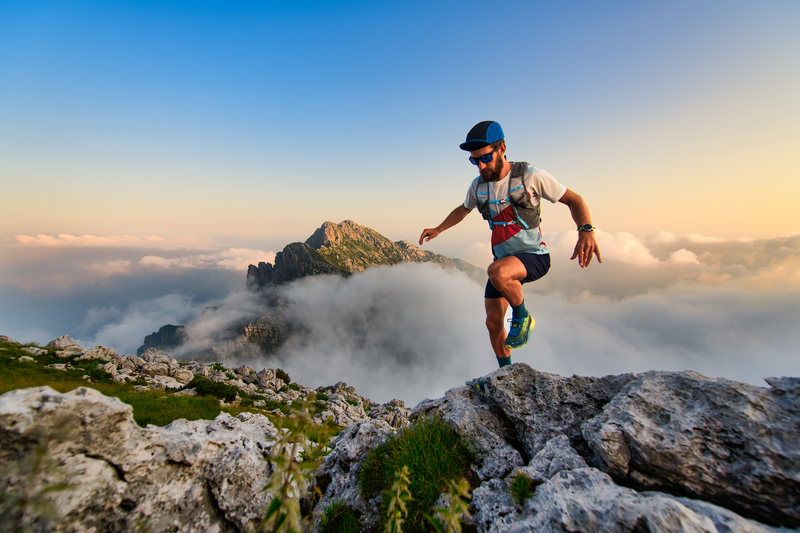
94% of researchers rate our articles as excellent or good
Learn more about the work of our research integrity team to safeguard the quality of each article we publish.
Find out more
ORIGINAL RESEARCH article
Front. Educ. , 17 July 2024
Sec. Higher Education
Volume 9 - 2024 | https://doi.org/10.3389/feduc.2024.1380865
Participation in non-formal adult education (NFE) is considered an essential part of lifelong learning, aiming to foster the development of individuals and societies. This significance is particularly evident today, given the era of extensive digitalization and disruptive technological advances. Nevertheless, not all adults participate in organized learning and have equal chances. Therefore, this study addresses the absence of up-to-date comparative findings on participation in NFE in the post-COVID-19 world. To this end, we examine current trends in NFE participation in four European countries: Sweden, Germany, the United Kingdom, and the Czech Republic (RQ1), where we explore the impact of key micro (social and behavioral), meso (job-related), and macro-level (country-specific) factors on this participation (RQ2). Our findings indicate that NFE participation remained relatively stable in 2022 compared to 2016, except for Sweden and Germany, which achieved higher participation rates. However, differences in participation between countries are diminishing. Simultaneously, NFE participation is becoming increasingly job-oriented and receives more support from employers. However, it is important to note that inequality in access to NFE persists, as the main predictors of NFE participation, such as learning intentions, educational attainment, economic and occupational status, remain consistent, regardless of the participants’ economic activity and country of birth. This underscores the enduring significance of a key concept behind this study: the willingness to engage in organized NFE exhibits a complex structure with multiple layers.
In an age defined by rapid technological progress and evolving workforce requirements (ILO, 2022; WEF, 2023), the importance of non-formal adult education (NFE) has never been more relevant (UNESCO, 2020). As individuals strive to adapt and enhance their skills to remain competitive in the job market and cope with changes in their everyday lives, NFE emerges as a vital pathway for personal and professional growth (CEDEFOP, 2018). To underscore its significance, participation in organized adult learning has become a foundational element of the European Union’s social rights (EC, 2017). Furthermore, engagement in NFE is recognized as a catalyst for fostering social cohesion, encouraging civic participation, and enhancing individual well-being (EC, 2020; Iňiguez-Berrozpe et al., 2020; Boyadjieva and Ilieva-Trichkova, 2022; Holford et al., 2022). However, understanding the dynamics of NFE participation and the persistent inequalities within this educational domain is essential to ensure equitable access to opportunities for all.
The NFE represents institutionalized learning activities organized by an educational provider that are happening outside of the formal educational system, such as courses, workshops, seminars, on-the-job training, or private lessons for either personal or job-related reasons (CLA, 2016). In this regard, NFE serves as a crucial instrument for lifelong learning and skill development. It is pivotal in empowering adults to acquire new competencies, bridge knowledge gaps, and enhance their capabilities (Boyadjieva and Ilieva-Trichkova, 2022).
This article delves into the contemporary landscape of NFE, shedding light on two compelling aspects of this educational domain. First, it examines the trends in NFE participation, drawing on new comparative research from 2022. The second aspect under investigation is inequality in NFE participation. Despite the numerous benefits associated with NFE, such as personal growth and enhanced employability, certain segments of the population need to be more represented in NFE activities. This includes individuals with lower levels of formal education (ISCED 0–3), those not currently in the workforce, individuals in low-skilled occupations and older adults. Persistent inequalities in NFE access persist (Rubenson, 2018; Desjardins, 2020; Jenkins, 2021; Boeren, 2023), highlighting the need for a comprehensive understanding of the underlying causes.
To address these two aspects of NFE participation, this article takes a comparative approach, focusing on four distinct countries: Sweden, Germany, the United Kingdom, and the Czech Republic. The countries are selected based on the principle of the Most Different Systems Design (Landman and Carvalho, 2017), representing distinct types of adult learning systems (see part 1.2 below for more details). These systems emerge from unique institutional clusters, each placing varying emphasis on NFE as a tool for continuous human capital investment strategy (Desjardins, 2017). Consequently, the selection provides a valuable perspective on the universality of NFE trends and inequalities across diverse national institutional settings.
The first topic of this article is related to the question of how many adults are involved in NFE. To address this question, comparative research, based predominantly on data from large-scale international surveys like the International Adult Literacy Survey (IALS), the Programme for the International Assessment of Adult Competencies (PIAAC) organized by OECD and the Adult Education Survey (AES) by Eurostat, conducted between the 1990s and the 2010s, has revealed two main trends in NFE within advanced industrial and post-industrial countries.
On the one hand, participation in organized adult learning has significantly increased over the last three decades. While countries with high participation rates in the 1990s have experienced modest growth, countries with lower participation rates at the start underwent a much steeper rise (Desjardins et al., 2016; Rubenson, 2018; Desjardins, 2020; Desjardins and Ioannidou, 2020). On the other hand, there has been a growing emphasis on the job-related NFE, particularly those financially supported by employers (Boeren and Holford, 2016; Desjardins et al., 2016; Rubenson, 2018; Desjardins, 2020). These trends have reinforced each other, with countries with a profound job orientation of NFE tending to have higher overall rates of adults involved in organized learning.
The second topic addressed in this article explores the factors that influence why some individuals participate in NFE more than others. While the scientific literature mentions extensive individual and societal benefits associated with NFE (Desjardins, 2019; Iňiguez-Berrozpe et al., 2020; Boyadjieva and Ilieva-Trichkova, 2022), numerous studies (e.g., Dämmrich et al., 2014; Blossfeld et al., 2020; Desjardins and Ioannidou, 2020; Jenkins, 2021; Boeren, 2023) have highlighted persistent inequalities in access to NFE opportunities and the resulting unequal advantages.
Typically, individuals with lower levels of the highest attained education (ISCED 0–2), those who are not currently in the workforce, those in low-skilled occupations, older adults, and migrants tend to participate in NFE less than other segments of the population (Boeren, 2016, 2023; Rubenson, 2018; Cabus et al., 2020). Although some scholars (Desjardins and Kim, 2023) have pointed out that overall participation inequality has decreased since the 1990s, certain inequalities continue to exist (Desjardins, 2020; Jenkins, 2021).
Following the previous discussion, this article aims to illuminate two aspects: (1) current participation trends in NFE, examining whether these trends have persisted since the last systematic assessment conducted in the mid-2010s by AES (RQ1); (2) the factors contributing to unequal participation in NFE activities, with a focus on identifying persistent inequalities and understanding their underlying sources (RQ2).
We will address these two questions in a comparative context, focusing on four countries: Sweden, Germany, the United Kingdom, and the Czech Republic. The impact of various factors traditionally associated with variations in NFE participation and related inequality – such as educational attainment, occupation, and age – is influenced by the specific welfare and educational institutions within each country (Thelen, 2014). To explore the universality of trends in NFE participation and inequality across different institutional settings (see Table 1), we intentionally selected four countries with very distinct adult learning systems (Roosmaa and Saar, 2012, 2017; Desjardins, 2017, 2023; Saar and Räis, 2017). Within the European context, these countries are characterized by various welfare state regimes represented by different institutional arrangements (i.e., employment protection and family policies) with impact perceived barriers to participation in adult education (Rubenson and Desjardins, 2009; Roosmaa and Saar, 2017).
Sweden is among the countries that have prominently included NFE in their social investment policy from the outset, boasting a robust welfare state and consistently high participation rates in adult education, with relatively low levels of inequality (Rubenson, 2006; Rubenson and Desjardins, 2009). This extensive Nordic countries’ state policies, which constitute the social-democratic welfare regime type (Esping-Andersen, 1999), show a great emphasis on the equalization of employment chances, particularly regarding continuing training (Dieckhoff and Steiber, 2011).
In contrast, the United Kingdom is a classic example of a liberal welfare regime with extensive commodification of public services, minimized role of the state, and limited social investment policies with low employment protection, social benefits and support for NFE (Esping-Andersen, 1990, 1999; Rees, 2013). However, high employer investment in employee training, stemming from the reliance on general initial education and ongoing workplace training (Hall and Soskice, 2001), results in relatively high NFE participation, albeit accompanied by inequality related to labor market integration (Roosmaa and Saar, 2012, 2017).
Germany exemplifies a typical dual adult learning system (Desjardins, 2017, 2023) where both the state and stakeholders share responsibility for coordinating organized adult training. Due to early occupational specialization within the formal education system, the demand for NFE is lower, compared to the United Kingdom. Nevertheless, the level of inequality is influenced by stronger investments in the skills of workers who require support due to the stronger position of professional associations and unions (Roosmaa and Saar, 2012, 2017; Rees, 2013; Desjardins, 2017).
Finally, the current adult learning system in the post-socialist Czech Republic only began to take shape after the fall of socialism, signifying an important institutional break from the past. It has been characterized by limited social investment policies and increasing liberalization (Kopecký and Šerák, 2015). Along with moderate employment protection for regular workers (ILO, 2004), this pattern suggests a relatively lower level of participation and substantial inequality (Roosmaa and Saar, 2012, 2017).
The theoretical foundation of this paper draws from the multilayer approach to participation inequality in lifelong learning, originally developed by Boeren (2016, 2017, 2023); also explored by Boeren et al. (2010), and alternatively discussed by Cabus et al. (2020). Additionally, it is influenced by the conceptual model of participation in adult education presented by Desjardins (2014), which was further empirically expanded upon by Saar and Räis (2017).
Boeren (2016, 2023) initially formulated an Integrated Lifelong Participation Model, which categorizes the factors influencing participation in adult education and training into three main sets. The first set encompasses micro-level factors related to participation, which include the social and behavioral characteristics of adults. They involve variables commonly examined in sociological research, such as age, gender, highest attained education, and occupation status. Behavioral characteristics, on the other hand, involve psychological constructs frequently used in research, such as motivation, needs, learning intentions, or attitudes toward lifelong learning.
The second set explores meso-level factors, i.e., how participation in lifelong learning is influenced by job-related characteristics including learning providers, which usually take two forms. One form involves learning institutions, with their specific attributes, including accessibility, cost, and flexibility. The other form centers on workplaces, which play a pivotal role in providing opportunities for job-related NFE. Factors like company size, specialization, and NFE funding determine participation in this context.
The third layer in Boeren’s integrated model pertains to the macro-level factors in the form of country-specific institutional features and adult education policies. This, for example, includes aspects such as investments in active labor market policies (ALMPs), the standardization of lifelong learning, and key economic characteristics with varying requirements for continuous skill development.
In line with the political economy of education and building upon Desjardins’s (2014) conceptual model (also discussed by Saar and Räis, 2017), this model operates with two overarching sets of factors. The first set consists of micro-demographic characteristics of adults, encompassing micro-level factors like age, immigrant status, education, and skill level. These factors pertain to the skill supply within the adult population and the corresponding need for structured learning opportunities. The second set relates to institutional macro-demographics of the workplace and job, which includes factors such as firm size, occupation, industry, and the utilization of skills in the workplace. These factors account for the demand for training among economically active adults and correspond to meso-level factors in Boeren’s (2016, 2023) integrated model.
Much like Boeren’s approach, this model does not solely focus on individual characteristics and their intention for participation. It also considers the structural aspects responsible for contributing to inequality in access to lifelong learning opportunities. Drawing from data collected in the PIAAC survey conducted between 2010 and 2012, Saar and Räis (2017) discovered that these structural meso-level features exert a more substantial influence on participation compared to micro-level factors. Unlike the Integrated Lifelong Participation Model, this approach does not encompass the conceptualization and measurement of behavioral characteristics that are usually overlooked by the political economy of education.
Building upon these two theoretical frameworks, we posit that the decision to participate is not solely dependent on individual resources and learning intentions (micro-level factors). It also hinges on adults’ access to NFE opportunities and their position within the labor market structure (meso-level factors) that is framed by the specific country’s characteristics (macro-level factors). As a result, we concentrate on key sets of factors that help us understand the main determinants contributing to participation inequality in NFE (see Figure 1). In our study, these factors are:
1. Social (micro-level) factors encompass crucial social characteristics of adults, including their highest level of education, age, and gender. These factors correspond to the sociodemographic characteristics as outlined in Desjardins’s (2014) conceptualization.
2. Behavioral (micro-level) factors in our model refer to the learning intentions of adults on two levels: (a) their intentional commitment to learning and (b) their actual efforts in seeking information related to lifelong learning. In line with current literature (Baert et al., 2006; Van Nieuwenhove and De Wever, 2021), they represent some of the key behavioral variables responsible for participation.
3. Job-related (meso-level) factors can be thought of as the institutional macro-demographics of the workplace and job. They play a significant role (institutional structure) in representing and influencing the demand for NFE among economically active adults.
4. Country-specific (macro-level) factors in the form of different types of skill formation regimes (Busemeyer and Trampusch, 2012) and adult learning systems with various degrees of social investment and coordination of NFE (Desjardins, 2017).
Based on the state-of-the-art and research gaps outlined above, we aim to study the following two research questions while describing descriptive (RQ1) and multivariate (RQ2) results of the analysis on participation in NFE:
RQ1: What is the extent of adult participation in NFE in selected countries? (Current trends in participation in NFE).
RQ2: What are the main micro, meso, and macro-level determinants of participation in NFE? (Effect of the multilayer structure influencing participation in NFE).
The research presented here was situated within the field of adult organized learning, where participation in NFE has been typically measured by a periodical international AES, which tracks adult engagement in learning activities (Eurostat, 2023a). Four waves (2007, 2011, 2016, and 2022) of the AES (Eurostat, 2023b) for Sweden, Germany, the United Kingdom, and the Czech Republic, were used as part of the answer to RQ1 referring to the declared NFE participation of individuals 25 to 64-year-olds in the past 12 months.
The AES made use of the Labour Force Survey (LFS) as a sampling frame comprised of respondents who had completed their final LFS interview and had provided contact details for future research. A two-stage representative stratified random sample was drawn for AES (Eurostat, 2023a). This involved the stratification of the individuals on the sample frame into three age bands (aged 19–24, 25–64, and 65 years and overs), the selection of households where at least one adult had provided details for re-contact (primary sampling unit) within each age band, and a subsequent selection of one adult per household (final sampling unit). Within each selected household, one eligible adult was selected at random. The total net sample size of AES is around 230,000 individuals.
The second data source answering RQ1 and RQ2 consisted of 4,000 respondents drawn from a representative stratified random sample of adults aged 25–64 years from four European countries: Sweden, Germany, the United Kingdom, and the Czech Republic. In terms of size, the sample was set equally for all countries (1,000 per country) with regard to the planned analytical procedures and recommended sample size (Bujang et al., 2018). In terms of internal structure, it reflected gender, age, education, region, and size of residential locations ratio to the overall population.
Data collection was carried out online through a research panel during the summer of 2022 by a specialized agency using the Computer-Assisted Web Interview (CAWI) method. This means that only registered persons were addressed. In the Czech Republic, the response rate was 28%. The agency also ensured adequate collection of data in other monitored countries through cooperating partners within their international network. Although sample selection was designed to reflect the representativeness of the adult population in each country, it was not possible to achieve a representative distribution of educational attainment in the United Kingdom and Germany. Therefore, these samples exhibited a higher proportion of adults in tertiary education (see Supplementary Table S1 and the Limitations section for further details). There were no missing data included.
The average age of the respondents was 44.7 years (SD = 11.3 years) with equal distribution by gender. The proportion of respondents according to attained educational level showed that 20.4% of adults achieved lower levels of education (ISCED 3c or lower), and 79.6% of adults achieved higher levels of education: 30.2% with upper and post-secondary non-tertiary education (ISCED 3ab−4), and 49.4% with higher tertiary education (ISCED 5–8), respectively. A dominant proportion of respondents were economically active (3,031, 75.8%), and mostly full-time employed workers (2,241, 56%). Out of these numbers, 1,946 (48.7%) of respondents participated in any type of NFE in the past 12 months. The detailed descriptive sample distribution is included in Supplementary Tables S1, S2.
In all phases of the survey process, emphasis was placed on the ethical principles of research, especially anonymity, respecting the ICC/ESOMAR International Code (International Chamber of Commerce/ESOMAR, 2016). Verbal informed consent was obtained from all participants, and ethical implications were respected together with safety and rights of all respondents.
In order to compare our participation rates with data from AES in 2007, 2011, 2016, and 2022 (Eurostat, 2023b) for selected countries, we adhered to the uniform NFE definition used in the AES waves. The national languages (i.e., Swedish, German, English, and Czech) of the participating countries were used to create a questionnaire that included selected variables used in the present analysis (Supplementary Table S3). The target was to keep the national versions of the questionnaire as similar as possible applying an adequate rather than literal translation of the instructions and items. To do so, skilled translators were used to produce the national version, which was then critically assessed within the national group of participating researchers. Semantic disparities were discussed until an agreement was reached.
The dependent variable, participation in NFE, was for all data sources measured comparably by a dichotomous variable of the involvement in various forms of NFE within the last 12 months. NFE was defined as any organized adult education outside the formal education system that does not result in official certification at the ISCED level, such as courses, workshops, seminars, on-the-job training, or private lessons for either personal or job-related reasons (Eurostat, 2023a).
Based on previous research in the field, key sets of factors serving as independent variables were included in our analysis (see Figure 1). First, the social (micro-level) variables were measured by gender, age, and level of educational attainment. The second set of micro-level predictors involved behavioral variables in the form of an expressed need for education and real action in the form of seeking out learning possibilities. At meso-level, we included job-related variables in the form of economic, occupational, and employment status, type of employment contract, and company size. At macro-level, the country of birth for assessing country-specific variables was included in the analysis. An overview of the scale variables and their categories can be found in Supplementary Tables S1–S3.
To compare the primary results of the AES 2007–2022 (Eurostat, 2023b) on participation in NFE and to explore the current trend of NFE participation across selected countries, descriptive statistics in the form of absolute and relative frequencies and their differences were applied (RQ1). To analyze the impact of key factors on participation in NFE expressed by binary response variable, logistic regression models (Wuensch, 2021) were calculated (RQ2).
Since the influence of key variables is mostly considered descriptively in current studies, it is questionable whether its impact remains valid in a multivariate analysis or whether it persists under the interaction with other variables. Therefore, key micro (social and behavioral) and meso-level (job-related) variables were hierarchically (sequentially) entered into the equation by predetermined order in steps (or blocks), with macro-level (country-specific) variables serving as a baseline of all models. Therefore, each independent variable was assessed in terms of what it adds to the prediction of the dependent variable after the previous variables have been controlled for (Tabachnick and Fidell, 2007). Within each block, explanatory variables were added by the Enter method for simultaneously entering variables into a regression, whether it is or is not significant.
More specifically, Model 1 was restricted to social (micro-level) variables; Model 2 included social and behavioral (micro-level) variables and Model 3 represented the final model that included all tested variables. To better understand outcomes of the Most Different Systems Design (Landman and Carvalho, 2017), country-related (macro-level) variables were included in all blocks (models) to see how well each variable and a model as a whole is able to predict participation outcome. Based on our knowledge targeting employment status as a crucial precondition for participation in adult education and training (Boeren, 2016; Saar and Räis, 2017; Rubenson, 2018), we decided to conduct separate regression analysis for all respondents (n = 4,000) and respondents active in the job market (n = 3,031). Therefore, we distinguished between all respondents (Models 1–3) and respondents who are active in the job market (Models 4–6); otherwise, they share the same variables strategy.
From the long-term overview, the descriptive results show that on average within EU and Euro area countries the participation rate of adults aged 25–64 years rose up to 44 and 47% in 2022 with the most noticeable increase between 2007 and 2022, as demonstrated by included countries except Sweden (see Table 1). The increasing tendency of participation is clearly significant in the case of Germany, in which participation rose by 15 percentage points from 2007, with the historically highest number of adults participating in NFE (58%) in 2022. However, during the past 15 years, participation in Sweden was persistently the highest among countries; followed by Germany and the United Kingdom.
Overall, participation rates have increased between 2007 and 2016 in all countries except Sweden, reaching slow stagnation in 2022 for the participation rates in the Czech Republic (0 p.p.). Moreover, the change in participation rate between 2016 and 2022 was not as high as the chance in percentage points 9 years earlier in 2007 (see the last two columns of Table 1). Although all four countries started with widely different participation rates, they have converged over time and reached EU and Euro area average or above-average values as measured by the AES 2007–2022 (Eurostat, 2023a).
The increasing importance of active status in the labor market is highlighted in Table 2, showing our data collection. Almost three-quarters of participants involved in NFE in 2022 (between 73 and 76%) participated in mostly job-related training, while this training was entirely or in part paid by the employer (between 81 and 87%). Therefore, NFE in the post-COVID-19 world has become an activity that is mainly job-oriented as well as employer-sponsored. Furthermore, we should point out that participation in NFE that is not directly job-related is also considerably supported by employers, especially in Sweden (48% of mostly non-job-related NFE).
Looking more closely, participation in NFE is by no means evenly distributed among the samples (see Supplementary Table S2). As for social factors, adults over 45 years of age and people with secondary or lower education (ISCED 3c or lower) had significantly lower participation rates in NFE in all countries. Moreover, there were significant differences in all tested job-related variables across all samples. As mentioned above, our findings indicate an increasing importance of economic status on participation in NFE, as employed adults comprise 85.7–89.5% of all participants in NFE.
The results also show that NFE primarily remains the domain of high-skilled white-collar workers (ISCO 1–2, including legislators, managers, professionals, technicians, and associate professionals). However, in Sweden and the United Kingdom, the percentage of involvement of adults in medium-skilled occupations (ISCO 3–7, including clerks, service, shop, and market sales workers) was almost the same. The findings that participation in NFE depends on employment status, type of contract, and company size were also confirmed by the present descriptive results. In this regard, adults who worked full-time with a permanent contract reported significantly higher participation rates in NFE (see Supplementary Table S2).
While employers play an essential role in NFE, the expressed need for education and training is of utmost importance. When it comes to behavioral factors, adults’ intent to participate in any form of adult education and training is a predominant factor among NFE participants (see Supplementary Table S2). Similarly, actively seeking information about potential educational opportunities and skill development distinguishes individuals who participate in NFE from those who do not.
Table 3 contains the results of regression models for participation in NFE of all respondents (Models 1–3) and respondents active in the job market (Models 4–6). All models demonstrated statistical significance and accurately classified between 61.7% (in the case of Model 4) and 73.6% (in the case of Model 3) of cases, with the most significant improvement of 9.9 percentage points seen in Model 5. Collectively, the final models (Models 3 and 6) explained between 23% (Cox and Snell R2) and 35% (Nagelkerke R2) of the variability in NFE participation. For a detailed summary of the quality parameters of the models, see the notes in Table 3.
Regarding the assessment of each independent variable added in the first step (block), it is worth noting that the likelihood of participating in NFE significantly decreased with increasing age, even when considering other factors in the complete models. To be more specific, older adults (aged 45 and above) were less likely to participate in NFE compared to younger adults (aged 25–44), with an average odds ratio of 0.627, signifying a 1.6 times lower chance for older adults to engage in NFE. In other words, older adults had a 37% lower likelihood of participating in NFE compared to the younger age category. This trend remained consistent even after incorporating other factors into the models, both for all respondents and respondents who are active in the job market.
The influence of educational background played a substantial role in NFE participation. Adult learners with higher education (ISCED 5–8) had a 3.5 times higher chance (Model 1) and a 3.2 times higher chance (Model 4) of participating in NFE compared to those with secondary or lower education (ISCED 3c or lower). While this effect remained statistically significant across all models, we observed a gradual reduction in its strength after introducing interactions with other variables into the models. Furthermore, gender did not emerge as a significant predictor.
On the other hand, behavioral (micro-level) factors emerged as the most influential predictors among all tested variables. The expressed need for education in any organized learning context, whether at work or during leisure, in the past 12 months was the most significant explanatory factor for NFE participation. More specifically, adults without a need for further education and training were, on average, 4.5 times less likely to participate in NFE than those with such intentions, which is valid for all respondents (M2 and M3), as well as respondents active in the job market (M5 and M6). Put another way, adults without a need for further education and training had a 77% lower likelihood to participate in NFE compared to those who expressed this need. However, actively seeking information about potential education or skill development in the past year, while still significant, had a lesser impact, roughly three times less.
Job-related (meso-level) factors made substantial contributions to the final models. Specifically, economically inactive adults had a 2.6 times lower chance of being involved in NFE than adults active in the job market. It means that adults outside the labor market had a 62% lower likelihood to participate in NFE compared to their economically active counterparts. The assumption that NFE primarily attracts adults in high-skilled occupations was confirmed. Adults in medium-skilled (ISCO 3–7) and low-skilled (ISCO 8–9) occupations had a lower chance to participate than high-skilled white-collar workers (ISCO 1–2). Similarly, part-time or self-employed workers had, on average, a 1.5 times lower likelihood or had a 31% lower chance to participate in NFE than full-time employees. The higher participation rates of employees in large companies were only partially confirmed; participants working for medium-sized companies (50–250 employees) had about a 1.5 times higher chance to participate in NFE than participants working for small-sized companies (less than 50 employees). Finally, employment contract did not significantly influence participation in NFE in the tested models.
The relevance of the country-specific (macro-level) factors was evident until the inclusion of other layers of behavioral and job-related variables in the models, encompassing all respondents and those active in the job market. It appears that, when accounting for adults’ learning intentions and job-related factors, the influence of the country of birth becomes less pronounced (as seen in baseline Models 2 and 5) or no longer significant (see baseline Models 3 and 6).
Upon evaluating the quality of the tested models (see the notes in Table 3), it is evident that the influencing factors play a role that is both statistically and substantially significant. This suggests that the predictor variables possess a strong explanatory power. It is worth noting that, although we distinguish adults based on their economic status on all respondents (Models 1–3) and these active in the job market (Models 4–6), they exhibit a consistent pattern of significant predictors across all models. As a result, these same variables remain valid in multivariate analysis and interact with each other in nearly identical ways. When examining the amount of variation explained by the models (Cox and Snell R2 and Nagelkerke R2), it becomes clear that behavioral factors contribute significantly to the explained variance in NFE participation. This underscores their high relevance among the tested predictors.
The focus of the present study was to investigate participation rates in NFE (RQ1) and analyze the impact of selected factors on participation in NFE of economically active and all respondents across four European countries with various adult learning systems (RQ2).
In terms of overall participation in NFE, it is worth noting that data from 2022 for four selected countries show a different trend compared to the initial prominent pattern of increasing participation rates in NFE (Desjardins et al., 2016; Rubenson, 2018; Desjardins, 2020; Desjardins and Ioannidou, 2020). In 2022, participation rates have either remained stable or, in the case of Sweden and Germany, have seen a slight increase as compared to previous data collection in 2016. This suggests that in advanced post-industrial European societies, there may be a limit to how much NFE participation can reach over a 12-month period, making it challenging to raise participation rates further.
These findings deserve two discussion points. First, it is the comparative dimension and long-term convergence trend in participation rates that we have identified. Although these four adult learning systems initially consolidated as highly different (Roosmaa and Saar, 2012, 2017; Boeren et al., 2017; Desjardins, 2017; Boeren, 2019; Desjardins and Ioannidou, 2020), both participation rates and the factors causing inequality in them have slowly converged over the time, albeit this converge was stronger in 2016 than in 2022. Although Sweden still has a slight comparative advantage in NFE participation and related levels of inequality in participation, this advantage is almost negligible compared to the situation in the 2000s. On the other hand, the capacity of the German system to boost participation levels in NFE has improved significantly since the late 2000s.
The interesting trends in Sweden (slight decline from 2007 to 2016) and Germany (continuous growth) could be interpreted on the background of different “varieties of liberalisms” (Thelen, 2014) that have been evolving in those countries. In Sweden, the liberalization of the robust welfare state has been driven by factors such as an aging population, increased immigration, financial challenges, and the pandemic crisis. Over the past decade, this liberalization has resulted in the implementation of austerity measures that have impacted various aspects, including the reduction of support for certain active labor market policies, including the previously generous support for NFE (Green, 2021). However, it seems that newly implemented social investment policies have stopped this trend.
On the other hand, Germany has followed a different trajectory in its approach to liberalization. NFE has become a crucial domain for private employers, who have turned to job-oriented NFE as a strategy to address the notably high labor market shortages (CEDEFOP, 2018) and use NFE more as a protection for employees in some sectors of the labor market.
Regarding the second global trend in NFE participation, which involves a growing emphasis on job-oriented training and an increasing share of employer-sponsored training (Boeren and Holford, 2016; Desjardins et al., 2016; Rubenson, 2018; Kalenda et al., 2019; Desjardins, 2020), our findings strongly support this direction. This finding also indicates that access to NFE is highly dependent on the characteristics of the enterprises where the employee currently works, their degree of innovation, role and value they see in continuous education (Boeren, 2016, 2023). In all analyzed countries, NFE remained the domain of full-time white-collar workers in high-skilled occupations.
Our hierarchical regression models, including micro-level sets of variables (social and behavioral factors), job-related (meso-level) factors, and country-specific (macro-level) factors of participation in NFE yielded statistically significant results, demonstrating that tested variables effectively explain participation in NFE. For a comprehensive overview of the quality parameters, please refer to the notes in Table 3.
Upon closer examination, it becomes evident that when evaluating the typical sources of NFE participation inequality, as suggested by the existing literature (Dämmrich et al., 2014; Boeren, 2016; Rubenson, 2018; Blossfeld et al., 2020; Cabus et al., 2020; Desjardins, 2020; Jenkins, 2021), factors such as age and the highest level of education remain crucial. However, to provide a more comprehensive explanation of NFE participation within the country-specific framework, it is essential to include additional layers, specifically those related to behavior (Boeren, 2023) and job-related factors (Desjardins, 2014; Saar and Räis, 2017).
In summary, our findings suggest that incorporating behavioral and job-related factors into more complex models significantly enhances the model quality and identifies robust predictors of NFE participation. The results, therefore, confirm a key concept behind this study: the willingness to engage in organized NFE exhibits a complex structure with multiple layers, as demonstrated in prior theoretical elaboration (Boeren, 2016, 2023). This means that considering a broader set of factors enhances the model’s ability to explain NFE participation. Hence, solely focusing on individual social factors or broad societal factors connected to a country’s welfare or educational policies is insufficient for comprehensively understanding the various factors and interactions that impact an individual’s decision to participate or not in NFE.
In response to our second research question (RQ2), it becomes evident that an individual’s behavioral characteristics (micro-level factors), particularly their (intrinsic) learning intention, have a significant impact on their likelihood of participating in NFE. In fact, it served as the strongest substantively significant predictor of participation in NFE under our investigation. Therefore, the ability of learners to recognize their own learning needs, whether in their workplace or daily life, emerges as a dominant predictor of their engagement in educational activities. However, it is worth noting that, to date, limited research has been conducted on this aspect. For instance, foundational work in the European context by Boeren et al. (2010, 2012) aimed to uncover differences in intentions to participate in formal adult education, and a decade later, Van Nieuwenhove and De Wever (2021) found that learning intentions are also vital of participation as well as individuals with a lower needs for training tend to have a lower educational background. As a result, there is a pressing need to shift our focus toward exploring factors related not only to the intention to learn but also other behavioral characteristics of adults, as emphasized by Boeren (2016, 2023). These factors encompass motivation, attitudes, values, and self-efficacy, all of which play crucial roles in shaping an individual’s decision to participate in NFE.
Our research has confirmed the influence of several social (micro-level) factors on participation in NFE. Among these social factors, educational attainment stands out. Individuals with higher levels of education are more inclined to engage in ongoing education and training activities. This observation aligns with the concept of the “Matthew effect” (Boeren, 2016; Blossfeld et al., 2020), which suggests that those already in advantageous positions tend to benefit even more, while the opposite holds true for those in less privileged positions. In our specific context, this translates to a higher rate of NFE participation among adults with higher education (especially ISCED 5–8). Their positive educational experiences might foster a greater willingness to pursue further education, underpinned by positive expectations (Rubenson, 2018).
Moreover, since highly educated adults have spent more time in formal education and successfully completed educational programs, their decisions are likely driven by a positive motivation that is particularly important for participating in NFE beyond the scope of company-sponsored training. However, it is worth noting that we observed a gradual reduction in the impact of education after incorporating all interactions into our comprehensive models. This could be due to the fact that individuals with a university degree are more frequently employed full-time in white-collar positions (Desjardins, 2020). Therefore, when controlling for these variables, the influence of educational background tends to diminish somewhat.
As anticipated, our research aligns with the commonly observed trend that the benefits of further education tend to be more significant for younger adults (Desjardins et al., 2006; Dämmrich et al., 2014; Rubenson, 2018; Blossfeld et al., 2020; Desjardins, 2020). Consequently, in our study, we found that higher age served as a barrier to participation. This pattern is consistent even in the context of OECD countries, as evidenced by the AES data from 2016 (OECD iLibrary, 2019), where younger adults (25–34-year-olds) are more likely to be involved in NFE compared to older age groups.
To address these age-related barriers, there is a compelling need for enhanced social and political recognition of older individuals in disadvantaged situations, particularly those working in companies with limited or no educational opportunities. As suggested by Lischewski et al. (2020), establishing legal rights to continuous education and training, such as granting employees study leave during working hours or recognizing competences acquired through NFE in family-friendly time slots or formats, can play a pivotal role in promoting participation and reducing barriers, particularly for disadvantaged groups.
Moreover, among the various job-related (meso-level) factors, economic status plays a prominent role. It exerts a significant influence on participation, as evident in our regression models. Specifically, we found that individuals inactive in the job market had nearly three times lower odds of engaging in NFE compared to those who were economically active. According to Rubenson et al. (2008), employed individuals had, on average, 1.5 times higher chances of being educated. This finding underlines the increasing importance of economic status on participation in NFE, as employed adults, on average, comprise 88% of all participants in NFE (see Supplementary Table S2). Although a high percentage of individuals participate in NFE (see Table 1), it is predominantly for job reasons (see Table 2) that makes NFE subject to market forces and employers’ interests.
Furthermore, holding a managerial position or position of highly skilled professional (ISCO 1–2) with a full-time contract in a medium-sized company (with 50–250 employees) had a significant impact on the participation rates of all adults, regardless their activity in the job market. Interestingly, the type of employment contract did not have a significant influence on participation in NFE. This suggests that investments made by companies in enhancing their employees’ skills and knowledge have become increasingly independent of the specific terms of employment, whether they are short-term or long-term in nature. If we compare our results with Kalenda et al. (2019), according to which full-time workers were more likely to be educated in 2016 as compared with 2011 according to regression models for the AES in the Czech Republic, the significance of whether people participate in NFE under the full-time employment contracts reached similar importance a half decade later.
These results suggest a direct connection between the increasing tendency of participation rates (see Table 1) and key factors affecting it (see Table 3). Based on the expansion of the mostly job-related NFE paid by employers (see Table 2), the impact of job-related factors has also increased. Put differently, the growing interest of employers in educating their employees to reach economic growth and the transformation of the economy was, in turn, reinforced by the employee need to participate in NFE.
Finally, in relation to the country-specific (macro-level) factors, we observed disparities in inequality between Germany and the United Kingdom, with both countries exhibiting lower levels of inequality when only social factors were considered. However, when accounting for adults’ learning intentions and job-related factors, the influence of the country of birth becomes less pronounced or vanishes. From this perspective, we can conclude that all four advanced post-industrial countries in this case, despite having different adult learning systems, share a similar pattern of inequality in NFE. As a result, NFE has been transformed into primarily labor market-based activity with a strong influence of employers’ goals and interests. Therefore, country-specific factors were somewhat overshadowed by social and job-related characteristics, which demonstrate substantial general determinants. This trend is also supported by our results introducing that although all four countries (macro-level factors) started with widely different NFE participation rates, they have converged over time and were constantly influenced by micro and meso-level factors.
The multivariate results we have examined, based on data from four different European countries combined into a single dataset, may appear to have several limitations. However, it is important to note that the general conclusions we have presented pertain to specific European adult learning systems that were selected for this analysis. In each of these countries, separate regression models were performed, although the details of these models are not presented here. While there may be slight variations across the countries we sampled, it is noteworthy that certain common features seem to prevail.
In relation to the AES, although this survey allows comparison of participation in adult learning from the individuals’ perspective across all countries of the European Union and several others, it carries known limitations of self-reporting. It is questionable how comparable AES data are with other large-scale international surveys like the IALS or PIAAC if they do not share the same methodological approach in terms of item wording, validity of measurement (measurement error), including errors in data processing, or method of sampling (sampling error). For example, Widany et al. (2019) address consequences for the interpretation of survey results on adult education and training participation with the Total Survey Error approach (TSE) used as a conceptual framework.
The AES requirement of a 12-month reference period has faced criticism (Widany et al., 2019), with contrasting views from data analysts, policymakers, and survey respondents. From an analytical and policymaker perspective, this period is relatively short, potentially limiting the ability to capture long-term trends. Adults might participate intensely in beneficial training activities initially, such as seeking new employment or career advancement, but their participation might dwindle later. Conversely, survey respondents could find this period too long, struggling to recall all learning activities in such detail. However, a context-based retrieval process (participation in specific employment) supports respondents’ accurate recall even within several times longer reference period (Dürnberger et al., 2010).
In this study, NFE was measured using a binary variable. The distinction between long or short-term NFE activities and their quantity was not considered, posing another limitation. Additionally, there is a limitation related to the purpose of participation in NFE – distinguishing between job-related and non-job-related activities. Respondents might have difficulty categorizing, as there could be a mix of both purposes within each category. For instance, job-related NFE might include non-job-related learning activities and vice versa. Moreover, our sample did not attain a representative distribution in the case of the highest level of educational achievement within the British and German samples. Our results may, therefore, show slightly different (elevated) rates of participation in NFE in these countries compared to the general population. The future research should address this discrepancy.
It would be intriguing for future research to delve into an examination of economically inactive adults (e.g., pensioners, the unemployed and those on parental leave) who have participation intentions but lack learning opportunities, i.e., non-participants willing to participate or participants who want to participate more, and to identify the barriers they encounter as they form 10.2 and 15.7% of the EU-27 population, respectively (Eurostat, 2024c). This analysis could provide insights into the difficulties these individuals face when considering participation in NFE. Specifically, it could shed light on the circumstances under which the potential benefits, including financial and job-related advantages, do not surpass the associated costs in terms of invested time, energy and finances.
Future research faces challenges due to the lack of information on (meso-level) NFE providers. Employers often procure NFE from external bodies, leading to variations in quality, format, duration, and location. Understanding how NFE offerings are communicated, the reach of potential learners, and whether participation is driven by job requirements or personal/career development could uncover valuable insights. The quality of data on participation in adult education and training, together with methodological issues, should be addressed. Lastly, longitudinal data on participation is widely lacking in adult education research including insights on its quality and long-term effects.
The raw data supporting the conclusions of this article will be made available by the authors, without undue reservation.
Ethical review and approval was not required for the study on human participants in accordance with the local legislation and institutional requirements. Written informed consent from the patients/participants or patients/participants legal guardian/next of kin was not required to participate in this study in accordance with the national legislation and the institutional requirements.
JV: Conceptualization, Data curation, Formal analysis, Funding acquisition, Investigation, Methodology, Project administration, Resources, Software, Supervision, Validation, Visualization, Writing – original draft, Writing – review & editing. JK: Writing – original draft, Writing – review & editing. IK: Writing – review & editing.
The author(s) declare that no financial support was received for the research, authorship, and/or publication of this article.
We acknowledge the contributions of Ellu Saar (8 October 1955 – 2 June 2024), who provided valuable feedback in the review process of this manuscript. We extend our condolences to their family and colleagues.
The authors declare that the research was conducted in the absence of any commercial or financial relationships that could be construed as a potential conflict of interest.
All claims expressed in this article are solely those of the authors and do not necessarily represent those of their affiliated organizations, or those of the publisher, the editors and the reviewers. Any product that may be evaluated in this article, or claim that may be made by its manufacturer, is not guaranteed or endorsed by the publisher.
The Supplementary material for this article can be found online at: https://www.frontiersin.org/articles/10.3389/feduc.2024.1380865/full#supplementary-material
Baert, H., De Rick, K., and Van Valckenborgh, K. (2006). “Towards the conceptualisation of learning climate” in Adult education: new routes in a new landscape. eds. R. Vieira de Castro, A. V. Sancho, and V. Guimaraes (Braga: University de Minho), 87–111.
Blossfeld, H.-P., Kilpi-Jakonen, E., Vono de Vilhena, D., and Buchholz, S. (2020). “Is there a Matthew effect in adult learning? Results from a cross-national comparison” in Monetary and non-monetary effects of adult education and training. eds. J. Schrader, A. Ioannidou, and H.-P. Blossfeld (Wiesbaden), 1–26.
Boeren, E. (2016). Lifelong learning participation in a changing policy context. An interdisciplinary theory. London: Palgrave Macmillan.
Boeren, E. (2017). Understanding adult lifelong learning participation as a layered problem. Stud. Contin. Educ. 39, 161–175. doi: 10.1080/0158037X.2017.1310096
Boeren, E. (2019). “Being an adult learner in Europe and the UK: persisting inequalities and the role of the welfare state” in Being an adult learner in austere times. Exploring the contexts of higher. Further and community education. eds. E. Boeren and N. James (London: Palgrave Macmillan), 21–45.
Boeren, E. (2023). “Conceptualizing lifelong learning participation – theoretical perspectives and integrated approaches” in International yearbook of adult education. Researching participation in adult education. ed. M. Schemann (Bielefeld: wbv Media), 17–31.
Boeren, E., and Holford, J. (2016). Vocationalism varies (a lot): a 12-country multivariate analysis of participation in formal adult learning. Adult Educ. Q. 66, 120–142. doi: 10.1177/0741713615624207
Boeren, E., Holford, J., Nicaise, I., and Baert, H. (2012). Why do adults learn? Developing motivational typology across twelve European countries. Glob. Soc. Educ. 10, 247–269. doi: 10.1080/14767724.2012.678764
Boeren, E., Nicaise, I., and Baert, H. (2010). Intentions and constraints of non-participants in adult education, in European Society for Research on the education of adult conference, 22–26 September 2010 (Linköping, Sweden).
Boeren, E., Whittaker, S., and Riddell, S. (2017). Provision of seven types of education for disadvantaged adults in ten countries: overview and cross-country comparison. ENLIVEN report, deliverable no. 2.1. Available at: https://h2020enliven.files.wordpress.com/2017/09/enliven-d2-1.pdf (accessed October 15, 2023)
Boyadjieva, P., and Ilieva-Trichkova, P. (2022). Does participation in non-formal adult education matter for individual subjective well-being as a multidimensional functioning? Eur. Educ. Res. J. 23, 125–144. doi: 10.1177/14749041221116261
Bujang, M. A., Sa'at, N., Sidik, T. M. I. T. A. B., and Joo, L. C. (2018). Sample size guidelines for logistic regression from observational studies with large population: emphasis on the accuracy between statistics and parameters based on real life clinical data. Malays. J. Med. Sci. 25, 122–130. doi: 10.21315/mjms2018.25.4.12
Busemeyer, M., and Trampusch, C. (2012). The political economy of collective skill formation. Oxford: Oxford University Press.
Cabus, S., Ilieva-Trichkova, P., and Štefánik, M. (2020). Multi-layered perspective on the barriers to learning participation of disadvantaged adults. Zeitschrift für Weiterbildungsforschungm 43, 169–196. doi: 10.1007/s40955-020-00162-3
CEDEFOP (2018). Insights into skill shortages and skill mismatch. Learning from Cedefop’s European skills and job survey. Luxembourg: Publications Office.
CLA (2016). Classification of learning activities (CLA). Manual. Luxembourg: Publications Office of the European Union.
Dämmrich, J., Vono, D., and Reichart, E. (2014). Participation in adult learning in Europe: the impact of country-level and individual characteristics, in Adult learning in modern societies: Patterns and consequences of participation from a life-course perspective, eds. H.-P. Blossfeld, E. Kilpi-Jakonen, and D. Vono de Vilhena, and Buchholz, S. (Cheltenham: Edward Elgar, 25–51.
Desjardins, R. (2014). Rewards to skill supply, skill demand and skill-mismatch. Studies using the adult literacy and life skills survey. Lund economic studies NO 176. Lund: Lund University.
Desjardins, R. (2017). Political economy of adult learning systems. Comparative study of strategies, policies, and constraints. New York, NY: Bloomsbury.
Desjardins, R. (2019). The relationship between attaining formal qualifications at older ages and outcomes related to active ageing. Eur. J. Educ. 54, 30–47. doi: 10.1111/ejed.12315
Desjardins, R. (2020). PIAAC thematic report on adult learning. OECD education working paper no. 223. Paris: OECD.
Desjardins, R. (2023). “Lifelong learning systems” in Third international handbook of lifelong learning. Springer international handbooks of education. eds. K. Evans, J. Markowitsch, W. O. Lee, and M. Zukas (Cham: Springer), 353–374.
Desjardins, R., and Ioannidou, A. (2020). The political economy of adult learning systems – some institutional features that promote adult learning participation. Zeitschrift Weiterbildungsforschungm 43, 143–168. doi: 10.1007/s40955-020-00159-y
Desjardins, R., and Kim, J. (2023). “Inequality in adult education participation across national contexts: is growing employer support exacerbating or mitigating inequality in participation?” in International yearbook of adult education 2023. ed. M. Schemann (New York, NY: Portico), 75–98.
Desjardins, R., Melo, V., and Lee, J. (2016). Cross-national patterns of participation in adult education and policy trends in Korea, Norway, and Vietnam. Prospects 46, 149–170. doi: 10.1007/s11125-016-9384-3
Desjardins, R., Rubenson, K., and Milana, M. (2006). Unequal chances to participate in adult learning: International perspectives. Paris: UNESCO.
Dieckhoff, M., and Steiber, N. (2011). A re-assessment of common theoretical approaches to explain gender differences in continuing training participation. Br. J. Ind. Relat. 49, 135–157. doi: 10.1111/j.1467-8543.2010.00824.x
Dürnberger, A., Drasch, K., and Matthes, B. (2010). Kontextgestützte Abfrage in Retrospektiverhebungen. Ein Kognitiver Pretest zu Erinnerungsprozessen bei Weiterbildungsereignissen. IAB-Discussion Paper. Available at: http://doku.iab.de/discussionpapers/2010/dp2010.pdf (accessed October 18, 2023).
EC (2020). European skills agenda for sustainable competitiveness, social fairness and resilience. Brussels: European Commission.
Esping-Andersen, G. (1990). The three worlds of welfare capitalism. Princeton: Princeton University Press.
Esping-Andersen, G. (1999). Social foundations of post-industrial economics. Oxford: Oxford University Press.
Eurostat. (2023a). Adult Education Survey (AES) Methodology. Available at: https://ec.europa.eu/eurostat/statistics-explained/index.php?title=Adult_Education_Survey_(AES)_methodology#Introduction (accessed May 26, 2024)
Eurostat. (2023b). Participation Rate in Education and Training by Sex. Available at: https://ec.europa.eu/eurostat/databrowser/view/trng_aes_100/default/table?lang=en (accessed February 2, 2024)
Eurostat. (2024c). Population by Will to Participate in Education and Training. Available at: https://ec.europa.eu/eurostat/databrowser/view/trng_aes_175__custom_11593473/default/table?lang=en (accessed May 29, 2024).
Green, A. (2021). Models of lifelong learning and their outcomes. How distinctive is the ‘Nordic model’ now? (LLAKES Research Papers 70). London, UK: Centre for Learning and Life Chances (LLAKES), UCL Institute of Education.
Hall, P. A., and Soskice, D. (2001). Varieties of capitalism: the institutional foundations of comparative advantage. New York: Oxford University Press.
Holford, J., Boyadjieva, P., Clancy, S., Hefler, G., and Studena, I. (2022). Lifelong learning, young adults and the challenges of disadvantage in Europe. London, UK: Palgrave-Macmillan.
ILO. (2004). National Labour Law Profile: The Czech Republic. Available at: https://www.ilo.org/ifpdial/information-resources/national-labour-law-profiles/WCMS_158893/lang--en/index.htm (accessed September 19, 2023)
ILO. (2022). World Employment and Social Outlook: Trends 2022. Available at: https://www.ilo.org/global/research/global-reports/weso/trends2022/lang--en/index.htm (accessed September 22, 2023).
Iňiguez-Berrozpe, T., Elboj-Saso, C., Flecha, A., and Marcaletti, F. (2020). Benefits of adult education for low-educated women. Adult Educ. Q. 70, 64–88. doi: 10.1177/0741713619870793
International Chamber of Commerce/ESOMAR. (2016). ICC/ESOMAR international code on market, opinion and social research and data analytics. ESOMAR. https://www.esomar.org/uploads/pdf/professional-standards/ICCESOMAR_Code_English.pdf
Jenkins, A. (2021). Patterns of participation and non-participation in learning in mid-life and their determinants. Int. J. Lifelong Educ. 40, 215–228. doi: 10.1080/02601370.2021.1937357
Kalenda, J., Kočvarová, I., and Vaculíková, J. (2019). Determinants of participation in non-formal education in the Czech Republic. Adult Educ. Q. 70, 99–118. doi: 10.1177/0741713619878391
Kopecký, M., and Šerák, M. (2015). “Adult education and learning policy in the CZE” in Global perspectives on adult education and learning policy. eds. M. Milana and T. Nesbit (New York: Palgrave Macmillan), 29–43.
Landman, T., and Carvalho, E. (2017). Issues and methods in comparative politics: an introduction. London, UK: Routledge.
Lischewski, J., Seeber, S., Wuttke, E., and Rosemann, T. (2020). What Influences Participation in Non-formal and Informal Modes of Continuous Vocational Education and Training? An Analysis of Individual and Institutional Influencing Factors. Front. Psychol. 11:534485. doi: 10.3389/fpsyg.2020.534485
OECD iLibrary. (2019). Education at a Glance 2019: OECD Indicators. Available at: https://www.oecd-ilibrary.org/sites/df2ec3d0-en/index.html?itemId=/content/component/df2ec3d0-en (accessed October 28, 2023)
Rees, G. (2013). Comparing adult learning systems: an emerging political economy. Eur. J. Educ. 48, 200–212. doi: 10.1111/ejed.12025
Roosmaa, E.-L., and Saar, E. (2012). Participation in non-formal learning in EU-15 and EU-8 countries: demand and supply side factors. Int. J. Lifelong Educ. 31, 477–501. doi: 10.1080/02601370.2012.689376
Roosmaa, E.-L., and Saar, E. (2017). Adults who do not want to participate in learning: a cross-national European analysis of their perceived barriers. Int. J. Lifelong Educ. 36, 254–277. doi: 10.1080/02601370.2016.1246485
Rubenson, K. (2006). The Nordic model of lifelong learning. Compare 36, 327–341. doi: 10.1080/03057920600872472
Rubenson, K. (2018). “Conceptualizing participation in adult learning and education. Equity issues” in The Palgrave international handbook on adult and lifelong education and learning. eds. M. Milana, S. Webb, J. Holford, R. Waller, and P. Jarvis (London: Palgrave Macmillan), 337–357.
Rubenson, K., and Desjardins, R. (2009). The impact of welfare state requirements on barriers to participation in adult education: a bounded agency model. Adult Educ. Q. 59, 187–207. doi: 10.1177/0741713609331548
Rubenson, K., Desjardins, R., and Yoon, E. S. (2008). Adult learning in Canada: a comparative perspective. Ottawa, Ontario, Canada: Statistics Canada.
Saar, E., and Räis, M. L. (2017). Participation in job-related training in European countries: the impact of skill supply and demand characteristics. J. Educ. Work. 30, 531–551. doi: 10.1080/13639080.2016.1243229
Tabachnick, B. G., and Fidell, L. S. (2007). Using multivariate statistics. 5th Edn. Boston: Pearson Education.
Thelen, K. (2014). Varieties of liberalization and the new politics of social solidarity. Cambridge: Cambridge University Press.
UNESCO (2020). Embracing a culture of lifelong learning. Contribution to the future of education initiative. Hamburg: UNESCO Institute for Lifelong Learning.
Van Nieuwenhove, L., and De Wever, B. (2021). Why are low-educated adults underrepresented in adult education? Studying the role of educational background in expressing learning needs and barriers. Stud. Contin. Educ. 44, 189–206. doi: 10.1080/0158037x.2020.1865299
Widany, S., Christ, J., Gauly, B., Massing, N., and Hoffmann, M. (2019). The quality of data on participation in adult education and training. An analysis of varying participation rates and patterns under consideration of survey design and measurement effects. Front. Sociol. 4:71. doi: 10.3389/fsoc.2019.00071
Wuensch, K. L. (2021). Binary logistic regression with SPSS©. Available at: https://core.ecu.edu/wuenschk/mv/multreg/logistic-spss.pdf (accessed May 29, 2024)
Keywords: adult education and training, non-formal adult education (NFE), participation in NFE, adult learning systems, multivariate analyses, multilayer approach
Citation: Vaculíková J, Kalenda J and Kočvarová I (2024) Participation in non-formal adult education within the European context: examining multilayer approach. Front. Educ. 9:1380865. doi: 10.3389/feduc.2024.1380865
Received: 02 February 2024; Accepted: 05 July 2024;
Published: 17 July 2024.
Edited by:
Petya Ilieva-Trichkova, Bulgarian Academy of Sciences (BAS), BulgariaReviewed by:
Denisa Fedakova, Centre of Social and Psychological Sciences, SlovakiaCopyright © 2024 Vaculíková, Kalenda and Kočvarová. This is an open-access article distributed under the terms of the Creative Commons Attribution License (CC BY). The use, distribution or reproduction in other forums is permitted, provided the original author(s) and the copyright owner(s) are credited and that the original publication in this journal is cited, in accordance with accepted academic practice. No use, distribution or reproduction is permitted which does not comply with these terms.
*Correspondence: Jitka Vaculíková, dmFjdWxpa292YUB1dGIuY3o=
Disclaimer: All claims expressed in this article are solely those of the authors and do not necessarily represent those of their affiliated organizations, or those of the publisher, the editors and the reviewers. Any product that may be evaluated in this article or claim that may be made by its manufacturer is not guaranteed or endorsed by the publisher.
Research integrity at Frontiers
Learn more about the work of our research integrity team to safeguard the quality of each article we publish.