality and usability of learning resources change regularly. Whilst limited-time projects share resources deemed important at the time, there is no guarantee that these will continue to be important to keep in perpetuity. The subsuming of limited-time project learning resources into longer-term catalogues is accompanied by a burden of quality assessment, revision and curation. Such activities are time-intensive and require considerable expertise. The evolution of communities, such as those fostered by OpenAIRE and FORRT will be crucial in taking such activities forward.
4.4 Balancing urgency of FAIRifying learning resources with future changes to FAIR standards
A number of the case studies, such as EMBL BioIT, SSHOC, and CLARIN outline the difficulties of applying FAIR to existing learning resources. In addition, they highlight an urgency to applying FAIR to their training catalogues—not only to enhance the reusability of their collections but also to inform the evolution of their platforms in the future. These case studies foreground a conundrum: defining what is “FAIR enough” for their purpose. Are there compromises on metadata standards that need to be made in developing possible solutions to implement that the communities will commit to follow?
Taking such decisions requires both leadership and community endorsement, but these are complicated by a rapidly changing FAIR landscape, leading to concerns about the future interoperability of catalogues and resources. The case studies note, however, that they do not have the luxury to wait, and that future revisions and changes are inevitable. Such observations are invaluable to other RIs, projects, and institutions as they highlight that waiting for standards and practises to stabilise can mean waiting indefinitely to start.
4.5 Quality assurance and “orphan” learning resources
Issues of quality featured prominently in all the case studies. The narratives highlighted not only the difficulty of setting out criteria with which to assess the quality of learning resources, but also the challenges relating to the future—continued quality, relevance and utility. It is important to note that the FAIR principles say nothing about quality, and that the reusability of resources does not equate to their utility.
Some of our case studies outlined curation sprints and other community practises. As the volume of learning resources continues to grow online it is likely that such activities will become ever more essential. In particular, community curation activities will assist in maintaining the quality of “orphan” resources whose creators are no longer active within (academic) training. For these sprints to be effective, it is important to note that consensus on the licences applied to learning resources is needed so as to facilitate the necessary amendment and updating activities.
5 Conclusion
The expansion of discussions on FAIR principles to learning resources is an important milestone in the field of OERs. The FAIRification of existing and future standards will address some of the recognised existing challenges relating to searchability/findability, interoperability/reuse and long-term curation/sustainability. Nonetheless, as with all aspects of FAIR, it must be recognised that applying the FAIR principles to learning resources is an evolving practise and requires considerable investment.
This paper presented the experiences of eight communities who have committed to making their learning resources FAIR. Through the experiential narratives, we highlight some of the complexities of implementing FAIR into learning resource management. These complexities relate not only to the nature of the learning resources, but also to the structural characteristics of the communities producing and managing them. Understanding the resources available to answer questions such as who will FAIRify the materials and curate them are critical to any successful FAIR venture.
The paper, together with the narratives in the Appendices, is intended to not only inspire other disciplinary communities to consider FAIRifying learning resources, but also to highlight the range of pragmatic and practical concerns necessary to confront. It is anticipated that this paper, together with the discussion it fosters, will strengthen the collaborative effort of research communities towards a future FAIR training landscape.
Data availability statement
The original contributions presented in the study are included in the article/Supplementary material, further inquiries can be directed to the corresponding author.
Author contributions
LP: Conceptualization, Data curation, Formal analysis, Investigation, Methodology, Supervision, Validation, Visualization, Writing – original draft, Writing – review & editing. LB: Conceptualization, Data curation, Formal analysis, Investigation, Methodology, Validation, Visualization, Writing – original draft, Writing – review & editing. SV: Resources, Conceptualization, Data curation, Formal analysis, Investigation, Methodology, Validation, Visualization, Writing – original draft, Writing – review & editing. IL: Conceptualization, Data curation, Formal analysis, Investigation, Methodology, Validation, Writing – original draft, Writing – review & editing. CG: Conceptualization, Data curation, Formal analysis, Investigation, Methodology, Validation, Writing – original draft, Writing – review & editing. IK: Conceptualization, Data curation, Formal analysis, Investigation, Methodology, Validation, Writing – original draft, Writing – review & editing. EL: Conceptualization, Data curation, Formal analysis, Investigation, Methodology, Writing – original draft. FA: Conceptualization, Investigation, Methodology, Writing – original draft, Writing – review & editing. IB: Conceptualization, Data curation, Writing – review & editing. LP: Data curation, Formal analysis, Writing – review & editing. HC: Conceptualization, Data curation, Writing – original draft. RB: Writing – review & editing.
Funding
The author(s) declare that no financial support was received for the research, authorship, and/or publication of this article.
Conflict of interest
The authors declare that the research was conducted in the absence of any commercial or financial relationships that could be construed as a potential conflict of interest.
Publisher’s note
All claims expressed in this article are solely those of the authors and do not necessarily represent those of their affiliated organizations, or those of the publisher, the editors and the reviewers. Any product that may be evaluated in this article, or claim that may be made by its manufacturer, is not guaranteed or endorsed by the publisher.
Supplementary material
The Supplementary material for this article can be found online at: https://www.frontiersin.org/articles/10.3389/feduc.2024.1390444/full#supplementary-material
Footnotes
1. ^We follow the definition from the ELIXIR Research Data Management toolkit for Life Sciences, https://rdmkit.elixir-europe.org/. Vocabularies and ontologies describe concepts and relationships within a knowledge domain. Used wisely, they can enable both humans and computers to understand data. There is no clear-cut division between the terms “vocabulary” and “ontology,” but the latter is more commonly used when dealing with complex (and perhaps more formal) collections of terms and relationships. Ontologies typically provide an identifier.
2. ^We follow the UNESCO definition of Open educational resources (https://www.unesco.org/en/open-educational-resources) which defines OERs as “learning, teaching and research materials in any format and medium that reside in the public domain or are under copyright that have been released under an open licence, that permit no-cost access, re-use, re-purpose, adaptation and redistribution by others.”
3. ^The narrative reflections discussed here consist of eight case studies carried out by the authors of this paper in their respective organisation, therefore no written informed consent was needed to participate in the study. These narratives are provided as Supplementary data. The aim of providing this collection of case studies (or narrative reflections) as appendices is to showcase different narratives from research communities on making their learning resources and catalogues FAIR.
References
Atenas, J., and Havemann, L. (2014). Questions of quality in repositories of open educational resources: a literature review. Res. Learn. Technol. 22:20889. doi: 10.3402/RLT.V22.20889
Beard, N., Bacall, F., Nenadic, A., Thurston, M., Goble, C. A., Sansone, S.-A., et al. (2020). TeSS: a platform for discovering life-science training opportunities. Bioinformatics 36, 3290–3291. doi: 10.1093/bioinformatics/btaa047
Directorate-General for Research and Innovation (European Commission) (2018). Turning FAIR into reality: final report and action plan from the European Commission expert group on FAIR data. Brussels: Publications Office of the European Union Available at: https://data.europa.eu/doi/10.2777/1524.
Directorate-General for Research and Innovation (European Commission) (2020). Six Recommendations for implementation of FAIR practice by the FAIR in practice task force of the European open science cloud FAIR working group. Brussels: Publications Office of the European Union Available at: https://data.europa.eu/doi/10.2777/986252.
Directorate-General for Research and Innovation (European Commission) (2022). European Research Area policy agenda: overview of actions for the period 2022–2024. Brussels: Publications Office of the European Union Available at: https://data.europa.eu/doi/10.2777/52110.
Fecher, B., and Friesike, S. (2014). “Open Science: one term, five schools of thought” in Opening science. eds. S. Bartling and S. Friesike (Cham: Springer International Publishing).
Garcia, L., Batut, B., Burke, M. L., Kuzak, M., Psomopoulos, F., Arcila, R., et al. (2020). Ten simple rules for making training materials FAIR. PLoS Comput. Biol. 16:e1007854. doi: 10.1371/journal.pcbi.1007854
Gurwitz, K. T., Singh Gaur, P., Bellis, L. J., Larcombe, L., Alloza, E., Balint, B. L., et al. (2020). A framework to assess the quality and impact of bioinformatics training across ELIXIR. PLoS Comput. Biol. 16:e1007976. doi: 10.1371/journal.pcbi.1007976
Higman, R., Bangert, D., and Jones, S. (2019). Three camps, one destination: the intersections of research data management, FAIR and Open. Insights: UKSG J. 32. doi: 10.1629/UKSG.468
Hoebelheinrich, N. J., Biernacka, K., Brazas, M., Castro, L. J., Fiore, N., Hellström, M., et al. (2022). Recommendations for a minimal metadata set to aid harmonised discovery of learning resources. Research Data Alliance. doi: 10.15497/RDA00073
Ossiannilsson, E. (2020). “Some challenges for universities, in a post crisis, as COVID-19” in Radical solutions for education in a crisis context. Lecture notes in educational technology. eds. D. Burgos, A. Tlili, and A. Tabacco (Singapore: Springer).
Roncevic, M. (2021). Open educational resources: the story of change and evolving perceptions. Open Research Community. Available at: https://openresearch.community/posts/open-educational-resources-the-story-of-change-and-evolving-perceptions
Keywords: accessible, interoperable, Open Science, training, research communities, open educational resource (OER), FAIR principles in open education, findable
Citation: Provost L, Bezuidenhout L, Venkataraman S, van der Lek I, van Gelder C, Kuchma I, Leenarts E, Azevedo F, Brvar IV, Paladin L, Clare H and Braukmann R (2024) Towards FAIRification of learning resources and catalogues—lessons learnt from research communities. Front. Educ. 9:1390444. doi: 10.3389/feduc.2024.1390444
Edited by:
Francis Thaise A. Cimene, University of Science and Technology of Southern Philippines, PhilippinesReviewed by:
Natasha Jeanne Gownaris, University of Washington, United StatesDasapta Erwin Irawan, Bandung Institute of Technology, Indonesia
Copyright © 2024 Provost, Bezuidenhout, Venkataraman, van der Lek, van Gelder, Kuchma, Leenarts, Azevedo, Brvar, Paladin, Clare and Braukmann. This is an open-access article distributed under the terms of the Creative Commons Attribution License (CC BY). The use, distribution or reproduction in other forums is permitted, provided the original author(s) and the copyright owner(s) are credited and that the original publication in this journal is cited, in accordance with accepted academic practice. No use, distribution or reproduction is permitted which does not comply with these terms.
*Correspondence: Lottie Provost, bG90dGllbWlhcHJvdm9zdEBjbnIuaXQ=